Two-stage hierarchical clustering for analysis and classification of mineral sunscreen and naturally occurring nanoparticles in river water using single-particle ICP-TOFMS†
Received
5th April 2024
, Accepted 5th July 2024
First published on 8th July 2024
Abstract
Titanium dioxide (TiO2) and zinc oxide (ZnO) engineered nanoparticles (NPs) are used in mineral-based sunscreens due to their excellent ultraviolet light protection abilities. Over time, surface water can become contaminated with these particles because of human recreational activities such as bathing, swimming, and other water sports. Thus, there is a need to measure these engineered particles present in surface waters to gain better understanding of anthropogenic inputs. In this study, we measure natural stream water spiked with mineral sunscreen along with naturally occurring NPs and microparticles (μPs) at the single-particle level using single-particle inductively coupled plasma time-of-flight mass spectrometry (spICP-TOFMS). We use two-stage hierarchical clustering analysis (HCA) to identify distinct multi-elemental compositions that are characteristic of sunscreen-derived particles. Specifically, sunscreen NPs can be isolated from naturally occurring NPs and μPs based on elevated Ti and Zn mass fractions in individual particles compared to natural particles that are rich in Fe, Al, Mn, Ti, Mg, Zn, Ce, La, and/or Pb. Based on clusters assigned by HCA, we demonstrate classification of sunscreen-derived Ti and Zn NPs across more than two orders of magnitude and at number concentrations up to 50 times lower than those of naturally occurring Ti- and Zn-containing particles. This study demonstrates the accurate class assignment of sunscreen released and naturally occurring particles in river water.
Environmental significance
The measurement of anthropogenic TiO2 and ZnO nanoparticles (NPs) from mineral sunscreens at environmentally relevant concentrations in real samples is a major analytical challenge and a limiting factor in assessing accuracy of nano-pollutant modelling and nano-toxicological effects of these particle types. We report a high-throughput single-particle inductively coupled plasma time-of-flight mass spectrometry (spICP-TOFMS) approach for the analysis of mineral sunscreen NPs in a background of naturally occurring NPs from river water. Our spICP-TOFMS and hierarchical clustering analysis (HCA) method offers a new strategy to separate and classify natural and mineral-sunscreen particles at number concentrations down to hundreds of particles per milliliter.
|
Introduction
Titanium dioxide (TiO2) and zinc oxide (ZnO) are among the most produced types of engineered nanoparticles due to their extensive use in personal care and consumer products. The current production of TiO2 particles is estimated to be 9.4 million tons and that of nano-ZnO is predicted to be up to 58
000 tons per year.1–3 The majority of produced TiO2 and ZnO particles are used as bright white pigment in plastics, paints, papers, and cosmetics.4,5 Due to their efficient photocatalytic activity, in recent years TiO2 and ZnO have also been extensively used in environmental and energy applications such as solar panels, water treatment, air purification, and self-cleaning surfaces.6–9 TiO2 and ZnO are often encountered by humans through consumables like food additives, drugs, clothing, and cosmetics.4,10–14 These engineered particles can ultimately find their way into environmental compartments such as water, soil, and air. Engineered particles present in personal care and consumer products often enter wastewater treatment plants (WWTPs) through household discharges and sewages.15 WWTPs can remove the majority of larger-sized NPs; however, these plants are less efficient for retention of NPs below 50 nm, which are then released into surface waters.16 Increased concentrations of Ti and Zn engineered particles in surface waters such as lakes and rivers are also due to bathing and other recreational activities especially during summer.17
Sunscreens are widely used as UV radiation filters and to enhance aesthetics. Both organic (oxybenzone, octinoxate, etc.) and mineral (TiO2 and ZnO) based filters provide protection; however, organic UV filters tend to exhibit potential adverse effects on human and environmental health.18 Thus, the US Food and Drug Administration (FDA) has called for additional safety evaluations of twelve commonly used organic filters.19 As an alternative, mineral UV filters are increasingly used in sunscreens. TiO2 NPs are more effective in the UVB range (290–320 nm) and ZnO NPs in UVA range (320–400 nm); the combination of these particles assures broadband UV protection.13 The European Commission's Scientific Committee on Consumer Safety (SCCS) also recommends usage of TiO2 and ZnO NPs in cosmetic products up to 25% for UV protection.20 There have been studies that show that these metal oxides act as UV filters with limited penetration into the skin and do not produce sensitization to skin. However, at very small sizes these particles can penetrate the skin and other tissues, and when exposed to UV radiation they may generate reactive oxygen species (ROS) and may induce cytotoxicity and genotoxicity.13,21–23 The International Agency for Research on Cancer (IARC) has listed TiO2 as a Group 2B carcinogen.24 To limit the generation of ROS the less photocatalytic rutile TiO2 is preferred to highly photocatalytic anatase.25 Additionally, to limit the photocatalytic activity, particles can be coated by an inert mineral layer of Al2O3 or SiO2. These coatings also provide hydrophobic or hydrophilic properties that manufacturers use to produce the desired texture and water resistance.26,27 The safety of cosmetic products containing TiO2 and ZnO NPs, particularly in sunscreens, has been a frequent topic of discussion.28,29
Identification and quantification of engineered NPs and μPs in complex environmental systems is a challenge due to low concentrations of engineered particles compared to high background concentrations of natural particles.30 The use of electron microscopy (EM) methods, such as scanning electron microscopy (SEM) and transmission electron microscopy (TEM), allows for the determination of the morphology, crystal structure and size of individual particles at the nanometer scale.17,31,32 When paired with energy dispersive X-ray spectroscopy (EDX), they also offer insight into the elemental composition. Although electron or X-ray-based microscopy techniques can be used to differentiate naturally occurring from anthropogenic particles,33–35 it is challenging to detect low particle number concentrations (PNCs) due to limited throughput, particularly in situations with high particle backgrounds.17,31 To overcome throughput limitations, several researchers have employed single-particle inductively coupled plasma mass spectrometry (spICP-MS) to measure Ti or Zn NP signatures in environmental samples.36–40 However, the inability to quantitatively measure multiple elements in individual particles with spICP-MS with quadrupole or sector-field mass analyzers limits the applicability of the approach to confidently classify anthropogenic Ti- of Zn-bearing particle types.41
In recent years, spICP-TOFMS has become a method of choice for single-particle analysis.42 It offers quasi-simultaneous analysis of ions across almost the entire atomic mass range—from 6Li to 238U—with sufficient time resolution to detect particle-produced transient events.43–45 Single-particle ICP-TOFMS analysis requires minimal sample preparation, is high throughput, and provides simultaneous determination of elemental composition, element mass amounts in particles, and PNCs. It also allows for quantitative untargeted multi-element measurements from individual particles, which can be used to differentiate natural from anthropogenic particles.15,42,46–49 With spICP-TOFMS, the critical mass (i.e. the amount of an element needed to be detected in an individual particle) for most elements is in the tens to hundreds of attograms.50 However, there are some naturally abundant elements, including carbon, nitrogen, oxygen, fluorine, and sulfur, that are not readily detectable at the single-particle level due to low sensitivities and/or high natural backgrounds. Several researchers have explored the specific detection of Ti- and Zn-containing anthropogenic and natural nanoparticles and their multi-element associations with spICP-TOFMS.42,49,51–53 In particular, associations of Ti with Al, V, Mn, Y, and Nb have been discussed as routes to classify the presence of anthropogenic particles.42,51
In spICP-TOFMS, a large amount of data is generated because thousands of particles can be measured per minute. To extract information from such large data files, automated and robust data processing and investigation strategies are required. In the past, researchers have used various machine learning (ML) methods for distinguishing between anthropogenic and natural Ce-containing NPs,48,54 linear classification models for identifying engineered Ti-particles in soil,55,56 and clustering analysis as a data reduction and analysis technique.57 Hierarchical clustering analysis (HCA) is a technique for separating data in groups based on measure of similarity between the data points. HCA analysis was first used in combination with spICP-TOFMS for the analysis of NPs measured from wastewater treatment plants.57 Since then, several research groups have adopted the approach and applied it for the analysis of NPs recorded by spICP-TOFMS from soil samples,58 urban rain and run-off,59,60 mineral dust aerosols,61 and artificial mixtures of engineered NPs (Au, Ag, FeCoNi and FeCoZn) and natural clay nanominerals (kaolinite and montmorillonite).62 In this study, we explore the ability to use spICP-TOFMS analysis to characterize and classify sunscreen-derived TiO2 and ZnO NPs against NPs and μPs found in a natural river sample by means of two-stage unsupervised HCA. Our study is the first to explore the use of HCA to classify and then quantify varying concentrations of anthropogenic particle types from a complex particle matrix.
Materials and methods
Sample preparation for spICP-TOFMS measurements
River water was collected from Clear Creek (Golden, Colorado) in a 500 mL high density polyethylene (HDPE) bottle. Analysis of mineral sunscreen particles and organic sunscreen agents in Clear Creek has been previously reported,41,63 with no conclusive measurements regarding the detection of mineral sunscreen particles. The water used here was collected on September 6, 2022 at a remote location upstream of Golden, and so likely has little-to-no anthropogenic input, and thus can be considered a pristine natural sample with regard to mineral sunscreen (SS) particulates. The water sample was processed with a previously established method.49 First, the sample was shaken vigorously for 30 seconds, and 1.5 mL of water was transferred into a 2 mL microcentrifuge tube. The sample was then ultrasonicated (VialTweeter, Hielscher UP200st, Germany) for 60 seconds (10 seconds on and 5 seconds off) at 100 W. Ultrasonication has been shown to be an effective sample preparation method for the analysis of mineral sunscreen-derived NPs.64 After ultrasonication, the samples were centrifuged for two minutes at 4000 rpm using a mini-centrifuge (Costar, USA). The estimated size cutoff from this centrifugation was 400 nm based on Stoke's law and a particle density of 4.2 g cm−3. After centrifugation, 900 μL of supernatant was transferred to a 2 mL microcentrifuge tube and the river water sample was further diluted in ultrapure water containing 5 ng mL−1 dissolved Cs. spICP-TOFMS analysis was used to determine the background particle content of river water.
Commercially available sunscreen (Cetaphil: Sheer Mineral Sunscreen Broad Spectrum SPF 50, United States) that, according to the label, contains 9% TiO2 and 7% ZnO as active ingredients was purchased from a local store and used as a source of Ti and Zn sunscreen particles. To prepare the sunscreen suspension, 0.2 g of sunscreen was placed in a 50 mL centrifuge tube, diluted with 20 mL of ultrapure water, and vigorously shaken for 30 seconds to disperse the sunscreen. The dispersed sample was then water-bath sonicated (VWR Ultrasonic Cleaner, VWR, PA, USA) for 30 minutes, vortexed for 30 seconds, and allowed to settle for 10 minutes. After settling, 1 mL of supernatant suspension was diluted in ultrapure water containing 5 ng mL−1 dissolved Cs and spICP-TOFMS analysis was used to determine PNCs from this suspension. Based on this initial spICP-TOFMS analysis, a stock sunscreen particle suspension containing ∼1 × 106 particles per mL was prepared and subsequently used to spike sunscreen particles into the river water sample at varying concentrations.
Mineral specimens of rutile, ilmenite, and biotite were obtained from the Colorado School of Mines Earth Science Museum. The mineral samples were processed with a previously established method.49,65 Ti-minerals were hand ground in a porcelain mortar and pestle, and neat suspensions were prepared by dispersing ∼5 mg of the ground minerals into 15 mL of ultrapure water (18.2 MΩ cm PURELAB flex, Elga LabWater, UK). Each of these samples were then water-bath sonicated (VWR Ultrasonic Cleaner, VWR, PA, USA) for 10 minutes, vortexed for 30 seconds, and allowed to settle for 10 minutes. A supernatant suspension of each sample was diluted in ultrapure water containing 5 ng mL−1 dissolved Cs and spICP-TOFMS analysis was used to estimate PNCs. Separate stock suspensions of each Ti-mineral containing ∼1 × 105 particles per mL were prepared.
River water samples containing 5 ng mL−1 dissolved Cs were spiked with a sunscreen suspension at varying number concentrations; the dilution scheme is provided in Table S1.† In another set of experiments, river water was first spiked with Ti-mineral particles, and then spiked with a sunscreen suspension at varying number concentrations. The dilution scheme for this experiment is provided in Table S2.†
Preparation of the microdroplet calibration solution
Online microdroplet experiments were performed using a multi-element calibration solution that was prepared using eighteen single-element standards (High-Purity Standards, SC, USA): Mg, Al, Ti, V, Mn, Fe, Co, Zn, Y, Zr, Nb, Cs, La, Ce, Nd, Ta, W and Pb. Concentrations of these elements in the microdroplet calibration solution are provided in Table S3.† Cesium (Cs) was used as the plasma uptake standard for online microdroplet calibration. All dilutions were made in 2% sub-boiled trace metal grade nitric acid (Fisher Scientific, Fair Lawn, NJ, USA) gravimetrically (ML204T/A00, Mettler-Toledo, Greifensee, Switzerland).
spICP-TOFMS measurements and data processing
An icpTOF-S2 instrument (TOFWERK AG, Thun, Switzerland) was used for all measurements. The operating parameters of the instrument are given in Table S4.† Online microdroplet calibration, which is described in detail elsewhere,46,66 was used to determine element masses per particle and PNCs.
TofDAQ Recorder (TOFWERK AG, Thun, Switzerland) was used for data collection and TOF Single-Particle Investigator (TOF-SPI) was used for data processing.67 TOF-SPI is an in-house developed LabVIEW program (LabVIEW 2018, National Instruments Corp., TX, USA) for batch analysis of online microdroplet calibration spICP-TOFMS data. The program is used to calculate element-specific background signals and single-particle critical values, determine absolute sensitivities, background subtract data, correct split-particle events,68 quantify element masses in single particles, and determine PNCs. With TOF-SPI, single-particle critical values (LC,sp) are determined for each element based on the known compound Poisson distribution of icpTOF data.69,70 These LC,sp values are used as thresholds to separate particle-derived signals from steady-state background signals. Critical values can also be expressed in terms of mass, i.e. “critical mass” Xmassc,sp, by dividing the LC,sp of a given element by the absolute sensitivity for that element.
Hierarchical clustering
Two-stage hierarchical clustering analysis (HCA) was performed using the hierarchical clustering library in MATLAB (verR2022 MathWorks, MA, USA). The script used in this work was adapted from the work of Mehrabi et al.57 HCA is an unsupervised data analysis approach that can be used to cluster/group particles of similar elemental composition and to identify their mean elemental composition.
In our two-stage HCA approach, first, intra-sample HCA is performed on mass-quantified spICP-TOFMS data using the correlation distance and a distance cutoff of 0.6 to separate the major clusters. For all found clusters composed of 5 or more particle events, the average mass of each element in the particles in the cluster is determined and then multiplied by the number of times the element is recorded among all particles in the cluster (i.e., the occurrence frequency). For all elements with occurrence frequency >0.2, their occurrence normalized average masses are compiled to create a representative particle-class proxy (PCP) for each cluster. All PCPs found from the initial intra-sample clusters are then used as data for the second, inter-sample, HCA analysis. In this second HCA, the correlation distance is again used and the distance cutoff for determining the major inter-sample clusters is reduced to 0.3. For both the first and second stage of HCA, we determined the cluster cutoff distance empirically through inspecting the resultant dendrograms from each clustering. The MATLAB script and data used to generate the two-dimensional cluster maps are available on GitHub: https://github.com/orgs/TOFMS-GG-Group in the “Two-Stage-HCA-sunscreen” repository. For all intra-sample clusters, the occurrence-normalized average mass of elements within each cluster and the PNCs are reported along with the dendrogram from the second inter-sample clustering. All particle-resolved spICP-TOFMS data are available from any cluster or sample. In this way, two-stage HCA can be used as a tool to explore similarities of particle composition within and between samples, and then as a means to sort data for deeper exploration of single-particle results. A graphical illustration of the two-stage HCA approach is provided in the ESI of Mehrabi et al.57
Results and discussion
Characterization of particles from sunscreen and river water
To obtain baseline particle numbers and elemental compositions from both the sunscreen (SS) and river water (RW), we first analyzed neat suspensions of both via spICP-TOFMS. According to the manufacturer label, the sunscreen (SS) product we analyzed consists of 9% TiO2 and 7% ZnO particles. The critical masses (Xmassc,sp,i) for all analyte elements, i, in these analyses are provided in Table S5.† For the SS sample, Xmassc,sp,Ti = 42 ag and Xmassc,sp,Zn = 319 ag. For the RW sample, Xmassc,sp,Ti = 212 ag and Xmassc,sp,Zn = 731 ag. Based on these critical masses and assuming spherical particle geometry and bulk densities, the critical diameters for TiO2 and ZnO are 32 and 54 nm, and 51 and 63 nm, for the SS and RW samples, respectively. Different critical masses are the result of different tuning parameters and background signal levels. In Fig. 1a, we provide boxplots of the mass distributions of Ti detected as single-metal (sm) particles and multi-metal (mm) particles with various secondary and tertiary elements from both the SS and the RW samples. Similar plots of Zn mass and Al mass are provided in Fig. S2.† Since spICP-TOFMS analysis is more sensitive for Ti than Zn, it is unsurprising that more Ti-containing than Zn-containing particles are measured from the SS and RW samples. With spICP-TOFMS, 80% of measured SS particles contain Ti, whereas only 20% of particles contain measurable amounts of Zn. As seen in Fig. 1a, as the number of elements measured in SS particles increases, so does the Ti mass. Two percent of the SS particles measured by spICP-TOFMS contain detectable amounts of Al. The higher Ti mass in AlTi and AlTiZn particles compared to sm-Ti particles suggests that Al is only detectable in larger particles, which supports the hypothesis that Al2O3 is used as a coating for these particles.26,71 SEM-EDS measurements (see Fig. S1†) also show the presence of Al, Ti and Zn in sunscreen particles.
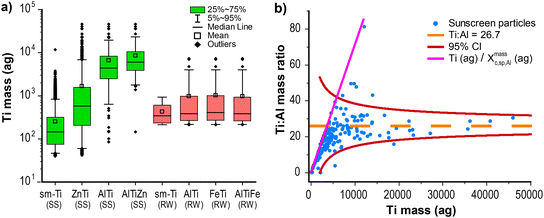 |
| Fig. 1 a) Boxplot showing mass distributions of titanium in titanium-containing particles in sunscreen and river water. b) Mass ratio of Ti : Al plotted against the Ti mass in each multi-element particle event. These ratios follow an estimated Poisson–Normal error. The red confidence interval (CI) shows 95% CI (±1.96σ), orange dashed line represents the Ti : Al mass ratio converging to 26.7 and the magenta line shows the critical mass limit, which is the measured mass of Ti divided by XmassC,sp,Al. | |
In Fig. 1b, we plot the ratio of Ti and Al in SS particles as a function of Ti mass. As seen, Ti and Al are well correlated with a mass ratio that converges to ∼27
:
1 Ti
:
Al, and the scatter in the measured element mass ratio matches predicted confidence bands based on Poisson statistics. Though Ti and Al are correlated in the SS particles, Al is only consistently detectable in large Ti particles (≳5000 ag Ti, 150 nm diameter TiO2), and so Al is not well suited as a fingerprint element to identify TiO2 NPs from SS. On the other hand, nearly half of all Zn particles from the SS are measured as ZnTi particles. There is poor mass correlation between Ti and Zn in these particles. It is likely that TiO2 and ZnO particles in the sunscreen form nanocomposite structures that are then recorded by spICP-TOFMS. To verify that the TiZn mmNP signals were real—and not the result of coincident particle detection or loose particle agglomerations—the SS samples were analyzed with various ultrasonic pre-treatment steps and at several dilutions. We observed no difference in the number of TiZn mmNPs across these measurements; results are provided in Fig. S3.†
Unlike the SS sample, in which particles are composed mostly of Ti, Zn, and Al (as measured by spICP-TOFMS), the RW sample has a much more diverse particle population. River water contains a blend of naturally occurring minerals and other colloids. In Fig. 2, we provide the PNCs of several elements detected as both single-metal (sm) and multi-metal (mm) particle types from the neat RW sample. The critical masses of all elements for this analysis are provided in Table S5.† As seen in Fig. 2, Fe is the most commonly measured element in single particles, with 68% of all particles containing Fe. Al is the next most common element with 21% of all particles containing Al. The high abundance of Fe and Al particles is expected because the crustal abundances of these elements are much higher compared to other metals.72 Manganese is the third most often detected element in RW particles, with 7% of particles having detectable amounts of Mn. Apart from the major elements (Fe, Al, and Mn), Ti, Ce, and Pb are detected in ∼1% of the particles from the RW sample. As described earlier, quantification of SS particles against a natural particle background relies on the measurement of the Ti- and Zn-containing particles. In the RW, 10–15% of Ti particles are detected as single-metal and 85–90% are detected as multi-metal particles, with the most common associated elements being Fe and Al. Zn-containing particles are infrequently detected (in ∼0.15% of all particles) in the RW sample, and most of the Zn-containing particles (>95%) are multi-metal, with most common associations with Fe and Al. Other elements detected in particles from the RW sample along with their percent abundances are Mg (0.40%), V (0.03%), Co (0.11%), Zr (0.06%), Nb (0.03%), La (0.20%) and Nd (0.02%).
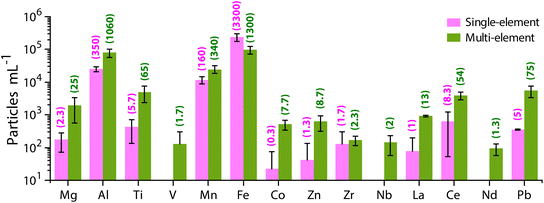 |
| Fig. 2 Multi-metal and single-metal particle number concentrations (PNCs) for each element from the river water sample diluted 50×. Average PNCs and standard deviations are based on triplicate measurements. The average number of particles recorded per spICP-TOFMS measurement is provided in parentheses. | |
Hierarchical clustering analysis of sunscreen spiked into river water
There are clear differences between particles measured from the SS and RW samples. The SS sample consists mostly of particles detected with elemental signatures of Ti, Zn, TiZn, and TiZnAl. On the other hand, the most commonly detected element signatures in RW particles are Fe, Al, Mn, FeAl, FeMn, and FeAlMn. Ti is detected infrequently in particles from the RW sample; however, as seen in Fig. 1, the mass of Ti is in the same range as that measured in the SS sample, and so separation of SS-derived and RW-derived Ti-containing particles in a mixture can be best achieved via multi-element signatures. Likewise, the multi-element signature of Zn-containing RW particles differs from that found in the SS particles. To explore the multi-elemental signature differences and ability of spICP-TOFMS to separate anthropogenic SS particles from naturally occurring RW particles, we analyzed spICP-TOFMS data from neat suspensions and mixtures of SS and RW via unsupervised two-stage hierarchical clustering analysis (HCA).
Results from two-stage analysis HCA are presented in Fig. 3. As seen, following the second stage (inter-sample) HCA, the clusters from the first-stage (intra-sample) HCA are grouped into nine major clusters, numbered 1–9. At the left of the heatmap in Fig. 3, we provide the names of each found intra-sample cluster (1st HCA stage), which we refer to as a “particle-class proxy” (PCP). Each PCP is identified according to the sample (i.e., SS, RW, S1–S6) and the elements that occur most often in the given cluster. The element symbols in the names of each PCP are those elements detected at 20% or greater occurrence frequency and ordered in terms of frequency with a maximum of three elements per name. The name of a PCP does not indicate all the elements present in that cluster; all elements are used in the creation of the PCP clusters. In Fig. 3, the occurrence-normalized mean elemental masses of each PCP are plotted on a false-color scale and the number concentration of each of these clusters is reported on the bar graph at the right. While the apparent elemental composition of each PCP is dominated by the primary element(s) in that PCP, less frequently occurring elements in a given particle class are accessible from the spICP-TOFMS data. In fact, a major benefit of two-stage HCA is the reduction of spICP-TOFMS data based on major element signatures and the subsequent investigation of minor or trace elements within found major clusters. For clarity, the arrangements of PCPs in clusters 4 and 5 (Zn-rich and Ti-rich clusters) are sorted according to PNCs of the PCPs rather than optimum dendrogram linkage distances.
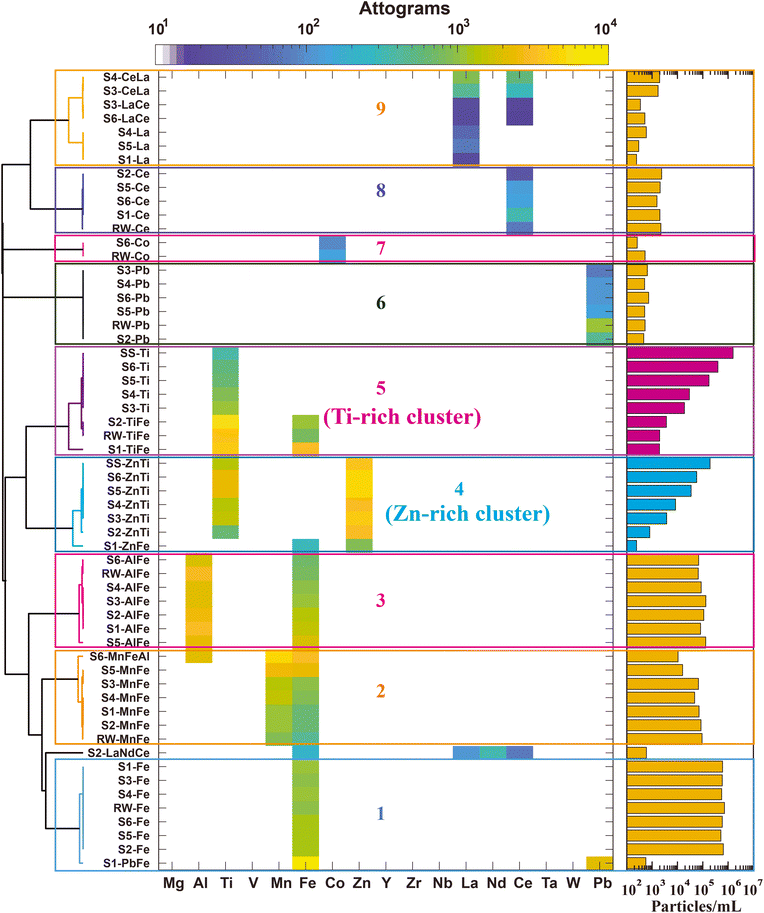 |
| Fig. 3 Dendrogram resulting from the two-stage unsupervised hierarchical clustering analysis (HCA). The heat map indicates the occurrence-normalized mean mass of each element in the found particle-class proxy (PCP) from the 1st-stage (intra-sample) clustering and the PNCs (particles per mL) of each PCP are provided as bars on the right. | |
As seen in Fig. 3, two-stage HCA reveals nine distinct major clusters across the eight samples (i.e., SS, RW, S1–S6). Samples S1–S6 are mixtures of SS and RW with increasing concentrations of SS particles. Importantly, the major clusters found from two-stage HCA allow the separation of SS-derived and RW-derived particle types. Specifically, clusters 1, 2, 3, 6, 7, and 8 have mixed samples (i.e., S1–S6) grouped with data from the neat RW sample and are thus attributable solely to natural RW particles. The consistent PNCs recorded for these natural PCPs (yellow bars in Fig. 3) across all the mixed samples and the RW sample also support the natural origin of these particle types because the RW sample was prepared at the same concentration for all samples. Cluster 9, which is rich in Ce and La, can also be considered a natural RW cluster, since no SS-originating PCPs are found in this cluster and the number concentration is consistent across all mixed samples. The natural RW clusters are rich in Fe, Al, and Mn, with lower mass amounts and number concentrations of Ce, La, Co, and Pb. While Fe, Al, Mn, Ce, and La are expected in natural RW samples, we did not anticipate detection of the Pb-rich and Co-rich PCPs. These PCPs are low-abundance, with PNCs of less than 1000 mL−1, and identification of these low-abundance unique particle types demonstrates utility of the unsupervised two-stage HCA approach.
The PCPs from the neat SS sample are found in two major clusters of the two-stage HCA: clusters 4 and 5, which we name the “Zn-rich” and “Ti-rich” clusters, respectively. As seen from the increase in PNCs from S1–S6, both clusters contain SS particles. In the Ti-rich cluster, there is also a RW-originating PCP (i.e., “RW-TiFe”), which indicates that some fraction of particles in the Ti-rich cluster are natural and cannot be distinguished from the Ti-rich SS particles based on HCA. This background of natural Ti-rich particles is also evident in the PNCs from the Ti-rich cluster, in which the PNC from the most dilute mixed sample (S1) is elevated compared to the expected value. On the other hand, the Zn-rich cluster is solely associated with SS particles. No RW PCP is found in the Zn-rich cluster and the PNC of the found particles increases linearly from S1 to S6. As seen from the heatmap, the PCPs in the Zn-rich cluster contain both Zn and Ti as commonly occurring elements, which indicates that particles with measurable amounts of both Zn and Ti are (for the samples presented here) useful in discriminating between SS and RW particle types.
The natural RW sample used here for SS + RW mixed samples had a low (<1%) number fraction of particles detected with Ti or Zn. In our experience, this number fraction is typical of the particle composition of freshwater streams.49 However, to test our method in a more challenging matrix, we spiked Ti-containing nanominerals (i.e. rutile, ilmenite, and biotite) into the RW sample and then analyzed this Ti-enriched RW sample with various SS particle concentrations. Results from this analysis are presented in Fig. S4,† and a discussion of this analysis is provided in the ESI.† In summary, we found similar major clusters from two-stage HCA as in the RW + SS mixture samples. However, a fraction of particles from the Ti-containing nanominerals were also grouped with the Ti-rich cluster. Even with elevated concentrations of common Ti-containing nanominerals, two-stage HCA demonstrated that particles assigned to the Zn-rich cluster were almost solely from the SS sample. Thus, we conclude that separation of anthropogenic SS particles from RW/Ti-nanomineral mixtures is possible at the single-particle level based on the presence of Zn and Ti in the SS particle. While Al is well correlated with Ti (cf.Fig. 1) because of its use as a coating for TiO2 particles in sunscreen, this element is not useful in the classification of anthropogenic SS particles in real matrices because of the low abundances of Al in the SS particles and the high abundance of Al in the natural background.
Correlation of the Zn and Ti mass in sunscreen particles
Our two-stage HCA suggests that the Zn-rich cluster is highly characteristic of SS particles. In this Zn-rich cluster, Ti and Zn are both detected in more than 20% of the particles and are often measured as mm-TiZn particles. We did not anticipate the detection of TiZn-containing particles from sunscreen; however, some reports of nanoaggregates of TiO2 and ZnO particles in sunscreen are consistent with our results.73–75 Following HCA, all particle data from 2nd-stage major clusters or the 1st-stage PCP clusters can be further analyzed. HCA allows us to perform targeted more detailed analysis of our spICP-TOFMS data. In Fig. 4, we plot the mass correlation of Zn and Ti in particles from the neat SS sample that are grouped into the Zn-rich and Ti-rich clusters. Plotting the mass correlation of Zn and Ti from the Zn-rich and Ti-rich clusters provides insight into how the unsupervised HCA separates particle masses into distinct clusters. Specifically, we find that the Zn-rich cluster is composed of sm-Zn particles and TiZn particles with a Zn
:
Ti mass ratio greater than of 1.1
:
1. The Ti-rich cluster is composed predominately of sm-Ti particles and TiZn particles with a Zn
:
Ti mass ratio less than of 1.1
:
1. The Zn-rich cluster has the majority of the TiZn-containing particles. The mass ratio cutoff for TiZn particle class assignment is established by the HCA protocol and depends on the cutoff distances used to define clusters in both the intra-sample and inter-sample clustering. Importantly, since all elements are used for the HCA analysis, these mass-ratio cutoffs may not be identical for all samples and may not be the defining characteristics for major cluster assignment. From Fig. 4, it is also apparent that, while Zn and Ti are measured together in many individual particles, they are not well correlated (R2 = 0.4). The lack of correlation between Zn and Ti further suggests that these particles are nanocomposites and not purposely created with a defined mass ratio.
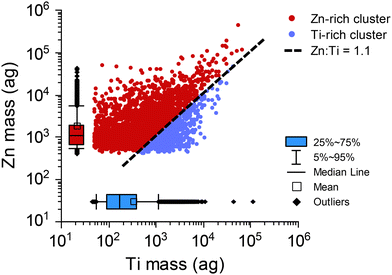 |
| Fig. 4 TiZn particles present in Zn-rich and Ti-rich clusters of the neat sunscreen particle suspension, which has the highest concentration of SS particles. Boxplot on the y-axis shows the single-metal Zn-mass distribution in the Zn-rich cluster and the x-axis shows the single-metal Ti-mass distribution in the Ti-rich cluster. | |
Quantification of Ti and Zn particles released from sunscreen against Ti and Zn particles present in river water
In Fig. 3, we plotted results from two-stage HCA of neat and mixed samples and showed that particles from clusters 4 and 5 (Zn-rich and Ti-rich) are predominately SS particles based on increasing PNCs in these clusters as a function of SS particle concentration. However, in addition to overall PNCs in each cluster, single-particle data from each cluster can be extracted to classify the Ti- and Zn-bearing particles as originating from spiked sunscreen or the river water matrix. In Fig. 5, we present the PNCs of Ti-bearing particles (Fig. 5a) and Zn-bearing particles (Fig. 5b) that are present in the Ti-rich cluster, the Zn-rich cluster, the AlFe-rich cluster (cluster 3), and the Fe-rich cluster (cluster 1) from the spiked samples (S1–S6). Here, we consider the Ti-rich and Zn-rich clusters to be characteristic of SS and the AlFe-rich and Fe-rich clusters to be characteristic of RW (i.e., natural background particles).
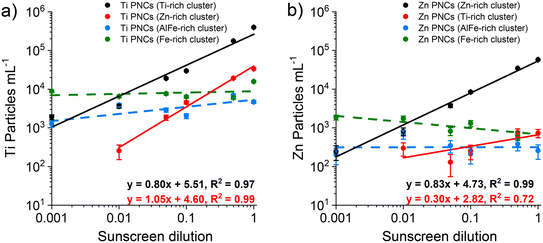 |
| Fig. 5 Quantification of Ti-bearing particles (a) and Zn-bearing particles (b) spiked from sunscreen into river water. Data points plotted are based on particles per mL present in merged (triplicate measurements merged for HCA) data files. Error bars plotted are the predicted standard deviation based on Poisson–Normal statistics, in which the RSD is calculated at and N is the number of particle events recorded. | |
Fig. 5 does not include Ti- and Zn-bearing particles from other clusters because such particles are either absent or occur at very low PNCs. Comparing the slopes and linearities of the curves in Fig. 5 allows us to assess the accuracy of classification and susceptibility for false-positive SS-particle classifications. On a log–log plot, a slope of 1 indicates perfect linearity. When we consider the most common particle types in each cluster, i.e., Ti particles in the Ti-rich cluster (Fig. 5a) and Zn particles in the Zn-rich cluster (Fig. 5b), we find that, while both particle types increase with sunscreen concentration, both curves have slopes less than 1. This non-linearity is likely due to non-negligible numbers of natural Ti- and Zn-containing particles in the RW at low SS concentrations (i.e., in samples S1 and S2). On average, 1% of measured RW particles contain Ti and 0.1% contain Zn, which corresponds to the measurement of 213 and 28 total Ti- and Zn-containing particles, respectively, from the RW sample. Of the RW particles assigned to cluster 5 (Ti-rich cluster), 49 contain Ti, which corresponds to a false-positive rate of 0.3% of RW particles classified as sunscreen and a PNC of ∼2000 mL−1. This low number of false positives interferes with the detection of very dilute SS particles, from which less than ∼50 SS particles are expected to be measured. Because there are very few Zn particles in the Ti-rich cluster, these particles are not suited for SS particle quantification.
Based on HCA analysis, we find that that classification of Ti-containing particles in the Zn-rich cluster enables the fewest false-positive classifications. In this case, the linearity of recorded PNC vs. dilution amount is excellent, with slope = 1.05. In the Zn-rich cluster, from sample S2 to sample S6 (100-fold concentration range), the total number of recorded Ti-containing particles spans from 6 to 779. The very low natural background of Ti particles in the Zn-rich cluster enables low false positive SS classifications and improved linearity. In fact, based on our measurements of over 25
000 RW particles per sample, we cannot establish a false positive rate of the RW water particles; in this case, the false-positive rate is simply too low. Linearity at the low concentration end of SS dilution is limited by low SS particle numbers. As seen in Fig. 5a, we demonstrate that Ti-bearing SS particles from the Zn-rich cluster can be measured at PNCs at least ∼50× lower than background Ti-containing particles.
In the Fig. S5,† we report the quantification of SS particles in a river water matrix supplemented with Ti-nanominerals (i.e., rutile, ilmenite, and biotite). A detailed description of these results is provided in the ESI.† In this Ti-particle-supplemented matrix, it is significantly more challenging to separate SS particles from background particles because the concentration of Ti-containing particles in this matrix is around 5-times higher than that in the RW alone. Moreover, as expected, many of the Ti-nanominerals tend to cluster along with the SS particles in the Ti-rich cluster. Nonetheless, we found that classification of Ti-containing particles from the Zn-rich cluster as SS particles produced accurate results with a PNC vs. dilution amount slope of 0.93 on a log–log plot and measurement of SS particles even when present at number concentrations 70× lower than those of background Ti-containing particles.
Conclusions
In this study, we demonstrated how spICP-TOFMS analysis can be used to separate anthropogenic sunscreen-derived nanoparticles (NPs) from naturally occurring NPs from a river water sample at the single-particle level. River water is a complex matrix that contains a large amount of Fe-, Al-, and Mn-bearing particles (97% of total particle number), and also significant amounts (∼1%) of Ti-, Ce- and Pb-bearing particles. With spICP-TOFMS, mineral sunscreen particles are characterized mostly as Ti, Zn, or TiZn particles. Through the use of two-stage hierarchical clustering analysis (HCA), we were able to group particle types according to multi-elemental signatures and develop distinct clusters linked to sunscreen and river water samples. Unlike previous measurements,76–78 we found that the measurement of Ti or Zn alone by spICP-MS is insufficient to classify measured particles as sunscreen derived because such classification results in many false positive results from naturally occurring particles and so is only relevant if SS PNCs are greater than that of the natural background. Here, we demonstrate that data reduction by 2-stage HCA and subsequent selection of Ti-containing particles from the Zn-rich cluster provides excellent SS-particle classification accuracy. To our knowledge, this is the first study to explore the use of 2-stage HCA data reduction as a tool for classification and quantitative analysis of minor anthropogenic particle type against a natural background. With this approach, we demonstrated measurement of sunscreen-derived PNCs across more than two orders of magnitude and at PNCs 50× lower than those of natural background Ti-containing particles.
While our results suggest that simultaneous detection of Ti and Zn from single particles may enable more definitive measurement of anthropogenic sunscreen particles in natural samples, these results are meant to demonstrate proof-of-principle. To more completely understand the limitations and potential utility of the method for real-world analyses, more sunscreen types, other anthropogenic Ti-containing NPs (e.g. from paint or cosmetics), and more background matrices should be explored. Through the analysis of sunscreen particles spiked into river water supplemented with common Ti-nanominerals (i.e. rutile, ilmenite, and biotite), we also demonstrated that our sunscreen-particle-classification approach is effective in complex, mineral-rich matrices. Our study emphasizes the advantage of using unsupervised HCA to group spICP-TOFMS data and enable targeted extraction of spICP-TOFMS data for classification and quantification. This strategy proved effective for the analysis of sunscreen particles in river water and could be extended to classify and quantify sunscreen (and other anthropogenic particle types) released into various surface waters such as river, ponds, and lakes or into other complex samples like soil, sewage sludge, and so on.
Data availability
The two-stage HCA code and spICP-TOFMS data are available at https://github.com/TOFMS-GG-Group/Two-Stage-HCA-sunscreen.
Conflicts of interest
The authors have no conflicts of interest to declare.
Acknowledgements
The authors acknowledge funding through the NSF career grant CHE-2237291. We also acknowledge Ed Raines, curator of the Mines Museum of Earth Science, as well as Dr. James Ranville and Dr. Aaron Goodman from Colorado School of Mines, for providing Ti-mineral samples and river water (Clear creek). We would also like to acknowledge the ISU glass and machine shop for the construction of the microdroplet introduction assembly.
References
- USGS, National Minerals Information Center, Titanium Statistics and Information, (https://www.usgs.gov/centers/nmic/titanium-statistics-and-information) (accessed 2023-10-01).
- ILZSG, International Lead and Zinc Study Group, (https://ilzsg.org/pages/document/p1/list.aspx?ff_aa_document_type=R&from=19 (accessed, 2023-10-01).
- R. Khan, M. A. Inam, S. Z. Zam, D. R. Park and I. T. Yeom, Assessment of Key Environmental Factors Influencing the Sedimentation and Aggregation Behavior of Zinc Oxide Nanoparticles in Aquatic Environment, Water, 2018, 10, 660 CrossRef.
- A. Weir, P. Westerhoff, L. Fabricius, K. Hristovski and N. von Goetz, Titanium dioxide nanoparticles in food and personal care products, Environ. Sci. Technol., 2012, 46, 2242–2250 CrossRef CAS PubMed.
- X. Song, R. Li, H. Li, Z. Hu, A. Mustapha and M. Lin, Characterization and Quantification of Zinc Oxide and Titanium Dioxide Nanoparticles in Foods, Food Bioprocess Technol., 2013, 7, 456–462 CrossRef.
- V. Puddu, H. Choi, D. D. Dionysiou and G. L. Puma, TiO2 photocatalyst for indoor air remediation: Influence of crystallinity, crystal phase, and UV radiation intensity on trichloroethylene degradation, Appl. Catal., B, 2010, 94, 211–218 CrossRef CAS.
-
D. G. Rickerby, in Nanomaterials for Environmental Protection, 2014, pp. 169–182, DOI:10.1002/9781118845530.ch10.
- T. T. Nguyen, M. Patel, S. Kim, R. A. Mir, J. Yi, V.-A. Dao and J. Kim, Transparent photovoltaic cells and self-powered photodetectors by TiO2/NiO heterojunction, J. Power Sources, 2021, 481, 228865 CrossRef CAS.
- A. Milionis, A. Tripathy, M. Donati, C. S. Sharma, F. Pan, K. Maniura-Weber, Q. Ren and D. Poulikakos, Water-Based Scalable Methods for Self-Cleaning Antibacterial ZnO-Nanostructured Surfaces, Ind. Eng. Chem. Res., 2020, 59, 14323–14333 CrossRef CAS PubMed.
- R. Dastjerdi and M. Montazer, A review on the application of inorganic nano-structured materials in the modification of textiles: focus on anti-microbial properties, Colloids Surf., B, 2010, 79, 5–18 CrossRef CAS PubMed.
- H. A. Foster, I. B. Ditta, S. Varghese and A. Steele, Photocatalytic disinfection using titanium dioxide: spectrum and mechanism of antimicrobial activity, Appl. Microbiol. Biotechnol., 2011, 90, 1847–1868 CrossRef CAS PubMed.
- R. Paul, L. Bautista, M. De la Varga, J. M. Botet, E. Casals, V. Puntes and F. Marsal, Nano-cotton Fabrics with High Ultraviolet Protection, Text. Res. J., 2009, 80, 454–462 CrossRef.
- T. G. Smijs and S. Pavel, Titanium dioxide and zinc oxide nanoparticles in sunscreens: focus on their safety and effectiveness, Nanotechnol., Sci. Appl., 2011, 4, 95–112 CrossRef CAS PubMed.
- D. Ziental, B. Czarczynska-Goslinska, D. T. Mlynarczyk, A. Glowacka-Sobotta, B. Stanisz, T. Goslinski and L. Sobotta, Titanium Dioxide Nanoparticles: Prospects and Applications in Medicine, Nanomaterials, 2020, 10, 387 CrossRef CAS PubMed.
- F. Loosli, J. Wang, S. Rothenberg, M. Bizimis, C. Winkler, O. Borovinskaya, L. Flamigni and M. Baalousha, Sewage spills are a major source of titanium dioxide engineered (nano)-particles into the environment, Environ. Sci.: Nano, 2019, 6, 763–777 RSC.
- P. Westerhoff, G. Song, K. Hristovski and M. A. Kiser, Occurrence and removal of titanium at full scale wastewater treatment plants: implications for TiO2 nanomaterials, J. Environ. Monit., 2011, 13, 1195–1203 RSC.
- A. P. Gondikas, F. von der Kammer, R. B. Reed, S. Wagner, J. F. Ranville and T. Hofmann, Release of TiO2 nanoparticles from sunscreens into surface waters: a one-year survey at the old Danube recreational Lake, Environ. Sci. Technol., 2014, 48, 5415–5422 CrossRef CAS PubMed.
- S. Narla and H. W. Lim, Sunscreen: FDA regulation, and environmental and health impact, Photochem. Photobiol. Sci., 2020, 19, 66–70 CrossRef CAS PubMed.
- US Food and Drug Administration Sunscreen Drug Products for Over-The-Counter Human Use; Proposed Rule, Federal Register. https://www.govinfo.gov/content/pkg/FR-2019-02-26/pdf/2019-03019.pdf, Published February 26, 2019.
- E. P. O. Additives, F. Products or Substances used in Animal, V. Bampidis, G. Azimonti, M. L. Bastos, H. Christensen, B. Dusemund, M. Fasmon Durjava, M. Kouba, M. Lopez-Alonso, S. Lopez Puente, F. Marcon, B. Mayo, A. Pechova, M. Petkova, F. Ramos, Y. Sanz, R. E. Villa, R. Woutersen, G. Aquilina, G. Bories, J. Gropp, J. Galobart and M. V. Vettori, Safety and efficacy of a feed additive consisting of titanium dioxide for all animal species (Titanium Dioxide Manufacturers Association), EFSA J., 2021, 19, e06630 Search PubMed.
- C. Antoniou, M. G. Kosmadaki, A. J. Stratigos and A. D. Katsambas, Sunscreens - what's important to know, J. Eur. Acad. Dermatol. Venereol., 2008, 22, 1110–1119 CrossRef CAS PubMed.
- W. G. Wamer, J. J. Yin and R. R. Wei, Oxidative damage to nucleic acids photosynthesized by titanium dioxide, Free Radical Biol. Med., 1997, 23, 851–858 CrossRef CAS PubMed.
- E. K. Dufour, T. Kumaravel, G. J. Nohynek, D. Kirkland and H. Toutain, Clastogenicity, photo-clastogenicity or pseudo-photo-clastogenicity: Genotoxic effects of zinc oxide in the dark, in pre-irradiated or simultaneously irradiated Chinese hamster ovary cells, Mutat. Res., 2006, 607, 215–224 CAS.
-
IARC, Carbon black, titanium dioxide, and talc, IARC monographs on the evaluation of carcinogenic risks to humans, International Agency for Research on Cancer, Lyon, France, 2006, vol. 93 Search PubMed.
- A. Fujishima, X. Zhang and D. Tryk, TiO2 photocatalysis and related surface phenomena, Surf. Sci. Rep., 2008, 63, 515–582 CrossRef CAS.
- E. Jang, K. Sridharan, Y. M. Park and T. J. Park, Eliminated Phototoxicity of TiO2 Particles by an Atomic-Layer-Deposited Al2 O3 Coating Layer for UV-Protection Applications, Chemistry, 2016, 22, 12022–12026 CrossRef CAS PubMed.
- D. M. King, X. Liang, B. B. Burton, M. K. Akhtar and A. W. Weimer, Passivation of pigment-grade TiO(2) particles by nanothick atomic layer deposited SiO(2) films, Nanotechnology, 2008, 19, 255604 CrossRef PubMed.
- M. D. Newman, M. Stotland and J. I. Ellis, The safety of nanosized particles in titanium dioxide- and zinc oxide-based sunscreens, J. Am. Acad. Dermatol., 2009, 61, 685–692 CrossRef CAS PubMed.
- K. Schilling, B. Bradford, D. Castelli, E. Dufour, J. F. Nash, W. Pape, S. Schulte, I. Tooley, J. van den Bosch and F. Schellauf, Human safety review of “nano” titanium dioxide and zinc oxide, Photochem. Photobiol. Sci., 2010, 9, 495–509 CrossRef CAS PubMed.
- H. Zanker and A. Schierz, Engineered nanoparticles and their identification among natural nanoparticles, Annu. Rev. Anal. Chem., 2012, 5, 107–132 CrossRef CAS PubMed.
- B. Kim, C.-S. Park, M. Murayama and M. F. Hochella, Jr., Discovery and Characterization of Silver Sulfide Nanoparticles in Final Sewage Sludge Products, Environ. Sci. Technol., 2010, 44, 7509–7514 CrossRef CAS PubMed.
- B. G. Kim and I. J. Kang, Evaluation of the effects of biodegradable nanoparticles on a vaccine delivery system using AFM, SEM, and TEM, Ultramicroscopy, 2008, 108, 1168–1173 CrossRef CAS PubMed.
- F. Tou, Y. Yang, J. Feng, Z. Niu, H. Pan, Y. Qin, X. Guo, X. Meng, M. Liu and M. F. Hochella, Environmental Risk Implications of Metals in Sludges from Waste Water Treatment Plants: The Discovery of Vast Stores of Metal-Containing Nanoparticles, Environ. Sci. Technol., 2017, 51, 4831–4840 CrossRef CAS PubMed.
- Y. Yang, B. Chen, J. Hower, M. Schindler, C. Winkler, J. Brandt, R. Di Giulio, J. Ge, M. Liu, Y. Fu, L. Zhang, Y. Chen, S. Priya and M. F. Hochella, Jr., Discovery and ramifications of incidental Magneli phase generation and release from industrial coal-burning, Nat. Commun., 2017, 8, 194 CrossRef PubMed.
- A. E. Pradas del Real, H. Castillo-Michel, R. Kaegi, C. Larue, W. de Nolf, J. Reyes-Herrera, R. Tucoulou, N. Findling, E. Salas-Colera and G. Sarret, Searching for relevant criteria to distinguish natural vs. anthropogenic TiO2 nanoparticles in soils, Environ. Sci.: Nano, 2018, 5, 2853–2863 RSC.
- A. P. Gondikas, F. v. d. Kammer, R. B. Reed, S. Wagner, J. F. Ranville and T. Hofmann, Release of TiO2 nanoparticles from sunscreens into surface waters: a one-year survey at the old Danube recreational Lake, Environ. Sci. Technol., 2014, 48, 5415–5422 CrossRef CAS PubMed.
- K. Phalyvong, Y. Sivry, H. Pauwels, A. Gélabert, M. Tharaud, G. Wille, X. Bourrat and M. F. Benedetti, Occurrence and Origins of Cerium Dioxide and Titanium Dioxide Nanoparticles in the Loire River (France) by Single Particle ICP-MS and FEG-SEM Imaging, Front. Environ. Sci., 2020, 8, 141 CrossRef.
- R. J. B. Peters, G. van Bemmel, N. B. L. Milani, G. C. T. den Hertog, A. K. Undas, M. van der Lee and H. Bouwmeester, Detection of nanoparticles in Dutch surface waters, Sci. Total Environ., 2018, 621, 210–218 CrossRef CAS PubMed.
- L. Fréchette-Viens, M. Hadioui and K. J. Wilkinson, Quantification of ZnO nanoparticles and other Zn containing colloids in natural waters using a high sensitivity single particle ICP-MS, Talanta, 2019, 200, 156–162 CrossRef PubMed.
- M. Hadioui, V. Merdzan and K. J. Wilkinson, Detection and Characterization of ZnO Nanoparticles in Surface and Waste Waters Using Single Particle ICPMS, Environ. Sci. Technol., 2015, 49, 6141–6148 CrossRef CAS PubMed.
- R. B. Reed, D. P. Martin, A. J. Bednar, M. D. Montaño, P. Westerhoff and J. F. Ranville, Multi-day diurnal measurements of Ti-containing nanoparticle and organic sunscreen chemical release during recreational use of a natural surface water, Environ. Sci.: Nano, 2017, 4, 69–77 RSC.
- A. Gondikas, F. von der Kammer, R. Kaegi, O. Borovinskaya, E. Neubauer, J. Navratilova, A. Praetorius, G. Cornelis and T. Hofmann, Where is the nano? Analytical approaches for the detection and quantification of TiO2 engineered nanoparticles in surface waters, Environ. Sci.: Nano, 2018, 5, 313–326 RSC.
- O. Borovinskaya, S. Gschwind, B. Hattendorf, M. Tanner and D. Gunther, Simultaneous mass quantification of nanoparticles of different composition in a mixture by microdroplet generator-ICPTOFMS, Anal. Chem., 2014, 86, 8142–8148 CrossRef CAS PubMed.
- O. Borovinskaya, B. Hattendorf, M. Tanner, S. Gschwind and D. Günther, A prototype of a new inductively coupled plasma time-of-flight mass spectrometer providing temporally resolved, multi-element detection of short signals generated by single particles and droplets, J. Anal. At. Spectrom., 2013, 28, 226–233 RSC.
- L. Hendriks, A. Gundlach-Graham, B. Hattendorf and D. Günther, Characterization of a new ICP-TOFMS instrument with continuous and discrete introduction of solutions, J. Anal. At. Spectrom., 2017, 32, 548–561 RSC.
- K. Mehrabi, D. Günther and A. Gundlach-Graham, Single-particle ICP-TOFMS with online microdroplet calibration for the simultaneous quantification of diverse nanoparticles in complex matrices, Environ. Sci.: Nano, 2019, 6, 3349–3358 RSC.
- S. Naasz, S. Weigel, O. Borovinskaya, A. Serva, C. Cascio, A. K. Undas, F. C. Simeone, H. J. P. Marvin and R. J. B. Peters, Multi-element analysis of single nanoparticles by ICP-MS using quadrupole and time-of-flight technologies, J. Anal. At. Spectrom., 2018, 33, 835–845 RSC.
- A. Praetorius, A. Gundlach-Graham, E. Goldberg, W. Fabienke, J. Navratilova, A. Gondikas, R. Kaegi, D. Günther, T. Hofmann and F. von der Kammer, Single-particle multi-element fingerprinting (spMEF) using inductively-coupled plasma time-of-flight mass spectrometry (ICP-TOFMS) to identify engineered nanoparticles against the elevated natural background in soils, Environ. Sci.: Nano, 2017, 4, 307–314 RSC.
- H. Karkee and A. Gundlach-Graham, Characterization and Quantification of Natural and Anthropogenic Titanium-Containing Particles Using Single-Particle ICP-TOFMS, Environ. Sci. Technol., 2023, 57, 14058–14070 CrossRef CAS PubMed.
- S. Harycki and A. Gundlach-Graham, Characterization of a high-sensitivity ICP-TOFMS instrument for microdroplet, nanoparticle, and microplastic analyses, J. Anal. At. Spectrom., 2022, 38, 111–120 RSC.
- F. Loosli, J. Wang, S. Rothenberg, M. Bizimis, C. Winkler, O. Borovinskaya, L. Flamigni and M. Baalousha, Sewage spills are a major source of titanium dioxide engineered (nano)-particle release into the environment, Environ. Sci.: Nano, 2019, 6, 763–777 RSC.
- S. Bevers, M. D. Montaño, L. Rybicki, T. Hofmann, F. von der Kammer and J. F. Ranville, Quantification and Characterization of Nanoparticulate Zinc in an Urban Watershed, Front. Environ. Sci., 2020, 8, 84 CrossRef.
- A. Azimzada, I. Jreije, M. Hadioui, P. Shaw, J. M. Farner and K. J. Wilkinson, Quantification and Characterization of Ti-, Ce-, and Ag-Nanoparticles in Global Surface Waters and Precipitation, Environ. Sci. Technol., 2021, 55, 9836–9844 CrossRef CAS PubMed.
- R. L. Buckman and A. Gundlach-Graham, Machine learning analysis to classify nanoparticles from noisy spICP-TOFMS data, J. Anal. At. Spectrom., 2023, 38, 1244–1252 RSC.
- G. D. Bland, M. Battifarano, A. E. Pradas Del Real, G. Sarret and G. V. Lowry, Distinguishing Engineered TiO(2) Nanomaterials from Natural Ti Nanomaterials in Soil Using spICP-TOFMS and Machine Learning, Environ. Sci. Technol., 2022, 56, 2990–3001 CrossRef CAS PubMed.
- G. D. Bland, P. Zhang, E. Valsami-Jones and G. V. Lowry, Application of Isotopically Labeled Engineered Nanomaterials for Detection and Quantification in Soils via Single-Particle Inductively Coupled Plasma Time-of-Flight Mass Spectrometry, Environ. Sci. Technol., 2022, 56, 15584–15593 CrossRef CAS PubMed.
- K. Mehrabi, R. Kaegi, D. Gunther and A. Gundlach-Graham, Emerging investigator series: automated single-nanoparticle quantification and classification: a holistic study of particles into and out of wastewater treatment plants in Switzerland, Environ. Sci.: Nano, 2021, 8, 1211–1225 RSC.
- M. Baalousha, J. Wang, M. Erfani and E. Goharian, Elemental fingerprints in natural nanomaterials determined using SP-ICP-TOF-MS and clustering analysis, Sci. Total Environ., 2021, 792, 148426 CrossRef CAS PubMed.
- J. Wang, M. D. M. Nabi, M. Erfani, E. Goharian and M. Baalousha, Identification and quantification of anthropogenic nanomaterials in urban rain and runoff using single particle-inductively coupled plasma-time of flight-mass spectrometry, Environ. Sci.: Nano, 2022, 9, 714–729 RSC.
- M. Erfani, M. Baalousha and E. Goharian, Unveiling elemental fingerprints: A comparative study of clustering methods for multi-element nanoparticle data, Sci. Total Environ., 2023, 905, 167176 CrossRef CAS PubMed.
- A. J. Goodman, A. Gundlach-Graham, S. G. Bevers and J. F. Ranville, Characterization of nano-scale mineral dust aerosols in snow by single particle inductively coupled plasma mass spectrometry, Environ. Sci.: Nano, 2022, 9, 2638–2652 RSC.
- M. Tharaud, L. Schlatt, P. Shaw and M. F. Benedetti, Nanoparticle identification using single particle ICP-ToF-MS acquisition coupled to cluster analysis. From engineered to natural nanoparticles, J. Anal. At. Spectrom., 2022, 37, 2042–2052 RSC.
- L. N. Rand, Y. Bi, A. Poustie, A. J. Bednar, D. J. Hanigan, P. Westerhoff and J. F. Ranville, Quantifying temporal and geographic variation in sunscreen and mineralogic titanium-containing nanoparticles in three recreational rivers, Sci. Total Environ., 2020, 743, 140845 CrossRef CAS PubMed.
- P. J. Lu, S. W. Fang, W. L. Cheng, S. C. Huang, M. C. Huang and H. F. Cheng, Characterization of titanium dioxide and zinc oxide nanoparticles in sunscreen powder by comparing different measurement methods, J. Food Drug Anal., 2018, 26, 1192–1200 CrossRef CAS PubMed.
- H. Karkee, C. Kyte and A. Gundlach-Graham, Classification of zirconium-rich engineered and natural nano particles using single particle ICP-TOFMS, J. Anal. At. Spectrom., 2024, 39, 1551–1559 RSC.
- S. Harycki and A. Gundlach-Graham, Online microdroplet calibration for accurate nanoparticle quantification in organic matrices, Anal. Bioanal. Chem., 2022, 414, 7543–7551 CrossRef CAS PubMed.
- A. Gundlach-Graham, S. Harycki, S. E. Szakas, T. L. Taylor, H. Karkee, R. L. Buckman, S. Mukta, R. Hu and W. Lee, Introducing “time-of-flight single particle investigator” (TOF-SPI): a tool for quantitative spICP-TOFMS data analysis, J. Anal. At. Spectrom., 2024, 39, 704–711 RSC.
- A. Gundlach-Graham and K. Mehrabi, Monodisperse microdroplets: a tool that advances single-particle ICP-MS measurements, J. Anal. At. Spectrom., 2020, 35, 1727–1739 RSC.
- A. Gundlach-Graham, L. Hendriks, K. Mehrabi and D. Gunther, Monte Carlo Simulation of Low-Count Signals in Time-of-Flight Mass Spectrometry and Its Application to Single-Particle Detection, Anal. Chem., 2018, 90, 11847–11855 CrossRef CAS PubMed.
- A. Gundlach-Graham and R. Lancaster, Mass-Dependent Critical Value Expressions for Particle Finding in Single-Particle ICP-TOFMS, Anal. Chem., 2023, 95, 5618–5626 CrossRef CAS PubMed.
- D. L. Slomberg, R. Catalano, V. Bartolomei and J. Labille, Release and fate of nanoparticulate TiO(2) UV filters from sunscreen: Effects of particle coating and formulation type, Environ. Pollut., 2021, 271, 116263 CrossRef CAS PubMed.
-
R. L. Rudnick, The crust, Elsevier, 2005 Search PubMed.
- J. Labille, J. Feng, C. Botta, D. Borschneck, M. Sammut, M. Cabie, M. Auffan, J. Rose and J.-Y. Bottero, Aging of TiO2 nanocomposites used in sunscreen. Dispersion and fate of the degradation products in aqueous environment, Environ. Pollut., 2010, 158, 3482–3489 CrossRef CAS PubMed.
- C. Botta, J. Labille, M. Auffan, D. Borschneck, H. Miche, M. Cabié, A. Masion, J. Rose and J.-Y. Bottero, TiO2-based nanoparticles released in water from commercialized sunscreens in a life-cycle perspective: Structures and quantities, Environ. Pollut., 2011, 159, 1543–1550 CrossRef CAS PubMed.
- Y. Zheng and B. Nowack, Size-Specific, Dynamic, Probabilistic Material Flow Analysis of Titanium Dioxide Releases into the Environment, Environ. Sci. Technol., 2021, 55, 2392–2402 CrossRef CAS PubMed.
- A. K. Venkatesan, R. B. Reed, S. Lee, X. Bi, D. Hanigan, Y. Yang, J. F. Ranville, P. Herckes and P. Westerhoff, Detection and Sizing of Ti-Containing Particles in Recreational Waters Using Single Particle ICP-MS, Bull. Environ. Contam. Toxicol., 2018, 100, 120–126 CrossRef CAS PubMed.
- R. Gonzalez de Vega, T. E. Lockwood, X. Xu, C. Gonzalez de Vega, J. Scholz, M. Horstmann, P. A. Doble and D. Clases, Analysis of Ti- and Pb-based particles in the aqueous environment of Melbourne (Australia) via single particle ICP-MS, Anal. Bioanal. Chem., 2022, 414, 5671–5681 CrossRef CAS PubMed.
- J. Vidmar, T. Zuliani, R. Milačič and J. Ščančar, Following the occurrence and origin of titanium dioxide nanoparticles in the Sava River by single particle ICP-MS, Water, 2022, 14, 959 CrossRef CAS.
|
This journal is © The Royal Society of Chemistry 2024 |