DOI:
10.1039/D0LC01040E
(Tutorial Review)
Lab Chip, 2021,
21, 458-472
Cardiovascular microphysiological systems (CVMPS) for safety studies – a pharma perspective
Received
15th October 2020
, Accepted 13th January 2021
First published on 15th January 2021
Abstract
The integrative responses of the cardiovascular (CV) system are essential for maintaining blood flow to provide oxygenation, nutrients, and waste removal for the entire body. Progress has been made in independently developing simple in vitro models of two primary components of the CV system, namely the heart (using induced pluripotent stem-cell derived cardiomyocytes) and the vasculature (using endothelial cells and smooth muscle cells). These two in vitro biomimics are often described as immature and simplistic, and typically lack the structural complexity of native tissues. Despite these limitations, they have proven useful for specific “fit for purpose” applications, including early safety screening. More complex in vitro models offer the tantalizing prospect of greater refinement in risk assessments. To this end, efforts to physically link cardiac and vascular components to mimic a true CV microphysiological system (CVMPS) are ongoing, with the goal of providing a more holistic and integrated CV response model. The challenges of building and implementing CVMPS in future pharmacological safety studies are many, and include a) the need for more complex (and hence mature) cell types and tissues, b) the need for more realistic vasculature (within and across co-modeled tissues), and c) the need to meaningfully couple these two components to allow for integrated CV responses. Initial success will likely come with simple, bioengineered tissue models coupled with fluidics intended to mirror a vascular component. While the development of more complex integrated CVMPS models that are capable of differentiating safe compounds and providing mechanistic evaluations of CV liabilities may be feasible, adoption by pharma will ultimately hinge on model efficiency, experimental reproducibility, and added value above current strategies.
Introduction
Cardiovascular (CV) physiological and structural alterations are a leading cause of market withdrawal and drug candidate failure.1,2 Many CV liabilities are identified at or during late stage clinical development or post-marketing, suggesting simpler nonclinical in vitro studies and more integrative animal models inadequately identify safety liabilities seen in patients.3,4 Goals for nonclinical safety evaluations include identifying and characterizing the mechanisms responsible for and risks associated with toxicities to better define risk/benefit assessments of evolving drug candidates. This is accomplished through the use of simpler (or more complex) assays (defined as the biology under study) as evaluated with various platforms (consisting of the techniques and technologies used to study the biology) and interpreted in context (defining the overall model or system). Simpler CV safety assays derived from animal tissues (or more recently, from human cells) may be helpful to define CV risks depending on the questions being addressed by the models and the capabilities of the platforms used. Given the complexity of the CV system and increasing variety of drug modalities, it is expected that at least a few in vitro microphysiological systems (MPS) will be needed to fully characterize the various types of CV liabilities in healthy and diseased conditions.5–7 The International Consortium for Innovation and Quality in Pharmaceutical Development (IQ) MPS Affiliate, of which all author companies are members is a group of pharmaceutical industry scientists who seek to expedite appropriate characterization and incorporation of MPS to potentially improve drug safety assessment and provide safer and more effective medicines to patients. The IQ-MPS Affiliate defines MPS models as going beyond traditional 2D sandwich culture or monolayer cell assays to include several of the following design aspects: a multi-cellular environment within biopolymer or tissue-derived matrix, a 3D structure, mechanical factors such as stretch or perfusion (e.g. breathing, gut peristalsis, flow), incorporate primary or stem cell-derived cells, and/or inclusion of immune system components.
Efficacious drugs can unintentionally cause adverse effects to the heart and CV system. Effects on the heart may arise from direct interactions with cellular components including cardiomyocytes (CMs), fibroblasts, endothelial cells, neural cells, or resident immune cells. Interactions between these cellular components (and potentially other organ systems) may also modulate and impair electrical conduction, contractile force generation, hemodynamic changes, matrix deposition, vascularization, and responses to injury. Although evaluating physiological and structural cardiac alterations in CM-only monolayer assays has been a significant advancement in recent years, this type of assay is likely insufficient to detect some CV functional and structural liabilities. Thus, human-derived CVMPS models are poised to play a role in detecting, defining, and avoiding cardiac as well as vascular liabilities in the nonclinical arena with human-derived preparations to better inform on potential clinical liabilities.3
The adoption of any novel biological model within drug discovery is rooted in providing a tangible benefit in terms of scientific need and practicality. The tangible use of more advanced, bioengineered cardiac in vitro assays over plate-based monolayer human induced pluripotent stem-cell derived-CMs (hiPS-CMs) is more likely for bespoke mechanistic investigations. This would allow for temporal assessments of an integrated cardiac safety signal in tandem with pharmacokinetics (PK) in a human-based model, hence providing additional benefit over current cardiac in vitro approaches. Such an approach should be amenable to both traditional small molecules and emerging newer therapeutics such as cell- or RNA-based modalities. The applications in the near term (<3 years) are less reliant on high throughput, thus emphasis should be placed on the biological function, physiology and maturity rather than miniaturization. Impact of such applications could subsequently lead to the long-term application for compound screening. To enable the use of such a model in these scenarios, several key characteristics of the biological assay as well as the experimental platform should be considered:
Biological assay characteristics
• Structural similarity to human myocardium and vasculature. Composing of multiple cell types including CMs (ventricular and arterial), nodal cells (sinoatrial and atrioventricular), smooth muscle cells, fibroblasts and endothelial cells in proportions and structure resembling the native myocardium. This includes elongated striated CMs, gene and protein expression representative of differentiated cell types with integrated physiological signalling pathways and appropriate density and geometry of t-tubules.
• Functional similarity to human myocardium and vasculature including integrated electrical stimulation. Aspects to consider include electrophysiology, contractility, energy dynamics (for myocardium, relative reliance on fatty acid oxidation) and a functional vascular system.
Platform characteristics
• Ability to incorporate cardiac pre- and after-load conditions (i.e. mirroring the stretch/force/pressure within the heart throughout the cardiac cycle) and mimic the cardiac physiological environment in terms of stretch and fluid dynamics (i.e. perfusion system design to influence sheer stress).
• Capabilities (technology) to monitor a range of functional (cardiac and vasculature), morphological, biomarker and PK endpoints simultaneously in a temporal manner facilitating the application to investigate PK–pharmacodynamic (PD) relationships aiding translation and mechanistic understanding, validated using pharmacological tools.
• Miniaturization is essential to enable higher throughput models with reduced consumption of limited cells, reagents, and test articles.
• Engineered using material compatible for use in biological research of drug substances.
An ideal CVMPS model would have these characteristics (summarized and expanded upon in Table 1) and be engineered in such a manner that it is as simple as possible while facilitating high levels of reproducibility and be suitable to support drug discovery. These concepts are further discussed below in ‘The Ideal CVMPS Model’. Realistically, no model will satisfy all these suggested ideal requirements. However, qualified models incorporating various subsystems could address specific needs in a practical and efficient manner.
Table 1 Biological, platform and practical ideal CVMPS model characteristics
Category |
Desired characteristic |
Details |
CMs, cardiomyocytes; CV, cardiovascular; PK, pharmacokinetics.
|
Biological |
Cell types |
CMs (ventricular and arterial), nodal cells (sinoatrial and atrioventricular), smooth muscle cells, fibroblasts and endothelial cells in proportions and structure resembling native human myocardium |
Structural integrity |
Elongated striated CMs with appropriate density and geometry of t-tubules with non-myocytes specially organised representative of human myocardium |
Gene/protein expression representative of a differentiated cell type with integrated physiological signalling pathways |
Electrical and mechanical functionality |
Electrophysiology and mechanical features representative of the adult heart, including a resting membrane potential ∼−90 mV, a positive force frequency relationship and vasodilation/vasoconstriction |
Physiological energy dynamics |
Reliance on physiological energy substrates, i.e. fatty acids for cardiomyocytes |
Lack of spontaneous beating |
Model does not beat spontaneously, but responses to integrated electrical stimulation up to 2 Hz (changes in beating rate of spontaneously active immature preparations may confound experimental findings) |
Extended tissue longevity |
Ability to assess acute (minutes) and chronic (>1 week) CV liabilities |
Induced diseased phenotypes |
Chemical and or genetic manipulation to induce CV disease phenotypes, i.e. ischemia reperfusion injury |
Physiological biotransformation |
Expression of metabolizing enzymes and transporters representative of physiology |
Incorporation of additional organ systems |
Vascularized flow connection to a kidney, liver, parasympathetic and sympathetic nervous system. Incorporation of immune component (minimum macrophages) into flow chamber |
Platform |
Mechanical stretch |
Incorporate cardiac pre- and after-load conditions, i.e. mirroring the stretch/force/pressure within the heart throughout the cardiac cycle |
Electrical stimulation |
Continued innate electrical stimulation |
Fluidics |
Perfusion representative of the CV system in terms of flow rate, volume and pressure |
Incorporation of non-disruptive endpoints |
Incorporation of electrical and mechanical sensors |
Ability to sample media and conduct live confocal imaging throughout experimental time course |
Pharmacological validation |
Concentration–response data for tool compounds for specific endpoint depending on context of use representing clinical findings (including positive and negative controls) |
In vivo like PK |
Ability to administer compound to mimic in vivo PK characteristics (i.e. compound Cmax and Tmax) |
Practicalities |
Throughput |
Amendable to scaling to increase throughput |
Data reproducible |
Minimum three replicates of validation with data within 2-fold |
Assay window |
Z-Prime > 0.5 (requires a large number of replicates, may not be appropriate measure for higher throughput model). Indeed, Z' values > 0 may be acceptable for an early screening assay |
Understanding CVMPS models
It is necessary to understand the CV system in order to evaluate the strengths and limitations of evolving CVMPS assays and models. The CV system is a functionally and structurally diverse and integrated system with the heart and vasculature comprising the two key components. The heart provides the force to generate pressures that enable blood flow through various portions of the vasculature. This includes volume-conducting large arteries, pressure-regulating smaller arterioles, capillaries for nutrient exchange, and venules and capacitance vessels that facilitate oxygenation, nutrient exchange, waste removal, and signalling between organs and within the CV system itself. This level of systems integration is likely impossible to duplicate in an in vitro CVMPS model at present. However, in vitro systems of lower complexity (representing lower levels of integration) are possible and have proven useful in defining acute cardiac and CV physiological and structural alterations (such as when screening known targets such as kinase inhibitors that generally require simpler models when adequate endpoints are measurable). These subsystems range from simpler CV tissues to systems incorporating fluidics to allow for superfusion (perfusion over a tissue) or perfusion (perfusion through a tissue) of cells or tissues (but rarely organs).
Although cardiac in vitro assays to date have enabled investigations using CMs (or other defined cellular populations) in a direct manner, most lack the complex phenotype and architecture of the cardiac myocardium to allow evaluation of responses to stress or injury such as cardiac remodelling. In general, the authors herein argue that the goals of a microphysiologic cardiac system (a component of a more complete CVMPS) are already lofty and equal to that of a biomimetic cardiac tissue; a multicellular highly aligned, contractile, and vascularized cardiac model in a three-dimensional microenvironment. Noteworthy advances in the field of tissue engineering have spawned multi-cellular cardiac constructs that hold promise and potential utility for in vitro safety assessment and this has been reviewed recently.8 At the time of that review (2018), the advancements in developing functional vascularized tissues were clear, but authors also concluded a more in-depth understanding of cellular components and their interactions are required to optimize these engineered and vascularized cardiac microtissues.
Context of use
In establishing a guidance for future development of various CVMPS models, the context of use will ultimately define what assay and platform attributes are required. Understanding the context, namely the toxicity or pathophysiological response suspected or observed, will help to determine the appropriate MPS for use (Table 2). Within the context of the CV system both physiological (electrical and mechanical) and/or structural alternations can arise in both the cardiac tissue and vasculature. These effects can be interconnected and influenced by both CV interactions and other organ systems (i.e., renal function). Some simple MPS assays may be suitable for hazard identification screening and ideally there would be movement towards a single system capable of understanding complex mechanisms of adverse effects in vivo. However, multiple fit-for-purpose MPS models will likely be necessary due to the inherent challenges of achieving all goals. For example, primary CMs and hiPSC-CMs assays in 2D cultures have been extensively and successfully used to evaluate electrophysiological changes and safety risk of evolving drug candidates (Table 2).9–18 Subsequent large-scale validation efforts are demonstrating the overall utility of these simpler assays for detecting distinct cardiac effects i.e. delayed proarrhythmic risk.13,15,16 Although Table 2 notes that there are many specific electrophysiological or contractile function endpoints that can be measured in two-dimensional (2D) cultures of cardiac myocytes (e.g. single cell-type, non-MPS systems), studies have demonstrated that three-dimensional (3D) co-cultures of hiPSC-CMs and cardiac fibroblasts may be superior in more closely mimicking the native state of the myocardium.19 The potential value of MPS is underscored in that pathological remodeling of the extracellular matrix in response to injury cannot be modeled by 2D single cell cultures or any of the existing cellular systems. One advantage of an engineered, cardiac microphysiological system is that it should replicate the responsive characteristics of extracellular matrix during remodeling via the use of synthetic and natural biomaterials and microfabrication techniques. Thus, a key advantage for a cardiac microphysiological system is that it would encompass physiologically relevant tissue structure and perfusion that closely mimics human vasculature. This would engage additional properties for more complex and integrated analysis of effects beyond what is attained by the more direct biochemical endpoints advancing beyond what is shown in Table 2. Further, immune-mediated changes in cardiac tissues that ultimately result in myocarditis (Table 2) would require a multicellular assay, potentially with fluidics, and the ability to respond to immune mediators. There are limitations to experiments conducted with a single cell type because these systems only reflect direct effects on CMs, missing likely modulatory components provided by other cell types. In short, understanding the biology or physiological responses in the tissue will define the performance of the model for the desired context of use (Table 2) and acceptance in decision making. Improvements in cellular maturation, recapitulation of tissue architecture and addition of a responsive contractile function by various means (e.g. micropatterning, discussed below) will broaden model utility and performance. The ultimate goal is to move away from merely detecting liabilities and towards understanding the often-complex mechanisms that cause adverse effects in vivo.
Table 2 CV physiological and structural responses, tool drugs/toxicants to assess mechanisms, and contexts of use
Pathophysiologic or toxic response |
Minimum cell system required |
Factors eliciting the response in vivo |
Pharmacologic tools for eliciting the response in vitro |
Context of use |
Electrophysiology/arrhythmias |
Delayed repolarization |
Single |
Hypokalaemia, antiarrhythmic and other drugs that inhibit cardiac K+ channels, inherited mutations in K+ and Na+ channels |
Dofetilide, haloperidol, quinidine, sotalol |
Evaluation of cardiac arrhythmia risk elicited by changes in ion currents |
Conduction delay/block |
Single or multiple (CMs, nodal cells fibroblasts) |
Fibrosis, ischemia, structural heart disease, drugs that inhibit Na+ channels |
Flecainide, lidocaine disopyramide, mexiletine, propafenone, diltiazem |
Evaluation of cardiac arrhythmias elicited by changes in normal conduction |
Delayed conduction across the heart |
Automaticity |
Single (immature CMs) or sinoatrial node cells |
Increased: catecholamines decreased: sick sinus syndrome, pericarditis, increased parasympathetic tone |
Isoproterenol, norepinephrine, dopamine |
Changes in automaticity in specialized CMs |
Acetylcholine, adenosine, ivabradine |
Triggered activity (EADs, DADs) |
Single |
Hypokalaemia, repolarization delay, K+ channel inhibitors, dysregulated SR calcium uptake or release, Na overload, excess catecholamines, ischemic heart disease, inherited CPVT |
Digoxin, quinidine, veratridine, dofetilide, sotalol, veratridine, caffeine, BayK8644 |
Prolongation of cardiac action potential duration and repolarization; Ca2+ overload and SR Ca2+ leak |
Re-entry arrhythmias |
Single or multiple (CMs, fibroblasts) |
Ischemic myocardium, fibrosis, slowed conduction |
Flecainide, lidocaine |
Slow conduction due to Na+ channel inhibition; structural or functional unidirectional blocks |
Contractile function |
Positive inotropy |
Single |
Catecholamines, stretch |
Omecamtiv mecarbil, levosimendan, milrinone, dobutamine, isoproterenol, digoxin |
Changes in myocardial contractility |
Negative inotropy |
Single |
ET-1, angII, histamine, acetylcholine, hypoxia |
Metoprolol, itraconazole, carbachol, nifedipine, verapamil, thapsigargin |
Decreased myocardial contractility |
Lusitropy |
Single/multiple (CMs, fibroblasts) |
Increased: catecholamines |
Isoproterenol, dobutamine, carvedilol, metoprolol, ET-1 |
Impaired myocardial relaxation |
Decreased: Ca2+ overload, reduced SERCA2A, fibrosis |
Calcium dysregulation |
Single |
Ischemic heart disease, HF, inherited CPVT |
Theophylline, rapamycin, propofol, amitriptyline, dobutamine |
Impaired beat-to-beat Ca2+ regulation |
Pathological remodelling |
Hypertrophy |
Single/multiple (CMs, VECs) |
HTN, RAAS activation, tachycardia, cardiac stimulants, pressure–volume overload |
ET-1, HDAC inhibitors, isoproterenol, norepinephrine dobutamine |
Simulate tissue architecture to enable mimicking pressure-overload mechanism |
Fibrosis |
Multiple (CMs, fibroblasts, VECs) |
Myocardial infarction, ischemia, RAAS activation, inflammation |
TGF-β AngII, aldosterone, ergotamine, methysergide, 5-HT |
Complex cellular responses resulting in activation of fibrotic changes |
Cardiomyopathy |
Single/multiple (CMs, fibroblasts, VECs); substrate scaffolding to mimic tissue architecture |
CAD, MI, HTN, high HR, metabolic disorders, inflammatory conditions, cardiac stimulants, chronic alcohol, multiple drug classes |
Doxorubicin, nilotinib, sunitinib, sorafenib, ponatinib, trastuzumab, 5-fluorouracil, cyclophosphamide, paclitaxel |
Broad multifactorial effects (e.g. ischemia, oxidative stress, mitochondrial injury, metabolic impairment, CM toxicity, dysregulated calcium homeostasis) |
ECM remodelling |
Multiple (CMs, fibroblasts, MSCs) |
Ischemia, tachyarrhythmia, inflammation, pressure overload |
Hypoxia, metalloproteinase-9, stretch |
Substrate scaffold to mimic tissue architecture would be important for ECM remodelling via mechanisms such as stretch or pressure overload |
Immune-mediated or inflammatory responses |
CMs, cardiomyocytes; VECs, vascular endothelial cells; MSCs, mesenchymal stem cells, EADs, early afterdepolarizations; ECM, extracellular matrix; DADs, delayed afterdepolarizations; SR, sarcoplasmic reticulum; CPVT, catecholaminergic ventricular tachycardia; HTN, hypertension; RAAS, renin–angiotensin–aldosterone system; CAD, coronary artery disease; MI, myocardial infarction; HR, heart rate; angII, angiotensin II; ET-1, endothelin-1; TNFα, tumour necrosis factor alpha.
|
Myocardial inflammation, myocarditis |
Multiple (CMs, VECs, lymphocytes) |
Viral and bacterial infection, ischemia, immune checkpoint inhibitors therapy for cancer |
Coxsackievirus B, anti-PD1, PDL1 mAbs, TNFα, IL10, IL8, sulfasalazine, amitriptyline |
Immune-mediated changes in cardiac tissues and normal function |
Outside of electrophysiological evaluation, drug-induced overt structural and functional alterations are of equal concern (Table 2). Development and implementation of in vitro cardiac screening models employing 2D hiPS-CMs to detect drug-induced cardiac alterations can help deconvolute the interconnection among electrical, contractile, and structural contributions to injury (Table 2). One such example use case is the application of CMs in the screening for potential cardiotoxicity of oncology agents.15,20 Through the inclusion of endpoints such as cell and mitochondrial health parameters or via impedance-based fingerprint pattern analysis, multiple platforms have demonstrated the utility of in vitro cardiotoxicity assays for exploring (and screening for) cardiac injury mechanisms.21–23 For certain compounds such as small molecule kinase inhibitors, an additional contextual layer can be gleaned from correlations between kinase inhibition patterns and beat profiles, to identify specific kinases that may influence CV biology, i.e. vascular endothelial growth factor receptor 2 inhibition has been mechanistically linked to alterations in hiPS-CM beat rate and cellular signaling pathways.24,25 This approach can be used upstream in drug discovery to identify cardiovascular liabilities based entirely on kinase inhibition profiles. Thus, context of use in drug discovery for cardiac injury can be used to provide mechanistic insight and guide drug discovery campaigns.
The absence of molecular understanding of all physiological and structural cardiac alterations is why in vitro functional assays with several receptors and active signalling pathways are needed. Positioning of this type of integrated biological assay early in drug discovery may offer the biggest rewards because it can be used to identify potential problems early on using minimum amounts of compound and some, albeit limited, molecular understanding of mechanism. A complex CVMPS may offer an efficient and higher throughput, integrated assessment of a candidate compound's activity on the myriad of ion channels, receptors, pumps, and basic signal transduction mechanisms directly impacting human cellular electrophysiology, key organelles and signalling pathways prior to (or without the) use of in vivo models. Success in this area would ultimately refine and replace the use of in vivo models resulting in a 3Rs benefit and allowing risk assessment to be conducted in a human based model system.
In determining the use of any model as a primary safety screen, several factors need to be taken into consideration including how the data will impact decision-making. For example, one may be willing to exclude drug candidates from further consideration based on in vitro models or one may consider rank-ordering compounds to reduce the number of advancing drug candidates. These considerations are likely to depend on the type of cardiac liability predicted, the confidence of translatability of the effect/liability, and the stage of drug discovery. If a model is developed to merely be representative of a general liability this may limit its use as a broad screening model for multiple cardiac safety liabilities (for example, cardiac failure as a result of a change in function and/or pathology, a model would need to be representative of a range of molecular mechanisms and multiple pathophysiological states). In contrast, if a model is only partly representative of particular in vivo model (i.e. changes in cardiac contractility), then it may be more suitable for a primary or secondary screening assay (i.e., mimicking clinical exposure components) if the sensitivity and specificity are acceptable, allowing a weight of evidence decision on compound progression.
HiPS-CM-based assays are currently routinely used for early cardiac safety screening and/or subsequent mechanistic investigations.14–17 Unlike primary cardiomyocytes isolated from humans or nonclinical species, hiPS-CMs offer the prospect to assess both acute and chronic effects with throughput amenable to early hazard identification. Overcoming these shortcomings of primary cardiomyocytes could enable wider applications. The current use of hiPS-CMs as a key component of an integrated group of cardiac safety models focused on different liabilities such that the totality of multiple sources can be used to assess overall risk. In making weight of evidence assessment of cardiac safety risk, there will be decreasing numbers of in vivo studies needed as the complexity of CVMPS assays and platforms increase. The CVMPS assays of value would improve upon hiPS-CM monolayer and primary cardiomyocyte approaches by increasing the clinical and translational relevance of in vitro approaches. Overcoming these limitations relies on an improved ability to mimic the architecture, physiology and energy dynamics of the adult human heart.
At present, hiPS-CMs are used predominantly for screening (i.e. risk detection) but have seen some use in mechanistic investigations when the receptor or pathway of interest is present. The context of use is influenced by the ability of a model system to mimic the adult human pathophysiology that is being perturbed by a particular compound.26–28 Improving the phenotypic relevance of ion channel expression and resulting action potential waveforms as well as cellular structural organization and composition are properties of hiPS-CMs that differ from adult intact myocardium and thus improvements to these characteristics should increase the impact of hiPS-CM-based assays in drug discovery applications. For example, improving a) the inotropic response associated with adrenergic stimulation as well as b) the force frequency relationship would move away from the identification of a general CV risk detection model to one where specific effects could be phenotypically and mechanistically characterised early and with translational relevance.
The ideal CVMPS model
Functionally, the ideal CVMPS is one that fully recapitulates an integrated system on a smaller scale and when challenged with drug, responds in a manner resembling that found in human, robustly and reproducibly. Key features include biological characteristics (cell type composition and signalling, gene/protein expression, morphological alignment, electrophysiology, contractility and energy dynamics) and platform characteristics (physiological environment of the heart and vasculature i.e. perfusion, sheer stress; endpoint incorporation) as discussed earlier in terms of ideal characteristics (Table 1). Ultimately, the value of a CVMPS model depends on properly controlled experimentation to define the fidelity of translation of the property of interest in a robust and reliable manner.
Proof-of-concept studies should demonstrate the ability of a system to yield an expected (qualitative) response. However, a higher standard is imposed when constructing concentration–response curves to calibrate in vitro responses to known clinical findings (calibrating or quantifying responses). Models demonstrating reproducibility, portability and clinical translatability of systems responses are essential to the further adoption of these models into drug discovery efforts.
Structurally, an ideal MPS platform should include a number of key components, namely a) microfluidic chip or culture chamber, b) live tissue/cell constructs containing multiple relevant cell types including CMs (ventricular and arterial), nodal cells (sinoatrial and atrioventricular), smooth muscle cells, fibroblasts and endothelial cells in proportions, function (electrical, mechanical and energy dynamics) and structure resembling the native human myocardium. c) Components for dynamic culture including in vivo like compound exposure profiles, electrical stimulation, superfusion or perfusion systems (e.g.: vasculature29) and cardiac pre- and after-load, and d) sensors for parameters of interest. Despite technical and engineering advances, many simpler biological preparations presently studied lack the complexity of native multicellular tissues, which may result in incomplete responses to physiological or pharmacological stimulation compared to native human tissues. As flow and pressure gradients in blood vessels result in unique signalling within the CV system, incorporating these elements into a CVMPS platform represents the simplest building block of a truly integrated CVMPS model. Platforms incorporating simpler fluidics may enhance translational fidelity through direct interaction across different tissue constructs and more closely mimic in vivo ‘like’ PK profiles. With any in vitro model increasing structural complexity (and integration, presumably leading to improved responses) comes the risk of reduced reproducibility and other practical limitations. All these factors need to be considered when developing the ideal CVMPS, be it a simpler organ-on-a-chip or a more fully integrated miniaturized CV system. This limitation imposed by bioengineering may pose the greatest limitation for evolving CVMPS platforms, a limitation which is also shared by the field of regenerative medicine.
To assist in identifying the level of complexity and integration of CVMPS models, we propose a multi-tier categorization scheme (Fig. 1). Progressing from level 0 a simple homogenous 2D-monocultures (e.g. cardiomyocyte cultures [with or without genetic manipulation]34 or vascular smooth muscle cells), the first CVMPS model (level I) would include co-cultures, engineered heart tissues, and simpler vascular tissues. These allow for interactions/integration between different cell types based on tissue composition, with the expected benefit of promoting phenotypic maturation (see below). Level II systems would include a tissue or organ biomimics that also contain an open fluidics component mimicking the flow dynamics and shear stress within the heart and within the vasculature being mimicked. Presently, these are more likely to be simple channels that flow solutions through tissue chambers due to difficulties in growing and perfusing media through ingrown vasculature (superfusion vs. perfusion). Level III systems would involve those enabling studies of integrated responses between at least two tissues or organs incorporating vascularized co-cultures fully integrating the cardiac and vascular system. A few such early systems have been used to characterize/describe the influence of hepatic metabolism of drugs on cardiac physiological or structural alterations.35 Finally, level IV CVMPS models would involve multiple organs on a chip integrated by fluidics, with the vasculature consisting of blood-vessel-like structures (i.e. endothelial lined) connecting organs (likely requiring an external pump to enable flow) enabling physiologically relevant substances to be passed from each organ. This would incorporate renal, hepatic and potential neural control, enabling the complex interplay of other organ systems on the CV system to be mimicked. For example, renal function influences CV pathophysiology, hence an integrated system is required to enable study of all aspects of the CV system.
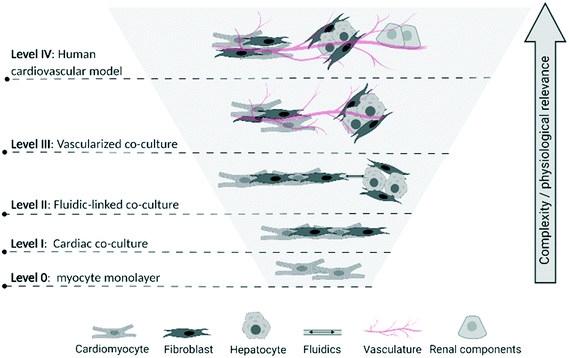 |
| Fig. 1 Proposed multi-tier categorization scheme of biological complexity levels for CVMPS models. Level 0: Homogenous 2D-monocultures; level I co-cultures, engineered heart tissues, and simpler vascular tissues; level II tissue or organ biomimics with open fluidics; level III integrated responses between at least two tissues or organs incorporating vascularized co-cultures; level IV human CVMPS connected by fluidics, with the vasculature consisting of blood-vessel-like structures connecting key organs influencing CV physiology (i.e. renal) and drug metabolism (i.e. liver). The reader is referred to articles cited in section entitled “Survey of Published CVMPS Models”, as well as the Fig. 1 of Schoneberg et al.,30 Gao et al.,31 Wimmer et al.32 and Kim et al.33 for excellent depictions of the various complexity levels we describe here. | |
Survey of published CVMPS models
While many in vitro models of various components of the CV system have been described, few meet the four criteria for a microphysiological system defined above (microfluidic chip or culture chamber; live tissue/cell constructs; components for dynamic culture and sensors for parameters of interest). However, the many types of models described represent CV components that have either been utilized or have potential application in the pharma industry and have the potential to be incorporated into more integrated CV assays. The complexity of these systems varies and extends from simple 2D assays (level 0) all the way to advanced multi-organ flow systems (level III). Similarly, the endpoints of interest may range from single- to multi-parametric analyses. In general, validation efforts for these models are often minimal and limited. As many of these systems have various informal nomenclatures (i.e. heart-on-a-chip), less colloquial subdivisions by MPS type and design are discussed below. It is important to point out that many of these systems span multiple categories that may improve on elements that might be lacking in the simpler systems. The value of these systems to the end user depends significantly on desired endpoints, and whether they support earlier hazard identification or are used to provide risk assessments and mechanistic insights. Some examples are discussed below in the context of our multi-tier categorization scheme.
2D micropatterning (level 0–I)
Although the utility of this one cell-type assay may be limited, it represents one of the most well-characterized with simple, defined endpoints, primarily focusing on cardiac contractility. These assays generally consist of a monolayer of usually ventricular CMs or simple co-cultures of ventricular CMs and cardiac fibroblasts grown on a flexible printed organosilicon matrix (usually polydimethylsiloxane) or aligned along nano-fibers printed onto rigid surfaces, to allow for assessing contractility along with electrical activity.36–41 This 2D micropatterning is sometimes referred to in the literature as “muscular thin film”.36 In some manifestations, fluorescent beads are embedded into a soft gel substrate underlying cells which enables contractility measurements via traction force microscopy.36–41 There are many iterations and types of matrices, and the culture can be monocellular or multicellular, as desired. Similarly, the system is amenable to include CMs from disease models or patients (i.e. Barth syndrome).
Cardiac spheroids and organoids (level I)
Due to overlap and sometimes imprecise use of these terms, the current section defines cardiac spheroids as self-aggregating CMs that are differentiated and subsequently co-cultured in the presence of endothelial cells or fibroblasts. Conversely, organoids are similarly multicellular but are derived from a single progenitor and ‘self-organize through cell sorting and spatially restricted lineage commitment’.42,46 With the rise of iPSC-derived cells, generation of spheroids is more common, most typically through the use of low-adhesion U-bottom plates to initiate aggregation.47–49 Unlike 2D CM monolayers, spheroids can incorporate elements of polarity and CM–matrix interactions. These aspects of 3D culture have been shown to improve the morphology, gene expression, and overall fidelity and performance in reproducing pharmacological responses to drugs focusing on cardiac contractility and structure via confocal images.51
The number of cells that can be incorporated into a nonvascularized sphere without leading to a hypoxic core remains a limitation, and the directionality of the contractile force is not representative of native tissue.48,52 Some additional drawbacks (e.g. limited cell numbers) could be mitigated through pooling of spheroids to provide sufficient tissue for desired analyses. However, this practice is relatively labour intensive and potentially introduces greater variability.
Regenerative medicine: 3D-bioprinted patch (level I)
There is significant medical value to generate cell therapy technologies (i.e. cell patches) capable of repairing or replacing damaged cardiac tissue. These approaches could equally benefit CVMPS models. From a functionality and model development perspective, these regenerative approaches contain many elements of traditional CVMPS models that the field can learn from. The regenerative approaches that could have utility in the CVMPS field typically contain organized structures of CMs that are bioprinted on a porous hyaluronic acid matrix to aid in improving engraftment.68 In addition to CMs, smooth-muscle and endothelial cells can also be included in the bio-weave;34 notably though a drawback might be that increased complexity can reduce the reproducibility of the patch. Thus, careful consideration in balancing the design, and functionality of these systems will continue to be a growing desire in this burgeoning area of research, however the learnings are highly relevant to CVMPS models and can be used to accelerate development.
Co-culture with and without fluidics (level I–II)
These systems often incorporate a matrix with an element of simple flow with perfusion of nutrients via microchannels.43,44 This type of co-culture structure is touted to be more physiologically relevant than simpler systems and usually consists of a hydrogel that is polymerized to generate anisotropic force. These systems are amenable to real-time measurements of contractility and in some cases field potential duration. The dynamic response to drugs has been shown in limited case studies to be more physiologically relevant compared to 2D systems.48–50 Manufacturing advancements in creating reproducible, filamentous matrixes with more control over porosity and improved mechanical force continue to refine the functionality of these systems. These approaches improve the geometrical arrangement of CMs from a random to aligned orientation, freeing CMs from 2D rigid to compliant substrates, coalescing cells into freely suspended fibres tethered to compliant pillars, and exercising the tissues well beyond their spontaneous activity. In other words, engineering features to create a more life-like environment within which the nascent tissues can continue to mature, and acquire characteristics associated with an adult phenotype.45 This approach has been very instructive, as it has led to some improvement of the inotropic responses based on the maturation of the Ca2+-induced Ca2+ release functionality of the CMs. This is due to formation of a t-tubule network and alignment of contractile fibres, resulting in a higher Ca2+ transient to force transduction efficiency. However, even the best of such models lack the feedback provided by a fluidically-linked “connected” cardiac and vascular system.
Vascularised co-cultures (level III)
This type of organ-on-a-chip incorporates elements of advanced flow and is designed to mimic shear force from liquid circulation representative of the vasculature. In simple models, this usually consists of a single cell type or a simple multicellular model lacking advanced vascular structure mimicking the physiological and cellular composition of different vessels (arteries, arterioles, and capillaries).53 Shear stress of the flow can be modulated as desired to mimic healthy or diseased circulation, and early experiments often altered oxygen levels to mimic conditions of hypoxia and subsequent reperfusion.54 These systems can also incorporate basic elements of electrical stimulation to improve the cardiac dynamics of the system.
More complex fluidics with an organized network of vascular structures (including endothelial and smooth muscle cells) have been developed due to recent advances in bio-printing.55 In these systems the CMs showed expression patterns more consistent with maturation of CMs, which is a notable advance towards a physiological and morphological phenotype of the adult myocardium.
3D cardiac tissues with or without microfluidics (level II–III)
Some 3D cardiac tissue technologies are designed to specifically grow differentiated CMs suspended in a matrix in a constrained manner to generate uniaxial stress either around one or between two fixed but flexible structures, forming a structure representative of a tissue.42,56–67 For example, such experiments may include ventricular differentiated iPSCs within a hydrogel matrix. Most of these systems also require a small stromal component, i.e. 10% of cells, to maintain tissue integrity, albeit fibroblast sources can vary across different assays.60 In these systems, contractility, calcium kinetics, and action/field potentials are typical endpoints that are monitored either with or without electric stimulation of the microtissue.56 Uniquely, these tissues form mechanically loaded systems in which force must be generated against static objects, thereby offering a more physiological representation of cardiac contraction. Several groups have demonstrated considerable success in maturation of iPSC-derived CMs by long-term, ramped pacing of tissues with or without serum-free media formulations as evidenced by loss of automaticity, a positive force–frequency relationship, physiological Ca2+ induced Ca2+ release functionality, increased post-rest potentiation, and an increased contractile response to beta-adrenergic stimulation.43,55 Furthermore, heteropolar tissues containing both ventricular and atrial CMs have been generated, enabling researchers to assess compound effects on two myocyte subtypes within a single tissue.53 Other platforms with similar constructs such as the use of flexible cantilevers or ‘posts’ built into a flow chamber where deformations of the cantilever are quantified as a surrogate for strain have also been described.58
Vascular models considerations
The role of the CV system is to provide for exchange of nutrients and oxygen for tissues throughout the body. In support of this activity a complex architecture consisting of larger conductance vessels branching to arteries (conductance vessels supporting flow) leading to arterioles (resistance vessels modulating flow and pressures). These arterioles lead to capillaries and microvessels (the latter considered as structures less than 100 μm in diameter) composed of multiple cell types (typically including endothelial cells, pericytes, and smooth muscle cells differing depending on macro vs. micro vessels). Capillary sites provide for the local exchange of fluid and solutes (including gases) and removal of wastes through the subsequent venous system. Blood flow to organs and tissues is regulated based on metabolic demand, with vascular tone provided by arteriolar resistance vessels under control of circulating as well as local vasoactive substances, central nervous system innervation and mechanical signalling. As optimal oxygen and nutrient delivery in tissues typically requires cells within 100–200 μm from capillary sources, strategies to support the growth and development of smaller scale vascular structures is essential for the growth of more complex spheroids/organoids and organ-chips as well as evolving tissue assays.
The structural complexities and multifunctional characteristics of the vascular system (conductance vs. resistance vs. exchange functions) present formidable challenges for any integrated in vitro vascular assay. Simple models have been described that are useful for investigating the physiology and drug responses of single and multiple component smaller scale resistance and exchange components from multiple organs (reviewed in ref. 69–71). Arguably, conductance vessels need not be considered for organ-on-a-chip systems.
In general, developing in vitro vascular assays have utilized two complementary strategies (reviewed in ref. 72). Angiogenesis describes microvascular growth by sprouting as part of normal vascular development. Vasculogenesis describes microvascular growth resulting from the combination of multiple vascular progenitor cell components. While promising for in vivo and clinical applications, it is difficult to replicate the essential components and signals necessary to generate microvascular growth on chips using either strategy.73 In contrast, microfluidic vascularization approaches promote vascular networks using scaffolds, perfusable channels and patterned biomaterials to promote microvessel growth and maturation. Perfusion pressures and flows can be controlled in such systems, allowing for more realistic simulation of physiologic signalling and drug delivery in these smaller vessels. Such systems (along with 3D bioprinting) have been used to support engineered tissues using CMs.74,75 Techniques to construct synthetic capillaries using human microvascular endothelial cells and live cell lithography have been described and such platforms have been used to explore metastasis in vitro.76,77
A recent elegant study described a perfusable and stretchable gelatin-based microfluidic system to simultaneously apply fluidic shear stress and stretch stress to in vitro 3D tissue culture to mimic the in vivo environment using a 3D extracellular matrix (ECM)-based scaffold.78 It is conceivable that combining this endothelial cell-based system with smooth muscle cells in co-culture would further refine physiological responses to the benefit of fully recapitulating in vivo responses. Such intricate and elegant studies may be challenging to routinely employ in drug discovery screening efforts due to issues of availability, efficiency, and reproducibility.
Another potential use of vascular MPS is the study of nanoparticle-based vascular drug delivery to tumour microenvironments. Recent reviews have highlighted challenges but also guidance for developing such models.79,80 These approaches also provide a potential technological solution to successfully incorporate a vasculature element into a cardiac model, ultimately developing a fully integrated CVMPS platform.
On-chip microfluidic vascularized platforms present a number of additional challenges. While providing a template for vascular growth, such platforms may not be stable for long term studies due to lack of scaffold-derived signals or metabolic signalling that support vascular networks. In addition, the link between parenchymal cells and vessels points to the need for broader tissue engineering efforts to support longer term vascular (and possibly organ-specific vascular network) studies. A benefit of such studies will be the ability to address tissue-specific vascular issues in normal tissues, e.g. blood–brain barrier microvessels as well as tumour tissues to understand the role of blood flow in controlling tumour biology.81,82
It is essential that vascular models be evaluated as “fit for purpose” based on the various components present, mechanisms and pathways operating in the assay, screening questions addressed, and throughput. As greater experience is gained by multiple groups in reproducibly using more complex and integrated assays, their application will likely extend from novel demonstrations and mechanism-specific investigations to more generalized screening for vascular responsiveness and injury.
CV biomarkers in MPS platforms
MPS platforms with a flow component offer a unique opportunity for in vitro systems. Specifically, they enable repeated sampling of media without destroying the cellular components allowing changes in released biomarkers or compound concentration to be measured from the same samples over a time course enabling an assessment of PKPD relationships. Combining such approaches with in-built technology facilitating the assessment of CV function will allow the investigation of the relationship between functional and structural changes in an integrated system.
Management of CV events is facilitated by biomarkers which can be used as indicators of the normal biological or pathological processes or biological response to drugs. There are an increasing number of biomarkers which have been identified over the years through advances made in research and development especially in the CV arena. Having a biomarker with high sensitivity and specificity in the early nonclinical stages will be helpful in screening for any CV liabilities. Inflammation and immunological response are critical elements of disease development and drug-induced toxicities. For example, cardiac troponin I (cTnI) and T (cTnT) are specific for the heart and are indicative of myocardial damage. Whereas N-terminal pro-B type natriuretic peptide (NT-proBNP) is an indicator of cardiac homeostatic responses and dysfunction and is used during the diagnosis of heart failure. In addition to cTn and NT-proBNP, immunological factors such as elevation of C-reactive protein (CRP), a biomarker for inflammation are increasing being applied for diagnostic purposes in cardiovascular disease.
Utilization of multiple biomarkers in MPS platforms is expected to improve safety risk prediction.63 Researchers have been able to incorporate biosensing devices to MPS platforms for rapid detection of heart disease protein biomarkers such as creatine kinase muscle brain (CK-MB), CRP, D-dimer and pregnancy-associated plasma protein A.83 Utilizing human embryonic stem cells, researchers have demonstrated that following chronic biomechanical stress, which is a manifestation of arterial hypertension or valvular disease, there is release of NT-proBNP and cTnT.84 Likewise, to monitor the effect of doxorubicin, continual monitoring of the secreted levels of CK-MB was achieved using a non-invasive biosensor in a heart-on-a-chip model.85
In addition to the protein CV biomarkers, researchers have proposed using biological cells such as white blood cells (biomarker for coronary artery disease) and circulating endothelial progenitor cells (biomarker for pulmonary arterial hypertension) as potential biomarkers using immunofluorescence.86
Identifying, characterizing, predicting, and monitoring drug-induced vascular injury (DIVI) remains a challenge in the industry. Histopathological changes observed in DIVI are generally similar among humans and nonclinical species.87 DIVI is thought to occur via hemodynamic activity (vasodilation or -constriction), primary toxicity to endothelial cells, or secondary injury to vascular tissue via immune complex-mediated inflammation.88 Despite the state of the knowledge of DIVI, one shortcoming has been the identification of biomarkers to predict and/or monitor DIVI in nonclinical or clinical studies.89 A DIVI finding in nonclinical studies would likely halt or considerably delay drug development, even if the finding has uncertain translation to humans, due to the lack of prodromal DIVI biomarkers. For example, several phosphodiesterase 4 (PDE4) inhibitors that were promising treatments for chronic obstructive pulmonary disease have been discontinued due to generation of vasculitis in the mesenteric vasculature of rats,90 though the clinical relevance of these nonclinical observations is uncertain and contentious.91–93
Due to their potential physiological relevance and higher throughput (relative to nonclinical in vivo studies), MPS models may provide a tool to identify and characterize new DIVI biomarkers, as well as investigate the underlying pathobiology related to changes in these potential biomarkers. Further, the testing of tissues from nonclinical species and humans in these models would aid interpretation of interspecies translatability. The Safer and Faster Evidence-based Translation (SAFE-T) Consortium, TransBioLine Consortium and Predictive Safety Testing Consortium (PSTC) have received support from the US FDA94 and EMA95 to further develop and validate DIVI biomarkers, including endothelial cell proteins, inflammatory factors, and smooth muscle cell proteins. Incorporating multiple cell types into CVMPS assays will be critical to the future state of the art.
PKPD modeling and ADME considerations
As highlighted in previous sections the ideal CVMPS model should mimic relevant clinical exposures for mechanistic understanding and translation of PK–PD or PK-toxicological responses. Key to assessing concentration–time profiles within MPS would be facile means of sample acquisition (e.g. media and cells). Furthermore, using plasma concentrations as a surrogate to cardiac exposure may not always be appropriate. A recent publication describes a four-compartment physiologically-based PK model for predicting active drug concentration in different locations of the heart tissue. This model also incorporates cardiac metabolism and, despite needing additional refinement shows promise.96 Another example of a MPS model employed a pumpless heart only chamber and a heart
:
liver chip fluidically linked to successfully describe the PK–PD relationship for terfenadine (a drug which exerts adverse electrophysiological effects at abnormally high plasma exposures). The authors also tested a proprietary AstraZeneca small molecule where they were able to correctly characterize the cardiotoxic response to a metabolite using an in vitro heart
:
liver fluidically linked system.97 More recently a first pass model of human drug absorption, metabolism, and excretion by fluidically coupled two channel microfluidic organ-on-chip platform of the gut, liver, and kidneys through vascular endothelium-lined channels has been described that employs robotic fluid coupling for interrogation of multiple vascularized organ chips.35,98
While cardiac tissue plays a limited role in overall systemic clearance of drugs, it is increasingly recognized that it may be an important determinant of cardiotoxicities and PK–PD responses where local drug or metabolite concentrations can contribute to or drive observed effects.104 Several CYP enzymes are expressed in human cardiac tissues, including CYPs 1A1, 2C8, 2C9, 2D6, 2E1, 2J2 and 4A11. Of these, expression of CYP2J2 is known to be unusually high (relative to other tissues with detectable CYP expression) and has been shown to catalyse the formation of epoxyeicosatrienoic acid metabolites (EETs) from arachidonic acid.99,100 EETs are thought to function as anti-inflammatory and anti-fibrotic agents in addition to regulating vascular tone.101,102 Modulation of this pathway by doxorubicin has been postulated to contribute to the dose-dependent cardiotoxicity observed.103 In addition to drug metabolizing enzymes, expression of transporters in the heart is important to consider in understanding distribution of systemically available xenobiotics and associated metabolites and their subsequent role in cardiotoxicity and drug effect. Multiple solute carrier transporters have been identified in the heart, including OATP4A1, OATP2A1, OAT2B1, OATP3A1, OCTN1, OCTN2 and OCTN3.104 OCTN2 is a high-affinity carnitine transporter confined to cardiac endothelial cells and has been shown to be involved in the transport of verapamil and spironolactone to cardiac tissue. Rare genetic defects at the protein level can lead to cardiomyopathy.105 It is further postulated that OCTN2 may function co-ordinately with P-gp, which along with MRP5 is also expressed in cardiac tissue.106 Immature iPSC assays are sometimes perceived as being unable to recapitulate physiologically relevant drug metabolism and transport and due to the limitations of culturing primary cardiomyocytes, these are not considered a viable alternative. Thus, CVMPS assays should ideally possess relevant protein expression and function of the appropriate drug metabolizing enzymes and transporters. Incorporating PK–PD translation of physiological and structural cardiac alterations in nonclinical CVMPS models may also build confidence in applying these systems towards mechanistic insights or prediction of cardiac liabilities in human.
Current limitations, future expectations and concluding remarks
Over the last few years significant progress has been made in developing and characterizing simpler 2D and more complex 3D human stem-cell derived cardiac assays to promote CM maturation (level 0–I models). Similar strides have been made for human-derived vascular models. With these advancements, the future state-of-the-art for CVMPS will hopefully combine these assays with fluidics (level II–III models) to more fully recapitulate the intended physiology delivering the ideal CVMPS model described (level IV). While considerable challenges and limitations exist, the progress made in the MPS field as a whole provides promise towards development of a more robust CVMPS. In order for the ideal CVMPS model to be realized (Table 1) a number of limitations need to be addressed to ensure CVMPS models are fit for the context of use for the future of drug discovery and development and enabling the diversity of cardiovascular safety findings to be both identified and investigated in a single model system. Specifically, mimicking in vivo CV responses in terms of cardiac structure, haemodynamics and energy dynamics requires further investment to ensure models can be applied in multiple context-of-use settings. Success would enable connecting key organs which physiologically influence the CV system together. In addition, as in vitro model systems increase in complexity so must the ability of platforms to monitor additional structural and functional biomarkers enabling full benefit to be realized. Considering the diversity of CV safety liabilities, a future CVMPS model would provide functional, morphological, PK, and mechanistic information in a temporal manner. Such an approach would allow the development of insights (insights into complex mechanisms) and detection that is not amenable to current non-human in vitro platforms. In addition, this approach opens the possibility of PK–PD or systems pharmacology modelling to be applied enabling the development of quantitative translational understanding based on human models. Further, moving towards a non-destructive, biomarker-based system or live cell imaging may allow for a better PK–PD understanding and insights into the relationship between function and structure that ultimately leads to improved translation. Currently the majority of platforms focus on developing the pathophysiology of the healthy CV system. While laudable, future expectations would be that diseased phenotype systems are developed to allow both efficacy and safety to be determined from one model system, as well as allowing safety questions requiring a patient phenotype to be investigated prior to clinical development.107,108 Developing such models for healthy and disease states will further expand the capability of in vitro CV safety strategies and the overarching goals of MPS systems, and the refinement and replacement of small animal in vivo studies over time. As these models continue to be developed increased emphasis needs to be placed on pharmacology validation and experimental reproducibility to ensure the resulting models can be used for quantitative decision-making during drug discovery.
The ultimate application of models within drug discovery and development is dependent on both the context of use and the ability to develop CVMPS models demonstrating biological responses and platform characteristics that provide functional, morphological, PK, and mechanistic information to be generated in a temporally relevant manner. These characteristics need to be balanced with model efficiency, experimental reproducibility, and added value above current strategies to facilitate adoption by pharma. To achieve this, further development of CVMPS models is required; these initiatives encompass broad and diverse areas of expertise and specialization (along with different expectations of different participants) that are best served by partnerships between government-funded initiatives, academics, the private sector, and pharma industry.
Author contributions
Amy Pointon, Jonathan Maher, Myrtle Davis, Thomas Baker, Joseph Cichocki, Diane Ramsden, Kyle L. Kolaja, Paul Levesque, Christopher Hale, Radhakrishna Sura, David M. Stresser, Gary Gintant all contributed to the writing, reviewing and editing of this manuscript.
Conflicts of interest
There are no conflicts to declare.
Acknowledgements
This manuscript was developed with the support of the International Consortium for Innovation and Quality in Pharmaceutical Development (IQ, http://www.iqconsortium.org). IQ is a not-for-profit organization of pharmaceutical and biotechnology companies with a mission of advancing science and technology to augment the capability of member companies to develop transformational solutions that benefit patients, regulators and the broader research and development community.
References
- D. Cook, D. Brown, R. Alexander, R. March, P. Morgan, G. Satterthwaite and M. N. Pangalos, Nat. Rev. Drug Discovery, 2014, 13, 419–431 CrossRef CAS.
- H. Laverty, C. Benson, E. Cartwright, M. Cross, C. Garland, T. Hammond, C. Holloway, N. McMahon, J. Milligan, B. Park, M. Pirmohamed, C. Pollard, J. Radford, N. Roome, P. Sager, S. Singh, T. Suter, W. Suter, A. Trafford, P. Volders, R. Wallis, R. Weaver, M. York and J. Valentin, Br. J. Pharmacol., 2011, 163, 675–693 CrossRef CAS.
- P. Morgan, D. G. Brown, S. Lennard, M. J. Anderton, J. C. Barrett, U. Eriksson, M. Fidock, B. Hamren, A. Johnson, R. E. March, J. Matcham, J. Mettetal, D. J. Nicholls, S. Platz, S. Rees, M. A. Snowden and M. N. Pangalos, Nat. Rev. Drug Discovery, 2018, 17, 167–181 CrossRef CAS.
- P. Ferdinandy, I. Baczko, P. Bencsik, Z. Giricz, A. Gorbe, P. Pacher, Z. V. Varga, A. Varro and R. Schulz, Eur. Heart J., 2019, 40, 1771–1777 CrossRef CAS.
- L. Ewart, E. M. Dehne, K. Fabre, S. Gibbs, J. Hickman, E. Hornberg, M. Ingelman-Sundberg, K. J. Jang, D. R. Jones, V. M. Lauschke, U. Marx, J. T. Mettetal, A. Pointon, D. Williams, W. H. Zimmermann and P. Newham, Annu. Rev. Pharmacol. Toxicol., 2018, 58, 65–82 CrossRef CAS.
- U. Marx, T. Akabane, T. B. Andersson, E. Baker, M. Beilmann, S. Beken, S. Brendler-Schwaab, M. Cirit, R. David, E. M. Dehne, I. Durieux, L. Ewart, S. C. Fitzpatrick, O. Frey, F. Fuchs, L. G. Griffith, G. A. Hamilton, T. Hartung, J. Hoeng, H. Hogberg, D. J. Hughes, D. E. Ingber, A. Iskandar, T. Kanamori, H. Kojima, J. Kuehnl, M. Leist, B. Li, P. Loskill, D. L. Mendrick, T. Neumann, G. Pallocca, I. Rusyn, L. Smirnova, T. Steger-Hartmann, D. A. Tagle, A. Tonevitsky, S. Tsyb, M. Trapecar, B. Van de Water, J. Van den Eijnden-van Raaij, P. Vulto, K. Watanabe, A. Wolf, X. Zhou and A. Roth, ALTEX, 2020, 37, 364–394 Search PubMed.
- U. Marx, T. B. Andersson, A. Bahinski, M. Beilmann, S. Beken, F. R. Cassee, M. Cirit, M. Daneshian, S. Fitzpatrick, O. Frey, C. Gaertner, C. Giese, L. Griffith, T. Hartung, M. B. Heringa, J. Hoeng, W. H. de Jong, H. Kojima, J. Kuehnl, M. Leist, A. Luch, I. Maschmeyer, D. Sakharov, A. J. Sips, T. Steger-Hartmann, D. A. Tagle, A. Tonevitsky, T. Tralau, S. Tsyb, A. van de Stolpe, R. Vandebriel, P. Vulto, J. Wang, J. Wiest, M. Rodenburg and A. Roth, ALTEX, 2016, 33, 272–321 Search PubMed.
- M. Zamani, E. Karaca and N. F. Huang, Front. Cardiovasc. Med., 2018, 5, 147 CrossRef CAS.
- R. Harmer, N. Abi-Gerges, M. J. Morton, G. F. Pullen, J. P. Valentin and C. E. Pollard, Toxicol. Appl. Pharmacol., 2012, 260, 162–172 CrossRef.
- O. Muller, Q. Tian, R. Zantl, V. Kahl, P. Lipp and L. Kaestner, Cell Calcium, 2010, 47, 224–233 CrossRef.
- M. J. Morton, D. Armstrong, N. Abi Gerges, M. Bridgland-Taylor, C. E. Pollard, J. Bowes and J. P. Valentin, Toxicol. Appl. Pharmacol., 2014, 279, 87–94 CrossRef CAS.
- L. Butler, C. Cros, K. L. Oldman, A. R. Harmer, A. Pointon, C. E. Pollard and N. Abi-Gerges, Toxicol. Sci., 2015, 145, 396–406 CrossRef CAS.
- K. Blinova, Q. Dang, D. Millard, G. Smith, J. Pierson, L. Guo, M. Brock, H. R. Lu, U. Kraushaar, H. Zeng, H. Shi, X. Zhang, K. Sawada, T. Osada, Y. Kanda, Y. Sekino, L. Pang, T. K. Feaster, R. Kettenhofen, N. Stockbridge, D. G. Strauss and G. Gintant, Cell Rep., 2018, 24, 3582–3592 CrossRef CAS.
- T. Bot, K. Juhasz, F. Haeusermann, L. Polonchuk, M. Traebert and S. Stoelzle-Feix, J. Pharmacol. Toxicol. Methods, 2018, 93, 46–58 CrossRef.
- G. Gintant, B. Fermini, N. Stockbridge and D. Strauss, Cell Stem Cell, 2017, 21, 14–17 CrossRef CAS.
- G. Gintant, P. Burridge, L. Gepstein, S. Harding, T. Herron, C. Hong, J. Jalife and J. C. Wu, Circ. Res., 2019, 125, e75–e92 CrossRef CAS.
- T. de Korte, P. A. Katili, N. A. N. Mohd Yusof, B. J. van Meer, U. Saleem, F. L. Burton, G. L. Smith, P. Clements, C. L. Mummery, T. Eschenhagen, A. Hansen and C. Denning, Annu. Rev. Pharmacol. Toxicol., 2020, 60, 529–551 CrossRef CAS.
- A. Satsuka and Y. Kanda, Curr. Pharm. Biotechnol., 2020, 21, 765–772 CAS.
- P. Beauchamp, C. B. Jackson, L. C. Ozhathil, I. Agarkova, C. L. Galindo, D. B. Sawyer, T. M. Suter and C. Zuppinger, Front. Mol. Biosci., 2020, 7, 14 CrossRef CAS.
- T. Force and K. L. Kolaja, Nat. Rev. Drug Discovery, 2011, 10, 111–126 CrossRef CAS.
- D. R. Talbert, K. R. Doherty, P. B. Trusk, D. M. Moran, S. A. Shell and S. Bacus, Toxicol. Sci., 2015, 143, 147–155 CrossRef CAS.
- B. Koci, G. Luerman, A. Duenbostell, R. Kettenhofen, H. Bohlen, L. Coyle, B. Knight, W. Ku, W. Volberg, J. R. Woska, Jr. and M. P. Brown, Toxicol. Appl. Pharmacol., 2017, 329, 121–127 CrossRef CAS.
- D. L. Misner, C. Frantz, L. Guo, M. R. Gralinski, P. B. Senese, J. Ly, M. Albassam and K. L. Kolaja, Br. J. Pharmacol., 2012, 165, 2771–2786 CrossRef CAS.
- A. Sharma, P. W. Burridge, W. L. McKeithan, R. Serrano, P. Shukla, N. Sayed, J. M. Churko, T. Kitani, H. Wu, A. Holmstrom, E. Matsa, Y. Zhang, A. Kumar, A. C. Fan, J. C. Del Alamo, S. M. Wu, J. J. Moslehi, M. Mercola and J. C. Wu, Sci. Transl. Med., 2017, 9, eaaf2584 CrossRef.
- S. D. Lamore, E. Ahlberg, S. Boyer, M. L. Lamb, M. P. Hortigon-Vinagre, V. Rodriguez, G. L. Smith, J. Sagemark, L. Carlsson, S. M. Bates, A. L. Choy, J. Stalring, C. W. Scott and M. F. Peters, Toxicol. Sci., 2017, 158, 213–226 CrossRef CAS.
- D. Millard, Q. Dang, H. Shi, X. Zhang, C. Strock, U. Kraushaar, H. Zeng, P. Levesque, H. R. Lu, J. M. Guillon, J. C. Wu, Y. Li, G. Luerman, B. Anson, L. Guo, M. Clements, Y. A. Abassi, J. Ross, J. Pierson and G. Gintant, Toxicol. Sci., 2018, 164, 550–562 CrossRef CAS.
- I. Mannhardt, A. Eder, B. Dumotier, M. Prondzynski, E. Kramer, M. Traebert, K. D. Sohren, F. Flenner, K. Stathopoulou, M. D. Lemoine, L. Carrier, T. Christ, T. Eschenhagen and A. Hansen, Toxicol. Sci., 2017, 158, 164–175 CrossRef CAS.
- A. Pointon, N. Abi-Gerges, M. J. Cross and J. E. Sidaway, Toxicol. Sci., 2013, 132, 317–326 CrossRef CAS.
- P. Sasmal, P. Datta, Y. Wu and I. T. Ozbolat, Microphysiol. Syst., 2018, 2, 9 Search PubMed.
- J. Schoneberg, F. De Lorenzi, B. Theek, A. Blaeser, D. Rommel, A. J. C. Kuehne, F. Kiessling and H. Fischer, Sci. Rep., 2018, 8, 10430 CrossRef.
- G. Gao, J. Y. Park, B. S. Kim, J. Jang and D. W. Cho, Adv. Healthcare Mater., 2018, 7, e1801102 CrossRef.
- R. A. Wimmer, A. Leopoldi, M. Aichinger, D. Kerjaschki and J. M. Penninger, Nat. Protoc., 2019, 14, 3082–3100 CrossRef CAS.
- S. Kim, W. Kim, S. Lim and J. S. Jeon, Bioengineering, 2017, 4 Search PubMed.
- J. T. Hinson, A. Chopra, N. Nafissi, W. J. Polacheck, C. C. Benson, S. Swist, J. Gorham, L. Yang, S. Schafer, C. C. Sheng, A. Haghighi, J. Homsy, N. Hubner, G. Church, S. A. Cook, W. A. Linke, C.
S. Chen, J. G. Seidman and C. E. Seidman, Science, 2015, 349, 982–986 CrossRef CAS.
- A. Herland, B. M. Maoz, D. Das, M. R. Somayaji, R. Prantil-Baun, R. Novak, M. Cronce, T. Huffstater, S. S. F. Jeanty, M. Ingram, A. Chalkiadaki, D. Benson Chou, S. Marquez, A. Delahanty, S. Jalili-Firoozinezhad, Y. Milton, A. Sontheimer-Phelps, B. Swenor, O. Levy, K. K. Parker, A. Przekwas and D. E. Ingber, Nat. Biomed. Eng., 2020, 4, 421–436 CrossRef CAS.
- A. Grosberg, P. W. Alford, M. L. McCain and K. K. Parker, Lab Chip, 2011, 11, 4165–4173 RSC.
- G. Wang, M. L. McCain, L. Yang, A. He, F. S. Pasqualini, A. Agarwal, H. Yuan, D. Jiang, D. Zhang, L. Zangi, J. Geva, A. E. Roberts, Q. Ma, J. Ding, J. Chen, D. Z. Wang, K. Li, J. Wang, R. J. Wanders, W. Kulik, F. M. Vaz, M. A. Laflamme, C. E. Murry, K. R. Chien, R. I. Kelley, G. M. Church, K. K. Parker and W. T. Pu, Nat. Med., 2014, 20, 616–623 CrossRef CAS.
- A. J. Ribeiro, Y. S. Ang, J. D. Fu, R. N. Rivas, T. M. Mohamed, G. C. Higgs, D. Srivastava and B. L. Pruitt, Proc. Natl. Acad. Sci. U. S. A., 2015, 112, 12705–12710 CrossRef CAS.
- A. Lee, A. R. Hudson, D. J. Shiwarski, J. W. Tashman, T. J. Hinton, S. Yerneni, J. M. Bliley, P. G. Campbell and A. W. Feinberg, Science, 2019, 365, 482–487 CrossRef CAS.
- N. Noor, A. Shapira, R. Edri, I. Gal, L. Wertheim and T. Dvir, Adv. Sci., 2019, 6, 1900344 CrossRef.
- M. Takeda, S. Miyagawa, S. Fukushima, A. Saito, E. Ito, A. Harada, R. Matsuura, H. Iseoka, N. Sougawa, N. Mochizuki-Oda, M. Matsusaki, M. Akashi and Y. Sawa, Tissue Eng., Part C, 2018, 24, 56–67 CrossRef CAS.
- R. J. Mills, B. L. Parker, G. A. Quaife-Ryan, H. K. Voges, E. J. Needham, A. Bornot, M. Ding, H. Andersson, M. Polla, D. A. Elliott, L. Drowley, M. Clausen, A. T. Plowright, I. P. Barrett, Q. D. Wang, D. E. James, E. R. Porrello and J. E. Hudson, Cell Stem Cell, 2019, 24, 895–907 CrossRef CAS , e896.
- A. Aung, I. S. Bhullar, J. Theprungsirikul, S. K. Davey, H. L. Lim, Y. J. Chiu, X. Ma, S. Dewan, Y. H. Lo, A. McCulloch and S. Varghese, Lab Chip, 2016, 16, 153–162 RSC.
- Z. Ma, S. Koo, M. A. Finnegan, P. Loskill, N. Huebsch, N. C. Marks, B. R. Conklin, C. P. Grigoropoulos and K. E. Healy, Biomaterials, 2014, 35, 1367–1377 CrossRef CAS.
- K. Ronaldson-Bouchard and G. Vunjak-Novakovic, Cell Stem Cell, 2018, 22, 310–324 CrossRef CAS.
- M. A. Lancaster and J. A. Knoblich, Science, 2014, 345, 1247125 CrossRef.
- P. Bartholoma, E. Gorjup, D. Monz, A. Reininger-Mack, H. Thielecke and A. Robitzki, J. Biomol. Screening, 2005, 10, 814–822 CrossRef CAS.
- C. R. Archer, R. Sargeant, J. Basak, J. Pilling, J. R. Barnes and A. Pointon, Sci. Rep., 2018, 8, 10160 CrossRef.
- L. Polonchuk, M. Chabria, L. Badi, J. C. Hoflack, G. Figtree, M. J. Davies and C. Gentile, Sci. Rep., 2017, 7, 7005 CrossRef.
- A. Pointon, J. Pilling, T. Dorval, Y. Wang, C. Archer and C. Pollard, Toxicol. Sci., 2017, 155, 444–457 CrossRef CAS.
- O. Sirenko, M. K. Hancock, C. Crittenden, M. Hammer, S. Keating, C. B. Carlson and G. Chandy, Assay Drug Dev. Technol., 2017, 15, 280–296 CrossRef CAS.
- I. Pitaktong, C. Lui, J. Lowenthal, G. Mattson, W. H. Jung, Y. Bai, E. Yeung, C. S. Ong, Y. Chen, S. Gerecht and N. Hibino, Tissue Eng., Part C, 2020, 26, 80–90 CrossRef CAS.
- J. Ribas, H. Sadeghi, A. Manbachi, J. Leijten, K. Brinegar, Y. S. Zhang, L. Ferreira and A. Khademhosseini, Appl. In Vitro Toxicol., 2016, 2, 82–96 CrossRef.
- T. Chen and G. Vunjak-Novakovic, Tissue Eng., Part A, 2019, 25, 711–724 CrossRef.
- Y. S. Zhang, J. Aleman, S. R. Shin, T. Kilic, D. Kim, S. A. Mousavi Shaegh, S. Massa, R. Riahi, S. Chae, N. Hu, H. Avci, W. Zhang, A. Silvestri, A. Sanati Nezhad, A. Manbohi, F. De Ferrari, A. Polini, G. Calzone, N. Shaikh, P. Alerasool, E. Budina, J. Kang, N. Bhise, J. Ribas, A. Pourmand, A. Skardal, T. Shupe, C. E. Bishop, M. R. Dokmeci, A. Atala and A. Khademhosseini, Proc. Natl. Acad. Sci. U. S. A., 2017, 114, E2293–E2302 CrossRef CAS.
- K. Ronaldson-Bouchard, S. P. Ma, K. Yeager, T. Chen, L. Song, D. Sirabella, K. Morikawa, D. Teles, M. Yazawa and G. Vunjak-Novakovic, Nature, 2018, 556, 239–243 CrossRef CAS.
- A. K. Schroer, M. S. Shotwell, V. Y. Sidorov, J. P. Wikswo and W. D. Merryman, Acta Biomater., 2017, 48, 79–87 CrossRef CAS.
- N. Thavandiran, N. Dubois, A. Mikryukov, S. Masse, B. Beca, C. A. Simmons, V. S. Deshpande, J. P. McGarry, C. S. Chen, K. Nanthakumar, G. M. Keller, M. Radisic and P. W. Zandstra, Proc. Natl. Acad. Sci. U. S. A., 2013, 110, E4698–E4707 CrossRef CAS.
- Y. Zhao, N. Rafatian, N. T. Feric, B. J. Cox, R. Aschar-Sobbi, E. Y. Wang, P. Aggarwal, B. Zhang, G. Conant, K. Ronaldson-Bouchard, A. Pahnke, S. Protze, J. H. Lee, L. Davenport Huyer, D. Jekic, A. Wickeler, H. E. Naguib, G. M. Keller, G. Vunjak-Novakovic, U. Broeckel, P. H. Backx and M. Radisic, Cell, 2019, 176, 913–927 CrossRef CAS , e918.
- M. Tiburcy, J. E. Hudson, P. Balfanz, S. Schlick, T. Meyer, M. L. Chang Liao, E. Levent, F. Raad, S. Zeidler, E. Wingender, J. Riegler, M. Wang, J. D. Gold, I. Kehat, E. Wettwer, U. Ravens, P. Dierickx, L. W. van Laake, M. J. Goumans, S. Khadjeh, K. Toischer, G. Hasenfuss, L. A. Couture, A. Unger, W. A. Linke, T. Araki, B. Neel, G. Keller, L. Gepstein, J. C. Wu and W. H. Zimmermann, Circulation, 2017, 135, 1832–1847 CrossRef CAS.
- I. Mannhardt, K. Breckwoldt, D. Letuffe-Breniere, S. Schaaf, H. Schulz, C. Neuber, A. Benzin, T. Werner, A. Eder, T. Schulze, B. Klampe, T. Christ, M. N. Hirt, N. Huebner, A. Moretti, T. Eschenhagen and A. Hansen, Stem Cell Rep., 2016, 7, 29–42 CrossRef CAS.
- S. S. Nunes, J. W. Miklas, J. Liu, R. Aschar-Sobbi, Y. Xiao, B. Zhang, J. Jiang, S. Masse, M. Gagliardi, A. Hsieh, N. Thavandiran, M. A. Laflamme, K. Nanthakumar, G. J. Gross, P. H. Backx, G. Keller and M. Radisic, Nat. Methods, 2013, 10, 781–787 CrossRef CAS.
- N. T. Feric, I. Pallotta, R. Singh, D. R. Bogdanowicz, M. Gustilo, K. Chaudhary, R. N. Willette, T. Chendrimada, X. Xu, M. P. Graziano and R. Aschar-Sobbi, Toxicol. Sci., 2019, 172, 89–97 CrossRef CAS.
- S. S. Nunes, J. W. Miklas, J. Liu, R. Aschar-Sobbi, Y. Xiao, B. Zhang, J. Jiang, S. Masse, M. Gagliardi, A. Hsieh, N. Thavandiran, M. A. Laflamme, K. Nanthakumar, G. J. Gross, P. H. Backx, G. Keller and M. Radisic, Nat. Methods, 2013, 10, 781–787 CrossRef CAS.
- M. N. Hirt, J. Boeddinghaus, A. Mitchell, S. Schaaf, C. Bornchen, C. Muller, H. Schulz, N. Hubner, J. Stenzig, A. Stoehr, C. Neuber, A. Eder, P. K. Luther, A. Hansen and T. Eschenhagen, J. Mol. Cell. Cardiol., 2014, 74, 151–161 CrossRef CAS.
- E. J. Lee, D. E. Kim, E. U. Azeloglu and K. D. Costa, Tissue Eng., Part A, 2008, 14, 215–225 CrossRef CAS.
- R. A. Li, W. Keung, T. J. Cashman, P. C. Backeris, B. V. Johnson, E. S. Bardot, A. O. T. Wong, P. K. W. Chan, C. W. Y. Chan and K. D. Costa, Biomaterials, 2018, 163, 116–127 CrossRef CAS.
- A. Marsano, C. Conficconi, M. Lemme, P. Occhetta, E. Gaudiello, E. Votta, G. Cerino, A. Redaelli and M. Rasponi, Lab Chip, 2016, 16, 599–610 RSC.
- A. Cochrane, H. J. Albers, R. Passier, C. L. Mummery, A. van den Berg, V. V. Orlova and A. D. van der Meer, Adv. Drug Delivery Rev., 2019, 140, 68–77 CrossRef CAS.
- D. S. Y. Lin, F. Guo and B. Zhang, Nanotechnology, 2019, 30, 024002 CrossRef.
- A. R. Henderson, H. Choi and E. Lee, Micromachines, 2020, 11, 2 CrossRef.
- J. Tien, Compr. Physiol., 2019, 9, 1155–1212 Search PubMed.
- H. G. Song, R. T. Rumma, C. K. Ozaki, E. R. Edelman and C. S. Chen, Cell Stem Cell, 2018, 22, 340–354 CrossRef CAS.
- K. Sakaguchi, T. Shimizu, S. Horaguchi, H. Sekine, M. Yamato, M. Umezu and T. Okano, Sci. Rep., 2013, 3, 1316 CrossRef.
- C. S. Ong, L. Nam, K. Ong, A. Krishnan, C. Y. Huang, T. Fukunishi and N. Hibino, BioMed Res. Int., 2018, 2018, 6497242 Search PubMed.
- K. Sarveswaran, V. Kurz, Z. Dong, T. Tanaka, S. Penny and G. Timp, Sci. Rep., 2016, 6, 21885 CrossRef CAS.
- L. A. Hapach, J. A. Mosier, W. Wang and C. A. Reinhart-King, npj Precis. Oncol., 2019, 3, 20 CrossRef.
- A. Shimizu, W. H. Goh, S. Itai, M. Hashimoto, S. Miura and H. Onoe, Lab Chip, 2020, 20, 1917–1927 RSC.
- D. Caballero, S. M. Blackburn, M. de Pablo, J. Samitier and L. Albertazzi, Lab Chip, 2017, 17, 3760–3771 RSC.
- Y. Li, K. Zhu, X. Liu and Y. S. Zhang, Curr. Drug Metab., 2018, 19, 100–109 CrossRef CAS.
- R. M. Linville, J. G. DeStefano, M. B. Sklar, Z. Xu, A. M. Farrell, M. I. Bogorad, C. Chu, P. Walczak, L. Cheng, V. Mahairaki, K. A. Whartenby, P. A. Calabresi and P. C. Searson, Biomaterials, 2019, 190–191, 24–37 CrossRef CAS.
- N. Dhiman, P. Kingshott, H. Sumer, C. S. Sharma and S. N. Rath, Biosens. Bioelectron., 2019, 137, 236–254 CrossRef CAS.
- K. Mitsakakis and E. Gizeli, Anal. Chim. Acta, 2011, 699, 1–5 CrossRef CAS.
- E. Ovchinnikova, M. Hoes, K. Ustyantsev, N. Bomer, T. V. de Jong, H. van der Mei, E. Berezikov and P. van der Meer, Stem Cell Rep., 2018, 10, 794–807 CrossRef CAS.
- S. R. Shin, Y. S. Zhang, D. J. Kim, A. Manbohi, H. Avci, A. Silvestri, J. Aleman, N. Hu, T. Kilic, W. Keung, M. Righi, P. Assawes, H. A. Alhadrami, R. A. Li, M. R. Dokmeci and A. Khademhosseini, Anal. Chem., 2016, 88, 10019–10027 CrossRef CAS.
- J. Wu, M. Dong, S. Santos, C. Rigatto, Y. Liu and F. Lin, Sensors, 2017, 17, 12 CrossRef.
-
S. Guionaud, P. Cacoub, M. Lawton, K. Bendjama and H. Firat, 30th Annual Symposium of Society of Toxicologic Pathology, Denver, CO, 2011, 24.
- I. Mikaelian, M. Cameron, D. A. Dalmas, B. E. Enerson, R. J. Gonzalez, S. Guionaud, P. K. Hoffmann, N. M. King, M. P. Lawton, M. S. Scicchitano, H. W. Smith, R. A. Thomas, J. L. Weaver, T. S. Zabka and C. Vascular Injury Working Group of the Predictive Safety, Toxicol. Pathol., 2014, 42, 635–657 CrossRef.
- K. Bendjama, S. Guionaud, G. Aras, N. Arber, L. Badimon, U. Bamberger, D. Bratfalean, D. Brott, M. David, L. Doessegger, H. Firat, J. F. Gallas, J. C. Gautier, P. Hoffmann, S. Kraus, T. Padro, D. Saadoun, P. Szczesny, P. Thomann, G. Vilahur, M. Lawton and P. Cacoub, Toxicol. Pathol., 2014, 42, 658–671 CrossRef CAS.
- N. Dagues, V. Pawlowski, C. Sobry, G. Hanton, F. Borde, S. Soler, J. L. Freslon and S. Chevalier, Toxicol. Sci., 2007, 100, 238–247 CrossRef CAS.
- J. T. Sobota, Toxicol. Pathol., 1989, 17, 193–202 CrossRef CAS.
- D. Spina, Br. J. Pharmacol., 2008, 155, 308–315 CrossRef CAS.
- M. A. Giembycz, Br. J. Clin. Pharmacol., 2006, 62, 138–152 CrossRef CAS.
-
FDA, https://www.fda.gov/media/101648/download, (accessed January 2020).
-
EMA, https://www.ema.europa.eu/en/documents/other/letter-support-drug-induced-vascular-injury-divi-biomarker_en.pdf, (accessed January 2020).
- Z. Tylutki and S. Polak, Sci. Rep., 2017, 7, 39494 CrossRef CAS.
- C. W. McAleer, A. Pointon, C. J. Long, R. L. Brighton, B. D. Wilkin, L. R. Bridges, N. Narasimhan Sriram, K. Fabre, R. McDougall, V. P. Muse, J. T. Mettetal, A. Srivastava, D. Williams, M. T. Schnepper, J. L. Roles, M. L. Shuler, J. J. Hickman and L. Ewart, Sci. Rep., 2019, 9, 9619 CrossRef.
- R. Novak, M. Ingram, S. Marquez, D. Das, A. Delahanty, A. Herland, B. M. Maoz, S. S. F. Jeanty, M. R. Somayaji, M. Burt, E. Calamari, A. Chalkiadaki, A. Cho, Y. Choe, D. B. Chou, M. Cronce, S. Dauth, T. Divic, J. Fernandez-Alcon, T. Ferrante, J. Ferrier, E. A. FitzGerald, R. Fleming, S. Jalili-Firoozinezhad, T. Grevesse, J. A. Goss, T. Hamkins-Indik, O. Henry, C. Hinojosa, T. Huffstater, K. J. Jang, V. Kujala, L. Leng, R. Mannix, Y. Milton, J. Nawroth, B. A. Nestor, C. F. Ng, B. O'Connor, T. E. Park, H. Sanchez, J. Sliz, A. Sontheimer-Phelps, B. Swenor, G. Thompson, 2nd, G. J. Touloumes, Z. Tranchemontagne, N. Wen, M. Yadid, A. Bahinski, G. A. Hamilton, D. Levner, O. Levy, A. Przekwas, R. Prantil-Baun, K. K. Parker and D. E. Ingber, Nat. Biomed. Eng., 2020, 4, 407–420 CrossRef.
- M. Solanki, A. Pointon, B. Jones and K. Herbert, Drug Metab. Dispos., 2018, 46, 1053–1065 CrossRef CAS.
- S. Wu, C. R. Moomaw, K. B. Tomer, J. R. Falck and D. C. Zeldin, J. Biol. Chem., 1996, 271, 3460–3468 CrossRef CAS.
- I. Fleming, Circ. Res., 2001, 89, 753–762 CrossRef CAS.
- K. N. Theken and C. R. Lee, Pharmacogenomics, 2007, 8, 1369–1383 CrossRef CAS.
- W. R. Arnold, J. L. Baylon, E. Tajkhorshid and A. Das, Biochemistry, 2017, 56, 6700–6712 CrossRef CAS.
- E. A. Hausner, S. A. Elmore and X. Yang, Drug Metab. Dispos., 2019, 47, 673–688 CrossRef CAS.
- M. Grube, S. Ameling, M. Noutsias, K. Kock, I. Triebel, K. Bonitz, K. Meissner, G. Jedlitschky, L. R. Herda, M. Reinthaler, M. Rohde, W. Hoffmann, U. Kuhl, H. P. Schultheiss, U. Volker, S. B. Felix, K. Klingel, R. Kandolf and H. K. Kroemer, Am. J. Pathol., 2011, 178, 2547–2559 CrossRef CAS.
- M. Grube, H. E. Meyer zu Schwabedissen, D. Prager, J. Haney, K. U. Moritz, K. Meissner, D. Rosskopf, L. Eckel, M. Bohm, G. Jedlitschky and H. K. Kroemer, Circulation, 2006, 113, 1114–1122 CrossRef.
- P. W. Burridge, Y. F. Li, E. Matsa, H. Wu, S. G. Ong, A. Sharma, A. Holmstrom, A. C. Chang, M. J. Coronado, A. D. Ebert, J. W. Knowles, M. L. Telli, R. M. Witteles, H. M. Blau, D. Bernstein, R. B. Altman and J. C. Wu, Nat. Med., 2016, 22, 547–556 CrossRef CAS.
- P. Liang, F. Lan, A. S. Lee, T. Gong, V. Sanchez-Freire, Y. Wang, S. Diecke, K. Sallam, J. W. Knowles, P. J. Wang, P. K. Nguyen, D. M. Bers, R. C. Robbins and J. C. Wu, Circulation, 2013, 127, 1677–1691 CrossRef CAS.
|
This journal is © The Royal Society of Chemistry 2021 |
Click here to see how this site uses Cookies. View our privacy policy here.