DOI:
10.1039/D2SC03750E
(Review Article)
Chem. Sci., 2022,
13, 12309-12325
Multi-analyte sensing strategies towards wearable and intelligent devices
Received
6th July 2022
, Accepted 17th September 2022
First published on 24th September 2022
Abstract
Continuous efforts to produce functional nanomaterials and flexible/stretchable devices have promoted cumbersome, laboratorial, detection processes toward wearable and portable intelligent sensing approaches. Responding to the challenges of the multiple analytes, mixtures, and complex components of practical samples, sensing array and multivariate analysis techniques have a significant advantage in terms of superior analytical capabilities, i.e., they are convenient, rapid, sensitive and have high-throughput for multi-analyte identification in food safety, clinical diagnoses, and environmental monitoring. Besides traditional molecular design and recognition mechanisms, materials with micro/nano structures also contribute to strong signals, sensitive responses, and novel properties. In this review, through a new perspective of signal amplification for responsive discrimination, we summarize progress in developing sensing arrays based on diverse micro/nanomaterials and their integrated devices for multi-analyte discrimination. An overview of strategies for constructing sensing arrays through various micro or nano building blocks, including 0D nanoparticle assembly and modification, 1D nanowires and fibers, 2D graphene and textiles, is schematized. Then, portable and wearable devices integrating colorimetric sensors or flexible electrochemical electrodes with the newest microelectronic units and circuit boards are presented. Meanwhile, the latest artificial intelligence (AI) algorithms are introduced for massive data analysis in complex biological and environmental systems. With future developments in facile and accurate discrimination for multi-analyte research, extended applications will gear up in various fields.
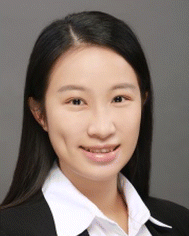 Yu Huang | Yu Huang received her PhD degree (2013) from the Institute of Chemistry, Chinese Academy of Sciences. Then she worked in Pennsylvania State University as a postdoctoral fellow. In 2018, she worked as a professor at the Faculty of Materials Science and Chemistry, China University of Geosciences (Wuhan). Her scientific interest is focused on interfacial super-wettability and biosensors. |
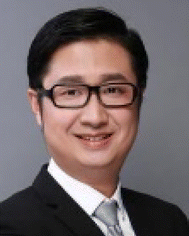 Fan Xia | Fan Xia obtained his PhD degree from the Institute of Chemistry, Chinese Academy of Sciences in 2008. He worked as a postdoc in the University of California, Santa Barbara. In 2012, he joined Huazhong University of Science and Technology as a professor. In 2016, he joined China University of Geosciences (Wuhan), as the dean and professor. His main research interests are focused on bioanalytical chemistry. |
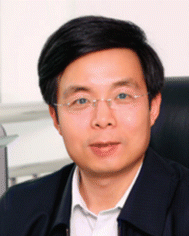 Yanlin Song | Yanlin Song received his PhD degree from the Department of Chemistry at Peking University in 1996. He then worked as a postdoctoral fellow in the Department of Chemistry of Tsinghua University from 1996 to 1998. He then joined the Institute of Chemistry, Chinese Academy of Sciences in 1998. He is currently a professor and the director of the Key Laboratory of Green Printing, at the Institute of Chemistry, Chinese Academy of Sciences. His research interests cover the areas of high density storage materials, smart photonic crystals and green printing technology. |
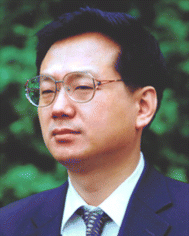 Lei Jiang | Lei Jiang is a professor at the Technical Institute of Physics and Chemistry, Chinese Academy of Sciences (TIPCCAS) and Beihang University. He is also an academician of the Chinese Academy of Sciences, Academy of Sciences for the Developing World, and National Academy of Engineering, USA. He received his Bachelor's and Master's degrees from Jilin University, and his PhD from the University of Tokyo. He worked as a post-doctoral fellow with Prof. Akira Fujishima and then as a senior researcher in the Kanagawa Academy of Sciences and Technology with Prof. Kazuhito Hashimoto. In 1999, he joined the Institute of Chemistry, Chinese Academy of Sciences as part of the Hundred Talents Program. In 2015, he and his group moved to TIPCCAS. His scientific interests focus on bio-inspired, smart, multi-scale interfacial materials with superwettability. |
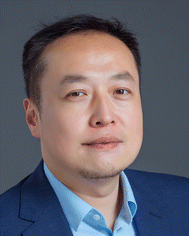 Fengyu Li | Fengyu Li is a full-time professor of Jinan University. He obtained his PhD degree from the Institute of Chemistry, Chinese Academy of Sciences in 2008. Then he conducted research as a postdoctoral follow in Bowling Green State University from 2008 to 2011. His research interests include photonic crystal materials, multianalyte sensing, flexible electronics, printed assembly, and 3D printing manufacture. He promoted high-performance multianalyte recognition with a single and simple dye, and achieved the integration of multiresolution photonic or electronic devices. |
1. Introduction
Wearable and intelligent sensing devices have attracted great interest due to their promising prospects in environmental protection and medical health.1–4 Their unique advantages in monitoring motion and continuous non-invasive monitoring of dynamic biological fluids, such as tears,5 saliva,6 and sweat,7,8 have also been well demonstrated. Multiple analytes, mixtures, and complex components are the principal features of biological fluids9–12 or environmental samples,13–17 which provide critical challenges in terms of accuracy, time-consumption, sophisticated instrumentation, and high-cost. Unlike a traditional “lock-and-key” sensor model with highly selective binding interactions to realize a low limit of detection in specific testing, multisensor platforms mimic biological sensory systems (i.e., the human nose and tongue) and adopt arrays of broadly cross-reactive sensors to realize “artificial olfactory systems” or an “electronic/chemistry tongue” to discriminate analytes in the gas phase or liquid phase, respectively.18 Cross-reactive sensors interact with analytes based on differential binding, and then generate differential response patterns. These response patterns could be considered “finger-prints” of analytes because they are unique for each analyte. Through comparing response patterns, molecular recognition could be successfully realized. Since Persaud et al. made models based on a biological olfactory system and adopted different metal oxides as sensors to discriminate similar gases in 1982,19 an increasing number of high-throughput sensor arrays have been developed in past decades for multiple analytes, including ions,20,21 proteins,10 bacteria,22–24 and cells.25,26 Through changes in chemical or physical properties, such as optical, electrochemical, or electrical, after sensors have combined with analytes, various recognition mechanisms and operating principles have been employed in sensing arrays.27
Generally, the biggest challenge in multi-analyte discrimination is the fabrication of multiresolution sensors and the analysis of various and complex data.28,29 In traditional sensing arrays, large numbers of sensor compounds or indicators have to be employed to obtain sufficient sensing information, which always involves complicated synthesis or massive screening, which severely limits the development of advanced sensing arrays.30,31 Recently, instead of adopting large numbers of sensor compounds or indicators, investigation of micro or nanomaterials has also promoted enormous progress in sensing systems, response mechanisms, testing methods and measurement modes.32 Various nanomaterials provide a diversity of morphologies with kaleidoscopic combinations of functional groups and abundant optical or electronical properties. They offer convenient, rapid, sensitive and high-throughput multi-analyte discriminative sensing via devices assembled from modified nanomaterials.33 Additionally, the integration of devices opens up tremendous opportunities for wild applications based on multi-analyte sensing. In general, sensing arrays provide a large amount of data for processing through statistical analysis, such as principal component analysis (PCA), linear discriminant analysis (LDA), and clustering analyses, i.e., hierarchical cluster analysis (HCA).34–39 Briefly, the goal of PCA is to find the greatest extent of variance in a set of data. Linear projection is the critical process for reduction of dimensions and extraction of the main features; that is, reducing the dimensionality of the dataset while identifying and retaining the most important features to describe the original variation. LDA finds a linear combination of features that characterizes or separates multiple analytes, and then adopts distances between centroids to classify analytes. Utilizing the whole dimensionality of the data, HCA creates a dendrogram of clusters to show the similarities between each analyte. Whatever statistical analysis or clustering analyses are adopted to realize successful multi-analyte differential sensing, the key point is to guarantee the sensor array a yield of appreciably differential signals for analysis through array design. A response data matrix is the prerequisite, which contains lots of columns corresponding to the number of detected features, and many rows corresponding to the number of recorded observations.34 There are numerous factors contributing to the probability of success in multi-analyte analysis. Deduction suggests a monotonically increasing function for the universality of a strategy applied in multitarget analysis.40 The calculated probability of success obviously increases when the number of observations increases. The massive number of different and relevant features will guarantee that the generated signals are cross-reactive and differential. Therefore, features contribute to successfully realizing high-performance multi-analyte discriminative classification and identification. In the response data matrix, an extended or excavated feature column can enrich the data matrix, resulting in an obvious reduction in dimensionality and clear hierarchical linear projection processing. Facing the demands of abundant data for successful multi-analysis, we propose an improvement in multi-analyte recognition via response signal and data excavation or extension. Progress in sensing arrays based on the assembly of diverse nanomaterials, the integration of various devices, and the latest developments in data analysis using artificial intelligence (AI) assisted algorithms are summarized to promote multi-analyte identification. A hierarchical outline is expanded with the development of nanomaterial sensors for multi-analysis. A multi-analyte sensor array firstly refers to diversity from nanomaterials to excavate new sensing information or enhance the signal-to-noise ratio. For example, devices containing a novel sensor array are endowed with ergonomic compatibility, multifunctional integration, and deep-learning mathematical operation. Firstly, the diversity of nanomaterials is unfolded in morphology from zero-dimensional (0D) to one-dimensional (1D) and two-dimensional (2D). We describe nanoparticle (NP) assembled nanomatrices for the construction of high-performance optical sensor arrays, and various modifications on NPs for efficient multi-analyte detection.
Optical properties, including structural color, slow-photon fluorescence enhancement, and the plasmon effect, are discussed as features to be excavated to promote more sensitive detection and higher resolution multi-analyte discrimination. Secondly, typical examples of monitoring of multiple vital signs via electronic signals are illustrated. The adoption of flexible patterns, e-textiles and graphene gels, and the fascinating prospects for clinical diagnosis and health assessment via extending multi-analysis features using piezo-resistance, field effect transistors, and capacitance monitoring are described. Finally, examples of practical devices with the rational integration of materials, structures, and mechanisms to approach portable, wearable, or attachable multi-analysis sensors are given. Beyond the design of devices, integration also involves the advanced mathematic operation of machine-learning. Facing up to a complex realistic environment or the requirements of dynamic monitoring calls for sensitive, high-throughput, commercial, and versatile multi-analyte recognition instruments with good user experience. Nanomaterial-based sensor devices present various reinforced optical or electronical properties via morphological construction. The extended and excavated response features will contribute to a tremendous database and result in high-resolution discrimination or abundant multi-analyte recognition.
In this review, an overview of strategies for constructing sensing arrays through various micro or nano building blocks, including 0D NP assembly and modification, 1D nanowires and fibers, and 2D graphene and textiles are presented. Then, typical sensing array devices integrated through chemometric methods for the differential sensing of clinical samples are displayed. Additionally, recent developments in AI-assisted algorithm data analysis over the last two or three years are summarized (Fig. 1). Finally, perspectives on challenges and future developments are presented. With the development of facile and accurate discrimination for multi-analysis research, extended applications would gear up in various fields.
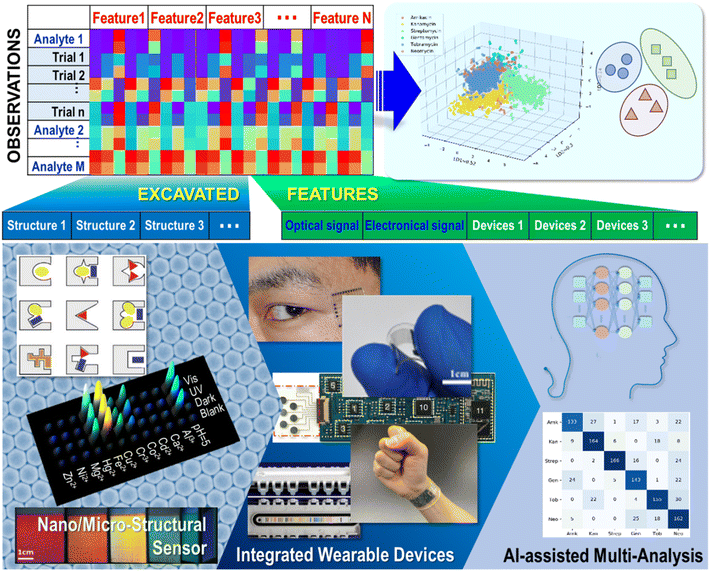 |
| Fig. 1 Schematic of promoted multi-analyte discriminative sensing via structural diversity, wearable device integration, and artificial intelligence (AI) assisted strategies. Reproduced with permission from ref. 17,28, 40, 68, 73, 117, 128 and 129. Copyright 2001, Wiley-VCH. Copyright 2016, Nature Publishing Group. Copyright 2015, Nature Publishing Group. Copyright 2016, Wiley-VCH. Copyright 2015, ACS publishing group. Copyright 2021, Wiley-VCH. Copyright 2022, ACS publishing group. | |
2. Diversity of nanomaterial optical sensors
Most recently, tremendous efforts have been made with low-dimensional nanomaterials as highly sensitive sensor transducers, including NPs, nanowires, nanotextiles, nanotubes, nanofibers, and graphene. For fluorescent sensing arrays, the rational design of a series of molecular sensors with similar chemical formulae is the most typical method. In general, these series of sensors are derivatives of a substance, which usually come from chemical modification, such as substitution with extended conjugated fluorescence.41–45 However, the rational design of sensors involves complex synthesis, purification processing and compound screening to achieve serials for valid sensors. Now, the field of multi-analyte molecular recognition has reached a stage where one can develop new principles and techniques to design and synthesize a feasible receptor with a good degree of predictability and selectivity. In particular, based on molecular design, improvements in methods and integration of devices, it is crucial to achieve correlative differential analysis without complicated chemical synthesis or screening of valid compounds. Through analyzing the characteristics of the sensor (i.e., optical, photo luminescent, and fluorescent) before and after binding with targets, a serial of targets with similar molecular structures, chemical or physiologic properties (i.e., proteins, anions, or human mobility) can be distinguished. Meanwhile, a series of new issues appear in the multi-analyte analyzing process from an analogical perspective. With the development of nanotechnology, new micro/nanostructure processing techniques have been combined with multi-analyte discrimination to avoid complex rational molecular design, which has obviously geared up their applications in related device integration, such as in biological detection and the electronics industry. In this section, sensing array assemblies and modifications through various micro/nano building blocks, including 0D NPs, 1D nanowires and fibers, 2D graphene and textiles, and their integrated devices for bio-target discrimination are discussed.
2.1 NP-assembled photonic crystal (PC) sensing arrays
As the most basic nanomaterial element, 0D NPs have undergone mass exploration since nanomaterial and nanotechnology research began to boom. Various kinds of NPs have been fabricated, diversely coordinated, and assembled with various novel functions. Specifically, through a facile and cost-effective bottom-up self-assembly strategy, photonic crystals (PCs) with a periodic dielectric structure have received increasing interest.46–52 PCs are capable of affecting light propagation and create a forbidden gap (a photonic stopband) in their photonic band structure.53 In past decades, PCs have attracted enormous research interest and increasing awareness of their potential applications in sensing, detection, catalysts, displays, solar cells and other fields, owing to their specific optical properties. Through reducing the group velocity at the edge of the photonic stopband of PCs, PCs have demonstrated outstanding modulation of spontaneous emission. Therefore, on photonic stopband matched PCs, the light output shows effective modulation and optical signal selective amplification. For PCs fabricated from self-assembled NPs, their photonic stopband can be easily tuned through NP sizes, chemical constitutions, assembly structures, and light incident angles, according to Bragg's law:54
λmax = 2d111neff sin θ |
Here, λmax is the maximum wavelength of reflectance, also called the photonic stopband; d111 refers to lattice spacing, which is related to size of the NPs and their assembly structure; neff is the effective refractive index of the PCs, decided by the materials of the NPs and the materials between the NPs; and θ is the glancing angle. The photonic stopband of the PCs was decided by the materials and size of the NPs, their assembly structure, the light incident angle, and the PCs' functionalized molecular recognition agents during the sensing process. With a matched photonic stopband, PCs would transduce diverse external stimulations to measurable optical responses and selectively enhanced fluorescence signals. Based on this principle, PC-based sensors with high efficiency and selectivity have been well developed, including analytes of metal ions, cations, pH, humidity, oil, DNA, cells, antibodies, glucose, creatinine, and ethanol.55–58
Inspired by PC-based sensors, researchers figured out that PCs could provide an opportunity for efficient and facile multi-analyte detection based on the achievement of increased sensing information Cunningham et al. extended the DNA detection capabilities of a standard microarray test on PCs by enhancing the fluorescence intensity from microarray spots.59,60 Another compelling advantage of a PC-based sensing array in multi-analyte recognition is avoiding a large number of lab-synthesized chemical compounds adopted as sensors. By introducing 6 commercial dyes into 7 PCs with different stopbands, Gu's group developed a PC sensor array for the sensing of 8 vapor-phase chemical molecules.61
When the photonic stopband of PCs is in the visible light region, the changes in the photonic stopband are actually displayed as a change in structural color, which can be perceived by the human eye. For example, Li et al. combined ionic liquids and appropriate PCs to realize anion detection by the naked eye.62 When sensing different anions, counter anion exchange led to changes in the PCs' periodic structure. As a result, the film's color varied according to the different anions. Unlike a traditional PC-based sensor adopting signals in the equilibrium state, Ge and co-workers developed a PC gel to distinguish solvents with similar structures through monitoring its dynamic reflection spectrum.63 Similarly, Ozin et al. fabricated a “PC nose” through a PC sensor with multiple layers of alternating refractive index.64 Nine kinds of various functionalized PCs were adopted to realize identification of similar molecules and bacteria. PCs can also be flexibly combined with other sensing materials: for example, molecularly imprinted polymers. Combining molecularly imprinted and inverse PCs as sensing elements in a sensing array, identification of 6 analytes was reported by Li's group for the first time.65 Compared with chromatographic methods, molecularly-imprinted spatially patterned PC arrays demonstrated a significant advance in the low-cost fabrication of sensors, a simple and fast analytical process, and the ability to detect trace amounts.
Although multi-stopband PC chips combined with a serial of chemical sensors have demonstrated their ability to discriminate multiple analytes, researchers are still working on simplifying the sensing array to a mono-chemical sensor or mono-stopband PC chips. In these cases, the complicated synthesis and screening process of serial sensors, and the complex fabrication and integration process of a multiplex PC materials matrix can be avoided. We constructed a multi-stopband PC microchip for the recognition of 12 metal ions based on only one simple and commercialized sensor: 8-hydroxyquinoline (8-HQ).66 This PC microchip was fabricated through a hydrophilic–hydrophobic patterned surface. Due to the different surface energies of the hydrophilic area and hydrophobic area, NPs would selectively adsorb and self-assemble on the hydrophilic areas of the patterned surface.67 The multi-stopband PC microchip selectively performed fluorescence signal enhancement through the matched photonic stopbands, which contributes to the correlative differential analysis. Instead of adopting 6 kinds of 8-HQ derivatives as sensors to achieve enough differential responsive signal for discriminating metal ions, this work used PCs to amplify the responsive signal after metal ions had combined with 8-HQ, which also successfully realized metal ion recognition. This research provides significant insight into high-performance discriminant analysis through introducing micro/nanodevices.
Furthermore, we developed a rainbow structural-color mono-stopband PC chip assembled from single-size NPs. This PC chip displayed angle-dependent structural colors from red and orange to bluish violet, as shown in Fig. 2a.68 According to Bragg's law, besides the lattice spacing and refractive index of the PCs, the angle of incidence of light (θ) also plays a key role in changing the photonic stopband position of PCs
:
PCs displayed various colors when θ changed from 0° to 90°. The change in the color implied a shift in the position of the photonic stopband of the PCs, that is, the maximum wavelength of reflectance. The detection process was carried out under various reflection angles, endowing this PC mono-stopband chip with the ability to enhance the fluorescence in different spectral regions. As a result, this mono-stopband PC chip realized the recognition of 12 saccharides through PCs assembled from one size of NPs. Based on this understanding, recently we further developed PC chips with diverse micromorphology assembled from one size of NPs, as shown in Fig. 2b.69 The PC pixels displayed various structural colors and provided abundant differential fluorescence information. As a result, 14 metal ions and 12 groundwater samples were successfully distinguished.
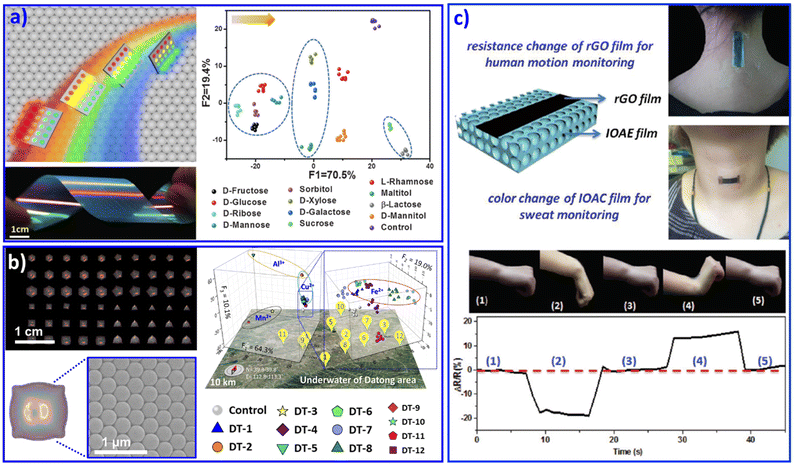 |
| Fig. 2 (a) A rainbow structural-color mono PC chip selectively enhances fluorescence with various viewing angles, resulting in the identification of 12 saccharides. Reproduced with permission from ref. 68. Copyright 2016, Wiley-VCH. (b) The diverse micromorphology of a PC chip realized the discrimination of various metal ions and 12 groundwater samples. Reproduced with permission from ref. 69. Copyright 2021, Wiley-VCH. (c) Multifunctional wearable sensor based on a composite of rGO and PCs. The wearable sensor attached on the wrist joint and neck. Reproduced with permission from ref. 71. Copyright 2018, Royal Society of Chemistry. | |
Similar to opal PC, inverse opal PC can also be adopted for sensing systems. Combined with poly(ionic liquids) as a sensing unit, the imidazolium moieties generated various products by a counterion exchange reaction of ionic liquids, resulting in the discrimination of 5 anions and 8 organic solvents with different polarities. Poly(ionic liquid) inverse opal PC beads doped with AIE dye were exploited for the recognition of 20 natural amino acids in human urine.70 Combined with a flexible substrate, PC-structured wearable sensors with the abilities of in situ monitoring of motion and sweat were feasibly achieved. For example, Gu's group developed a multifunctional wearable sensor based on a reduced oxide graphene (rGO) film on a PC film, as shown in Fig. 2c. The wearable sensor can be attached on the wrist joint and the neck to realize in situ motion monitoring and in situ analysis of the ion concentration in sweat.71 Besides polymer NPs, metal NPs could also be adopted to form micro/nanostructural sensor arrays.72 We assembled Ag NPs on flexible poly(dimethylsiloxane) (PDMS) with a patterned silicon pillar to integrate curve arrays.73 The as-prepared Ag NP curve arrays displayed discriminative resistance with different deformations, which endowed it as a wearable microelectrode sensor for discriminating monitored human facial expressions, including relaxed, anger, disgust, fear, laughter, sadness, smiling, and surprise. We believe that with burgeoning inkjet printing technology, the design and fabrication of patterned PC-based sensing arrays for the recognition of various multiple analytes will become a remarkable part of wearable sensing chips, due to their advantages of efficient use of materials, low cost, and elimination of waste. This idea may open an efficient avenue for the multi-analyte recognition research field.
2.2 Nanofiber aligned sensor array
Over past decades, 1D nanoscale building blocks with superior electrical and mechanical properties, such as nanowires, nanofibers, and nanotubes, have emerged as key components in a number of advanced and miniaturized electronic, sensing, optical, electromechanical and thermal systems. Meanwhile, because of their high surface-to-volume ratios, 1D materials are considered particularly sensitive chemical sensors. Introducing 1D nanomaterials as different active materials into array sensing devices has also attracted immense interest. For instance, Heath et al. and Li et al. developed functionalized Si nanowires (NWs)74 and carbon nanotubes75,76 as sensing elements in sensor arrays to distinguish vapors. Most recently, Dou et al. designed and fabricated an optoelectronic SiNWs/ZnO/reduced graphene oxide (rGO) Schottky sensing system for the recognition of explosive vapors.77 The ultrasensitive light response property of SiNWs and the response to explosive vapors of SiNWs and ZnO NPs contributed to a real-time sensing array, as shown in Fig. 3a.
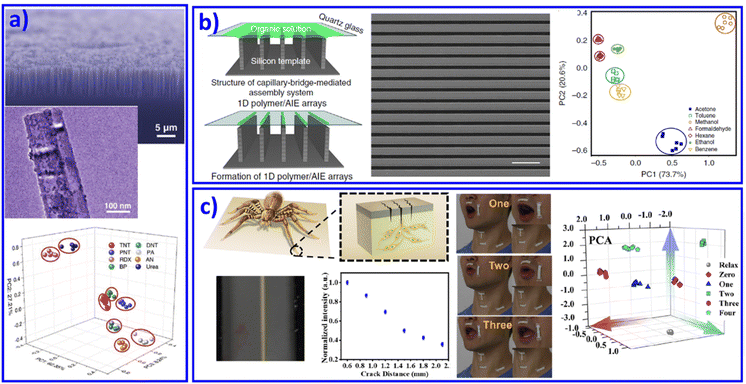 |
| Fig. 3 1D nanomaterial based sensor arrays. (a) A SiNWs/ZnO/rGO Schottky sensor array for vapor recognition. Reproduced with permission from ref. 77. Copyright 2017, Wiley-VCH. (b) Organic vapor discrimination. Reproduced with permission from ref. 79. Copyright 2018, Nature Publishing Group. (c) Inspired by slit in spider's leg joint, ultrasensitive mechanoluminescence sensors were achieved for visualizable vocalization. REF Reproduced with permission from ref. 80. Copyright 2021, American Chemical Society. | |
Although metal and non-metal nanowire based sensing arrays have been demonstrated, challenges still remain in their effective fabrication at large scale. For polymer nanowires, the approaches are much more flexible, providing a significant perspective insight for advanced 1D material based sensing chips. Typical examples include the development of fluorescent sensors combined with a polymer nanofiber mat to achieve sensing of 10 metal ions.78 Through an electro-spun method, the fiber's chemical components and orientations in the mat can be easily tuned, demonstrating its potential for various target analytes, even as wearable sensors. Recently, AIE molecules combined with polymer microwires were further developed to form an organic vapor sensing array (Fig. 3b).79 The polymer microwires came from a liquid-bridge-mediated assembly method. With organic vapor present, the AIE/polymer microwires would swell and change the aggregated state of the AIE molecules, resulting in varying fluorescent intensity. This new mechanism of swelling-induced varying fluorescent signals provided a new method for optical organic vapor sensing arrays. With this understanding, very recently we mimicked joint slits in spider legs and developed ultrasensitive mechanoluminescence sensors. Under the driving of 0.1 N stress, these sensors with a slit-based pattern performed distinct mechanoluminescence, which contributed to bright luminescence while people talked and during visible vocalization (Fig. 3c).80 Further improvement in the performance of the 1D nanomaterial based sensing array, such as selectivity and sensitivity, will generate a significant practical impact on defense applications, environmental monitoring, and medical diagnosis. However, device fabrication and system integration processes still require significant development for economically viable applications.
2.3 Graphene-based 2D nanosheet matrix
Among various 2D materials, graphene is absolutely a rapidly rising star on the horizon of condensed-matter physics and materials science, with its outstanding properties of good electrical conductivity, high mobility, surface area, high Young's modulus, optical transmittance and so on.81 As a precursor, graphite oxide (GO) has emerged, offering the cost-effective, large-scale production of graphene-based materials. For discriminative sensing, GO usually works as a quenching substrate or signal transducer for detecting proteins,10,82 therapeutic antibodies,83 and distinguishing differences in human mobility84 and human hemoglobin.85 For example, Fan et al. combined nanographene oxide (NGO) and 7 kinds of DNA tagged with fluorescent dye to form a sensing array system.10 The fluorescence of the system was quenched at first. With various proteins, the bound DNA strands were released into solution due to competitive displacement of the proteins. As a result, differential fluorescent response patterns were achieved. In this work, they demonstrated that the size and shape of NGO may result in various fluorescent quenching effects. Later on, based on NGO and fluorophores combined into a sensor array, Dravid and co-workers confirmed that the fluorescent response was enhanced as the size of the NGO became smaller, due to a more pronounced GO–protein binding and release response through fluorescence displacement transduction, as shown in Fig. 4a.82
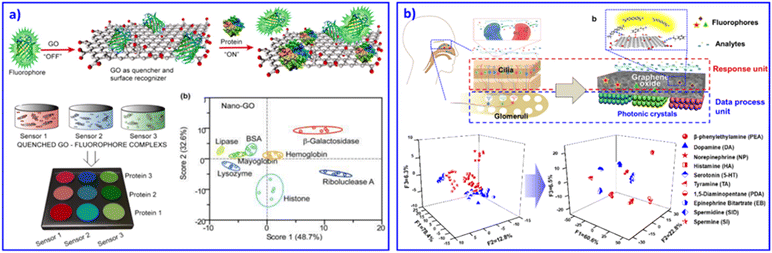 |
| Fig. 4 2D nanomaterial-based sensor arrays. (a) GO as receptors for biomolecular interactions and sensing. Fluorescent reporters were initially quenched through GO binding. Displacement of quenched fluorophore by analyte proteins would restore the fluorescence. Therefore, GO–protein interactions can be analysed and discriminated through differential fluorescent change. Reproduced with permission from ref. 82. Copyright 2012, American Chemical Society. (b) An olfactory system inspired sensor chip based on a GO and PC bilayer structure sensing system. The GO layer effectively captures the analytes, and PCs selectively enhance fluorescence signals, contributing to multi-amine recognition. Reproduced with permission from ref. 87. Copyright 2018, American Chemical Society. | |
Besides GO working as a quencher and changing the fluorescent signal in a sensing array, the conductivity property of GO also contributes to discriminating analytes. Based on inkjet printing, our group developed a wearable graphene aerogel sensing array for deformation responses.86 Through the reversible mechanical deformation induced electrical conductivity change of the wearable device, discrimination of complicated movement perceptions could be achieved. Inspired by the olfactory system, we mimicked the cilia and glomeruli to develop a GO and PC bilayer structural sensing chip to discriminate 7 drug amines.87 As shown in Fig. 4b, the GO layer captured the analytes and generated fluorescent signals based on its microporous structure. Then, PCs selectively enhanced the fluorescent signals through their periodic structure. As a result, the bilayer integrated sensing chip successfully realized the recognition of similar drug amines. Other 2D materials, such as MnO2,88 MoS2, textiles,84 and WS2 nanosheets89 were also adopted to construct sensor arrays. For example, our group developed a wearable electronic textile for human motion recognition based on commercially available polyester fabrics and silver precursors.84 Compared with GO-based devices, the polymer fabric-based devices displayed better deformation performance and much simpler fabrication by just dipping the commercial polyester fabrics into the silver precursor. This strain–electric responsive sensor displayed outstanding sensitivity, durability, and permeability, benefiting its promising applications as wearable devices for sports training, health care monitoring and so on. Based on 2D materials as blocks, the further development of comfortable wearable devices for sensing human health is strongly desired.
3. Ergonomic electronic sensor array
Current detecting devices and analysis methods for complex systems are stuck because of the bulky instruments, limited or simple response function, and complex analysis operation. The tremendous desire in clinical diagnosis and health assessment for the monitoring of vital signs calls for high-resolution discrimination and a sensitive response with good user experience. Wearable electronics is fascinating because of its advantages of convenience and non-invasiveness, which combines ergonomic contents with flexible and bio-friendly materials, multi-integration, bionic design, and a smooth human–machine interface. In the following, we will present some examples of flexible patterns, electronic textiles and graphene combinations for human symptoms or monitoring of signs. Static and dynamic basic data of body movement, and changes in humidity and temperature were detected and analyzed for earlier medical diagnosis, long-term chronic monitoring, auxiliary apparatus for people with handicaps or paraplegia, daily health assessment and so on.
3.1 Flexible patterns
Flexible electronics,90,91 dispersing conductive or semiconductive nanomaterials into elastomeric matrices,92 is blooming with the tremendous and increasing demand for integrated circuits, wearable devices and attached or embeddable intelligent instruments. Fractal and serpentine patterns are commonly adopted to separate and release stress during stretching, bending or twisting flexible operations. Generally, in order to achieve a sensitive and accurate elastomeric deformation response, flexible electronics are required to adopt arbitrary curve patterns and tunable tortuous morphologies. As shown in Fig. 5a, we assembled silver NPs into curved arrays with various curvatures via pillar-patterned template printing.73 The curves with various tortuous morphologies have differential resistive strain sensitivities, which exhibit sensitive and stable resistance responses to deformation. With multichannel monitoring of the delicate deformation of human facial expression, 6 positions on facial skin were selected. These 6 positions contained the basic muscle groups for most expressive movements. Finally, 8 main expressions (anger, disgust, fear, laughter, sadness, smiling, surprise, and a relaxed one as a control) were analyzed. Originating from the distinctive changes in resistance during facial expression, PCA and HCA statistical operations showed complete and clear classification of all 8 monitored expressions. The sensitive perception of skin movement with facial expression recognition contributes to remarkable applications in auxiliary apparatus for skin micromotion manipulation, especially for people with paraplegia.
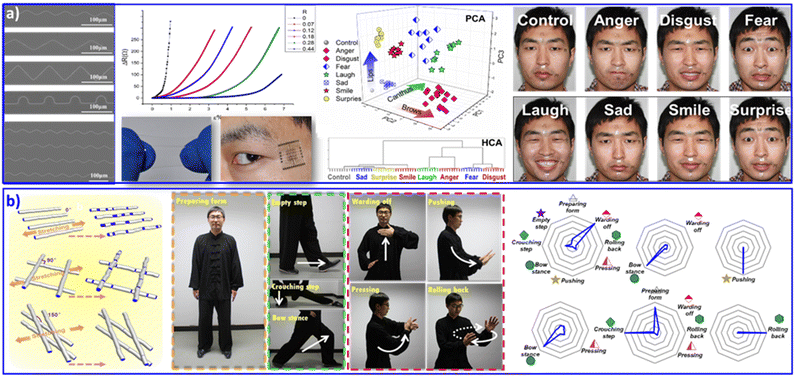 |
| Fig. 5 (a) Wearable sensing device based on silver NP assembled curved arrays, with increased electrical resistance in response to strain deformation. The wearable sensing devices were attached at six selective positions on facial skin, contributing to discriminant analysis of eight facial expressions. Reproduced with permission from ref. 73. Copyright 2016, Wiley-VCH. (b) Wearable sensing device based on a microfiber-knitted sensor array. The wearable devices were attached at the shoulders, elbows, and knees with different cross-weave angles, contributing to whole-body movement monitoring and multifactor quantitative analysis. Reproduced with permission from ref. 94. Copyright 2018, Wiley-VCH. | |
3.2 Electronic textiles
For modern detection and analysis technology, experience is recognized and acknowledged as a critical pursuit. Successful wearable devices will face burgeoning requirements in terms of multifunctionality, adaptability to complex environments and convenience of setup. Textiles, as the most successful ergonomic materials, contribute light weight, permeability, and durable features for human comfort. Textile knitting endows inherent flexibility with diverse knitting morphology to achieve integrated wearable electronic sensing instruments.93 As shown in Fig. 5b, we developed arrays of conductive microfibers and then knitted them with various cross-weave patterns to construct multiresolution flexible electronical sensors.94 The cross-weave angles of fibers knitted in various patterns exhibit tunable resistive strain sensitivity. Then, sensors with different cross-weave patterns were integrated into multiresolution wearable sensors for whole-body joint kinematic monitoring. Six microfiber-knitted sensors were attached to the human shoulder, elbow and knee joints to monitor shadowboxing movements. Another example is to construct an e-textile sensor on a commercial polyester textile via a simple and effective dyeing method. Low-viscosity and low-surface-tension ink with reactive silver ions ([Ag(NH3)2]+[C2H3O2]−) was adopted in this case.95 The stable electronic dyeing and various textile knitting morphologies respond to specific differentials of imperceptible movements. Therefore, multi-analyte investigation results in delicate distinctions for the experimenter undertaking the same action. E-textiles with multipoint sensing and complicated data analysis could precisely perceive or distinguish the complexity and similarity of human motions, displaying potential applications in movement-related health status and clinical practice.
3.3 Graphene combinations
Graphene, as an excellent 2D material that combines many advantages, such as conductivity,96 thermal conductivity97 and flexibility,98 can be easily processed into different morphological nanostructures, including ultrathin films,99 nanosheets,100 paper,101 fiber,102 nanoribbons,103 foams104 and sponges.105 The response diversity and mechanical flexibility of graphene materials has contributed to flexible sensitive sensors for collecting a large amount of valid data.106 Meanwhile, qualitative or quantitative ergonomic measurement results were also obtained through multivariate analysis. For example, Ryhanen et al. designed an ultra-fast graphene film as a human body humidity and temperature sensor.107
Through PCA analysis, different whistled songs can also be qualitatively identified. Based on 3D microstructures of graphene aerogel, we printed GO aerogel patterns to monitor human motions.86 The 3D architectures of reduced graphene oxide (rGO) can respond to complex mechanical stimulations and achieve multi-gesture recognition (Fig. 6a). Although graphene aerogel contributes to a sensitive flexible response, its performance is still a big obstacle because of its high resistance. Porous structures will result in poor electron transfer between graphene sheets, and the laminar assembled graphene will bring about much higher electron transfer efficiency. Various assembly structures exhibit different electrical behavior with unitary graphene material. Their improved progress combines laminar graphene and aerogel graphene in an integrated sensing device. The porous-structured graphene pattern sensitively responds to micromovements, and the laminar graphene pattern behaves as a good conductor to transmit the sensing signal smoothly. Touch-sensitive graphene skin can perform clear multiresolution orientation, locational kinesis tracking and tactile-sensing control (Fig. 6b).108 Recently, we developed graphene aerogels with porous serpentine patterns, displaying various multidimensional deformation responses.109 Then, wrist movements can be successfully recognized, as shown in Fig. 6c. The graphene skin developed by our group showed promising adoptability in the future multifunctional integration of electronics.109 The introduction of multi-analysis technology enables efficient discrimination of various and complex data. Multi-analyte detection of complex environments enables sensor components with high sensitivity, fast response, and low detection limits for high-resolution signal reception and efficient transmission.110
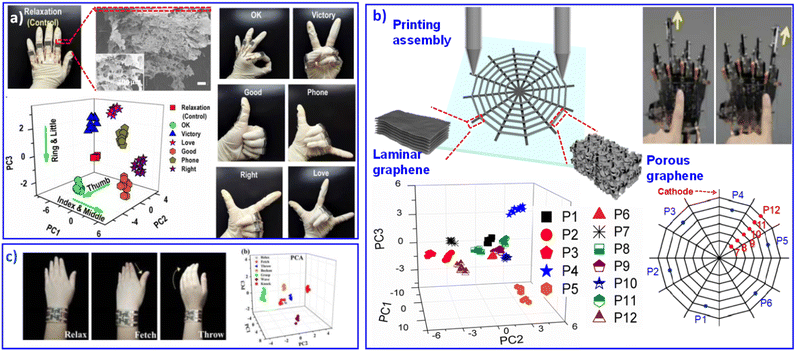 |
| Fig. 6 Flexible sensors based on graphene-combination materials. (a) Printed rGO aerogel sensor with multidimensional motion response for gesture recognition. Reproduced with permission from ref. 86. Copyright 2016, Royal Society of Chemistry. (b) Touch-sensitive rGO skin with different microstructures to balance stimulated response and signal transmission. Reproduced with permission from ref. 108. Copyright 2018, Royal Society of Chemistry. (c) Graphene aerogels with porous serpentine patterns for recognizing wrist movements. Reproduced with permission from ref. 109. Copyright 2019, Royal Society of Chemistry. | |
4. Device integration
In previous sections, we discussed nanomaterial-based multi-analyte bio/chemical sensing arrays, as well as design and assembly strategies for wearable electronics with unique electrical and mechanical properties for the monitoring of multiple vital signs. Additionally, the integration of circuits and systems opens up tremendous opportunities for multitarget sensing applications spanning bio/chemical sensing, stimulation, to clinical pathology, diagnosis and therapy. In this section, examples of such integrated systems in the forms of hand-held devices and multifunctional skin are reviewed, and the latest developments in AI-assisted data analysis methods are also discussed.
4.1 Hand-held devices
The development of fundamental research into sensing array systems calls for the generation of user-friendly, highly sensitive, inexpensive and disposable sensing devices. Various materials, such as paper-based,111 organic field effect based,112 and gel based113 sensing arrays were developed as integrated sensing devices for reliable qualitative and quantitative analyses. However, the above-mentioned instruments still require a digital reader to form on-site analytical devices. For truly hand-held multitarget sensing devices, Suslick's group combined an optoelectronic reader and an advanced optical sensor array to develop a series of hand-held analytical devices for on-site collection and analysis, including explosives,114 biomarkers,115 and food.116 Hand-held devices with reading colorimetric sensor arrays were based on digital imaging technology, such as contact image sensors (CISs), flatbed scanners, digital cameras, and cell phones. For example, CISs usually contain a linear complementary metal-oxide semiconductor sensor array for optical transduction and colorimetric data rapid collection, as shown in Fig. 7a.117 The detectors could receive light reflected off the object and then produce an image. Software installed in this hand-held device would process the data by comparing and analyzing each spot's color values through red, green and blue values. Based on different color values, statistical and quantitative analyses can be performed, which finally contribute to fast, sensitive, on-site sensing. For example, the quantification of urinary trimethylamine N-oxide (Fig. 7b),118 monitoring CO and ethylene (Fig. 7c),119 and examining trimethylamine from mouth and skin odor through a cell phone camera, were achieved.115 This portable sensing of vapors and liquids at ppb concentrations shows particular potential in point-of-care medical monitoring and diagnosis.
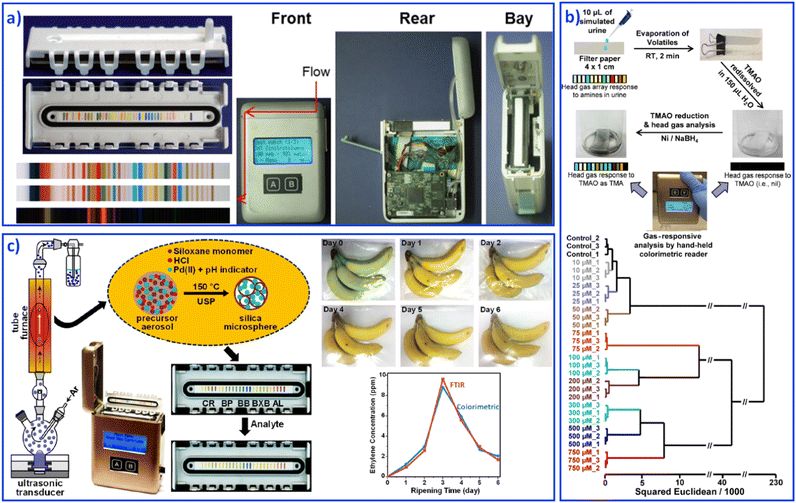 |
| Fig. 7 Hand-held devices containing colorimetric sensor arrays. (a) Structure of hand-held devices. Reproduced with permission from ref. 117. Copyright 2015, American Chemical Society. (b) Quantification of urinary trimethylamine N-oxide. Reproduced with permission from ref. 118. Copyright 2018, American Chemical Society. (c) Monitoring CO and ethylene. Reproduced with permission from ref. 119. Copyright 2019, American Chemical Society. | |
4.2 Multifunctional skin
Continuous efforts have resulted in the development of flexible/stretchable physical and bio/chemical sensors that are capable of sensing electrophysiological signals (e.g., electrocardiography,120 electromyography121), physiological signals (e.g., pulses,122 temperature123,124), and bio/chemical parameters (e.g., glucose125), paving the way toward the realization of multifunctional skin-like electronic systems.27 Therefore, we include advances in thin-film electronic systems, which enable high-performance monitoring of human activities, personal healthcare, and/or interfacing with machines. For example, inflammation-free, highly gas-permeable, ultrathin, lightweight and stretchable sensors with an Au-coated conductive nanomesh structure can be directly attached to irregular human skin for long-term effective performance. Due to the asymmetric interlocking geometry, the bioinspired e-skin is capable of real-time measurement and discrimination of normal and shear forces, with increased sensitivity, minimal hysteresis, excellent cycling stability, and response time in the millisecond range. The e-skin provided sensing feedback for controlling a robot arm during various tasks, illustrating its potential application in robotics with tactile feedback. Improved sensor characteristics in terms of spatial resolution and sensitivity, as well as the uniformity of the array, can be achieved by integrating functional sensors into active matrices. Through this approach, more delicate e-skin sensors with spatial mapping capability could be developed.
In addition to wearable sensors capable of tracking the user's physical activities and vital signs, continuous monitoring of bio/chemical parameters is of great importance to providing insight into the user's state of health at the molecular level. Measurements of human sweat, which contains rich physiological and metabolic information, could enable such insight non-invasively. Given the complexity of sweat secretions, simultaneous and multiplexed screening of target biomarkers is critical and requires full system integration to ensure the accuracy of measurements.28,126 Javey and co-workers developed a wearable fully integrated sensor array (FISA) for multiplexed in situ perspiration analysis, which simultaneously and selectively screens a panel of biomarkers in sweat, including multiple sweat metabolites (glucose and lactate), electrolytes (sodium and potassium), as well as skin temperature (Fig. 8).28 By integrating the FISA and a wireless flexible printed circuit board (FPCB), the wearable system integrated signal transduction, conditioning, processing and wireless transmission for the realization of a practical wearable sensor device. The system was applied to measure the detailed sweat profile of human subjects engaged in prolonged indoor and outdoor physical activities, and to make a real-time assessment of the physiological state of the subjects. The further integration of electrochemical sensors with energy modules with self-powered capability achieved noninvasive sweat monitoring, and real-time signal processing/display in a single platform.126
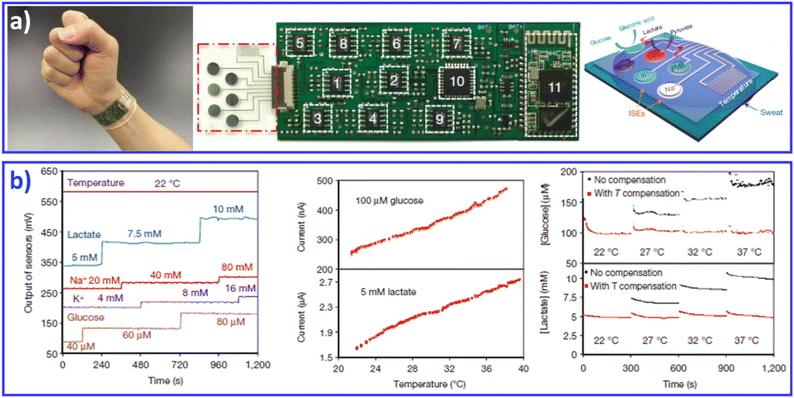 |
| Fig. 8 A FISA integrating a multiplexed sweat sensor array and a wireless flexible printed circuit board FPCB for the simultaneous and selective screening of a panel of biomarkers in sweat. (a) Images and schematic illustrations of the FISA. (b) System-level characterization of the sensor array. Reproduced with permission from ref. 28. Copyright 2016, Nature Publishing Group. | |
5. Artificial intelligence assisted perception
Realistic environments or in vivo samples are inherently endowed with the complexity of multiple variables and multiple features. A complete analysis will certainly yield a tremendous amount of data. In addition to performing a fast and portable response, most wearable sensing devices compromise on sensitivity or accuracy. Progress in artificial intelligence (AI) contributes convenient approaches to emancipate us from tedious work in data processing. Meyer and collaborators have endeavored to provide odor molecule recognition via machine-learning algorithms.29 They collected a psychophysical dataset from 49 individuals with profiling of 476 structurally and perceptually diverse molecules, and then quantized 4884 physicochemical features, including atom types and functional groups, as well as topological and geometrical properties. Using a large olfactory psychophysical dataset, the authors developed machine-learning algorithms to predict sensory attributes of molecules based on their chemoinformatic features. Random-forest and regularized linear models exhibit exceptionally higher performances than other common predictive model types, while tracking the observed perceptual values. This results in all predictions being reflected in a hidden test set, avoiding the pitfall of inflated correlations due to overfitting of the experimental data. The AI-assisted massive data processing and attributive correlation analysis could be run for reverse-engineering of desired perceptual profiles, to identify certain molecules from vast chemoinformatic databases and closely approach the theoretical limits of accuracy from individual variability. Recently, Tomono's group combined nanopores with machine learning to recognize four coronaviruses with high sensitivity.127 This simple procedure does not require a reverse transcription-polymerase chain reaction (RT-PCR), demonstrating the great potential applications of AI-assisted sensing devices.
The complexity and multivariate nature of biological and environmental systems are critical challenges for a high-throughput sensing methods and multianalyte identification. Deep learning (DL) algorithms present a big advantage in analyzing multidimensional data and nonlinear operation. Through efficient qualitative and quantitative contract analysis of the ten typical machine-learning algorithms, naïve-Bayes (NB), linear discriminant analysis (LDA), logistic-regression (LR), decision-tree (DT), random-forest (RF), support-vector-machine (SVM), K-nearest neighbors (KNN), extreme gradient boosting (XGBoost), multilayer perceptron (MLP) and convolutional neural network (CNN), we can conclude that the DL algorithm CNN could realize the clearer prediction of various analytes (biogenic amines, aminoglycoside antibiotics etc.), with 100% accuracy and multicomponent quantification (Fig. 9a).128,129 For example, the classification of six aminoglycoside antibiotics (AGs) was first performed through three-dimensional LDA, as shown in Fig. 9b. Then, CNN regression models were constructed to predict the concentration of AGs. The histogram of the error distribution shows many predictions with an error of ±2.5 mg L−1 (Fig. 9c). CNN demonstrated greater confidence and convenience than the other non-deep-learning algorithms to distinguish various analytes with clear fingerprint images.
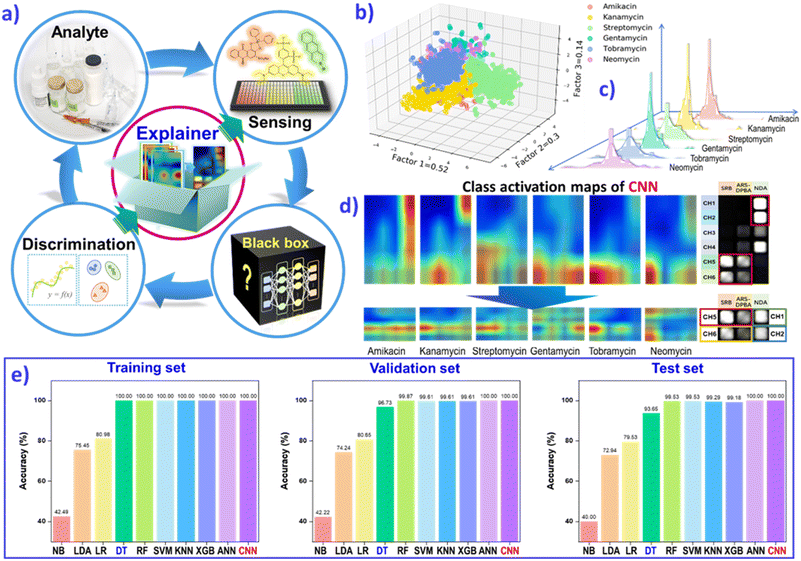 |
| Fig. 9 (a) The feedback mechanism of explainable deep learning (DL) guides sensor array evolution to use less material, for simplified operation or for efficient data acquisition. (b) The LDA classification plot for 6 AGs. (c) The prediction error distribution of the test set. (d) The class activation maps of AGs based on the result of the CNN model, and class activation maps of six AGs based on the result of the rebuilt CNN model after feature extraction. (e) Comparison of prediction accuracies for the AG category by employing different classification models, including NB, LDA, LR, DT, RF, SVM, KNN, XGB, MLP and CNN, on the training set, validation set and test set. The resulting assessment will guide the sensor array evolution, and approach an “end-to-end” strategy. Reproduced with permission from ref. 129. Copyright 2022, ACS publishing group. | |
However, for most machining-learning, especially DL, there is one puzzling cloud hanging over algorithm operations. The data-driven black boxes suffer from non-transparent inner workings. A feedback mechanism is required to explain the detailed feature extraction, and to guide the evolution of the sensor array to use less material, for simplified operation or for efficient data acquisition. Class-activation mapping assessment has been developed to explain how the CNN model assesses the importance of sensor elements and to make discrimination decisions (Fig. 9d and e). The explainable DL assisted analysis method establishes an “end-to-end” strategy, to ascertain the black-box of the DL algorithm and promote hardware design or principal optimization. The explainable AI strategy can offer a feedback mechanism to verify the design of the sensor unit, and optimize sensor devices. For future wearable platforms, the programmable sensing chip may be normalized to meet the tremendous complexity and multivariate nature of advanced health monitoring and point-of-care diagnosis, and even new scientific discoveries.
6. Summary and outlook
Multi-analyte discrimination has been well developed over past decades, and is still rapidly growing in terms of both sensing materials and analytical techniques. Sensor arrays have demonstrated their usefulness as mainstream tools in addressing a wide range of analytical challenges. In particular, from this review, it is apparent that micro/nanotechnology-based sensor arrays provide powerful platforms for various serials of multi-analyte discrimination. Through a combination of sensor arrays and nanotechnology, high-density sensor arrays could be miniaturized to the nanoscale. Meanwhile, new chemical and physical properties would also accompany the micro/nanometer effect, which will provide reliable sensing processes with novel sensing principles and mechanisms. For instance, facile and commercial sensor arrays combined with nanostructural substrates could replace serials of derivatives as sensors, avoiding complex rational design and fabrication processes for sensors. In addition, various sensing signals, including color, fluorescence, and resistivity, could also be adopted in a dataset for high-throughput statistical analysis. The excavation or extension of sensing signals will tremendously augment and expand the featured data matrix for discriminatory analysis with higher resolution. The advanced mathematical operation of machine-learning has been adopted to analyse extremely complex systems. Therefore, besides molecules, ions, and biomolecules as analysis targets in traditional sensor arrays, a large number of similar targets could be considered as analytes, such as transformation positions, human motions, human facial expressions, and human olfactory perception. These novel targets would greatly extend the application areas of sensor arrays in differential detection.
Although much progress has been achieved in recent years, great challenges still remain in micro–nanostructural sensor arrays with miniaturization, integration and high sensitivity, especially customizable features for the target-oriented discrimination of a broad spectrum of multiple analytes. Based on the bottom layer of the response data matrix and cross-reactive operation, three promoted strategies could be summarized: (1) creating material diversity, (2) designing the organization of a sensor array structure, and (3) integrating multifunctional devices. The excellent optical or electronical properties on nanoparticles, nanofibers or nanomatrices contribute significant sensitivity and selectivity for traditional or novel sensing processes. Ingenious and well-organized or assembled materials to design structures, can endow them with particular response features for ergonomic analysis. Device integration can collect various functions to match practical requirements. With the rational design of micro–nanostructural sensor arrays, responsive materials after binding with target analytes could be introduced into sensor arrays as flat forms to achieve chemical and physical property tunability. The adoption of deep-learning (DL) algorithms will contribute a big advantage to analysing nonlinear, multidimensional and massive data. But most DL models are confronted with a “black-box” dilemma: the non-transparency of neural networks. By opening the “black-box” and pointing out the connection between input and output, the explanatory AI analysis method can establish an end-to-end strategy. Ascertaining the activation and decision-making mechanisms of the AI algorithm can help to assess the efficiency of sensor units, which will promote hardware design, principle optimization, even new scientific discoveries. In the end, there are still big challenges to the performance of micro–nanostructural sensor arrays in practical applications, such as the lack of a corresponding platform, signal singularity, the inconvenience of information extraction, integration with wearable devices, and an incomplete system. Especially for on-site application, a sensor array's long-term durability and mechanical stability still need improvement. On the other hand, since sensor arrays and multi-analyte discrimination have been demonstrated, their applications in areas related to the monitoring of human health, such as blood, urine, sweat, and motion, the construction of sensor arrays on wearable devices with biocompatibility and bioactive functions should be taken into consideration to adapt the tendency of wearable or implantable intelligent devices.
Author contributions
The manuscript was written through contributions of all authors. All authors have given approval to the final version of the manuscript.
Conflicts of interest
There are no conflicts to declare.
Acknowledgements
This work is supported by the National Natural Science Foundation of China (21874056, 22176180, 22090050, 21874121, 21525523, 21974128, 21722507, and 52003103), the National Key R&D Program of China (2021YFA1200400, 2018YFE0206900, 2017YFA0208000), Zhejiang Provincial Natural Science Foundation of China under Grant No. LY20B050002, LD21B050001, and the Science and Technology Foundation of State Key Laboratory JZX7Y201901SY007301.
Notes and references
- Z. Ma, D. Kong, L. Pan and Z. Bao, J. Semicond., 2020, 41, 041601 CrossRef CAS.
- Y. Gong, Y.-Z. Zhang, S. Fang, C. Liu, J. Niu, G. Li, F. Li, X. Li, T. Cheng and W. Lai, Appl. Phys. Rev., 2022, 9, 021403 CAS.
- Z. Ren, D. Qi, P. Sonar and Z. Wei, J. Semicond., 2020, 41, 040402 CrossRef.
- D. Li, W.-Y. Lai, Y. Zhang and W. Huang, Adv. Mater., 2018, 30, 1704738 CrossRef PubMed.
- J. Park, J. Kim, S. Y. Kim, W. H. Cheong, J. Jang, Y. G. Park, K. Na, Y. T. Kim, J. H. Heo, C. Y. Lee, J. H. Lee, F. Bien and J. U. Park, Sci. Adv., 2018, 4, eaap9841 CrossRef PubMed.
- P. Tseng, B. Napier, L. Garbarini, D. L. Kaplan and F. G. Omenetto, Adv. Mater., 2018, 30, 1703257 CrossRef PubMed.
- M. Bariya, H. Y. Y. Nyein and A. Javey, Nat. Electron., 2018, 1, 160–171 CrossRef.
- H. Lee, C. Song, Y. S. Hong, M. S. Kim, H. R. Cho, T. Kang, K. Shin, S. H. Choi, T. Hyeon and D. H. Kim, Sci. Adv., 2017, 3, e1601314 CrossRef PubMed.
- W. Liu, L. Shang, F. Zheng, J. Lu, J. Qian, Y. Zhao and Z. Gu, Small, 2014, 10, 88–93 CrossRef CAS PubMed.
- H. Pei, J. Li, M. Lv, J. Wang, J. Gao, J. Lu, Y. Li, Q. Huang, J. Hu and C. Fan, J. Am. Chem. Soc., 2012, 134, 13843–13849 CrossRef CAS PubMed.
- A. Grinthal and J. Aizenberg, Chem. Soc. Rev., 2013, 42, 7072–7085 RSC.
- J. Cui, W. Zhu, N. Gao, J. Li, H. Yang, Y. Jiang, P. Seidel, B. J. Ravoo and G. Li, Angew. Chem., Int. Ed., 2014, 53, 3844–3848 CrossRef CAS PubMed.
- S. H. Lim, L. Feng, J. W. Kemling, C. J. Musto and K. S. Suslick, Nat. Chem., 2009, 1, 562–567 CrossRef CAS PubMed.
- Z. Xie, K. Cao, Y. Zhao, L. Bai, H. Gu, H. Xu and Z. Gu, Adv. Mater., 2014, 26, 2413–2418 CrossRef CAS PubMed.
- R. H. Perry, M. Splendore, A. Chien, N. K. Davis and R. N. Zare, Angew. Chem., Int. Ed., 2011, 50, 250–254 CrossRef CAS PubMed.
- Y. Yang, S. K. Seidlits, M. M. Adams, V. M. Lynch, C. E. Schmidt, E. V. Anslyn and J. B. Shear, J. Am. Chem. Soc., 2010, 132, 13114–13116 CrossRef CAS PubMed.
- J. J. Lavigne and E. V. Anslyn, Angew. Chem., Int. Ed., 2001, 40, 3118–3130 CrossRef CAS PubMed.
- Y. Geng, W. J. Peveler and V. M. Rotello, Angew. Chem., Int. Ed., 2019, 58, 5190–5200 CrossRef CAS PubMed.
- K. Persaud and G. Dodd, Nature, 1982, 299, 352–355 CrossRef CAS PubMed.
- W. Xu, C. Ren, C. L. Teoh, J. Peng, S. H. Gadre, H.-W. Rhee, C.-L. K. Lee and Y. -T. Chang, Anal. Chem., 2014, 86, 8763–8769 CrossRef CAS PubMed.
- Z. Wang, M. A. Palacios and P. Anzenbacher, Anal. Chem., 2008, 80, 7451–7459 CrossRef CAS PubMed.
- W. Chen, Q. Li, W. Zheng, F. Hu, G. Zhang, Z. Wang, D. Zhang and X. Jiang, Angew. Chem., Int. Ed., 2014, 53, 13734–13739 CrossRef CAS PubMed.
- J. Chen, S. M. Andler, J. M. Goddard, S. R. Nugen and V. M. Rotello, Chem. Soc. Rev., 2017, 46, 1272–1283 RSC.
- C. Zhou, W. Xu, P. Zhang, M. Jiang, Y. Chen, R. T. K. Kwok, M. M. S. Lee, G. Shan, R. Qi, X. Zhou, J. W. Y. Lam, S. Wang and B. Tang, Adv. Funct. Mater., 2019, 29, 1805986 CrossRef.
- C. Liu, J. Zhao, F. Tian, J. Chang, W. Zhang and J. Sun, J. Am. Chem. Soc., 2019, 141, 3817–3821 CrossRef CAS PubMed.
- N. D. B. Le, G. Y. Tonga, R. Mout, S. T. Kim, M. E. Wille, S. Rana, K. K. Dunphy, D. J. Jerry, M. Yazdani, R. Ramanathan, C. M. Rotello and V. M. Rotello, J. Am. Chem. Soc., 2017, 139, 8008–8012 CrossRef CAS PubMed.
- T. Someya and M. Amagai, Nat. Biotechnol., 2019, 37, 382–388 CrossRef CAS PubMed.
- W. Gao, S. Emaminejad, H. Y. Y. Nyein, S. Challa, K. V. Chen, A. Peck, H. M. Fahad, H. Ota, H. Shiraki, D. Kiriya, D. H. Lien, G. A. Brooks, R. W. Davis and A. Javey, Nature, 2016, 529, 509–514 CrossRef CAS PubMed.
- A. Keller, R. C. Gerkin, Y. Guan, A. Dhurandhar, G. Turu, B. Szalai, J. D. Mainland, Y. Ihara, C. W. Yu, R. Wolfinger, C. Vens, L. Schietgat, K. De Grave, R. Norel, G. Stolovitzky, G. A. Cecchi, L. B. Vosshall, P. Meyer and D. O. Prediction, Science, 2017, 355, 820–826 CrossRef CAS PubMed.
- Y. Liu, T. Minami, R. Nishiyabu, Z. Wang and P. Anzenbacher, J. Am. Chem. Soc., 2013, 135, 7705–7712 CrossRef CAS PubMed.
- X. Zhang, L. You, E. V. Anslyn and X. Qian, Chem.–Eur. J., 2012, 18, 1102–1110 CrossRef CAS PubMed.
- C. Choi, Y. Lee, K. W. Cho, J. H. Koo and D. H. Kim, Acc. Chem. Res., 2019, 52, 73–81 CrossRef CAS PubMed.
- Q. Wang and L. Wang, Nanoscale, 2020, 12, 7485–7499 RSC.
- P. Anzenbacher, P. Lubal, P. Bucek, M. A. Palacios and M. E. Kozelkova, Chem. Soc. Rev., 2010, 39, 3954–3979 RSC.
- J.-S. Moon, W.-G. Kim, D.-M. Shin, S.-Y. Lee, C. Kim, Y. Lee, J. Han, K. Kim, S. Y. Yoo and J.-W. Oh, Chem. Sci., 2017, 8, 1665 RSC.
- J. Tropp, M. H. Ihde, A. K. Williams, N. J. White, N. Eedugurala, N. C. Bell, J. D. Azoulay and M. Bonizzoni, Chem. Sci., 2019, 10, 10247–10255 RSC.
- S. Tomita, M. Sakao, R. Kurita, O. Niwa and K. Yoshimoto, Chem. Sci., 2015, 6, 5831–5836 RSC.
- P. Qin, S. Okur, C. Li, A. Chandresh, D. Mutruc, S. Hecht and L. Heinke, Chem. Sci., 2021, 12, 15700–15709 RSC.
- P. Zhang, X. Zhou and Y. Zeng, Chem. Sci., 2019, 10, 5495–5504 RSC.
- Y. Huang, F. Li, C. Ye, M. Qin, W. Ran and Y. Song, Sci. Rep., 2015, 5, 9724 CrossRef CAS PubMed.
- E. Bardez, I. Devol, B. Larrey and B. Valeur, J. Phys. Chem. B, 1997, 101, 7786–7793 CrossRef CAS.
- A. D. Gill, B. L. Hickey, W. Zhong and R. J. Hooley, Chem. Commun., 2020, 56, 4352–4355 RSC.
- J. Chen, B. L. Hickey, L. Wang, J. W. Lee, A. D. Gill, A. Favero, R. Pinalli, E. Dalcanale, R. J. Hooley and W. Zhong, Nat. Chem., 2021, 13, 488–495 CrossRef CAS PubMed.
- Y. Qi, W. Xu, R. Kang, N. Ding, Y. Wang, G. He and Y. Fang, Chem. Sci., 2018, 9, 1892–1901 RSC.
- Q. Lin, T.-T. Lu, X. Zhu, T.-B. Wei, H. Li and Y.-M. Zhang, Chem. Sci., 2016, 7, 5341–5346 RSC.
- G. von Freymann, V. Kitaev, B. V. Lotschz and G. A. Ozin, Chem. Soc. Rev., 2013, 42, 2528–2554 RSC.
- F. Wu, Y. Huang, X. Yang, J.-J. Hu, X. Lou, F. Xia, Y. Song and L. Jiang, Anal. Chem., 2021, 93, 16257–16263 CrossRef CAS PubMed.
- B. Gao, Z. He, B. He and Z. Gu, Sens. Actuators, B, 2019, 288, 734–741 CrossRef CAS.
- J. Liao, C. Ye, J. Guo, C. E. Garciamendez-Mijares, P. Agrawal, X. Kuang, J. O. Japo, Z. Wang, X. Mu, W. Li, T. Ching, S. M. Luis, C. Zhu, X. Zhang, Z. Gu and Y. S. Zhang, Mater. Today, 2022, 56, 29–41 CrossRef CAS.
- K. Liu, H. Ding, S. Li, Y. Niu, Y. Zeng, J. Zhang, X. Du and Z. Gu, Nat. Commun., 2022, 13, 4563 CrossRef CAS PubMed.
- J. Chi, D. Wu, M. Su and Y. Song, Sci. Bull., 2022, 67, 1191–1193 CrossRef CAS PubMed.
- X.-Y. Wang, K.-D. Zhu, J. Zhu and S.-N. Ding, Anal. Chem., 2021, 93, 2959–2967 CrossRef CAS PubMed.
- E. Yablonovitch, Phys. Rev. Lett., 1987, 58, 2059–2062 CrossRef CAS PubMed.
- A. Richel, N. P. Johnson and D. W. McComb, Appl. Phys. Lett., 2000, 76, 1816–1818 CrossRef CAS.
- L. Cui, W. Shi, J. Wang, Y. Song, H. Ma and L. Jiang, Anal. Methods, 2010, 2, 448–450 RSC.
- H. Li, J. Wang, L. Yang and Y. Song, Adv. Funct. Mater., 2008, 18, 3258–3264 CrossRef CAS.
- E. Tian, J. Wang, Y. Zheng, Y. Song, L. Jiang and D. Zhu, J. Mater. Chem., 2008, 18, 1116–1122 RSC.
- M. Li, F. He, Q. Liao, J. Liu, L. Xu, L. Jiang, Y. Song, S. Wang and D. Zhu, Angew. Chem., Int. Ed., 2008, 47, 7258–7262 CrossRef CAS PubMed.
- C.-S. Huang, S. George, M. Lu, V. Chaudhery, R. M. Tan, R. C. Zangar and B. T. Cunningham, Anal. Chem., 2011, 83, 1425–1430 CrossRef CAS PubMed.
- C.-S. Huang, V. Chaudhery, A. Pokhriyal, S. George, J. Polans, M. Lu, R. M. Tan, R. C. Zangar and B. T. Cunningham, Anal. Chem., 2012, 84, 1126–1133 CrossRef CAS PubMed.
- L. Bai, Z. Xie, K. Cao, Y. Zhao, H. Xu, C. Zhu, Z. Mu, Q. Zhong and Z. Gu, Nanoscale, 2014, 6, 5680–5685 RSC.
- X. Hu, J. Huang, W. Zhang, M. Li, C. Tao and G. Li, Adv. Mater., 2008, 20, 4074–4078 CrossRef CAS.
- Y. Zhang, Q. Fu and J. Ge, Nat. Commun., 2015, 6, 7510 CrossRef CAS PubMed.
- L. D. Bonifacio, D. P. Puzzo, S. Breslav, B. M. Willey, A. McGeer and G. A. Ozin, Adv. Mater., 2010, 22, 1351–1354 CrossRef CAS PubMed.
- D. Xu, W. Zhu, C. Wang, T. Tian, J. Cui, J. Li, H. Wang and G. Li, Chem.–Eur. J., 2014, 20, 16620–16625 CrossRef CAS PubMed.
- Y. Huang, F. Li, M. Qin, L. Jiang and Y. Song, Angew. Chem., Int. Ed., 2013, 52, 7296–7299 CrossRef CAS PubMed.
- I. B. Burgess, L. Mishchenko, B. D. Hatton, M. Kolle, M. Loncar and J. Aizenberg, J. Am. Chem. Soc., 2011, 133, 12430–12432 CrossRef CAS PubMed.
- M. Qin, Y. Huang, Y. Li, M. Su, B. Chen, H. Sun, P. Yong, C. Ye, F. Li and Y. Song, Angew. Chem., Int. Ed., 2016, 55, 6911–6914 CrossRef CAS PubMed.
- Y. Huang, L. Liu, X. Yang, X. Zhang, B. Yan, L. Wu, P. Feng, X. Lou, F. Xia, Y. Song and F. Li, Small, 2021, 17, 2006723 CrossRef CAS PubMed.
- W. Zhang, N. Gao, J. Cui, C. Wang, S. Wang, G. Zhang, X. Dong, D. Zhang and G. Li, Chem. Sci., 2017, 8, 6281–6289 RSC.
- H. Xu, Y. Lu, J. Xiang, M. Zhang, Y. Zhao, Z. Xie and Z. Gu, Nanoscale, 2018, 10, 2090–2098 RSC.
- J. Sun, Y. Lu, L. He, J. Pang, F. Yang and Y. Liu, TrAC, Trends Anal. Chem., 2020, 122, 115754 CrossRef CAS.
- M. Su, F. Li, S. Chen, Z. Huang, M. Qin, W. Li, X. Zhang and Y. Song, Adv. Mater., 2016, 28, 1369–1374 CrossRef CAS PubMed.
- M. C. McAlpine, H. Ahmad, D. Wang and J. R. Heath, Nat. Mater., 2007, 6, 379–384 CrossRef CAS PubMed.
- Y. Lu, C. Partridge, M. Meyyappan and J. Li, J. Electroanal. Chem., 2006, 593, 105–110 CrossRef CAS.
- S. Freddi, A. V. Emelianov, I. I. Bobrinetskiy, G. Drera, S. Pagliara, D. S. Kopylova, M. Chiesa, G. Santini, N. Mores, U. Moscato, A. G. Nasibulin, P. Montuschi and L. Sangaletti, Adv. Healthcare Mater., 2020, 9, 2000377 CrossRef CAS PubMed.
- L. Guo, Z. Yang and X. Dou, Adv. Mater., 2017, 29, 1604528 CrossRef PubMed.
- P. Anzenbacher, F. Li and M. A. Palacios, Angew. Chem., Int. Ed., 2012, 51, 2345–2348 CrossRef CAS.
- X. Jiang, H. Gao, X. Zhang, J. Pang, Y. Li, K. Li, Y. Wu, S. Li, J. Zhu, Y. Wei and L. Jiang, Nat. Commun., 2018, 9, 3799 CrossRef PubMed.
- H. Ji, Y. Tang, B. Shen, X. Qian, Z. Cai, F. Li, M. Su, L. Wu, Y. Ma and Y. Song, ACS Appl. Mater. Interfaces, 2021, 13, 60689–60696 CrossRef CAS PubMed.
- H. Yang, T. Xue, F. Li, W. Liu and Y. Song, Adv. Mater. Technol., 2019, 4, 1800574 Search PubMed.
- S. S. Chou, M. De, J. Luo, V. M. Rotello, J. Huang and V. P. Dravid, J. Am. Chem. Soc., 2012, 134, 16725–16733 CrossRef CAS PubMed.
- S. Tomita, A. Matsuda, S. Nishinami, R. Kurita and K. Shiraki, Anal. Chem., 2017, 89, 7818–7822 CrossRef CAS PubMed.
- Y. Li, Y. Li, M. Su, W. Li, Y. Li, H. Li, X. Qian, X. Zhang, F. Li and Y. Song, Adv. Electron. Mater., 2017, 3, 1700253 CrossRef.
- M. Lin, W. Li, Y. Wang, X. Yang, K. Wang, Q. Wang, P. Wang, Y. Chang and Y. Tan, Chem. Commun., 2015, 51, 8304–8306 RSC.
- B. An, Y. Ma, W. Li, M. Su, F. Li and Y. Song, Chem. Commun., 2016, 52, 10948–10951 RSC.
- W. Ren, M. Qin, X. Hu, F. Li, Y. Wang, Y. Huang, M. Su, W. Li, X. Qian, K. Tang and Y. Song, Anal. Chem., 2018, 90, 6371–6375 CrossRef CAS PubMed.
- W. Huang, Y. Deng and Y. He, Biosens. Bioelectron., 2017, 91, 89–94 CrossRef CAS PubMed.
- M. S. Hizir, N. M. Robertson, M. Balcioglu, E. Alp, M. Rana and M. V. Yigit, Chem. Sci., 2017, 8, 5735–5745 RSC.
- N. Matsuhisa, X. Chen, Z. Bao and T. Someya, Chem. Soc. Rev., 2019, 48, 2946–2966 RSC.
- C. Ning, K. Dong, R. Cheng, J. Yi, C. Ye, X. Peng, F. Sheng, Y. Jiang and Z. Wang, Adv. Funct. Mater., 2021, 31, 2006679 CrossRef CAS.
- J. A. Fan, W.-H. Yeo, Y. W. Su, Y. Hattori, W. Lee, S.-Y. Jung, Y. H. Zhang, Z. J. Liu, H. Y. Cheng, L. Falgout, M. Bajema, T. Coleman, D. Gregoire, R. J. Larsen, Y. Huang and J. A. Rogers, Nat. Commun., 2014, 5, 3266 CrossRef PubMed.
- B. Nie, R. Huang, T. Yao, Y. Zhang, Y. Miao, C. Liu, J. Liu and X. Chen, Adv. Funct. Mater., 2019, 29, 1808786 CrossRef.
- M. Su, Z. Huang, F. Li, Z. Zhang, Y. Guo, Z. Cai, Y. Li, W. Li, X. Qian, Y. Li, X. Zhang and Y. Song, Adv. Mater. Technol., 2018, 3, 1800107 CrossRef.
- S. B. Walker and J. A. Lewis, J. Am. Chem. Soc., 2012, 134, 1419–1421 CrossRef CAS PubMed.
- K. S. Novoselov, V. I. Fal'ko, L. Colombo, P. R. Gellert, M. G. Schwab and K. Kim, Nature, 2012, 490, 192–200 CrossRef CAS PubMed.
- S. Chen, Q. Wu, C. Mishra, J. Kang, H. Zhang, K. J. Cho, W. Cai, A. A. Balandin and R. S. Ruoff, Nat. Mater., 2012, 11, 203–207 CrossRef CAS PubMed.
- G. Tsoukleri, J. Parthenios, K. Papagelis, R. Jalil, A. C. Ferrari, A. K. Geim, K. S. Novoselov and C. Galiotis, Small, 2009, 5, 2397–2402 CrossRef CAS PubMed.
- K. S. Kim, Y. Zhao, H. Jang, S. Y. Lee, J. M. Kim, K. S. Kim, J. H. Ahn, P. Kim, J. Y. Choi and B. H. Hong, Nature, 2009, 457, 706–710 CrossRef CAS PubMed.
- C. Zhu, S. Guo, Y. Fang and S. Dong, ACS Nano, 2010, 4, 2429–2437 CrossRef CAS PubMed.
- H. Chen, M. B. Muller, K. J. Gilmore, G. G. Wallace and D. Li, Adv. Mater., 2008, 20, 3557–3561 CrossRef CAS.
- T. Ma, H.-L. Gao, H.-P. Cong, H.-B. Yao, L. Wu, Z.-Y. Yu, S.-M. Chen and S.-H. Yu, Adv. Mater., 2018, 30, 1706435 CrossRef PubMed.
- X. Li, X. Wang, L. Zhang, S. W. Lee and H. Dai, Science, 2008, 319, 1229–1232 CrossRef CAS PubMed.
- X. Huang, K. Qian, J. Yang, J. Zhang, L. Li, C. Yu and D. Zhao, Adv. Mater., 2012, 24, 4419–4423 CrossRef CAS PubMed.
- B. -X. Zhang, Z. -L. Hou, W. Yan, Q. -L. Zhao and K. -T. Zhan, Carbon, 2017, 125, 199–206 CrossRef CAS.
- M. T. Hwang, Z. Wang, J. Ping, D. Ban, Z. C. Shiah, L. Antonschmidt, J. Lee, Y. Liu, A. G. Karkisaval, A. T. C. Johnson, C. Fan, G. Glinsky and R. Lal, Adv. Mater., 2018, 30, 1802440 CrossRef PubMed.
- S. Borini, R. White, D. Wei, M. Astley, S. Haque, E. Spigone, N. Harris, J. Kivioja and T. Ryhanen, ACS Nano, 2013, 7, 11166–11173 CrossRef CAS PubMed.
- L. Liu, Y. Huang, F. Li, Y. Ma, W. Li, M. Su, X. Qian, W. Ren, K. Tang and Y. Song, Chem. Commun., 2018, 54, 4810–4813 RSC.
- T. Xue, H. Yang, B. Shen, F. Li, M. Su, X. Hu, W. Liu and Y. Song, J. Mater. Chem. C, 2019, 7, 6317–6322 RSC.
- K. Welsher and H. Yang, Nat. Nanotechnol., 2014, 9, 198–203 CrossRef CAS PubMed.
- M. G. Caglayan, S. Sheykhi, L. Mosca and P. Anzenbacher, Chem. Commun., 2016, 52, 8279–8282 RSC.
- T. Minami, Y. Sasaki, T. Minamiki, P. Koutnik, P. Anzenbacher and S. Tokito, Chem. Commun., 2015, 51, 17666–17668 RSC.
- Q. Lin, T.-T. Lu, X. Zhu, B. Sun, Q.-P. Yang, T.-B. Wei and Y.-M. Zhang, Chem. Commun., 2015, 51, 1635–1638 RSC.
- Z. Li, W. P. Bassett, J. R. Askim and K. S. Suslick, Chem. Commun., 2015, 51, 15312–15315 RSC.
- Z. Li, H. Li, M. K. LaGasse and K. S. Suslick, Anal. Chem., 2016, 88, 5615–5620 CrossRef CAS PubMed.
- Z. Li and K. S. Suslick, ACS Sens., 2016, 1, 1330–1335 CrossRef CAS.
- J. R. Askim and K. S. Suslick, Anal. Chem., 2015, 87, 7810–7816 CrossRef CAS PubMed.
- Z. Li and K. S. Suslick, ACS Appl. Mater. Interfaces, 2018, 10, 15820–15828 CrossRef CAS PubMed.
- Z. Li and K. S. Suslick, Anal. Chem., 2019, 91, 797–802 CrossRef CAS PubMed.
- T. Kim, J. Park, J. Sohn, D. Cho and S. Jeon, ACS Nano, 2016, 10, 4770–4778 CrossRef CAS PubMed.
- H. Jin, N. Matsuhisa, S. Lee, M. Abbas, T. Yokota and T. Someya, Adv. Mater., 2017, 29, 1605848 CrossRef PubMed.
- N. Luo, W. Dai, C. Li, Z. Zhou, L. Lu, C. C. Y. Poon, S.-C. Chen, Y. Zhang and N. Zhao, Adv. Funct. Mater., 2016, 26, 1178–1187 CrossRef CAS.
- B. W. An, S. Heo, S. Ji, F. Bien and J. U. Park, Nat. Commun., 2018, 9, 2458 CrossRef PubMed.
- R. Wu, L. Ma, C. Hou, Z. Meng, W. Guo, W. Yu, R. Yu, F. Hu and X. Liu, Small, 2019, 15, 1901558 CrossRef PubMed.
- H. Lee, Y. J. Hong, S. Baik, T. Hyeon and D.-H. Kim, Adv. Healthcare Mater., 2018, 7, 1701150 CrossRef PubMed.
- H. Y. Y. Nyein, L.-C. Tai, Q. P. Ngo, M. Chao, G. B. Zhang, W. Gao, M. Bariya, J. Bullock, H. Kim, H. M. Fahad and A. Javey, ACS Sens., 2018, 3, 944–952 CrossRef CAS PubMed.
- M. Taniguchi, S. Minami, C. Ono, R. Hamajima, A. Morimura, S. Hamaguchi, Y. Akeda, Y. Kanai, T. Kobayashi, W. Kamitani, Y. Terada, K. Suzuki, N. Hatori, Y. Yamagishi, N. Washizu, H. Takei, O. Sakamoto, N. Naono, K. Tatematsu, T. Washio, Y. Matsuura and K. Tomono, Nat. Commun., 2021, 12, 3726 CrossRef CAS PubMed.
- X. Tan, Y. Ye, H. Liu, J. Meng, L. Yang and F. Li, Chin. J. Chem., 2021, 39, 609–616 CrossRef.
- X. Tan, Y. Liang, Y. Ye, Z. Liu, J. Meng and F. Li, Anal. Chem., 2022, 94, 829–836 CrossRef CAS PubMed.
|
This journal is © The Royal Society of Chemistry 2022 |