DOI:
10.1039/D0MA00573H
(Paper)
Mater. Adv., 2020,
1, 2818-2830
A water destructible SnS2 QD/PVA film based transient multifunctional sensor and machine learning assisted stimulus identification for non-invasive personal care diagnostics†
Received
6th August 2020
, Accepted 21st September 2020
First published on 22nd September 2020
Abstract
With the advent of the internet of things, where millions of sensors are connected, they come with two important problems: disposability of the fabricated sensors and the accurate classification of the sensor data. Even though there are reports on multifunctional sensors, research into tackling both the disposability and the accurate frontend processing of the sensor data remains limited. This report demonstrates for the first time the use of a water soluble SnS2 QD/PVA film as a multifunctional sensor for both physical (strain, pressure) and chemical (breath) stimuli and further incorporating machine learning algorithms for the accurate classification of the sensor data. The water-soluble nature of the fabricated sensor allows for its easy disposability wherein the sensor completely dissolves in ∼360 seconds when immersed in water. The fabricated sensor exhibited an excellent pressure sensitivity of ∼10.14 kPa−1 and a gauge factor of ∼3.08 and can distinguish various respiration patterns. The multifunctional sensor data were subjected to machine learning algorithms wherein the data were trained to classify the stimulus with the highest accuracy of 87.7%. Combining both, the sensor has been projected for real time applications such as human motion monitoring, touch sensors, and breath analysers.
1. Introduction
The development of modern silicon-based CMOS electronics has typically focused on long lifetime, miniaturization, and stability over time. In contrast, water soluble electronics takes an opposite approach wherein the fabricated device can be disintegrated upon use. The development of transient electronics has gained researchers’ attention, offers unique possibilities and allows a wide variety of applications in biosensors,1,2 flexible and stretchable electronics,3 environmental sensors,4 and hardware-secure electronics.5 The conventional materials used for transient electronics (water soluble) include silk protein, cellulose, polyvinyl alcohol (PVA), and poly lactic-co-glycolic acid (PLGA), however, these only serve as substrates and for embedding devices.6 Even though there are reports on the use of various materials for transient electronics, one of the major issues that remain unaddressed is the ability of the device to utilize functional nanomaterials, which are mostly not soluble in water.7 Such functional nanomaterials offer exciting electronic, chemical, and mechanical properties which not only enhance the device functionality but also offer a plethora of applications that can be targeted.
Personal health monitoring has become an indispensable part of human life and if it is cost-effective, accessible, and user-friendly, then it can be used to assist and monitor the health of an individual even without the use of sophisticated equipment.8,9 Monitoring of personal healthcare requires sensitive multifunctional sensors that can sense both physical (strain and pressure) and chemical (breath) stimuli.10 Achieving such high sensitivity along with the detection of different stimuli requires extremely sensitive transducers that can provide a high signal to noise ratio and a particular sensing pattern that is extremely specific to particular stimuli. Multifunctional sensors handle complex inputs from different stimuli and differentiating such complex input data is a challenging task and usage of the same sensor for multiple sensing applications often leads to data misclassification.11 One of the major issues of the multifunctional sensor is the inability of the frontend processing to accurately predict the stimuli because of low recognition and high cross-sensitivity.12 Many methods have been utilized such as principal component analysis, pattern recognition, etc. which do not accurately predict the stimuli.13 Hence there is an urgent need to develop a robust multifunctional sensor system that would not only sense various stimuli but also accurately predict the stimuli.
Machine learning techniques have gained widespread interest in many fields, particularly in identifying psychophysical signals from human activities, which aid in easy diagnosis.14–16 Electrocardiogram (ECG) and photoplethysmogram (PPG) signals obtained from a wearable sensor, wherein 21 features are extracted from those signals were used to train the various machine learning algorithms for the estimation of cardiovascular dysfunction.15 The extracted features from worn miniature inertial and magnetic sensors were used in different machine learning classifiers for the detection of human activity.16 Similarly, smart watch sensor data obtained from inertial sensors were used to extract optimum features using filter and wrapper approaches for human activity identification, validation, and testing via a support vector machine (SVM).17 In another reported work, the digital shade matching device for dental colour determination was adopted to identify the optimal solution for quantitative tooth colour measurement.18 The above literature suggests that machine learning has huge potential for the frontend classification of the multifunctional sensor but the study towards it remains unexplored.
In search of materials for transient electronics, various natural biopolymers have been utilized as a substrate but the major issue that needs to be tackled is the use of functional nanomaterials.19 Since most of the functional nanomaterials are water insoluble, proper functionalization is required to make them available for water soluble electronics. Transition metal dichalcogenides (TMDs) are two-dimensional (2D) materials and have gained considerable interest in recent years due to their excellent chemical, mechanical and electronic properties.20 One such transition metal dichalcogenide is tin disulfide (SnS2), which exhibits excellent electronic and chemical properties and is non-toxic.21–23 Furthermore, its earth abundance makes it a low-cost alternative to conventional TMDs such as MoS2 and WS2.24 But, SnS2 in its 2D form is water insoluble and hence cannot be utilized directly for water soluble electronics. On the other hand, SnS2 Quantum Dots (QDs) are the 0D counterpart of 2D SnS2 exhibiting similar excellent electronic properties and due to their very small size, they are not visible to the naked eye after dissolving them in water which makes them ideal for transient (water soluble) electronics.23 Also, the mechanical properties of PVA have been studied extensively25,26 and it has been utilized as a substrate for pressure and strain sensors.27,28 Furthermore, research into SnS2 QDs for their usage as a multifunctional sensor is rare and it would be very interesting to study the response of SnS2 QD/PVA towards different physical and chemical stimuli.
This paper demonstrates for the first time the use of three important and emergent technologies i.e. transient electronics and multifunctional sensors with stimulus identification using machine learning. SnS2 QDs were utilized which were synthesized using the hydrothermal and sonochemistry method. QDs have a very large surface to volume ratio and high reactivity which is essential for breath sensing applications. Furthermore, the stain dependent bandgap of quantum dots has paved the way for their use in physical sensors such as pressure and strain sensors.29 Also, SnS2 in its natural 2D form is water insoluble but SnS2 in 0D exhibits similar exciting properties to those of 2D SnS2 with the advantage of extremely low size and after dissolving in water is not visible to the naked eye. In addition, the band gap of QDs is tunable and has extremely high photo stability and chemical stability.30 Detailed structural and morphological characterization was performed and the results confirmed the formation of SnS2 quantum dots. Embedding a particular concentration of SnS2 QDs in PVA leads to the formation of a conducting water soluble film which was utilized for physical (strain and pressure) and chemical (breath) sensing. It is important to note that the plain PVA film showed very high resistance (∼5.4 GΩ) and the conductivity of the fabricated device is due to SnS2 QDs only. Furthermore, the use of machine learning algorithms allowed for the accurate prediction of the stimulus as for all the sensors, the transduction mechanism is resistive. Also, the fabricated sensors were tested in real time by integrating onto human body parts for human motion monitoring, embedding the device in a face mask for monitoring different respiration rates, and finally a human touch sensor. The detailed physical mechanism for each sensor is explained in terms of charge transport and tunnelling phenomena and also the transience (water solubility) is explained by the hydrolysis process. Successful demonstration of the SnS2 QD/PVA (non-toxic and biodegradable) based water soluble multifunctional sensor with accurate stimulus identification holds potential applications in the field of security, biomedicine, E-waste, internet of things, etc.
2. Experimental section
A SnS2 QD/PVA film was fabricated via a two-step process wherein in the first step SnS2 QDs were synthesized using the hydrothermal and exfoliation method and in the second step, PVA (Mw: 61
000 g mol−1, 98.0–98.8 mol% hydrolysis) was dispersed in SnS2 QDs to form a SnS2 QD/PVA conducting film.
2.1. Synthesis of SnS2 QDs
The hydrothermal method was utilized to synthesize SnS2. In brief, 262 mg tin(IV) chloride (SnCl4.5H2O) and 114 mg of thiourea (NH2CSNH2) were ultra-sonicated in 30 mL deionized (DI) water for 15 minutes to obtain a transparent solution. Afterward, the precursor solution was transferred to the hydrothermal reactor and held at 200 °C for 7 h followed by natural cooling. Thereafter, the solution was centrifuged 3 times with ethanol at 4000 rpm for 10 minutes to remove the residue of the reactant and then dried at 60 °C for 4 h. The obtained SnS2 powder (10 mg) was dispersed in 20 mL of NMP and was sonicated with an ice bath continuously for 6 h to obtain the SnS2 QDs.
2.2. Fabrication of the SnS2 QD/PVA film
The SnS2 QD/PVA film was fabricated by dispersing 300 mg of PVA (3 wt%) in 10 mL of SnS2 QD solution by continuous stirring for 6 hours at 60 °C. After the proper dispersion, the solution casting method was employed wherein the solution was poured in a Petri dish and then dried in an oven at 60 °C for 24 h. The complete process including the synthesis and the fabrication is shown in Fig. 1.
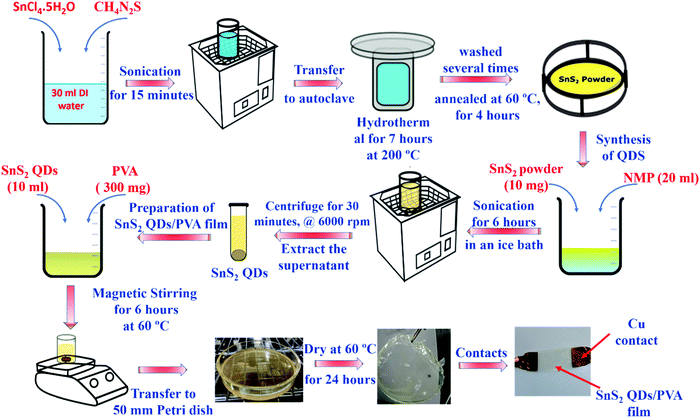 |
| Fig. 1 Schematic demonstration of the synthesis and fabrication of the SnS2 QD/PVA film. | |
2.3. Sensor fabrication
The fabrication of the multifunctional sensor was done by cutting the obtained SnS2 QD/PVA film into required dimensions (2 cm × 2 cm), and the contacts were fabricated using copper tape. Fig. 1 shows the complete synthesis and schematic procedure.
3. Results and discussion
The structure and morphology of the as-synthesized SnS2 QDs were studied by transmission electron microscopy (TEM). Fig. 2a and b shows the TEM image of well dispersed SnS2 QDs which have nearly spherical morphology with varying particle size indicating polydispersity. The average diameter of the SnS2 QDs determined from the TEM image lies in the range of 3.99 ± 1.58 nm, as depicted in Fig. 2c. The high-resolution transmission electron microscopy (HRTEM) images of SnS2 QDs given in Fig. 2d show the lattice fringes separated by 0.275 nm, which corresponds to the (101) crystal planes of hexagonal structured SnS2 (JCPDS No: 23-0677).24 The corresponding selective area electron diffraction (SAED) pattern displays multiple rings and scattered dots revealing the polycrystalline nature and dominant (001) and (101) diffraction planes as shown in Fig. 2e.
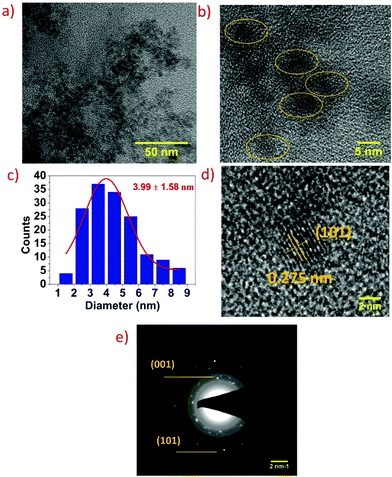 |
| Fig. 2 (a and b) TEM image of the synthesized SnS2 QDs, (c) size distribution of SnS2 QDs, calculated average diameter of SnS2 QDs is 3.99 ± 1.58 nm, (d) HRTEM lattice fringes of SnS2 QDs, and (e) SAED pattern of SnS2 QDs. | |
The optical properties of the as-synthesized SnS2 QDs were investigated using UV-vis spectroscopy and photoluminescence (PL) spectroscopy. Fig. 3a shows the UV-vis spectra of SnS2 QDs, and the spectra were obtained over a wide wavelength range of 300–800 nm. The direct bandgap of SnS2 QDs was calculated from the (αhν)2 data plotted against the energy (hν) as shown in the inset of Fig. 3a and it was found to be ∼3.13 eV. It should be noted that the bandgap measured is for SnS2 QDs and not bulk SnS2 and hence the measured bandgap is higher than the reported value of bulk SnS2. The calculated bandgap is in agreement with the reported literature for SnS2 QDs.31,32 The increase in the bandgap when compared to the bulk or 2D counterpart is due to the quantum confinement effect wherein as the size decreases the bandgap increases.33 SnS2 QDs at an excitation wavelength of 360 nm showed a strong blue fluorescence with an intense peak at ∼430 nm and a red shift was observed in the emission peak of SnS2 QDs as the excitation wavelength increases from 345 nm to 390 nm as shown in Fig. 3b. The red shift is due to the different radii of quantum dots and it decreases with an increase in the radius of QDs.34 The red shift, principally, occurs if either the top of the valence band is an optically passive state or if the electron and the hole are in a triplet state.34 The red shift is also referred to as a Stokes shift and it arises due to the distance between the optically active states, and as the optical ground state increases there is an increase of the Stokes shift.35 To further study the elemental composition, binding characteristics, and surface chemical states of SnS2 QDs, XPS analysis was performed. Fig. 3c shows the wide range XPS spectra of SnS2 QDs wherein binding energy peaks confirm the presence of Sn, S, C, and O elements. Oxygen was present in spectra due to the partial oxidation of SnS2 QDs upon exposure to ambient conditions and carbon was present because of the carbon tape used while performing the XPS measurements. The deconvoluted carbon 1s spectra show the presence of C–C and C–O as seen in Fig. 3d. High resolution spectra of Sn 3d are shown in Fig. 3e, wherein the characteristic peaks corresponding to Sn 3d5/2 and Sn 3d3/2 were observed at 486.6 eV and 495.2 eV and the difference of ∼8.4 eV between these two peaks clearly shows the +4 oxidation state of Sn in SnS2 QDs.36,37Fig. 3f shows the high-resolution spectra of S 2p wherein two peaks at 162.9 eV and 161.6 eV signify the presence of S 2p1/2 and S 2p3/2 which correspond to the 2-oxidation state of S in SnS2 QDs.38
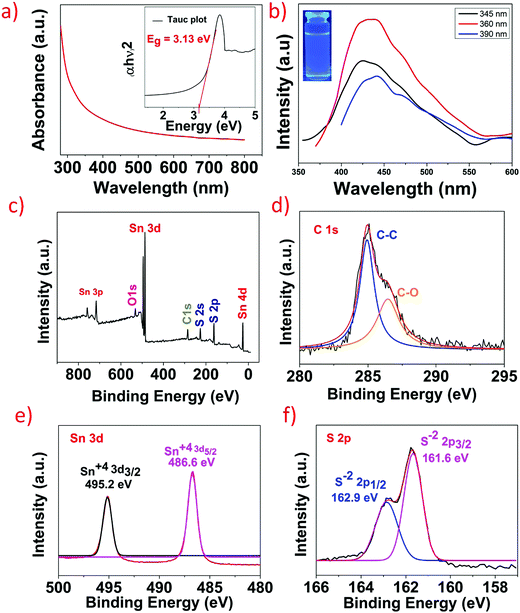 |
| Fig. 3 (a) UV-Visible absorption spectra of SnS2 QDs; the inset is the Tauc plot showing a bandgap of ∼3.13 eV. (b) Photoluminescence spectra of SnS2 QDs at different excitation from 345 nm to 390 nm. (c) XPS survey spectra of SnS2 QDs. (d) High resolution spectra of C 1s. (e) High resolution spectra of Sn 3d. (f) High resolution spectra of S 2p. | |
3.1. Strain sensing
Wearable strain sensors have been intensively studied to monitor human motion.39–41 The development of high sensitivity and low-cost devices is critical to meet the needs of wearable electronics. The sensing material SnS2QDs are embedded in a water soluble polyvinyl alcohol (PVA) polymer substrate for the fabrication of a flexible strain sensor, which is resistant to various strains. The average conductance of the SnS2 QD/PVA film was measured to be 2.04 × 10−8 S (N = 4). The characteristics of current vs. voltage (I–V) under various strains are shown in Fig. 4a and b. A linear relationship is exhibited by the I–V characteristics indicating that the fabricated sensor exhibits ohmic contacts. By increasing the applied compressive strain, the slope of the I–V curve increases suggesting the increase in the sensor current. Conversely, when increasing tensile strains were applied, the slope of the I–V characteristics decreases suggesting the decrease in the sensor current under the external tensile strain. To verify whether the fabricated sensor was able to repeatedly sense the applied tensile and compressive strain, a constant compressive and tensile strain of ∼15% was applied and the temporal response is shown in Fig. 4c and d respectively. The fabricated sensor was able to repeatedly measure the constant strain with much accuracy suggesting the reliability of the fabricated sensor. Fig. 4e and f shows the temporal response of the fabricated sensor upon increasing compressive strain and tensile strains and as it is evident from the graph, the sensor current increases upon increasing the compressive strain and the sensor current decreases upon increasing the tensile strain which is in good agreement with the I–V characteristics. The gauge factor of the strain sensor is defined as
, where ΔR is the resistance variation upon strain loading, ε is the applied strain, and R0 is the initial resistance. As seen in Fig. 4g, the gauge factor of the fabricated strain sensor under tensile strain was calculated to be ∼3.08. It is worth noting that the gauge factor calculated was comparable to the gauge factor of the devices fabricated using sophisticated techniques.42,43 To verify the reliability of the fabricated sensor, the device was subjected to 1000 cycles of compressive strain, as can be observed from Fig. 4h in which a negligible change in the device performance was observed suggesting the excellent reliability of the fabricated SnS2 QD/PVA sensor. Response time is one of the important figures of merit for the strain sensor and is calculated by taking the time difference of the sensor signal to reach from 10% to 90% of its maximum value. As can be seen from Fig. 4i and j, the rise time and fall time of the sensor subjected to compressive strain were found to be ∼1.2 s and ∼690 ms respectively.
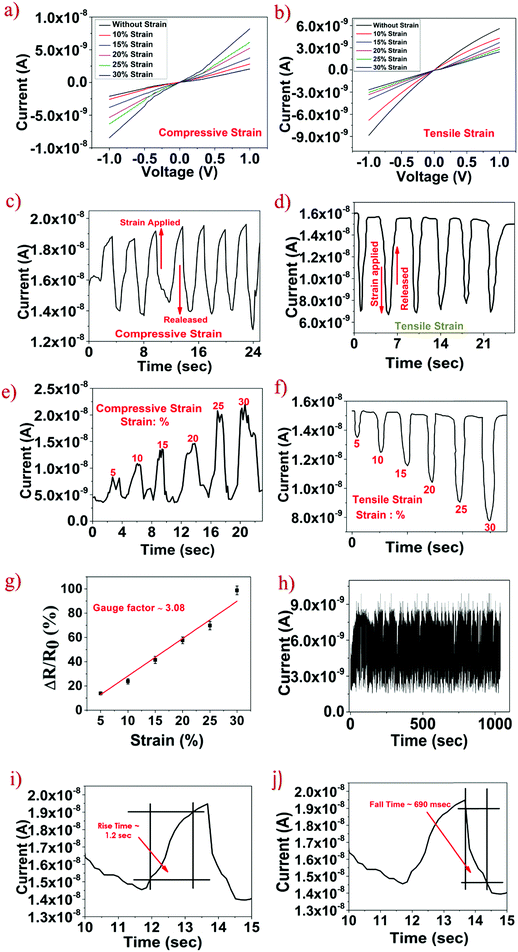 |
| Fig. 4 (a and b) I–V characteristics of the SnS2 QD/PVA film under compressive and tensile strain respectively. (c and d) Continuous-time sensor response under constant compressive and tensile strains. (e and f) Temporal response of the sensor with an increase in compressive and tensile strains. (g) Graph showing the normalized resistance (%) vs. strain (%), gauge factor calculated to be ∼3.08. (h) Response of the fabricated sensor for 1000 strain cycles. (i and j) Rise time and fall time of the strain sensor were ∼1.2 s and ∼690 ms. | |
The increase in the sensor current due to the applied strain can be understood by the piezoresistive phenomenon in QDs. The homogeneous distribution of aggregated QDs in the SnS2 QD/PVA film facilitates the conduction current. The piezoresistive effect in QDs can be ascribed to the tunneling effect between adjacent QDs. In the SnS2 QD/PVA film, quantum dots are separated in the subnanometer scale (1–3 nm) as evident from the TEM image. When compressive strain is applied on the device, nanoscopic gaps between the adjacent QDs reduce which decreases the tunneling resistance. The decrease in the tunneling resistance eventually increases the overall sensor current. Similarly, when tensile strain (i.e. bent outwards) is applied on the SnS2 QD/PVA film, the distance between the QDs increases which further increases the tunneling resistance that leads to a decrease in the sensor current. Furthermore, the fabricated sensor exhibits a low hysteresis graph (ESI,† Fig. S1) which can be attributed to no cracks or ruptures in the SnS2 QD/PVA film due to an external strain applied thereby suggesting that the tunneling process plays a prominent role in the sensor current change.
To test the fabricated strain sensor for real time human motion monitoring, the flexible SnS2 QD/PVA device was mounted on the human body to monitor the movement of the thumb finger joint, elbow, knee, and human neck as shown in Fig. 5. Two ends of the sensor are attached with adhesive tape for safe contact of the sensor on the body. As shown in Fig. 5a the sensor attached to the thumb experiences the tensile strain resulting from thumb bending. The resistance increases when the thumb bends and recovered to its initial value during thumb straightening. When a sensor is mounted on the outer surface of the elbow and knee, a similar sensor resistance response behaviour was observed as shown in Fig. 5b and c, furthermore, when the sensor was integrated onto the neck to monitor movements it induces compressive strain during the bending of the neck which resulted in reduced resistance as shown in Fig. 5d. The results clearly show that the fabricated SnS2 QD/PVA sensor is capable of monitoring the joint movements whether it is compressive or tensile movement.
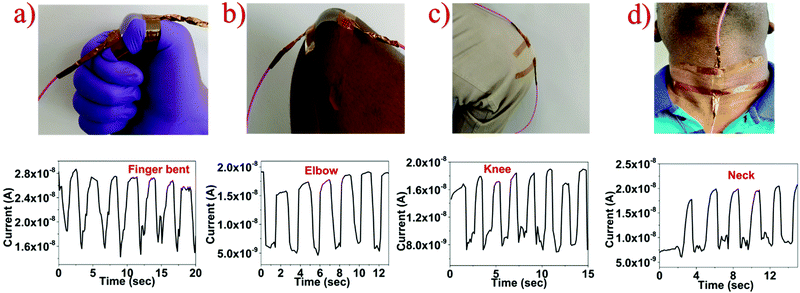 |
| Fig. 5 Human motion monitoring with a fabricated strain sensor integrated on different body parts: (a) bent finger, (b) elbow, (c) knee and (d) neck. | |
3.2. Breath sensing
Another parameter for monitoring personal health is human breath. The breath pattern of an individual is one of the most significant aspects of physiological condition assessment in real-time monitoring and it plays a vital role in the management of patients in the biomedical sector.44 The prepared SnS2 QD/PVA film was cut into 2 cm × 2 cm dimensions, and copper tape was used for contacts. Fig. 6a shows the cross-section schematic wherein the active area of a breath sensor is 1.4 cm × 2 cm. The sensor was placed at a distance of 4 cm from the nose of an individual as illustrated in Fig. 6b. As shown in Fig. 6c the sensor responds towards the normal breath pattern of a healthy individual and as can be seen upon exhalation the sensor current increases and during inhalation the sensor current decreases. It is worth noting that the sensor current does not reach the baseline (initial) value during the inhalation process which can be attributed to the slower desorption process of water molecules from the SnS2 QD/PVA film surface. However, the unstable baseline is not important in the application targeted and depends on the number of peaks to monitor various respiration patterns. To test whether the fabricated sensor was able to sense and differentiate between the respiration patterns, the device was subjected to various modulations of human breath. The frequency and the amplitude of the sensor current peaks were utilized to distinguish between the different breathing patterns. Fig. 6d shows the temporal response of the fabricated sensor towards slow breaths (9 breaths per min) wherein the sensor was able to recover the initial current because of the larger time gaps between the consecutive exhalations. Fig. 6e displays the response of the sensor towards faster breaths (42 breaths per min) and because of the lesser time gap between the consecutive exhalations the sensor was not able to regain its initial current/resistance value. The results suggest that the fabricated device was able to distinguish between the respiration rates based on the frequency of sensor current peaks. To verify whether the sensor was able to repeatedly sense human breath exhalations, iterative breath was taken wherein the exhalation was performed 3 times and then stopped and the same was repeated 5 times. As seen in Fig. 6f the sensor was able to repeatedly sense exhalation suggesting excellent repeatability and reliability of the fabricated sensor. Furthermore, the rise time and fall time of the fabricated sensor were calculated by taking the time difference of the sensor response to reach from 10% to 90%. As seen in Fig. 6g and h, the rise time of the fabricated breath sensor was ∼300 ms and the fall time measured was ∼710 ms.
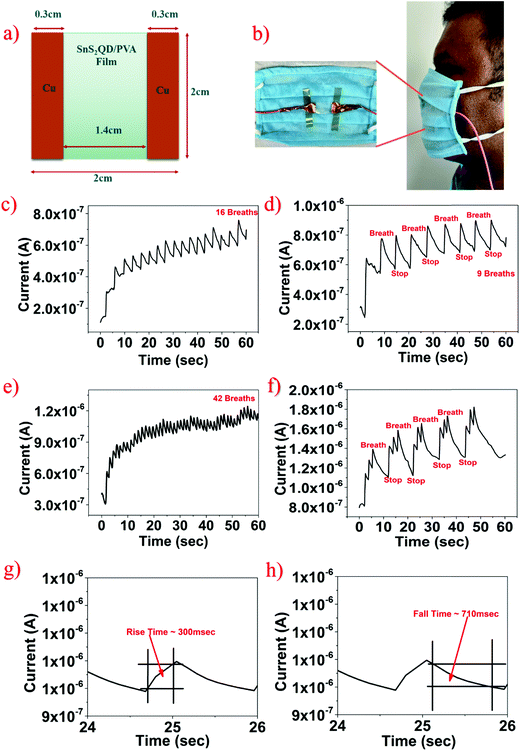 |
| Fig. 6 (a) Schematic of a breath sensor. (b) Integration of the sensor in the breath mask. (c) Continuous-time response of SnS2 QD/PVA under normal breath. (d and e) Slow and fast breath patterns over a period of 60 seconds. (f) Three breath iterative breathing patterns for five cycles. (g and h) The rise time and fall time of the fabricated sensor were ∼300 ms and ∼710 ms. | |
The sensing mechanism of the SnS2 QD/PVA film can be attributed to the water (H2O) molecule adsorption and desorption phenomena. During the exhalation process, the surface of the SnS2 QD/PVA film adsorbs breath water (H2O) molecules, and since these molecules are electron donor species, upon adsorption the sensor current increases. In the presence of an electric field, human breath water molecules get ionized and form H3O+ ions and an electron. SnS2 is an n-type material and hence upon exhalation the majority carrier concentration increases increasing the sensor current. Similarly, during inhalation, the water molecules get desorbed due to the ambient temperature, and hence the sensor current decreases.
3.3. Pressure sensing
Furthermore, the fabricated SnS2 QD/PVA film was utilized for pressure sensing. To investigate the pressure sensing properties, 1 V external voltage was applied to the 2 cm × 2 cm SnS2 QD/PVA sensor. Different pressures were applied to test the pressure sensor and the temporal response was measured. The digital photo shows that the human finger touches the surface of the fabricated pressure sensor as shown in Fig. 7a demonstrating the real time application. Fig. 7b illustrates the measured I–V characteristics of the fabricated pressure sensor under increasing pressure and as observed as the pressure increases the device current increases. Fig. 7c shows the temporal response wherein constant pressure was applied and a change in the sensor current was monitored and it was observed that the sensor was able to repeatedly sense the pressure applied. A similar measurement was performed for increasing pressure and as can be seen from Fig. 7d, as the pressure increases the sensor current increases which is consistent with the I–V characteristics. The important parameter of the pressure sensor is sensitivity which is defined as S = (ΔR/R0)/ΔP, where R0 is the initial resistance when no pressure is applied, ΔP is the change in the pressure applied, and ΔR is the change in the resistance due to the applied pressure. Fig. 7e shows the graph plotted between normalized resistance and pressure applied, the sensitivity of the fabricated device was found to be ∼10.14 kPa−1. To verify the reliability of the fabricated pressure sensor, the device was subjected to 1000 cycles and as can be seen from Fig. 7f, a negligible change in the device performance was observed suggesting the high robustness of the fabricated device. Also, similar to a strain and breath sensor, the rise time and fall time of the pressure sensor were calculated to be ∼987 ms and ∼523 ms respectively as shown in Fig. 7g and h.
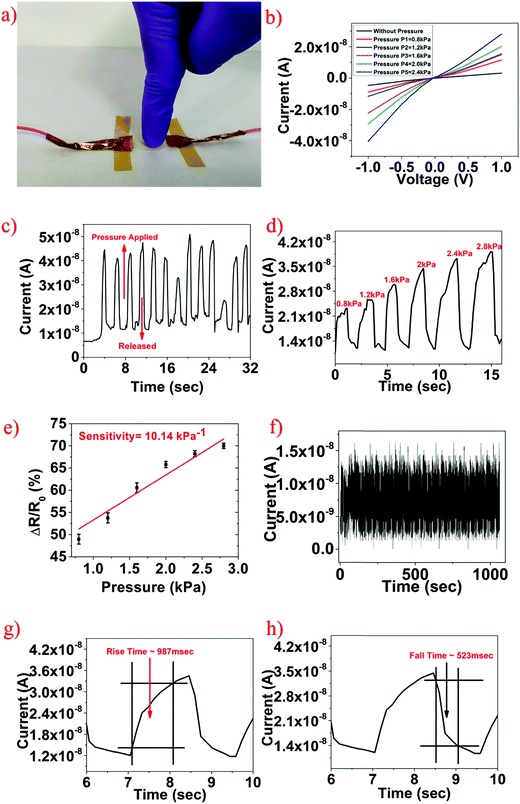 |
| Fig. 7 (a) The digital photo of a finger touching the pressure sensor. (b) I–V characteristics of a SnS2 QD/PVA based pressure sensor with different applied pressures. (c) Temporal response of the fabricated sensor under constant pressure. (d) Temporal response of the fabricated sensor under different applied pressures. (e) Sensitivity graph showing a sensitivity value of ∼10.14 kPa−1. (f) The response of the fabricated sensor for 1000 cycles. (g and h) The rise time and fall time of the fabricated sensor were ∼987 ms and ∼523 ms. | |
The change in the device current under external pressure can be understood by the following phenomenon similar to the strain sensing. The current flow in the SnS2 QD/PVA film is realized through continuous conductive pathways formed by aggregated QDs. When the external pressure is applied on the device, quantum dots come closer which increases the conductive pathways thereby increasing the sensor current. Secondly, quantum dots are separated by very few nanometers (∼1–2 nm) as seen in the TEM image (Fig. 2a and b), which can be considered as tunneling distance. When the external pressure is applied on the sensor, it reduces the distance between QDs which promotes the tunneling process and increases the overall sensor current. The fabricated pressure sensor has a negligible hysteresis as seen in Fig. S2 (ESI†) which confirms that there are no cracks or ruptures in the device under external pressure and this can further be attributed to the excellent mechanical and flexible properties of the fabricated SnS2 QD/PVA film.
The hydrolysis of the fabricated SnS2 QD/PVA sensor is shown in Fig. 8a–e where the images are taken in a sequence displaying the dissolution of the fabricated sensor in DI water. The PVA is a water-soluble substrate, and is made up of vinyl acetate monomer; during the dissolution process, vinyl acetate is replaced by a reactive hydroxyl group. The PVA substrate with SnS2 QDs dissolved completely in ∼360 s, which shows that the fabricated sensor has good transient behaviour. Furthermore, it should be noted that the time taken for dissolution can be adjusted by changing the thickness and physical dimensions of the device. Also, only the active material (SnS2 QDs) and the substrate (PVA) are water soluble and the metal contacts are not water soluble thereby making the technology as destructible electronics instead of transient electronics.
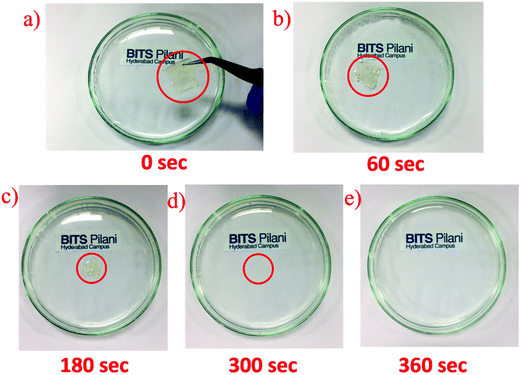 |
| Fig. 8 Images of different stages of dissolution of SnS2 QDs with the PVA substrate in DI water: (a) 0 s, (b) 60 s, (c) 180 s, (d) 300 s and (e) 360 s. | |
3.4. Machine learning
Since all the three stimuli discussed have the transduction mechanism to be resistive, it becomes very difficult to identify the stimulus due to which the sensor resistance varied. Machine learning is commonly used to tackle such critical issues concerning the identification of variation in the resistance due to any one of the stimuli. As identification of stimuli requires manual intervention, machine learning algorithms are capable of learning to predict the data themselves. At first, they learn data from the prior information of the stimuli and then categorize a supplied dataset for validation. In this work, four machine learning algorithms such as k-nearest neighbor (KNN), decision tree (DT), random forest (RF), and extra tree (ET) classifiers are used for classifying multi-class sensor output data for a reliable frontend processing. The data collected from the multifunction sensor for pressure, breath, and strain were used to train and test the four ML classifiers. The machine learning classifier model was trained on the collected data sets from the different stimuli and validated against a labelled test data set. The accuracy of the model of machine learning algorithms depends on the values chosen for the hyperparameter. The hyperparameters are used to look at different tuning parameters to find a configuration that provides the best output. The grid search method is used to change different hyperparameters to boost the accuracy of algorithms in machine learning (ML) over an iterative process. Here, both binary and multi-classification was done on data by using the Scikit learning python platform.45 The data acquired from multifunctional sensors were categorized into three sets: strain & breath, pressure & breath, and pressure & strain. The experimental research showed that current and resistance with respect to time are two key features of sensory data that are used to train and test various classifiers. The SMOTE technique46 was utilized as a pre-processing tool for handling any imbalance datasets and subsequently was divided into two parts; trains (80%) and tests (20%). The classifier model's performance was assessed using a blend of tuning parameters with the grid search method to improve the classification accuracy, and the same is tabulated in Table 1. Extra tree gives a testing accuracy of 84.7% for the breath & pressure dataset, 84.9% for the breath & strain dataset, and 87.8% for the pressure & strain dataset. The model accuracy evaluation is performed by cross-validation. Here, two cross-validation methods, k-fold and repeated k-fold stratified with tuning parameters, were used to validate classifier performance at k = 10 as shown in Fig. 9a–c, respectively. It is found that the extra tree bi-classifier with repeated stratified k-fold could identify breath & pressure, breath & strain, and pressure & strain with 84.7%, 85.1%, and 87.7% classification accuracy, respectively. Multi-classifiers use a one-vs.-rest strategy involving training a single classifier per class, with the samples of that class as positive samples and all other samples as negatives. The extra tree gives a testing accuracy of 77.5%, as reported in Table 1, whereas Fig. 9d shows 78.4% validation accuracy on the multifunction sensory dataset. Therefore, the extra tree with and without the cross-validation method was best suited for multifunctional sensory data sets.
Table 1 Testing the accuracy of binary and multi-classification
S. no. |
Algorithm |
Breath and pressure |
Breath and strain |
Pressure and strain |
Multi-class |
1 |
k-Nearest neighbors |
82.3% |
82.3% |
85.8% |
74.2% |
2 |
Decision tree algorithm |
83.8% |
80.1% |
86% |
74.1% |
3 |
Random forests |
84.5% |
82.9% |
87.7% |
76.2% |
4 |
Extra trees |
84.7% |
84.9% |
87.8% |
77.5% |
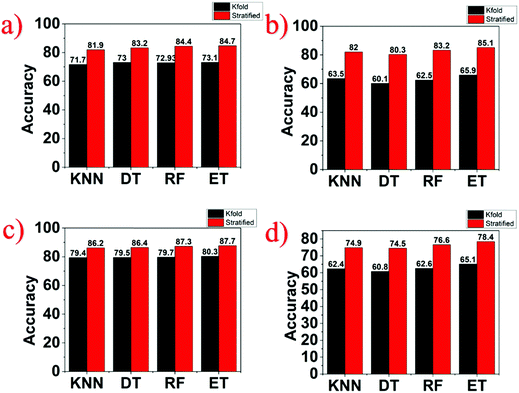 |
| Fig. 9
k-Fold and repeated stratified k-fold validation methods used for the performance evaluation of both binary and multi-class classifications: (a) breath and pressure, (b) breath and strain, (c) pressure and strain and (d) breath, pressure, and strain respectively. | |
Several works have reported sensing different physical and chemical stimuli on the multifunctional sensor. Yang et al. developed sensors using network-MXene/polyurethane (M/P mat) to detect subtle physiological signals (e.g. respiration and pulse waves) and a wide variety of body behaviours.47 Zhao et al. demonstrated a variety of possible applications such as walking gaits, finger-tapping, finger flexure, and human swallowing with the aid of nanofiber film made from carbonised electrospun polyacrylonitrile/barium titanate (PAN-C/BTO).48 Zhai et al. proposed flexible conductive carbon black (CB)/thermoplastic polyurethane (TPU) foam used as a wearable sensor to monitor different physiological signals from human motion which deals with water pollution through the separation of oil and water.49 Xu et al. used ecoflex rubber, 3D graphene foam (3D-GrF), and modified silicon rubber (MSR) to fabricate a strain sensor with a long cycle life of more than 10
000 cycles for pressure and strain sensing.50 Hua et al. presented a conformable matrix network (SCMN) that successfully expands the e-skin sensing functionality including in-plane strain, magnetic field, pressure, humidity, proximity, and temperature.51 Shin et al. fabricated a PEDOT:PSS functionalized AgNW electrode integrated into the polymer layer acting as a flexible multifunctional sensor to monitor motion and temperature change.52 Although various multifunctional sensors exhibit excellent mechanical and electrical properties, the abovementioned state-of-the-art multifunctional sensor does not have a proper frontend processing for stimulus identification. Also, most of the reported sensors are fabricated using materials that cause a lot of E-waste (Table 2). Furthermore, the authors would like to mention that the term “multifunctional” in this work refers to applying the stimulus independently and not simultaneously. Applying simultaneous stimulus requires a much sophisticated frontend processing of the data which is beyond the scope of the current work. In this work, the authors report the development of water soluble film SnS2 QD/PVA used for a multifunctional sensor with machine learning to identify different stimuli even though the transduction mechanism is resistive in all cases which utilizes important recent technological advances.
Table 2 Comparison of various multifunctional sensors
S. no. |
Materials & substrate |
Application |
Transient |
Stimuli identification |
Ref. |
1 |
Network-M/P mat |
Tensile strain/vertical pressure/bending and subtle vibrations |
No |
No |
47
|
2 |
PAN-C/BTO |
Pressure and curvature |
No |
No |
48
|
3 |
CB/TPU |
Strain/pressure |
No |
No |
49
|
4 |
3D-GrF/MSR |
Strain/pressure |
No |
No |
50
|
5 |
Pt, Ni/Cu, ZnO, Co/Cu, Ag/PDMS |
Humidity/UV light/magnetic field/strain/pressure/proximity |
No |
No |
51
|
6 |
ZnO/PDMS |
Motion/temperature |
No |
No |
52
|
7
|
SnS
2
QDs/PVA
|
Breath/pressure/strain |
Yes
|
Yes
|
This Work
|
4. Conclusions
In conclusion, this report demonstrates, for the first time, a transient, cost-effective, and flexible multifunctional sensor (strain, pressure, and breath) based on a water soluble SnS2 QD/PVA film and the accurate classification of the stimuli using machine learning algorithms. The fabricated SnS2 QD/PVA film with Cu contacts was utilized for real-time human motion monitoring application and the GF of the device was calculated to be 3.08. Also, the fabricated device was tested to track various breath patterns and it is capable of identifying the breathing frequency of an individual. Furthermore, the pressure sensing ability of the fabricated device was tested and the device exhibits a sensitivity of 10.14 kPa−1. The machine learning algorithm has been used to recognize patterns and differentiate sensor-generated signals upon exposure to physical and chemical stimuli with the highest accuracy of 87.7%. Moreover, the fabricated device displays complete water solubility within ∼360 s, which is a proof of evidence for its phenomenal applications in transient electronics. The successful demonstration of a multifunctional sensor based on SnS2 QD/PVA enables new opportunities in flexible and eco-friendly transient electronics for medical applications.
Conflicts of interest
The authors declare no conflict of interest.
Acknowledgements
P. S. acknowledges the Research Initiation Grant (RIG and ACRG) Birla Institute of Technology and Science Pilani, Hyderabad Campus. The authors thank Central Analytical Laboratory, BITS Pilani Hyderabad Campus, for the assistance in material characterization. A portion thereof was performed using facilities at CeNSE, Indian Institute of Science, Bengaluru, funded by the Ministry of Human resource development (MHRD), Ministry of electronics and information technology (MeitY), and Nanomission, Department of Science and Technology (DST), Govt. of India.
References
- S. W. Hwang, C. H. Lee, H. Cheng, J. W. Jeong, S. K. Kang, J. H. Kim, J. Shin, J. Yang, Z. Liu, G. A. Ameer and Y. Huang, Nano Lett., 2015, 15, 2801–2808 CrossRef CAS.
- S. W. Hwang, J. K. Song, X. Huang, H. Cheng, S. K. Kang, B. H. Kim, J. H. Kim, S. Yu, Y. Huang and J. A. Rogers, Adv. Mater., 2014, 26, 3905–3911 CrossRef CAS.
- L. Teng, S. Ye, S. Handschuh-Wang, X. Zhou, T. Gan and X. Zhou, Adv. Funct. Mater., 2019, 29, 1808739 CrossRef.
- S. W. Hwang, J. K. Song, X. Huang, H. Cheng, S. K. Kang, B. H. Kim, J. H. Kim, S. Yu, Y. Huang and J. A. Rogers, Adv. Mater., 2014, 26, 3905–3911 CrossRef CAS.
- S. K. Ganeshan, V. Selamneni and P. Sahatiya, New J. Chem., 2020, 44, 11941–11948 RSC.
- K. Liu, H. Tran, V. R. Feig and Z. Bao, MRS Bull., 2020, 45, 96–102 CrossRef.
- P. Sahatiya, A. Shinde, A. Kadu and S. Badhulika, Mater. Sci. Semicond. Process., 2019, 93324–93330 Search PubMed.
- V. Selamneni, P. Barya, N. Deshpande and P. Sahatiya, IEEE Sens. J., 2019, 19, 11255–11261 CAS.
- L. Wang, Z. Lou, K. Jiang and G. Shen, Adv. Intell. Syst., 2019, 1, 1900040 CrossRef.
- S. Veeralingam, P. Sahatiya, A. Kadu, V. Mattela and S. Badhulika, ACS Appl. Electron. Mater., 2019, 1, 558–568 CrossRef CAS.
- P. Sahatiya, A. Kadu, H. Gupta, P. T. Gomathi and S. Badhulika, ACS Appl. Mater. Interfaces, 2018, 10, 9048–9059 CrossRef CAS.
- L. Wang, Z. Lou, K. Jiang and G. Shen, Adv. Intell. Syst., 2019, 1, 1900040 CrossRef.
- Z. Shen and Q. Wang, IEEE Trans. Instrum. Meas., 2012, 61, 3351–3362 Search PubMed.
- D. Zhang, J. Liu, C. Jiang, A. Liu and B. Xia, Sens. Actuators, B, 2017, 240, 55–65 CrossRef CAS.
- F. Miao, X. Wang, L. Yin and Y. Li, IEEE Sens. J., 2018, 19, 1426–1434 Search PubMed.
- K. Altun, B. Barshan and O. Tunçel, Pattern Recognit., 2010, 43, 3605–3620 CrossRef.
- N. Ahmed, J. I. Rafiq and M. R. Islam, Sensors, 2020, 20, 317 CrossRef.
- M. Kim, B. Kim, B. Park, M. Lee, Y. Won, C. Y. Kim and S. Lee, Sensors, 2018, 18, 3051 CrossRef.
- K. K. Fu, Z. Wang, J. Dai, M. Carter and L. Hu, Chem. Mater., 2016, 28, 3527–3539 CrossRef CAS.
- G. Giovannetti, P. A. Khomyakov, G. Brocks, P. J. Kelly and J. Van Den Brink, Phys. Rev. B: Condens. Matter Mater. Phys., 2007, 76, 073103 CrossRef.
- D. Zhang, X. Zong, Z. Wu and Y. Zhang, ACS Appl. Mater. Interfaces, 2018, 10, 32631–32639 CrossRef CAS.
- D. Zhang, Z. Xu, Z. Yang and X. Song, Nano Energy, 2020, 67, 104251 CrossRef CAS.
- D. Zhang, X. Zong and Z. Wu, Sens. Actuators, B, 2019, 287, 398–407 CrossRef CAS.
- S. H. Chaki, M. P. Deshpande, D. P. Trivedi, J. P. Tailor, M. D. Chaudhary and K. Mahato, Appl. Nanosci., 2013, 3, 189–195 CrossRef CAS.
- T. M. Maria, R. A. De Carvalho, P. J. Sobral, A. M. B. Habitante and J. Solorza-Feria, J. Food Eng., 2008, 87, 191–199 CrossRef CAS.
- N. Jain, V. K. Singh and S. Chauhan, J. Mech. Behav. Mater., 2017, 26, 213–222 CAS.
- N. D. Alexopoulos, C. Bartholome, P. Poulin and Z. Marioli-Riga, Compos. Sci. Technol., 2010, 70, 1733–1741 CrossRef CAS.
- Y. Zhu, W. Lu, Y. Guo, Y. Chen, Y. Wu and H. Lu, RSC Adv., 2018, 8, 36999–37007 RSC.
- B. Ram and A. K. Singh, Phys. Rev. B, 2017, 95, 075134 CrossRef.
- X. Huang, X. Tong and Z. Wang, J. Electron. Sci. Technol., 2020, 100018 CrossRef.
- Y. Li, L. Tang, R. Li, J. Xiang, K. S. Teng and S. P. Lau, Chin. Phys. B, 2019, 28, 037801 CrossRef CAS.
- X. Fu, P. Ilanchezhiyan, G. M. Kumar, H. D. Cho, L. Zhang, A. S. Chan, D. J. Lee, G. N. Panin and T. W. Kang, Nanoscale, 2017, 9, 1820–1826 RSC.
- T. Edvinsson, R. Soc. Open Sci., 2018, 5, 180387 CrossRef CAS.
- A. Bagga, P. K. Chattopadhyay and S. Ghosh, Int. Workshop Phys. Semicond. Devices, 2007, 876–879 Search PubMed.
- A. L. Efros, M. Rosen, M. Kuno, M. Nirmal, D. J. Norris and M. Bawendi, Phys. Rev. B: Condens. Matter Mater. Phys., 1996, 54, 4843 CrossRef CAS.
- Y. Li, L. Tang, R. Li, J. Xiang, K. S. Teng and S. P. Lau, Chin. Phys. B, 2019, 28, 037801037801 Search PubMed.
- Z. Qin, K. Xu, H. Yue, H. Wang, J. Zhang, C. Ouyang, C. Xie and D. Zeng, Sens. Actuators, B, 2018, 262, 771–779 CrossRef CAS.
- Y. Zhang, Y. Guo, Y. Wang, T. Peng, Y. Lu, R. Luo, Y. Wang, X. Liu, J. K. Kim and Y. Luo, Nanoscale Res. Lett., 2018, 13, 389 CrossRef.
- G. Ge, W. Huang, J. Shao and X. Dong, J. Semicond., 2018, 39, 011012 CrossRef.
- M. Lee, C. Y. Chen, S. Wang, S. N. Cha, Y. J. Park, J. M. Kim, L. J. Chou and Z. L. Wang, Adv. Mater., 2012, 24, 1759–1764 CrossRef CAS.
- L. Cai, L. Song, P. Luan, Q. Zhang, N. Zhang, Q. Gao, D. Zhao, X. Zhang, M. Tu, F. Yang and W. Zhou, Sci. Rep., 2013, 3, 1–9 Search PubMed.
- R. Matsuzaki and K. Tabayashi, Adv. Funct. Mater., 2015, 25, 3806–3813 CrossRef CAS.
- M. K. Shin, J. Oh, M. Lima, M. E. Kozlov, S. J. Kim and R. H. Baughman, Adv. Mater., 2010, 22, 2663–2667 CrossRef CAS.
- K. H. Kim, S. A. Jahan and E. Kabir, TrAC-Trend Anal. Chem., 2012, 33, 1–8 CrossRef CAS.
- G. Varoquaux, L. Buitinck, G. Louppe, O. Grisel, F. Pedregosa and A. Mueller, Mob. Comput. Commun. Rev., 2015, 19, 29–33 Search PubMed.
- G. Lemaître, F. Nogueira and C. K. Aridas, J. Mac. Learn. Res., 2017, 18, 559–563 Search PubMed.
- K. Yang, F. Yin, D. Xia, H. Peng, J. Yang and W. Yuan, Nanoscale, 2019, 11, 9949–9957 RSC.
- G. Zhao, X. Zhang, X. Cui, S. Wang, Z. Liu, L. Deng, A. Qi, X. Qiao, L. Li, C. Pan and Y. Zhang, ACS Appl. Mater. Interfaces, 2018, 10, 15855–15863 CrossRef CAS.
- Y. Zhai, Y. Yu, K. Zhou, Z. Yun, W. Huang, H. Liu, Q. Xia, K. Dai, G. Zheng, C. Liu and C. Shen, Chem. Eng. J., 2020, 382, 122985 CrossRef CAS.
- M. Xu, F. Li, Z. Zhang, T. Shen, Q. Zhang and J. Qi, Sci. China Mater., 2019, 62, 555–565 CrossRef CAS.
- Q. Hua, J. Sun, H. Liu, R. Bao, R. Yu, J. Zhai, C. Pan and Z. L. Wang, Nat. Commun., 2018, 9, 1–11 CrossRef CAS.
- S. H. Shin, D. H. Park, J. Y. Jung, M. H. Lee and J. Nah, ACS Appl. Mater. Interfaces, 2017, 9, 9233–9238 CrossRef CAS.
Footnote |
† Electronic supplementary information (ESI) available. See DOI: 10.1039/d0ma00573h |
|
This journal is © The Royal Society of Chemistry 2020 |