A computationally efficient model to represent the chemistry, thermodynamics, and microphysics of secondary organic aerosols (simpleSOM): model development and application to α-pinene SOA†
Received
26th February 2021
, Accepted 26th May 2021
First published on 7th June 2021
Abstract
Secondary organic aerosols (SOA) constitute an important fraction of fine-mode atmospheric aerosol mass. Frameworks used to develop SOA parameters from laboratory experiments and subsequently used to simulate SOA formation in atmospheric models make many simplifying assumptions about the processes that lead to SOA formation in the interest of computational efficiency. These assumptions can limit the ability of the model to predict the mass, composition, and properties of SOA accurately. In this work, we developed a computationally efficient, process-level model named simpleSOM to represent the chemistry, thermodynamic properties, and microphysics of SOA. simpleSOM simulates multigenerational gas-phase chemistry, phase-state-influenced kinetic gas/particle partitioning, heterogeneous chemistry, oligomerization reactions, and vapor losses to the walls of Teflon chambers. As a case study, we used simpleSOM to simulate SOA formation from the photooxidation of α-pinene. This was done to demonstrate the ability of the model to develop parameters that can reproduce environmental chamber data, to highlight the chemical and microphysical processes within simpleSOM, and discuss implications for SOA formation in chambers and in the real atmosphere. SOA parameters developed from experiments performed in the chamber at the California Institute of Technology (Caltech) reproduced observations of SOA mass yield, O
:
C, and volatility distribution gathered from other experiments. Sensitivity simulations suggested that multigenerational gas-phase aging contributed to nearly half of all SOA and that in the absence of vapor wall losses, SOA production in the Caltech chamber could be nearly 50% higher. Heterogeneous chemistry did not seem to affect SOA formation over the short timescales for oxidation experienced in the chamber experiments. Simulations performed under atmospherically relevant conditions indicated that the SOA mass yields were sensitive to whether and how oligomerization reactions and the particle phase state were represented in the chamber experiment from which the parameters were developed. simpleSOM provides a comprehensive, process-based framework to consistently model the SOA formation and evolution in box and 3D models.
Environmental significance
Secondary organic aerosols (SOA) constitute an important fraction of atmospheric aerosol mass and affect climate, air quality, and human health. The simplified treatment of SOA in three-dimensional atmospheric models limits the ability of these models to predict the distribution and environmental impacts of SOA accurately. In this work, we develop a computationally efficient framework to simulate the formation, evolution, and properties of SOA with an emphasis on modeling SOA consistently over scales ranging from the laboratory to global models. In this first application, we demonstrate the model's capabilities by simulating SOA formation from the oxidation of α-pinene, an important aerosol precursor.
|
1. Introduction
Secondary organic aerosols (SOA) constitute a ubiquitous and large fraction of atmospheric aerosol mass and play an important role in air quality, climate, and human health.1,2 Atmospheric SOA, formed from the oxidation of volatile organic compounds (VOCs), arise from a multitude of sources and pathways. When compared to other aerosol types such as inorganic aerosols, black carbon, and dust, SOA are much more complex.3 SOA are a mixture of thousands of compounds with physical and chemical properties that vary over logarithmic scales and constantly evolve in the atmosphere.4 This evolving complexity is challenging to represent in atmospheric models and especially burdensome to capture in a computationally efficient manner. Since the atmospheric impacts of SOA are largely dependent on the amount formed and their evolving properties, there is a continued need to find computationally efficient methods to represent the formation, evolution, and properties of SOA in atmospheric models.
There is a significant diversity in modeling approaches to represent SOA in large-scale atmospheric (i.e., 3D) models, which include chemistry-climate models and chemical transport models. A thorough review of this diversity has been undertaken before5 and an updated review is beyond the scope of this work. Briefly, in simpler representations, SOA are either formed immediately upon emission (e.g., for biogenic VOCs) or parameterized to observations of SOA formation in urban plumes (e.g., for anthropogenic VOCs). The SOA so formed are assumed to be non-volatile and non-reactive. An excellent example of this is the ‘simple’ treatment of SOA in GEOS-Chem, a global chemical transport model that considers a limited number of SOA forming VOCs with the SOA formed represented by two model species.6 In more complex representations, the VOC oxidation is assumed to result in the formation of several lumped products that vary in their volatility. The volatility characterized by the effective saturation concentration (c*) informs the partitioning of these lumped products between the gas and particle phases.7 When the products are assumed to be logarithmically spaced in volatility, the representation is referred to as the volatility basis set (VBS).8 Often the gas- and particle-phase products participate in additional chemical reactions that lead to changes in c*. Furthermore, either reversible or irreversible schemes are used to model the aqueous production of SOA in aerosol and cloud water.9 A typical example of this is the treatment of SOA in the Community Multiscale Air Quality Model (CMAQ), a regional chemical transport model that dedicates between 35 and 50 model species to represent SOA, somewhat independent of the gas-phase chemical mechanism used.10,11 What is common to these various representations of SOA in atmospheric models, even in more complex schemes, is that several simplifying assumptions are generally made about SOA processes that are likely to significantly affect model predictions. A few of these assumptions and their potential impacts are discussed below.
First, it is well understood that SOA are a mixture of organic compounds arising from multiple generations of oxidation starting from a VOC precursor.12 This multigenerational aging includes both chemical reactions occurring on (i.e., heterogeneous chemistry13) and inside (e.g. oligomerization reactions14–16) the particle surface. VBS-type parameters derived from environmental chamber data typically assume all SOA to be a ‘first generation’ product, noting that the parameters capture the effects of multigenerational aging within the chamber experiment.8,17 As lower-volatility products from multigenerational aging are produced later in the chamber experiment (except for products of autoxidation reactions18), VBS-type parameters typically underestimate the formation of lower-volatility products.19–21 Furthermore, when implemented in atmospheric models, multigenerational aging is either ignored or represented using generalized schemes that are not constrained to laboratory data. For instance, a commonly used gas-phase aging scheme17 in atmospheric models only considers functionalization reactions despite evidence that fragmentation reactions become increasingly relevant with oxidation.22–24 This scheme has been shown to overestimate ambient SOA mass concentrations.25–27 Heterogeneous chemistry is rarely simulated in chamber and atmospheric models, which is likely because this oxidation pathway is believed to be significantly slower than gas-phase chemistry. Finally, oligomer formation, if accounted for at all, is modeled as a slow irreversible process (lifetime of ∼1 day), in contrast to more recent laboratory evidence that oligomers are formed much more rapidly28 and can dissociate with dilution or heating back into the original monomers.29
Second, most if not all atmospheric models assume instantaneous partitioning of SOA between the gas and particle phases. Theoretical work has argued that the timescales for gas/particle partitioning in the atmosphere are short enough for this assumption to be valid for most particle sizes and locations in the atmosphere.30,31 However, instantaneous partitioning dictated by Raoult's law results in SOA condensation according to the volume distribution when kinetic models have shown that low-volatility SOA condense according to the Fuchs-corrected surface area distribution.31,32 When the timescales for partitioning are short, both instantaneous and kinetic partitioning approaches will result in similar SOA mass condensation but will predict a vastly different evolution of the aerosol size distribution that is controlled by the SOA volatility.33,34 Furthermore, more recent work has shown that the timescales for partitioning can also be controlled by the particle phase state, often characterized using the bulk diffusion coefficient (Db).31,35,36 Depending on the aerosol composition (e.g., aerosol water and oligomers) and environmental conditions (e.g., temperature and relative humidity), the aerosol Db can vary over ten orders of magnitude in the atmosphere (from liquid-like to nearly solid) and consequently produce a ten order-of-magnitude difference in partitioning timescales (from near instantaneous to several years, respectively).37 The aerosol Db might be especially important to consider in chamber experiments since they have been often performed under dry conditions, conducive to producing semisolid SOA.38,39 With emerging evidence that both laboratory and atmospheric SOA might be semisolid under certain circumstances,40 it may not be appropriate to assume instantaneous partitioning for SOA in atmospheric models.
Third, losses of vapors to the walls of the Teflon chamber41–43 have been shown to influence SOA formation in chamber experiments.44–47 When accounted for, they have resulted in significant increases and improvements in SOA predictions in urban atmospheres.48,49 Yet, very rarely are the SOA parameters used in atmospheric models corrected for vapor wall losses. They are corrected for particle wall losses and, occasionally, for the losses of vapors to the particles on the wall.50 Vapor wall loss impacts are likely to vary with the chamber size (e.g., surface area to volume ratio), operation (e.g., actively mixing), and conditions (e.g., OH concentrations and NOX) under which the SOA experiments have been performed. Hence, SOA parameters cannot be simply adjusted to account for the effects of vapor wall losses. What is required is a kinetic treatment of vapor uptake and release from the chamber walls that is now understood to be a function of the vapor volatility.38,42,43
SOA representations in atmospheric models have typically focused on only predicting the total SOA mass concentrations largely because observational datasets were until recently primarily limited to SOA mass concentrations.5 However, many more ambient observations of SOA composition and properties are now available. For instance, aerosol mass spectrometers are regularly deployed across the globe and analyses of these data provide information on source contributions (e.g., urban anthropogenic SOA), elemental composition (e.g., O
:
C), and inferred volatility that could be directly compared against model predictions.51–53 Yet, there are no atmospheric models that we know of that track the dynamic evolution of the mass, O
:
C, and volatility of SOA subject to the processes mentioned above. In summary, there is a need for an explicit treatment within SOA models for multiphase and multigenerational aging processes and phase-state-influenced kinetic gas/particle partitioning and corrections for vapor wall losses encountered in chamber experiments. Additionally, these models need to be applied consistently between the laboratory experiments that are used to determine SOA parameters and the atmospheric simulations that are used to simulation SOA formation and evolution.
In this work, we have developed a computationally efficient model named simpleSOM to represent the chemistry, thermodynamics, and microphysics of SOA. Philosophically, the simpleSOM model is similar to the statistical oxidation model (SOM) originally developed by Cappa and Wilson54 in that it uses a statistical approach to represent the processes relevant to SOA formation and evolution, but is much more efficient. The computational efficiency stems from integration with the VBS framework first developed by Donahue et al.8 In the sections below, we first describe the physical and chemical processes represented in simpleSOM along with the corresponding governing differential equations (Section 2) and computational code (Section 3). Later, we describe the application of the simpleSOM model to simulate SOA formation from the photooxidation of α-pinene (Section 4). Finally, in the Summary and discussion (Section 5), we discuss the feasibility, implications, and limitations of incorporating simpleSOM in a 3D atmospheric model.
2. Model description
2.1 Volatility basis set (VBS) framework
In simpleSOM, all gas- and particle-phase model species except the parent VOC are tracked in logarithmically spaced volatility ‘bins’ of effective saturation concentration, c*. This is similar to the treatment of model species in the VBS framework of Donahue et al.,8 where c* determines the partitioning of the species between the gas and particle phases. This simpleSOM VBS is hereafter referred to as a simpleSOM set. As will be explained later, each simpleSOM set will have its own set of parameters that dictate the oxidation chemistry and thermodynamic properties of the model species in that set. Tracking the model species along a single volatility dimension substantially reduces the number of species that need to be accounted for, relative to SOM (which tracks model species in a 2D carbon- and oxygen-number space), making it well suited for integration in atmospheric models. A lot of atmospheric models already include VBS-type frameworks to model OAs.6,10,55–57 Hence, simpleSOM parameters can be represented within the framework of existing VBS schemes in atmospheric models, with some modifications.
2.2 Gas phase chemistry
All gas-phase model species in simpleSOM, which include VOCs and their oxidation products, are considered as precursor species that are reactive towards the hydroxyl radical (OH). The VOC precursor is referred to as the ‘parent’ species, to distinguish it from other gas-phase reactive products. Each functionalization reaction of the model species with OH is characterized in terms of the probability of adding a certain number of oxygen atoms (POn) and the associated decrease in the c* of the product (fragmentation reactions are described later in this section). It is assumed that a given reaction can add 1 to 4 oxygen atoms which characterizes the addition of various functional groups to the precursor's carbon backbone (e.g., alcohol, carbonyl, acid, ester, ether). Alternatively, the reaction can add a sufficient number of oxygen atoms to produce an ‘extremely low volatility organic compound’ (ELVOC; PELVOC). ELVOCs are included to reflect the formation of multifunctional organic compounds58 and products of autoxidation reactions.18 In theory, all precursors including the parent should be able to form ELVOCs but the pathways for ELVOC formation are not very well understood.59 For simplicity, we assume that only the parent species can directly produce ELVOCs but this could be revised in future versions of the model. For the functionalization reactions, the total probability for the parent and precursor species is thus: | 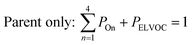 | (1) |
| 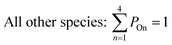 | (2) |
The functionalization reactions are characterized by a decrease in the c* of the product relative to the precursor compound. The average decrease in the volatility of the product due to the addition of one oxygen atom is characterized by the parameter Δlog
c*, and we use the shorthand Δ1c* to indicate a one order of magnitude decrease in volatility, Δ2c* to indicate a two order of magnitude decrease in volatility, and so on. We assume that each oxygen added leads to a decrease of either one or two orders of magnitude in c*, which corresponds approximately to the addition of either a ketone (Δ1c*) or alcohol (Δ2c*); the addition of other functional groups (e.g. hydroperoxides and nitrates) will typically fall between those two limits.60–62 The probability that the addition of n oxygen atoms (PnO) leads to a decrease in the volatility of Δmc* (termed pn,m) is related to the value of Δlog
c* through the relationship:
| 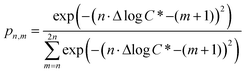 | (3) |
where
n is the number of oxygen atoms added and
m ranges from
n to 2
n.
Eqn (3) weights the decrease in volatility for the product distribution according to the average decrease in volatility (
i.e., Δlog
c*). For example, there are two probabilities associated with the addition of one oxygen atom,
p1,1 that corresponds to the product fraction that is one order of magnitude lower than the precursor and
p1,2 that corresponds to the product fraction that is two orders of magnitude lower than the precursor. Similarly, there are three probabilities associated with the addition of two oxygen atoms,
p2,2 that corresponds to the product fraction that is two orders of magnitude lower than the precursor,
p2,3 that corresponds to the product fraction that is three orders of magnitude lower than the precursor, and
p2,4 that corresponds to the product fraction that is four orders of magnitude lower than the precursor. As four oxygen atoms can be added in a single reaction step, the functionalization reactions can produce a maximum, one-step, decrease in
c* of up to a factor of eight (
i.e., Δ8
c*). By definition, the parent is not allowed to participate in fragmentation reactions.
The functionalization reactions, described above, are dealt with much more simply for VOCs that react with two other oxidants relevant to the atmosphere, O3 and NO3. The VOC reaction with the oxidant can lead to the formation of functionalized oxidation products and ELVOCs but the oxidation products are only allowed to further react with OH and not with O3 and NO3. Although simpleSOM can accommodate reactions with all 3 oxidants, we only focus on reactions with OH in this work.
Reaction with OH also leads to the fragmentation of the precursor into higher volatility species24 and this is characterized using the probability of fragmentation (Pfrag). Since the likelihood of fragmentation increases as a molecule becomes more functionalized (i.e. has more oxygen atoms),23 and since it is oxygen addition (functionalization) that leads to decreases in c*, the Pfrag values are parameterized as:
|  | (4) |
where

is the maximum value of
c* considered and
mfrag is an adjustable parameter. Here, we assume

to be equal to the
c* value of the parent VOC (rounded up or down to the nearest decade) but it could also be set to a fixed value (
e.g.,

of 10
7 μg m
−3). It follows that the probability of functionalization
Pfunc is equal to 1 −
Pfrag. If fragmentation occurs, we assume that some of the resulting products are volatile enough that they are unlikely to ever form low volatility products after subsequent reactions and the remaining products increase only marginally in volatility such that subsequent reactions may convert them to lower volatility products. With these assumptions, the fragmentation reactions are dealt with as follows. We introduce a parameter,
Ploss, that describes the probability that fragmentation leads to permanent loss of potentially condensable mass and the remainder (1 −
Ploss) has a volatility that is one or two orders of magnitude larger than that of the precursor compound,
i.e. +Δ1
c* or +Δ2
c*. We assume, that of the mass that is not irreversibly lost, fragmentation leads equally to products with +Δ1
c* and +Δ2
c*. All fragmented products are assumed to gain one oxygen atom over the precursor.
Taken together, the gas-phase oxidation chemistry in simpleSOM is controlled by the following eight adjustable parameters: (i–iv) POn, the probability of adding n oxygen atoms to the precursor where n varies from 1 to 4, (v) PELVOC, the probability of forming ELVOCs, (vi) Δlog
c*, the average decrease in volatility in logarithmic space per oxygen added, (vii) mfrag, the parameter that determines the probability of fragmentation, Pfrag, and (viii) Ploss, the probability that fragmentation will lead to permanent loss of precursor mass. Since pn,m is calculated from Δlog
c* as per eqn (3), pn,m is not a directly adjustable parameter. The values for these parameters, including pn,m, can be either specified (when that information is available a priori, e.g., PELVOC in this work) or fit by comparing model predictions to observations. The differential equations, considering the functionalization and fragmentation schemes described above, for a generic precursor or model species in a simpleSOM set (i.e., [Δ0c*]) and its oxidation products are given as (5) and (6), respectively.
| 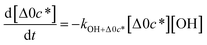 | (5) |
|  | (6a) |
|  | (6b) |
|  | (6c) |
|  | (6d) |
|  | (6e) |
|  | (6f) |
|  | (6g) |
|  | (6h) |
|  | (6i) |
|  | (6j) |
|  | (6k) |
|  | (6l) |
where
kOH+Δ0c* is the rate coefficient for the reaction of the precursor species (
i.e. the Δ0
c* species) with OH in cm
3 per molecule per s and [OH] is the OH concentration in molecules per cm
3. The
kOH for the parent species is based on literature values while, for all other species, the
kOH values (in cm
3 per molecule per s) are calculated using
eqn (7), which is a reduced version of the parameterization used in SOM.
63 |  | (7) |
where
a1 = 1.56 × 10
−13,
a2 = −5.62 × 10
−13,
b1 = −7.12 × 10
−13,
b2 = −5.69 × 10
−13,
c1 = −8.22 × 10
−12, and
c2 = 6.63 × 10
−11.
The overall differential equations for the gas-phase parent and precursor model species in simpleSOM are as follows:
| 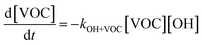 | (8a) |
|  | (8b) |
where [VOC] and [
Cgi] are the gas-phase concentrations of the parent and model species
i in μg m
−3, respectively. Because a simple SOM set is configured for a fixed number of
c* model species or volatility bins, the reaction mechanisms and differential equations are adjusted to account for the production of species that are smaller or larger in volatility than the pre-defined
c* range. For instance, for a simpleSOM set of 14 bins that ranges from a log
c* of −6 to 7, any reactions leading to species with a log
c* smaller than −6 are placed in the lowest log
c* bin of −6 and any reactions leading to species with a log
c* larger than 7 are placed in the largest log
c* bin of 7. ELVOCs, by default, are placed in the lowest log
c* bin. In this work, we set the lowest log
c* bin to −6 and the highest log
c* bin is set to the log
c* of the parent rounded up or down to the nearest integer. This example simpleSOM set of 14 bins was used in this work to model SOA formation from the photooxidation of α-pinene.
In addition to tracking the gas-phase concentrations of the oxidation products, we also track the oxygen concentrations in each c* bin by precisely knowing the oxygen atoms added to the precursor through both functionalization and fragmentation reactions. These concentrations are used offline to determine the c*-resolved and bulk O
:
C of the SOA assuming that most of the particle-phase products retain the carbon backbone of the parent.64 This assumption should produce a lower bound estimate for the SOA O
:
C since some of the oxidation products in the particle phase are likely to be composed of species that have a smaller carbon number than the precursor. In the future, we will consider tracking the carbon number of the c* bin in addition to tracking the oxygen number to perform a more precise calculation of the aerosol O
:
C ratio.
2.3 Kinetic gas–particle partitioning and particle phase state
The kinetic gas/particle partitioning of a model species influenced by the particle phase state is modeled in simpleSOM using the approach outlined in Zaveri et al.65 The differential equations used to model the evaporation and condensation of the simpleSOM species i, for a polydisperse size distribution, are as follows: |  | (9) |
|  | (10) |
where [Cpi,j] is the particle-phase concentration of the species i in size bin j in μg m−3, dj is the diameter of the particle in size bin j in m, Nj is the particle number concentration in size bin j in m−3, Ki,j is the overall gas-side mass transfer coefficient for species i in size bin j in m s−1, [OAj] is the total OA mass concentration in size bin j in μg m−3,
is the effective saturation concentration of species i in μg m−3, and Sj is the Kelvin ratio.66Ki,j is calculated as follows: | 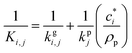 | (11) |
|  | (12) |
|  | (13) |
where kgi,j is the gas-side mass transfer coefficient in m s−1, kpj is the particle-side mass transfer coefficient in m s−1, ρp is the SOA density in μg m−3, Dgi is the gas-phase diffusion coefficient of the model species in m2 s−1,67 FSj is the Fuchs–Sutugin correction factor,68Db is the particle-phase diffusion coefficient of the model species in m2 s−1, and lj is the length scale over which diffusion in the particle phase takes place. In this study, a ρp of 1.18 g cm−3 was used based on the estimates produced by Bahreini et al. (2005)69 for α-pinene SOA and a mass accommodation coefficient of unity was used when calculating FSj based on recent work by Krechmer et al. (2017)70 and Liu et al. (2019)71. The same Db was used for all model species. At the two extremes, lj equals dj/2 for a homogeneously mixed particle and approaches the coating thickness for a core–shell morphology that assumes an SOA coating on an inert solid seed. For a liquid-like aerosol when Db is greater than 10−10 m2 s−1, Ki,j approaches kgi,j and eqn (9) and (10) resemble the condensation/evaporation equation expressed in the continuum regime.68Eqn (9) to (13) are valid only when the model species do not participate in additional particle-phase reactions (i.e., heterogeneous chemistry and oligomerization). When particle-phase reactions are considered, the equations take on a slightly modified form depending on the effective reaction rate and these equations have been covered in our previous work.76
2.4 Heterogeneous chemistry
All particle-phase model species in simpleSOM are assumed to participate in heterogeneous oxidation reactions with the OH radical. This is modeled as a surface reaction wherein a certain fraction of the OH collisions with the particle surface, given by the uptake coefficient, is assumed to lead to a reaction.13 The differential equation that determines the loss of the model species due to these heterogeneous or surface reactions is given as: |  | (14) |
where γOH is the uptake coefficient,
is the root mean square speed of the OH molecules in m s−1, dj is the diameter of the particle in size bin j in m, Nj is the number concentration of the particles in size bin j in m−3, and FOHFS,j is the Fuchs–Sutugin correction for OH for size bin j. For simplicity, we assume that the heterogeneous oxidation reaction leads to the formation of model species one order of magnitude lower in volatility (i.e., functionalization) and one order of magnitude higher in volatility (i.e., fragmentation) than the precursor species. The probability of functionalization and fragmentation is calculated as per eqn (4). The uptake coefficient, γOH, is an adjustable parameter but is assumed to be equal to 1 in this work. This value is within the range of uptake coefficients determined in previous experimental work performed on OA model systems (0.1 to 6).72–74
2.5 Oligomerization reactions
All monomeric particle-phase species in simpleSOM (Mpi,j) are allowed to participate in reversible oligomerization reactions that include forward or formation reactions and reverse or dissociation reactions. Our approach to representing oligomerization in simpleSOM is borrowed from that described in Trump and Donahue75 and He et al.76 For simplicity, we only model dimer formation to represent general oligomer formation, where we allow all combinations of monomers to form those dimers but ignore higher-order oligomers formed from the reactions of dimers with monomers or other dimers and so on. Instead of tracking all potential dimer pairs formed from all combinations of monomers, we model dimer formation by keeping track of the monomers that have undergone a reaction to form a dimer; we call them ‘dimerized monomers’. All dimerized monomers are assumed to be non-volatile. These dimerized monomers from each c* bin are tracked separately, which doubles the number of particle-phase species that need to be tracked for a given simpleSOM set. Dimer dissociation is modeled by simply returning the dimerized monomer back to its original monomer form. The dimer formation rates are characterized by the forward reaction rate, kf in cm3 molecules per s, and the reverse reaction rate, kr in s−1. For simplicity and in the absence of any specific experimental data, the same kf and kr are assumed to be valid for all monomers and dimers and treated as adjustable parameters in simpleSOM. The oligomerization reactions are modeled in simpleSOM as follows: |  | (15) |
|  | (16) |
where [Mpi,j] and [Oi,j] are the monomer and dimerized monomer concentrations for species i and size bin j in the particle phase in molecules per cm3 of particle volume.
2.6 Vapor wall losses
The losses of vapors to the Teflon walls have been shown to influence SOA formation in chamber experiments41,42,45,77 and need to be explicitly accounted for when developing SOA parameters from chamber data. The losses of simpleSOM species to the Teflon walls are modeled following the methods described in Zhang et al.45 and Krechmer et al.42 The first-order uptake of vapors by the walls is assumed to be equal to kw,on and the release of vapors from the walls, kw,off, is modeled using absorptive partitioning theory with the Teflon wall serving as an absorbing mass with an effective mass concentration of Cwall mg m−3. kw,on for a model species is calculated using the following equation that assumes that the mass accommodation coefficient of the vapor with the wall is larger than 10−6 (above which, this accommodation coefficient has little effect on the overall mass transfer):78 | 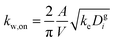 | (17) |
where A/V is the surface area to volume ratio for the Teflon chamber in m−1 and ke is the coefficient of eddy diffusion in s−1. kw,off is calculated using the following equation:41 | 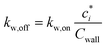 | (18) |
As the ke is not known a priori, we use an empirically determined kw,on. Zhang et al.45 determined a kw,on of 2.5 × 10−4 s−1 for the environmental chamber at the California Institute of Technology (Caltech) based on SOA experiments performed on toluene, but this was later revised by Huang et al.46 to a value of 4 × 10−4 s−1 (the value used in this work). Based on the observations of Krechmer et al.,42Cwall is varied with the c* of the model species, with higher values used for more volatile species (e.g., Cwall = 10 mg m−3 for c* > 104 μg m−3) and vice versa (e.g., Cwall = 0.016 mg m−3 for c* < 1 μg m−3). Here, as in our previous work, we only consider absorptive reversible losses of vapors to the chamber wall and assume that other potential modes of vapor loss (e.g., heterogeneous chemistry on the wall surface, uptake by water adsorbed on the wall) are unimportant. Vapor wall losses are turned on when performing the chamber simulations and turned off when performing the atmospheric simulations (explained later).
2.7 Computational methods
The simpleSOM model is coded in IGOR (Wavemetrics Inc., OR) and the latest version is available at the permanent archival links shared later (Section 6). All differential equations are solved using the semi-implicit Euler method previously described in Jacobson79 and Zaveri et al.80 Within the code, the processes described in Sections 2.2–2.6 are solved sequentially in the following order: gas-phase chemistry, vapor wall losses, kinetic gas/particle partitioning, heterogeneous chemistry, and oligomerization reactions. A time step of 60 seconds was used for all simulations performed in this work. On an ordinary desktop computer (circa 2017), chamber simulations were computed in <5 s and atmospheric simulations were computed in <30 s for a single VOC precursor (i.e., α-pinene). This translates to a computation time of <0.4 s per wall hour of simulation. Fitting to chamber data (explained later) took slightly longer, e.g., ∼15 minutes, as this required on the order of 100 iterative chamber simulations. Fitting was performed using a local optimization scheme, the Levenberg–Marquardt algorithm, available in IGOR. Because we use a local optimization scheme, we tested the robustness of our fitting results by trying several initial guesses of the parameter sets for fitting. For all fitting performed, the optimal fits were not sensitive across a sensible range of initial guesses, showing that our optimal parameter estimates were robust.
We simulate the SOA formation from a single VOC precursor, α-pinene, in this work. These simulations used a simpleSOM set of 14 c* bins that spanned a log
c* range from −6 to 7 and 30 size bins that initially ranged from 10 to 714 nm. These size and volatility dimensions required 868 model species to track the gas- and particle-phase species arising from the oxidation of a single VOC. This number includes tracking the dimerized monomers and the oxygen atoms for each c* bin. Although this number can be easily accommodated in box model applications, these are too many to include for a single precursor in most 3D models since multiple VOCs (or VOC classes) are generally simulated. Hence, the simpleSOM model will need to be optimized when incorporated within a 3D model, and this is discussed in Section 5.
3. Experimental data and simulations
In this work, we used the simpleSOM model to simulate the SOA formation from α-pinene photooxidation. We do this to demonstrate the ability of the model to develop parameters that can reproduce environmental chamber data, to highlight the chemical and microphysical processes within simpleSOM, and discuss the implications for SOA formation in chambers and in the real atmosphere. To develop SOA parameters, we used the environmental chamber data described in Chhabra et al.,81 where they formed SOA from the photooxidation of α-pinene in separate low and high NOX experiments. We chose to examine α-pinene SOA and this set of specific chamber experiments for several reasons. First, α-pinene is a widely studied biogenic VOC81–84 that, as part of monoterpene emissions, is an important SOA precursor on regional and global scales.6,85–87 The wealth of historical chamber data for α-pinene SOA allowed us to compare simpleSOM predictions to a subset of prior observations. Second, experiments performed in the environmental chamber at Caltech have been characterized for the losses of vapors to the chamber walls45,46 and corrected for particle wall losses.45,88 Wall losses are experimental artifacts that need to be corrected for or explicitly modeled since they are likely to significantly influence SOA parameters determined from chamber data.48,49 Third, the α-pinene SOA data from Chhabra et al.81 have been previously used to develop SOA parameters for monoterpenes for use in box89 and 3D25,48,49 models. In subsequent publications, we will aim to develop parameters for other important SOA precursors.
The α-pinene photooxidation experiments have been described in detail in Chhabra et al.,81 but we provide a brief description below for completeness. Ammonium sulfate seeds added to a clean 30 m3 Teflon chamber provided a surface area for the condensation of SOA. OH radicals were produced through the photolysis of hydrogen peroxide (H2O2) in the case of the low NOX experiment and of methyl nitrite (CH3ONO) in the case of the high NOX experiment. The initial NO and NO2 concentrations were below the instrument's limit of detection (<2 ppbv) during the low NOX experiment and were 447 and 400 ppbv, respectively, during the high NOX experiment. Photooxidation was initiated by turning the chamber's UV lights on, which produced an NO2 photolysis rate of ∼1.5 min−1. α-Pinene concentrations were measured using gas chromatography while aerosol concentrations were measured using a scanning mobility particle sizer (SMPS) and a high resolution-aerosol mass spectrometer (HR-AMS). The decay in the VOC concentrations was used to determine OH concentrations. The SMPS and HR-AMS data were used, along with the knowledge of the particle wall loss rates (calculated separately), to estimate the particle wall-loss-corrected SOA mass concentrations. The HR-AMS data were also used to determine the elemental ratios of H
:
C and O
:
C.
The simpleSOM model was used along with the experimental data of Chhabra et al.81 to perform the following set of chamber and atmospheric simulations:
1. A base version of the simpleSOM model that included gas-phase oxidation chemistry and kinetic gas/particle partitioning was fit to chamber data to determine parameters that could reproduce the observations of SOA mass concentration and O
:
C. The fitting, here and elsewhere, was performed using an objective function that weighed the SOA mass concentration and SOA O
:
C equally. Fitting was performed separately for low and high NOX conditions. In these simulations, we assumed a liquid aerosol (Db = 10−6 cm2 s−1). After fitting, we performed sensitivity simulations with multigenerational aging, vapor wall losses, or heterogeneous chemistry turned off. The results from these simulations are shown in Fig. 1 and 2.
2. With oligomerization reactions added to the base version. The forward (kf) and reverse (kr) reaction rates for the oligomers, in addition to the simpleSOM parameters, were optimized to achieve different oligomer fractions (folig,c) at the end of the chamber simulation, while still reproducing the observations of SOA mass concentration and O
:
C. Fitting was performed only for the low NOX conditions to assess the sensitivity of the model results to the inclusion of oligomerization. The parameters were then used to simulate SOA formation and evolution in a simplified atmospheric simulation, following the methods described in Hodzic et al.90 and He et al.91 Here, we injected a trace amount of α-pinene (1 pptv) into a box with a constant OH concentration of 1.5 × 106 molecules per cm3 and an OA mass concentration of 10 μg m−3 and modeled the formation and evolution of SOA over 72 hours. The results from these simulations are shown in Fig. 3.
3. With phase state-influenced kinetic/gas particle partitioning added to the base version. Different Db,chamber values were assumed for the α-pinene SOA in the chamber simulation that spanned from a liquid (10−6 cm2 s−1) to nearly a solid (10−17 cm2 s−1). Separate SOA parameters were determined for these different Db,chamber values that reproduced the observations of SOA mass concentration and O
:
C. Fitting was performed only for the low NOX conditions to assess the sensitivity of the model results to mass transfer limitations. The parameters were then used to simulate the SOA formation and evolution in atmospheric simulations, similar to that described in #2, for different Db,atmos values for the atmospheric OA. The results from these simulations are shown in Fig. 4.
4. Results
4.1 Fitting to chamber data and effects of multigenerational aging and vapor wall losses
Results from the application of simpleSOM to the α-pinene SOA data from Chhabra et al.81 are shown in Fig. 1. These results are presented to provide a general overview of how simpleSOM parameters are developed for a given SOA precursor and what the parameter values potentially convey. The simpleSOM parameters (PO1–PO4, Δlog
c*, mfrag, and Ploss) were determined by simultaneously fitting to the observations of the time-dependent SOA mass concentration and O
:
C ratio. PELVOC was assumed to be 3.4% for the low NOX case based on the estimate for HOM production from the photooxidation of α-pinene.59,92 No ELVOCs were produced in the high NOX case (PELVOC = 0). Low- and high-NOX experiments were fit separately, and the results for these two different NOX cases are shown in panels (a) and (b), respectively. At both NOX levels, the simpleSOM model predictions were able to closely follow the observations of SOA mass concentration and O
:
C and were well within the uncertainty ranges for both measurements. These comparisons, along with the results shown later, attest to the ability of simpleSOM to represent the oxidation chemistry and gas/particle partitioning of SOA. Varying the initial guesses for the simpleSOM parameters across a reasonable range of values did not have a large influence on the optimal parameter set, and hence, searching for additional acceptable local minima was not explored further.
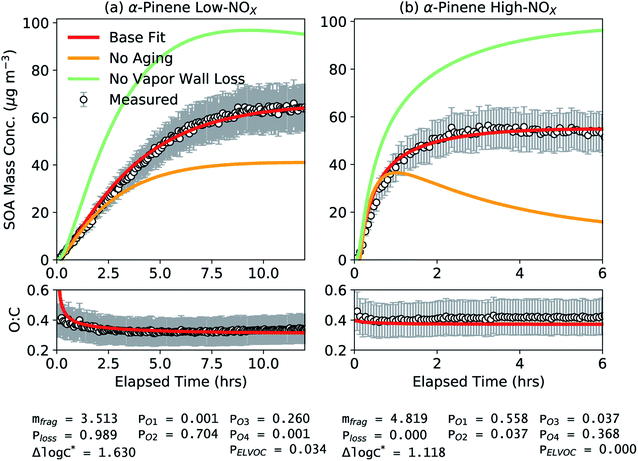 |
| Fig. 1 SimpleSOM predictions of SOA mass concentration and SOA O : C ratio compared to measurements for (a) low and (b) high NOX photooxidation experiments performed on α-pinene.81 Model predictions based on fits to the SOA mass concentration and O : C are shown in solid red while those for simulations using the base fit parameters but with no multigenerational aging or no vapor wall losses are shown in solid mint and orange, respectively. The fit parameters for the respective NOX conditions are listed at the bottom of the figure. | |
While simpleSOM does not perfectly represent the mechanisms of VOC oxidation and SOA formation, the associated parameters derive from physical and chemical processes. As such, there is some utility in interrogating the optimized parameter values in terms of what they indicate about the chemical evolution of the system. The first-generation, functionalized oxidation products appeared to be dominated by species with 2 and 3 additions of oxygen to the precursor for the low NOX case and dominated by species with 1 and 4 additions of oxygen to the precursor for the high NOX case. Being a statistical model, the differences in the oxygen additions to the precursor are not directly interpretable. For the fit Δlog
c* (1.630 and 1.118, respectively), this resulted in a one- to five-fold decrease in c* for these oxidation products compared to the precursor (c* of ∼107.4 μg m−3) but these were still squarely within the semivolatile (c* = 1–103 μg m−3) and intermediate-volatility (c* = 103–106 μg m−3) ranges.93 However, a significant fraction of the total SOA was composed of a much lower volatility material (c* < 1 μg m−3) (61 and 83% for the low and high NOX simulations, respectively) than that suggested by the first-generation oxidation products; c*-resolved contributions to SOA are shown in Fig. S1.† This implied that the lower-volatility oxidation products, separate from HOMs, were principally formed through multigenerational aging. Simulations performed without multigenerational aging, where the first-generation oxidation products did not react any further, reproduced only about half of the observed, end-of-experiment SOA mass (Fig. 1). In the high-NOX simulations without multigenerational aging, as the first-generation oxidation products in the gas-phase were not allowed to react, their loss to the walls drove the evaporation of the SOA that had condensed up to that point. These results provide evidence that multigenerational aging needs to be explicitly modeled in chamber and atmospheric simulations to better represent the formation and properties of low-volatility SOA. The mfrag term was modestly large (3.513 and 4.819) wherein the probability of fragmentation for reactions of the first-generation oxidation products with OH was between 53 and 95%. The Ploss term was close to unity for the low NOX experiment (0.989) indicating that nearly all of the oxidation products from fragmentation reactions were too volatile to be tracked in the simpleSOM set. The Ploss term was zero for the high NOX experiment, although values greater than zero cannot be ruled out given the uncertainty in the fitting process and the potential for non-unique solutions.
When vapor wall losses were turned off, after the original fitting, simpleSOM predicted a 51% and 76% increase in SOA formation in the low and high NOX experiments, respectively. These findings are largely consistent with estimates in Zhang et al.45 who found a 60% and 30% increase in SOA production with no vapor wall losses under low and high NOX conditions, respectively, for the same experimental data. The consistent results increase our confidence in the simpleSOM framework that it could be used to develop SOA parameters corrected for vapor wall losses for use in atmospheric models.
We modeled the effect of heterogeneous chemistry on the simulations performed as shown in Fig. 1 although even with an uptake coefficient of 1 this had little to no effect on the SOA evolution (see Fig. S2†). The end-of-experiment SOA mass concentration decreased by 1–5% and the SOA O
:
C increased by ∼1%. Hence, for all results shown in this work, we turned heterogeneous chemistry off. Heterogeneous oxidation reactions are likely to be important when modeling the SOA formation and evolution in oxidation flow reactors where the oxidant concentrations are much higher than those in chamber experiments123 and could be important in atmospheric models depending on the timescales simulated. This process will be examined in more detail in future applications of the simpleSOM model.
4.2 Comparisons with historical data for mass yields, O
:
C, and volatility distribution
We assessed the generalizability of our parameters by comparing the simpleSOM model predictions to historical experimental data. The comparison is qualitative by design and not meant to be a substitute for a more systematic evaluation where one would need to account for differences in initial concentrations (e.g., VOC, NOX, and oxidant), chamber characteristics (e.g., vapor and particle loss rates, and photolysis rates), and environmental conditions (e.g., temperature and relative humidity). In Fig. 2(a and b), we compare the model predictions of the SOA mass yields from simpleSOM to historical data gathered from photooxidation and ozonolysis experiments performed on α-pinene.84,96–98 The end-of-experiment observational data were recently summarized in Afreh et al.94 The model predictions were from simpleSOM simulations performed at two initial VOC concentrations (40 and 160 ppbv) and across 24 hours of photochemical aging at a constant OH concentration of 1.5 × 106 molecules per cm3. All other model inputs were the same as those used in Fig. 1 (e.g., initial seed surface area, vapor wall loss rate, and temperature). The initial VOC concentrations and OH exposure were chosen such that the predictions could be compared to the observations over a similar range of SOA mass concentrations (1–400 μg m−3).
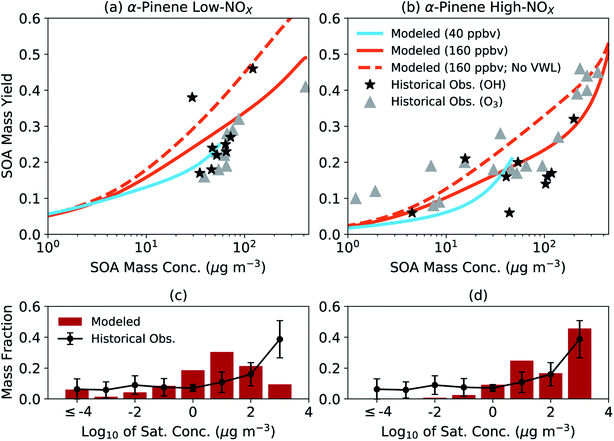 |
| Fig. 2 SimpleSOM predictions of (a and b) SOA mass yields and (c and d) volatility distributions compared with historical data. Predictions in panels (a) and (b) are based on simulations with an initial VOC concentration of 40 and 160 ppbv with vapor wall losses modeled and an initial VOC of 160 ppbv without vapor wall losses modeled (no VWL). Predictions in panels (c) and (d) are normalized, end-of-experiment gas + particle volatility distributions based on simulations with an initial VOC concentration of 160 ppbv with vapor wall losses modeled. Historical data for SOA mass yields have been tabulated in the ESI† in Afreh et al.94 and those for volatility distributions can be found in Morino et al.95 | |
The simpleSOM predictions of SOA mass yields were found to be generally consistent with the historical photooxidation and ozonolysis data with a few exceptions. Under low NOX conditions, predictions from simulations performed with an initial VOC concentration of 40 ppbv seemed to reproduce the cluster of observed SOA mass yields between 0.15 and 0.31 but predictions from an initial VOC concentration of 160 ppbv slightly overestimated the main cluster of observed points. Differences in the model predictions from the two different simulations indicated the sensitivity of the SOA formation to the initial VOC concentration, which could be attributed to differences in multigenerational aging and different SOA production rates relative to the condensation-sink and vapor-wall-loss timescales. The cluster of observed SOA mass yields between 0.15 and 0.31 did not seem to point to any differences between the photooxidation and ozonolysis data for the low NOX conditions. In contrast, under high NOX conditions, predictions from simulations performed with either initial VOC concentration were more consistent with the photooxidation data but slightly lower compared to the ozonolysis data. Differences in the observed SOA mass yields could likely be a result of lower HOM yields measured in photooxidation experiments (1.2%) compared to those in ozonolysis experiments (3.4%).59 Simulations performed without vapor wall losses increased the predictions of the SOA mass yields. At both NOX levels for the photooxidation simulations, the average predicted SOA mass yield was higher than the average of the observed SOA mass yield (a factor of 1.4 for low NOX and 1.5 for high NOX) although this increase was much more variable for the high NOX conditions. Model predictions of SOA O
:
C at both initial VOC concentrations and NOX levels (0.29–0.37) seemed to agree with historical observations for α-pinene SOA (0.27–0.55)19,38,99–104; a compilation of historical O
:
C observations in chamber experiments can be found in Table S1.†
In Fig. 2(c, d), we compare the model predictions of the SOA volatility distribution from the end of the experiment to the average volatility distribution measured in earlier work. The average measured volatility distribution was based on the measurements of the chemical composition of SOA and the response of SOA to heating.12,20,105,106 We should note that these volatility distribution data, which were recently summarized in Morino et al.,95 were mostly gathered from experiments performed with OH and O3 as oxidants under low NOX conditions. These volatility distribution data were averaged because there was significant uncertainty (∼1 order of magnitude) in the individual estimates and significant variability across studies. Hence, the comparison presented here needs to be interpreted with caution. The simpleSOM model predictions of the volatility distribution under low NOX conditions were qualitatively similar to the measurements in the lower-volatility bins (c* < 1 μg m−3), in that the predictions reproduced the distribution of oxidation products over a broad range of c* values. The comparison was much less favorable over the higher-volatility bins (c* ≥ 1 μg m−3). The trends in the model-measurement comparison seemed to flip for the high NOX conditions. These comparisons likely indicate the differences expected in the SOA volatility distribution under different NOX conditions and the ability of the model to capture those differences. With new approaches to measure and quantify volatility (e.g., Chhabra et al.105), SOA parameters could be better constrained and evaluated in the future by comparing model predictions to observations of the volatility distribution.
Although the comparisons in Fig. 2 suggest that the simpleSOM parameters developed from the work of Chhabra et al.81 can generally reproduce the historical data, they emphasize the need to test the generalizability of SOA parameters developed from one set of chamber experiment(s) to similar experimental data in the literature before these parameters are used in atmospheric models.
4.3 Oligomerization reactions
Monoterpene oxidation leading to SOA formation has been shown to produce high molecular-weight, low volatility oligomers in the particle phase14–16,107 and yet the chemistry and abundance of oligomers have remained largely uncertain for most VOCs including monoterpenes. We performed a case study to understand the impact of accounting for oligomerization reactions in chamber experiments on SOA mass yields and properties in the real atmosphere. The results from this case study are shown in Fig. 3 for a representative low NOX experiment where the chamber data are the same as those shown earlier in Fig. 1 and 2.81 Results for the high NOX case were generally similar and are shown in Fig. S3.† simpleSOM parameters were determined for four different target oligomer fractions (folig,c) to be achieved by the end of the chamber experiment: 0, 20, 50, and 80%. Apart from the 0% target, the other target oligomer fractions were chosen to span the oligomer fractions observed in previous experiments involving α-pinene SOA.28,108,109 The target oligomer fractions were achieved by fixing the dimer formation rate constant (kf of 10−24 cm3 molecules per s) and adjusting the dimer dissociation rate constant (kr, s−1). The kf value was chosen based on the median value described for oligomer formation in Ziemann and Atkinson110 and Roldin et al.111 The kr values for the four target oligomer fractions were found to be 0, 0.0150, 0.0024, and 0.0003 s−1, respectively, and seemed to be generally consistent with observed ranges in the literature.75,110,111 The simpleSOM parameters are listed in Table S2.†
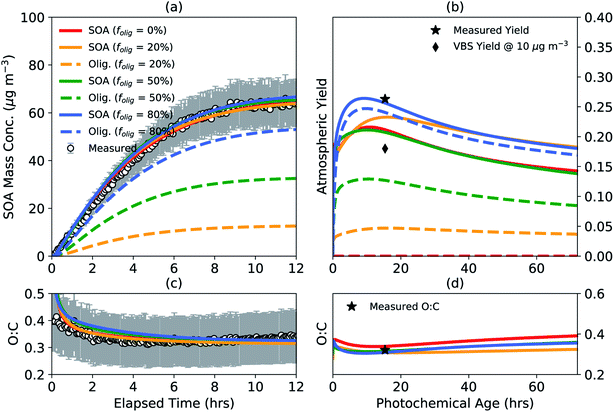 |
| Fig. 3 SimpleSOM predictions of the (a) SOA mass concentration and (c) SOA O : C ratio based on fits to the observations compared to measurements for a low NOX photooxidation experiment performed on α-pinene81 for different target end-of-experiment oligomer fractions. simpleSOM predictions of (b) SOA mass yields and (d) the SOA O : C ratio from atmospheric simulations performed under low NOX conditions. Predictions of total SOA mass are shown in solid lines and the oligomer mass are shown in dashed lines. Symbols in panel (b) show the measured, end-of-experiment SOA mass yield and the estimated VBS SOA mass yield at an OA mass concentration of 10 μg m−3. Symbol in panel (d) shows the measured, end-of-experiment SOA O : C. | |
As shown in Fig. 3(a, c), model predictions based on the fit parameters matched the observations of SOA mass concentration and O
:
C. Since oligomerization reactions do not affect the particle-phase O
:
C ratio, the model predictions of SOA O
:
C were insensitive to folig,c. This fitting exercise suggests that it is unlikely that we will be able to constrain oligomer formation by only using the observations of SOA mass concentration and O
:
C. The use of parameters with different folig,c resulted in slightly different SOA mass yields in the atmospheric simulations, which are presented in Fig. 3(b, d). Generally, a lower target folig,c for the chamber resulted in lower atmospheric SOA mass yields. For example, the peak SOA mass yield with no oligomer formation (i.e., folig,c = 0) was approximately 30% lower than the peak SOA mass yield for parameters with an folig,c of 80%. This was presumably because the atmospheric simulations were performed at a constant OA mass concentration of 10 μg m−3, which was lower than the average OA mass concentration in the chamber experiment (∼30 μg m−3). A lower OA mass concentration in the atmospheric simulation resulted in a smaller proportion of semivolatile monomers condensing to the particle phase, which slowed oligomer production and additional uptake of semivolatile monomers and eventually depressed the SOA mass yields. Regardless, since all SOA parameters were constrained to the same chamber observations, the use of a lower folig,c resulted in parameters that produced increasing quantities of lower-volatility oxidation products to compensate for reduced oligomer formation. This is probably the primary reason for why model predictions of the atmospheric SOA mass yields were much closer than anticipated for all assumed values of folig,c. The model predictions of SOA O
:
C in the atmospheric simulations did not trend with folig,c as the SOA mass yields did.
Sensitivity simulations performed with a slower (10−25 cm3 molecules per s) and faster (10−23 cm3 molecules per s) kf,110,111 but still constrained to match the chamber observations of SOA mass concentration and O
:
C, produced similar trends in the atmospheric simulations as shown in Fig. 3(b, d); the results of these sensitivity simulations are presented in Fig. S4 and S5,† respectively. Overall, these simulations suggest that the predictions of the atmospheric SOA mass yields are not very sensitive to whether oligomerization reactions are explicitly accounted for when the SOA parameters are developed and that accounting for oligomerization reactions might marginally boost the atmospheric SOA mass yields. Another point to consider is that most OA modules in atmospheric models simulate oligomer formation from the SOA monomers generated from parameters developed assuming an folig,c of 0% during the underlying experiments. These schemes should produce more SOA than that shown in Fig. 3(b) for folig,c = 0% and could overestimate SOA formation in atmospheric models.
The model predictions of the atmospheric SOA mass yields for all folig,c were lower than the SOA mass yield measured at the end of the chamber experiment presumably because the average OA mass concentration in the chamber experiment (∼30 μg m−3) was larger than that used in the atmospheric simulation (10 μg m−3). In contrast, however, the model predictions of the atmospheric SOA mass yields for all folig,c were larger than the SOA mass yield predicted using a VBS fit to the chamber data potentially from the absence of processes in the VBS model to simulate the production of lower-volatility SOA via multigenerational aging and particle-phase reactions.
4.4 Particle phase state
The phase state of SOA might be important to account for when modeling laboratory-based SOA experiments since these experiments are typically performed under low relative humidities (<20%) that may encourage the production of semisolid/viscous aerosols. Building on our recent work,76 we performed a case study to understand the impact of assuming the phase state in chamber experiments on SOA mass yields in the real atmosphere. The results from this case study are shown in Fig. 4 for the same low NOX experiment shown in earlier figures. The simpleSOM parameters were determined for three assumed bulk diffusion coefficients (Db,chamber) for the chamber SOA: 10−6, 3 × 10−15, and 10−17 cm2 s−1. The largest Db,chamber value (10−6 cm2 s−1) was chosen to represent a liquid aerosol that reflects the current approach to modeling the gas/particle partitioning of SOA with no limitations to mass transfer. The intermediate Db,chamber value (3 × 10−15 cm2 s−1) was chosen based on our recent work where we showed that the observations of SOA mass concentration and the aerosol size distribution in an α-pinene chamber experiment could only be reproduced for a narrow range of Db values around the chosen value.76 And finally, the smallest Db,chamber value (10−17 cm2 s−1) was chosen to represent an even more viscous SOA that is consistent with the direct measurements of viscosity for α-pinene SOA.38,39
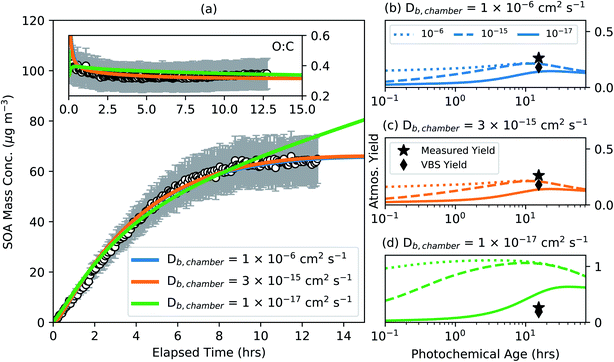 |
| Fig. 4 (a) simpleSOM predictions of SOA mass concentration and O : C based on fits to the observations compared to measurements in a low NOX photooxidation experiment performed on α-pinene81 for different assumed Db,chamber values. (b–d) simpleSOM predictions of the SOA mass yields from atmospheric simulations performed under low NOX conditions for different Db,atmos values. Note the significantly different y-axis in panel (d). Symbols in panels (b) through (d) show the measured, end-of-experiment SOA mass yield and the estimated VBS SOA mass yield at an OA mass concentration of 10 μg m−3. | |
Each of the fit parameter sets (tabulated in Table S3) reproduced the observations of SOA mass concentration and O
:
C with the exception that the parameters for a Db,chamber of 10−17 cm2 s−1 slightly overestimated the observations of SOA mass concentration near the end of the chamber experiment. Interestingly, the parameter sets for an assumed Db,chamber of 10−6 and 3 × 10−15 cm2 s−1 were nearly identical and so were the parameters for all Db,chamber values in between (not tabulated). The primary reason for this was that even though the Db value varied over nine orders of magnitude, there were few limitations to mass transfer over this Db range. This was because the timescales for mixing were sufficiently short over the length scale over which the bulk-phase diffusion took place (lj). To remind the reader, the length scale for bulk-phase diffusion in these experiments was restricted to the SOA coating on the solid ammonium sulfate seed particles; at the end of the chamber experiment lj for the mass median particle (300 nm) was 150 nm. For a 150 nm coating on a 300 nm seed particle, the characteristic mixing timescale for a Db between 10−6 and 3 × 10−15 cm2 s−1 would be between ∼0 and 2 hours, relatively shorter than the timescale over which SOA were produced (>12 hours). However, the parameter set for an assumed Db,chamber of 10−17 cm2 s−1 was substantially different from those for the larger assumed Db,chamber values (i.e., 10−6 and 3 × 10−15 cm2 s−1). This difference was because the timescales for mixing were now much longer, posing significant limitations for mass transfer. For a 150 nm coating on a 300 nm seed particle, the characteristic mixing timescale for a Db of 10−17 cm2 s−1 would be more than 26 days. To reproduce the chamber observations, the parameter sets for an assumed Db,chamber of 10−17 cm2 s−1 resulted in an excess of condensable SOA mass that had not entirely condensed to the particle phase by the end of the chamber experiment. Evidence for this line of reasoning can be found by examining the model response beyond the duration of the chamber experiment. The predictions of the SOA mass concentration from an assumed Db,chamber of 10−6 and 3 × 10−15 cm2 s−1 were relatively flat while those from an assumed Db,chamber of 10−17 cm2 s−1 continued to increase as more of the condensable mass partitioned into the particle phase beyond the duration of the chamber experiment.
We performed atmospheric simulations with three different parameter sets from the chamber simulations, one for each assumed Db,chamber (10−6, 3 × 10−15, and 10−17 cm2 s−1), and three different Db,atmos values (10−6, 10−15, and 10−17 cm2 s−1) assumed for the atmospheric OA. These results are presented in Fig. 4(b–d). For example, in Fig. 4(c), we show results for the parameter set which were determined by assuming a semisolid aerosol (Db = 3 × 10−15 cm2 s−1) in the chamber simulation but assuming a Db,atmos of 10−6, 10−15, and 10−17 cm2 s−1 for the OA in the atmospheric simulation. With a few differences in the parameter sets for an assumed Db,chamber of 10−6 and 3 × 10−15 cm2 s−1, the results in Fig. 4(b) and (c) were nearly identical. However, as a larger Db,atmos was associated with shorter mixing timescales and faster uptake of SOA, the predictions of the SOA mass yield for the same parameter set were higher with the use of a larger Db,atmos value. We saw differences in the model predictions with the use of a Db,atmos value of 10−6 and 3 × 10−15 because, in contrast to the chamber simulations where α-pinene SOA were assumed to condense into an SOA coating on a solid seed, the SOA in the atmospheric simulations were assumed to condense into an absorbing OA particle. For simulations performed with a given Db,chamber value, differences in SOA mass yields were substantial initially (e.g., a factor of ∼3 and ∼5 higher for a Db,atmos of 10−6 compared to a Db,atmos of 10−15 and 10−17 cm2 s−1, respectively), but the SOA mass yields seemed to converge with photochemical aging. Simulation results for OAs with a Db,atmos of 10−15 cm2 s−1 converged with those for OAs with a Db,atmos of 10−6 cm2 s−1 in a few hours while those for OAs with a Db,atmos of 10−17 cm2 s−1 started to converge after a day of photochemical aging. As the parameter sets for an assumed Db,chamber of 10−17 cm2 s−1 produced more condensable SOA mass, it was understandable that the predicted SOA mass yields in Fig. 4(d) were larger than those shown in Fig. 4(b) and (c). The peak SOA mass yields for a Db,chamber of 10−17 cm2 s−1 were nearly a factor of 5 larger than those for a Db,chamber of 10−6 and 10−15 cm2 s−1, suggesting that such a low Db value might be unrealistic for α-pinene SOA. Similar to the findings described in Fig. 3, the atmospheric SOA mass yields for a Db,chamber value of 10−6 and 3 × 10−15 cm2 s−1 were lower than the SOA mass yield measured at the end of the chamber experiment but larger than the SOA mass yield predicted using a VBS fit to the chamber data.
For SOA parameters that are determined from typical chamber experiments performed under dry conditions (RH < 20%) and with an inert solid seed available for SOA condensation, there are two key implications for the results presented above. First, the atmospheric SOA mass yields are unlikely to be sensitive to the phase state assumed in the chamber experiments if the Db value for the chamber SOA is approximately higher than 10−15 cm2 s−1. This would appear to be the case for α-pinene SOA, which have recently been shown to exhibit a Db on the order of 10−15 cm2 s−1.76,109,112 Second, the atmospheric SOA mass yields are sensitive to the Db value of the absorbing OA, but this sensitivity is limited to the first few hours of photochemical aging if this Db value is higher than 10−15 cm2 s−1, extending to a few days for Db values lower than 10−15 cm2 s−1. The phase state of atmospheric OAs is largely uncertain since there are only a handful of observations38,113,114 and modeling studies37,115 that have attempted to do so. Shiraiwa et al.37 used a global model to argue that the surface-level OA at lower latitudes is likely to be liquid-like (>10−15 cm2 s−1) from the presence of some aerosol water and warmer temperatures but could take on a semisolid (10−15 to 10−25 cm2 s−1) or solid phase (<10−25 cm2 s−1) at higher latitudes and altitudes where the OA experiences lower temperatures and drier conditions on average. Based on those findings, we would expect the atmospheric SOA mass yields to be largely unaffected at the surface level but they could be influenced by low Db values for SOA produced at higher altitudes. Nonetheless, to evaluate the model predictions and its potential impacts, future work should aim to estimate the Db of SOA formed from different precursors in laboratory experiments and estimate the Db of atmospheric OAs from ambient measurements.
5. Summary and discussion
In this work, we developed a state-of-the-science, process-level model called simpleSOM to simulate the chemistry, thermodynamic properties, and microphysics of SOA. simpleSOM is a reduced and computationally efficient version of the statistical oxidation model (SOM) of Cappa and Wilson54 and was specifically designed to be used in 3D atmospheric models. simpleSOM leverages a VBS framework to track the oxidation chemistry of gas- and particle-phase species and to calculate the properties of interest (e.g., kOH, c*, Pfrag, and O
:
C). An important feature of simpleSOM is that it is parameterizable, which allows the user to determine SOA parameters that can reproduce laboratory observations of SOA. To demonstrate the capabilities of the model, we used simpleSOM to simulate SOA formation from the photooxidation of α-pinene. For this model system, we were able to demonstrate that a simpleSOM parameter set, fit to representative environmental chamber data, was able to generally reproduce historical observations of SOA formation (i.e., mass yields), composition (i.e., O
:
C), and properties (i.e., volatility). We performed two separate case studies to better understand the impact of accounting for oligomerization reactions and the particle phase state when developing simpleSOM parameters from chamber data. We found that accounting for oligomerization reactions and assuming a semisolid aerosol, both of which have been found to be relevant for α-pinene SOA, tended to increase SOA mass yields in the real atmosphere when compared to the SOA mass yields derived in the absence of oligomerization reactions and assuming a liquid-like aerosol.
Recently, a growing body of work has quantified the emissions and volatility distribution of primary organic aerosols (POAs) and the speciation and SOA formation from semi-volatile and intermediate-volatility organic compounds (S/IVOCs) from a range of combustion sources including gasoline and diesel vehicles, aircraft, and biomass burning.116–118 So while the model development and application in this work have centered around SOA formation from VOCs, simpleSOM can be easily extended to model the oxidation chemistry, thermodynamic properties, and microphysics of OAs from combustion sources. In this work, we only leveraged a subset of commonly gathered data from historical experiments to study the processes influencing SOA formation, composition, and properties. By leveraging a more complete set of gas and aerosol measurements including those gathered through novel experimentation, we argue that these measurements, old and new, when integrated with the simpleSOM model have the potential to better constrain process-specific SOA parameters. For instance, several studies have been able to leverage the evolution of the aerosol size distribution with kinetic SOA models to constrain the oxidation chemistry,119 volatility,33,120 and phase state76,109 of SOA. Analogous to the use of the aerosol size distribution, the detailed speciation of gas- and particle-phase organic compounds (e.g., D'Ambro et al.28 and Isaacman-VanWertz et al.12) made possible by advanced mass spectrometry techniques could be put to similar use. Finally, we will also aim to study the ability of the simpleSOM parameters to simulate the SOA formation from α-pinene (and other SOA precursors) under different and continuously varying NOX conditions.
A modern-day 3D model typically simulates SOA formation from several classes of VOC precursors that include, but are not limited to, isoprene, terpenes, alkanes, alkenes, aromatics, and S/IVOCs.3 While some of the SOA precursors are modeled separately (e.g., isoprene and benzene), those with a similar potential to form SOA are frequently lumped together for computational efficiency (e.g., C8+ single-ring aromatics). Regardless, there is a fair amount of diversity in 3D models as to the precise number of SOA precursors included and this precursor number can vary between 2 and 10.5 To calculate, as an example, the computational burden simpleSOM would impose on a 3D model, let us assume that a typical 3D model simulates the SOA formation from 5 unique precursors. An advantage of a simpleSOM set is that oxidation products from multiple VOCs can be tracked in the same set, assuming that the parameters can be extended to simulate the SOA formation from those VOCs. Hence, SOA from VOCs with similar characteristics could be ‘lumped’ into the same simpleSOM set, akin to how VOCs are lumped together in gas-phase chemical mechanisms for computational efficiency. For each precursor, let us assume that the simpleSOM set spans across 12 log
c* bins (e.g., −3 to 8). The lowest log
c* bin could be set to −3 (instead of −6 as used in this work) since species with a log
c* of −3 are functionally non-volatile under most conditions relevant to the atmosphere. This simpleSOM set could be reduced to track a subset of the model species. For instance, we could choose to ignore the gas-phase species for the low log
c* bins (e.g., log
c* from −3 to 0) and ignore the particle-phase species for the high log
c* bins (e.g., log
c* from 4 to 8) because these model species are expected to exclusively reside in the gas and particle phases, respectively, under most atmospherically relevant conditions. The exact reduction would need to be configured depending on the atmospheric conditions being simulated. Up to 12 additional species will be needed to track the oxygen content in each c* bin although the O
:
C could be parameterized to the c* bin for a given simpleSOM set to further optimize the implementation. In total, a minimum of ∼27 model species will need to be tracked per simpleSOM set. For 5 unique SOA precursors, a simpleSOM representation in a 3D model with no particle size resolution would require a minimum of 40 gas-phase, 35 particle-phase, and 60 oxygen model species. If the 3D model included a particle size-resolved model with, e.g., 10 size sections, the number of particle-phase model species would be 350. Altogether, a basic simpleSOM representation would require a total of 135 (no size resolution) or 425 (with size resolution) species in a 3D model. Several recent studies with regional and global models have included a similar range of gas- and particle-phase species to model SOA and the estimates provided here are now well within reach of modern-day 3D models.49,121,122
The simpleSOM model is available as an IGOR code in the dataset published along with this manuscript (Section 6). This model can be used to perform both chamber and atmospheric simulations and be used to determine SOA parameters that can reproduce laboratory observations of SOA mass concentration and O
:
C. In theory, simpleSOM should also be able to simulate SOA formation in oxidation flow reactors (OFRs) and non-Teflon chambers124,125 noting that certain processes (e.g., vapor wall losses and batch versus steady-state mode) will need to be modeled differently. OFRs are increasingly being used to study SOA formation in laboratory123,126,127 and field128–131 environments, and chamber-based SOA parameters could be evaluated and updated based on the application of simpleSOM to OFR data. In subsequent publications, we aim to use simpleSOM to develop parameters for other important SOA precursors based on the chamber data from Caltech and other research groups. Additionally, simpleSOM has been coupled with the Model for Simulating Aerosol Interactions and Chemistry (MOSAIC)65,80 for eventual implementation in the Weather Research and Forecasting Chemistry (WRF-Chem) model. The simpleSOM-MOSAIC model was developed in Fortran with a Python wrapper and a benchmarked version of the code for this model has been made available in the dataset published along with this manuscript. The Fortran-Python version may be better suited for adoption by the wider atmospheric chemistry and air quality community.
The simpleSOM version described in this work is able to simulate multiphase and multigenerational aging processes and phase-state-influenced kinetic gas/particle partitioning. However, there are two key processes that are not currently modeled in simpleSOM but will need to be represented in future work. First, aerosols and cloud water can take up and chemically process oxygenated VOCs to form SOA through aqueous phase reactions.9,132 In 3D models, aqueous chemistry modeling has so far focused on simulating the SOA formation from a few unique oxygenated VOCs that are produced in large quantities in the atmosphere (e.g., isoprene epoxydiol, glyoxal, and methylglyoxal).133,134 These aqueous SOA precursors and pathways have been included in 3D models explicitly and it is unclear whether the statistical approach within simpleSOM offers the right framework to model the aqueous chemistry for all organic compounds. Second, gas- and particle-phase organic compounds within SOA can photolyze, which have been shown to result in the loss of SOA mass in controlled laboratory experiments.135,136 The earlier photolysis work has mostly focused on SOA produced from biogenic VOCs, where organic peroxides and carbonyls have been identified as key product classes that are sensitive to photolysis.137,138 Similar to the argument made earlier, it is unclear whether models could benefit from modeling the photolysis of a few key species/classes or if there is a benefit in representing photolysis statistically from all organic compounds. If, however, we chose to represent aqueous chemistry and photolysis within simpleSOM, a statistical approach would require developing parameterizations to model solubility, pH-dependent reactivity, photolysis rates, and parameters to determine product yields and volatility. In the case of aqueous chemistry, we may have to further develop the code to account for interactions and separation of the organic and aqueous phases, as modulated by the aerosol composition and environmental conditions (e.g., relative humidity).139,140 These parameterizations and parameters could be informed by highly detailed kinetic models that have been developed over the past decade.141,142
Overall, simpleSOM provides a comprehensive, process-based, and kinetic framework to consistently model the formation, evolution, and properties of POA-SOA-OA in box, Lagrangian, and 3D models. The application of simpleSOM to laboratory and field data is likely to provide insight into the key processes that drive SOA formation and evolution and ultimately improve the representation of OAs in 3D atmospheric models.
Data availability
Experimental data used in this work are from Dr John Seinfeld’s group at the California Institute of Technology and are now available as part of the Index of Chamber Atmospheric Research in the United States (ICARUS; https://icarus.ucdavis.edu). The latest versions of the IGOR and Fortran models for simpleSOM/simpleSOM-MOSAIC along with the simulation data are archived with Colorado State University Libraries (http://dx.doi.org/10.25675/10217/232634) and Github (https://github.com/ARM-Synergy/simpleSOM_boxmodel). The data from all the figures in the paper are also available with Colorado State University Libraries (http://dx.doi.org/10.25675/10217/232634).
Author contributions
SHJ and CDC designed the model framework and overall study. CDC developed the IGOR model and YH and WC updated the model. YH developed the Fortran-Python model and YH and KRB benchmarked this model against the IGOR model. YH performed the simulations and YH and SHJ analyzed the data. JHS provided the experimental data. RAZ, MS, and JRP supported the model development and analysis. KRB helped with archiving the code and model output. SHJ and YH wrote the paper with contributions from all co-authors.
Conflicts of interest
There are no conflicts to declare.
Acknowledgements
This work was supported by the U.S. Department of Energy (DOE), Office of Science (DE-SC0017975). RAZ acknowledges support from the U.S. DOE Office of Science as part of the Atmospheric System Research Program at the Pacific Northwest National Laboratory (PNNL). MS was supported by the U.S. DOE, Office of Science, and Office of Biological and Environmental Research through the Early Career Research Program. PNNL is operated for DOE by Battelle Memorial Institute under contract DE-AC05-76RL0 1830. We thank Dr Benjamin Murphy at the U.S. Environmental Protection Agency for helpful conversations concerning CMAQ.
References
- J. L. Jimenez, M. R. Canagaratna, N. M. Donahue, A. S. H. Prevot, Q. Zhang, J. H. Kroll, P. F. DeCarlo, J. D. Allan, H. Coe, N. L. Ng, A. C. Aiken, K. S. Docherty, I. M. Ulbrich, A. P. Grieshop, A. L. Robinson, J. Duplissy, J. D. Smith, K. R. Wilson, V. A. Lanz, C. Hueglin, Y. L. Sun, J. Tian, A. Laaksonen, T. Raatikainen, J. Rautiainen, P. Vaattovaara, M. Ehn, M. Kulmala, J. M. Tomlinson, D. R. Collins, M. J. Cubison, E. J. Dunlea, J. A. Huffman, T. B. Onasch, M. R. Alfarra, P. I. Williams, K. Bower, Y. Kondo, J. Schneider, F. Drewnick, S. Borrmann, S. Weimer, K. Demerjian, D. Salcedo, L. Cottrell, R. Griffin, A. Takami, T. Miyoshi, S. Hatakeyama, A. Shimono, J. Y. Sun, Y. M. Zhang, K. Dzepina, J. R. Kimmel, D. Sueper, J. T. Jayne, S. C. Herndon, A. M. Trimborn, L. R. Williams, E. C. Wood, A. M. Middlebrook, C. E. Kolb, U. Baltensperger and D. R. Worsnop, Evolution of Organic Aerosols in the Atmosphere, Science, 2009, 326(5959), 1525–1529 CrossRef CAS PubMed.
- S. Fuzzi, U. Baltensperger, K. Carslaw, S. Decesari, H. Denier van der Gon, M. C. Facchini, D. Fowler, I. Koren, B. Langford, U. Lohmann, E. Nemitz, S. Pandis, I. Riipinen, Y. Rudich, M. Schaap, J. G. Slowik, D. V. Spracklen, E. Vignati, M. Wild, M. Williams and S. Gilardoni, Particulate Matter, Air Quality and Climate: Lessons Learned and Future Needs, Atmos. Chem. Phys., 2015, 15(14), 8217–8299 CrossRef CAS.
- M. Shrivastava, C. D. Cappa, J. Fan, A. H. Goldstein, A. B. Guenther, J. L. Jimenez, C. Kuang, A. Laskin, S. T. Martin and N. L. Ng, Recent Advances in Understanding Secondary Organic Aerosol: Implications for Global Climate Forcing, Rev. Geophys., 2017, 55(2), 509–559 CrossRef.
- A. H. Goldstein and I. E. Galbally, Known and Unknown Organic Constituents in the Earth’ S Atmosphere, Environ. Sci. Technol., 2007, 41(5), 1514–1521 CrossRef CAS.
- K. Tsigaridis, N. Daskalakis, M. Kanakidou, P. J. Adams, P. Artaxo, R. Bahadur, Y. Balkanski, S. E. Bauer, N. Bellouin, A. Benedetti, T. Bergman, T. K. Berntsen, J. P. Beukes, H. Bian, K. S. Carslaw, M. Chin, G. Curci, T. Diehl, R. C. Easter, S. J. Ghan, S. L. Gong, A. Hodzic, C. R. Hoyle, T. Iversen, S. Jathar, J. L. Jimenez, J. W. Kaiser, A. Kirkevåg, D. Koch, H. Kokkola, Y. H. Lee, G. Lin, X. Liu, G. Luo, X. Ma, G. W. Mann, N. Mihalopoulos, J.-J. Morcrette, J.-F. Müller, G. Myhre, S. Myriokefalitakis, N. L. Ng, D. O'Donnell, J. E. Penner, L. Pozzoli, K. J. Pringle, L. M. Russell, M. Schulz, J. Sciare, Ø. Seland, D. T. Shindell, S. Sillman, R. B. Skeie, D. Spracklen, T. Stavrakou, S. D. Steenrod, T. Takemura, P. Tiitta, S. Tilmes, H. Tost, T. van Noije, P. G. van Zyl, K. von Salzen, F. Yu, Z. Wang, Z. Wang, R. A. Zaveri, H. Zhang, K. Zhang, Q. Zhang and X. Zhang, The AeroCom Evaluation and Intercomparison of Organic Aerosol in Global Models, Atmos. Chem. Phys., 2014, 14(19), 10845–10895 CrossRef.
- S. J. Pai, C. L. Heald, J. R. Pierce, S. C. Farina, E. A. Marais, J. L. Jimenez, P. Campuzano-Jost, B. A. Nault, A. M. Middlebrook, H. Coe, J. E. Shilling, R. Bahreini, J. H. Dingle and K. Vu, An Evaluation of Global Organic Aerosol Schemes Using Airborne Observations, Atmos. Chem. Phys., 2020, 20(5), 2637–2665 CrossRef CAS.
- J. F. Pankow, An Absorption Model of Gas/particle Partitioning of Organic Compounds in the Atmosphere, Atmos. Environ., 1994, 28,, 185–188 CrossRef.
- N. M. Donahue, A. L. Robinson, C. O. Stanier and S. N. Pandis, Coupled Partitioning, Dilution, and Chemical Aging of Semivolatile Organics, Environ. Sci. Technol., 2006, 40(8), 2635–2643 CrossRef CAS PubMed.
- V. F. McNeill, Aqueous Organic Chemistry in the Atmosphere: Sources and Chemical Processing of Organic Aerosols, Environ. Sci. Technol., 2015, 49(3), 1237–1244 CrossRef CAS PubMed.
- B. N. Murphy, M. C. Woody, J. L. Jimenez, A. M. G. Carlton, P. L. Hayes, S. Liu, N. L. Ng, L. M. Russell, A. Setyan, L. Xu, J. Young, R. A. Zaveri, Q. Zhang and H. O. T. Pye, Semivolatile POA and Parameterized Total Combustion SOA in CMAQv5.2: Impacts on Source Strength and Partitioning, Atmos. Chem. Phys., 2017, 17(18), 11107–11133 CrossRef CAS PubMed.
-
K. W. Appel, J. O. Bash, K. M. Fahey, K. M. Foley, R. C. Gilliam, C. Hogrefe, W. T. Hutzell, D. Kang, R. Mathur, B. N. Murphy, S. L. Napelenok, C. G. Nolte, J. E. Pleim, G. A. Pouliot, H. O. T. Pye, L. Ran, S. J. Roselle, G. Sarwar, D. B. Schwede, F. I. Sidi, T. L. Spero and D. C. Wong, The Community Multiscale Air Quality (CMAQ) Model Versions 5.3 and 5.3.1, System Updates and Evaluation, 2020, DOI: DOI:10.5194/gmd-2020-345.
- G. Isaacman-VanWertz, P. Massoli, R. O'Brien, C. Lim, J. P. Franklin, J. A. Moss, J. F. Hunter, J. B. Nowak, M. R. Canagaratna, P. K. Misztal, C. Arata, J. R. Roscioli, S. T. Herndon, T. B. Onasch, A. T. Lambe, J. T. Jayne, L. Su, D. A. Knopf, A. H. Goldstein, D. R. Worsnop and J. H. Kroll, Chemical Evolution of Atmospheric Organic Carbon over Multiple Generations of Oxidation, Nat. Chem., 2018, 10(4), 462–468 CrossRef CAS.
- J. D. Smith, J. H. Kroll, C. D. Cappa, D. L. Che, C. L. Liu, M. Ahmed, S. R. Leone, D. R. Worsnop and K. R. Wilson, The Heterogeneous Reaction of Hydroxyl Radicals with Sub-Micron Squalane Particles: A Model System for Understanding the Oxidative Aging of Ambient Aerosols, Atmos. Chem. Phys., 2009, 9(9), 3209–3222 CrossRef CAS.
- K. C. Barsanti and J. F. Pankow, Thermodynamics of the Formation of Atmospheric Organic Particulate Matter by Accretion reactions—Part 1: Aldehydes and Ketones, Atmos. Environ., 2004, 38(26), 4371–4382 CrossRef CAS.
- K. C. Barsanti and J. F. Pankow, Thermodynamics of the Formation of Atmospheric Organic Particulate Matter by Accretion reactions—2. Dialdehydes, Methylglyoxal, and Diketones, Atmos. Environ., 2005, 39(35), 6597–6607 CrossRef CAS.
- K. C. Barsanti and J. F. Pankow, Thermodynamics of the Formation of Atmospheric Organic Particulate Matter by Accretion reactions—Part 3: Carboxylic and Dicarboxylic Acids, Atmos. Environ., 2006, 40(34), 6676–6686 CrossRef CAS.
- A. L. Robinson, N. M. Donahue, M. K. Shrivastava, E. A. Weitkamp, A. M. Sage, A. P. Grieshop, T. E. Lane, J. R. Pierce and S. N. Pandis, Rethinking Organic Aerosols: Semivolatile Emissions and Photochemical Aging, Science, 2007, 315(5816), 1259–1262 CrossRef CAS.
- M. Ehn, J. A. Thornton, E. Kleist, M. Sipilä, H. Junninen, I. Pullinen, M. Springer, F. Rubach, R. Tillmann, B. Lee, F. Lopez-Hilfiker, S. Andres, I.-H. Acir, M. Rissanen, T. Jokinen, S. Schobesberger, J. Kangasluoma, J. Kontkanen, T. Nieminen, T. Kurtén, L. B. Nielsen, S. Jørgensen, H. G. Kjaergaard, M. Canagaratna, M. D. Maso, T. Berndt, T. Petäjä, A. Wahner, V.-M. Kerminen, M. Kulmala, D. R. Worsnop, J. Wildt and T. F. Mentel, A Large Source of Low-Volatility Secondary Organic Aerosol, Nature, 2014, 506(7489), 476–479 CrossRef CAS.
- J. E. Shilling, Q. Chen, S. M. King, T. Rosenoern, J. H. Kroll, D. R. Worsnop, P. F. DeCarlo, A. C. Aiken, D. Sueper, J. L. Jimenez and S. T. Martin, Loading-Dependent Elemental Composition of α-Pinene SOA Particles, Atmos. Chem. Phys., 2008, 8(4), 15343–15373 Search PubMed.
- P. K. Saha and A. P. Grieshop, Exploring Divergent Volatility Properties from Yield and Thermodenuder Measurements of Secondary Organic Aerosol from α-Pinene Ozonolysis, Environ. Sci. Technol., 2016, 50(11), 5740–5749 CrossRef CAS PubMed.
- T. Chen, Y. Liu, C. Liu, J. Liu, B. Chu and H. He, Important Role of Aromatic Hydrocarbons in SOA Formation from Unburned Gasoline Vapor, Atmos. Environ., 2019, 201, 101–109 CrossRef CAS.
- J. H. Kroll, J. D. Smith, D. L. Che, S. H. Kessler, D. R. Worsnop and K. R. Wilson, Measurement of Fragmentation and Functionalization Pathways in the Heterogeneous Oxidation of Oxidized Organic Aerosol, Phys. Chem. Chem. Phys., 2009, 11(36), 8005–8014 RSC.
- H. J. Chacon-Madrid, A. A. Presto and N. M. Donahue, Functionalization vs. Fragmentation: N-Aldehyde Oxidation Mechanisms and Secondary Organic Aerosol Formation, Phys. Chem. Chem. Phys., 2010, 12(42), 13975–13982 RSC.
- J. H. Kroll, N. M. Donahue, J. L. Jimenez, S. H. Kessler, M. R. Canagaratna, K. R. Wilson, K. E. Altieri, L. R. Mazzoleni, A. S. Wozniak, H. Bluhm, E. R. Mysak, J. D. Smith, C. E. Kolb and D. R. Worsnop, Carbon Oxidation State as a Metric for Describing the Chemistry of Atmospheric Organic Aerosol, Nat. Chem., 2011, 3(2), 133–139 CrossRef CAS.
- S. H. Jathar, C. D. Cappa, A. S. Wexler, J. H. Seinfeld and M. J. Kleeman, Simulating Secondary Organic Aerosol in a Regional Air Quality Model Using the Statistical Oxidation Model--Part 1: Assessing the Influence of Constrained Multi-Generational Ageing, Atmos. Chem. Phys., 2016, 16(4), 2309–2322 CrossRef CAS.
- M. Shrivastava, A. Zelenyuk, D. Imre, R. Easter, J. Beranek, R. A. Zaveri and J. Fast, Implications of Low Volatility SOA and Gas-Phase Fragmentation Reactions on SOA Loadings and Their Spatial and Temporal Evolution in the Atmosphere, J. Geophys. Res., 2013, 118(8), 3328–3342 CAS.
- M. Shrivastava, R. C. Easter, X. Liu, A. Zelenyuk, B. Singh, K. Zhang, P.-L. Ma, D. Chand, S. Ghan, J. L. Jimenez, Q. Zhang, J. Fast, P. J. Rasch and P. Tiitta, Global Transformation and Fate of SOA: Implications of Low-Volatility SOA and Gas-Phase Fragmentation Reactions, J. Geophys. Res., 2015, 120(9), 4169–4195 CAS.
- E. L. D'Ambro, S. Schobesberger, R. A. Zaveri, J. E. Shilling, B. H. Lee, F. D. Lopez-Hilfiker, C. Mohr and J. A. Thornton, Isothermal Evaporation of α-Pinene Ozonolysis SOA: Volatility, Phase State, and Oligomeric Composition, ACS Earth Space Chem., 2018, 2(10), 1058–1067 CrossRef.
- K. R. Kolesar, Z. Li, K. R. Wilson and C. D. Cappa, Heating-Induced Evaporation of Nine Different Secondary Organic Aerosol Types, Environ. Sci. Technol., 2015, 49(20), 12242–12252 CrossRef CAS PubMed.
- Z. Meng, D. Dabdub and J. H. Seinfeld, Chemical Coupling Between Atmospheric Ozone and Particulate Matter, Science, 1997, 277(5322), 116–119 CrossRef CAS.
- M. Shiraiwa and J. H. Seinfeld, Equilibration Timescale of Atmospheric Secondary Organic Aerosol Partitioning, Geophys. Res. Lett., 2012, 39(24) DOI:10.1029/2012gl054008.
- X. Zhang, S. N. Pandis and J. H. Seinfeld, Diffusion-Limited Versus Quasi-Equilibrium Aerosol Growth, Aerosol Sci. Technol., 2012, 46(8), 874–885 CrossRef CAS.
- J. R. Pierce, I. Riipinen, M. Kulmala, M. Ehn, T. Petäjä, H. Junninen, D. R. Worsnop and N. M. Donahue, Quantification of the Volatility of Secondary Organic Compounds in Ultrafine Particles during Nucleation Events, Atmos. Chem. Phys., 2011, 11(17), 9019–9036 CrossRef CAS.
- I. Riipinen, J. R. Pierce, T. Yli-Juuti, T. Nieminen, S. Häkkinen, M. Ehn, H. Junninen, K. Lehtipalo, T. Petäjä, J. Slowik, R. Chang, N. C. Shantz, J. Abbatt, W. R. Leaitch, V.-M. Kerminen, D. R. Worsnop, S. N. Pandis, N. M. Donahue and M. Kulmala, Organic Condensation: A Vital Link Connecting Aerosol Formation to Cloud Condensation Nuclei (CCN) Concentrations, Atmos. Chem. Phys., 2011, 11(8), 3865–3878 CrossRef CAS.
- T. Koop, J. Bookhold, M. Shiraiwa and U. Pöschl, Glass Transition and Phase State of Organic Compounds: Dependency on Molecular Properties and Implications for Secondary Organic Aerosols in the Atmosphere, Phys. Chem. Chem. Phys., 2011, 13(43), 19238–19255 RSC.
- Y. Li and M. Shiraiwa, Timescales of Secondary Organic Aerosols to Reach Equilibrium at Various Temperatures and Relative Humidities, Atmos. Chem. Phys., 2019, 19(9), 5959–5971 CrossRef CAS.
- M. Shiraiwa, Y. Li, A. P. Tsimpidi, V. A. Karydis, T. Berkemeier, S. N. Pandis, J. Lelieveld, T. Koop and U. Pöschl, Global Distribution of Particle Phase State in Atmospheric Secondary Organic Aerosols, Nat. Commun., 2017, 8, 15002 CrossRef PubMed.
- X. Zhang, R. C. McVay, D. D. Huang, N. F. Dalleska, B. Aumont, R. C. Flagan and J. H. Seinfeld, Formation and Evolution of Molecular Products in α-Pinene Secondary Organic Aerosol, Proc. Natl. Acad. Sci. U. S. A., 2015, 112(46), 14168–14173 CrossRef CAS PubMed.
- W.-S. W. DeRieux, Y. Li, P. Lin, J. Laskin, A. Laskin, A. K. Bertram, S. A. Nizkorodov and M. Shiraiwa, Predicting the Glass Transition Temperature and Viscosity of Secondary Organic Material Using Molecular Composition, Atmos. Chem. Phys., 2018, 18(9), 6331–6351 CrossRef.
- J. P. Reid, A. K. Bertram, D. O. Topping, A. Laskin, S. T. Martin, M. D. Petters, F. D. Pope and G. Rovelli, The Viscosity of Atmospherically Relevant Organic Particles, Nat. Commun., 2018, 9(1), 956 CrossRef PubMed.
- A. Matsunaga and P. J. Ziemann ‡, Gas-Wall Partitioning of Organic Compounds in a Teflon Film Chamber and Potential Effects on Reaction Product and Aerosol Yield Measurements, Aerosol Sci. Technol., 2010, 44(10), 881–892 CrossRef CAS.
- J. E. Krechmer, D. Pagonis, P. J. Ziemann and J. L. Jimenez, Quantification of Gas-Wall Partitioning in Teflon Environmental Chambers Using Rapid Bursts of Low-Volatility Oxidized Species Generated in Situ, Environ. Sci. Technol., 2016, 50(11), 5757–5765 CrossRef CAS PubMed.
- Q. Ye, E. S. Robinson, X. Ding, P. Ye, R. C. Sullivan and N. M. Donahue, Mixing of Secondary Organic Aerosols versus Relative Humidity, Proc. Natl. Acad. Sci. U. S. A., 2016, 113(45), 12649–12654 CrossRef CAS.
- R. C. McVay, C. D. Cappa and J. H. Seinfeld, Vapor-Wall Deposition in Chambers: Theoretical Considerations, Environ. Sci. Technol., 2014, 48(17), 10251–10258 CrossRef CAS PubMed.
- X. Zhang, C. D. Cappa, S. H. Jathar, R. C. McVay, J. J. Ensberg, M. J. Kleeman and J. H. Seinfeld, Influence of Vapor Wall Loss in Laboratory Chambers on Yields of Secondary Organic Aerosol, Proc. Natl. Acad. Sci. U. S. A., 2014, 111(16), 5802–5807 CrossRef CAS PubMed.
- Y. Huang, R. Zhao, S. M. Charan, C. M. Kenseth, X. Zhang and J. H. Seinfeld, Unified Theory of Vapor-Wall Mass Transport in Teflon-Walled Environmental Chambers, Environ. Sci. Technol., 2018, 52(4), 2134–2142 CrossRef CAS PubMed.
- L. Xing, M. Shrivastava, T.-M. Fu, P. Roldin, Y. Qian, L. Xu, N. L. Ng, J. Shilling, A. Zelenyuk and C. D. Cappa, Parameterized Yields of Semivolatile Products from Isoprene Oxidation under Different NO X Levels: Impacts of Chemical Aging and Wall-Loss of Reactive Gases, Environ. Sci. Technol., 2018, 52(16), 9225–9234 CrossRef CAS PubMed.
- C. D. Cappa, S. H. Jathar, M. J. Kleeman, K. S. Docherty, J. L. Jimenez, J. H. Seinfeld and A. S. Wexler, Simulating Secondary Organic Aerosol in a Regional Air Quality Model Using the Statistical Oxidation Model--Part 2: Assessing the Influence of Vapor Wall Losses, Atmos. Chem. Phys., 2016, 16(5), 3041–3059 CrossRef CAS.
- A. Akherati, C. D. Cappa, M. J. Kleeman, K. S. Docherty, J. L. Jimenez, S. M. Griffith, S. Dusanter, P. S. Stevens and S. H. Jathar, Simulating Secondary Organic Aerosol in a Regional Air Quality Model Using the Statistical Oxidation Model – Part 3: Assessing the Influence of Semi-Volatile and Intermediate-Volatility Organic Compounds and NO X, Atmos. Chem. Phys., 2019, 19(7), 4561–4594 CrossRef CAS.
- L. Hildebrandt, N. M. Donahue and S. N. Pandis, High Formation of Secondary Organic Aerosol from the Photo-Oxidation of Toluene, Atmos. Chem. Phys., 2009, 9(9), 2973–2986 CrossRef CAS.
- P. L. Hayes, A. M. Ortega, M. J. Cubison, K. D. Froyd, Y. Zhao, S. S. Cliff, W. W. Hu, D. W. Toohey, J. H. Flynn, B. L. Lefer, N. Grossberg, S. Alvarez, B. Rappenglück, J. W. Taylor, J. D. Allan, J. S. Holloway, J. B. Gilman, W. C. Kuster, J. A. de Gouw, P. Massoli, X. Zhang, J. Liu, R. J. Weber, A. L. Corrigan, L. M. Russell, G. Isaacman, D. R. Worton, N. M. Kreisberg, A. H. Goldstein, R. Thalman, E. M. Waxman, R. Volkamer, Y. H. Lin, J. D. Surratt, T. E. Kleindienst, J. H. Offenberg, S. Dusanter, S. Griffith, P. S. Stevens, J. Brioude, W. M. Angevine and J. L. Jimenez, Organic Aerosol Composition and Sources in Pasadena, California, during the 2010 CalNex Campaign, J. Geophys. Res.: Atmos., 2013, 118(16), 9233–9257 Search PubMed.
- R. U. Shah, E. S. Robinson, P. Gu, A. L. Robinson, J. S. Apte and A. A. Presto, High-Spatial-Resolution Mapping and Source Apportionment of Aerosol Composition in Oakland, California, Using Mobile Aerosol Mass Spectrometry, Atmos. Chem. Phys., 2018, 18(22), 16325–16344 CrossRef CAS.
- C. D. Cappa and J. L. Jimenez, Quantitative Estimates of the Volatility of Ambient Organic Aerosol, Atmos. Chem. Phys., 2010, 10(12), 5409–5424 CrossRef CAS.
- C. D. Cappa and K. R. Wilson, Multi-Generation Gas-Phase Oxidation, Equilibrium Partitioning, and the Formation and Evolution of Secondary Organic Aerosol, Atmos. Chem. Phys., 2012, 12(20), 9505–9528 CrossRef CAS.
- R. Ahmadov, S. A. McKeen, A. L. Robinson, R. Bahreini, A. M. Middlebrook, J. A. de Gouw, J. Meagher, E.-Y. Hsie, E. Edgerton, S. Shaw and M. Trainer, A Volatility Basis Set Model for Summertime Secondary Organic Aerosols over the Eastern United States in 2006, J. Geophys. Res.: Atmos., 2012, 117(D6) DOI:10.1029/2011JD016831.
- M. Shrivastava, M. O. Andreae, P. Artaxo, H. M. J. Barbosa, L. K. Berg, J. Brito, J. Ching, R. C. Easter, J. Fan, J. D. Fast, Z. Feng, J. D. Fuentes, M. Glasius, A. H. Goldstein, E. G. Alves, H. Gomes, D. Gu, A. Guenther, S. H. Jathar, S. Kim, Y. Liu, S. Lou, S. T. Martin, V. F. McNeill, A. Medeiros, S. S. de Sá, J. E. Shilling, S. R. Springston, R. A. F. Souza, J. A. Thornton, G. Isaacman-VanWertz, L. D. Yee, R. Ynoue, R. A. Zaveri, A. Zelenyuk and C. Zhao, Urban Pollution Greatly Enhances Formation of Natural Aerosols over the Amazon Rainforest, Nat. Commun., 2019, 10(1), 1046 CrossRef PubMed.
- L. N. Posner, G. Theodoritsi, A. Robinson, G. Yarwood, B. Koo, R. Morris, M. Mavko, T. Moore and S. N. Pandis, Simulation of Fresh and Chemically-Aged Biomass Burning Organic Aerosol, Atmos. Environ., 2019, 196, 27–37 CrossRef CAS.
- B. H. Lee, C. Mohr, F. D. Lopez-Hilfiker, A. Lutz, M. Hallquist, L. Lee, P. Romer, R. C. Cohen, S. Iyer, T. Kurtén, W. Hu, D. A. Day, P. Campuzano-Jost, J. L. Jimenez, L. Xu, N. L. Ng, H. Guo, R. J. Weber, R. J. Wild, S. S. Brown, A. Koss, J. de Gouw, K. Olson, A. H. Goldstein, R. Seco, S. Kim, K. McAvey, P. B. Shepson, T. Starn, K. Baumann, E. S. Edgerton, J. Liu, J. E. Shilling, D. O. Miller, W. Brune, S. Schobesberger, E. L. D'Ambro and J. A. Thornton, Highly Functionalized Organic Nitrates in the Southeast United States: Contribution to Secondary Organic Aerosol and Reactive Nitrogen Budgets, Proc. Natl. Acad. Sci. U. S. A., 2016, 113(6), 1516–1521 CrossRef CAS.
- F. Bianchi, T. Kurtén, M. Riva, C. Mohr, M. P. Rissanen, P. Roldin, T. Berndt, J. D. Crounse, P. O. Wennberg, T. F. Mentel, J. Wildt, H. Junninen, T. Jokinen, M. Kulmala, D. R. Worsnop, J. A. Thornton, N. Donahue, H. G. Kjaergaard and M. Ehn, Highly Oxygenated Organic Molecules (HOM) from Gas-Phase Autoxidation Involving Peroxy Radicals: A Key Contributor to Atmospheric Aerosol, Chem. Rev., 2019, 119(6), 3472–3509 CrossRef CAS PubMed.
- J. H. Kroll and J. H. Seinfeld, Chemistry of Secondary Organic Aerosol: Formation and Evolution of Low-Volatility Organics in the Atmosphere, Atmos. Environ., 2008, 42(16), 3593–3624 CrossRef CAS.
- J. F. Pankow and W. E. Asher, SIMPOL.1: A Simple Group Contribution Method for Predicting Vapor Pressures and Enthalpies of Vaporization of Multifunctional Organic Compounds, Atmos. Chem. Phys., 2008, 8(10), 2773–2796 CrossRef CAS.
- S. Compernolle, K. Ceulemans and Müller J.-F., EVAPORATION: A New Vapour Pressure Estimation Method for Organic Molecules Including Non-Additivity and Intramolecular Interactions, Atmos. Chem. Phys., 2011, 11(18), 9431–9450 CrossRef CAS.
- S. H. Jathar, C. D. Cappa, A. S. Wexler, J. H. Seinfeld and M. J. Kleeman, Multi-Generational Oxidation Model to Simulate Secondary Organic Aerosol in a 3-D Air Quality Model, Geosci. Model Dev., 2015, 8, 2553–2567 CrossRef CAS.
- D. S. Tkacik, A. A. Presto, N. M. Donahue and A. L. Robinson, Secondary Organic Aerosol Formation from Intermediate-Volatility Organic Compounds: Cyclic, Linear, and Branched Alkanes, Environ. Sci. Technol., 2012, 46(16), 8773–8781 CrossRef CAS.
- R. A. Zaveri, R. C. Easter, J. E. Shilling and J. H. Seinfeld, Modeling Kinetic Partitioning of Secondary Organic Aerosol and Size Distribution Dynamics: Representing Effects of Volatility, Phase State, and Particle-Phase Reaction, Atmos. Chem. Phys., 2014, 14(10), 5153–5181 CrossRef.
- J. Tröstl, W. K. Chuang, H. Gordon, M. Heinritzi, C. Yan, U. Molteni, L. Ahlm, C. Frege, F. Bianchi, R. Wagner, M. Simon, K. Lehtipalo, C. Williamson, J. S. Craven, J. Duplissy, A. Adamov, J. Almeida, A.-K. Bernhammer, M. Breitenlechner, S. Brilke, A. Dias, S. Ehrhart, R. C. Flagan, A. Franchin, C. Fuchs, R. Guida, M. Gysel, A. Hansel, C. R. Hoyle, T. Jokinen, H. Junninen, J. Kangasluoma, H. Keskinen, J. Kim, M. Krapf, A. Kürten, A. Laaksonen, M. Lawler, M. Leiminger, S. Mathot, O. Möhler, T. Nieminen, A. Onnela, T. Petäjä, F. M. Piel, P. Miettinen, M. P. Rissanen, L. Rondo, N. Sarnela, S. Schobesberger, K. Sengupta, M. Sipilä, J. N. Smith, G. Steiner, A. Tomè, A. Virtanen, A. C. Wagner, E. Weingartner, D. Wimmer, P. M. Winkler, P. Ye, K. S. Carslaw, J. Curtius, J. Dommen, J. Kirkby, M. Kulmala, I. Riipinen, D. R. Worsnop, N. M. Donahue and U. Baltensperger, The Role of Low-Volatility Organic Compounds in Initial Particle Growth in
the Atmosphere, Nature, 2016, 533(7604), 527–531 CrossRef PubMed.
- S. Eluri, C. D. Cappa, B. Friedman, D. K. Farmer and S. H. Jathar, Modeling the Formation and Composition of Secondary Organic Aerosol from Diesel Exhaust Using Parameterized and Semi-Explicit Chemistry and Thermodynamic Models, Atmos. Chem. Phys., 2018, 18(19), 13813–13838 CrossRef CAS.
-
S. N. Pandis; J. H. SeinfeldAtmospheric Chemistry and Physics: from Air Pollution to Climate Change, Wiley, 2006 Search PubMed.
- R. Bahreini, M. D. Keywood, N. L. Ng, V. Varutbangkul, S. Gao, R. C. Flagan, J. H. Seinfeld, D. R. Worsnop and J. L. Jimenez, Measurements of Secondary Organic Aerosol from Oxidation of Cycloalkenes, Terpenes, and M-Xylene Using an Aerodyne Aerosol Mass Spectrometer, Environ. Sci. Technol., 2005, 39(15), 5674–5688 CrossRef CAS PubMed.
- J. E. Krechmer, D. A. Day, P. J. Ziemann and J. L. Jimenez, Direct Measurements of Gas/Particle Partitioning and Mass Accommodation Coefficients in Environmental Chambers, Environ. Sci. Technol., 2017, 51(20), 11867–11875 CrossRef CAS PubMed.
- X. Liu, D. A. Day, J. E. Krechmer, W. Brown, Z. Peng, P. J. Ziemann and J. L. Jimenez, Direct Measurements of Semi-Volatile Organic Compound Dynamics Show near-Unity Mass Accommodation Coefficients for Diverse Aerosols, Commun. Chem., 2019, 2(1), 1–9 CrossRef.
- J. D. Smith, J. H. Kroll, C. D. Cappa, D. L. Che, C. L. Liu, M. Ahmed, S. R. Leone, D. R. Worsnop and K. R. Wilson, The Heterogeneous Reaction of Hydroxyl Radicals with Sub-Micron Squalane Particles: A Model System for Understanding the Oxidative Aging of Ambient Aerosols, Atmos. Chem. Phys., 2009, 9(9), 3209–3222 CrossRef CAS.
- J. H. Kroll, J. D. Smith, D. L. Che, S. H. Kessler, D. R. Worsnop and K. R. Wilson, Measurement of Fragmentation and Functionalization Pathways in the Heterogeneous Oxidation of Oxidized Organic Aerosol, Phys. Chem. Chem. Phys., 2009, 11(36), 8005–8014 RSC.
- M. A. Miracolo, A. A. Presto, A. T. Lambe, C. J. Hennigan, N. M. Donahue, J. H. Kroll, D. R. Worsnop and A. L. Robinson, Photo-Oxidation of Low-Volatility Organics Found in Motor Vehicle Emissions: Production and Chemical Evolution of Organic Aerosol Mass, Environ. Sci. Technol., 2010, 44(5), 1638–1643 CrossRef CAS PubMed.
- E. R. Trump and N. M. Donahue, Oligomer Formation within Secondary Organic Aerosols: Equilibrium and Dynamic Considerations, Atmos. Chem. Phys., 2014, 14(7), 3691–3701 CrossRef.
- Y. He, A. Akherati, T. Nah, N. L. Ng, L. A. Garofalo, D. K. Farmer, M. Shiraiwa, R. A. Zaveri, C. D. Cappa, J. R. Pierce and S. H. Jathar, Particle Size Distribution Dynamics Can Help Constrain the Phase State of Secondary Organic Aerosol, Environ. Sci. Technol., 2021, 55(3), 1466–1476 CrossRef CAS PubMed.
- J. E. Krechmer, D. A. Day and J. L. Jimenez, Always Lost but Never Forgotten: Gas-Phase Wall Losses Are Important in All Teflon Environmental Chambers, Environ. Sci. Technol., 2020, 54(20), 12890–12897 CrossRef CAS.
- P. H. McMurry and D. Grosjean, Gas and Aerosol Wall Losses in Teflon Film Smog Chambers, Environ. Sci. Technol., 1985, 19(12), 1176–1182 CrossRef CAS PubMed.
-
M. Z. Jacobson
Fundamentals of Atmospheric Modeling, Cambridge University Press, 2005 Search PubMed.
- R. A. Zaveri, R. C. Easter, J. D. Fast and L. K. Peters, Model for Simulating Aerosol Interactions and Chemistry (MOSAIC), J. Geophys. Res., 2008, 113(D13), 1591 CrossRef.
- P. S. Chhabra, N. L. Ng, M. R. Canagaratna, A. L. Corrigan, L. M. Russell, D. R. Worsnop, R. C. Flagan and J. H. Seinfeld, Elemental Composition and Oxidation of Chamber Organic Aerosol, Atmos. Chem. Phys., 2011, 11(17), 8827–8845 CrossRef CAS.
- R. J. Griffin, D. R. III Cocker, R. C. Flagan and J. H. Seinfeld, Organic Aerosol Formation from the Oxidation of Biogenic Hydrocarbons, J. Geophys. Res., 1999, 104(D3), 3555–3567 CrossRef CAS.
- A. A. Presto, K. E. H. Hartz and N. M. Donahue, Secondary Organic Aerosol Production from Terpene Ozonolysis. 2. Effect of NOx Concentration, Environ. Sci. Technol., 2005, 39(18), 7046–7054 CrossRef CAS.
- N. L. Ng, J. H. Kroll, M. D. Keywood, R. Bahreini, V. Varutbangkul, R. C. Flagan, J. H. Seinfeld, A. Lee and A. H. Goldstein, Contribution of First- versus Second-Generation Products to Secondary Organic Aerosols Formed in the Oxidation of Biogenic Hydrocarbons, Environ. Sci. Technol., 2006, 40(7), 2283–2297 CrossRef CAS PubMed.
- S. C. Farina, P. J. Adams and S. N. Pandis, Modeling Global Secondary Organic Aerosol Formation and Processing with the Volatility Basis Set: Implications for Anthropogenic Secondary Organic Aerosol, J. Geophys. Res., 2010, 115(D9) DOI:10.1029/2009JD013046.
- H. O. T. Pye, A. W. H. Chan, M. P. Barkley and J. H. Seinfeld, Global Modeling of Organic Aerosol: The Importance of Reactive Nitrogen (NOx and NO3), Atmos. Chem. Phys., 2010, 10(22), 11261–11276 CrossRef CAS.
- P. S. Kim, D. J. Jacob, J. A. Fisher, K. Travis, K. Yu, L. Zhu, R. M. Yantosca, M. P. Sulprizio, J. L. Jimenez, P. Campuzano-Jost, K. D. Froyd, J. Liao, J. W. Hair, M. A. Fenn, C. F. Butler, N. L. Wagner, T. D. Gordon, A. Welti, P. O. Wennberg, J. D. Crounse, J. M. St. Clair, A. P. Teng, D. B. Millet, J. P. Schwarz, M. Z. Markovic and A. E. Perring, Sources, Seasonality, and Trends of Southeast US Aerosol: An Integrated Analysis of Surface, Aircraft, and Satellite Observations with the GEOS-Chem Chemical Transport Model, Atmos. Chem. Phys., 2015, 15(18), 10411–10433 CrossRef CAS.
- C. L. Loza, P. S. Chhabra, L. D. Yee, J. S. Craven, R. C. Flagan and J. H. Seinfeld, Chemical Aging of m-Xylene Secondary Organic Aerosol: Laboratory Chamber Study, Atmos. Chem. Phys., 2012, 12(1), 151–167 CrossRef CAS.
- A. Akherati, Y. He, M. M. Coggon, A. R. Koss, A. L. Hodshire, K. Sekimoto, C. Warneke, J. de Gouw, L. Yee, J. H. Seinfeld, T. B. Onasch, S. C. Herndon, W. B. Knighton, C. D. Cappa, M. J. Kleeman, C. Y. Lim, J. H. Kroll, J. R. Pierce and S. H. Jathar, Oxygenated Aromatic Compounds Are Important Precursors of Secondary Organic Aerosol in Biomass-Burning Emissions, Environ. Sci. Technol., 2020, 54(14), 8568–8579 CrossRef CAS PubMed.
- A. Hodzic, P. S. Kasibhatla, D. S. Jo, C. D. Cappa, J. L. Jimenez, S. Madronich and R. J. Park, Rethinking the Global Secondary Organic Aerosol (SOA) Budget: Stronger Production, Faster Removal, Shorter Lifetime, Atmos. Chem. Phys., 2016, 16(12), 7917–7941 CrossRef CAS.
- Y. He, B. King, M. Pothier, L. Lewane, A. Akherati, J. Mattila, D. K. Farmer, R. L. McCormick, M. Thornton, J. R. Pierce, J. Volckens and S. H. Jathar, Secondary Organic Aerosol Formation from Evaporated Biofuels: Comparison to Gasoline and Correction for Vapor Wall Losses, Environ. Sci.: Processes Impacts, 2020, 22(7), 1461–1474 RSC.
- J. Kirkby, J. Duplissy, K. Sengupta, C. Frege, H. Gordon, C. Williamson, M. Heinritzi, M. Simon, C. Yan, J. Almeida, J. Tröstl, T. Nieminen, I. K. Ortega, R. Wagner, A. Adamov, A. Amorim, A.-K. Bernhammer, F. Bianchi, M. Breitenlechner, S. Brilke, X. Chen, J. Craven, A. Dias, S. Ehrhart, R. C. Flagan, A. Franchin, C. Fuchs, R. Guida, J. Hakala, C. R. Hoyle, T. Jokinen, H. Junninen, J. Kangasluoma, J. Kim, M. Krapf, A. Kürten, A. Laaksonen, K. Lehtipalo, V. Makhmutov, S. Mathot, U. Molteni, A. Onnela, O. Peräkylä, F. Piel, T. Petäjä, A. P. Praplan, K. Pringle, A. Rap, N. A. D. Richards, I. Riipinen, M. P. Rissanen, L. Rondo, N. Sarnela, S. Schobesberger, C. E. Scott, J. H. Seinfeld, M. Sipilä, G. Steiner, Y. Stozhkov, F. Stratmann, A. Tomé, A. Virtanen, A. L. Vogel, A. C. Wagner, P. E. Wagner, E. Weingartner, D. Wimmer, P. M. Winkler, P. Ye, X. Zhang, A. Hansel, J. Dommen, N. M. Donahue, D. R. Worsnop, U. Baltensperger, M. Kulmala, K. S. Carslaw and J. Curtius, Ion-Induced Nucleation of Pure Biogenic Particles, Nature, 2016, 533(7604), 521–526 CrossRef CAS PubMed.
- B. N. Murphy, N. M. Donahue, A. L. Robinson and S. N. Pandis, A Naming Convention for Atmospheric Organic Aerosol, Atmos. Chem. Phys., 2014, 14(11), 5825–5839 CrossRef.
-
I. K. Afreh, B. Aumont, M. Camredon and K. C. Barsanti, Using GECKO-A to Derive Mechanistic Understanding of SOA Formation from the Ubiquitous but Understudied Camphene, 2020, DOI: DOI:10.5194/acp-2020-829.
- Y. Morino, K. Sato, S. H. Jathar, K. Tanabe, S. Inomata, Y. Fujitani, S. Ramasamy and C. D. Cappa, Modeling the Effects of Dimerization and Bulk Diffusion on the Evaporative Behavior of Secondary Organic Aerosol Formed from α-Pinene and 1,3,5-Trimethylbenzene, ACS Earth Space Chem., 2020, 4(11), 1931–1946 CrossRef CAS.
- A. Lee, A. H. Goldstein, J. H. Kroll, N. L. Ng, V. Varutbangkul, R. C. Flagan and J. H. Seinfeld, Gas-Phase Products and Secondary Aerosol Yields from the Photooxidation of 16 Different Terpenes, J. Geophys. Res., 2006, 111(D17) DOI:10.1029/2006jd007050.
- H. Kim and S. E. Paulson, Real Refractive Indices and Volatility of Secondary Organic Aerosol Generated from Photooxidation and Ozonolysis of Limonene, α-Pinene and Toluene, Atmos. Chem. Phys., 2013, 13(15), 7711–7723 CrossRef.
- R. C. McVay, X. Zhang, B. Aumont, R. Valorso, M. Camredon, Y. S. La, P. O. Wennberg and J. H. Seinfeld, SOA Formation from the Photooxidation of α-Pinene: Systematic Exploration of the Simulation of Chamber Data, Atmos. Chem. Phys., 2016, 16(5), 2785–2802 CrossRef CAS.
- A. C. Aiken, P. F. Decarlo, J. H. Kroll, D. R. Worsnop, J. A. Huffman, K. S. Docherty, I. M. Ulbrich, C. Mohr, J. R. Kimmel, D. Sueper, Y. Sun, Q. Zhang, A. Trimborn, M. Northway, P. J. Ziemann, M. R. Canagaratna, T. B. Onasch, M. R. Alfarra, A. S. H. Prevot, J. Dommen, J. Duplissy, A. Metzger, U. Baltensperger and J. L. Jimenez, O/C and OM/OC Ratios of Primary, Secondary, and Ambient Organic Aerosols with High-Resolution Time-of-Flight Aerosol Mass Spectrometry, Environ. Sci. Technol., 2008, 42(12), 4478–4485 CrossRef CAS PubMed.
- P. S. Chhabra, R. C. Flagan and J. H. Seinfeld, Elemental Analysis of Chamber Organic Aerosol Using an Aerodyne High-Resolution Aerosol Mass Spectrometer, Atmos. Chem. Phys., 2010, 10(9), 4111–4131 CrossRef CAS.
- E. Järvinen, K. Ignatius, L. Nichman, T. B. Kristensen, C. Fuchs, C. R. Hoyle, N. Höppel, J. C. Corbin, J. Craven, J. Duplissy, S. Ehrhart, I. El Haddad, C. Frege, H. Gordon, T. Jokinen, P. Kallinger, J. Kirkby, A. Kiselev, K.-H. Naumann, T. Petäjä, T. Pinterich, A. S. H. Prevot, H. Saathoff, T. Schiebel, K. Sengupta, M. Simon, J. G. Slowik, J. Tröstl, A. Virtanen, P. Vochezer, S. Vogt, A. C. Wagner, R. Wagner, C. Williamson, P. M. Winkler, C. Yan, U. Baltensperger, N. M. Donahue, R. C. Flagan, M. Gallagher, A. Hansel, M. Kulmala, F. Stratmann, D. R. Worsnop, O. Möhler, T. Leisner and M. Schnaiter, Observation of Viscosity Transition in α-Pinene Secondary Organic Aerosol, Atmos. Chem. Phys., 2016, 16(7), 4423–4438 CrossRef.
- T. Nah, R. C. McVay, X. Zhang, C. M. Boyd, J. H. Seinfeld and N. L. Ng, Influence of Seed Aerosol Surface Area and Oxidation Rate on Vapor Wall Deposition and SOA Mass Yields: A Case Study with α-Pinene Ozonolysis, Atmos. Chem. Phys., 2016, 16(14), 9361–9379 CrossRef CAS.
- H. Kim, S. Liu, L. M. Russell and S. E. Paulson, Dependence of Real Refractive Indices on O : C, H : C and Mass Fragments of Secondary Organic Aerosol Generated from Ozonolysis and Photooxidation of Limonene and α-Pinene, Aerosol Sci. Technol., 2014, 48(5), 498–507 CrossRef CAS.
- K. J. Heaton, R. L. Sleighter, P. G. Hatcher, W. A. Hall, 4th and M. V. Johnston, Composition Domains in Monoterpene Secondary Organic Aerosol, Environ. Sci. Technol., 2009, 43(20), 7797–7802 CrossRef CAS PubMed.
- P. S. Chhabra, A. T. Lambe, M. R. Canagaratna, H. Stark, J. T. Jayne, T. B. Onasch, P. Davidovits, J. R. Kimmel and D. R. Worsnop, Application of High-Resolution Time-of-Flight Chemical Ionization Mass Spectrometry Measurements to Estimate Volatility Distributions of α-Pinene and Naphthalene Oxidation Products, Atmos. Meas. Tech., 2015, 8(1), 1–18 CrossRef CAS.
- K. Sato, Y. Fujitani, S. Inomata, Y. Morino, K. Tanabe, S. Ramasamy, T. Hikida, A. Shimono, A. Takami, A. Fushimi, Y. Kondo, T. Imamura, H. Tanimoto and S. Sugata, Studying Volatility from Composition, Dilution, and Heating Measurements of Secondary Organic Aerosols Formed during α-Pinene Ozonolysis, Atmos. Chem. Phys., 2018, 18(8), 5455–5466 CrossRef CAS.
- M. Kalberer, D. Paulsen, M. Sax, M. Steinbacher, J. Dommen, A. S. H. Prevot, R. Fisseha, E. Weingartner, V. Frankevich, R. Zenobi and U. Baltensperger, Identification of Polymers as Major Components of Atmospheric Organic Aerosols, Science, 2004, 303(5664), 1659–1662 CrossRef CAS PubMed.
- S. Gao, M. Keywood, N. L. Ng, J. Surratt, V. Varutbangkul, R. Bahreini, R. C. Flagan and J. H. Seinfeld, Low-Molecular-Weight and Oligomeric Components in Secondary Organic Aerosol from the Ozonolysis of Cycloalkenes and α-Pinene, J. Phys. Chem. A, 2004, 108(46), 10147–10164 CrossRef CAS.
- R. A. Zaveri, J. E. Shilling, A. Zelenyuk, M. A. Zawadowicz, K. Suski, S. China, D. M. Bell, D. Veghte and A. Laskin, Particle-Phase Diffusion Modulates Partitioning of Semivolatile Organic Compounds to Aged Secondary Organic Aerosol, Environ. Sci. Technol., 2020, 54(5), 2595–2605 CrossRef CAS PubMed.
- P. J. Ziemann and R. Atkinson, Kinetics, Products, and Mechanisms of Secondary Organic Aerosol Formation, Chem. Soc. Rev., 2012, 41(19), 6582–6605 RSC.
- P. Roldin, A. C. Eriksson, E. Z. Nordin, E. Hermansson, D. Mogensen, A. Rusanen, M. Boy, E. Swietlicki, B. Svenningsson, A. Zelenyuk and J. Pagels, Modelling Non-Equilibrium Secondary Organic Aerosol Formation and Evaporation with the Aerosol Dynamics, Gas- and Particle-Phase Chemistry Kinetic Multilayer Model ADCHAM, Atmos. Chem. Phys., 2014, 14(15), 7953–7993 CrossRef.
- R. A. Zaveri, J. E. Shilling, A. Zelenyuk, J. Liu, D. M. Bell, E. L. D'Ambro, C. J. Gaston, J. A. Thornton, A. Laskin, P. Lin, J. Wilson, R. C. Easter, J. Wang, A. K. Bertram, S. T. Martin, J. H. Seinfeld and D. R. Worsnop, Growth Kinetics and Size Distribution Dynamics of Viscous Secondary Organic Aerosol, Environ. Sci. Technol., 2018, 52(3), 1191–1199 CrossRef CAS PubMed.
- A. P. Bateman, A. K. Bertram and S. T. Martin, Hygroscopic Influence on the Semisolid-to-Liquid Transition of Secondary Organic Materials, J. Phys. Chem. A, 2015, 119(19), 4386–4395 CrossRef CAS PubMed.
- A. Pajunoja, A. T. Lambe, J. Hakala, N. Rastak, M. J. Cummings, J. F. Brogan, L. Hao, M. Paramonov, J. Hong, N. L. Prisle, J. Malila, S. Romakkaniemi, K. E. J. Lehtinen, A. Laaksonen, M. Kulmala, P. Massoli, T. B. Onasch, N. M. Donahue, I. Riipinen, P. Davidovits, D. R. Worsnop, T. Petäjä and A. Virtanen, Adsorptive Uptake of Water by Semisolid Secondary Organic Aerosols, Geophys. Res. Lett., 2015, 42(8), 3063–3068 CrossRef CAS.
- R. Schmedding, Q. Z. Rasool, Y. Zhang, H. O. T. Pye, H. Zhang, Y. Chen, J. D. Surratt, F. D. Lopez-Hilfiker, J. A. Thornton, A. H. Goldstein and W. Vizuete, Predicting Secondary Organic Aerosol Phase State and Viscosity and Its Effect on Multiphase Chemistry in a Regional-Scale Air Quality Model, Atmos. Chem. Phys., 2020, 20(12), 8201–8225 CrossRef CAS PubMed.
- Q. Lu, Y. Zhao and A. L. Robinson, Comprehensive Organic Emission Profiles for Gasoline, Diesel, and Gas-Turbine Engines Including Intermediate and Semi-Volatile Organic Compound Emissions, Atmos. Chem. Phys., 2018, 18(23), 17637–17654 CrossRef CAS.
- G. T. Drozd, Y. Zhao, G. Saliba, B. Frodin, C. Maddox, M.-C. Oliver Chang, H. Maldonado, S. Sardar, R. J. Weber, A. L. Robinson and A. H. Goldstein, Detailed Speciation of Intermediate Volatility and Semivolatile Organic Compound Emissions from Gasoline Vehicles: Effects of Cold-Starts and Implications for Secondary Organic Aerosol Formation, Environ. Sci. Technol., 2019, 53(3), 1706–1714 CrossRef CAS PubMed.
- C. N. Jen, L. E. Hatch, V. Selimovic, R. J. Yokelson, R. Weber, A. E. Fernandez, N. M. Kreisberg, K. C. Barsanti and A. H. Goldstein, Speciated and Total Emission Factors of Particulate Organics from Burning Western US Wildland Fuels and Their Dependence on Combustion Efficiency, Atmos. Chem. Phys., 2019, 19(2), 1013–1026 CrossRef CAS.
- M. Shiraiwa, A. Zuend, A. K. Bertram and J. H. Seinfeld, Gas-Particle Partitioning of Atmospheric Aerosols: Interplay of Physical State, Non-Ideal Mixing and Morphology, Phys. Chem. Chem. Phys., 2013, 15(27), 11441–11453 RSC.
- A. L. Hodshire, B. B. Palm, M. L. Alexander, Q. Bian, P. Campuzano-Jost, E. S. Cross, D. A. Day, S. S. de Sá, A. B. Guenther, A. Hansel, J. F. Hunter, W. Jud, T. Karl, S. Kim, J. H. Kroll, J.-H. Park, Z. Peng, R. Seco, J. N. Smith, J. L. Jimenez and J. R. Pierce, Constraining Nucleation, Condensation, and Chemistry in Oxidation Flow Reactors Using Size-Distribution Measurements and Aerosol Microphysical Modeling, Atmos. Chem. Phys., 2018, 28, 12433 CrossRef.
- J. Li, M. Cleveland, L. D. Ziemba, R. J. Griffin, K. C. Barsanti, J. F. Pankow and Q. Ying, Modeling Regional Secondary Organic Aerosol Using the Master Chemical Mechanism, Atmos. Environ., 2015, 102, 52–61 CrossRef CAS.
- M. Shrivastava, J. Fast, R. Easter, W. I. Jr Gustafson, R. A. Zaveri, J. L. Jimenez, P. Saide and A. Hodzic, Modeling Organic Aerosols in a Megacity: Comparison of Simple and Complex Representations of the Volatility Basis Set Approach, Atmos. Chem. Phys., 2011, 11(13), 6639–6662 CrossRef CAS.
- A. T. Lambe, P. S. Chhabra, T. B. Onasch, W. H. Brune, J. F. Hunter, J. H. Kroll, M. J. Cummings, J. F. Brogan, Y. Parmar, D. R. Worsnop, C. E. Kolb and P. Davidovits, Effect of Oxidant Concentration, Exposure Time, and Seed Particles on Secondary Organic Aerosol Chemical Composition and Yield, Atmos. Chem. Phys., 2015, 15(6), 3063–3075 CrossRef CAS.
- A. Kiendler-Scharr, J. Wildt, M. Dal Maso, T. Hohaus, E. Kleist, T. F. Mentel, R. Tillmann, R. Uerlings, U. Schurr and A. Wahner, New Particle Formation in Forests Inhibited by Isoprene Emissions, Nature, 2009, 461(7262), 381–384 CrossRef CAS PubMed.
- J. Kirkby, J. Curtius, J. Almeida, E. Dunne, J. Duplissy, S. Ehrhart, A. Franchin, S. Gagné, L. Ickes, A. Kürten, A. Kupc, A. Metzger, F. Riccobono, L. Rondo, S. Schobesberger, G. Tsagkogeorgas, D. Wimmer, A. Amorim, F. Bianchi, M. Breitenlechner, A. David, J. Dommen, A. Downard, M. Ehn, R. C. Flagan, S. Haider, A. Hansel, D. Hauser, W. Jud, H. Junninen, F. Kreissl, A. Kvashin, A. Laaksonen, K. Lehtipalo, J. Lima, E. R. Lovejoy, V. Makhmutov, S. Mathot, J. Mikkilä, P. Minginette, S. Mogo, T. Nieminen, A. Onnela, P. Pereira, T. Petäjä, R. Schnitzhofer, J. H. Seinfeld, M. Sipilä, Y. Stozhkov, F. Stratmann, A. Tomé, J. Vanhanen, Y. Viisanen, A. Vrtala, P. E. Wagner, H. Walther, E. Weingartner, H. Wex, P. M. Winkler, K. S. Carslaw, D. R. Worsnop, U. Baltensperger and M. Kulmala, Role of Sulphuric Acid, Ammonia and Galactic Cosmic Rays in Atmospheric Aerosol Nucleation, Nature, 2011, 476(7361), 429–433 CrossRef CAS PubMed.
- B. Friedman and D. K. Farmer, SOA and Gas Phase Organic Acid Yields from the Sequential Photooxidation of Seven Monoterpenes, Atmos. Environ., 2018, 187, 335–345 CrossRef CAS.
- Y. Zhao, A. T. Lambe, R. Saleh, G. Saliba and A. L. Robinson, Secondary Organic Aerosol Production from Gasoline Vehicle Exhaust: Effects of Engine Technology, Cold Start, and Emission Certification Standard, Environ. Sci. Technol., 2018, 52(3), 1253–1261 CrossRef CAS PubMed.
- A. M. Ortega, D. A. Day, M. J. Cubison, W. H. Brune, D. Bon, J. A. de Gouw and J. L. Jimenez, Secondary Organic Aerosol Formation and Primary Organic Aerosol Oxidation from Biomass-Burning Smoke in a Flow Reactor during FLAME-3, Atmos. Chem. Phys., 2013, 13(22), 11551–11571 CrossRef.
- D. S. Tkacik, A. T. Lambe, S. Jathar, X. Li, A. A. Presto, Y. Zhao, D. Blake, S. Meinardi, J. T. Jayne, P. L. Croteau and A. L. Robinson, Secondary Organic Aerosol Formation from in-Use Motor Vehicle Emissions Using a Potential Aerosol Mass Reactor, Environ. Sci. Technol., 2014, 48(19), 11235–11242 CrossRef CAS PubMed.
- B. B. Palm, P. Campuzano-Jost, A. M. Ortega, D. A. Day, L. Kaser, W. Jud, T. Karl, A. Hansel, J. F. Hunter, E. S. Cross, J. H. Kroll, Z. Peng, W. H. Brune and J. L. Jimenez, In Situ Secondary Organic Aerosol Formation from Ambient Pine Forest Air Using an Oxidation Flow Reactor, Atmos. Chem. Phys., 2016, 16(5), 2943–2970 CrossRef CAS.
- B. B. Palm, S. S. de Sá, D. A. Day, P. Campuzano-Jost, W. Hu, R. Seco, S. J. Sjostedt, J.-H. Park, A. B. Guenther, S. Kim, J. Brito, F. Wurm, P. Artaxo, R. Thalman, J. Wang, L. D. Yee, R. Wernis, G. Isaacman-VanWertz, A. H. Goldstein, Y. Liu, S. R. Springston, R. Souza, M. K. Newburn, M. L. Alexander, S. T. Martin and J. L. Jimenez, Secondary Organic Aerosol Formation from Ambient Air in an Oxidation Flow Reactor in Central Amazonia, Atmos. Chem. Phys., 2018, 18(1), 467–493 CrossRef CAS.
- B. Ervens, B. J. Turpin and R. J. Weber, Secondary Organic Aerosol Formation in Cloud Droplets and Aqueous Particles (aqSOA): A Review of Laboratory, Field and Model Studies, Atmos. Chem. Phys., 2011, 11(21), 11069–11102 CrossRef CAS.
- H. O. T. Pye, R. W. Pinder, I. R. Piletic, Y. Xie, S. L. Capps, Y.-H. Lin, J. D. Surratt, Z. Zhang, A. Gold, D. J. Luecken, W. T. Hutzell, M. Jaoui, J. H. Offenberg, T. E. Kleindienst, M. Lewandowski and E. O. Edney, Epoxide Pathways Improve Model Predictions of Isoprene Markers and Reveal Key Role of Acidity in Aerosol Formation, Environ. Sci. Technol., 2013, 47(19), 11056–11064 CrossRef CAS PubMed.
- C. Knote, A. Hodzic, J. L. Jimenez, R. Volkamer, J. J. Orlando, S. Baidar, J. Brioude, J. Fast, D. R. Gentner, A. H. Goldstein, P. L. Hayes, W. B. Knighton, H. Oetjen, A. Setyan, H. Stark, R. Thalman, G. Tyndall, R. Washenfelder, E. Waxman and Q. Zhang, Simulation of Semi-Explicit Mechanisms of SOA Formation from Glyoxal in Aerosol in a 3-D Model, Atmos. Chem. Phys., 2014, 14(12), 6213–6239 CrossRef.
- K. M. Henry and N. M. Donahue, Photochemical Aging of α-Pinene Secondary Organic Aerosol: Effects of OH Radical Sources and Photolysis, J. Phys. Chem. A, 2012, 116(24), 5932–5940 CrossRef CAS PubMed.
- M. A. Zawadowicz, B. H. Lee, M. Shrivastava, A. Zelenyuk, R. A. Zaveri, C. Flynn, J. A. Thornton and J. E. Shilling, Photolysis Controls Atmospheric Budgets of Biogenic Secondary Organic Aerosol, Environ. Sci. Technol., 2020, 54(7), 3861–3870 CrossRef CAS PubMed.
- S. A. Epstein, S. L. Blair and S. A. Nizkorodov, Direct Photolysis of α-Pinene Ozonolysis Secondary Organic Aerosol: Effect on Particle Mass and Peroxide Content, Environ. Sci. Technol., 2014, 48(19), 11251–11258 CrossRef CAS PubMed.
- M. Krapf, I. El Haddad, E. A. Bruns, U. Molteni, K. R. Daellenbach, A. S. H. Prévôt, U. Baltensperger and J. Dommen, Labile Peroxides in Secondary Organic Aerosol, Chem, 2016, 1(4), 603–616 CAS.
- M. A. Freedman, Phase Separation in Organic Aerosol, Chem. Soc. Rev., 2017, 46(24), 7694–7705 RSC.
- H. O. T. Pye, B. N. Murphy, L. Xu, N. L. Ng, A. G. Carlton, H. Guo, R. Weber, P. Vasilakos, K. W. Appel, S. H. Budisulistiorini, J. D. Surratt, A. Nenes, W. Hu, J. L. Jimenez, G. Isaacman-VanWertz, P. K. Misztal and A. H. Goldstein, On the Implications of Aerosol Liquid Water and Phase Separation for Organic Aerosol Mass, Atmos. Chem. Phys., 2017, 17(1), 343–369 CrossRef CAS PubMed.
- B. Ervens and R. Volkamer, Glyoxal Processing by Aerosol Multiphase Chemistry: Towards a Kinetic Modeling Framework of Secondary Organic Aerosol Formation in Aqueous Particles, Atmos. Chem. Phys., 2010, 10(17), 8219–8244 CrossRef CAS.
- V. F. McNeill, J. L. Woo, D. D. Kim, A. N. Schwier, N. J. Wannell, A. J. Sumner and J. M. Barakat, Aqueous-Phase Secondary Organic Aerosol and Organosulfate Formation in Atmospheric Aerosols: A Modeling Study, Environ. Sci. Technol., 2012, 46(15), 8075–8081 CrossRef CAS.
Footnote |
† Electronic supplementary information (ESI) available. See DOI: 10.1039/d1ea00014d |
|
This journal is © The Royal Society of Chemistry 2021 |