DOI:
10.1039/D0AN02443K
(Paper)
Analyst, 2021,
146, 2591-2599
A combined flow injection/reversed-phase chromatography–high-resolution mass spectrometry workflow for accurate absolute lipid quantification with 13C internal standards†‡
Received
23rd December 2020
, Accepted 24th February 2021
First published on 1st March 2021
Abstract
We propose a fully automated novel workflow for lipidomics based on flow injection, followed by liquid chromatography–high-resolution mass spectrometry (FI/LC–HRMS). The workflow combined in-depth characterization of the lipidome achieved via reversed-phase LC–HRMS with absolute quantification by using a large number of lipid species-specific and/or retention time (RT)-matched/class-specific calibrants. The lipidome of 13C-labelled yeast (LILY) provided a large panel of cost-effective internal standards (ISTDs) covering triacylglycerols (TG), steryl esters (SE), free fatty acids (FA), diacylglycerols (DG), sterols (ST), ceramides (Cer), hexosyl ceramides (HexCer), phosphatidylglycerols (PG), phosphatidylethanolamines (PE), phosphatidic acids (PA), cardiolipins (CL), phosphatidylinositols (PI), phosphatidylserines (PS), phosphatidylcholines (PC), lysophosphatidylcholines (LPC) and lysophosphatidylethanolamines (LPE). The workflow in combination with the LILY lipid panel enables simultaneous quantification via (1) external multi-point calibration with internal standardization and (2) internal one-point calibration with LILY as a surrogate ISTD, increasing the coverage while keeping the accuracy and throughput high. Extensive measures on quality control allowed us to rank the calibration strategies and to automatically select the calibration strategy of the highest metrological order for the respective lipid species. Overall, the workflow enabled a streamlined analysis, with a limit of detection in the low femtomolar range, and provided validation tools together with absolute concentration values for >350 lipids in human plasma on a species level. Based on the selected standard panel, lipids from 7 classes (LPC, LPE, PC, PE, PI, DG, TG) passed stringent quality filters, which included QC accuracy, a precision and recovery bias of <30% and concentrations within the 99% confidence interval of the international laboratory comparison of SRM 1950, NIST, USA. The quantitative values are independent of common deuterated or non-endogenous ISTDs, thus offering cross-validation of different lipid methods and further standardizing lipidomics.
Introduction
LC–MS-driven lipidomics strives to quantify lipids in biological entities comprehensively. However, to date, validation of quantitative omics-type analysis has remained a challenge.1,2 Appropriate standardization strategies regarding different analytical platforms and their compliance with guidelines are currently under debate. Internal standardization has been propagated as the method of choice for absolute quantification, and the selection criteria of internal standards (ISTDs) in lipidomics are well defined.3 Ideally, the lipid selected as the ISTD is added as early as possible to the sample in the analytical process. In the best case, it is an isotopically labeled analog of the investigated lipid. Otherwise, at least the prerequisites of co-ionization (e.g., co-eluting compounds when using liquid chromatography) and structural similarity have to be met. Different calibration approaches have been developed to minimize the number of required ISTDs by using a class-specific surrogate standard.4 However, co-elution is a must and only possible in direct infusion mass spectrometry and chromatographic techniques separating lipids according to their head group chemistry (as enabled via hydrophilic interaction liquid chromatography (HILIC), normal-phase liquid chromatography (NP-LC) or supercritical fluid chromatography (SFC)). However, nowadays, reversed-phase liquid chromatography–mass spectrometry (RP-LC–MS), which separates lipids according to their fatty acyl chain chemistry, is the most widely used analytical lipidomics technique as revealed by a recent survey1 among expert laboratories and a comprehensive literature review.5 It provides efficient matrix separation6 and excellent chromatographic selectivity and retentivity for lipids due to the possibility of separating lipids with different chain lengths and different numbers/positions of double bonds, resulting in high sensitivity and dynamic range when combined with MS detection. While the method is unrivaled in terms of lipid separation and thus identification (combined with high-resolution MS (HRMS)), appropriate standardization approaches imply the use of multiple standards per lipid class; otherwise, quantification accuracy is compromised (see ESI Fig. S6‡).3,6–9 Only quantification based on external calibration with internal standardization by an (isotopically labeled) analog10–14 is the method of the highest metrological order and complies with the FDA guideline for Bioanalytical Method Validation,15 commonly used in clinics and biomarker evaluation. In fact, recent large-scale clinical studies have resorted to targeted analysis of a small panel of lipids.16
In this work, we want to increase the number of quantified lipids within one analytical run on RP-LC–HRMS without compromising accuracy. A lipid extract provided by 13C fully labeled biomass (Pichia pastoris), coined as lipidome isotope labeling of yeast (LILY),10 is used as the ISTD, providing over 250 uniformly 13C-labeled lipid species from 19 lipid classes. However, the number of accurately quantified lipid species is also limited by the number of external calibrants.11,12 As the quantitative information is lacking for LILY, a workflow has been designed to quantify LILY on a day-to-day basis with reverse isotope dilution prior to RP-LC–HRMS analysis of samples. This strategy enables implementing different calibration strategies within one lipidomics workflow: (1) external multi-point calibration with internal standardization and (2) surrogate internal standardization without external standardization. The reverse isotope dilution of LILY coped with the fact that comprehensive lipidome stability and storage conditions are still ill-defined for such cost-effective materials. It is well known that certain lipid species are prone to oxidation and degradation, making frequent recalibration a prerequisite.17–19
Human plasma lipidomics serves as a prime example to show the presented workflow's validity as it represents the most frequently analyzed sample matrix in the field.1,5 Quantification on the lipid species level will simplify the calculation and serve as a proof of concept as the implementation of quantification on the MS2 level was proven elsewhere.10 The presented validation will capitalize on healthy donor samples20–24 and reference materials23 with published consensus values of an interlaboratory comparison and the results of the workflow will be compared to shotgun lipidomics data.
Materials and methods
Materials
Human plasma samples were purchased from Innovative Research (Novi, Michigan). Standard reference material (SRM) 1950 from the National Institute of Standards and Technology (NIST, Gaithersburg, USA) was used. Reference standards (endogenous compounds for external calibration) and SPLASH® LIPIDOMIX® Mass Spec Standard were obtained from Avanti (Alabaster, USA) and Merck KGaA (Darmstadt, Germany). The internal standard LILY was obtained via the procedure described by Neubauer et al.25 and Schoeny et al.26
Methods
Two different methods (flow injection (FI) and RP-LC) were applied in the same analytical sequence as enabled by a 6-port valve controlled using MS software (see ESI Fig. S1‡). A detailed description of extraction, analysis, and data processing can be found in the ESI's‡ Extended Materials and Methods section.
Briefly, a Vanquish Horizon HPLC system and a high-field Q Exactive HF™ quadrupole-Orbitrap mass spectrometer (both from Thermo Fisher Scientific) were used. For RP chromatography of lipids, an Acquity HSS T3 column (2.1 mm × 150 mm, 1.8 μm, Waters) with a VanGuard Pre-column (2.1 × 5 mm, 100 Å, 1.8 μm) was used. The column temperature was set to 40 °C and the flow rate was set to 250 μL min−1. Acetonitrile (ACN)/H2O (3
:
2, v/v) was used as solvent A and isopropanol (IPA)/ACN (9
:
1, v/v) as solvent B, both containing 0.1% formic acid and 10 mM ammonium formate. A gradient of 23 min was applied. MS1 acquisition was used for quantification. An injection volume of 2 μL was selected, and polarity switching was performed. For data-dependent acquisition (DDA), the LC method was identical, but the injection volume was increased to 5 μL, the positive mode and negative mode were acquired separately, and only the pooled sample together with the extraction blank and a highly concentrated external standard were analyzed. For FI, the column was by-passed via a 6-port valve. 25 μL was injected at a 5 μL min−1 flow rate to obtain a constant signal for around 5 min. The eluents were kept constant at 50% A/50% B. Each FI measurement lasted 10 min, including washing. Polarity switching was triggered after 2.5 min (afterwards 10 s for equilibration). For each polarity, 200 data-independent acquisition (DIA) scans (precursor isolation width m/z 1) were alternated with an MS1 scan for quantification. MS1 RP-LC lipid data were processed using Skyline (version 20.1), data-dependent acquisition (DDA) files were processed using LipidSearch 4.2 from Thermo Scientific, and FI data were evaluated using LipidXplorer (version 1.2.8). All final data processing was performed in the R/R studio environment.
Results and discussion
RP-LC–HRMS is the gold standard for the in-depth characterization of lipidomes.1,5 While the superiority of this method with regard to sensitivity and dynamic range is widely accepted, its quantification capability is currently under debate. It becomes increasingly evident that a large number of lipid standards is mandatory for accurate omics-type quantification by RP-LC-based methods.3,6–9 In this work, an FI/LC–HRMS workflow integrated isotopically labeled yeast (LILY) as an internal standard (see Fig. 1) to simultaneously quantify a large number of lipids either via external multi-point calibration with internal standardization or internal one-point calibration with LILY as the surrogate ISTD. This increased the coverage while keeping the accuracy and throughput high. Several proof-of-concept studies showed the potential of LILY.10–12 However, the applied internal standardization strategies lacked the quantitative characterization of the 13C yeast lipidome. As the stability data for such an omics-type quantitative standard were beyond the possibility of this study, the integration of FI into the RP-LC workflow enables on-demand control of the ISTD for each sequence with only a slight increase in time (10%). This approach follows reverse isotope dilution, as it is often performed in quantitative atomic spectrometry to improve the accuracy by characterizing the spike material on a day-to-day basis.27 Internal standardization was accomplished by spiking samples and external standards with known amounts of LILY.
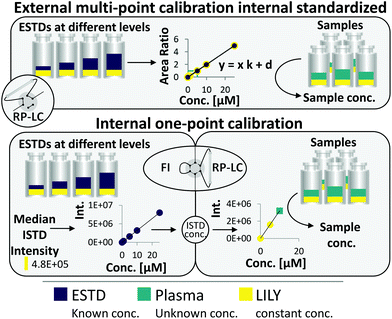 |
| Fig. 1 The two combined quantification strategies: (1) external multi-point calibration with internal standardization and (2) internal one-point calibration. LILY (yellow) was added to the plasma sample (blue) and the ESTDs (purple) in the same concentration. ESTDs were measured via RP-LC–HRMS and FI-HRMS. Samples were only measured via RP-LC–HRMS. | |
The combined FI/RP-LC–HRMS workflow for lipid quantification
An FI analysis step preceded RP-LC–HRMS analysis and enabled LILY's quantitative characterization on a day-to-day basis by reverse isotope dilution. For this purpose, FI was selected as a direct infusion method,28 given the possibility of automated switching to subsequent RP-LC-based lipidomic analysis (see ESI Fig. S1‡). An acquisition time of 5 min per sample was obtained by injecting 25 μL at a flow rate of 5 μL min−1 so that polarity switching and DIA MS/MS can be applied. As a prerequisite, the UHPLC system used in this work delivers highly precise flow rates in the flow regime from 1 μL min−1 to 5 mL min−1. Regarding sensitivity and precision, a comparable performance was observed for the optimized FI-HRMS approach and the chip-based infusion nano-ESI-MS29 (limits of detection (LOD) comparable to shotgun data were found in this work; see also Table S1‡).
Fig. 1 shows the two quantification strategies that are possible with the suggested workflow. For (1), the area ratio of ESTD/ISTD was used for linear regression models, and the area ratio of analyte/ISTD in samples was used to calculate the final analyte concentration. In (2), ESTDs measured via FI generated a linear regression model with their intensities. The median intensity value of the ISTD (present in all ESTDs) was used to calculate the ISTD concentration in a reverse isotope dilution manner. This calculated concentration was used in samples for analytes, which could not be analyzed with strategy 1, to quantify them via one-point calibration. Therefore, the accuracy and coverage can be combined via RP-LC with only a small increase in time (+10% of total run time) even when a vast number of standards and different blanks were included (see ESI Fig. S4 and ESI Excel table/Sequence‡). By tailoring the calibration levels, the measurement time could be reduced even further. The measurement sequence starts with FI analysis of external standards spiked with LILY. Fully automated switching from FI analysis to RP-LC–HRMS is accomplished via a 6-port valve. A typical lipidomics RP-LC–HRMS run in 23 min was performed to analyze samples and external standards spiked with LILY lipids. Excellent retention time (RT) stability (see ESI Fig. S3A‡) supported lipid identification and quantification across samples. Despite the short chromatographic separation time, for some lipid species, isomer separation was achieved for LPC, LPE, PC, PE and DG. Exemplarily, baseline separation of two lipid species (PC 18:1(9Z)/18:1(9Z) and PC 18:1(9E)/18:1(9E)) is shown in ESI Fig. S3B.‡ However, quantification was based on the sum integral to simplify data evaluation. The streamlined workflow involved RP-LC–HRMS analysis of all samples and standards in full MS mode (MS1, mass resolution 120
000, dynamic polarity switching) and a pooled sample in data-dependent MS/MS for quantification and identification, respectively. The measured calibration dilution series covered 4 orders of magnitude (low nM to low μM range). For data evaluation, only the linear working range was considered.
As a key advance, the large panel of external and internal standards paved the way for different applicable calibration strategies expanding the number of lipid species amenable to absolute quantification. Moreover, an extensive dilution series of external calibrants allowed tailored calibration levels for lipid species evaluation, both in FI-HRMS and RP-LC–HRMS analyses. Thus, the method allowed accurate quantification without an initial screening of every single analyte's linear range. Following stringent quality criteria, an automatic selection of the calibration strategy for each lipid species was implemented. Wherever applicable, species-specific standardization (denoted as level 1, according to the definition of LSI,30 see ESI Fig. S5‡) was preferred over class-specific calibration (denoted as level 2 in the case of RT matching, otherwise level 3). The ISTD and ESTD were chosen in the following decreasing preference order: level of standard,30 number of hydroxy groups (necessary only for sphingolipids), number of double bonds, and number of carbons in the fatty acyl chain. The quantification approach of the highest metrological order is lipid species-specific standardization by external multi-point calibration with internal LILY standardization. Evidently, this calibration approach is limited to the selection of external standards and lipids present in LILY. Otherwise, level 2 (RT match was accepted for maximal ±0.5 min RT shift) external calibration using the species-specific (level 1) LILY ISTD was applied, followed by one-point calibration using the quantified level 1 LILY ISTD. If no species-specific calibration was available, lipid class-specific RT-matched standardization was applied. The remaining species were assessed using level 3 standards.
Quantification of LILY by FI-HRMS
169 of the 250 13C fully labeled lipid species (identified on the lipid species level) were present in all samples with an intensity high enough for internal standardization (>10% of ISTD Blank). Out of these, 67 lipids were accurately quantified by reverse isotope dilution on an FI-HRMS routine by using either level 1 or 2 type standardization. Depending on the lipid class, MS quantification was based on the precursor, the head group fragment or the fatty acyl chain fragments in either positive or negative mode. Lipids from 8 classes (DG, TG, ST, PC, PE, PG, LPC, HexCer; see the ESI‡ for lipid class abbreviations) ranging in concentrations from 3 to 1500 nM were quantified (see ESI Excel table/LILY-lipids_FI‡). For all obtained LILY standard concentrations, stringent quality criteria were met in the FI-HRMS data. A detailed description of the quality criteria used for filtering is given in the ESI.‡ Overall, experimental uncertainty in QC samples, signal stability, linear dynamic range, and LOQ were considered. Thus, out of the 169 LILY lipids, 67 compounds were potential one-point ISTDs with experimentally assessed concentrations. All other LILY lipids served as ISTDs in external calibrations requiring no quantitative information to compensate for variations in sample preparation and instrument performance (see strategies 1 and 2, respectively, in the ESI‡/absolute lipid quantification).
Evaluation of different calibration strategies
The two possible quantification strategies in this workflow, external multi-point calibration with internal standardization and internal one-point calibration in combination with the three different levels30 for the ISTD and ESTD, enable multiple calibration strategies that need to be evaluated. Fig. 2 shows concentration values for selected lipid species obtained by these different calibration strategies in SRM 1950 with respect to the published consensus values.23,31 More specifically, the quantification of 4 lipid species, i.e. DG 34:1, TG 48:3, PE 36:2 and PC 36:2, is addressed. The isomers of PC 36:2 (PC 18:1_18:1 and PC 18:0_18:2) were separated by RP-LC. However, quantification was performed with the sum integral over all isomers. The importance of species-specific calibration or at least RT matching in class-specific calibration can be readily observed, emphasizing that a small number of standards is not practical in RP-LC analysis. Regardless of whether level 1 or level 2 calibration was applied, all concentrations were within the 99% confidence interval as published for the SRM 1950 material. However, the international lipidomics interlaboratory comparison revealed a rather broad distribution of measured concentration values for single lipid species32 (DG 34:1: <1–22 μmol L−1, TG 48:3: 1–10 μmol L−1, PE 36:2: 2–30 μmol L−1, PC 36:2: 75–350 μmol L−1), making it difficult to validate the different calibration strategies based on these consensus values only. Only certified reference material would allow an actual accuracy assessment.21,33
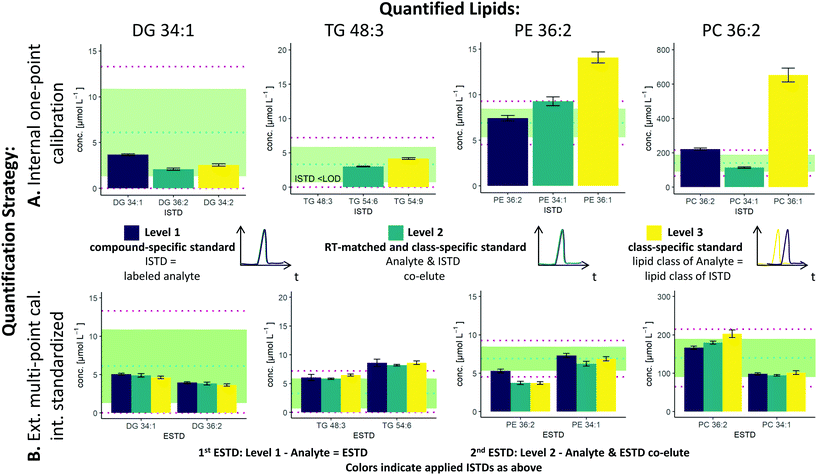 |
| Fig. 2 Influence of the selected ESTD and ISTD on (A) internal one-point calibration and (B) external multi-point calibration with internal standardization. Different results were obtained with different levels of ESTD and ISTD. Exemplarily, the quantitative results of the lipids DG 34:1, TG 48:3, PE 36:2 and PC 36:2 in SRM 1950 are shown. The blue dotted line shows the median of the mean (MEDM), the light green region shows the 95% confident interval (CI) of the interlaboratory comparison and the red dotted line shows the 99% CI. (A) The x-axis and the color for the one-point calibration show the applied ISTD. (B) The x-axis for the multi-point calibration shows the applied ESTD (left: level 1, right: level 2) and the colors show the applied ISTD (purple: level 1, blue: level 2, yellow: level 3). The color code for the ISTD in (B) is the same as that in (A). | |
As already mentioned, species-specific external calibration and internal standardization is the method of the highest metrological order. The isotope dilution strategy ensures accuracy by compensating for losses during sample preparation and for variations in MS measurement, provided that (1) high-purity external standards with certified concentrations are used and (2) equilibration of the LILY spike material and the sample is given. Considering typical experimental uncertainties of measured MS intensity ratios and sample preparation, the latter governed by extraction efficiencies and recoveries, typical total combined uncertainties of 4–7%, were expected (see Fig. 3). In fact, the experimentally observed uncertainties for biological replicates ranged from 2–7% when using level 1 ESTD and ISTD calibrations. When using level 2 external standardization with level 1 internal standardization, the ionization bias between the class-specific standard (ESTD) and investigated species contributes to the total combined uncertainty, while the contribution of sample preparation and MS detection was still minimized due to the species-specific ISTD. Introducing a correction factor – as an estimate of the contribution of the ionization efficiency distribution within the lipid class – in the model equation of the uncertainty budgets results in estimated uncertainties of up to 30% for neutral lipids. However, for polar lipids, this contribution was significantly lower, resulting in calculated uncertainties ranging at 10% (ESTD level 2, ISTD level 1), in accordance with the widely accepted hypothesis that the ionization efficiency is determined through the head group.3,34 When using level 2 ESTD and level 2 ISTD, for polar lipids, total combined uncertainties of 12–15% were estimated, while for neutral lipids, uncertainties of again up to 35% were calculated. The combined uncertainty of species-specific internal standardization (one-point calibration) by LILY was mainly determined by the uncertainty of the LILY quantification based on FI-HRMS. Error propagation results in estimated combined uncertainties ranging at 14% (assuming that the measured ratios are within the dynamic range and level 1 standardization). Again, in the case of quantifying LILY via level 2 standardization, the uncertainty for neutral lipids increased to 33% as the differences in ionization efficiency cannot be overcome without correction. When performing species-specific ISTD calibration (one-point calibration) with certified standards, 5–7% uncertainty has been estimated. It has to be mentioned that the estimated uncertainties of level 2 ISTD calibration is comparable regardless of whether synthetic certified standards or cost-saving LILY ISTDs were used due to the fact that the correction factor for ionization bias is the major contribution to the total combined uncertainty. Consequently, the quantitative output of the workflow was ranked according to these considerations and labeled accordingly. Quantification resorted to level 3 standardization only when level 1/2 ESTD and ISTD were not available. Again, multi-point calibration was preferred over one-point calibration.
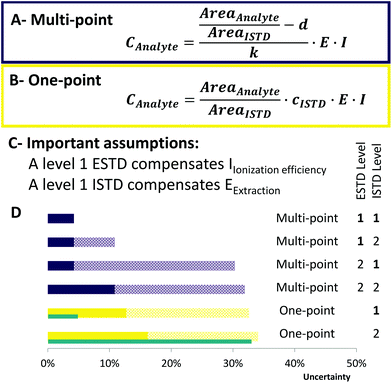 |
| Fig. 3 Uncertainty calculation of the applied methods. Concentration formula for (A) multi-point calibration and (B) one-point calibration. AreaAnalyte/AreaISTD: standard uncertainty of 3% in RP-LC, d – intercept, k – slope, E – extraction factor: E = 1, associated uncertainty 10%,35I – ionization efficiency factor: I = 1, associated uncertainty 30% (only for neutral lipids). (C) Assumptions that have been made to estimate the uncertainty of each calibration. (D) Ranking of the applied quantification strategies. Purple bars indicate multi-point calibration and yellow one-point calibration. The blue bars next to one-point calibration show the uncertainty if synthetic certified standards (uncertainty of 2%) had been used. Full color bars show the uncertainty for polar lipids, whereas patterned stacked bars show the uncertainty that can occur for neutral lipid classes (e.g. TG, CE and DG). One-point calibration considers LILY quantified upon level 2 standardization. | |
Application to human plasma lipidomics
Although the yeast lipidome might be less complex, retention time windows matching the respective plasma lipid classes were obtained (see Fig. 4A). Co-elution and thus co-ionization were supported by the inverse retention order observed with respect to increased carbon number versus increased double bond number, also described in the equivalent carbon number (ECN) model.36 For example, TG 48:3 (ECN: 42) co-eluted with TG 50:4, TG 52:5, TG 54:6, etc. (all ECN: 42, exemplarily, the TG elution profile is shown in ESI Fig. S3C,‡ also true for DG, LPC, LPE, PC, PI). This phenomenon increased the number of co-eluting LILY and plasma lipid species. For example, when analyzing the human plasma sample SRM 1950, LILY provided a species-specific ISTD (level 1) for 26% out of the 351 quantified lipid species (see Fig. 4B). For the great majority of lipid species (54%), a class-specific and RT-matched ISTD (level 2) was offered. Quantification of 9% of plasma lipids had to be based on a class-specific ISTD (level 3) only. Lipids from classes not present in LILY (SM, Hex2Cer and AcCa) could be quantified with external calibration, only resampling to 11% of the total quantified lipid species. These lipids followed a rather relative quantification approach and were excluded from accuracy assessments.
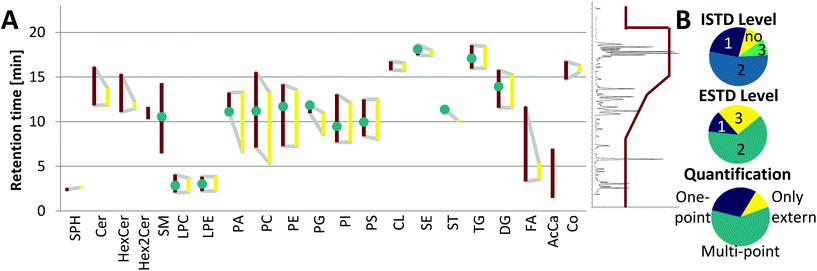 |
| Fig. 4 Application of LILY with RP-LC and its benefits. (A) Broad RT coverage: the RT coverage for different lipid classes (each polygon corresponds to one lipid class) is shown, the left red side of the polygon shows the elution range (1st to last eluting lipid of each class) of human plasma lipids, the right yellow side shows the elution range of LILY lipids, lipid classes shown as the vertical line are only present in plasma, and green dots show the elution time of the deuterated single-lipid-per-class mixture SPLASH® LIPIDOMIX® Mass Spec Standard. A total ion chromatogram and the gradient starting at 55% B and going up to 100% B are shown on the right side as visual help. (B) Distribution of the used ISTD, quantification strategies and the ESTD. ISTD: 80% of analytes have RT-matched ISTD; the level (defined by LSI)30 of the ISTD is shown. Quantification strategies: 59% of analytes are quantified via multi-point calibration; three different quantification strategies have been applied: multi-point, external multi-point calibration with internal standardization; one-point, surrogate internal standardization without external standardization; only external, multi-point calibration without the use of the ISTD and ESTD; 12% of analytes are available as the ESTD and 63% have co-ionized ESTD. | |
The FI/LC–HRMS lipidomics workflow was applied to the analysis to human plasma samples from 20 healthy donors striving to provide high lipidome coverage and accurate quantification. Lipid extraction of human plasma is well established.8,37–40 Spiking plasma with LILY increased the overall lipid amount of the sample by approximately 10%. Thus, the established Matyash protocol41 was adopted by reducing the plasma sample amount to a minimum of 10 μL, which still ensures homogeneity,17 and increasing the sample versus solvent ratio to 1
:
325. By automatizing the workflow and streamlining the data evaluation, manual curation of data was reduced to a minimum (for more details, see the ESI‡). The reference material for human plasma SRM 1950 – providing consensus values for 339 lipid species based on an international interlaboratory comparison – was used for method validation and data filtering.23
Three independent methods (QC accuracy and precision tests, recovery test considering standard addition of non-endogenous standards and Z-score calculation of SRM 1950) were implemented as quality control measures of the workflow next to the filter criteria described in the extended methods part in the ESI.‡ In total, quantitative values for 351 lipid species were obtained. 86 lipids from 7 lipid classes (LPC, LPE, PC, PE, PI, DG, TG) passed all quality filters. More specifically, accurate quantification was based on ESTD and ISTD panels passing the QC accuracy (accepting <30% trueness bias) and precision tests (accepting <30% relative standard deviation (RSD)) and the recovery test with bias <30% for the respective lipid class and obtaining a calculated Z-score within the 99% CI.
The total quantitative output of the novel workflow was compared to the state-of-the-art shotgun lipidomics strategy measured previously26 with regard to the calculated Z-score based on SRM 1950 data. As can be readily observed in Fig. 5A, the quantitative performance was comparable after both data sets were filtered by strict criteria to avoid misidentifications, including signal stability (RSD < 30%), mass accuracy (<3 ppm) and LOQ. However, the novel workflow benefits from increased sensitivity, leading to a higher number of quantified lipids, an increased selectivity, a higher number of standards and the possibility to control accuracy by the mentioned tests. Moreover, the quantitative values of the proposed workflow are traceable to external standards, offering an additional method independent of the widely used ISTD (deuterated lipids or lipids with odd, short or long chains, respectively).8 This enables cross-validation of different lipid methods and brings lipidomics a step forward in terms of standardization and harmonization.
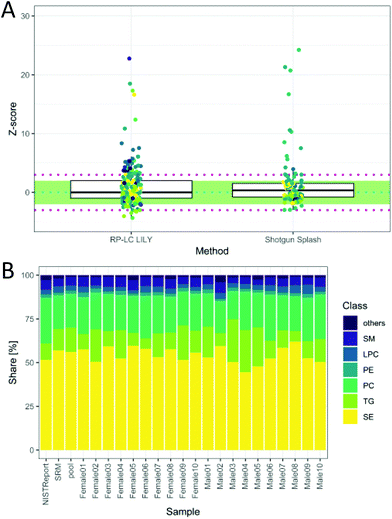 |
| Fig. 5 (A) Z-Score distribution of the presented workflow (RP-LC LILY) covering 208 lipids in comparison with the state-of-the-art quantification using SPLASH® LIPIDOMIX® in a shotgun nano-ESI experiment covering 99 lipids (coverage is determined by the NIST report). In ESI Fig. S6 and Table S2,‡ a more detailed version can be found. Data for shotgun experiment taken from a previous publication (Schoeny et al.26). (B) Relative lipid class distribution of the major lipid classes in human plasma without cholesterol. “Others” contains all lipid classes with a smaller contribution to the total lipid content than 2%. The NIST report shows the distribution from the consensus values, whereas SRM is the analyzed SRM 1950 sample. | |
The obtained relative lipid class distribution resembling the biological variance of the 20 healthy donors can be seen in Fig. 5B.21,42 On average, state-of-the-art lipidomics methods mentioned in the literature reported 50–300 annotated lipid species when quantification was mentioned.5 In this work, 351 lipids out of 429 identified lipids were quantified in human plasma providing new tools for data validation and ranking quantitative values after a stringent metrological order. Additionally, new tools enabling data validation have been introduced, offering a new way of method control and improving the reliability of all quantitative results.
Conclusions
Lipidome wide absolute quantification and validation were enabled via high-resolution mass spectrometry coupled with reversed-phase chromatography. As a prerequisite, the implementation of a reversed isotope dilution step increased the number of available lipid standards provided by fully labelled 13C yeast extracts in a cost-effective manner. Indeed, our experiments showed that the designed workflow provided a comparable analytical performance with regard to accuracy, uncertainty and number of absolute quantifications compared to established lipidomics platforms with the additional possibility to control accuracy via actual analyte QC accuracy and precision tests, recovery test considering standard addition of non-endogenous standards and Z-score calculation of SRM 1950. Hence, all acknowledged benefits of RP-LC–HRMS-based lipidomics, including possible isomer separation and superior sensitivity and selectivity, are now amenable without compromising the aspect of quantification. We think that the workflow increases the option of quantifying in the field of lipid analysis, offering an independent calibration strategy relying on different calibrants and standard resources, which is important regarding harmonization and standardization. Furthermore, due to the implementation of reversed isotope dilution via FI, this workflow can be used for any isotopically labeled biomass as the ISTD, enabling adaptation or self-production. Our study was restricted to quantification on a lipid species level based on MS1 measurements. In the future, the quantification capability could be extended to the molecular species level (i.e. known fatty acyl chain composition) by integrating MS2 measurements and the LC-separated isomers in the workflow.
Author contribution
Conceptualization: Schoeny, H.; Koellensperger, G.; data curation: Schoeny, H.; formal analysis: Schoeny, H.; funding acquisition: Koellensperger, G.; Rampler, E.; investigation: Schoeny, H.; Zach, O.; methodology: Schoeny, H.; Koellensperger, G.; project administration: Schoeny, H.; Koellensperger, G.; resources: Hermann, G.; Koellensperger, G.; software: Schoeny, H.; El Abiead, Y.; Hildebrand, F.; supervision: Koellensperger, G.; validation: Schoeny, H.; visualization: Schoeny, H.; writing—original draft preparation: Schoeny, H.; Koellensperger, G.; writing—review and editing: Schoeny, H.; Koellensperger, G.; El Abiead, Y.; Rampler, E.; all authors have read and agreed to the published version of the manuscript.
Conflicts of interest
This work is supported by the University of Vienna, the Faculty of Chemistry, the Vienna Metabolomics Center (VIME; http://metabolomics.univie.ac.at/) and the research platform Chemistry Meets Microbiology. The project was funded by the aws PRIZE prototype from the Austrian BMWFW Federal Ministry. ISOtopic solutions supplied the yeast cells for internal standardization.
Acknowledgements
The Mass Spectrometry Center (MSC), Faculty of Chemistry, University of Vienna, is acknowledged for providing mass spectrometric instrumentation. The authors thank all members of the environmental analysis (University of Vienna) group for continuous support.
References
- J. A. Bowden, C. Z. Ulmer, C. M. Jones, J. P. Koelmel and R. A. Yost, Metabolomics, 2018, 14(53), 1–5 CAS.
-
P. R. Baker, Current State of Quantitation in Lipidomics Analysis, https://avantilipids.com/discussions/current-state-of-quantitation-in-lipidomics-analysis, (accessed 12 February 2020).
- M. Wang, C. Wang and X. Han, Mass Spectrom. Rev., 2017, 36, 693–714 CrossRef CAS PubMed.
- E. Rampler, Y. El Abiead, H. Schoeny, M. Rusz, F. Hildebrand, V. Fitz and G. Koellensperger, Anal. Chem., 2021, 93(1), 519–545 CrossRef CAS PubMed.
- T. Cajka and O. Fiehn, Trends Anal. Chem., 2014, 1, 192–206 CrossRef PubMed.
- M. Lange, Z. Ni, A. Criscuolo and M. Fedorova, Chromatographia, 2019, 82, 77–100 CrossRef CAS.
- T. Hyötyläinen, L. Ahonen, P. Pöhö, M. Orešič and M. Oresic, Biochim. Biophys. Acta, Mol. Cell Biol. Lipids, 2017, 1862, 800–803 CrossRef PubMed.
- M. Holčapek, G. Liebisch and K. Ekroos, Anal. Chem., 2018, 90, 4249–4257 CrossRef PubMed.
- B. Burla, M. Arita, M. Arita, A. K. Bendt, A. Cazenave-gassiot, E. A. Dennis, K. Ekroos, X. Han, K. Ikeda, G. Liebisch, M. K. Lin, T. P. Loh, P. J. Meikle, M. Oreši, O. Quehenberger, A. Shevchenko, F. Torta, M. J. O. Wakelam, C. E. Wheelock and M. R. Wenk, J. Lipid Res., 2018, 59, 2001–2017 CrossRef CAS PubMed.
- E. Rampler, C. Coman, G. Hermann, A. Sickmann, R. Ahrends and G. Koellensperger, Analyst, 2017, 142, 1891–1899 RSC.
- E. Rampler, D. Egger, H. Schoeny, M. Rusz, M. P. Pacheco, G. Marino, C. Kasper, T. Naegele and G. Koellensperger, Molecules, 2019, 24(3615), 1–19 Search PubMed.
- E. Rampler, A. Criscuolo, M. Zeller, Y. El Abiead, H. Schoeny, G. Hermann, E. Sokol, K. Cook, D. A. Peake, B. Delanghe and G. Koellensperger, Anal. Chem., 2018, 90, 6494–6501 CrossRef CAS PubMed.
- C. S. Ejsing, M. Bilgin and A. Fabregat, PLoS One, 2015, 10, 1–17 CrossRef PubMed.
- A. Demirkan, A. Isaacs, P. Ugocsai, G. Liebisch, M. Struchalin, I. Rudan, J. F. Wilson, P. P. Pramstaller, U. Gyllensten, H. Campbell, G. Schmitz, B. A. Oostra and C. M. Van Duijn, J. Psychiatr. Res., 2013, 47, 357–362 CrossRef PubMed.
-
Food and Drug Administration, Bioanalytical Method Validation Guidance for Industry, 2018 Search PubMed.
- J. Kurz, M. J. Parnham, G. Geisslinger and S. Schiffmann, Trends Mol. Med., 2019, 25, 20–32 CrossRef CAS PubMed.
- L. A. Heiskanen, M. Suoniemi, H. X. Ta, K. Tarasov and K. Ekroos, Anal. Chem., 2013, 85, 8757–8763 CrossRef CAS PubMed.
- W. Yang, Y. Chen, C. Xi, R. Zhang, Y. Song, Q. Zhan, X. Bi and Z. Abliz, Anal. Chem., 2013, 85, 2606–2610 CrossRef CAS PubMed.
- M. Haid, C. Muschet, S. Wahl, W. Römisch-Margl, C. Prehn, G. Möller and J. Adamski, J. Proteome Res., 2018, 17, 203–211 CrossRef CAS PubMed.
- M. Pietzner, A. Kaul, A. Henning, G. Kastenmüller, A. Artati, M. M. Lerch, J. Adamski, M. Nauck and N. Friedrich, BMC Med., 2017, 15, 1–12 CrossRef PubMed.
- A. Triebl, B. Burla, J. Selvalatchmanan, J. Oh, S. H. Tan, M. Y. Chan, N. A. Mellet, P. J. Meikle, F. Torta and M. R. Wenk, J. Lipid Res., 2020, 61, 105–115 CrossRef CAS PubMed.
- T. Cajka, J. T. Smilowitz and O. Fiehn, Anal. Chem., 2017, 89, 12360–12368 CrossRef CAS PubMed.
- J. A. Bowden, A. Heckert, C. Z. Ulmer, C. M. Jones, J. P. Koelmel, L. Abdullah, L. Ahonen, Y. Alnouti, A. Armando, J. M. Asara, T. Bamba, J. R. Barr, J. Bergquist, C. H. Borchers, J. Brandsma, S. B. Breitkopf, T. Cajka, A. Cazenave-Gassiot, A. Checa, M. A. Cinel, R. A. Colas, S. Cremers, E. A. Dennis, J. E. Evans, A. Fauland, O. Fiehn, M. S. Gardner, T. J. Garrett, K. H. Gotlinger, J. Han, Y. Huang, A. H. Neo, T. Hyötyläinen, Y. Izumi, H. Jiang, H. Jiang, J. Jiang, M. Kachmann, R. Kiyonami, K. Klavins, C. Klose, H. C. Köfeler, J. Kolmert, T. Koal, G. Koster, Z. Kuklenyik, I. J. Kurland, M. Leadley, K. Lin, K. R. Maddipati, M. Danielle, P. J. Meikle, N. A. Mellett, C. Monnin, M. A. Moseley, R. Nandakumar, M. Oresic, R. Patterson, D. Peake, J. S. Pierce, M. Post, A. D. Postle, R. Pugh, Y. Qiu, O. Quehenberger, P. Ramrup, J. Rees, B. Rembiesa, D. Reynaud, M. R. Roth, S. Sales, K. Schuhmann, M. L. Schwartzman, C. N. Serhan, A. Shevchenko, S. E. Sommerville, L. St John-Williams, M. A. Surma, H. Takeda, R. Thakare, J. W. Thompson, F. Torta, A. Triebl, M. Trötzmüller, K. Ubhayasekera, D. Vuckovic, J. M. Weir, R. Welti, M. R. Wenk, C. E. Wheelock, L. Yao, M. Yuan, X. H. Zhao and S. Zhou, J. Lipid Res., 2017, 58(12), 2275–2288 CrossRef CAS PubMed.
- J. W. Thompson, K. J. Adams, J. Adamski, Y. Asad, D. Borts, J. A. Bowden, G. Byram, V. Dang, W. B. Dunn, F. Fernandez, O. Fiehn, D. A. Gaul, A. Fr, A. Kalli, T. Koal, S. Koeniger, R. Mandal, F. Meier, F. J. Naser, D. O. Neil, A. Pal, G. J. Patti, H. Pham-tuan, C. Prehn, F. I. Raynaud, T. Shen, A. D. Southam, L. S. John-williams, K. Sulek, C. G. Vasilopoulou, M. Viant, C. L. Winder, D. Wishart, L. Zhang, J. Zheng and M. A. Moseley, Anal. Chem., 2019, 91, 14407–14416 CrossRef CAS PubMed.
- S. Neubauer, C. Haberhauer-Troyer, K. Klavins, H. Russmayer, M. G. Steiger, B. Gasser, M. Sauer, D. Mattanovich, S. Hann and G. Koellensperger, J. Sep. Sci., 2012, 35, 3091–3105 CrossRef CAS PubMed.
- H. Schoeny, E. Rampler, G. Hermann, U. Grienke, J. M. Rollinger and G. Koellensperger, Anal. Bioanal. Chem., 2020, 412, 2365–2374 CrossRef CAS PubMed.
-
J. I. G. Alonso and P. Rodriguez-González, Isotope Dilution Mass Spectrometry, Royal Society of Chemistry, 2013 Search PubMed.
- K. Schuhmann, R. Almeida, M. Baumert, R. Herzog, S. R. Bornstein and A. Shevchenko, J. Mass Spectrom., 2012, 47, 96–104 CrossRef CAS PubMed.
- M. Höring, C. S. Ejsing, M. Hermansson and G. Liebisch, Anal. Chem., 2019, 91, 3459–3466 CrossRef PubMed.
-
Lipidomics Standards Initiative, Lipid Species Quantifciaton, https://lipidomics-standards-initiative.org/guidelines/lipid-species-quantification, (accessed 12 February 2020) Search PubMed.
- C. Z. Ulmer, J. M. Ragland, J. P. Koelmel, A. Heckert, C. M. Jones, T. Garrett, R. A. Yost and J. A. Bowden, Anal. Chem., 2017, 89, 13069–13073 CrossRef CAS PubMed.
-
J. A. Bowden, A. Heckert, C. Z. Ulmer and C. M. Jones, Lipid Concentrations in Standard Reference Material (SRM) 1950: Results from an Interlaboratory Comparison Exercise for Lipidomics, 2017 Search PubMed.
- M. Lange and M. Fedorova, Anal. Bioanal. Chem., 2020, 412, 3573–3584 CrossRef CAS PubMed.
- M. Koivusalo, P. Haimi, L. Heikinheimo, R. Kostiainen and P. Somerharju, J. Lipid Res., 2001, 42, 663–672 CrossRef.
- R. E. Patterson, A. J. Ducrocq, D. J. Mcdougall, T. J. Garrett and R. A. Yost, J. Chromatogr. B: Anal. Technol. Biomed. Life Sci., 2015, 1002, 260–266 CrossRef CAS PubMed.
- M. Lísa and M. Holcapek, J. Chromatogr. A, 2008, 1199, 115–130 CrossRef PubMed.
- J. Folch, M. Lees and G. H. Sloane Stanley, J. Biol. Chem., 1957, 226, 497–509 CrossRef CAS.
- E. G. Bligh and W. J. Dyer, Can. J. Biochem. Physiol., 1959, 37, 911–917 CrossRef CAS PubMed.
- C. Z. Ulmer, C. M. Jones, R. A. Yost, T. J. Garrett and J. A. Bowden, Anal. Chim. Acta, 2018, 1037, 351–357 CrossRef CAS PubMed.
- X. Han, Front. Biosci., 2007, 12, 2601–2615 CrossRef CAS PubMed.
- V. Matyash, G. Liebisch, T. V. Kurzchalia, A. Shevchenko and D. Schwudke, J. Lipid Res., 2008, 49, 1137–1146 CrossRef CAS PubMed.
- O. Quehenberger, A. M. Armando, A. H. Brown, S. B. Milne, D. S. Myers, A. H. Merrill, S. Bandyopadhyay, K. N. Jones, S. Kelly, R. L. Shaner, C. M. Sullards, E. Wang, R. C. Murphy, R. M. Barkley, T. J. Leiker, C. R. H. Raetz, Z. Guan, G. M. Laird, D. a. Six, D. W. Russell, J. G. McDonald, S. Subramaniam, E. Fahy and E. a. Dennis, J. Lipid Res., 2010, 51, 3299–3305 CrossRef CAS PubMed.
- K. Haug, K. Cochrane, V. C. Nainala, M. Williams, J. Chang, K. V. Jayaseelan and C. O. Donovan, Nucleic Acids Res., 2020, 48, 440–444 Search PubMed.
Footnotes |
† Raw data available on MetaboLights43http://www.ebi.ac.uk/metabolights/MTBLS1876. |
‡ Electronic supplementary information (ESI) available: Abbreviations, extended materials and methods, additional figures and tables; XLSX—quantitative results, quantification strategy comparison, figures of merit, quantified LILY lipids with FI-HRMS, the MS sequence list; ZIP—MFQL files for LipidXplorer. See DOI: 10.1039/d0an02443k |
|
This journal is © The Royal Society of Chemistry 2021 |