DOI:
10.1039/C8LC00829A
(Paper)
Lab Chip, 2019,
19, 410-421
Introducing an automated high content confocal imaging approach for Organs-on-Chips
Received
10th August 2018
, Accepted 5th December 2018
First published on 21st January 2019
Abstract
Organ-Chips are micro-engineered systems that aim to recapitulate the organ microenvironment. Implementation of Organ-Chips within the pharmaceutical industry aims to improve the probability of success of drugs reaching late stage clinical trial by generating models for drug discovery that are of human origin and have disease relevance. We are adopting the use of Organ-Chips for enhancing pre-clinical efficacy and toxicity evaluation and prediction. Whilst capturing cellular phenotype via imaging in response to drug exposure is a useful readout in these models, application has been limited due to difficulties in imaging the chips at scale. Here we created an end-to-end, automated workflow to capture and analyse confocal images of multicellular Organ-Chips to assess detailed cellular phenotype across large batches of chips. By automating this process, we not only reduced acquisition time, but we also minimised process variability and user bias. This enabled us to establish, for the first time, a framework of statistical best practice for Organ-Chip imaging, creating the capability of using Organ-Chips and imaging for routine testing in drug discovery applications that rely on quantitative image data for decision making. We tested our approach using benzbromarone, whose mechanism of toxicity has been linked to mitochondrial damage with subsequent induction of apoptosis and necrosis, and staurosporine, a tool inducer of apoptosis. We also applied this workflow to assess the hepatotoxic effect of an active AstraZeneca drug candidate illustrating its applicability in drug safety assessment beyond testing tool compounds. Finally, we have demonstrated that this approach could be adapted to Organ-Chips of different shapes and sizes through application to a Kidney-Chip.
Introduction
In the drug discovery process, the ability to accurately detect and predict both efficacy and safety relies on the availability of robust assay models that reproduce, as closely as possible, the effects at both a human volunteer and patient level. The relative simplicity of 2D cell culture models means that findings often do not translate into the clinical phases of drug development.1 Therefore, complex culture systems (including 3D and co-culture systems) are an area of intense interest because of their potential for improved translatability whilst retaining the control and ease of handling such systems.2 Organ-Chip systems, variously known as Organs-on-a-Chip, tissue chips, or microphysiological systems, aim to recapitulate the complex physiology and microenvironment of an in vivo organ through spatiotemporal control of tissue architecture and addition of fluid dynamics. The use of Organ-Chips that more accurately recapitulate organ and disease biology have the potential to impact all aspects of drug discovery from target validation, elucidation of mechanism of action, and compound efficacy, through to safety and pharmacokinetic profiling of drugs destined for the clinic.3,4 A key role for Organ-Chips will also be towards understanding on- and off-target species differences and how this translates to human physiology. However, there are challenges involved with extracting meaningful and robust data that must be overcome to enable full exploitation of these high-fidelity models. For example, the scale of these systems (approximately 35
000 to 70
000 cells) can in some cases hinder detection of analytes due to the small quantities produced and the small media volumes available for analysis. Imaging via microscopy offers an orthogonal approach for capturing cell phenotype in response to drug exposure. In addition, imaging data and the fact that Organ-Chips provide cellular and molecular level resolution of biology can enable new insights into biological processes and enable mechanistic insights, e.g. mechanism of action of drug for efficacy. However, routine application of imaging has been limited due to difficulties in the ability to image Organ-Chips reproducibly at scale.
Automated microscopes take advantage of the standard layout of multi-well plates to remove the need for manual control of the stage, allowing higher throughput acquisition on large numbers of plates.5 By contrast, the recent emergence of Organ-Chip systems means that a standard architecture has not yet emerged; indeed, multiple Organ-Chip architectures are presently used for various applications or modular combination of organs. Furthermore, due to the narrow width of channels on some Organ-Chips, fields of view (FOV) must be accurately placed to correctly capture the cells, meaning that field positions often need to be adjusted on a chip-by-chip basis to avoid introducing imaging biases. There are a wide range of options for on-the-fly microscope control based on feedback from acquired images, including the MetaXpress journal capability, the Wako Software Suite for Yokogawa systems, through to full microscope control using tools such as MicroManager.
Here, we describe the development of an intelligent scanning workflow to a Liver-Chip model. Microfluidic Liver-Chips have enormous potential as predictive models for drug-induced liver injury (DILI) which remains a major cause of drug attrition during drug discovery and development.6,7 We further developed a framework for the statistical analysis of Liver-Chips that not only reduces bias and variability but also ensures that the questions posed by the study can be robustly answered. Using benzbromarone, a compound associated with clinical hepatotoxicity, we determine the sample size needed to detect a hepatotoxic effect with an appropriate experimental power. Accurate sample size calculations minimise experimental cost since they prevent us from running studies that are inconclusive or use too many chips. This analysis further identifies different sources of variability and allows us to test for adverse effects while controlling for unwanted influences. We have now used this framework to design and prosecute a study to evaluate the hepatotoxic effects of an active AstraZeneca compound that is in clinical development.
Finally, to demonstrate broader application of this workflow to different chip formats, we conducted a preliminary investigation of a Kidney-Chip from a different manufacturer that is designed to generate a complete tubular structure with unidirectional perfusion. The Kidney-Chip differs from the Liver-Chip firstly in thickness and it also incorporates a glass window on the bottom face designed to facilitate imaging but its overall size was still compatible with our bespoke chip adaptors.
Materials and methods
Preparation of Liver-Chips
Liver-Chips, made from polydimethylsiloxane (PDMS) were obtained from Emulate Inc. (USA) and are illustrated in Fig. 1. The Liver-Chips contain 2 parallel channels that are separated by a porous PDMS membrane which is 50 μm thick and has a 7 μm pore diameter. The “top” channel of the Liver-Chip is where hepatocytes are seeded and measures 1 mm wide by 1 mm tall whereas the “bottom” channel is where the endothelial cells are seeded and measures 1 mm wide by 0.2 mm tall. The overlapping portion of the two channels measures 18 mm. Prior to cell seeding, both channels of the Liver-Chips were coated with a proprietary mixture of extracellular matrix prior to overnight incubation in a humidified chamber at 37 °C. Primary human cryopreserved hepatocytes (ThermoFisher), contained in Liver-Chip hepatocyte plating media (Williams E Media (WEM) containing phenol red, 1% penicillin/streptomycin, 1% L-glutamax, 0.1% fungin, 4 μg per mL insulin, 0.05 mg per mL ascorbic acid, 1 μM dexamethasone and 5% FBS) were seeded quickly using a pipette into the top channel of the Liver-Chip at a density of 3.5 × 106 cells per mL, taking care to ensure a homogenous cell distribution was achieved. Cells were left to form an attached monolayer at 37 °C in a humidified incubator with 95%/5% air/CO2. Twenty-four hours post seeding, the hepatocyte monolayer was overlaid with Matrigel Matrix (Corning, USA). Primary human cryopreserved liver sinusoidal endothelial cells (LSECs; cell systems) were seeded quickly into the bottom channel of the chip at a density of 2 to 4 × 106 cells per mL in endothelial medium (CSC medium containing 1% penicillin/streptomycin, 0.1% fungin, 2% culture boost and 10% FBS). Chips were inverted for 2 hours to allow attachment underneath the membrane, and then inverted back before being connected to a platform containing a pneumatic pump (Emulate). Media was flowed through both channels at a flow rate of 30 μL per hour and was refreshed every other day. Compound treatment started 4 days after commencement of flow (7 days and 5 days post seeding hepatocytes and LSECs to the Liver-Chips respectively).
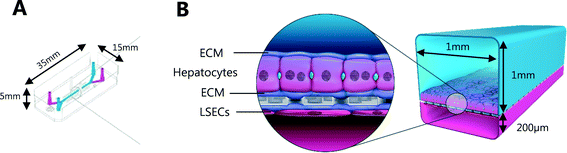 |
| Fig. 1 (A) Schematic of Liver-Chip illustrating dimensions; (B) each chip incorporates a hepatocyte and liver sinusoidal endothelial cell (LSEC) interface held within extracellular matrix (ECM). Incorporation of microfluidics allows physiological flow of media/drug treatment across the cell layers. | |
The effect of benzbromarone and staurosporine on cell phenotype was evaluated. Benzbromarone is a known hepatotoxicant8 while staurosporine is widely used to induce apoptosis.9 Both channels of the chips were perfused with benzbromarone (0 and 300 μM) for 24 hours or staurosporine (0 and 10 μM) for 6 hours at a flow rate of 30 μL per hour. Benzbromarone or staurosporine were prepared in hepatocyte dosing media (WEM without phenol red containing 1% penicillin/streptomycin, 1% L-glutamax; 0.1% fungin, 1% ITS + premix (human recombinant insulin (12.5 mg), human transferrin (12.5 mg), selenous acid (12.5 μg), bovine serum albumin (BSA) (2.5 g), linoleic acid (10.7 mg)), 0.05 mg mL−1 ascorbic acid, 0.1 μM dexamethasone and 2% FBS) when applied to the top hepatocyte channel or in LSEC dosing media (CSC medium containing 1% penicillin/streptomycin, 0.1% fungin, 2% culture boost and 2% FBS) when applied to the bottom LSEC channel. The concentration of both compounds was chosen to be towards the top of their concentration–response curve for toxicity. This was to ensure that a large, positive effect on cellular morphology could be detected confirming the applicability of the imaging approach. For benzbromarone, the reported IC50 in primary human hepatocytes over a 48 hour incubation was 170 μM8 whereas the apoptotic effect of staurosporine9 in murine hepatocytes was 10 μM over a 16 hour incubation. An AstraZeneca compound was also tested in the Liver-Chips. As above, both channels of the chips were perfused with this compound (0.1 μM, 1 μM or 10 μM) for 3 hours using hepatocyte or LSEC dosing media as appropriate. This protocol was chosen because it was analogous to the 3 hour intravenous administration of this compound when it was evaluated in vivo during preclinical development. A concentration–response relationship was also desired in this scenario to provide additional information on the sensitivity of detection of hepatotoxicity using the imaging approach. After a further 3 or 21 hours with compound-free media flow, chips were fixed with formaldehyde and stored in phosphate buffered saline (PBS) at 4 °C as described below.
Preparation of Kidney-Chips
Dual-channel chips, consisting of an inner PDMS body and plastic outer casing, were obtained from Nortis Inc (USA) and were prepared as previously described.10 Briefly, chips were filled with a freshly prepared solution of rat tail collagen I (Corning), at a concentration of 7 mg mL−1, to create the extracellular matrix, and left to polymerize overnight in a humidified incubator at 37 °C. Subsequently, the glass micro-pins embedded in the chip collagen matrix were removed to expose the two parallel micro-channels. The left channel was coated with human collagen IV (5 μg mL−1), 1 hour prior to cell injection. Primary human cryopreserved proximal tubule epithelial cells – RPTEC (Biopredic) were cultured according to supplier specifications. The RPTEC culture medium used was based in DMEM F-12 GlutaMAX (Invitrogen) containing 1% penicillin/streptomycin supplemented with 1% FBS, 10 ng mL−1 hEGF, 32 ng mL−1 hydrocortisone, 4 pg mL−1 triiodothyronine and ITS (insulin: 10 μg mL−1, transferrin: 10 μg mL−1, sodium selenite: 10 ng mL−1). Cell were harvested with trypsin-EDTA, flushed through a 100 μM cell strainer (Corning) and injected via the upstream injection port in the left channel of the chips, using a Hamilton 5 μL micro-syringe as a single cell suspension at a density of 1.0 × 106 cells per mL (5 μL per chip). Chips were kept in a humidified incubator with 95%/5% air/CO2 at 37 °C for 24 hours to allow cells to adhere to the collagen matrix. Each chip was connected to a Nortis perfusion platform (Nortis Inc), reservoirs were added and filled with 10 mL RPTEC culture medium, afterwards flow was initiated at a rate of 0.5 μL min−1. Chips were cultured for 7 days in a humidified incubator with 95%/5% air/CO2 at 37 °C and both medium and waste reservoirs levels were monitored daily to ensure adequate perfusion. Tube formation and morphology were monitored daily by visual inspection using a bench transmitted-light microscope.
Immunofluorescence
The Liver-Chips and Kidney-Chips were fixed with 4% formaldehyde for 15 and 30 minutes respectively, at room temperature before being washed and permeabilized (Triton X 0.1% with 2% BSA in PBS). Blocking and incubation with the primary antibody (overnight in PBS containing 2% BSA) was followed by 2 hours of incubation with fluorescently conjugated secondary antibodies (Invitrogen) also containing Phalloidin-488 (1
:
500, Thermofisher) and Hoechst 33342 (1
:
1000, Invitrogen) in the same buffer. Liver-Chip immunostaining was performed with primary antibodies specific for the beta subunit of the ATP synthase enzyme (ATPB; 1
:
100, Abcam, ab14730), Ki67 D3B5 (1
:
50, cell signalling 12075S) and cleaved caspase 3 (CC3) Asp175 (1
:
100, cell signalling, 9661S). An antibody specific for Zonula-occludens 1 (ZO1) (1
:
100, BD Biosciences) along with Phalloidin-488 (1
:
500) was used in the Kidney-Chips.
Design and printing of adaptors
Bespoke adaptors were designed, and 3D printed to allow compatibility with the plate based, automated confocal microscope (Fig. 2). Two chip holder designs were created to capture the different formats of the Liver-Chips and Kidney-Chips. Fig. 2A shows the production parts for the Liver-Chip, and Fig. 2B shows the design for the Kidney-Chip. All designs were made in house using SolidWorks 3D CAD and printed on a Stratasys OBJET30 Prime 3D printer. All parts were made using a Stratasys OBJET MED610 Biocompatible material (Part # OBJ-04057).
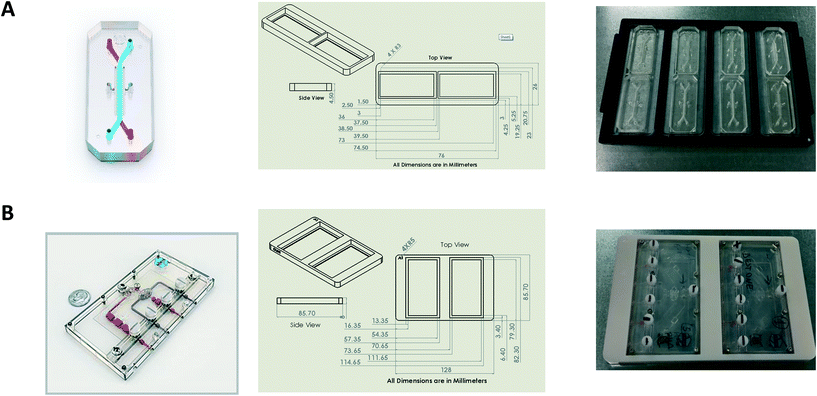 |
| Fig. 2 Design and 3D printing of adaptors allows compatibility with plate based, automated microscopes. (A) Left; schematic of Liver-Chip, middle; adaptor design, Right; Liver-Chips within adaptors to fit footprint of standard multi-well plate. (B) Left; schematic of Kidney-Chip, middle; adaptor design, right; Kidney-Chips within adaptors to fit footprint of standard multi-well plate. | |
Confocal image acquisition
Chips were imaged on a Cell Voyager 7000 (CV7000, Yokogawa Inc.). Confocal fluorescent images were captured using a long working distance 20× objective (Olympus LUCPLFLN 0.45 NA, WD 6.6–7.8 mm) and an Andor Neo sCMOS camera with a 2 × 2 bin. Hoechst was imaged using a 405 nm excitation laser (405 ± 5 nm, 100 mW, Coherent) with a 445/45 nm band pass emission filter. CC3 and ZO1 were imaged using a 561 nm excitation laser (561 ± 2 nm, 200 mW, Coherent) with a 600/37 nm band pass emission filter. Phalloidin was imaged using a 488 nm excitation laser (488 ± 2 nm, 200 mW, Coherent) with a 525/50 nm band pass emission filter and Ki67/ATPB imaged using a 640 nm excitation laser (640 +4/−5 nm, 100 mW, Coherent) with a 676/37 nm band pass emission filter.
For the Liver-Chips, images were captured over a 120 μm range at 5 μm Z intervals to cover both the LSECs and hepatocyte cell layers. For the Kidney-Chips, images were captured over a 120 μm range at 1 μm Z intervals. In the first pass scan, bright-field images were acquired using a 4× objective (Olympus UPLSAPO 0.16 NA, WD 13 mm) using a 100 W halogen lamp as an illumination source.
Image analysis
Intelligent scanning algorithm.
For control of the CV7000 automated confocal microscope, we used the SearchFirst functionality from the Wako Software Suite (Wako Automation), which defines a workflow where a first round of acquisition takes place, followed by launch of an analysis script, which calculates coordinates from the first-round images. The coordinates are then passed to the microscope for a second round of acquisition at higher magnification.
In the first pass, seven bright field images were captured for each Liver-Chip. To define the same FOV on every chip, we defined a common coordinate system, based on features which are present in each Liver-Chip (Fig. 3). The images were corrected for variation in illumination across each image, then stitched together to form one image covering the whole of the chip area. We then applied Hessian-based trough detection to enhance dark lines in the image, followed by a radon transform to detect straight lines in the images (Fig. 3A). The edges of the main channel were detected as two lines close to the vertical orientation and with the expected separation, defining the horizontal location of the main channel. The vacuum channels to either side of the main channel were detected as two lines perpendicular to the main channel, with the correct spacing. Together, these lines defined the standard coordinate system for the Liver-Chip. We choose coordinates equally spaced along the centre of the main channel, with a gap marginally larger than the size of the FOV. Points defined in these coordinates will lie at the same position on every chip.
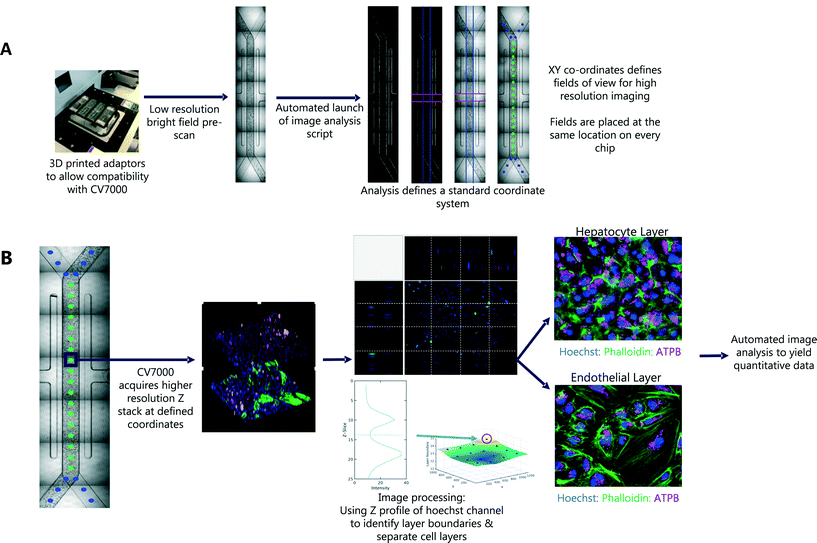 |
| Fig. 3 Imaging workflow and intelligent scanning strategy of Liver-Chip model. (A) Outline of first pass imaging workflow and intelligent scanning strategy. The CV7000 automated microscope first performs a low resolution (4×) bright field scan of all 8 chips within each holder. This is followed by the automatic launch of an analysis script that stitches the images together and defines XY co-ordinates for fields of view required for high resolution imaging. (B) Outline of second pass imaging. CV7000 microscope acquires a high resolution (20×) Z stack at the XY co-ordinates defined by first pass imaging. Image processing accurately separates out the cell layers ready for image analysis. | |
This general approach of identifying landmarks at low magnification can be applied to any chip architecture. We illustrated this by implementing intelligent scanning of Kidney-Chips from a different manufacturer. To generalise the approach for any Organ-Chip system, one needs to apply the following two steps. First, coordinates of fields of interest are placed manually on a reference image set. Second, for the intelligent scanning run, the first pass images were aligned to the reference image using a rigid registration algorithm, to determine where to place the field coordinates on the first pass images. To account for brightness and focus variations in the bright field images, both reference and test images were normalised, smoothed and edge filtered, before performing the registration.
Quantification of fluorescent images.
In the Liver-Chip, the fluorescent images were acquired as a single stack comprising the endothelial layer and hepatocyte layer together, separated by the membrane. First, the stack was separated into two layers by finding the minimum in the Hoechst channel along the z-direction. To account for variations in layer locations across the image was broken down into 4 × 4 sub regions, the height of the minimum was located in each region and then this height was interpolated over the whole image, giving a surface, which separated the two layers (Fig. 3B). For morphological measurements of hepatocytes, nuclei were segmented in 3 dimensions, and measures of cell number and organisation computed. For fluorescence measurements, a maximum projection was calculated for each layer, and cell regions were segmented using the phalloidin channel, starting from nuclear regions as seeds, to calculated single cell intensity values. Where appropriate, the fluorescence images were separated into distinct length scales of signal using morphological opening operations.
Statistical analysis
We have established a framework of statistical best practice for Organ-Chip studies to reduce bias and variability. We first used principles of optimal experimental design to randomise the order in which the chips are imaged. This distributed all experimental conditions equally across the different rows (within a chip holder) and between holders to reduce bias associated with chip location or imaging order.
We then analysed the relationship between the image analysis endpoints and treatment or time effects while controlling for the chip-to-chip variability, as well as row or holder effects. Specifically, we fitted a Bayesian multilevel linear regression model with uninformative priors, treating the fluorescence signal of the fields of view of a chip as repeated measurements. We estimated treatment and time effects as well as the variability induced by chip, row and holder. We report Bayes p-values, each representing the probability that the true effect is in the opposite direction than what we considered.11 Statistical significance was accepted with a Bayes p-value of less than 0.025. The analysis was implemented using the “brms” package for the R programming language.12
Modelling data from chips with the positive control benzbromarone, we were able to conduct a power analysis enabling us to identify the minimum sample size necessary to detect a given phenotypic effect. To do so, we simulated data from the fitted model (parametric bootstrap) where we varied the treatment effect relative to the positive control and the sample size. We used summary statistics on the simulations to assess the power of the statistical analysis.
Results
Here we set out to develop an end-to-end workflow that enables the automation of confocal microscopy of Organ-Chips to reduce user input and experimental bias, and increase throughput. Furthermore, we applied statistical modelling to create a framework that guides future experimental design. The improvements in throughput and reproducibility move towards the possibility of using Organ-Chips in a drug screening setting.
Imaging workflow of Liver-Chip model
An outline of the workflow is illustrated in Fig. 3. We first acquired low magnification bright field images, tiled to cover the whole chip. After the first pass, a script was automatically launched in MATLAB, which tiled the images and identified the location of 30 standard FOV corresponding to the same locations on each chip. These coordinates were used in the second pass of imaging, at higher magnification, multiple fluorescent channels and a 3D Z-stack to capture both cell layers of the Liver-Chip. The whole process is fully automated, requiring no user intervention other than to load the chips into the adapter. Each holder can contain up to 8 chips and the imaging acquisition process takes 70 minutes per holder but requires less than 5 minutes of user interaction time. As well as increasing the number of chips that can be imaged within a single study, this approach allowed more FOV to be acquired, increasing the amount of data collected from a single chip. Importantly, the FOV were defined to be in the same location on every chip, enabling standardisation of acquisition and statistical modelling of the sources of uncertainty in chip measurements, and potential spatial effects such as zonation, which are not systematically studied in Liver-Chip systems unless oxygen biosensors are incorporated.
Capturing cellular phenotype changes following drug exposure
Exemplar confocal fluorescent images from the higher resolution, second pass imaging step of both the hepatocyte and endothelial cell layers on the Liver-Chip are shown in Fig. 4. It is possible to obtain a clear separation of the cell layers with single cell resolution as well as signals for well validated stains of cellular morphology (via F-actin visualisation by phalloidin staining), proliferation (Ki67), apoptosis (CC3) and mitochondrial structure (ATP synthase beta subunit, ATPB).
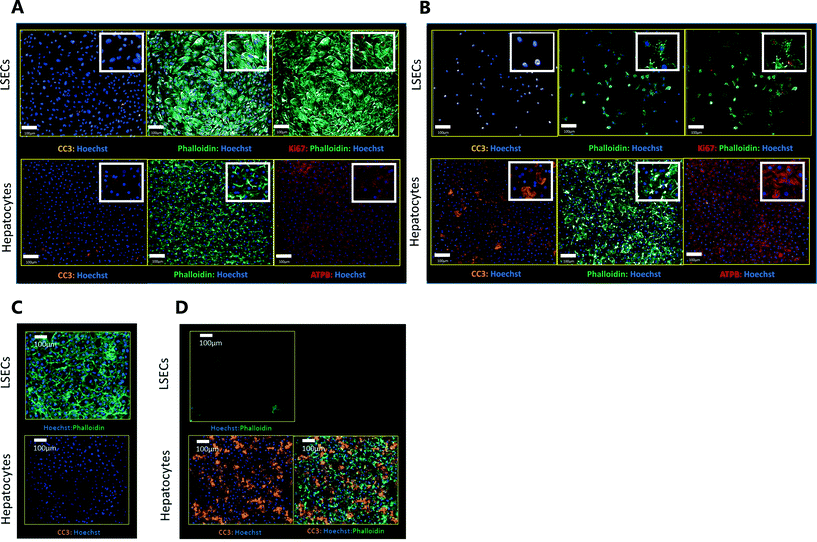 |
| Fig. 4 Exemplar confocal images taken at 20× magnification. All images are representative and taken from the mid region of each chip. Images shown include markers of F-actin morphology (Phalloidin), proliferation (Ki67), mitochondrial structure (ATPB – ATP synthase beta subunit), apoptosis (CC3 – cleaved caspase 3) and nuclei (Hoechst). (A and B) Images captured from a chip treated with (A) DMSO or (B) 300 μM benzbromarone for 24 hours. (C and D) Images captured from a chip treated with (C) DMSO or (D) staurosporine (10 μM) for 6 hours. | |
Benzbromarone has been reported to cause cytotoxicity to hepatocytes through a mitochondrial mechanism.13 We evaluated the effects of this drug on cell phenotype in the Liver-Chip system to validate the end-to-end workflow and to understand the interplay between the different imaging endpoints (Fig. 5). We treated 2 chips with benzbromarone (300 μM) and 3 chips with a corresponding DMSO control for 24 hours. We fitted a Bayesian multilevel linear regression model enabling us to adjust for chip-to-chip variability when estimating the treatment and time effects. For each imaging endpoint, we fitted a separate model using time, concentration, FOV, and chip ID as explanatory variables. Instead of using each FOV on its own, we estimated a linear field effect reflecting how the endpoint changed along the FOV within a chip, discussed in more detail below. Fig. 5 illustrates the difference in mean and variability between vehicle and treated chips for 6 endpoints of interest and shows the Bayes p values for testing for a treatment effect between vehicle and benzbromarone. We observed a significant treatment effect of benzbromarone on the resulting proliferation (Fig. 5B) and overall LSEC cell number (Fig. 5A). Because LSECs are highly proliferative, the increase in proliferation is most likely to be a repair mechanism as a consequence of the profound benzbromarone-induced cytotoxicity. Whilst, no significant effect of benzbromarone was observed on overall hepatocyte cell number (Fig. 5D), a significant effect was seen on hepatocyte apoptosis (Fig. 5C), changes in hepatocyte F-actin morphology (Fig. 5F) and mitochondria structure (Fig. 5E). The apparent increase in mitochondrial ATPB staining seen following benzbromarone treatment could either be the result of a compensatory mitochondrial upregulation, or perhaps more likely the result of mitochondrial swelling, caused by benzbromarone's reported mitochondrial permeability transition effect.14 Due to the resolution limitations of light microscopy this could not be confirmed. In addition, a key advantage of imaging is that it allows analysis of cells from different positions within each chip. Fig. 6 gives an example of this analysis where the changes in benzbromarone induced changes in hepatocyte ATPB stain was more significant at the chip inlet (zone 1) compared with the outlet (zone 3). However, the full significance of this finding would require further experimentation.
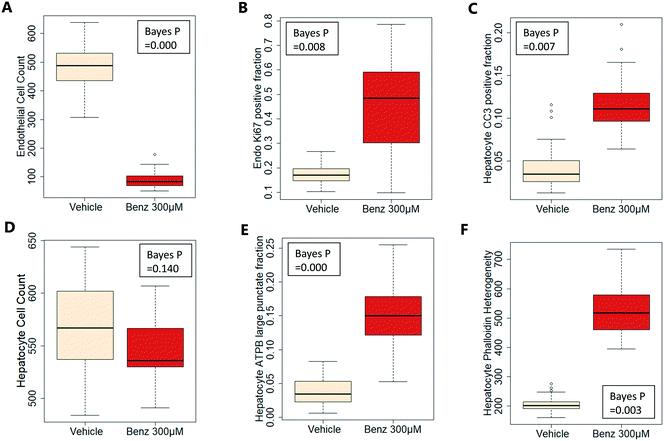 |
| Fig. 5 Effect of 300 μM benzbromarone on LSEC (A and B) and hepatocyte (C–F) cellular phenotypes. The boxplots illustrate the difference in mean and variability between vehicle and treated chips. A low Bayes p value indicates that there is a significant difference between vehicle and treated chips. We tested for cell count, proliferation (Ki67 positive fraction), apoptosis (CC3 positive fraction), mitochondrial changes (ATPB large punctate fraction), and F-actin morphology (Phalloidin heterogeneity). Data is based on 3 chips (vehicle) and 2 chips (300 μM benzbromarone), within each chip there are 18 fields of view. | |
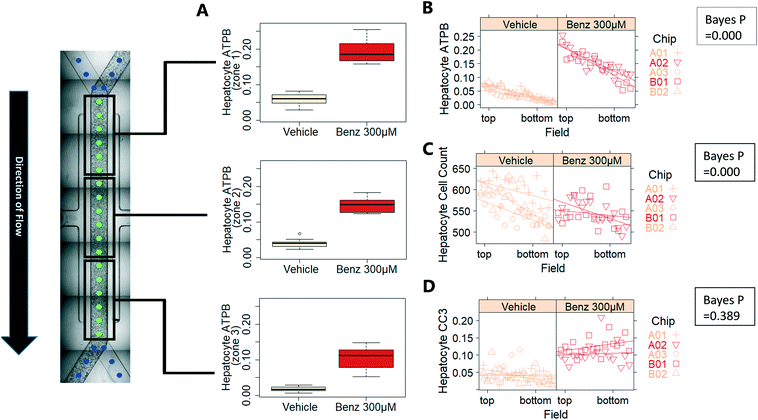 |
| Fig. 6 Measurement of field effect: imaging allows correlation of cell position within chip with phenotype. In this example, we observed a field effect for the ATPB endpoint in the hepatocyte layer (A and B). Similarly, there is a field effect for hepatocyte cell count (C). In contrast, no field effect was observed for hepatocyte CC3 positive fraction (D). | |
We also performed similar imaging techniques to Liver-Chips treated with staurosporine, a compound reported to induce apoptosis in hepatocytes through activation of caspsase-3.9 Staurosporine (10 μM) produced marked increases in the apoptotic marker (CC3) with little if any effect on hepatocyte cell number and complete loss of LSECs after a 6 hour exposure (Fig. 4C and D).
Statistical analysis: proof of concept and experimental design
To inform future experimental design, we performed a power analysis to compute the number of replicate chips required to ensure sufficient statistical power to detect a hepatotoxic effect comparable to benzbromarone. We computed a power analysis for each endpoint except for hepatocyte cell count (where no significant effect was observed). Table 1 shows the results of hepatocyte CC3 positive fraction, hepatocyte phalloidin heterogeneity and LSEC cell count endpoints which were representative for all the power analyses. Each table illustrates how many replicate chips are required to detect an effect equivalent to 50%, 80% or 100% of benzbromarone along with the % statistical power. For instance, to detect a hepatocyte CC3 response comparable to 80% of benzbromarone with a power of 80%, 3 replicate chips would be required. It is common practice to aim for at least 80% power, therefore this analysis concludes that for all endpoints of interest at least 3 replicate chips are required per condition to achieve sufficient statistical power to detect a phenotype comparable to 80% of the positive control.
Table 1 Use of power analysis to determine appropriate sample size within a study to achieve statistical significance when the effect is comparable to the positive control data. Three examples are given; (A) analysis based on CC3 (cleaved caspase 3) signal in hepatocytes in response to positive control (300 μM benzbromarone). (B) Analysis based on F-actin (phalloidin) heterogeneity in hepatocytes. (C) Analysis based on LSEC cell count
A Power analysis for hepatocyte CC3 positive fraction |
Treatment effecta [%] |
No. replicates [chips] |
Power [%] |
50 |
3 |
56 |
4 |
92 |
80 |
3 |
80 |
4 |
94 |
100 |
3 |
88 |
B Power analysis for hepatocyte phalloidin heterogeneity |
Treatment effecta [%] |
No. replicates [chips] |
Power [%] |
50 |
3 |
53 |
4 |
72 |
80 |
3 |
75 |
4 |
88 |
100 |
3 |
86 |
C Power analysis for LSEC cell count |
Treatment effecta [%] |
No. replicates [chips] |
Power [%] |
Relative to positive control.
|
50 |
2 |
91 |
3 |
96 |
80 |
2 |
97 |
3 |
99 |
100 |
2 |
100 |
Decoupling sources of variability
To demonstrate how to apply this workflow for the safety profiling of potential drugs, we analysed the hepatotoxic effect of an active AstraZeneca drug candidate currently in clinical development. We analysed 80 chips that contained hepatocytes from 2 different human donors at 3 time points (3 hours, 6 hours and 24 hours) and 4 concentrations (vehicle, 0.1 μM, 1 μM, 10 μM), with each group consisting of at least 3 replicate chips. As described above, we fitted a Bayesian multilevel linear regression model. Applying methods of model selection has shown that the donor-to-donor variability is negligible compared to the other sources of variabilities. To avoid over-fitting, we therefore excluded donor as a descriptor for the statistical model. The high number of chips imaged allowed us to decouple the different sources of variability, including chip, row and holder effects, by including hyperparameters describing the standard deviation associated with each error source. Accordingly, the fitting procedure automatically identified the chip-to-chip, row-to-row and holder-to-holder variability and allowed us to estimate the biological treatment effect after adjusting for these unwanted influences. Fig. 7 displays the posterior probability densities estimating the standard deviation corresponding to the different sources of variability as well as the treatment and field effects for two representative endpoints: LSEC cell count and hepatocyte CC3 positive fraction. Fig. 7A illustrates that the highest source of variability with respect to LSEC cell count comes from chip-to-chip variability, as the posterior density was high and comparably tight (in contrast, the holder-to-holder and row-to-row variability's posterior densities were wide spread and close to zero, indicating low holder-to-holder and row-to-row variability). This is an encouraging result, indicating that the holder and imaging system are not a significant source of noise in our system for this sort of measurement. In contrast, Fig. 7B illustrates that the highest source of variability with respect to hepatocyte CC3 response was the variability across holders, i.e. the order in which the chips are imaged. This highlights the importance for randomisation of the position in which the chips are placed in the holders and hence the order in which they are imaged. With the various sources of measurement error characterized, we proceeded to derive an adjusted estimate of the treatment effect at each concentration and timepoint. We concluded that the drug treatment showed no significant effect at any of the endpoints that were analysed.
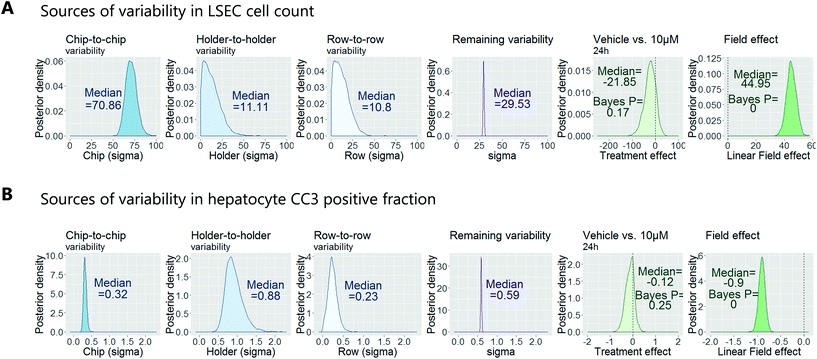 |
| Fig. 7 Bayesian analysis of a test compound to understand the variability in the data allowing the decoupling of the variability due to technical error (in blue) from experimental conditions (in green). Two examples are given; (A) analysis based on LSEC cell count. (B) Analysis based on CC3 (cleaved caspase 3) signal in hepatocytes. | |
Confirmation of approach using chips from a different manufacturer
Traditional in vitro renal cell cultures lack functionality and retain a poor epithelial phenotype.15 Organ-Chips have been designed to provide renal proximal tubule cells with a microenvironment that has continuous luminal flow promoting the self-assembly of a tight epithelium and recreating the characteristic barrier function.16 Imaging and reconstruction of a whole kidney tubule is a powerful tool to understand how nephrotoxicity develops over time, where the injury is manifest and impact on individual cells. The potential to multiplex high resolution imaging with quantitative analysis of metabolites and injury markers in Kidney-Chips can dramatically improve the reach of these systems in renal research and disease mechanism elucidation.
Using 3D printing we created bespoke adaptors to enable the automated confocal imaging of these Kidney-Chips (see Fig. 8). To improve the flexibility of the intelligent scanning algorithm, avoiding the need to design custom image processing for different chip designs, we manually placed FOV coordinates on a single reference image. First-pass scans are then registered to this reference image, aligning based on strong edges in the images, to determine where to place the fields for the second pass. By basing the algorithm on global features of the image, this approach improves the robustness of the field identification, because some elements can be missing or altered, and the alignment will still be successful. Fig. 8B shows the location of the fields identified for high resolution imaging in the second pass, overlaid on a stitched image of the 4 first pass bright field images. Slices from the high resolution second pass stacks are also displayed in Fig. 8D along with a 3D reconstruction of the proximal tubule (Fig. 8C).
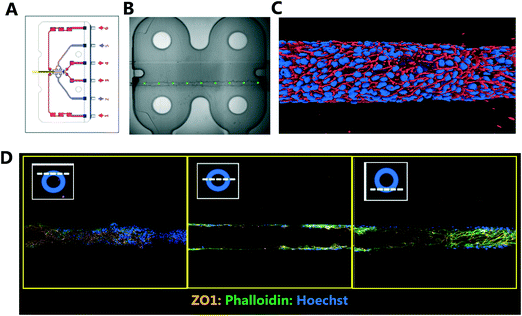 |
| Fig. 8 Automated confocal imaging of Kidney-Chips. (A) Schematic of Kidney-Chip. (B) Bright field image (4× magnification) from first pass acquisition with defined fields of view highlighted (green) for second pass imaging. (C) 3D render of proximal tube (rendered from 20× magnification Z stack images). (D) Exemplar confocal images taken from the mid region of a kidney tubule taken at 20× magnification. Cells have been stained with Phalloidin (green), Z01 (orange) and Hoechst (blue). | |
Discussion
This paper describes, to the best of our knowledge, the first setup of an end-to-end, automated workflow to capture and analyse confocal images of a multicellular Organ-Chip model. This process allows detailed cellular phenotypes (such as morphology, proliferation, apoptosis, and mitochondrial structure) to be captured across large batches of chips. By automating this process, we have reduced acquisition and user interaction time. Prior to this, 3D confocal imaging of the Liver-Chips in a manual fashion (i.e. manually locating and defining FOV) took more than 2 hours per chip. Whilst we may not require the capacity for high throughput screening, a single study to evaluate hepatoxicity of a test compound in a dose dependent manner, across multiple donors typically requires analysis of upwards of 50 chips. Even at this scale, manual acquisition of images would take weeks and could also suffer from variability.
We have also built a framework of statistical best practice for Organ-Chip studies. We first applied principles of optimal experimental design to randomise chip imaging location and order. This removed all bias associated with the imaging workflow. We then used a Bayesian analysis to understand the variability in the data and could decouple variability due to technical error from experimental conditions. Finally, we ran a power analysis to identify the minimum sample size necessary to detect a given effect size. This best practice standard guides the experimental design and analysis of all our Organ-Chip studies at AstraZeneca and its application is important in order to extract robust data from these complex model systems.
The Liver-Chips described in this manuscript are designed to recapitulate in vivo liver function. The chips display physiologically relevant levels of albumin and urea secretion as well as metabolic competency for at least 14 days.17 Although biomarkers of cellular injury in response to drug exposure can be measured, we have demonstrated here that high-content imaging offers a complimentary approach of capturing cell phenotype changes in response to a hepatotoxic compound, thereby enhancing the application of these systems for mechanistic studies. Image analysis confirmed the benzbromarone-induced hepatotoxicity, with a statistically significant reduction in endothelial cell count and increase in hepatocyte actin heterogeneity, indicative of altered cellular morphology, and the staurosporine-induced apoptosis with marked increases in CC3 levels. We have described morphology, proliferation and apoptosis endpoints as examples, but with the rapid advancement of sophisticated image analysis algorithms and use of machine learning, the potential phenotypes that could be analysed can extend to any available antibodies and markers. Moreover, this approach is not limited by the small number of cells within a chip. By imaging in 3 dimensions we can separate the cell layers, and read cellular phenotype at the single cell level allowing quantification of heterogeneity, for example in the phalloidin intensity, and an understanding of phenotype which would not be apparent in population averaged measures.
The benzbromarone induced mitochondrial perturbations (as identified by altered ATPB staining) were greater at the chip inlet. The possible reasons underlying this are predominantly due to exposure levels of benzbromarone or its metabolites. The hepatocytes at the inlet of the chip will be exposed to higher concentrations of parent compound. However, due to the high concentration of benzbromarone, constant flow and number of hepatocytes in the chip, parent compound exposure is a likely differentiating factor when comparing the response of a hepatocyte at the inlet versus the outlet. To understand this further the differential mechanisms of toxicity of benzbromarone would need to be evaluated. Parent benzbromarone can affect mitochondrial function in its own right;18 however, it undergoes metabolism to reactive metabolites (quinones and epoxides), which have been reported to also affect mitochondrial function.14,19,20 Finally, benzbromarone is also a potent inhibitor of Bile salt efflux pump (BSEP) and causes downregulation of BSEP expression21 as well as multidrug resistance-associated protein (MRP2) expression.22 There is potential for benzbromarone to inhibit bile acid secretion and these bile acids could also contribute to the ATPB response. Imaging using fluorescent bile acids would shed further insights into the mechanism of toxicity of benzbromarone.
In summary, we have established an end-to-end, automated workflow to acquire and analyse confocal images of multicellular Organ-Chips of different formats to probe cellular phenotype across large batches of chips. By automating this process, we reduce acquisition time, process variability and user bias. Taken together, this has enabled us to establish a unique framework of statistical best practice for Organ-Chip imaging that will enhance the data we can obtain from these model systems going forward and enhance their utility and applications. We believe that by establishing the best practice framework we can contribute to the growing platform of evidence that shows these micro-engineered systems accurately recapitulate in vivo functionality. In addition, the framework can play a role in the development of methodology guidelines that assess the reproducibility, robustness and clinical translatability of Organ-Chips.
Author contributions
Samantha Peel: conceptualization, data curation and writing-original draft.
Adam M Corrigan, Beate Ehrhardt: conceptualization, formal analysis, software and writing-original draft.
Kyung-Jin Jang, Pedro Pinto, Jonathan Rubins, Konstantia Kodella, Debora B. Petrpopolis, Janey Ronxhi, Gauri Kulkarni, Alison J. Foster: data curation and investigation.
Matt Boeckeler: software.
Dominic Williams: writing-original draft.
Geraldine A. Hamilton: writing-original draft.
Lorna Ewart: supervision, conceptualization, and writing-original draft.
Abbreviations
ATPB | Beta subunit of ATP synthase enzyme ATPB |
BSA | Bovine serum albumin |
BSEP | Bile salt export pump |
CC3 | Cleaved caspase 3 |
EGF | Epidermal growth factor |
FOV | Fields of view |
LSEC | Liver sinusoidal endothelial cells |
MRP2 | Multidrug resistance-associated protein |
PBS | Phosphate buffered saline |
ZO1 | Zonula-occludens 1 |
Conflicts of interest
There are no conflicts to declare.
Acknowledgements
The authors would like to acknowledge Beverley Isherwood and James Pilling for their contribution to establishing automated, high content confocal microscopy within AstraZeneca, and Johan Karlsson for helpful discussions on intelligent scanning. We would also like to acknowledge Nortis Inc for support in setting up the Kidney-Chip platform and specifically Carl Hulit, Christopher Smoke and Henning Mann for valuable scientific advice and discussion on optimising the Kidney-Chip cultures. Finally, we would like to thank Daniel Levner for his critical review of this manuscript.
References
- P. Horvath,
et al., Screening out irrelevant cell-based models of disease, Nat. Rev. Drug Discovery, 2016, 15(11), 751–769 CrossRef CAS PubMed.
- S. N. Bhatia and D. E. Ingber, Microfluidic organs-on-chips, Nat. Biotechnol., 2014, 32(8), 760–772 CrossRef CAS PubMed.
- L. Ewart,
et al., Application of Microphysiological Systems to Enhance Safety Assessment in Drug Discovery, Annu. Rev. Pharmacol. Toxicol., 2018, 58, 65–82 CrossRef CAS PubMed.
- E. W. Esch, A. Bahinski and D. Huh, Organs-on-chips at the frontiers of drug discovery, Nat. Rev. Drug Discovery, 2015, 14(4), 248–260 CrossRef CAS PubMed.
- M. Boutros, F. Heigwer and C. Laufer, Microscopy-Based High-Content Screening, Cell, 2015, 163(6), 1314–1325 CrossRef CAS PubMed.
- P. B. Watkins, Drug safety sciences and the bottleneck in drug development, Clin. Pharmacol. Ther., 2011, 89(6), 788–790 CrossRef PubMed.
- D. Cook,
et al., Lessons learned from the fate of AstraZeneca's drug pipeline: a five-dimensional framework, Nat. Rev. Drug Discovery, 2014, 13(6), 419–431 CrossRef CAS PubMed.
- W. R. Proctor,
et al., Utility of spherical human liver microtissues for prediction of clinical drug-induced liver injury, Arch. Toxicol., 2017, 91(8), 2849–2863 CrossRef CAS PubMed.
- G. Feng and N. Kaplowitz, Mechanism of staurosporine-induced apoptosis in murine hepatocytes, Am. J. Physiol., 2002, 282(5), G825–G834 CAS.
- K. P. Van Ness,
et al., Microphysiological Systems to Assess Nonclinical Toxicity, Curr. Protoc. Toxicol., 2017, 73, 14181–141828 CrossRef PubMed.
- A. Gelman and F. Tuerlinckx, Type S error rates for classical and Bayesian single and multiple comparison procedures, Comput. Stat., 2000, 15(3), 373–390 CrossRef.
- P.-C. Bürkner, brms: An R Package for Bayesian Multilevel Models Using Stan, J. Stat. Softw., 2017, 80(1), 1–28 Search PubMed.
- P. Kaufmann,
et al., Mechanisms of benzarone and benzbromarone-induced hepatic toxicity, Hepatology, 2005, 41(4), 925–935 CrossRef CAS PubMed.
- M. Shirakawa,
et al., Metabolic activation of hepatotoxic drug (benzbromarone) induced mitochondrial membrane permeability transition, Toxicol. Appl. Pharmacol., 2015, 288(1), 12–18 CrossRef CAS PubMed.
- S. E. Jenkinson,
et al., The limitations of renal epithelial cell line HK-2 as a model of drug transporter expression and function in the proximal tubule, Pflugers Arch, 2012, 464(6), 601–611 CrossRef CAS PubMed.
- E. J. Weber,
et al., Development of a microphysiological model of human kidney proximal tubule function, Kidney Int., 2016, 90(3), 627–637 CrossRef PubMed.
-
K. Jang, L. Ewart, M. Otieno, K. Kodella, J. Ronxhi, D. Petropolis, A. Srivastava, L. Andersson, K. Maratea, I. Barrett, M. Clausen, S. Lazic, D. Williams, M. Singer, J. Rubins, G. Kulkarni, B. Jones, D. Simic, J. Silva, S. Dallas, P. Guzzie-Peck, K. Karalis, D. Ingber and G. Hamilton, Liver-Chip Model for Determination of Species Differences and Risk Assessment of Hepatotoxicity in Humans, Annual Meeting Abstract Supplement Society of Toxicology, 2018, vol. 1, p. 1360 Search PubMed.
- A. Felser,
et al., Hepatocellular toxicity of benzbromarone: effects on mitochondrial function and structure, Toxicology, 2014, 324, 136–146 CrossRef CAS PubMed.
- M. G. McDonald and A. E. Rettie, Sequential metabolism and bioactivation of the hepatotoxin benzbromarone: formation of glutathione adducts from a catechol intermediate, Chem. Res. Toxicol., 2007, 20(12), 1833–1842 Search PubMed.
- K. Wang,
et al., Identification of Epoxide-Derived Metabolite(s) of Benzbromarone, Drug Metab. Dispos., 2016, 44(4), 607–615 CrossRef PubMed.
- B. Garzel,
et al., The role of bile salt export pump gene repression in drug-induced cholestatic liver toxicity, Drug Metab. Dispos., 2014, 42(3), 318–322 CrossRef PubMed.
- K. Jemnitz,
et al., Biliary efflux transporters involved in the clearance of rosuvastatin in sandwich culture of primary rat hepatocytes, Toxicol. In Vitro, 2010, 24(2), 605–610 CrossRef CAS PubMed.
Footnotes |
† Authors contributed equally to this manuscript. |
‡ Current address: Institute for Mathematical Innovation, University of Bath, Bath, UK. |
|
This journal is © The Royal Society of Chemistry 2019 |