DOI:
10.1039/D2NH00331G
(Communication)
Nanoscale Horiz., 2022,
7, 1540-1552
Multiplexed molecular imaging with surface enhanced resonance Raman scattering nanoprobes reveals immunotherapy response in mice via multichannel image segmentation†
Received
13th July 2022
, Accepted 14th October 2022
First published on 20th October 2022
Abstract
Visualizing the presence and distribution of multiple specific molecular markers within a tumor can reveal the composition of its microenvironment, inform diagnosis, stratify patients, and guide treatment. Raman imaging with multiple molecularly-targeted surface enhanced Raman scattering (SERS) nanoprobes could help investigate emerging cancer treatments preclinically or enable personalized treatment assessment. Here, we report a comprehensive strategy for multiplexed imaging using SERS nanoprobes and machine learning (ML) to monitor the early effects of immune checkpoint blockade (ICB) in tumor-bearing mice. We used antibody-functionalized SERS nanoprobes to visualize 7 + 1 immunotherapy-related targets simultaneously. The multiplexed images were spectrally resolved and then spatially segmented into superpixels based on the unmixed signals. The superpixels were used to train ML models, leading to the successful classification of mice into treated and untreated groups, and identifying tumor regions with variable responses to treatment. This method may help predict treatment efficacy in tumors and identify areas of tumor variability and therapy resistance.
New concepts
Imaging multiple molecular markers in vivo is crucial for revealing therapeutic mechanisms and effecting personalized medicine. Raman imaging with surface enhanced Raman scattering nanoprobes (SERS NPs) can provide data-rich spectral images of multiple markers, owing to the NPs’ distinct spectral signatures. Here, we present a comprehensive methodology for developing, employing, and visualizing multiple molecularly targeted SERS NPs to map the tumor microenvironment. We demonstrate this technique via the in vivo administration of SERS NPs and imaging in a mouse model of immune checkpoint blockade therapy. The multi-channel images are color-coded to produce molecular maps of the tumor microenvironment, and subsequently analyzed using a super-pixel segmentation approach with supervised machine learning, to classify tumor areas as responsive or naïve to immunotherapy. This work provides a generalized guideline for multiplexed SERS imaging that may be applied to preclinical studies and personalized treatment.
|
Introduction
Molecular imaging is key to precision medicine.1 Today, ever richer images are acquired by using multiple modalities to scan a patient2 or by using multiplexed analysis methods to image many target molecules in a single sample.3 Multiplexed molecular imaging can prove especially beneficial for the advancement of new cancer treatments, such as immunotherapy. In particular, immune checkpoint blockade (ICB) therapy has great potential as cancer treatment, however, the benefit of therapy has been limited to a minority of patients.4 The ability to detect the presence of important immune cells and other crucial markers could predict treatment efficacy and elucidate immunotherapy mechanisms.5–7 By imaging multiple markers in vivo, namely immune cell infiltration and activation markers directly in the tumor, it may become possible to overcome these challenges.8 A promising approach is multiplexed IHC, where immunity-associated targets (typically immune cell infiltration) are imaged in excised fixed tumors, and the spatial distribution and colocalization of the markers have been shown to predict treatment outcome.9–11 Newer imaging techniques based on mass spectrometry, such as multiplexed ion beam imaging (MIBI), image hundreds of biomarkers on a sample.12,13 However, these techniques require excised samples, limiting their potential for in vivo imaging. A method that provides comparable features (i.e., high-resolution imaging of multiple molecular markers) while being amenable to in vivo use, will be useful for preclinical research and patient care alike.
An emerging optical imaging method has shown great promise in preclinical oncology imaging, namely Raman spectroscopy with surface enhanced Raman scattering (SERS) nanoparticles.14
The phenomenon of SERS, was discovered in the 1970s15,16 and has since proved beneficial in many fields.17 SERS nanoparticles typically used in biomedical imaging rely on a plasmonic metal core that enhances the light inelastically scattered by Raman reporter molecules adsorbed on its surface. The core is encapsulated within a silica shell, on which antibodies or other targeting moieties can be attached to produce a molecularly targeted nanoprobe. The multiplexing capacity of the technique has been known for years. Proof-of-concept imaging of 10 distinct nanoparticles (each with a distinct Raman reporter but no targeting) has been shown18 along with various examples of tagging and imaging of several targets in excised tissues.19–22 Recently, SERS-based multiplexed imaging has enjoyed renewed interest. Multiplexed imaging of 5 untargeted nanoparticles at different ratios simultaneously in vivo has been reported,23 as well as the spatial encoding of 26 distinct nanoparticles with targeting shown for 5 targets in vitro.24
Here, we report a general framework for employing the technique for imaging the tumor microenvironment. Specifically, we present how to select Raman reporter molecules for SERS nanoprobe synthesis, a quantitative way of imaging formation by color-coding the multichannel pixel-wise spectra, an example application of 8-plexed imaging in a mouse model of cancer immunotherapy, and an algorithmic approach for parsing and classifying the multiplexed images based on machine learning (ML).
Results and discussion
Raman reporters
The Raman reporter molecule is a critical component of the nanoprobe. Although a handful of commercial dyes can be employed, we pursued a systematic custom reporter synthesis approach, which can provide many new distinct Raman nanoprobes, greatly augmenting the multiplexing capacity of the platform. Chalcogenopyrylium infrared (ChPIR) dyes have been shown to be effective SERS reporter molecules.25,26 The absorbance wavelength maximum of these synthetic molecules can be tuned, as it is proportional to the molecule's length27 and sequential bathochromic shifts are produced with the incorporation of heavier chalcogens in the order of oxygen, sulfur, selenium, and tellurium.28 This versatility in tuning the absorption maxima allows for structural variety without sacrificing the resonance enhancement effect that arises from having the absorption wavelength close to the excitation wavelength.29 Variability in structure enables different Raman signatures to be incorporated into different dyes, augmenting multiplexing capacity. An additional benefit to using chalcogenopyrylium-based Raman reporters is that substituents can be introduced at the 2- and 6-positions with high affinity for the gold and silver surfaces, which are used as typical SERS substrates.30–32 With these tools at our disposal, we have produced a library of ChPIR Raman reporter molecules. The synthesis and characterization of dyes are described in the electronic supplementary information (ESI†). Their structures and UV/Vis absorbance spectra are shown in Fig. S1 (ESI†).
Raman nanoprobe characterization
To determine the best candidate dyes for the synthesis of SERS nanoprobes and produce a panel of SERS nanoparticle contrast agents, we followed a comprehensive screening approach using 20 ChPIR and 8 commercial NIR dyes – all using the same synthesis methodology33,34 with minor modifications as described in Table S1 (ESI†). After synthesis, the nanoparticles were characterized to determine morphology, stability, and yield. Transmission electron microscopy (TEM) images of the synthesized nanoparticles and their absorbance spectra are shown in Fig. S2(a) (ESI†). The wide absorbance profile of the nanoparticles allows plasmonic excitation with the 785 nm laser and intense signal, consistent with our previous work35,36 and reveals that the plasmonic core contributes most of the extinction, while the absorbance of the reporter molecules is negligible.
The SERS signal of the nanoprobes is shown in Fig. 1, after baseline subtraction, and the raw spectrum including the fluorescence background in Fig. S2(b) (ESI†). The nanoparticles were placed in a plastic 1536 well plate vertically in order of chemical similarity of the reporter molecules37 (Fig. 1a) and horizontally in increasing concentrations from left to right (Fig. 1b), and Raman scanned to establish limits of detection. We observed that not all reporters provided strong SERS signals (Fig. 1c). The signal intensity depends on many parameters, including plasmonic enhancement, the reporter affinity to the gold core, and colloidal stability of the core during silica encapsulation. Chemical similarity appears to be a predictor of signal intensity and also, it can be seen that chemically similar dyes exhibit related spectral features. The reporter dyes can be clustered into four classes, based on chemical similarity, namely, (I) commercial cyanine-type dyes with moderate to good intensities and complex spectra, (II) ChPIR dyes with high intensities and single peak in the high-wave region, (III) ChPIR dyes with weak to moderate intensities and more complex spectra, and finally (IV) ChPIR dyes with very low intensity, likely due to poor interactions with the gold substrate, e.g., zwitterionic compounds and compounds with aliphatic sidechains. Chemical similarity can help in directing the selection of reporter dyes for curating a multiplex panel, or for the rational design of new reporter molecules. The Raman intensity of the best performing nanoparticles can quantify the nanoparticle concentration over at least three orders of magnitude, at least down to about 200 femtomolar, as shown in Fig. 1d.
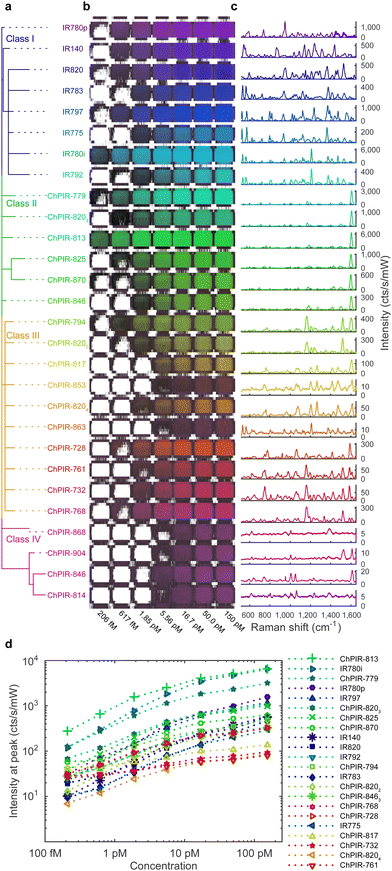 |
| Fig. 1 SERS nanoparticle library based on 8 commercially available IR dyes and 20 ChPIR dyes as Raman reporters. (a) Raman reporters are ordered by chemical similarity for a systematic approach to developing a SERS nanoparticle library, forming four classes with related signal intensity and complexity. (b) SERS nanoparticles synthesized with the 28 reporters and imaged in a standard plastic 1536 well plate at various concentrations. Compounds grouped by chemical similarity demonstrate similar spectral features and intensities. (c) Each nanoparticle type provides a uniquely identifiable spectrum, shown here after baseline subtraction and averaging of 98 point spectra. (d) Peak signal intensity for each of the 22 brightest nanoparticles at various concentrations shows the dynamic range of the SERS signal. | |
Image formation
Linear regression with a least-squares fit was used for spectral demultiplexing, as it can readily decompose a complex spectrum into its constituents based on a set of known reference spectra. Here, we use non-negative least squares (nn-LS) to assign a set of scores on each point spectrum from the image, using reference spectra derived from pure nanoparticle populations. By using the constrained (non-negative) fitting algorithm, we avoid having negative scores, as this would not be physically meaningful. This process yields a set of positive scores for every pixel indicating the contribution of each of the reference spectra as is demonstrated in Fig. 2, where SERS nanoparticles with four distinct Raman reporters were imaged in a microwell plate, in different concentrations and ratios (Fig. 2a). Here, the nn-LS scores on each point were calculated using the reference spectra shown in Fig. 2c (see Methods for preprocessing). The point-wise derived scores (Fig. 2d) show the distribution for each tag, clearly separated and quantified, as well as the background signal of the plastic plate. The intensity before spectral processing follows a clear increasing trend (Fig. 2e); the derived nn-LS scores for each dye correspond to the maximum intensity of the analyzed spectrum after baseline subtraction (Fig. 2f).
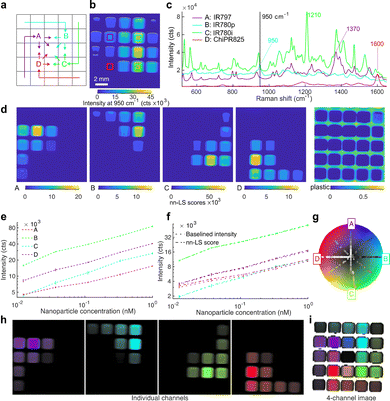 |
| Fig. 2 Spectral unmixing and visualization for multiplex Raman imaging. (a) Four SERS nanoparticle configurations featuring distinct reporter molecules were placed in a well plate, at various concentrations and ratios. (b) Raman image showing the intensity at a single wavenumber cannot separate the signals. (c) Reference spectra from pure populations used for spectral unmixing. (d) The nn-LS score obtained for each reference at each pixel unmixes the signals spatially. (e) Raw Raman intensity of representative peaks. (f) The nn-LS score for each configuration (dashed line) matches the corresponding Raman signal intensity after baseline subtraction at the characteristic peak (dotted line). (g) Radial coordinate system where the references are spaced angularly on the circumference of a modified HSV color wheel. The color of each pixel is determined based on its projection onto the color wheel coordinates. Spectra from pure populations are projected on lines from the center (low intensity) towards the direction of their reference (high intensity), whereas mixtures lie in areas between the corresponding references (e.g., blue is a 1 : 1 mixture of purple A and cyan B). The signal corresponding to “plastic” was excluded from the coordinate system. (h) Single-channel images show the signal quantitation for each nanoparticle configuration. (i) Final image showing the distribution and intensity of the four nanoparticle configurations. In the central well, an equal mixture of all four dyes yields a small vector sum (assigned to black). Pixels with signal (from the included references) below the threshold are rendered transparent. For panels (e–f) the values reported are averages of 648 spectra, with error bars showing the standard deviation from the mean. | |
To visualize multiplex images in a standardized, consistent, and meaningful fashion, we have developed a quantitative and generalizable way of color-coding the Raman signatures of the nanoprobes after spectral unmixing, where each set of scores is assigned a pseudocolor based on radially projected coordinates on a color wheel. Our method was inspired by RadViz,38 a method for visualizing similarities in samples after dimensional reduction, by projecting them onto a new radial coordinate system where basis vectors (anchors) are placed around the circumference of a circle. To generate a single image summarizing the measurement, the calculated scores for each pixel are projected onto a new coordinate system, where they are assigned a pseudocolor. The new coordinate system is circular, with radial basis vectors that correspond to any number of the reference spectra. In this way, the scores are now reduced to a 2D disk space, which can be superimposed on a color wheel (Fig. 2g). Here, we opted for a modified HSV wheel with full saturation; we used a different hue to mark each channel and the intensity to encode the magnitude of the scores. This method can be used to generate single-channel images corresponding to each tag (Fig. 2h) each with a unique color assigned to its maximum value and black for the minimum value or a single image with all the channels of interest (Fig. 2i). As the coordinate system depends on the superposition of radial vectors, diametrically opposite components add to zero. To differentiate such points from points of actually low signal, we assigned a transparency threshold, based on an absolute summation of the score magnitudes. In this way, the well-plate scan is rendered as shown in Fig. 2i, with the point projections shown on the color wheel (Fig. 2g), and the corresponding pixels colored accordingly. Channels with irrelevant information, like the plastic plate here or paraffin in histology blocks, can be seamlessly excluded from the visualization. This method goes beyond traditional methods that show only the presence of a nanoprobe or the ratio of two, and it allows quantification and visualization of mixtures.
Imaging the tumor immune microenvironment
We selected a panel of targets that can reveal information about the immune profile of a tumor, the state of the tumor cells, and their response to therapy, as well as non-specific nanoparticle uptake controls. We synthesized SERS nanoprobes functionalized with antibodies (Fig. S3, ESI†) against the following targets: CD8a (cytotoxic T lymphocytes); CD4 (helper T-cells); GITR and 4-1BB (T-cell activation/costimulatory receptors); CD11b (myeloid phagocytes implicated in immunotherapy and nanoparticle opsonization); NKp46 (natural killer cells); PD-L1 (expressed by tumor cells and implicated in PD-1 mediated immune suppression); and a non-targeted IgG isotype control. All of these markers are actively investigated in various studies of ICB.39–41 SERS nanoprobes (7 targeted + 1 untargeted control) were synthesized with reporter molecules and targeting antibodies as stated in Fig. S3 and Table S2 (ESI†). The Raman reporters were selected to yield high intensities when interrogated with the 785 nm laser, and to have sufficiently distinct spectral features to facilitate unmixing. The hydrodynamic diameter was measured via Dynamic Light Scattering (DLS) at 69 nm for the star core, at 146 ± 16.5 nm for the silicated nanoparticles (all configurations), and 166 ± 13.8 nm after antibody functionalization.
A mouse model of cancer immunotherapy was employed to test our imaging approach in a preclinical in vivo scenario. To induce the tumors, BALB/c mice were injected subcutaneously with CT26 (colon) and 4T1 (breast) murine cancer cells on opposite flanks. We selected this model because the BALB/c mice are immunocompetent and the cancer cell lines are widely available and have been used previously to assess the effects of immunotherapy.42–45 The animals were randomly divided into two groups: the treatment group received ICB treatment (anti-CTLA-4 and anti-PD-1), and the control group received sham treatment (PBS), as described in Fig. S4a (ESI†). We elected to perform the imaging before any therapy-induced reduction in tumor size was observed, to discern the initial effects of the treatment. After only two rounds of treatment, we proceeded to nanoparticle administration and imaging. Tumors from another cohort of mice, subjected to the same conditions (treatment and control) were examined via flow cytometry. The results (Fig. S4b, ESI†) suggest that early, yet not robust, changes are occurring in the activation markers (GITR, 4-1BB) and tumor expression of PD-L1.
The nanoprobes were administered to the tumor-bearing mice as a cocktail bolus and allowed to circulate overnight. The mice were subjected to Raman imaging of the tumor regions, simulating a non-invasive imaging scenario through the intact skin and an intraoperative scenario with the skin removed and the tumors imaged in situ (Fig. 3a and d). Subsequently, the tumors were excised, fixed with 4% paraformaldehyde, and embedded in paraffin. The paraffin blocks were subjected to Raman imaging at high resolution to produce Raman multiplex images (Fig. 3b and e). The SERS signal of the injected nanoprobes remained detectable in the excised tumors at least for several days after fixation. Single-channel images were compared to histologically stained sections from the same blocks (Fig. 3c and f). Our first observation was that there was no detectable nanoprobe signal from tumor areas that showed necrosis or hemorrhage. Such areas were identified by the H & E stain and were typically in the central regions of 4T1 tumors (Fig. S6, ESI†). This observation was expected, as the necrotic areas are poorly vascularized preventing nanoprobe delivery via the bloodstream; which produces imaging contrast between viable and necrotic areas of the tumor, irrespective of targeting moiety. This creates a complication when comparing the multiplexed Raman images to IHC stains: IHC stains are applied to thin tissue sections of the tumor, and, as such, are naïve to blood flow, staining necrotic and viable areas alike. This observation is exemplified in Fig. S5 (ESI†) where IHC for CD11b, for example, stains necrotic areas more strongly than viable positive areas, and for PD-L1 where practically no contrast appears between viable positive and necrotic areas. The physiological differences between the different tumor regions are clearly seen with the H & E stain. Conversely, the images obtained with our method reveal the presence of the target molecules in the viable regions alone, without confounding signals from necrotic areas.
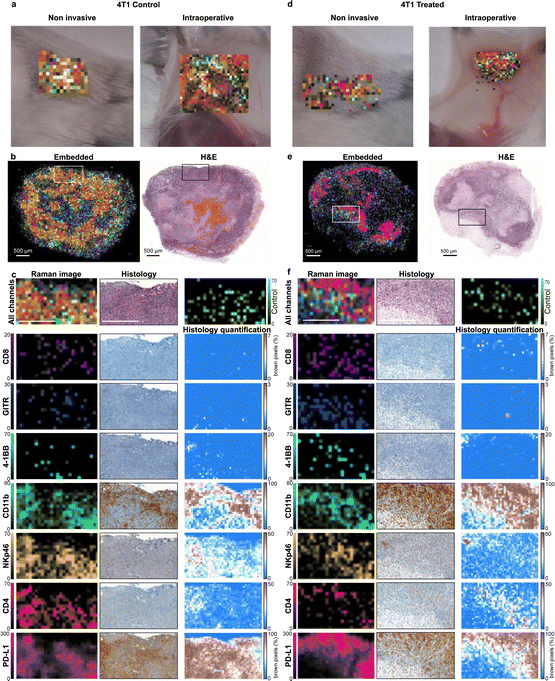 |
| Fig. 3 Raman imaging of tumor immune composition with histological validation. Tumors were scanned in different settings to simulate potential clinical scenarios such as non-invasive and intraoperative imaging (a and d) and histological analysis (b and e). Validation of imaging efficacy was performed by comparing subregions (indicated with the box in b and e) of single-channel Raman images (for the 7 targets + control) to histological stains of the same subregions with related antibodies (c and f). The histological stains were quantified as percentage of stained pixels for clarity. Raman imaging reveals the relative abundance of different markers in the tumors, in agreement with IHC. Scale bars signify 500 μm. | |
When examining the viable tumor areas, IHC staining corroborated the Raman images well, as the spatial patterns of all the markers are similar between the two techniques. Some spatial discrepancies were expected due to image sampling parameters. For example, in the IHC images, cells that appear in low numbers (such as CD8+ cells seen in Fig. 3c and f) can only be detected at high magnifications and are not discernible in low magnification images; whereas in the Raman scan, the larger pixels allow for the signal to be detected even though their exact location of the cells within the pixel is not revealed. An additional hurdle to the direct comparison of the two techniques is the innate variability of IHC stains, as the affinity of different antibodies used in the immunostaining process yields different background levels and contrast for every IHC image. Overall good agreement between the Raman images and IHC was noted in areas of viable tumor tissue: both Raman imaging and IHC reveal high expression of some markers (CD11b, PD-L1), moderate (NKp46, CD4), and lower of others (CD8, GITR, 4-1BB). The non-targeted control NP (IgG) provided low signals in areas correlated to the expression of CD11b, which may indicate non-specific uptake by phagocytes, but in low numbers. Although CD8, GITR, and 4-1BB are detected at similar intensity levels as the non-targeted control, their spatial distribution is distinct, and it is likely not attributed to the same (non-specific) uptake mechanism.
Tumor treatment response assessment
The imaged tumors demonstrated heterogeneous morphology; typically, with a necrotic center and proliferative areas in the periphery or the vicinity of blood vessels, while immune cells infiltrated the tumor in different areas and to different extents. A few consistent changes in expression level and localization were observed between the treatment group versus control, which agree with the expected effects of ICB on the tumor microenvironment.5–7,46,47 For example, moderately increased levels of CD8 and GITR and higher levels of PD-L1 were observed in the periphery of 4T1 tumors of treated animals around areas of necrotic tumor, indicating T-cell infiltration and activation, as well as an increased immune-suppressing response from the tumor, respectively. Conversely, for the control group, these markers are dispersed throughout the viable tumor area and do not point to an active immune response. A similar pattern was noted for CT26 tumors but to a lesser extent. These qualitative differences can be seen in the tumors shown in Fig. S6 and S7 (ESI†), where tumors from animals that received immunotherapy exhibited, in certain areas, elevated PD-L1 (and moderately increased CD8 and GITR) compared to their PBS-treated counterparts. However, when averaged for the whole tumor without considering their spatial distribution, the unmixed scores of the markers were found to not show any statistically significant differences (Fig. S8, ESI†), similar to flow-cytometry analysis. A spatially aware approach (taking into consideration marker distribution and co-localization) can provide more useful information than simply averaging the signal of the markers in bulk.48,49
Segmentation and classification of tumor regions
To take into consideration morphological features of the imaged tissue, a suitable method must be employed to segment the tumor in subregions with similar features. We selected to follow a superpixel clustering algorithm (SLIC),50 which groups pixels by minimizing the distance in the 8-dimensional space span by the nn-LS scores derived on the markers as well as in the two-dimensional physical space of the image. The scores on each channel were mean-centered and normalized to unit variance. The spatial coordinates were also scaled, as described in Methods, to control the superpixel compactness. The results of the superpixel segmentation are shown in Fig. 4a and b.
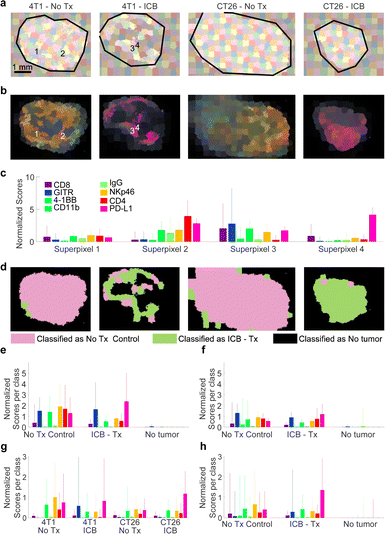 |
| Fig. 4 Segmentation and classification of tumor regions. (a) Superpixel clustering was used to group together adjacent pixels with similar nanoprobe uptake profiles into superpixels. (b) The superpixels colored based on their average Raman signal. (c) Example nanoparticle uptake profiles from the 4 superpixels indicated in (b). The bars show the mean signal on each of the markers from the constituent pixels, and the whiskers the standard deviation from the mean. Each superpixel contains about 100 pixels. (d) Classification of the superpixels using ANN. Tumors from treated mice are classified overwhelmingly as “ICB Tx” whereas control tumors as “No Tx”. Necrotic areas are classified as “No tumor”. (e) Mean expression profile from the 4T1 tumor shown in (d) according to class. The bars show the mean profile of the superpixels in each class, and the whiskers the standard deviation. A total of 1100 superpixels were considered. (f) Same for the CT26 tumor shown in (d). (g) Expression profile of superpixels from (d) based on experimental condition. (h) Overall expression profile based on class for all superpixels representing all of the 20 tumors examined in this study. | |
After SLIC-based segmentation, the superpixels were used to train classification models based on different ML approaches, to classify the superpixels into categories. The complete analysis procedure from raw Raman spectra to classification is demonstrated in Fig. S9 (ESI†), and described in more detail in Methods. Approximately 150 superpixels were generated per tumor (plus the adjacent paraffin region), as seen in Fig. 4a. The nn-LS scores were averaged within each superpixel, to provide the mean superpixel nanoprobe uptake image (Fig. 4b). Each superpixel is characterized by a nanoparticle uptake profile, four exemplary superpixel uptake profiles are shown in Fig. 4c.
The superpixels were then used as training data to calibrate several models based on supervised machine learning (SML). Classes for calibration were assigned as ‘ICB’, ‘No treatment’, and ‘No tumor’ based on whether the superpixel was from a treated animal, a control animal, or an area without a tumor, respectively. We elected that intra-tumoral variation not be taken into consideration for model calibration, meaning that areas within a tumor (either from a treated or control animal) were assigned to the same class, regardless of being viable, necrotic, or distinct in any other way. This decision reduces the bias introduced to the model during training by limiting input from a human user but leads to decreased goodness of fit statistics because varied tumor regions are treated as the same class. Several SML methods were assessed, including artificial neural networks (ANN), support vector machines (SVM), partial least squares discriminant analysis (PLS-DA), and boosted gradient trees (XBG). In all methods cross-validation was performed, to avoid over-fitting of the data, by leaving a set of 4 tumors out of calibration and using it for validation. This was performed for all tumors in the dataset (in five iterations). The classification results are summarized in Fig. S10 (ESI†).
The resulting classification is shown in Fig. 4d for one set of tumors, and in Fig. S10 (ESI†) for the whole dataset. Superpixels classified as ‘ICB’, ‘No treatment’, or ‘No tumor’ are shown in green, pink, and black, respectively. Almost all models classified areas from naïve and treated CT26 tumors correctly, but indicated that ICB-treated 4T1 tumors contained areas more closely resembling the “No Tx” group. This indicates that 4T1 tumors are more resistant to ICB therapy compared to CT26, consistent with reports in the literature.51
Overall, the ANN model performed adequately, with class error of cross-validation around 0.10, and root mean square error of cross-validation (RMSECV) around 0.26, while XBG performed better, with class error of cross-validation of 0.07. As expected, most of the error stems from intra-tumoral variability, as necrotic areas provided negligible signal and were classified as ‘No tumor’. Even so, our methodology was able to classify the tumors appropriately based on the multiplexed SERS signal, and indicate if any (and which) tumor areas are not aligned with the expected outcome. We expect that by fine-tuning the calibration data, or using morphological image processing approaches, better models can be generated, where necrotic areas and areas of effective treatment or emerging resistance can be identified.
Other SML models corroborated the results of the ANN model, indicating that the approach is model-independent. PLS-DA identified the variables with the most descriptive power as PD-L1 and GITR with T2 weights of 0.77 and 0.59, respectively, compared to CD4 and CD11b, which were the least important for classification with weights of 0.27 and 0.19, respectively. XBG also identified PD-L1 expression as the single most important predictor for classification, with a gain of 95, compared to 13 for the weakest predictor, 4-1BB.
Conclusions
New techniques with high multiplexing capacity, such as the one we present here, can greatly benefit the field of molecular imaging. Today, the detection and visualization of a single molecular target can help the physician reach a diagnosis and monitor disease progression. By detecting a panel of multiple relevant targets, the physician can gain access to synergistically more information, which also can be used for computer-aided diagnosis via ML.
In this work, we present a comprehensive methodology for multiplexed molecular Raman imaging with SERS nanoprobes, demonstrating the high potential of this modality. Initially, we examined how to discover and select new Raman reporter molecules. For in vivo applications, lasers in the IR are advantageous, and reporters with resonances close to the excitation wavelength are preferable as they provide higher signals, creating a need for good Raman reporters in the IR. Another important consideration is the affinity of the molecule to the gold core, as the plasmonic enhancement rapidly decays with distance. These effects may be predicted from the structure of a reporter molecule by using a chemical similarity metric. This approach can indicate whether a molecule will yield high-intensity SERS signal, as well as whether the spectrum will be distinct from other molecules in a multiplexed imaging panel.
Once we assembled a library of 8 distinct and bright nanoprobes, we were able to perform molecularly targeted multiplexed imaging. The data-rich images acquired, consisting of 8 channels or data, are not easily interpreted visually. It is established that ratiometric approaches can be used to show the relative prevalence of one probe vs. another. We generalized this philosophy to accommodate arbitrary numbers of markers, and provide the relative prevalence of one marker vs. all others based on a circular coordinate system. We then used this approach to assign colors based on these relative abundances. In this way, we were able to generate images that convey information from 8 channels (or more) in a single snapshot.
Our multiplexed imaging approach showcases that the immune microenvironment is not uniform throughout a tumor, with different areas exhibiting different profiles, that change drastically with certain treatments, as shown here for ICB. The local complexity within the tumor can only be effectively observed with a multiplexed imaging approach that highlights the variations in biomarker expression and immune infiltration. By visualizing this variability and identifying regions that do not fit the expected phenotype it may become possible to predict therapeutic efficacy and guide the treatment approach.
The multichannel images (Fig. S6 and S7, ESI†) can serve as a first description of the tumor microenvironment, indicating the spatial abundance of immune cells or the expression of PD-L1. However, a single image is only a qualitative visual indicator of the cellular composition of the tumor, and does not convey the complete quantitative information obtained through the scan. The complete multi-channel dataset can be analyzed algorithmically to provide a guiding model encompassing all the relevant information. A pixel-by-pixel approach has several disadvantages, as it is prone to random noise stemming from the distribution of a small number of nanoparticles in the tumor. Subsampling the image and averaging the signal over many pixels can lead to better statistics. However, to preserve physiological features we must segment the multichannel image in a way that reflects the signal of the multiple nanoparticles as well as their relative positions. We did this by using a superpixel algorithm to segment the image, grouping pixels together in clusters, that are spatially cognate, and similar in molecular expression. These clusters provide improved signal statistics and correspond to physiologically similar regions within the tumor microenvironment, allowing us to classify them algorithmically into responsive and naïve regions, after ICB.
Multiplexed imaging with SERS nanoparticles has now matured, and provides a new way of thinking about molecular imaging. Even though nanoparticle contrast agents are still a long way from clinical use, this versatile method can be applied readily in preclinical studies as well as ex vivo, using excised tissues. Overall, multiplexed molecular imaging, with SERS nanoprobes or another agent with comparable multiplexing capacity, can usher in a new era of high-capacity, computer-aided, and personalized imaging.
Materials and methods
ChPIR synthesis
All reagents were purchased from commercial sources and, unless otherwise stated, were used without further purification. Reactions run under anhydrous and/or inert conditions are noted in the procedure as such. All other reactions were performed exposed to atmospheric conditions. Tetrahydrofuran (THF) was distilled from a sodium benzophenone ketyl, and other anhydrous solvents were dried over 4 Å molecular sieves before use. Concentration in vacuo was performed on a rotary evaporator. NMR spectra were recorded on either a 300 MHz Gemini or a 500 MHz Varian Inova spectrometer for 1H NMR spectroscopy and a 300 MHz Gemini spectrometer operating at 75.5 MHz for 13C NMR spectroscopy. Residual solvent signal was used as the internal standard. If a mixture of CD2Cl2 and CD3OD was used, the residual peak for CH2Cl2 was used as the internal standard. UV/VIS-near-IR spectra were recorded in quartz cuvettes with a 1 cm path length on a PerkinElmer Lambda 12 spectrophotometer. Melting points were determined with a Büchi capillary melting point apparatus and are uncorrected. 13C NMR spectra were not recorded for some pyrylium dyes due to limited solubility in common NMR solvents. Detailed synthesis for each is presented in the ESI.†
SERS nanoprobe synthesis
SERS nanoparticles were synthesized as reported previously, with a gold nanostar core encapsulated by a silica shell.33 Briefly, gold nanostars were synthesized by quickly adding 10 mL of 20 mM tetrachloroauric acid (HAuCl4) solution per 1 L of 60 mM ascorbic acid under vigorous stirring at 4 °C. The resulting nanostars were concentrated 160-fold (to approximately 1 nM) via centrifugation (20 min, 3220 g) and dialyzed (MWCO 3.5 kDa) against DI water at 4 °C for at least three days. The silica shell was formed via a modified Stöber reaction, during which the Raman reporter molecules (commercial IR or ChPIR dyes) were also introduced to yield the various SERS spectra. In a 500 mL plastic bottle 90 mL isopropanol, 4.5 mL tetraethylorthosilicate, 1.8 mL DI, and the corresponding Raman reporter were mixed. All nanoparticle configurations were synthesized using the same procedure with different amounts of reporter molecule (at a concentration of 20 mM in dimethylformamide), as listed in Table S1 (ESI†). In a 50 mL conical tube, 27 mL ethanol, 10.8 mL nanostars from dialysis, and 1.8 mL ammonium hydroxide were mixed quickly and then poured into the plastic bottle under vigorous stirring. The reaction was allowed to proceed for 15 minutes and was then quenched by adding ethanol to a final volume of 400 mL. The nanoparticles were collected via centrifugation (20 min, 3220 g), concentrated 250-fold to approximately 3 nM, and washed repeatedly via centrifugation (3 min, 11
000 g) followed by aspiration of the supernatant and redispersion (via ultrasonication) in fresh ethanol. For antibody conjugation, the silicated nanoparticles were dispersed in a solution of 10% (3-mercaptopropyl)trimethoxysilane and 5% DI in ethanol, for one hour at room temperature. Antibodies (as listed in Table S2, ESI†) were reacted with 10× molar excess of a heterobifunctional PEG crosslinker ((poly(ethylene glycol)(N-hydroxysuccinimide 5-pentanoate) ether N′-(3-maleimidopropionyl) aminoethane (CAS: 851040-94-3)) in HEPES buffer (pH 7.1) for 30 min at room temperature. Excess crosslinker was removed by centrifuging the antibody-PEG solution using a centrifugal filter (MWCO 100 kDa). The thiolated nanoparticles were washed (2× in ethanol and 2× in DI) and finally resuspended in HEPES buffer (pH 7.1) and mixed with the antibody-PEG conjugate. The solution was allowed to react overnight at 4 °C. The functionalized SERS nanoprobes were washed one last time to remove unreacted antibodies, and the different configurations were mixed prior to injection.
The nanoparticle morphology was tested at every step of the synthesis via TEM. A droplet of the suspension was placed on a carbon film-coated copper grid (300 mesh, Electron Microscopy Sciences) and allowed to air-dry. Images were acquired at 250
000× magnification using a JEOL JEM-1400 (JEOL USA, Inc.) operated at 100 kV. The concentration and size of the nanoparticles were monitored via nanoparticle tracking analysis (NanoSight NS500, Malvern Instruments). DLS was used to measure the size distribution, zeta-potential, of the nanoparticles before and after silication and functionalization, and establish successful conjugation and stability of each sample. For DLS measurements, the nanoparticle samples were diluted 1
:
1000 or 1
:
500 in HEPES buffer at pH 7.1.
SERS nanoprobe characterization
Aliquots of each of the synthesized nanoparticle configurations at varying concentrations were scanned in a 1536 well microplate via Raman imaging. The scan results are shown in Fig. 1, where the signal from the nanoparticles is detected using a non-negative Least Squares (nn-LS) fit (see Methods) (Fig. 1b). The distinct spectra of each of the configurations at the highest concentration (Fig. 1c) were used as references for the mathematical fitting model.
For in vivo administration, eight of the best performing nanoparticle configurations were selected and surface-functionalized with antibodies to yield 7 targeted (and one non-targeted control) SERS nanoprobes. The antibodies used (Table S2, ESI†) were validated independently via flow-cytometry for these targets in live cells. Successful functionalization of the nanoparticles was confirmed by dynamic light scattering (DLS), revealing changes in size and ζ-potential for all nanoparticle-antibody pairs (Fig. S3, ESI†).
Cell culture
The murine cancer cell lines CT26 and 4T1 were obtained from ATCC and were maintained in RPMI medium supplemented with 7.5% FCS and penicillin with streptomycin. All of the cell lines were tested and found to be negative for mycoplasma contamination. The cell lines were not reauthenticated after their receipt from the original sources.
Animal model/treatment
All animal studies were conducted under a protocol approved by the Institutional Animal Care and Use Committee of Memorial Sloan Kettering Cancer Center following the NIH guidelines for the care and use of laboratory animals (NIH Publication No. 85–23 Rev. 1985). BALB/c mice (female, 4–6 weeks) were acquired from Jackson Labs. Bilateral flank CT26 and 4T1 tumors were established by subcutaneous implantation of 106 and 3 × 105 cells, respectively. The mice were randomized into a treatment group (n = 5) and a control group (n = 5). The treatment group received intraperitoneal injections of anti-CTLA-4 (9H10, 100 μg) and anti-PD-1 (RMP1-14, 250 μg) antibodies in PBS on days 8 and 11 post tumor inoculation, whereas the control group received intraperitoneal injections of pure PBS. For the 8-plex experiment, all animals were administered a bolus of the SERS nanoprobes mixture (200 μL, 3 nM each configuration, in HEPES buffer pH 7.1) on day 13 via tail vein injection. The mice were observed for an hour after nanoparticle injection, noting no abnormal behavior or signs of major discomfort. The animals were sacrificed approximately 12–16 hours after injection via carbon dioxide asphyxiation.
Flow cytometry
Each tumor was extracted, mechanically cut, enzymatically lysed and processed for surface labeling. The lysates were stained for CD8, GITR, 4-1BB, CD11b, NKp46, CD4, and PD-L1. Data were acquired using the LSRII Flow cytometer (BD Biosciences) and analyzed using FlowJo software (Treestar).
Raman imaging
Raman imaging was performed on a Renishaw InVia Raman imaging microscope, via the Wire software v4 interface. The mice were imaged twice, once through the intact skin and once after surgical exposure of the tumor area, with alternating order between control and treatment groups. One animal at a time was fastened on a platform and mounted on the microscope's mechanized stage. Prior to each Raman scan, the focal plane was selected to intersect the tumors using white-light parfocal optics, and a widefield image was acquired. The tumor areas were selected, and scanned with the Raman laser (wavelength 785 nm, objective ×5, power 161 mW, acquisition 0.5 s, resolution 300 μm). The platform was then removed from the microscope, the tumors exposed surgically, and the imaging procedure repeated with the same settings. After imaging each animal, the tumors were excised and processed for histological analysis as described below. Prior to sectioning, the paraffin blocks were subjected to Raman imaging (wavelength 785 nm, objective ×20, power 134 mW, acquisition 100 ms, resolution 40 μm) to acquire the high-resolution images for histological comparison.
Histology
Tumors were excised, fixed in 4% paraformaldehyde in PBS overnight, processed, and embedded in paraffin. Sets of four tumors (from two animals) were placed in each block to represent all experimental conditions (4T1-treated, 4T1-control, CT26-treated, CT26-control). The blocks were trimmed to reveal the four tumors and subjected to Raman imaging as described above. Next, the blocks were sectioned (5 μm thick) and stained with H&E for histological comparison (Fig. 3b and e). Additional sections were subjected to immunohistochemical staining against the same targets as the SERS nanoprobes, but using antibodies for different epitopes, independently titered and tested (Table S2, ESI†). EDTA-based antigen retrieval was used on a Ventana Discovery XT (Roche diagnostics). Optical imaging of the stained sections was performed on a Mirax digital slide scanner (Zeiss). Image quantitation was performed in MATLAB (Mathworks). RGB histological images were converted to the L*a*b* colorspace and segmented to three channels (white, blue, and brown) using a K-nearest neighbor algorithm based on the a* and b* values alone. The image was divided in square subareas and the percentage of pixels classified as ‘brown’ was counted.
Computational – spectral processing
Data analysis was performed using MATLAB (Mathworks) and PLS Toolbox (EigenVector Research Inc.). Raman images were subjected to spectral unmixing using non-negative least squares (nn-LS) regression. Reference spectra for the regression analysis were acquired from suspensions of each nanoparticle configuration in a well plate. The reference spectra underwent baseline subtraction (Whittaker filter, λ = 200) and normalization by the maximum value. The spectral data from the image were subjected to pointwise baseline subtraction (Whittaker filter, λ = 200), but no normalization was performed. Regression analysis provided a score on each of the N reference spectra, corresponding to the unmixed signal of that spectral component for every point. Specifically, for Fig. 1, N = 29 (28 reference spectra of the various nanoparticles plus the spectrum of the plastic well-plate); for Fig. 2, N = 5 (four nanoparticle spectra plus for the plastic well-plate); for Fig. 3 and Fig. S5–S9 (ESI†), N = 9 (8 nanoprobe spectra + paraffin). In all cases, as the reference spectra were normalized but the sample spectra were not, the scores retain their natural units and can be used as such for quantitative analysis, or normalized for visualization via pseudocolor assignment.
Computational-visualization
For pseudocolor representation, a color wheel was constructed as a 201 × 201 × 3 matrix using the HSV color model. Hue was set to change with the azimuth, saturation as an all-ones matrix, and value increasing radially, with 0 at the center. This matrix was then converted to a 201 × 201 × 3 RGB matrix to display the color wheel. For each visualization, the N radial reference vectors were placed evenly along the circumference of the wheel (r = 100), and their cartesian coordinates (xi, yi) were calculated.
For each point spectrum, the color was computed as follows: the coordinates on the disk were calculated as
where Si are the scores of the spectrum on each reference. Since all scores were constrained between 0 (non-negative fit) and 1 (normalization) the resulting coordinates were within the bounds of the color wheel (xp, yp between −100 and 100). The coordinates for each point were then rounded to the nearest integer, and assigned a color corresponding to the position of the point on the wheel. In this way, each point of the Raman scan was converted into a pixel on the pseudocolor image.
Computational – machine learning models
The images derived from nn-LS scores were used as input for the ML models. Initially, each of the 8 data channels was mean-centered and standardized (μ = 0 and σ = 1). Then, a SLIC algorithm was followed to group individual pixels from the Raman scans into superpixels. The same constraints and superpixel density were used for all images. The algorithm minimized the distance
for each pixel i and each superpixel n, with coordinates (x,y) and the corresponding normalized scores on each of the N = 8 multiplexed image channels. The compactness parameter a, used for weighing physical distance (in 2D space) against score distance (in 8D space), was determined empirically as a = 5 × 10−3 m−2. The process was repeated for 20 iterations, each time recalculating new coordinates and mean scores for the n superpixels. Each of the resulting superpixels represented the average of similar pixels within its vicinity. To create the dataset, each superpixel was assigned to a class (Tx, C, or none) based on whether the physical location of its center was located within the tumor boundaries.
For the classification models, the physical location of the superpixels was ignored, and only the aggregate nn-LS scores and the assigned class of the superpixel were considered. For cross-validation, a set of 4 tumors was excluded from training and was used as validation. Training with cross-validation was performed 5 times to include all 20 tumors for validation. Several SML methods were tested. After classification, the predicted class (of calibration) with the highest probability (most likely class) was used to set the color of each pixel in the image, based on the superpixel classification. The main results are presented in Fig. 4 and Fig. S10 (ESI†).
Author contributions
The manuscript was written through contributions of all authors. All authors have approved the final version of the manuscript. Conceptualization, M. F. K., M. D., T. M., C. A.; methodology, C. A., K. P., D. Z., T. M., M. F. K., A. O., S. P.; software, C. A.; validation, N. B., L. E. R.; formal analysis, C. A.; investigation, C. A., K. P., N. B., M. G., R. M.; resources, K. P., L. E. R., M. G., M. D., T. M.; data curation, C. A., K. P., N. B.; writing – original draft, C. A., M. F. K.; writing – review & editing, C. A., K. P., N. B., M. G., R. M., S. P., T. M., M. F. K.; visualization, C. A.; supervision, C. A., M. F. K.; project administration, C. A., M. F. K.; funding acquisition, M. F. K., T. M., C. A.
Conflicts of interest
M. F. K. is a co-founder of RIO Imaging, which did not contribute to this manuscript. All patents associated with M. F. K. have been assigned to and are handled by Stanford University or Memorial Sloan Kettering Cancer Center, respectively. Other authors declare no competing interests.
Acknowledgements
We thank Sebastien Monette for the helpful discussion regarding histological interpretation, Rebecca Gelman and Andreas Artemiou for valuable input on the statistical analysis, the MSKCC Molecular Cytology Core Facility for histology support, Hsiao-Ting Hsu for assisting with animal handling, and Shukree Abdul-Rashed for assistance with the dye synthesis. This work was performed with funds from the following sources: Pershing Square Sohn Prize by the Pershing Square Sohn Cancer Research Alliance; Damon Runyon-Rachleff Innovation Award DRR-29-14; NIH R01 EB017748 and R01 CA222836; and MSKCC Center for Molecular Imaging & Nanotechnology (CMINT) and Technology Development Grants. Acknowledgments are also extended to the grant-funding support provided by the MSKCC NIH Core Grant (P30-CA008748).
References
- National Research Council, Toward Precision Medicine: Building a Knowledge Network for Biomedical Research and a New Taxonomy of Disease, The National Academies Press, Washington, DC, 2011 DOI:10.17226/13284.
- K. Heinzmann, L. M. Carter, J. S. Lewis and E. O. Aboagye, Multiplexed imaging for diagnosis and therapy, Nat. Biomed. Eng., 2017, 1(9), 697–713 CrossRef.
- C. Andreou, R. Weissleder and M. F. Kircher, Multiplexed imaging in oncology, Nat. Biomed. Eng., 2022, 6(5), 527–540 CrossRef PubMed.
- P. Sharma and J. P. Allison, The future of immune checkpoint therapy, Science, 2015, 348(6230), 56–61 CrossRef CAS PubMed.
- M. Rashidian, M. W. LaFleur, V. L. Verschoor, A. Dongre, Y. Zhang, T. H. Nguyen, S. Kolifrath, A. R. Aref, C. J. Lau, C. P. Paweletz, X. Bu, G. J. Freeman, M. I. Barrasa, R. A. Weinberg, A. H. Sharpe and H. L. Ploegh, Immuno-PET identifies the myeloid compartment as a key contributor to the outcome of the antitumor response under PD-1 blockade, Proc. Natl. Acad. Sci. U. S. A., 2019, 116(34), 16971–16980 CrossRef CAS.
- R. Tavare, H. Escuin-Ordinas, S. Mok, M. N. McCracken, K. A. Zettlitz, F. B. Salazar, O. N. Witte, A. Ribas and A. M. Wu, An Effective Immuno-PET Imaging Method to Monitor CD8-Dependent Responses to Immunotherapy, Cancer Res., 2016, 76(1), 73–82 CrossRef CAS PubMed.
- M. Yi, D. C. Jiao, H. X. Xu, Q. Liu, W. H. Zhao, X. W. Han and K. M. Wu, Biomarkers for predicting efficacy of PD-1/PD-L1 inhibitors, Mol. Cancer, 2018, 17, 1–14 CrossRef.
- D. Bethmann, Z. P. Feng and B. A. Fox, Immunoprofiling as a predictor of patient's response to cancer therapy-promises and challenges, Curr. Opin. Immunol., 2017, 45, 60–72 CrossRef CAS PubMed.
- R. Remark, T. Merghoub, N. Grabe, G. Litjens, D. Damotte, J. D. Wolchok, M. Merad and S. Gnjatic, In-depth tissue profiling using multiplexed immunohistochemical consecutive staining on single slide, Sci. Immunol., 2016, 1(1), aaf6925 Search PubMed.
- E. C. Stack, C. Wang, K. A. Roman and C. C. Hoyt, Multiplexed immunohistochemistry, imaging, and quantitation: a review, with an assessment of Tyramide signal amplification, multispectral imaging and multiplex analysis, Methods, 2014, 70(1), 46–58 CrossRef CAS.
- E. C. Stack, C. C. Wang, K. A. Roman and C. C. Hoyt, Multiplexed immunohistochemistry, imaging, and quantitation: A review, with an assessment of Tyramide signal amplification, multispectral imaging and multiplex analysis, Methods, 2014, 70(1), 46–58 CrossRef CAS.
- M. Angelo, S. C. Bendall, R. Finck, M. B. Hale, C. Hitzman, A. D. Borowsky, R. M. Levenson, J. B. Lowe, S. D. Liu, S. Zhao, Y. Natkunam and G. P. Nolan, Multiplexed ion beam imaging of human breast tumors, Nat. Med., 2014, 20(4), 436–442 CrossRef CAS PubMed.
- L. Keren, M. Bosse, D. Marquez, R. Angoshtari, S. Jain, S. Varma, S. R. Yang, A. Kurian, D. Van Valen, R. West, S. C. Bendall and M. Angelo, A Structured Tumor-Immune Microenvironment in Triple Negative Breast Cancer Revealed by Multiplexed Ion Beam Imaging, Cell, 2018, 174(6), 1373–1387.e19 CrossRef CAS PubMed.
- C. Andreou, S. A. Kishore and M. F. Kircher, Surface-Enhanced Raman Spectroscopy: A New Modality for Cancer Imaging, J. Nucl. Med., 2015, 56(9), 1295–1299 CrossRef CAS PubMed.
- D. L. Jeanmaire and R. P. Vanduyne, Surface Raman Spectroelectrochemistry. 1. Heterocyclic, Aromatic, and Aliphatic-Amines Adsorbed on Anodized Silver Electrode, J. Electroanal. Chem., 1977, 84(1), 1–20 CAS.
- M. Fleischmann, P. J. Hendra and A. J. Mcquillan, Raman-Spectra of Pyridine Adsorbed at a Silver Electrode, Chem. Phys. Lett., 1974, 26(2), 163–166 CrossRef CAS.
- J. Langer, D. Jimenez de Aberasturi, J. Aizpurua, R. A. Alvarez-Puebla, B. Auguie, J. J. Baumberg, G. C. Bazan, S. E. J. Bell, A. Boisen, A. G. Brolo, J. Choo, D. Cialla-May, V. Deckert, L. Fabris, K. Faulds, F. J. Garcia de Abajo, R. Goodacre, D. Graham, A. J. Haes, C. L. Haynes, C. Huck, T. Itoh, M. Kall, J. Kneipp, N. A. Kotov, H. Kuang, E. C. Le Ru, H. K. Lee, J. F. Li, X. Y. Ling, S. A. Maier, T. Mayerhofer, M. Moskovits, K. Murakoshi, J. M. Nam, S. Nie, Y. Ozaki, I. Pastoriza-Santos, J. Perez-Juste, J. Popp, A. Pucci, S. Reich, B. Ren, G. C. Schatz, T. Shegai, S. Schlucker, L. L. Tay, K. G. Thomas, Z. Q. Tian, R. P. Van Duyne, T. Vo-Dinh, Y. Wang, K. A. Willets, C. Xu, H. Xu, Y. Xu, Y. S. Yamamoto, B. Zhao and L. M. Liz-Marzan, Present and Future of Surface-Enhanced Raman Scattering, ACS Nano, 2020, 14(1), 28–117 CrossRef CAS PubMed.
- C. L. Zavaleta, B. R. Smith, I. Walton, W. Doering, G. Davis, B. Shojaei, M. J. Natan and S. S. Gambhir, Multiplexed imaging of surface enhanced Raman scattering nanotags in living mice using noninvasive Raman spectroscopy, Proc. Natl. Acad. Sci. U. S. A., 2009, 106(32), 13511–13516 CrossRef CAS.
- Y. W. Wang, N. P. Reder, S. Kang, A. K. Glaser and J. T. C. Liu, Multiplexed Optical Imaging of Tumor-Directed Nanoparticles: A Review of Imaging Systems and Approaches, Nanotheranostics, 2017, 1(4), 369–388 CrossRef.
- Y. W. Wang, N. P. Reder, S. Kang, A. K. Glaser, Q. Yang, M. A. Wall, S. H. Javid, S. M. Dintzis and J. T. C. Liu, Raman-Encoded Molecular Imaging with Topically Applied SERS Nanoparticles for Intraoperative Guidance of Lumpectomy, Cancer Res., 2017, 77(16), 4506–4516 CrossRef CAS.
- X. P. Wang, Y. Zhang, M. Konig, E. Papadopoulou, B. Walkenfort, S. Kasimir-Bauer, A. Bankfalvi and S. Schlucker, iSERS microscopy guided by wide field immunofluorescence: analysis of HER2 expression on normal and breast cancer FFPE tissue sections, Analyst, 2016, 141(17), 5113–5119 RSC.
- Y. W. Wang, Q. Yang, S. Kang, M. A. Wall and J. T. C. Liu, High-speed Raman-encoded molecular imaging of freshly excised tissue surfaces with topically applied SERRS nanoparticles, J. Biomed. Opt., 2018, 23(4), 1–8 CAS.
-
J.-H. Yu, I. Steinberg, R. M. Davis, A. V. Malkovskiy, A. Zlitni, D. T. Chung, L. D. Curet, A. L. D’Souza, E. Chang and J. Campbell, Noninvasive and Highly Multiplexed Five-Color Tumor Imaging of Near-Infrared Resonant Surface-Enhanced Raman Nanoparticles In Vivo, 2020.
- O. E. Eremina, A. T. Czaja, A. Fernando, A. Aron, D. B. Eremin and C. Zavaleta, Expanding the Multiplexing Capabilities of Raman Imaging to Reveal Highly Specific Molecular Expression and Enable Spatial Profiling, ACS Nano, 2022, 16(7), 10341–10353 CrossRef CAS.
- F. Nicolson, L. E. Jamieson, S. Mabbott, K. Plakas, N. C. Shand, M. R. Detty, D. Graham and K. Faulds, Through tissue imaging of a live breast cancer tumour model using handheld surface enhanced spatially offset resonance Raman spectroscopy (SESORRS), Chem. Sci., 2018, 9(15), 3788–3792 RSC.
- F. Nicolson, L. E. Jamieson, S. Mabbott, K. Plakas, N. C. Shand, M. R. Detty, D. Graham and K. Faulds, Surface enhanced resonance Raman spectroscopy (SERRS) for probing through plastic and tissue barriers using a handheld spectrometer, Analyst, 2018, 143(24), 5965–5973 RSC.
- M. R. Detty and B. J. Murray, Telluropyrylium Dyes. 1. 2,6-Diphenyltelluropyrylium Dyes, J. Org. Chem., 1982, 47(27), 5235–5239 CrossRef CAS.
- M. R. Detty, J. M. Mckelvey and H. R. Luss, Tellurapyrylium Dyes. 2. The Electron-Donating Properties of the Chalcogen Atoms to the Chalcogenapyrylium Nuclei and Their Radical Dications, Neutral Radicals, and Anions, Organometallics, 1988, 7(5), 1131–1147 CrossRef CAS.
- M. A. Bedics, H. Kearns, J. M. Cox, S. Mabbott, F. Ali, N. C. Shand, K. Faulds, J. B. Benedict, D. Graham and M. R. Detty, Extreme red shifted SERS nanotags, Chem. Sci., 2015, 6(4), 2302–2306 RSC.
- S. Harmsen, M. A. Bedics, M. A. Wall, R. M. Huang, M. R. Detty and M. F. Kircher, Rational design of a chalcogenopyrylium-based surface-enhanced resonance Raman scattering nanoprobe with attomolar sensitivity, Nat. Commun., 2015, 6(1), 1–9 RSC.
- H. Kearns, S. Sengupta, I. R. Sasselli, L. Bromley, K. Faulds, T. Tuttle, M. A. Bedics, M. R. Detty, L. Velarde, D. Graham and W. E. Smith, Elucidation of the bonding of a near infrared dye to hollow gold nanospheres - a chalcogen tripod, Chem. Sci., 2016, 7(8), 5160–5170 RSC.
- N. G. Rule, M. R. Detty, J. E. Kaeding and J. A. Sinicropi, Syntheses of 4h-Thiopyran-4-One 1,1-Dioxides as Precursors to Sulfone-Containing Analogs of Tetracyanoquinodimethane, J. Org. Chem., 1995, 60(6), 1665–1673 CrossRef CAS.
- C. Andreou, A. Oseledchyk, F. Nicolson, N. Berisha, S. Pal and M. F. Kircher, Surface-enhanced Resonance Raman Scattering Nanoprobe Ratiometry for Detecting Microscopic Ovarian Cancer via Folate Receptor Targeting, JoVE, 2019,(145), e58389 CAS.
- S. Harmsen, M. A. Wall, R. Huang and M. F. Kircher, Cancer imaging using surface-enhanced resonance Raman scattering nanoparticles, Nat. Protoc., 2017, 12(7), 1400–1414 CrossRef CAS PubMed.
- S. Harmsen, R. Huang, M. A. Wall, H. Karabeber, J. M. Samii, M. Spaliviero, J. R. White, S. Monette, R. O'Connor, K. L. Pitter, S. A. Sastra, M. Saborowski, E. C. Holland, S. Singer, K. P. Olive, S. W. Lowe, R. G. Blasberg and M. F. Kircher, Surface-enhanced resonance Raman scattering nanostars for high-precision cancer imaging, Sci. Transl. Med., 2015, 7(271), 271ra7 Search PubMed.
- T. R. Nayak, C. Andreou, A. Oseledchyk, W. D. Marcus, H. C. Wong, J. Massague and M. F. Kircher, Tissue factor-specific ultra-bright SERRS nanostars for Raman detection of pulmonary micrometastases, Nanoscale, 2017, 9(3), 1110–1119 RSC.
- T. W. H. Backman, Y. Q. Cao and T. Girke, ChemMine tools: an online service for analyzing and clustering small molecules, Nucleic Acids Res., 2011, 39, W486–W491 CrossRef CAS PubMed.
- J. Sharko, G. Grinstein and K. A. Marx, Vectorized Radviz and Its Application to Multiple Cluster Datasets, IEEE Trans. Visualization Comput. Graphics, 2008, 14(6), 1444–1451 Search PubMed.
- L. Gauthier, A. Morel, N. Anceriz, B. Rossi, A. Blanchard-Alvarez, G. Grondin, S. Trichard, C. Cesari, M. Sapet, F. Bosco, H. Rispaud-Blanc, F. Guillot, S. Cornen, A. Roussel, B. Amigues, G. Habif, F. Caraguel, S. Arrufat, R. Remark, F. Romagne, Y. Morel, E. Narni-Mancinelli and E. Vivier, Multifunctional Natural Killer Cell Engagers Targeting NKp46 Trigger Protective Tumor Immunity, Cell, 2019, 177(7), 1701–1713.e16 CrossRef CAS.
- W. Ma, B. M. Gilligan, J. Yuan and T. Li, Current status and perspectives in translational biomarker research for PD-1/PD-L1 immune checkpoint blockade therapy, J. Hematol. Oncol., 2016, 9(1), 47 CrossRef PubMed.
- L. Strauss, M. A. A. Mahmoud, J. D. Weaver, N. M. Tijaro-Ovalle, A. Christofides, Q. Wang, R. Pal, M. Yuan, J. Asara, N. Patsoukis and V. A. Boussiotis, Targeted deletion of PD-1 in myeloid cells induces antitumor immunity, Sci. Immunol., 2020, 5(43), eaay1863 CrossRef CAS PubMed.
- V. S. Fear, C. Tilsed, J. Chee, C. A. Forbes, T. Casey, J. N. Solin, S. M. Lansley, W. J. Lesterhuis, I. M. Dick, A. K. Nowak, B. W. Robinson, R. A. Lake and S. A. Fisher, Combination immune checkpoint blockade as an effective therapy for mesothelioma, Oncoimmunology, 2018, 7(10), e1494111 CrossRef PubMed.
- K. Kim, A. D. Skora, Z. Li, Q. Liu, A. J. Tam, R. L. Blosser, L. A. Diaz, Jr, N. Papadopoulos, K. W. Kinzler, B. Vogelstein and S. Zhou, Eradication of metastatic mouse cancers resistant to immune checkpoint blockade by suppression of myeloid-derived cells, Proc. Natl. Acad. Sci. U. S. A., 2014, 111(32), 11774–11779 CrossRef CAS.
- Z. Ma, W. Li, S. Yoshiya, Y. Xu, M. Hata, Y. El-Darawish, T. Markova, K. Yamanishi, H. Yamanishi, H. Tahara, Y. Tanaka and H. Okamura, Augmentation of Immune Checkpoint Cancer Immunotherapy with IL18, Clin. Cancer Res., 2016, 22(12), 2969–2980 CrossRef CAS.
- Q. Chen, L. Xu, C. Liang, C. Wang, R. Peng and Z. Liu, Photothermal therapy with immune-adjuvant nanoparticles together with checkpoint blockade for effective cancer immunotherapy, Nat. Commun., 2016, 7, 13193 CrossRef CAS.
- F. Petitprez, M. Meylan, A. de Reynies, C. Sautes-Fridman and W. H. Fridman, The Tumor Microenvironment in the Response to Immune Checkpoint Blockade Therapies, Front. Immunol., 2020, 11, 784 CrossRef CAS PubMed.
- R. Noy and J. W. Pollard, Tumor-Associated Macrophages: From Mechanisms to Therapy, Immunity, 2014, 41(1), 49–61 CrossRef CAS PubMed.
- S. M. Lewis, M. L. Asselin-Labat, Q. Nguyen, J. Berthelet, X. Tan, V. C. Wimmer, D. Merino, K. L. Rogers and S. H. Naik, Spatial omics and multiplexed imaging to explore cancer biology, Nat. Methods, 2021, 18(9), 997–1012 CrossRef CAS.
- A. Rao, D. Barkley, G. S. Franca and I. Yanai, Exploring tissue architecture using spatial transcriptomics, Nature, 2021, 596(7871), 211–220 CrossRef CAS.
- R. Achanta, A. Shaji, K. Smith, A. Lucchi, P. Fua and S. Susstrunk, SLIC superpixels compared to state-of-the-art superpixel methods, IEEE Trans Pattern Anal Mach Intell, 2012, 34(11), 2274–2282 Search PubMed.
- J. Xu, R. Saklatvala, S. Mittal, S. Deshmukh and A. Procopio, Recent Progress of Potentiating Immune Checkpoint Blockade with External Stimuli-an Industry Perspective, Adv. Sci., 2020, 7(8), 1903394 CrossRef CAS.
Footnotes |
† Electronic supplementary information (ESI) available: Online Methods, Fig. S1–S10 and Tables S1, S2. See DOI: https://doi.org/10.1039/d2nh00331g |
‡ These authors contributed equally |
§ Deceased. |
|
This journal is © The Royal Society of Chemistry 2022 |