DOI:
10.1039/D4TB00865K
(Review Article)
J. Mater. Chem. B, 2024,
12, 6735-6756
A nanoparticle-assisted signal-enhancement technique for lateral flow immunoassays
Received
21st April 2024
, Accepted 12th June 2024
First published on 13th June 2024
Abstract
Lateral flow immunoassay (LFIA), an affordable and rapid paper-based detection technology, is employed extensively in clinical diagnosis, environmental monitoring, and food safety analysis. The COVID-19 pandemic underscored the validity and adoption of LFIA in performing large-scale clinical and public health testing. The unprecedented demand for prompt diagnostic responses and advances in nanotechnology have fueled the rise of next-generation LFIA technologies. The utilization of nanoparticles to amplify signals represents an innovative approach aimed at augmenting LFIA sensitivity. This review probes the nanoparticle-assisted amplification strategies in LFIA applications to secure low detection limits and expedited response rates. Emphasis is placed on comprehending the correlation between the physicochemical properties of nanoparticles and LFIA performance. Lastly, we shed light on the challenges and opportunities in this prolific field.
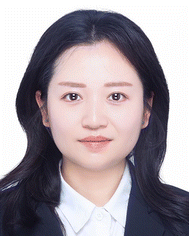
Fang Gao
| Fang Gao earned her PhD from the University of Queensland, Australia, in 2022. She then worked as an Analytical Technical Officer at Sanitarium Health Food Company in Australia from 2022 to 2023. Since 2023, she has served as a Research Professor at the University of Shanghai for Science and Technology. Her primary research focuses on developing quantum dot-based biosensors and signal amplification systems for analytical chemistry, with applications in point-of-care diagnostics. |
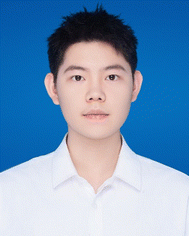
Shaonian Ye
| Shaonian Ye received his BEng degree (2019) from Jingdezhen Ceramic University. He then joined Professor Fang Gao's group and is currently a Master's student at the University of Shanghai for Science and Technology. His major research focuses on the synthesis of quantum dot-based fluorescence biosensors and their application in point-of-care diagnosis. |
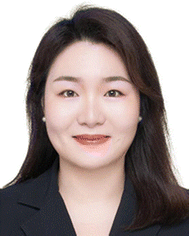
Lin Huang
| Lin Huang received her PhD in biomedical engineering from Shanghai Jiao Tong University, China. Currently, she is a Senior Research Fellow (Principal Investigator) at Shanghai Chest Hospital, Shanghai Jiao Tong University School of Medicine, China. Her research interests focus on the development of novel materials for in vitro diagnostics based on various analytical methods. |
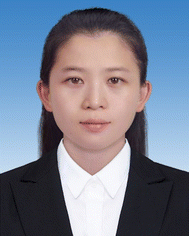
Zhengying Gu
| Zhengying Gu received her PhD in biomedical engineering from the University of Queensland, Australia. She is currently an Assistant Research Fellow at Shanghai Chest Hospital, Shanghai Jiao Tong University, School of Medicine, China. Her research interests focus on the study of immuno-metabolic interaction and the development of diagnostic and therapeutic nanotechnologies. |
1. Introduction
The lateral flow immunoassay (LFIA) strip is a simple paper-based point-of-care (POC) testing device designed to rapidly and cost-effectively detect target analytes.1 It fully aligns with the ASSURED criteria outlined by the World Health Organization for an ideal POC diagnostic device that is affordable, sensitive, specific, user-friendly, rapid and robust, equipment-free and deliverable.2 Recently, the application of LFIA has expanded beyond clinical diagnostics3–5 to include uses in food safety6,7 and environmental monitoring.8 The global market revenue for LFIAs is projected to increase from $20.5 billion in 2022 to an estimated $22.6 billion by 2027.9 The COVID-19 pandemic has underscored the widespread applicability and effectiveness of LFIA testing for clinical and public health purposes.10 However, the significant occurrence of false negatives has spurred the need for new generation LFIA technologies with a lower limit of detection (LoD).11,12 Given the low concentration (10−16–10−12 M) of crucial biomarkers in life-threatening diseases such as cancers and contagious diseases,13,14 achieving a lower LoD with LFIA is essential for effective interventions and therapies to help reduce mortality and morbidity.15,16
Significant efforts have been dedicated to improving detection sensitivity, as evidenced by numerous high-quality reviews in the field.17–22 These endeavours encompass a range of strategies, including orientating antibodies on the labels and membranes,23,24 exploring alternative recognition units such as aptamers25,26 and glycans27in lieu of antibodies, integrating isothermal amplification methods4 and/or clustered regularly interspaced short palindromic repeats (CRISPR) and CRISPR-associated (Cas) system28–30 with LFIA, controlling the flow dynamics in LFIA,31 developing dedicated reader devices,32 and customizing labelling materials10,33–35 like noble metal nanomaterials,36–39 quantum dots (QDs),40 upconversion nanomaterials,41 and enzymes42 to amplify colorimetric, surface-enhanced Raman scattering (SERS), fluorescence, chemiluminescence, and electrochemical43 signals.
The utilization of nanoparticle-assisted signal amplification represents an innovative approach aimed at augmenting LFIA sensitivity. In this context, the term “nanoparticle” refers to a nanomaterial that serves to enhance the signal generated by the label material,44 rather than functioning as the primary label material45 for target analyte detection. For instance, while polystyrene spheres and silica nanoparticles are unable to independently generate a detectable signal, they boost the recognition process by enriching a large number of labels (e.g., Eu(III) chelates and QDs).46,47 Another illustration is provided by magnetic nanoparticles, which can effectively concentrate target analytes from complex samples and also carry a substantial quantity of labels for signal amplification to improve LFIA performance.48 The physicochemical properties of nanoparticles, including size and surface chemistry, significantly impact the transport dynamics, reaction kinetics, and signal production that ultimately determine LFIA performance. However, there has been limited discourse on how to engineer nanoparticles to enhance their performance in LFIA applications.22,40 Understanding the relationship between the physicochemical properties of a nanoparticle and its performance in LFIA signal amplification is crucial for advancing the development of LFIA with sub-picomolar LoD.49 To date, there has been no comprehensive review of nanoparticle-assisted signal amplification strategies for LFIA, with a particular focus on the crucial involvement of nanoparticles.
Herein, we present a timely overview of the latest nanoparticle-assisted strategies for signal amplification in LFIA, aimed at achieving a lower LoD. The review is structured into three main sections: (1) utilizing nanoparticles to incorporate diverse labels (e.g., small molecules, biomacromolecules, and nanomaterials) for multi-signal enhancement; (2) examining the impacts of key properties (size and surface chemistry) on their performance; and (3) discussing the challenges and prospects in applying tailored nanoparticles for the ultrasensitive LFIA. This review is intended to facilitate and enhance the evolution of the next generation of LFIA technology.
1.1. Nanoparticles for LFIA signal amplification
The LFIA, also known as the immunochromatographic assay, combines the principles of chromatography and immunochemical reactions.50 As depicted in Scheme 1A, a typical LFIA strip consists of four key components: the sample pad, the conjugate pad, the reaction membrane, and the absorbent pad. The sample pad serves as the entry point for the sample, while the conjugate pad houses the labelled primary antibodies. The reaction membrane, usually made of nitrocellulose (NC), leverages the robust dipole interactions between the nitrate esters and the peptide bonds in the proteins to immobilize the primary and secondary antibodies.51 This enables the formation of a test line and a control line based on the target antigen–antibody interaction. The absorbent pad functions as a waste reservoir, collecting the excess sample and reagents as they flow through the system.52 The LFIA works via the capillary flow of the sample across the sequential pads, enabling rapid and user-friendly immunoassays on NC membranes. This integration of chromatographic separation and immunochemical reactions allows for the efficient detection and analysis of target analytes. Compared to other immunoassay techniques like enzyme-linked immunosorbent assay (ELISA), LFIA offers advantages such as simplicity, rapid analysis, portability, and cost-effectiveness, making it a preferred choice for POC and on-site applications.53
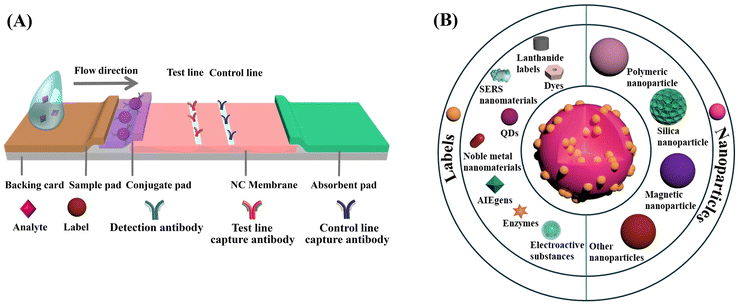 |
| Scheme 1 Schematic diagram of (A) LFIA and (B) nanoparticles for LFIA signal amplification. | |
Embedding sufficient labels onto a single nanoparticle is a highly effective strategy for improving the detection sensitivity of low abundance biomarkers. Much effort has been devoted to developing diverse nanoparticles for label enrichment and signal amplification, including polymeric nanoparticles, silica nanoparticles, magnetic nanoparticles, etc. (Scheme 1B).
1.1.1. Polymeric nanoparticles.
Carboxyl-modified polystyrene nanospheres provide a useful platform for diagnostic applications.54 They can be loaded with various fluorescent labels, such as dyes,55 europium(III)–chelate,56 AIEgens,57 and QDs,58 and can be conjugated with recognition molecules, such as antibodies and antigens.59 The label materials can be enriched in polymeric nanoparticles through embedding into pre-synthesized nanoparticles, incorporating into an emulsion assembly, or via polymerization processes (Fig. 1).
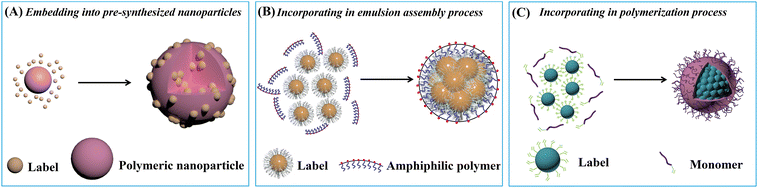 |
| Fig. 1 Enrichment of label materials in polymeric nanoparticles through (A) embedding into pre-synthesized nanoparticles, (B) incorporating in emulsion assembly and (C) polymerization processes. | |
1.1.1.1. Embedding into pre-synthesized nanoparticles.
The swelling-coupled evaporation or centrifugation method is the most widely adopted approach for encapsulating labels such as dyes or QDs within polymeric nanoparticles. This method involves swelling polymer spheres in an organic solvent such as hexane,58 chloroform,60 dichloromethane,61 or tetrahydrofuran.57,59,62 After the labels permeate the interior of the spheres, the organic solvent is removed, causing the polymer network to contract and encapsulate the labels within particles. For example, Zhong et al. utilized hexane as the swelling solvent to prepare QD-embedded polystyrene nanobeads via this method.58 The resulting nanobeads achieved a low LoD of 0.299 ng mL−1 for fetuin-B. The LoD of QD nanobead-based LFIA was 2.5 to 6.25 times lower than that of the colloidal Au-based LFIA in the detection of anticoagulant rodenticides.60 Recently, the near-infrared emissive AIEgen-incorporated carboxyl-modified polystyrene nanospheres have been successfully fabricated through the swelling–centrifugation method and applied to LFIA (Fig. 2).59 An estimated amount of 3.18 × 106 AIEgens was enriched in one polystyrene microsphere of 300 nm diameter, greatly amplifying the fluorescence signal of the obtained near-infrared label. The antibodies further modified on these polystyrene nanoparticles enable the detection of IgM and IgG against the severe acute respiratory syndrome coronavirus 2 (SARS-CoV-2) in clinical serum samples, with LoD values of 0.236 and 0.125 μg mL−1, respectively. These values are comparable to those obtained by the ELISA, which yielded LoD values of 0.040 and 0.039 μg mL−1. The near-infrared labelled-LFIA demonstrated a sensitivity of 78% for IgM detection and 95% for IgG detection as evaluated with 172 serum samples. The sensitivities are comparable to those observed in ELISA, which were found to be 85 and 95% but superior to a commercial colloidal Au-based LFIA with a sensitivity of 41 and 85%. Owing to the significantly enhanced detection sensitivity of the near-infrared label-based LFIA, the test can detect the IgM or IgG antibodies in as early as one to seven days after the onset of symptoms, which is considerably earlier than the eight to fifteen days required by colloidal Au-based LFIA.
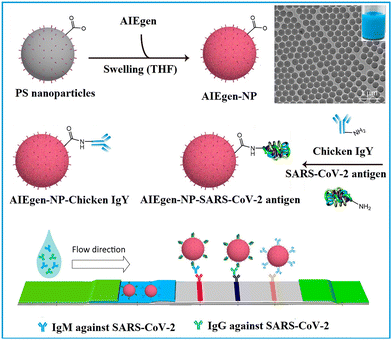 |
| Fig. 2 The enrichment of labels to pre-synthesised polymeric nanoparticles. Reproduced with permission from ref. 59. Copyright 2021 American Chemical Society. | |
Besides the swelling-coupled evaporation or centrifugation method, the labels can be anchored on the surface of polymeric nanoparticles through electrostatic attraction,63 or in situ growth.64 For instance, the surface of commercial carboxyl-modified polystyrene nanospheres was rendered positively charged using poly(diallyldimethyl) ammonium chloride. These nanospheres were then incubated with an excess of negatively charged QDs under ultrasonication.63 The resulting QD-decorated polystyrene nanospheres were coated with a silica shell to protect the fluorescence and for further bio-functionalization. The QD nanobeads were incorporated into a LFIA for SARS-CoV-2 antibody detection, resulting in more than a tenfold increase in detection sensitivity compared to the commercial assay based on colloidal Au. Matsumura et al. designed latex particles consisting of poly(2-vinylpyridine) cross-linked with divinylbenzene. Because of its positively charged pyridine moieties, metal ions (AuCl4−) can be adsorbed on their surface through electrostatic interactions. The Au labels were subsequently formed in situ by the reduction of adsorbed metal ions (AuCl4−).65 The developed latex–Au nanocomposite contains multiple Au labels. Compared with conventional colloidal Au-labelled LFIA, the LoD of influenza A virus H1N1 antigen based on this nanocomposite-labelled LFIA was significantly lowered by 64 times.
1.1.1.2. Incorporating into an emulsion assembly process.
Labels can be encapsulated into polymeric nanoparticles through emulsion strategy during the self-assembly of polymeric micelles. Typically, the hydrophobic label materials are initially dispersed in a nonpolar solvent (e.g. chloroform,66–70 cyclohexane71 and toluene72) containing amphiphilic poly(maleic anhydride-alt-1-octadecene) (PMAO) and/or hydrophobic poly(methyl methacrylate) (PMMA) to form the oil phase. The oil phase is subsequently combined with the aqueous phase containing surfactant and emulsified using an ultrasonic homogeniser to form the oil-in-water emulsion. Subsequently, the nonpolar solvent is evaporated to obtain the nanobeads. This approach has been used to fabricate nanobeads embedded with QDs,66,69,73 gold nanomaterials68,70,72 and AIEgens.67,74
Notably, the amphiphilic nature of PMAO allows for the co-assembly of functional building blocks with different solubility, resulting in a core–shell structure that spatially separates the building blocks. This separation is beneficial for maintaining the functionality of the building blocks and minimizing mutual interferences among them.75–77 As exhibited in Fig. 3, hydrophobic Fe3O4 nanoparticles, AIEgens, and PMAO were dissolved in chloroform and then proceeded through micelle encapsulation in the aqueous phase, with SDS acting as the surfactant. The distinct solubility of Fe3O4 nanoparticles and AIEgens led to the formation of a core–shell nanostructure comprising a dense AIEgen core and a discretely packed Fe3O4 shell. The resultant AIEgen@Fe3O4 nanocomposite exhibits high fluorescence intensity attributed to enhanced photoluminescence from AIEgens stacking and reduced photon loss from the magnetic shell, as well as notable magnetic activity due to minimised magnetic shielding through magneto extraposition. With the assistance of magnetic operation, an LoD of 0.016 ng mL−1 was achieved by AIEgen@Fe3O4-based LFIA in the detection of lipoarabinomannan, representing a 44-fold higher sensitivity than colloidal Au-based LFIA.77
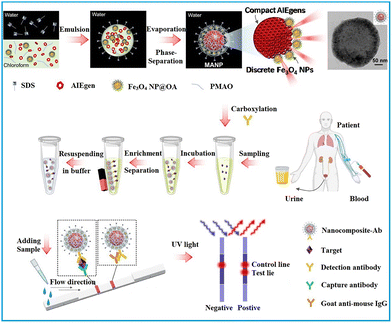 |
| Fig. 3 The enrichment of labels in polymeric nanoparticles through mini-emulsion polymerisation. Reproduced with permission from ref. 77. Copyright 2021 Elsevier. | |
1.1.1.3. Incorporating into a polymerization process.
The labels including europium(III)–chelate,47,78,79 QDs,80,81 dyes82 and AIEgens83 can also be embedded into polymeric nanoparticles through polymerization.47,78–80,83 Typically, hydrophobic label materials are present in the oil phase, while carboxyl-providing monomers such as acrylic acid,47 methacrylic acid,80 methyl methacrylate,82 and maleic anhydride83 are present in the aqueous phase. Styrene monomers may be present in either the oil phase or aqueous phase with the aid of a surfactant, and all these components form a mini-emulsion. This emulsion then undergoes polymerization in the presence of an initiator (e.g. potassium persulfate,80 azobisiobutyronitrile83 and 4,4′-azobis(4-cyanovaleric acid)78) or under heat, resulting in the formation of polymeric nanospheres. As shown in Fig. 4, the carboxyl-modified Eu(III) chelate nanospheres were synthesised by using a one-step mini-emulsion polymerisation method.78 The aqueous phase consisted of surfactant sodium dodecyl benzenesulfonate, carboxyl-providing acrylic acid and initiator 4,4′-azobis(4-cyanovaleric acid), which were mixed with styrene monomers, Eu(III) chelate labels and n-hexadecane in the oil phase. The mixture was emulsified under ultrasonication in an ice bath, followed by heating the mini-emulsion to 70 °C in an inlet atmosphere to allow polymerisation. During the one-step polymerisation, the Eu(III) chelates were immobilised within the interior hydrophobic environment and dispersed throughout the entire nanospheres. Compared with the swelling method, the Eu(III) chelate nanospheres prepared by the one-step polymerisation exhibited higher fluorescence intensity. The Eu(III) chelate nanosphere-based LFIAs demonstrated a LoD of 0.683 ng mL−1 in the detection of alpha-fetoprotein.78
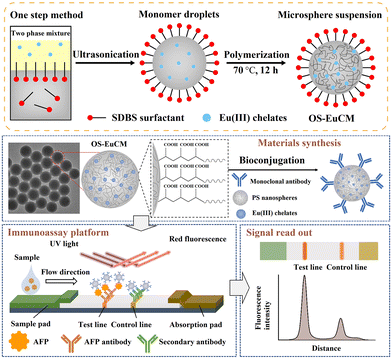 |
| Fig. 4 The emulsion assembly strategy enabled spatial separation of different functional building blocks. Reproduced from ref. 78 with open access from John Wiley and Sons. | |
This section illustrates three strategies for nanoparticle-based label enrichment. The swelling method is widely utilized for its operational simplicity and the consistent, reproducible sizes of the resulting nanobeads. Nevertheless, in this approach, labels tend to be predominantly located in the superficial layer of the polymeric nanoparticles, potentially restricting the loading capacity of labels. In contrast, nanobeads fabricated by the emulsion assembly and polymerization techniques may offer enhanced loading capacity. However, these strategies could pose challenges such as reduced reproducibility and label aggregation within the polymer frameworks.84 The emulsion assembly strategy enables the concurrent assembly of diverse functional components with spatial separation. Nonetheless, nanobeads prepared using this approach often display non-uniform size distribution, potentially affecting assay reproducibility.84,85
1.1.2. Silica nanoparticles.
Silica nanoparticles have been widely used for signal amplification in analytical applications,86–88 although their specific applications in LFIA have been less discussed. Silica nanoparticles possess superior characteristics such as adjustable particle size and pore size, uniform particle size distribution, ease of functionalisation, and good stability, making them highly suitable for a wide range of applications in label accumulation and signal amplification.89 Various types of silica nanoparticles, including nonporous silica nanoparticles, small-pored silica nanoparticles, and large-pored silica nanoparticles, have been extensively employed for signal amplification in LFIA platforms (Fig. 5).90,91 These nanoparticles exhibit distinct features in terms of surface area, pore size, and pore structure, influencing their compatibility with different types of labels.
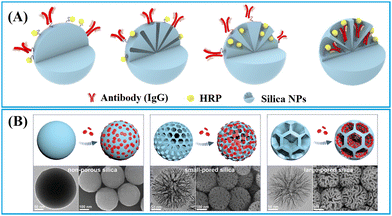 |
| Fig. 5 Strategies for enriching (A) biomacromolecules and (B) nanomaterials to nonporous, small-pored, and large-pored silica nanoparticles. Reprinted with permission from ref. 90 and 91. Copyright 2021 American Chemical Society and 2024 John Wiley and Sons. | |
1.1.2.1. Nonporous silica nanoparticles.
The nonporous silica nanoparticles can have labels enriched on their surface through physical or chemical adsorption.92–97 A typical physical adsorption approach involves rendering silica nanoparticles positively charged by coating them with a cationic polyethyleneimine (PEI) layer92,93 or functionalising them with amino groups,94 which facilitate the adsorption of water-soluble negatively charged QDs98,99 or gold nanomaterials.94 Silica nanoparticles can be also functionalised with thiol groups to facilitate the assembly of hydrophobic QDs through thiol–metal coordination, which is a common chemical adsorption method used for label enrichment.95–97 However, the loading capacity of surface enrichment is constrained by the limited surface area of nonporous silica nanoparticles.
To enhance the loading capacity, a layer-by-layer assembly approach has been developed.98–100 Through repetitive self-assembly of functional molecules and label adsorption, multi-shell labelled silica nanoparticles can be obtained. For example, the QD nanobeads with a triple-QD shell were obtained through a repeated PEI self-assembly followed by QD-adsorption.99 The presence of numerous QDs not only significantly enhanced the fluorescence signal but also provide sufficient carboxyl groups for subsequent antibody conjugation. The QD nanobeads with enhanced fluorescence signal markedly lowered the LoD of LFIA in clinical diagnostics. The LoD of QD nanobead-based LFIA for detecting SARS-CoV-2 and influenza A virus H1N1 was found to be 5 pg mL−1 and 50 pfu mL−1, respectively. Moreover, QD nanobead-based LFIA demonstrated exceptional accuracy and specificity when tested with throat swab samples. Its LoD was two orders of magnitude lower compared to the conventional colloidal Au-based LFIA.99
This layer-by-layer approach allows for facile co-assembly of various labels on the silica nanoparticles.46,101,102 For instance, using this method, a multilayered nanocomposite composed of SiO2–Au core and QD shell has been fabricated (Fig. 6A).46 This composite material enables powerful colorimetric and fluorescent dual-signal output for rapid and sensitive in situ monkeypox virus antigen determination. The detection limits for the colorimetric and fluorometric modes were 0.5 and 0.0021 ng mL−1, respectively. In the fluorometric mode, this method demonstrated a substantial enhancement in sensitivity for detecting the monkeypox virus, showing 238-fold and 3.3-fold improvements compared to the colloidal Au-based LFIA and ELISA methods, respectively.46 Dou et al. prepared the dye-doped silica nanobeads with intensified fluorescence intensity through the PEI and poly(sodium-p-styrenesulfonate)-mediated layer-by-layer self-assembly approach,103 The fluorescence signal of the resultant nanobeads (dyeing four times) was significantly enhanced compared to the once-dyed silica nanoparticles.103 The dye-doped silica nanobead-based LFIA realised the quantitative detection of hemagglutinin antigen of H7N2 and H7N9 avian influenza viruses (AIVs), in the linear range of 0.1–10 ng mL−1 and LoD of 0.08 pg mL−1.103
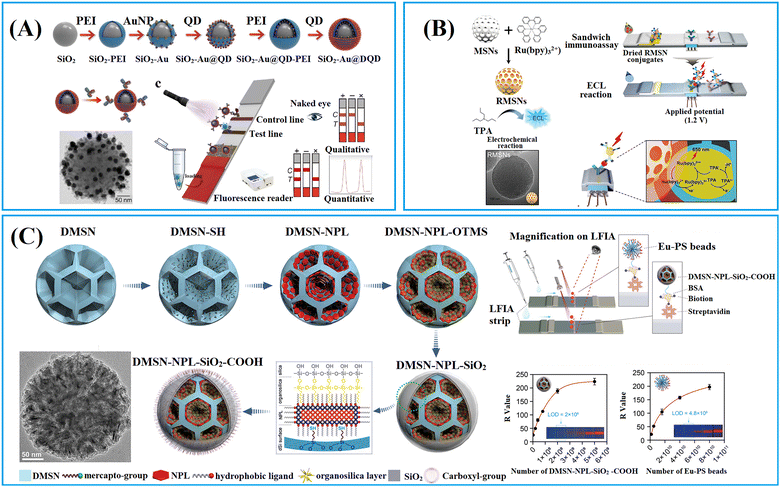 |
| Fig. 6 Strategies for enriching labels to silica nanoparticles. (A) The enrichment of labels on the surface of nonporous silica nanospheres by a layer-by-layer assembly approach. Reproduced from ref. 46 with open access from Springer Nature. (B) The enrichment of small molecules in small-pored silica nanoparticles. Reproduced with permission from ref. 104. Copyright 2020 John Wiley and Sons. The enrichment (C) nanomaterials in large-pored silica nanoparticles. Reproduced with permission from ref. 91. Copyright 2024 John Wiley and Sons. | |
The noble metal nanomaterials, such as Au,105–107 and Ag,108 can be formed in situ on the surface of silica nanoparticles. Typically, the process begins with mixing the silica nanoparticles with solutions containing seeds105–107 or ions108 to form the seed/ion-decorated silica nanoparticles. The noble metal shell is then formed through the additional reduction of noble metal ions. For example, Au seeds are initially decorated on the surface of silica nanoparticles to facilitate the subsequent growth of the Au–shell.107 During the Au–shell growth process, a growth solution is prepared, in which Au hydroxide is formed through the alkaline hydrolysis of chloroauric acid.107 Subsequently, a suspension of Au seed-coated nanoparticles, a reducing agent such as hydroxylamine hydrochloride, and polyvinylpyrrolidone (PVP) are sequentially added to the growth solution, leading to the reduction of Au hydroxide ions [Au(OH)4]− and the formation of Au shells.107 The resulting silica nanoparticles, adorned with abundant Au labels, have exhibited a LoD of 0.5 ng mL−1 in the detection of heart-type fatty acid binding protein.107
1.1.2.2. Small-pored silica nanoparticles.
Conventional mesoporous silica nanoparticles (MSNs) are a type of nanoparticles with pore size smaller than 10 nm,109 suitable for enrichment and signal amplification of small molecular dyes such as tris(2,2′-bipyridyl)ruthenium(II) (Ru(bpy)32+).104 As illustrated in Fig. 6B, Hong et al. have developed an electrochemiluminescent LFIA for detection of cTnI at low concentrations using Ru(bpy)32+-loaded MSNs as labels.109 In comparison to non-porous silica, MSNs reduced the LoD by two orders of magnitude. This improvement is attributed to the porous structure of MSNs, facilitating a more effective loading of Ru(bpy)32+, surpassing the loading capacity of non-porous silica by over 100 times. The Ru(bpy)32+-loaded MSNs exhibited strong electrochemiluminescent signals in reaction to tripropylamine, generating an electrochemiluminescent image that can be captured by a CCD camera for quantification analysis. The electrochemiluminescent LFIA based on Ru(bpy)32+-loaded MSNs enables the rapid detection of cTnI-spiked human serum within 20 min, achieving detection levels as low as 0.81 pg mL−1.109
1.1.2.3. Large-pored silica nanoparticles.
Innovative synthesis approaches have enabled the preparation of dendritic mesoporous silica nanoparticles (DMSNs) with a distinctive three-dimensional centre-radial pore structure, resulting in significant attention towards these DMSNs owing to their unique dendritic architecture.110 The DMSNs exhibited large open pore channels and a highly accessible internal surface, distinguishing them as promising nanocarriers for biomacromolecules90 and nanomaterials91,111–113 when compared to conventional MSNs.
Jung et al. designed DMSNs with a pore size of 13 nm capable of size-selective immobilisation of antibodies (recognition molecules) and enzymes (labels).90 The enzyme horseradish peroxidase (HRP) catalyses the oxidation of luminol using hydrogen peroxide to generate a product known as 3-aminophthalate, which can produce chemiluminescent signals emitting at a wavelength of 428 nm.90 Given that the pore size (13 nm) of DMSNs is smaller than that of anti-AIV antibodies (10 nm × 15 nm) but larger than HRP (3.0 × 6.5 × 7.5 nm3),114 the anti-AIV antibodies were initially conjugated onto the outer surface of DMSNs to enhancing binding to AIV antigens. Subsequently, HRPs were loaded into the pores of DMSNs to amplify the chemiluminescence signals. It is noteworthy that, despite having higher loading capacity for HRP and anti-AIV antibodies, the DMSNs with larger pore size (19.2 nm) do not exhibit the lowest LoD due to the anchoring of anti-AIV antibodies within the inner pores. Therefore, the DMSNs with a 13 nm pore size were identified as the optimal nanoparticles. The chemiluminescent-LFIA using optimal DMSNs loaded with HRP and anti-AIV antibodies as labels for the nucleoprotein of the H3N2 AIV virus demonstrated a LoD of 5 pM. For avian influenza H9N2, H1N1 and H5N9 viruses, the LoD values were 103.5, 102.5, and 104 50% egg infective dose (EID50) mL−1, respectively. These values are 20 to 100 times lower compared to a commercial AIV rapid test kit.90
DMSNs have been proven to effectively enrich various nanomaterial-based labels, including QDs,111,112,115,116 Au labels,117 carbon dots,113,118,119 and nanoplatelets.91 For example, fluorescent silica nanospheres with controlled nanoplatelets incorporation have been developed to enhance the performance of LFIAs.91 The synthesis method involved the controlled assembly of hydrophobic nanoplates into thiolated DMSNs through thiol–metal interaction, followed by the phase-transfer of the hydrophobic DMSN-nanoplate nanoassemblies through organosilica encapsulation and growth of the outer silica shell. The large open pore channels and highly accessible inner surface of DMSNs enabled the incorporation of flat/slit-shape nanoplatelets (24 nm in length, 11 nm in width and 4.4 nm in thickness) within DMSNs to amplify signal (Fig. 6C).91 This unique structure has been shown to significantly enhance the fluorescence intensity, achieving a 246-fold and 4-fold increase in brightness compared to a standalone nanoplate and commercial europium-doped polystyrene, respectively.91
By employing a repeated coordination-driven assembly and phase-transfer process, various functional units, such as Fe3O4 nanoparticles, QDs and Au labels can be readily co-assembled into DMSNs.120–123Fig. 7A shows plasmonic–fluorescent silica nanocomposite composed of DMSNs loaded with Au labels and red-light emitting QDs using this method.120 These nanocomposites were employed as the signal nanosphere in a CRISPR-based LFIA (Fig. 7B).121 In negative detection mode, Cas12a enzyme remains inactive, resulting in the capture of all signal nanospheres by the test line. The inner colour filter effect of Au and red-light emitting QDs in the signal nanospheres cause a change in colour from green to red. Conversely, in the context of positive detection, the presence of target amplicons activates the Cas12a enzyme, triggering the collateral trans-cleavage of digoxin–ssDNA–biotin. In this scenario, only a few signal nanoparticles emitting red-light will be captured at the test line, remaining green. The cleavage activity of Cas12a and colorimetric readout capability, the assay can achieve a rapid visual detection limit of 1 copy μL−1 for Salmonella typhimurium genomic DNA within 1 h, surpassing the sensitivity of commercial colorimetric LFIAs.121
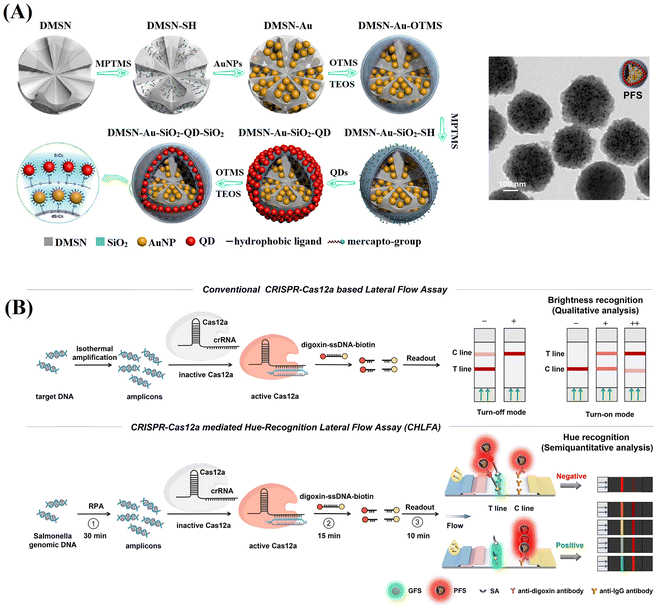 |
| Fig. 7 Schematic representation of the enrichment of various functional units co-assembled into DMSNs through the repeated coordination-driven assembly and phase-transfer process. (A) The fabrication of plasmonic-fluorescent silica nanospheres by loading DMSNs with Au labels and QDs through the repetition of the coordination-driven assembly and phase-transfer process. Reproduced from ref. 120, Copyright 2020 American Chemical Society. (B) Implementation of plasmonic-fluorescent silica nanospheres as the signal nanosphere in a CRISPR-based LFIA. Reprinted from ref. 121, Copyright 2024 American Chemical Society. | |
This section presents strategies for silica nanoparticles to enrich multiple labels for signal amplification. These labels can be adsorbed on the surface of the non-porous silica nanoparticles or embedded internally through layer-by-layer assembly and in situ synthesis. Selecting mesoporous nanoparticles with a matching pore structure based on the size of the label molecules or nanomaterials is another effective strategy to further enhance the concentration of label enrichment. Non-porous silica nanoparticles, known for their controllable particle size, excellent monodispersity and colloid stability, and ease of functionalisation, are widely used for label enrichment and LFIA signal amplification. Due to their limited surface area, non-porous silica nanoparticles have lower label loading capacity. In contrast, MSNs with a porous structure possess higher label loading capacity due to their larger surface area, significantly boosting the detection performance of LFIA. While conventional MSNs with pore size less than 10 nm are primarily used for the enrichment of small molecular labels, the DMSNs with a three-dimensional centre-radial pore structure excel in the enrichment of biomacromolecule and nanomaterial-based labels.
1.1.3. Magnetic nanoparticles.
Magnetic nanoparticles (MNPs), such as Fe3O4 nanoparticles,124–128 Fe3O4@silica nanoparticles,129–132 Fe3O4@MOF nanoparticles,48 and MnFe2O4 nanobeads,133 exhibit superparamagnetic properties that enable the magnetic enrichment of target analytes in a complex matrix when subjected to an external magnetic field.134 This feature streamlines LFIA operation following the magnetic enrichment of target analytes, thereby improving the detection performance significantly.135
A series of magnetic nanocomposite-based sensitive LFIA biosensors with amplified fluorescence, SERS, colorimetric and photothermal signals have been developed, such as the MNP@QD,124,129,132,133,136 MNP@SERS,125,128,137,138 MNP@nanozyme,48,127,135,139 and MNP@Au.140,141 Furthermore, Fe3O4 nanoparticles possess peroxidase-like properties and photothermal properties, offering advantages in enhancing the catalytic activity of Fe3O4@nanozyme48,135 and the photothermal effect of Fe3O4@Au140 respectively.
The PEI-mediated assembly approach is frequently utilized in the preparation of MNP@QD,124,133,136,142 MNP@SERS,125 and MNP@Au141 nanocomposites. For example, Wang et al. developed a high-performance MNP@QD nanocomposite with a triple-QD shell to decrease the LoD of LFIA.124 This nanocomposite consisted of an Fe3O4 core for magnetic separation and a triple-layer QD-shell containing numerous QDs for robust fluorescence signals. Upon conjugation with anti-SARS-CoV-2 spike antibodies/anti-nucleocapsid protein antibodies, the Fe3O4@QD was integrated into the LFIA system to detect SARS-CoV-2 spike and nucleocapsid protein antigens. The Fe3O4@QD-based LFIA offers two detection modes. The direct mode enables rapid screening of suspected samples in a time frame of 10 minutes, while the enrichment mode allows for quantitative analysis of SARS-CoV-2 antigens at low concentrations without interference from the “hook effect”. Owing to magnetic enrichment, the LoD for the two antigens in the enrichment mode was 0.5 pg mL−1 at a signal-to-noise ratio of 3, which is half as low as that in the direct mode.124 As illustrated in Fig. 8, multilayered magnetic-core dual-shell nanocomposites with precisely controlled nanogaps were engineered using a PEI-mediated layer-by-layer assembly process.125 The Fe3O4 core provided robust magnetic responsiveness, while the two layers Au@Ag satellites, separated by a 1 nm thick PEI interlayer, created narrow nanogaps and abundant hotspots. Furthermore, Raman reporters were integrated onto the surface of Au@Ag shells to enhance SERS signal. By modifying the nanocomposite with Raman reporter molecules and conjugating with four types of antibodies, the MNP@SERS nanocomposites could simultaneously detect four targets on two test lines with LoD reaching pg mL−1 levels. These detection limits were markedly lower than those of conventional Au-based LFIA and commercial ELISA kits, being at least 400 and 10 times lower, respectively.125
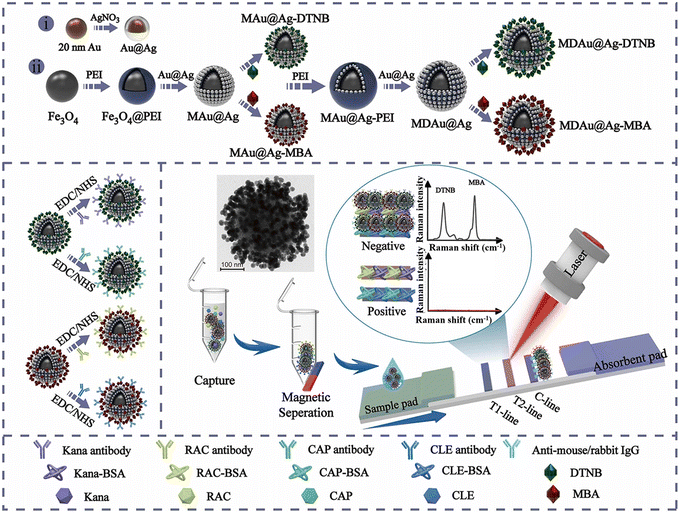 |
| Fig. 8 The enrichment of labels on the surface of MNPs by a PEI-mediated assembly approach. Reproduced from ref. 125 with open access from Elsevier. | |
The in situ growth method has been used to prepare MNP@SERS,128,137,138 MNP@nanozyme,48,127,135,139 and MNP@Au140 nanocomposites. For instance, Fe3O4@Pt nanocomposites135 were synthesised by reducing chloroplatinic acid onto Fe3O4 nanoparticles using sodium citrate. The magnetic properties of Fe3O4 nanoparticles enabled magnetic enrichment in liquid samples, whereas the synergistic catalytic properties of Fe3O4 nanoparticles and Pt satellites allowed for colorimetric signal amplification through enzyme-like reactions. The integration of Fe3O4@Pt nanocomposites in LFIA strips led to a two-order-of-magnitude reduction in LoD compared to conventional LFIA based on colloidal Au.135
Increasing the loading density of Pt labels reduced the saturation magnetization of Fe3O4 nanocomposites owing to the intrinsic magnetic shielding effect of noble metal components.48,128 In this context, an iron-based metal−organic framework (MOF) (MIL-100) with a thickness of 40 nm was utilized as a spacer layer to preserve the magnetism of Fe3O4 nanoparticles. The MIL-100 can accommodate Pt labels and improve the catalytic performance through the intrinsic peroxidase-like activity (Fig. 9).48 The resultant Fe3O4@MOF@Pt nanocomposites achieved enhanced colorimetric signal brightness, rapid magnetic response, and ultrahigh peroxidase-mimicking activity, contributing to the enhancement of the sensitivity of LFIA. Integration of the nanocomposites with the dual-antibody sandwich LFIA platform enabled the detection of procalcitonin with remarkable sensitivity of 0.5 pg mL−1. This level is approximately 2280 times greater than that of conventional Au-based LFIAs and surpasses previously reported immunoassays.48
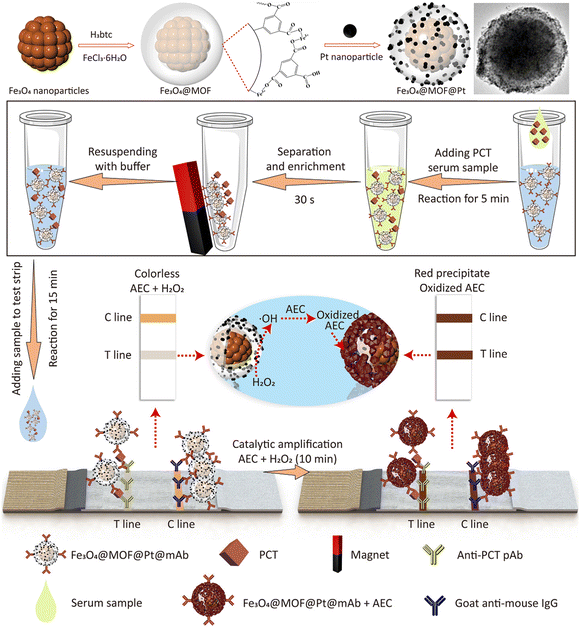 |
| Fig. 9 The enrichment of labels on MNPs by an in situ growth method. Reproduced from ref. 48, Copyright 2022 American Chemical Society. | |
Enrichment of labels onto MNPs can be achieved through chemical adsorption.129 Typically, the Fe3O4 nanoparticles are coated with a silica shell and amino functionalised using the Stöber method, followed by binding with carboxylated QDs using the EDC/NHS method.129 A multifunctional nanocomposite was synthesized, consisting of an Fe3O4 core with diameter of 160 nm, a SiO2 shell with thickness of 40 nm, and numerous small QD satellites.129 Incorporating MNPs into QD-based LFIAs enhanced the precision and anti-interference capability in quantitative analysis, as target analytes can be rapidly enriched from complex samples under the influence of an external magnetic field. The LoD was determined to be 1.2 × 103 copy mL−1, addressing the need for rapid and accurate adenovirus detection.129
In summary, the previously discussed MNP-label nanocomposites possess a core@shell structure, utilizing MNPs both as a magnetic separation tool and a stable nanocarrier for enriching diverse labels (e.g. QD, SERS labels, nanozyme, and Au labels). The assembly techniques predominantly employed for the preparation of MNP@QD and MNP@noble metals are the PEI-mediated assembly method and the in situ growth method. It is crucial to carefully select the reagent in the in situ growth method to ensure uniform plasmonic label coating. Compared to polymeric nanoparticles and silica nanoparticles, MNPs with intrinsic superparamagnetic properties enable direct operation of MNP-based LFIA after the magnetic enrichment of target analytes, which enhances the detection performance. It is essential to minimize the inherent magnetic shielding effect of noble metal components to preserve the saturation magnetization of Fe3O4 nanocomposites. Additionally, MNPs typically feature a nonporous structure, limiting label enrichment solely to the surface of the MNPs. In contrast, porous silica nanoparticles facilitate widespread label enrichment within their pores. Furthermore, polymeric nanoparticles can encapsulate numerous labels through the self-assembly of amphiphilic polymers in selective solvents, leading to a higher label loading capacity compared to MNPs without increasing particle size. While polymeric nanoparticles and silica nanoparticles boast exceptional optical clarity due to their amorphous nature, MNPs (particularly Fe3O4 nanoparticles) have a high absorbance index. The high absorbance index causes MNPs to absorb the fluorescence signal of QDs and diminishes the signal intensity of the MNP@QD nanocomposite.
1.1.4. Other types of nanoparticles.
In addition to polymeric nanoparticles, silica nanoparticles and MNPs, other types of nanoparticles including gold nanoparticles (AuNPs),143–147 MOFs,148–151 and carbon-based nanoparticles152,153 have also been employed for both label enrichment and LFIA signal amplification.
1.1.4.1. AuNPs.
AuNPs can serve as label materials for colorimetric assays and as a carrier for the enrichment of enzymes (e.g., horseradish peroxidase (HRP),154,155 polyHRP143 and nanozyme144) and luminescent labels (e.g., dyes145–147 and bimetallic nanoclusters156) in chemiluminescent, fluorescent, colorimetric and electrochemiluminescent LFIAs. The immobilisation of polyHRP onto AuNPs led to a significant increase in HRP content per AuNP compared to HRP alone, approximately 15.7-fold higher.143 The chemiluminescent signal generated by polyHRP-loaded AuNPs was 155-fold higher than that of HRP-loaded AuNPs. Integration of a time-programmable amplification step to the polyHRP-loaded AuNP-based LFIA platform enabled automation of the sequential enzyme/chemical reactions in the immunoassay and signal amplification. Using the developed LFIA platform, the LoD for cTnI was 0.84 pg mL−1, representing a 595-fold improvement over colloidal Au-based LFIA.143
AuNPs exhibit distinct surface plasmon resonance properties that have garnered significant attention over the decades. The effect, known as the fluorescence enhancement, based on surface plasmon resonance, is currently employed in enhancing LIFA signals. Fig. 10 depicts a plasmonic fluor fabricated with a core of gold nanorod (GNR), a mesoporous silica (mSiO2) shell, and a cyanine 5 (Cy5) fluorophore.147 The choice of GNRs as the core was due to their two distinct surface plasmon resonances transverse and longitudinal, which culminate in a robust plasmonic resonance compared to gold nanospheres. Cy5 was selected for its high spectral overlap with GNRs. The 10.3 nm mSiO2 shell not only encapsulates a considerable quantity of the Cy5 fluorescent material but also maintains an optimal distance between Cy5 and the GNRs. This step significantly amplified the fluorescence of Cy5 through plasmonic coupling between the GNRs and Cy5 in proximity, typically within 5–90 nm. The proposed plasmonic fluor LFIA facilitated sensitive detection of influenza A virus nucleocapsid protein with a remarkable LoD of 0.52 pg mL−1 within 20 min. Furthermore, it exhibited exceptional specificity and accuracy in analysing clinical samples for the influenza A virus.147
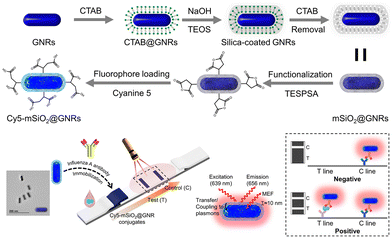 |
| Fig. 10 The use of AuNPs for labels enrichment and LFIA signal amplification. Reproduced with permission from ref. 147. Copyright 2023 American Chemical Society. | |
1.1.4.2. MOFs.
MOFs, a class of crystalline porous material composed of metal ions and organic ligands, have characteristics, such as large surface areas, facile synthesis approaches, abundant functional groups, and chemical stability.157 They have found extensive applications in biosensing,157–159 catalysis,160,161 and energy storage.162 However, the use of MOFs for label enrichment and LFIA signal amplification is still at an early stage.148–151,163,164 For example, the colorimetric catalytic nanocomposite in Fig. 11 utilized a multi-shell porous zeolitic imidazolate framework-8 (ZIF-8) to immobilize HRP, exhibiting commendable loading efficiency, catalytic activities, and signal luminosity.148 This structure fostered more cavities for enzyme sequestration and facilitated an effective diffusion pathway for catalytic substrates. Further augmentation of the nanocomposite surface with a polydopamine (PDA) layer amplified the signal brightness and proffered a flexible tethering site for HRP immobilization, thereby escalating the enzyme quantity efficiently. This ZIF-based LFIA platform achieved an ultrasensitive assay for cTnI with a post-catalytic naked-eye colorimetric detection limit of an impressive 0.01 ng mL−1, which was 100-fold higher than its counterpart Au@PDA-based LFIA.148 Zirconium-based MOFs have been reported to facilitate the in situ growth of the MOF–Au nanocomposite.164 Compared to the traditional colloidal Au-based LFIA, the LoD of MOF–Au-labelled LFIA for human chorionic gonadotropin was reduced by 10.6 times.
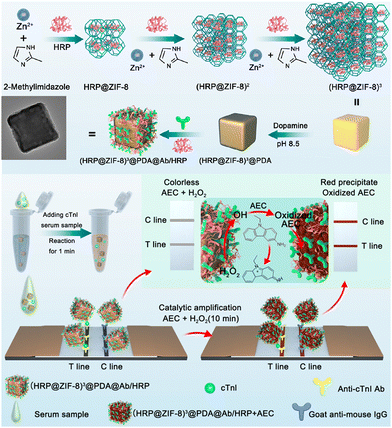 |
| Fig. 11 The use of MOF for labels enrichment and LFIA signal amplification. Reproduced with permission from ref. 148. Copyright 2023 American Chemical Society. | |
1.1.4.3. Carbon nanomaterials.
Carbon nanomaterials, known for their distinct properties, such as excellent chemical stability, large surface area and cost-effective synthesis, are ideal nanoparticles for label enrichment.152,153,165 Among these, the graphite-like g-C3N4 nanosheets with large surface areas have been applied to enrich abundant Au nanomaterials on their surface to amplify signals and improve the performance of g-C3N4–Au-based LFIA.153 The LoD of g-C3N4–Au-based LFIA for 17β-estradiol in foods was 0.5 ng mL−1, exhibiting a three-fold enhancement in analytical sensitivity compared to colloidal Au-based LFIA.153 A three-dimensional membrane-like SERS nanocomposite based on graphene oxide (GO) has been incorporated into LFIA for the sensitive detection of various pathogens.152 The process, as illustrated in Fig. 12, initiates by coating the GO sheet with a positively charged PEI layer to attract small Au seeds (3 nm). Subsequently, the Au shell was coated on the surface of the GO@Au seed nanosheet using hydroxylamine hydrochloride to reduce chloroauric acid. Then, a 0.5 nm PEI layer was applied to the synthesized GO@Au surface to act as an interlayer. This interlayer was instrumental in creating a precise nanogap to load Raman dye molecules and Ag satellites via electrostatic interaction. The process generated numerous SERS hotspots and offered a multitude of surface sites for bacterial adhesion. By integrating two different Raman reporter molecules with non-intersecting Raman peaks into GO@Au@Ag, and conjugating them with four antibodies, the biosensor facilitates the concurrent identification of four pathogens at dual test lines. The GO@Au@Ag nanostickers are highly effective at adhering to the bacteria surface, yielding stronger SERS signals and aiding in the mobility of bacterial nanocomplexes. This, in turn, leads to an improvement in the sensitivity of LFIA for bacteria detection. This biosensor can accurately quantify four distinct types of bacteria in actual clinical samples, achieving a minimal detection limit of 9 cells mL−1.152
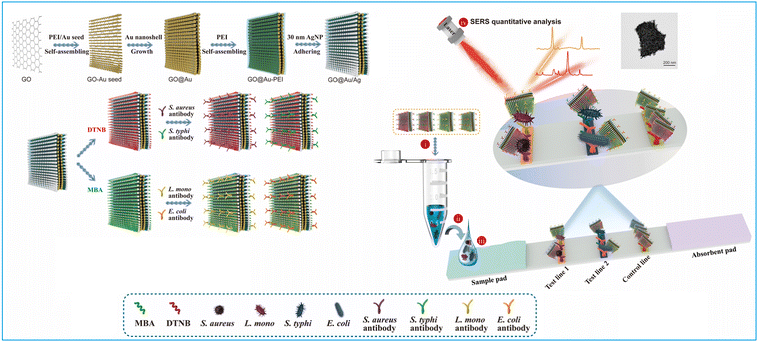 |
| Fig. 12 The use of graphene oxide nanosheets for labels enrichment and LFIA signal amplification. Reproduced with permission from ref. 152. Copyright 2022 Elsevier. | |
Table 1 summarises the advantages and limitations of different nanoparticles applied in LIFA signal amplification. Au nanomaterials have been extensively studied not only as labels of LFIA but also as nanocarriers for label enrichment and LFIA signal amplification due to their ease of preparation and conjugation, mass production capacity, and uniform particle size. Smaller AuNPs (<50 nm) exhibit reduced non-specific binding compared to other types of nanoparticles, although they have a lower loading capacity (∼10 times less) for labels. These smaller AuNPs also offer faster migration speed, allowing for a 15-minute one-step assay, considerably quicker than 50-minute two-step assay required for larger silica nanoparticles (200 nm). The plasmonic properties of AuNPs enable them to boost the fluorescence of fluorophores. MOFs with high surface areas and abundant functional groups are suitable for label enrichment, but their water instability poses a significant challenge when applied to LFIA signal amplification for detecting target analytes in serum or urine samples. Two-dimensional carbon nanomaterials with expansive surface areas and cost-effectiveness are promising for label enrichment, however their nonuniform size distribution may impair the reproducibility of the assays.
Table 1 Summary of advantages and limitations of different nanoparticles
Nanoparticles |
Advantages |
Limitations |
Ref. |
Polymer nanoparticles |
Embedding into pre-synthesized nanoparticles |
Excellent optical clarity |
Very easy operation, uniform size distribution and good reproducibility |
Label distribution inhomogeneity problem |
Limited label loading capacity of nano-sized labels |
59
|
Incorporating in an emulsion assembly process |
Easy operation, high label loading capacity and relatively controllable assembly process |
Nonuniform size distribution, and low reproducibility |
77
|
Incorporating in the polymerization process |
Medium label loading capacity and uniform size distribution |
Carefully controlled operation condition |
78 and 80
|
Silica nanoparticles |
Nonporous |
Excellent optical clarity, ease-of-functionalisation, very controllable assembly process |
Excellent colloidal stability |
Tedious operation process |
Limited label loading capacity |
46
|
Small-pored |
Suitable for molecular-sized labels |
Not very suitable for nano-sized labels |
109
|
Large-pored |
Very high label loading capacity |
|
91
|
MNPs |
Magnetic enrichment of target analytes, catalytic and photothermal properties |
Tedious operation process, limited label loading capacity and high absorbance index |
129 and 140
|
AuNPs |
Ease-of-preparation and conjugation, mass production capacity, uniform particle size, less non-specific binding, catalytic and plasmonic properties |
Limited label loading capacity and high absorbance index |
144 and 147
|
MOFs |
High label loading capacity and ease-of-functionalisation |
Water instability |
148
|
Carbon nanomaterials |
High label loading capacity and low cost |
Nonuniform size distribution |
152
|
1.2. Engineering nanoparticles for LFIA signal amplification
LFIA integrates the physical, chemical, and optical properties of label materials, with their transport dynamics, reaction kinetics and signal generation all playing significant roles in determining their performance within LFIA applications. The previous section demonstrated that the enrichment of labels on a single nanocarrier to form nanoparticle-label composites is an effective strategy for enhancing signal intensity. However, the size and surface chemical properties of these composite nano-labelling materials greatly influence the transport dynamics, reaction kinetics, and signal generation in LFIA. For example, particle size simultaneously impacts the label loading capacity (signal generation) and diffusion rate (transport dynamics).166 Moreover, surface chemistry greatly affects its water dispersibility (transport dynamics), antibody-antigen interaction (reaction kinetics) and label signal intensity (signal generation).66,115,167 The impact of size and surface chemistry of nanoparticle/resultant nanocomposite on the LFIA performance is complex. Therefore, a thorough comprehension of the relationship between the physicochemical properties of nanoparticles and their performance in LFIA signal amplification would facilitate the rational design of nanoparticle-label composites applied in LFIA. This section will delve into the impact of nanoparticle size and surface chemistry on their performance in LFIA applications.
1.2.1. Size-controlled signal amplification strategies in LFIA.
The size of a nanoparticle-label nanocomposite is a pivotal factor determining its performance in LFIA, impacting the signal generation, transport dynamics, and reaction kinetics of the nanocomposite in LFIA.72,83,116,166,168 Increasing the particle size enhances the loading capacity and binding affinity of nanocomposites due to a larger surface area, allowing for more labels and antibodies to be accommodated. However, based on the Stokes–Einstein equation, larger nanoparticles exhibited decreased diffusion rates, resulting in fewer nanoparticles binding to the antibodies immobilised on the NC pore walls. Therefore, achieving optimal performance in LFIA requires a delicate balance between signal strength and diffusion dynamics.
1.2.1.1. The impact on signal intensity.
The signal intensity of a nanoparticle-label nanocomposite typically correlates positively with its particle size, due to the increased surface area of individual nanoparticles, enhancing their capacity for label loading. For example, the colorimetric and fluorescence signals of nanobeads loaded with Au labels,68,70,72 QDs,116,166 and AIEgens83 enhance significantly as their particle size increases. In Fig. 13A, the optical density of Au-embedded nanobeads increases by over 10-fold as the size grows from 100 to 400 nm.72 These Au-embedded nanobeads were prepared using an emulsion assembly strategy.72 Within the emulsion system (oil-in-water), adjustment of surfactant concentration, oil-to-water ratio, and ultrasonic power enables facile control of particle size. Generally, an increase in surfactant concentration or oil-to-water ratio leads to particle size reduction, while higher ultrasonic power results in size increase.72 Similarly, the fluorescence intensity of AIEgen-embedded polymeric nanobeads escalates as their size expands.83 This mechanism also applies to nanoparticle-label composites using large-pore nanoparticles as a carrier. As shown in Fig. 13B, the fluorescence intensity of the DMSN-QD nanocomposite amplifies by over 30-fold as the DMSN size increases from 138 to 471 nm.116 Monodispersed DMSNs were synthesized using an anion-assisted approach169 with sodium salicylate as the structure-directing agent. Control over the size of DMSNs can be easily achieved by adjusting the amount of sodium salicylate and ethanol,116 improving the surface area of individual particles and label loading capacity.
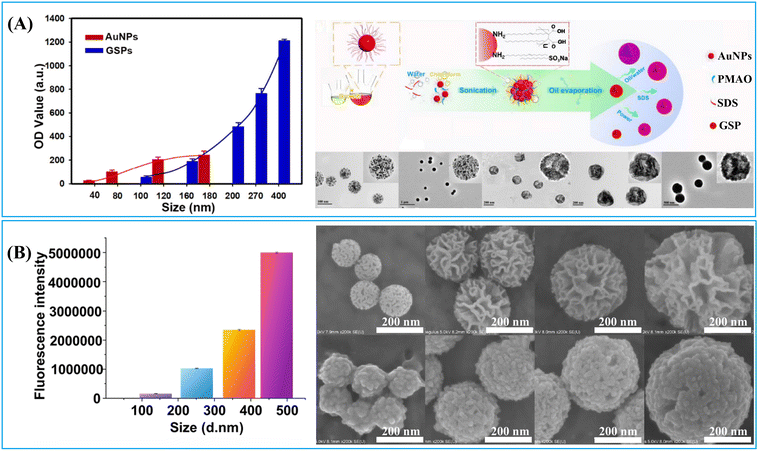 |
| Fig. 13 The impact of particle size on its signal generation and size-controlled synthesis of nanoparticle-label nanocomposite. (A) The optical density of Au-embedded nanobeads increases with particle size and schematic illustration of size control of nanobeads loaded with Au labels in a self-assembly process via altering surfactant concentrations, the volume ratio of chloroform/water and the power of sonication. Reproduced from ref. 72, with open access from Ivyspring International Publisher. (B) The fluorescence intensity of DMSN-QD nanobeads increases with particle size and SEM images of 4-sized DMSN-QD nanocomposites and DMSNs. Reproduced with permission from ref. 116. Copyright 2021 John Wiley and Sons. | |
1.2.1.2. The impact on binding quantity.
Since LFIA comprises a chromatographic system and immunochemical reactions, the nanocomposite must first reach the test line and then interacts with the pre-immobilised antibodies.50,170 In a typical sandwich LFIA system labelled with colloidal Au, the diffusion, advection and antibody–antigen reaction of the gold label collectively affect its binding efficiency at the test line (Fig. 14A).168 Exploring the kinetics of transport and reaction is a pivotal step in LFIA development and is essential for improving the LFIA performance.17 The kinetics of transport and reaction can be defined by dimensionless numbers, namely the Péclet number (Pe) and Damköhler number (Da).168,171 Pe describes the ratio of advective flux to diffusive flux, while the Da depicts the relative rates of reaction to diffusion.168 The theoretical calculation is outlined as follows:171 | 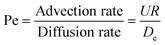 | (1) |
where U denotes the convective velocity (∼0.3 mm s−1 for CN140 membrane), R (8 μm) signifies the average radius of cylindrical pores from NC membrane, and De is the effective diffusivity in the NC membrane. De can be calculated by the following formula:In this context, D stands for the diffusivity of label in solution which can be determined using the Stokes–Einstein equation:kB represents the Boltzmann constant, T is solution temperature, μ is the solution viscosity, and r is the hydraulic radius of the label.171ϕ (0.85) is the membrane porosity and τ (∼1) is the tortuosity of the membrane.172 According to the above equations, Pe increases as the particle size increases. A calculated Pe ≫ 1 suggests that advection timescales are significantly shorter than those of diffusion within an LFIA, making diffusion the rate-limiting step in label transport to the test line. | 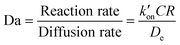 | (4) |
Meanwhile, Da can be determined by eqn (4), where
is effective forward reaction rate constant, C is surface density of antibodies at the test line.171
can be scaled as n·kon, where kon is forward reaction constant for individual antibody–antigen interaction in the NC membrane (∼7.26 × 102 M−1 s−1),171 and n reflects the effective number of antibodies that bind the test line per label, which can be estimated by dividing the label surface area (4πR2) by the IgG docking area (166 nm2).171 Based on the above equations, Da increases as the particle size increases. The calculated Da ≪ 1 indicates that diffusion timescales are much shorter than those of reaction within an LFIA. Therefore, reaction is the rate-limiting step in the binding of label at the test line.
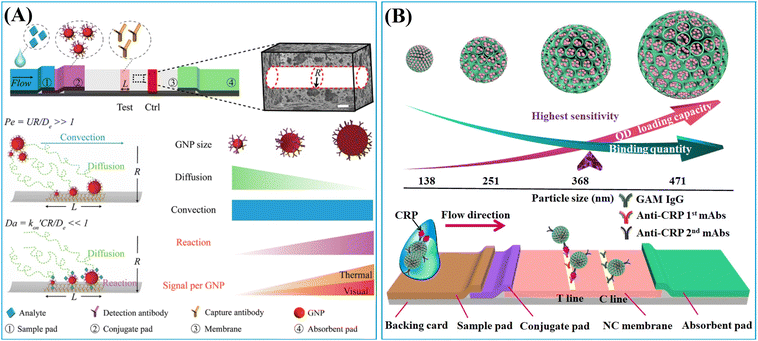 |
| Fig. 14 (A) Architecture of LFIA, assuming that the NC membrane is conceptually simplified as bundles of cylindrical pores with radius R; scale bar is 5 μm. Pe is the ratio of diffusion time to convection time of a colloidal Au label, where Pe ≫ 1 in LFIA implies the transport of the colloidal Au label to the test line is diffusion-limited, and Da is the ratio of reaction flux to diffusion flux, where Da ≪ 1 in LFIA implies the capture of the colloidal Au label at the test line is reaction-limited. Reprinted with permission from ref. 171. Copyright 2017 American Chemical Society. (B) The QD loading capacity and binding quantity of DMSN-QD as a function of their particle size, and the scheme of DMSN-QD-based LFIA test strip for the detection of CRP. Reprinted with permission from ref. 116. Copyright 2021 John Wiley and Sons. | |
For colloidal Au labels with size less than 100 nm, Pe ≫ 1 and Da ≪ 1 indicate that the reaction ultimately restricts the binding of label at the test line (Fig. 14A). Considering that the small-size Au labels (size ≤100 nm) do not settle before reaching the test line, a larger Au label can improve the binding efficiency due to increased n·kon associated with a larger surface area.171 When maintaining the same label concentration at various sizes, the binding quantity of a larger size label at the test line increases. In this context, the increase in particle size (size ≤100 nm) simultaneously heightens the signal intensity and the binding quantity of the label at the test line, thereby improving the detection performance of LFIA.171
In contrast, the nanoparticle-label composite typically has a size exceeding 100 nm, prompting a re-evaluation of the correlation between the binding quantity and the particle size.72,116,166 The particle size increment may lead to a transition of Da(
CR/De) to over 1 at a certain point as
enlarges and De diminishes with increasing particle size, shifting the rate-limiting step of nanocomposite binding from reaction to diffusion. In cases where Da ≫ 1, further enlarging nanocomposite size decreases its binding efficiency due to slower diffusion (Fig. 14B). According to the colloid filtration theory,173 the binding efficiency influenced by diffusion (ηB) can be determined as 0.487As1/3Pe−0.552. As depends on membrane porosity:
| As = 2(1 − γ5)/(2 − 3γ + 3γ5 − 2γ6) | (5) |
where
γ = (1 −
ϕ)
1/3. In addition, increased
n for large nanocomposite (
e.g. 400 nm) elevates the risk of non-specific interactions between antibodies on nanocomposite surface and pre-immobilised antibodies on the LFIA membrane, potentially causing false positives.
72,116,166 Thus, decreasing the concentration of large nanocomposite is essential to mitigate false positives. In this context, the binding quantity of larger size nanocomposite at the test line decreases as both the binding efficiency and concentration decrease with growing particle size. Consequently, the ultimate LFIA performance hinges on the signal intensity and binding quantity of nanocomposites at test line, with contrasting tendencies relative to particle size (
Fig. 14B). The optimal LFIA performance is achieved by utilizing a medium-sized nanocomposite with a balanced signal intensity and binding quantity.
72,116,166
1.2.2. Surface chemistry-controlled signal amplification strategies in LFIA.
To effectively develop the LFIA platform for detecting low-concentration biomarkers, it is essential that the nanocomposite possess high signal intensity, excellent water dispersibility and strong interaction efficiency and specificity.115,117,167 In this regard, the surface chemistry of the nanoparticles must be meticulously engineered to meet these criteria and accurately indicate biomolecular recognition events with high precision and a low LoD.174,175 This section reviews the key role of surface chemistry of nanoparticles in LFIA optimization.
1.2.2.1. The impact on signal intensity.
The signal intensity of a nanoparticle-label composite depends on the loading capacity of the nanoparticles and the preservation of label signals. As discussed above, labels can be enriched to nanoparticles through physical adsorption or chemical adsorption. Physical adsorption is primarily driven by hydrophobic–hydrophobic interactions,57,58,61 and electrostatic attractions.46,94 Hydrophobic–hydrophobic interactions predominantly occur between the alkyl chains (e.g. oleylamine,72 octadecylamine,66 oleic acid,69 and trioctylphosphine oxide58) of hydrophobic labels such as QDs and Au labels, and the hydrophobic framework of polymeric nanoparticles consisting of amphiphilic molecules or polystyrene. These interactions also occur between the aromatic functionalities of hydrophobic molecule labels (e.g. Nile red,61 AIEgens,57,59 Eu(III) chelates,47 and the hydrophobic polystyrene). For example, polystyrene, with its hydrophobic chains and steric phenyl rings, forms strong interactions with the aromatic phenyl rings of AIEgen, limiting intramolecular movements and enhancing AIEgen fluorescence considerably (Fig. 15A). These hydrophobic interactions take place between the native surface of the nanoparticle and the label, requiring no post-modification of the nanoparticles.
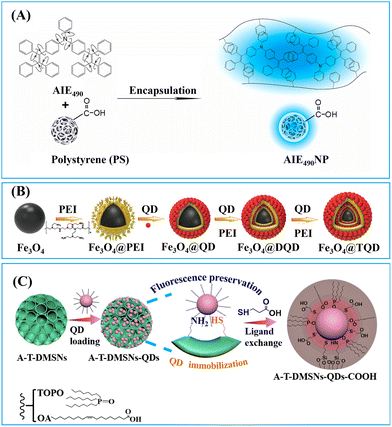 |
| Fig. 15 Surface chemistry of nanoparticles for label enrichment. (A) The hydrophobic–hydrophobic interactions between AIEgens and polystyrene enhance AIEgen fluorescence by restraining intramolecular motions. Reproduced with permission from ref. 57, Copyright 2022 Elsevier. (B) Surface coating of PEI to promote enhanced electrostatic interactions. Reproduced with permission from ref. 124. Copyright 2021 American Chemical Society. (C) Amino- and thiol-modified DMSNs for QD loading and signal preservation. Reproduced with permission from ref. 115. Copyright 2021 American Chemical Society. | |
Enzyme adsorption (e.g. artificial miniaturized peroxidase144) on the surface of AuNPs involves hydrophobic and/or electrostatic interactions. In cases of electrostatic interactions, PEI, a cationic polymer with a high positive charge density, is frequently utilised to coat the surface of nanoparticles (e.g. MNPs,124,125 AuNPs156 and nonporous silica nanoparticles46,98) to enhance label loading efficiency and maintain nanostructure stability in solution. The PEI-mediated layer-by-layer coating strategy can greatly improve loading efficiency (Fig. 15B).124,142 Furthermore, PEI coating can prevent nanoparticle aggregation.
The nanoparticle-label integration can be achieved through covalent bonding.80,113,129 The incorporation of labels with vinyl groups80,83 into polymeric nanoparticles can be achieved through alkene addition polymerization. The surface modification of nanoparticles with amino129 and thiol groups176 represents an effective strategy for enhancing label enrichment. Amines can be coupled with carboxyl groups of labels through carbodiimide-mediated EDC condensation,129–131 while thiols can coordinate with metal labels.96,111,116,176Fig. 15C shows amino and thiol commodified DMSNs for QD enrichment and fluorescence preservation. Amino groups contribute to QD fluorescence preservation through amino-based surface passivation, while thiol groups increase the loading capacity of QDs due to the thiol–metal coordination.
Notably, among these strategies, the hydrophobic functionality,57,59 PEI coating46,124,125 and thiol modification strategies96,111,116,176 emerge as the top three surface chemistry methods for the preparation of nanocomposite with high label loading, uniform label distribution and consistent size distribution.
1.2.2.2. The impact on binding quantity.
The binding quantity of nanocomposite at test line is greatly affected by its water-dispersity. Carboxylation emerges as the most promising strategy to enhance its water-dispersity. Currently, nearly all the commercially available fluorescent polystyrene spheres used in LFIA are carboxyl-modified.55,177,178 This modification is attributed not only to the ease of conjugating carboxyl group with antibodies through the EDC method,175 but also to their pKa of approximately 4,179 facilitating deprotonation in water and enhancing the nanocomposite dispersibility.176 Various approaches have been devised to introduce the carboxyl groups onto the outer surface of nanocomposite. For example, the carboxyl groups can be incorporated by using monomers containing carboxyl or acid anhydride functionalities (e.g., acrylic acid,47,78 methacrylic acid,80 methyl methacrylate,82 and maleic anhydride83) during the polymerisation process. Additionally, the maleic anhydride in an amphiphilic copolymer like poly (styrene-co-maleic anhydride)69 or polymer PMAO67,77 that is utilised in the emulsion assembly strategy can also contribute carboxyl groups to nanocomposites. Besides, the saline chemistry enables the facile surface functionalisation of the carboxyl groups. Typically, this involves initially coating a silica shell for the subsequent carboxyl modification. The carboxyl groups can be directly grafted on the silica surface by using 3-triethoxysilylpropyl succinic anhydride,147,180 or by converting the amino groups into carboxyl groups through reactions with the succinic anhydride111,176 or polyacrylic acid.167
The efficiency and specificity of the antibodies attached to the nanocomposites in recognizing the target analyte affects the amount of binding of the nanocomposites on the test line. The oriented immobilization of the antibodies on the nanocomposite surface played a pivotal role in improving the reaction efficiency and specificity. The accessibility of antigen binding sites (Fab fragment) determines the efficiency of antibody–antigen binding. A nucleophilic addition reaction between the hydrazide group of the nanocomposites and the aldehyde group of the Fc region of the antibodies enables the specific attachment of antibodies to the surface of the nanocomposites (Fig. 16A). Compared to the carbodiimide method, this hydrazide-mediated oriented coupling strategy reduced the LoD by approximately fourfold in the detection of the hepatitis B surface antigen.66
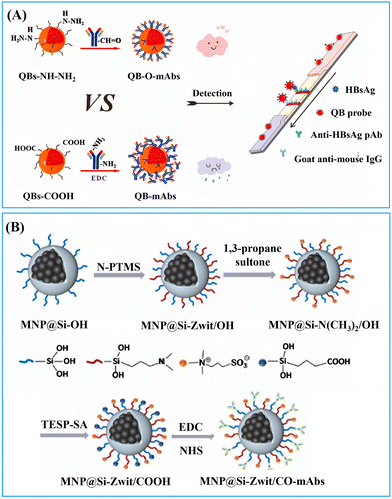 |
| Fig. 16 Strategies for enhancing reaction efficiency and specificity. (A) The hydrazide mediated oriented coupling strategy. Reprinted with permission from ref. 66. Copyright 2021 Elsevier. (B) Utilization of zwitterionic ligands to reduce nonspecific binding. Reproduced with permission from ref. 180. Copyright 2024 Elsevier. | |
Effective surface blocking of antibodies also benefits the reaction efficiency and specificity. The incorporation of zwitterionic ligands, which simultaneously possess positive and negative charges, leading to high hydration capacity and antifouling properties, can further reduce nonspecific binding. Fig. 16B depicts the introduction of zwitterionic groups through a nucleophilic addition reaction using 1,3-propanesultone following the co-hydrolysis of the silica precursor N,N-diethyl-3-(trimethoxysilyl) propylamine and tetraethyl orthosilicate. The zwitterionic groups effectively prevent nonspecific adhesion. Moreover, antibody-modified nanocomposites are usually blocked with 1–10% (w/v) bovine serum albumin (BSA) to prevent undesired non-specific binding.58,181 Alternative blocking regents include other protein products (e.g. casein131 and skim milk68) and polymers (e.g. PEG 20
000156 and PEG-400144). Additionally, quenching reagents such as glycine109 and ethanolamine,182 along with Tween-20,135 are often combined with BSA to enhance blocking performance.
In general, surface chemistry plays a crucial role in determining the binding quantity of nanocomposites by influencing water dispersibility, reaction efficiency and specificity. Carboxylation acts as a fundamental strategy to enhance water dispersibility, while the oriented immobilization of antibodies and effective surface blocking techniques are pivotal in improving reaction efficiency and specificity. The integration of recognition antibodies onto nanocomposites can be achieved through noncovalent or covalent bonding, with the latter providing control over orientation and stability. Furthermore, the introduction zwitterionic ligands and the use of agents to block antibody-modified nanocomposites can effectively reduce nonspecific binding.
2. Conclusion and perspectives
The utilization of nanocomposites for signal amplification in LFIA detection systems represents a significant advancement in the field of modern biosensing. Nanocomposites possess unique optical, electronic, catalytic, and magnetic properties that can markedly enhance detection sensitivity and specificity. This review delves into the engineering of nanoparticles for LFIA signal amplification, emphasizing the importance of comprehensively understanding the relationship between the physicochemical properties of nanoparticles and their performance in LFIA applications. The advantages and potentials of these nanoparticle-assisted strategies in LFIA can be summarized into five aspects: (1) high sensitivity: nanocomposites amplify detection signals, enabling the identification of analytes at lower concentrations. (2) Multifunctionality: certain nanoparticles can be employed for both signal amplification and target pre-concentration. (3) Diverse signal outputs: nanocomposites offer various signal outputs, such as optical, and electrochemical signals, and (4) quantitative detection: through integration with digital health technologies, highly sensitive nanoparticle assisted LFIA can offer more precise quantitative analyses, whereas traditional lateral flow strips only provide qualitative or semi-quantitative results.
Despite the numerous advantages of nanoscale signal amplification technologies, its application continues to encounter various limitations and challenges to be urgently addressed, including the following.
2.1. Balancing sensitivity and specificity
While enhancing sensitivity, precautions must be taken to avoid increasing the risk of false positives.
2.2. Stability
Nanocomposites may aggregate or degrade over time due to prolonged storage or extreme environmental conditions, affecting their functionality. Therefore, there is a need to develop more stable nanocomposites and optimize storage conditions.
2.3. Batch consistency
Maintaining high reproducibility and consistency in the preparation of nanocomposites is crucial to ensure the accuracy of detection results.
2.4. Ease of operation
The use of nanocomposites should not overly complicate the detection process, as it could impact the user-friendliness of LFIA detection systems.
2.5. Cost-effectiveness
Nanocomposites may elevate testing costs, necessitating the development of cost-efficient synthetic methods.
With the development of new materials and technologies, it is believed that addressing these limitations and fully harnessing the advantages of nanocomposites could lead the LFIA to achieve greater breakthroughs in sensitivity, stability, and applicability in the future.
Author contributions
FG and ZG conceptualized and wrote the manuscript. SY created the schemes and figures. LH provided oversight and made essential revisions. All authors reviewed and endorsed the final version of the manuscript.
Conflicts of interest
There are no conflicts to declare.
Acknowledgements
This work was supported by the National Natural Science Foundation of China (Grant no. 82372148) and the National Key Research and Development Program of China (no. 2021YFF0703500 and 2022YFE0103500).
References
- J. Budd, B. S. Miller, N. E. Weckman, D. Cherkaoui, D. Huang, A. T. Decruz, N. Fongwen, G. Han, M. Broto, C. S. Estcourt, J. Gibbs, D. Pillay, P. Sonnenberg, R. Meurant, M. R. Thomas, N. Keegan, M. M. Stevens, E. Nastouli, E. J. Topol, A. M. Johnson, M. Shahmanesh, A. Ozcan, J. J. Collins, M. F. Suarez, B. Rodriguez, R. W. Peeling and R. A. McKendry, Nat. Rev. Bioeng., 2023, 1, 13–31 CrossRef.
- M. Naseri, Z. M. Ziora, G. P. Simon and W. Batchelor, Rev. Med. Virol., 2022, 32, e2263 CrossRef CAS.
- P. Sadeghi, H. Sohrabi, M. Hejazi, A. Jahanban-Esfahlan, B. Baradaran, M. Tohidast, M. R. Majidi, A. Mokhtarzadeh, S. M. Tavangar and M. de la Guardia, Trends Anal. Chem., 2021, 145, 116460 CrossRef CAS PubMed.
- C. Zheng, K. Wang, W. Zheng, Y. Cheng, T. Li, B. Cao, Q. Jin and D. Cui, Analyst, 2021, 146, 1514 RSC.
- L. Khelifa, Y. Hu, N. Jiang and A. K. Yetisen, Lab Chip, 2022, 22, 2451–2475 RSC.
- F. Mazur, A. D. Tjandra, Y. Zhou, Y. Gao and R. Chandrawati, Nat. Rev. Bioeng., 2023, 1, 180–192 CrossRef PubMed.
- H. Sohrabi, M. R. Majidi, P. Khaki, A. Jahanban-Esfahlan, M. de la Guardia and A. Mokhtarzadeh, Compr. Rev. Food Sci. Food Saf., 2022, 21, 1868–1912 CrossRef PubMed.
- Q. Zhang, L. Fang, B. Jia, N. Long, L. Shi, L. Zhou, H. Zhao and W. Kong, Trends Anal. Chem., 2021, 144, 116427 CrossRef CAS.
- Z. Farka, J. C. Brandmeier, M. J. Mickert, M. Pastucha, K. Lacina, P. Skládal, T. Soukka and H. H. Gorris, Adv. Mater., 2024, 36, 2307653 CrossRef CAS PubMed.
- Y. Zhou, Y. Wu, L. Ding, X. Huang and Y. Xiong, Trends Anal. Chem., 2021, 145, 116452 CrossRef CAS PubMed.
- J. Dinnes, P. Sharma, S. Berhane, S. S. van Wyk, N. Nyaaba, J. Domen, M. Taylor, J. Cunningham, C. Davenport, S. Dittrich, D. Emperador, L. Hooft, M. M. G. Leeflang, M. D. F. McInnes, R. Spijker, J. Y. Verbakel, Y. Takwoingi, S. Taylor-Phillips, A. Van den Bruel and J. J. Deeks, Cochrane Database Syst. Rev., 2022,(7), CD013705 Search PubMed.
- E. Valera, A. Jankelow, J. Lim, V. Kindratenko, A. Ganguli, K. White, J. Kumar and R. Bashir, ACS Nano, 2021, 15, 7899–7906 CrossRef CAS PubMed.
- M. Cretich, G. G. Daaboul, L. Sola, M. S. Ünlü and M. Chiari, Trends Biotechnol., 2015, 33, 343–351 CrossRef CAS PubMed.
- S. O. Kelley, ACS Sens., 2017, 2, 193–197 CrossRef CAS PubMed.
- P. R. Srinivas, B. S. Kramer and S. Srivastava, Lancet Oncol., 2001, 2, 698–704 CrossRef CAS PubMed.
- J. Castillo-León, R. Trebbien, J. J. Castillo and W. E. Svendsen, Analyst, 2021, 146, 3750–3776 RSC.
- Y. Liu, L. Zhan, Z. Qin, J. Sackrison and J. C. Bischof, ACS Nano, 2021, 15, 3593–3611 CrossRef CAS PubMed.
- L. Roy, P. Buragohain and V. Borse, Biosens. Bioelectron. X, 2022, 10, 100098 CAS.
- H. R. Boehringer and B. J. O’farrell, Clin. Chem., 2022, 68, 52–58 CrossRef PubMed.
- A. C. Mirica, D. Stan, I. C. Chelcea, C. M. Mihailescu, A. Ofiteru and L. A. Bocancia-Mateescu, Front. Bioeng. Biotechnol., 2022, 10, 922772 CrossRef PubMed.
- Y. Deng, H. Jiang, X. Li and X. Lv, Microchim. Acta, 2021, 188, 379 CrossRef CAS PubMed.
- J. D. Bishop, H. V. Hsieh, D. J. Gasperino and B. H. Weigl, Lab Chip, 2019, 19, 2486–2499 RSC.
- S. Gao, L. Niu, R. Zhou, C. Wang, X. Zheng, D. Zhang, X. Huang, Z. Guo and X. Zou, Int. J. Biol. Macromol., 2024, 257, 128621 CrossRef CAS PubMed.
- A. K. Trilling, J. Beekwilder and H. Zuilhof, Analyst, 2013, 138, 1619–1627 RSC.
- L. F. Yang, M. Ling, N. Kacherovsky and S. H. Pun, Chem. Sci., 2023, 14, 4961 RSC.
- T. Wang, L. Chen, A. Chikkanna, S. Chen, I. Brusius, N. Sbuh and R. N. Veedu, Theranostics, 2021, 11, 5174–5196 CrossRef PubMed.
- A. N. Baker, G. W. Hawker-Bond, P. G. Georgiou, S. Dedola, R. A. Field and M. I. Gibson, Chem. Soc. Rev., 2022, 51, 7238–7259 RSC.
- L. Zhang, H. Jiang, Z. Zhu, J. Liu and B. Li, Talanta, 2022, 243, 123388 CrossRef CAS PubMed.
- M. M. Kaminski, O. O. Abudayyeh, J. S. Gootenberg, F. Zhang and J. J. Collins, Nat. Biomed. Eng., 2021, 5, 643–656 CrossRef CAS PubMed.
- Y. Tang, L. Gao, W. Feng, C. Guo, Q. Yang, F. Li and X. C. Le, Chem. Soc. Rev., 2021, 50, 11844–11869 RSC.
- S. Modha, C. Castro and H. Tsutsui, Biosens. Bioelectron., 2021, 178, 113026 CrossRef CAS PubMed.
- J. Park, Sensors, 2022, 22, 7398 CrossRef CAS PubMed.
- Z. Wang, J. Zhao, X. Xu, L. Guo, L. Xu, M. Sun, S. Hu, H. Kuang, C. Xu and A. Li, Small Methods, 2022, 6, 2101143 CrossRef CAS PubMed.
- Z. Su, W. Dou, X. Liu, J. Ping, D. Li, Y. Ying and L. Xie, TrAC, Trends Anal. Chem., 2022, 154, 116673 CrossRef CAS.
- V. T. Nguyen, S. Song, S. Park and C. Joo, Biosens. Bioelectron., 2020, 152, 112015 CrossRef CAS PubMed.
- X. Chen, L. Ding, X. Huang and Y. Xiong, Theranostics, 2022, 12, 574–602 CrossRef CAS PubMed.
- O. I. Guliy and L. A. Dykman, Biosens. Bioelectron. X, 2024, 17, 100457 CAS.
- L. Wang, X. Wang, L. Cheng, S. Ding, G. Wang, J. Choo and L. Chen, Biosens. Bioelectron., 2021, 189, 113360 CrossRef CAS PubMed.
- K. Kim, L. Kashefi-Kheyrabadi, Y. Joung, K. Kim, H. Dang, S. G. Chavan, M. H. Lee and J. Choo, Sens. Actuators, B, 2021, 329, 129214 CrossRef CAS PubMed.
- B. Fang, Q. Xiong, H. Duan, Y. Xiong and W. Lai, Trends Anal. Chem., 2022, 157, 116754 CrossRef CAS.
- W. He, M. Wang, P. Cheng, Y. Liu and M. You, Trends Anal. Chem., 2024, 173, 117641 CrossRef CAS.
- D. Calabria, M. M. Calabretta, M. Zangheri, E. Marchegiani, I. Trozzi, M. Guardigli, E. Michelini, F. Di Nardo, L. Anfossi, C. Baggiani and M. Mirasoli, Sensors, 2021, 21, 1–19 Search PubMed.
- J. Cheng, G. Yang, J. Guo, S. Liu and J. Guo, Analyst, 2022, 147, 554–570 RSC.
- I. Y. Goryacheva, E. S. Speranskaya, V. V. Goftman, D. Tang and S. De Saeger, Trends Anal. Chem., 2015, 66, 53–62 CrossRef CAS.
- O. S. Wolfbeis, Angew. Chem., Int. Ed., 2013, 52, 9864–9865 CrossRef CAS PubMed.
- X. Yang, X. Cheng, H. Wei, Z. Tu, Z. Rong, C. Wang and S. Wang, J. Nanobiotechnol., 2023, 21, 450 CrossRef CAS PubMed.
- M. Xu, J. Liu, X. Su, Q. Zhou, H. Yuan, Y. Wen, Y. Cheng and F. Li, Sci. China: Chem., 2021, 64, 2125–2133 CrossRef CAS.
- R. Chen, X. Chen, Y. Zhou, T. Lin, Y. Leng, X. Huang and Y. Xiong, ACS Nano, 2022, 16, 3351–3361 CrossRef CAS PubMed.
- Y. Wu, R. D. Tilley and J. Justin Gooding, J. Am. Chem. Soc., 2019, 141, 1162–1170 CrossRef CAS PubMed.
- E. B. Bahadır and M. K. Sezgintürk, Trends Anal. Chem., 2016, 82, 286–306 CrossRef.
-
M. Millipore, Rapid lateral flow test strips: considerations for product development, Billerica, MA, Germany, 2013 Search PubMed.
-
M. A. Mansfield, Lateral Flow Immunoassay, Humana Press, 2009, pp. 1–19 Search PubMed.
- R. Krska and A. Molinelli, Anal. Bioanal. Chem., 2008, 393, 67–71 CrossRef PubMed.
- F. Hou, S. Sun, S. W. Abdullah, Y. Tang, X. Li and H. Guo, Anal. Methods, 2023, 15, 2154–2180 RSC.
- R. Hu, T. Liao, Y. Ren, W. Liu, R. Ma, X. Wang, Q. Lin, G. Wang and Y. Liang, Nano Res., 2022, 15, 7313–7319 CrossRef CAS PubMed.
- D. Wang, S. He, X. Wang, Y. Yan, J. Liu, S. Wu, S. Liu, Y. Lei, M. Chen, L. Li, J. Zhang, L. Zhang, X. Hu, X. Zheng, J. Bai, Y. Zhang, Y. Zhang, M. Song and Y. Tang, Nat. Biomed. Eng., 2020, 4, 1150–1158 CrossRef CAS PubMed.
- L. Bian, Z. Li, A. He, B. Wu, H. Yang, Y. Wu, F. Hu, G. Lin and D. Zhang, Biomaterials, 2022, 288, 121694 CrossRef CAS PubMed.
- J. Hou, Y. Cao, Q. Deng, Q. Zhang, X. Deng, Z. Chen and Z. Zhong, Anal. Chim. Acta, 2024, 1288, 342143 CrossRef CAS PubMed.
- R. Chen, C. Ren, M. Liu, X. Ge, M. Qu, X. Zhou, M. Liang, Y. Liu and F. Li, ACS Nano, 2021, 15, 8996–9004 CrossRef CAS PubMed.
- H. Li, B. Dong, L. Dou, W. Yu, X. Yu, K. Wen, Y. Ke, J. Shen and Z. Wang, Sens. Actuators, B, 2020, 324, 128771 CrossRef CAS.
- Y. Cai, K. Kang, Y. Liu, Y. Wang and X. He, Anal. Biochem., 2018, 556, 129–135 CrossRef CAS PubMed.
- R. Chen, X. Zhou, Y. Wu, Q. Liu, Q. Liu, J. Huang and F. Li, Sens. Actuators, B, 2021, 328, 129050 CrossRef CAS.
- C. Li, Z. Zou, H. Liu, Y. Jin, G. Li, C. Yuan, Z. Xiao and M. Jin, Talanta, 2021, 225, 122064 CrossRef CAS PubMed.
- J. Xia, Y. Liu, M. Ran, W. Lu, L. Bi, Q. Wang, D. Lu and X. Cao, RSC Adv., 2020, 10, 29156–29170 RSC.
- Y. Matsumura, Y. Enomoto, M. Takahashi and S. Maenosono, ACS Appl. Mater. Interfaces, 2018, 10, 31977–31987 CrossRef CAS PubMed.
- J. Hu, S. Zhou, L. Zeng, Q. Chen, H. Duan, X. Chen, X. Li and Y. Xiong, Talanta, 2021, 223, 121723 CrossRef CAS PubMed.
- G. Chen, X. Chen, G. Xu, X. Wei, X. Lin, Y. Su, Y. Xiong and X. Huang, Food Chem., 2023, 412, 135580 CrossRef CAS PubMed.
- Y. Li, X. Chen, J. Yuan, Y. Leng, W. Lai, X. Huang and Y. Xiong, J. Dairy Sci., 2020, 103, 6940–6949 CrossRef CAS PubMed.
- C. Zhou, X. Yang, C. Zhang, S. Zhang and P. Zhang, Surf. Interfaces, 2021, 24, 101057 CrossRef CAS.
- X. Chen, X. Miao, T. Ma, Y. Leng, L. Hao, H. Duan, J. Yuan, Y. Li, X. Huang and Y. Xiong, Foods, 2021, 10, 1488 CrossRef CAS PubMed.
- Y. Zhou, Y. Chen, W. Liu, H. Fang, X. Li, L. Hou, Y. Liu, W. Lai, X. Huang and Y. Xiong, Sens. Actuators, B, 2021, 343, 130139 CrossRef CAS PubMed.
- X. Chen, Y. Leng, L. Hao, H. Duan, J. Yuan, W. Zhang, X. Huang and Y. Xiong, Theranostics, 2020, 10, 3737 CrossRef CAS PubMed.
- B. Liu, P. Li, Y. Wang, Y. Guo, H. Zhang, S. Dong, Y. Xiong and C. Zhang, Food Anal. Methods, 2020, 13, 1736–1745 CrossRef.
- G. Zhang, S. Xu, Y. Xiong, H. Duan, W. Chen, X. Li, M. Yuan and W. Lai, Biosens. Bioelectron., 2019, 135, 173–180 CrossRef CAS PubMed.
- L. Hao, Y. Leng, L. Zeng, X. Chen, J. Chen, H. Duan, X. Huang, Y. Xiong and X. Chen, Adv. Sci., 2020, 7, 1902433 CrossRef CAS PubMed.
- L. Hao, J. Chen, X. Chen, T. Ma, X. Cai, H. Duan, Y. Leng, X. Huang and Y. Xiong, Food Chem., 2021, 336, 127710 CrossRef CAS PubMed.
- Y. Su, X. Chen, H. Huang, Y. Wu, X. Shen, X. Lin, K. Sun, X. Fan, X. Huang and Y. Xiong, Aggregate, 2023, e459 Search PubMed.
- Z. Li, Q. Liu, Y. Li, W. Yuan and F. Y. Li, J. Rare Earths, 2021, 39, 11–18 CrossRef CAS.
- Z. Chen, Z. Zhang, X. Zhai, Y. Li, L. Lin, H. Zhao, L. Bian, P. Li, L. Yu, Y. Wu and G. Lin, Anal. Chem., 2020, 92, 7226–7231 CrossRef CAS PubMed.
- Q. Xu, Y. Wang, P. Gao and Y. Jiang, New J. Chem., 2021, 45, 10618–10625 RSC.
- C. Zhou, T. Liu, X. Yang, C. Zhang, S. Zhang and P. Zhang, Opt. Mater., 2020, 99, 109582 CrossRef CAS.
- F. Shi, Y. Tang, Z. H. Xu, Y. X. Sun, M. Z. Ma and C. F. Chen, J. Appl. Microbiol., 2021, 00, 1–10 Search PubMed.
- G. Wang, L. Yang, C. Li, H. Yu, Z. He, C. Yang, J. Sun, P. Zhang, X. Gu and B. Tang, Mater. Chem. Front., 2021, 5, 2452–2458 RSC.
- S. Zhou, Y. Peng, J. Hu, H. Duan, T. Ma, L. Hou, X. Li and Y. Xiong, Microchem. J., 2020, 159, 105533 CrossRef CAS.
- X. Lai, X. Lv, G. Zhang, Z. Xiong, W. Lai and J. Peng, Food Anal. Methods, 2020, 13, 2258–2268 CrossRef.
- C. Hofmann, A. Duerkop and A. J. Baeumner, Angew. Chem., Int. Ed., 2019, 58, 12840–12860 CrossRef CAS PubMed.
- S. Chatterjee, X.-S. Li, F. Liang and Y.-W. Yang, Small, 2019, 15, 1904569 CrossRef CAS PubMed.
- J. Wang, Q. Ma, Y. Wang, Z. Li, Z. Li and Q. Yuan, Chem. Soc. Rev., 2018, 47, 8766–8803 RSC.
- W. Wei, M. Wei and S. Liu, Rev. Anal. Chem, 2012, 31, 163–176 CrossRef CAS.
- H. Jung, S. H. Park, J. Lee, B. Lee, J. Park, Y. Seok, J. H. Choi, M. G. Kim, C. S. Song and J. Lee, Anal. Chem., 2020, 93, 792–800 CrossRef PubMed.
- Y. Zhu, L. Ao, S. Chu, Y. Liao, J. Wang, J. Hu and L. Huang, Adv. Funct. Mater., 2024, 2316147, 1–13 Search PubMed.
- B. Zhang, X. Yang, X. Liu, J. Li, C. Wang and S. Wang, RSC Adv., 2020, 10, 2483–2489 RSC.
- X. Yang, X. Liu, B. Gu, H. Liu, R. Xiao, C. Wang and S. Wang, Microchim. Acta, 2020, 187, 1–11 CrossRef PubMed.
- T. Dong, R. Yin, Q. Yu, W. Qiu, K. Li, L. Qian, H. Li, B. Shen and G. Liu, Anal. Chim. Acta, 2021, 1147, 56–63 CrossRef CAS PubMed.
- S. K. Kim, H. Sung, S. H. Hwang and M. N. Kim, Biochip. J., 2022, 16, 175–182 CrossRef CAS PubMed.
- S. Bock, H. M. Kim, J. Kim, J. An, Y. S. Choi, X. H. Pham, A. Jo, K. M. Ham, H. Song, J. W. Kim, E. Hahm, W. Y. Rho, S. H. Lee, S. M. Park, S. Lee, D. H. Jeong, H. Y. Lee and B. H. Jun, Nanomaterials, 2022, 12, 1–11 Search PubMed.
- H. M. Kim, C. Oh, J. An, S. Baek, S. Bock, J. Kim, H. S. Jung, H. Song, J. W. Kim, A. Jo, D. E. Kim, W. Y. Rho, J. Y. Jang, G. J. Cheon, H. J. Im and B. H. Jun, Nanomaterials, 2021, 11, 1–10 Search PubMed.
- J. Zhao, H. Han, Z. Liu, J. Chen, X. Liu, Y. Sun, B. Wang, B. Zhao, Y. Pang and R. Xiao, Anal. Chim. Acta, 2024, 1295, 342306 CrossRef CAS PubMed.
- C. Wang, X. Yang, S. Zheng, X. Cheng, R. Xiao, Q. Li, W. Wang, X. Liu and S. Wang, Sens. Actuators, B, 2021, 345, 130372 CrossRef CAS PubMed.
- S. Zheng, X. Yang, B. Zhang, S. Cheng, H. Han, Q. Jin, C. Wang and R. Xiao, Food Chem., 2021, 363, 130400 CrossRef CAS PubMed.
- C. Wang, X. Yang, B. Gu, H. Liu, Z. Zhou, L. Shi, X. Cheng and S. Wang, Anal. Chem., 2020, 92, 15542–15549 CrossRef CAS PubMed.
- C. Wang, D. Shi, N. Wan, X. Yang, H. Liu, H. Gao, M. Zhang, Z. Bai, D. Li, E. Dai, Z. Rong and S. Wang, Analyst, 2021, 146, 3908–3917 RSC.
- Z. Su, G. Zhao and W. Dou, Anal. Methods, 2021, 13, 2313–2319 RSC.
- D. Hong, E.-J. Jo, K. Kim, M.-B. Song and M.-G. Kim, Small, 2020, 16, 2004535 CrossRef CAS PubMed.
- X. Jia, K. Wang, X. Li, Z. Liu, Y. Liu, R. Xiao and S. Wang, Nanomed. Nanotechnol., Biol. Med., 2022, 41, 102522 CrossRef CAS PubMed.
- L. Shi, L. Xu, R. Xiao, Z. Zhou, C. Wang, S. Wang and B. Gu, Front. Microbiol., 2020, 11, 596005 CrossRef PubMed.
- V. G. Grigorenko, I. P. Andreeva, G. V. Presnova, D. E. Presnov, E. A. Yakovleva and A. P. Osipov, Moscow Univ. Chem. Bull., 2020, 75, 207–212 CrossRef.
- H. M. Kim, J. Kim, S. Bock, J. An, Y. S. Choi, X. H. Pham, M. G. Cha, B. Seong, W. Kim, Y. H. Kim, H. Song, J. W. Kim, S. min Park, S. H. Lee, W. Y. Rho, S. Lee, D. H. Jeong, H. Y. Lee and B. H. Jun, Sensors, 2021, 21, 4099 CrossRef CAS PubMed.
- R. Narayan, U. Y. Nayak, A. M. Raichur and S. Garg, Pharmaceutics, 2018, 10, 118 CrossRef CAS PubMed.
- C. Xu, C. Lei, Y. Wang and C. Yu, Angew. Chem., Int. Ed., 2022, 61, e202112752 CrossRef CAS PubMed.
- L. Ao, T. Liao, L. Huang, S. Lin, K. Xu, J. Ma, S. Qiu, X. Wang and Q. Zhang, Chem. Eng. J., 2022, 436, 135204 CrossRef CAS.
- F. Gao, C. Liu, Y. Yao, C. Lei, S. Li, L. Yuan, H. Song, Y. Yang, J. Wan and C. Yu, Biosens. Bioelectron., 2022, 199, 113892 CrossRef CAS PubMed.
- Y. Wang, C. Deng, S. Qian, H. Li, P. Fu, H. Zhou and J. Zheng, Food Chem., 2023, 399, 133970 CrossRef CAS PubMed.
- S. Tadepalli, Z. Wang, K. K. Liu, Q. Jiang, J. Slocik, R. R. Naik and S. Singamaneni, Langmuir, 2017, 33, 6611–6619 CrossRef CAS PubMed.
- F. Gao, C. Lei, Y. Liu, H. Song, Y. Kong, J. Wan and C. Yu, ACS Appl. Mater. Interfaces, 2021, 13, 21507–21515 CrossRef CAS PubMed.
- F. Gao, Y. Liu, C. Lei, C. Liu, H. Song, Z. Gu, P. Jiang, S. Jing, J. Wan and C. Yu, Small Methods, 2021, 5, 2000924 CrossRef CAS PubMed.
- D. Li, M. Huang, Z. Shi, L. Huang, J. Jin, C. Jiang, W. Yu, Z. Guo and J. Wang, Anal. Chem., 2022, 94, 2996–3004 CrossRef CAS PubMed.
- L.-D. Xu, F.-L. Du, J. Zhu and S.-N. Ding, Analyst, 2021, 146, 706–713 RSC.
- L. Di Xu, J. Zhu and S. N. Ding, Analyst, 2021, 146, 5055–5060 RSC.
- L. Huang, J. Jin, L. Ao, C. Jiang, Y. Zhang, H.-M. Wen, J. Wang, H. Wang and J. Hu, ACS Appl. Mater. Interfaces, 2020, 12, 58149–58160 CrossRef CAS PubMed.
- J. Yuan, L. Wang, L. Huang, K. He, H. Wang, X. Xu, B. Su and J. Wang, Anal. Chem., 2024, 96, 220–228 CrossRef PubMed.
- J. Wang, C. Jiang, J. Jin, L. Huang, W. Yu, B. Su and J. Hu, Angew. Chem., Int. Ed., 2021, 60, 13042–13049 CrossRef CAS PubMed.
- L. Huang, Y. Zhang, T. Liao, K. Xu, C. Jiang, D. Zhuo, Y. Wang, H. Wen, J. Wang, L. Ao and J. Hu, Small, 2021, 17, 2100862 CrossRef CAS PubMed.
- C. Wang, X. Cheng, L. Liu, X. Zhang, X. Yang, S. Zheng, Z. Rong and S. Wang, ACS Appl. Mater. Interfaces, 2021, 13, 40342–40353 CrossRef CAS PubMed.
- J. Tu, T. Wu, Q. Yu, J. Li, S. Zheng, K. Qi, G. Sun, R. Xiao and C. Wang, J. Hazard. Mater., 2023, 448, 130912 CrossRef CAS PubMed.
- C. Wang, C. Wang, X. Wang, K. Wang, Y. Zhu, Z. Rong, W. Wang, R. Xiao and S. Wang, ACS Appl. Mater. Interfaces, 2019, 11, 19495–19505 CrossRef CAS PubMed.
- L. Dou, Y. Bai, M. Liu, S. Shao, H. Yang, X. Yu, K. Wen, Z. Wang, J. Shen and W. Yu, Biosens. Bioelectron., 2022, 204, 114093 CrossRef CAS PubMed.
- X. Liu, K. Wang, B. Cao, L. Shen, X. Ke, D. Cui, C. Zhong and W. Li, Anal. Chem., 2021, 93, 3626–3634 CrossRef CAS PubMed.
- F. Wang, X. Li, Z. Liu, X. Zhao, C. Zhao, G. Hou, Q. Liu and X. Liu, Anal. Chem., 2024, 96, 2059–2067 CrossRef CAS PubMed.
- Z. Huang, Z. Xiong, Y. Chen, S. Hu and W. Lai, J. Agric. Food Chem., 2019, 67, 3028–3036 CrossRef CAS PubMed.
- Z. Huang, J. Peng, J. Han, G. Zhang, Y. Huang, M. Duan, D. Liu, Y. Xiong, S. Xia and W. Lai, Food Chem., 2019, 276, 333–341 CrossRef CAS PubMed.
- J. Wang, C. Jiang, J. Yuan, L. Tong, Y. Wang, D. Zhuo, L. Huang, W. Ni, J. Zhang, M. Huang, D. Li, B. Su and J. Hu, Anal. Chem., 2022, 94, 10865–10873 CrossRef CAS PubMed.
- Z. Bai, H. Wei, X. Yang, Y. Zhu, Y. Peng, J. Yang, C. Wang, Z. Rong and S. Wang, Sens. Actuators, B, 2020, 325, 128780 CrossRef CAS PubMed.
- L. Gloag, M. Mehdipour, D. Chen, R. D. Tilley and J. J. Gooding, Adv. Mater., 2019, 31, 1904385 CrossRef CAS PubMed.
- M. S. Kim, S. H. Kweon, S. Cho, S. S. A. An, M. Il Kim, J. Doh and J. Lee, ACS Appl. Mater. Interfaces, 2017, 9, 35133–35140 CrossRef CAS PubMed.
- Z. Xie, S. Feng, F. Pei, M. Xia, Q. Hao, B. Liu, Z. Tong, J. Wang, W. Lei and X. Mu, Anal. Chim. Acta, 2022, 1233, 340486 CrossRef CAS PubMed.
- W. Shen, C. Wang, X. Yang, C. Wang, Z. Zhou, X. Liu, R. Xiao, B. Gu and S. Wang, J. Mater. Chem. C, 2020, 8, 12854–12864 RSC.
- X. Liu, X. Yang, K. Li, H. Liu, R. Xiao, W. Wang, C. Wang and S. Wang, Sens. Actuators, B, 2020, 320, 128350 CrossRef CAS.
- S. Liu, L. Dou, X. Yao, W. Zhang, M. Zhao, X. Yin, J. Sun, D. Zhang and J. Wang, Biosens. Bioelectron., 2020, 169, 112610 CrossRef CAS PubMed.
- T. Zhang, L. Lei, M. Tian, J. Ren, Z. Lu, Y. Liu and Y. Liu, Talanta, 2021, 222, 121478 CrossRef CAS PubMed.
- M. Zhang, M. Li, Y. Zhao, N. Xu, L. Peng, Y. Wang and X. Wei, Food Res. Int., 2021, 142, 110102 CrossRef CAS PubMed.
- C. Wang, W. Shen, Z. Rong, X. Liu, B. Gu, R. Xiao and S. Wang, Nanoscale, 2020, 12, 795–807 RSC.
- G.-R. Han, H. Ki and M.-G. Kim, ACS Appl. Mater. Interfaces, 2020, 12, 1885–1894 CrossRef CAS PubMed.
- E. Renzi, A. Piper, F. Nastri, A. Merkoçi and A. Lombardi, Small, 2023, 19, 2207949 CrossRef CAS PubMed.
- D. Hong, K. Kim, E.-J. Jo and M.-G. Kim, Anal. Chem., 2021, 93, 7925–7932 CrossRef CAS PubMed.
- A. J. Qavi, Q. Jiang, M. J. Aman, H. Vu, L. Zetlin, J. M. Dye, J. W. Froude, D. W. Leung, F. Holtsberg, S. L. Crick and G. K. Amarasinghe, ACS Infect. Dis., 2024, 10, 57–63 CrossRef CAS PubMed.
- D. Hong, E. J. Jo, D. Bang, C. Jung, Y. E. Lee, Y. S. Noh, M. G. Shin and M. G. Kim, ACS Nano, 2023, 17, 16607–16619 CrossRef CAS PubMed.
- M. Song, J. Xing, H. Cai, X. Gao, C. Li, C. Liu, X. Li, X. Fu, S. Ding, W. Cheng and R. Chen, ACS Nano, 2023, 17, 10748–10759 CrossRef CAS PubMed.
- X. Yin, L. Dou, X. Yao, S. Liu, L. Zhang, M. Zhao, L. Su, J. Sun, J. Wang and D. Zhang, Food Chem., 2022, 367, 130737 CrossRef CAS PubMed.
- J. Zhang, Y. Li, F. Chai, Q. Li, D. Wang, L. Liu, B. Z. Tang and X. Jiang, Sci. Adv., 2022, 8, eabo1874 CrossRef CAS PubMed.
- K. He, T. Bu, X. Zheng, J. Xia, F. Bai, S. Zhao, X. yu Sun, M. Dong and L. Wang, J. Hazard. Mater., 2022, 425, 128034 CrossRef CAS PubMed.
- C. Wang, C. Wang, J. Li, Z. Tu, B. Gu and S. Wang, Biosens. Bioelectron., 2022, 214, 114525 CrossRef CAS PubMed.
- X. Yao, Z. Wang, M. Zhao, S. Liu, L. Su, L. Dou, T. Li, J. Wang and D. Zhang, Food Chem., 2021, 347, 129001 CrossRef CAS PubMed.
- G. R. Han and M. G. Kim, Sensors, 2020, 20, 2593 CrossRef CAS PubMed.
- J. Deng, M. Yang, J. Wu, W. Zhang and X. Jiang, Anal. Chem., 2018, 90, 9132–9137 CrossRef CAS PubMed.
- Y. Pan, X. Wei, X. Guo, H. Wang, H. Song, C. Pan and N. Xu, Biosens. Bioelectron., 2021, 194, 113611 CrossRef CAS PubMed.
- M. Lv, W. Zhou, H. Tavakoli, C. Bautista, J. Xia, Z. Wang and X. J. Li, Biosens. Bioelectron., 2021, 176, 112947 CrossRef CAS PubMed.
- Y. Zhao, H. Zeng, X.-W. Zhu, W. Lu and D. Li, Chem. Soc. Rev., 2021, 50, 4484–4513 RSC.
- Y. Wang, Y. Hu, Q. He, J. Yan, H. Xiong, N. Wen, S. Cai, D. Peng, Y. Liu and Z. Liu, Biosens. Bioelectron., 2020, 169, 112604 CrossRef CAS PubMed.
- Y. S. Wei, M. Zhang, R. Zou and Q. Xu, Chem. Rev., 2020, 120, 12089–12174 CrossRef CAS PubMed.
- A. Bavykina, N. Kolobov, I. S. Khan, J. A. Bau, A. Ramirez and J. Gascon, Chem. Rev., 2020, 120, 8468–8535 CrossRef CAS PubMed.
- L. Jiao and H. L. Jiang, Chem, 2019, 5, 786–804 CAS.
- J. Zou, X. Liu, X. Ren, L. Tan, C. Fu, Q. Wu, Z. Huang and X. Meng, Nanoscale, 2021, 13, 7844–7850 RSC.
- J. Yuan, X. Chen, H. Duan, X. Cai, Y. Li, L. Guo, X. Huang and Y. Xiong, Microchim. Acta, 2020, 187, 1–9 CrossRef PubMed.
- X. Cheng, S. Zheng, W. Wang, H. Han, X. Yang, W. Shen, C. Wang and S. Wang, Chem. Eng. J., 2021, 426, 131836 CrossRef CAS.
- H. Duan, X. Chen, Y. Wu, Y. Leng, X. Huang and Y. Xiong, Anal. Chim. Acta, 2021, 1141, 136–143 CrossRef CAS PubMed.
- J. Jia, L. Ao, Y. Luo, T. Liao, L. Huang, D. Zhuo, C. Jiang, J. Wang and J. Hu, Biosens. Bioelectron., 2022, 198, 113810 CrossRef CAS PubMed.
- D. Gasperino, T. Baughman, H. V. Hsieh, D. Bell and B. H. Weigl, Annu. Rev. Anal. Chem., 2018, 11, 219–244 CrossRef PubMed.
- Y. Yang, J. Tang, H. Song, Y. Yang, Z. Gu, J. Fu, Y. Liu, M. Zhang, Z. Qiao and C. Yu, Angew. Chem., Int. Ed., 2020, 59, 19610–19617 CrossRef CAS PubMed.
- C. Parolo, A. Sena-Torralba, J. F. Bergua, E. Calucho, C. Fuentes-Chust, L. Hu, L. Rivas, R. Álvarez-Diduk, E. P. Nguyen, S. Cinti, D. Quesada-González and A. Merkoçi, Nat. Protoc., 2020, 15, 3788–3816 CrossRef CAS PubMed.
- L. Zhan, S. Guo, F. Song, Y. Gong, F. Xu, D. R. Boulware, M. C. McAlpine, W. C. W. Chan and J. C. Bischof, Nano Lett., 2017, 17, 7207–7212 CrossRef CAS PubMed.
- C. J. Gommes, A.-J. Bons, S. Blacher, J. H. Dunsmuir and A. H. Tsou, AIChE J., 2009, 55, 2000–2012 CrossRef CAS.
- G. Boccardo, D. L. Marchisio and R. Sethi, J. Colloid Interface Sci., 2014, 417, 227–237 CrossRef CAS PubMed.
- S. Zhang, R. Geryak, J. Geldmeier, S. Kim and V. V. Tsukruk, Chem. Rev., 2017, 117, 12942–13038 CrossRef CAS PubMed.
- K. E. Sapsford, W. R. Algar, L. Berti, K. B. Gemmill, B. J. Casey, E. Oh, M. H. Stewart and I. L. Medintz, Chem. Rev., 2013, 113, 1904–2074 CrossRef CAS PubMed.
- L. Huang, T. Liao, J. Wang, L. Ao, W. Su and J. Hu, Adv. Funct. Mater., 2018, 28, 1705380 CrossRef.
- M. Zhou, X. Chen, X. Shen, X. Lin, P. Chen, Z. Qiao, X. Li, Y. Xiong and X. Huang, J. Agric. Food Chem., 2023, 71, 4408–4416 CrossRef CAS PubMed.
- X. Wei, X. Chen, Z. Liu, G. Xu, G. Chen, X. Lin, W. Zhan, X. Huang and Y. Xiong, Anal. Chim. Acta, 2023, 1247, 340869 CrossRef CAS PubMed.
-
D. D. Perrin, B. Dempsey and E. P. Serjeant, pKa Prediction for Organic Acids and Bases, Chapman and Hall, London, 1981 Search PubMed.
- G. Liu, J. Wang, J. Wang, X. Cui, K. Wang, M. Chen, Z. Yang, A. Gao, Y. Shen, Q. Zhang, G. Gao and D. Cui, Talanta, 2024, 273, 125868 CrossRef CAS PubMed.
- Q. Yu, W. Dou, J. Liu, G. Zhao, S. Yang, D. Zhu, Y. Zhao and L. Li, Anal. Methods, 2019, 11, 268–275 RSC.
- J. Li, Y. Lv, N. Li, R. Wu, J. Li, J. You, H. Shen, X. Chen and L. S. Li, Sens. Actuators, B, 2021, 344, 130275 CrossRef CAS.
|
This journal is © The Royal Society of Chemistry 2024 |