DOI:
10.1039/C6AN00200E
(Paper)
Analyst, 2016,
141, 3648-3656
Single-cell mass spectrometry with multi-solvent extraction identifies metabolic differences between left and right blastomeres in the 8-cell frog (Xenopus) embryo†
Received
26th January 2016
, Accepted 17th March 2016
First published on 17th March 2016
Abstract
Single-cell metabolic mass spectrometry enables the discovery (untargeted) analysis of small molecules in individual cells. Using single-cell capillary electrophoresis high-resolution mass spectrometry (CE-HRMS), we recently uncovered small-molecule differences between embryonic cells located along the animal–vegetal and dorsal–ventral axes of the 16-cell frog (Xenopus laevis) embryo, raising the question whether metabolic cell heterogeneity also exists along the left–right body axis. To address this question, we here advance single-cell CE-HRMS for identifying and quantifying metabolites in higher analytical sensitivity, and then use the methodology to compare metabolite production between left and right cells. Our strategy utilizes multiple solvents with complementary physicochemical properties to extract small molecules from single cells and improve electrophoretic separation, increasing metabolite ion signals for quantification and tandem HRMS. As a result, we were able to identify 55 different small molecules in D1 cells that were isolated from 8-cell embryos. To quantify metabolite production between left and right cells, we analyzed n = 24 different D1 cells in technical duplicate–triplicate measurements. Statistical and multivariate analysis based on 80 of the most repeatedly quantified compounds revealed 10 distinct metabolites that were significantly differentially accumulated in the left or right cells (p < 0.05 and fold change ≥1.5). These metabolites were enriched in the arginine–proline metabolic pathway in the right, but not the left D1 cells. Besides providing analytical benefits for single-cell HRMS, this work provides new metabolic data on the establishment of normal body asymmetry in the early developing embryo.
Introduction
Chemical analysis is central to understanding how molecular processes coordinate normal development such as breakage of the body symmetry in the early developing embryo.1,2 Studies in many different animals, based on gene-by-gene manipulations and more recent RNA-sequencing approaches, have identified differentially inherited or distributed molecules that form signaling centers, which then specify the formation of the animal–vegetal and dorsal–ventral axes of the developing embryo.2–8 However, despite significant research efforts, the molecular determinants of the left–right body axis are yet to be fully understood. In many animals, left–right asymmetry is influenced by directed flow of the Nodal signaling factor across the midline of the gastrula, but there also is evidence for earlier asymmetry in cytoskeletal elements, ion pumps and channels, and signaling molecules.9–13 By extending high-resolution mass spectrometry (HRMS) to single blastomeres, we recently quantified asymmetrical distribution for proteins14 and small-molecules (metabolites)15 along the animal–vegetal and dorsal–ventral body axes. Because metabolites are dynamically influenced by transcriptional and translational events,16 the discovery analysis of the metabolome raises a powerful tool to further investigate the initial molecular foundations of left–right patterning.
Metabolic analysis with single-cell resolution is enabled by several analytical techniques (see recent reviews in ref. 16–20). Single-cell mass spectrometry (MS) provides label-free detection, qualitative–quantitative information, and capability for targeted or untargeted (discovery) operation. Single-cell MS technologies include but are not limited to secondary ion MS (SIMS) for lipids in mating bacteria21 and single Xenopus embryos,22 matrix-assisted23–25 and matrix-free26,27 laser desorption ionization for endogenous metabolites and drugs in animal, plant, or microbial cells and cell cultures. Additionally, single-cell MS can be performed under atmospheric-pressure conditions. For example, lipid and fatty acid changes were monitored in bovine,28 porcine,29 and mouse30 oocytes and preimplantation embryos by desorption electrospray ionization as well as Xenopus eggs by laser ablation electrospray ionization.31 Laser desorption ionization32 and ablation33,34 found cellular heterogeneity and subcellular gradients, and direct-microsampling electrospray ionization (ESI)35–37 helped to track drug metabolism in live cells. Other single-cell MS strategies incorporate a separation step to reduce chemical complexity and matrix effects prior to ionization and detection of small molecules. For example, peptides were characterized in neurons using microsampling with liquid chromatography,38 metabolites from stimulated single heart cells were electrophoretically separated in lab-on-a-chip devices,39 and capillary electrophoresis (CE) with ESI-HRMS was used to detect metabolites in isolated molluskan and mammalian single neurons.40–42 We recently adapted CE-ESI-HRMS to single Xenopus blastomeres and quantified dorsal–ventral and animal–vegetal metabolic cell heterogeneity in the 16-cell embryo.15
Here we further the sensitivity of the single-cell CE-ESI-HRMS workflow and explore cell-to-cell differences along the left–right axis in the early developing Xenopus embryo. We use solvent systems with complementary physicochemical properties to enhance small-molecule extraction from blastomeres and to improve their separation by CE. The workflow allowed us to quantify ∼80 and identify ∼55 different metabolites in single D1 blastomeres that were isolated from the 8-cell Xenopus embryo. Next, multi-solvent extraction was used to quantify metabolic differences between left and right D1 cells in n = 3–5 biological replicates with each extract analyzed in technical duplicate–triplicate. The CE-ESI-HRMS metadata were evaluated using multivariate and statistical data analysis tools, revealing significant small-molecule differences between the left and right D1 cells. Metabolic pathway enrichment analysis identified the differentially accumulated metabolites to be represented in the arginine–proline pathway in the right, but not the left D1 blastomeres. Multi-solvent extraction presents analytical benefits for metabolomics by single-cell MS, which in turn fosters the understanding of basic biochemical processes underlying cell or organism development.
Experimental
Chemicals
Formic acid, acetic acid, methanol, acetonitrile, and water were LC-MS-grade, from Fisher Scientific (Fair Lawn, NJ). Ammonium hydroxide and acetylcholine were from Acros Organics (Fair Lawn, NJ). Eagle's minimum essential medium (Sigma Aldrich; St Louis, MO) was used as a mixture of the standard L-amino acids: Ala, Arg, Asn, Asp, Glu, Gln, Gly, His, Ile, Leu, Lys, Phe, Pro, Ser, Tyr, and Val. All solvents were LC-MS grade, and all chemical standards were reagent grade or higher.
Solutions
Fresh Steinberg's solution (100%) was prepared as previously described.15 Two-fold dilution of this solution yielded 50% Steinberg's solution. Metabolite extraction solutions spanned a range of organic content (polar to apolar) and pH to promote the extraction of complementary types of small molecules. The “polarpH4” solution was 50% (v/v) methanol prepared with 0.5% (v/v) acetic acid, yielding pH 3.89. The “apolarpH5” solution contained 40% (v/v) acetonitrile and 40% (v/v) methanol, yielding pH 4.70. The “apolarpH8” solution contained 40% (v/v) acetonitrile and 40% (v/v) methanol with pH titrated to 8.30 using ammonium hydroxide.
Animals and cell isolation
Adult male and female Xenopus laevis frogs were purchased from Nasco (Fort Atkinson, WI), and housed in a breeding colony at GWU (IACUC #A311). Fertilized 8-cell embryos were obtained by gonadotropin-induced egg laying and in vitro fertilization. The jelly coats of the embryos were removed as previously described.43 Dejellied embryos were selected at the 2-cell stage if the lightly pigmented animal hemisphere was bisected by the first cleavage furrow in order to accurately predict the dorsal–ventral axis.44 These embryos were transferred into a Petri dish containing 100% Steinberg's solution at room temperature and their cleavage patterns monitored with a stereomicroscope. Upon reaching the 8-cell stage, typically ∼2.25 h post-fertilization, those in which left and right D1 blastomeres could be identified based on pigmentation and location in reference to established cell-fate maps45 were transferred to 50% Steinberg's solution in an agarose-coated Petri dish. The left and right D1 blastomeres were dissected free using sharpened forceps following an earlier protocol.46 Each cell type was collected at n = 5 biological replicates. Each isolated blastomere was labeled with a unique cell identifier to aid interpretation of results.
Single-cell extracts
Each isolated blastomere was directly transferred into a distinct 0.6 mL Eppendorf microvial (Fisher) containing 20 μL of methanol chilled to ∼4 °C to quench15,41,47–49 enzymatic reactions. Subsequently, the methanol solution containing the blastomere was dried in a vacuum concentrator (Labconco; Kansas City, MO) at 4 °C. The sample was reconstituted in 5 μL of metabolite extraction solution, sonicated in an ice-cold water bath for 3 min, and vortexed for 1 min. Metabolite extracts were centrifuged at 8000g at 4 °C (Sorvall Legend X1R; Thermo Scientific, Waltham, MA) for 3 min and stored at −80 °C until measurement by CE-ESI-MS.
Single-cell CE-ESI-HRMS
Extracts were thawed, vortex-mixed, and centrifuged at 8000g at 4 °C to pellet cell debris before measurement using a CE-ESI-MS platform that we recently developed for small molecules in single Xenopus blastomeres.15 Briefly, the instrument consists of a custom-built CE platform capable of injecting ∼1–20 nL from ∼1 μL extract and separating small molecules, a co-axial sheath flow CE-ESI interface without nebulizer gas to ionize molecules, and a quadrupole orthogonal acceleration time-of-flight (q-oaTOF) high-resolution tandem mass spectrometer to mass-analyze ions (Impact HD, Bruker Daltonics, Billerca, MA). The mass spectrometer was tuned and mass-calibrated to <1 ppm accuracy following vendor instructions and operated at 40
000 FWHM resolution. Experimental parameters for CE were as follows: injection volume, 10 nL; CE fused silica dimensions, 40/105 μm internal/outer diameter × 90 cm length; background electrolyte, 1% (v/v) formic acid; electrophoretic separation voltage, 17–23 kV (applied to the injection end). ESI parameters included: electrospray solvent, 50% methanol containing 0.1% (v/v) formic acid; spray flow rate, 1 μL min−1; spray voltage, −1700 V (applied to the orifice plate of the mass spectrometer); electrospray regime, cone jet (controlled as previously described49). Mass spectrometer settings for single-stage and data-dependent acquisition (DDA) were: mass range for survey (MS1) and MS2, m/z 50–500; survey scan rate, 2 Hz; MS2 scan rate, 2 Hz; collision-induced dissociation energy/gas, 18 eV/nitrogen; fragmentation on 3 most-intense features; active exclusion, after 3 spectra for 60 s; smart exclusion, activated with 5×. Quality control and operation of the platform followed our recent protocol to enable 60 amol lower limit of detection and 3–5 log-order dynamic range of quantification.15 Instrumental repeatability was characterized daily using 50 nM acetylcholine. Over 10 days of continuous data collection, quantitative repeatability was 11% relative standard deviation (RSD) in migration time and 17% RSD in peak area for quantification. We required a minimum repeatability of <25% RSD in peak area and separation time before measuring cell extracts. The separation capillary was flushed with BGE for 5 min at the end of each separation experiment followed by a 2 min blank (BGE injected) analysis to test CE-ESI-MS signal stability before injection of the next sample.
Data analysis and software
Raw MS data files were processed using custom-written scripts in Compass Data Analysis ver. 4.0 (Bruker Daltonics) according to our previous protocol.49 Briefly, each raw file was externally mass-calibrated to <1 ppm accuracy (HPC calibration mode in data analysis) with sodium-formate clusters that formed in the CE-ESI ion source as sodium ions separated from the extracts, and molecular features (unique m/z vs. time domains) were manually searched between m/z 50–500 with a 500 mDa incremental step. To note the molecular feature, the accurate mass (m/z value) and the migration time were noted for the apex of each feature within its elution window. The resulting metadata were analyzed in MetaboAnalyst 3.0,50 a public web-based metabolomic pipeline, with the following settings: type of normalization, sum (to signal abundance); type of scaling, auto. Fisher's least significant difference analysis (LSD) was adopted post hoc. Statistical analysis utilized Student's two-tailed t-test (homoscedastic) with p < 0.05 chosen to mark significance for normally distributed data. A fold change of ≥1.5 was chosen to denote biological significance. Octanol–water distribution coefficients were calculated in MarvinSketch 16.1.11 (2016 ChemAxon, Budapest, Hungary). Ion fragmentation pathways during collision-induced dissociation were predicted in Mass Frontier 7.0 (Thermo Scientific) to aid molecular identifications.
Safety considerations
Standard safety procedures apply for handling chemicals. Capillaries, which present wounding hazard, must be handled with care using safety glasses. As high voltage poses electrical shock hazard, electrically conductive parts of the CE-ESI interface must be grounded or shielded to prevent accidental exposure; in this work, the CE system was housed in a Plexiglass enclosure with an interlock-enabled door controlling the CE high-voltage power supply as an active safety mechanism.
Results and discussion
Three-solvent small-molecule extraction for single cells
Our first goal was to enhance the detectable coverage of the metabolome for single blastomeres in the Xenopus embryo. In traditional metabolomic experiments, detection and identification of metabolites are enhanced by modulating compound distribution between the extraction solvent and the specimen.51,52 For example, amino acids are efficiently extracted using methanol-based solvent systems, and extraction performance can be improved by multiple extraction steps in sequence or fine adjustments to the solvent composition.53–55 Similarly, our accumulated experience showed 50% (v/v) methanol containing 0.5% (v/v) acetic acid to be capable of extracting an appreciable number of polar small molecules from single neurons41,49 and embryonic cells.15 Here we proposed that the utilization of multiple extraction solvents deepens the metabolic coverage in single-cell measurements using CE-ESI-HRMS.
This strategy was tested by evaluating metabolite extraction using different solvent systems. In addition to extraction by methanol, the study included apolar conditions to help lyse the cell membrane and facilitate the detection of apolar compounds. To guide the selection of pH for the extraction solvents, we calculated the octanol/water distribution coefficient (D) between pH 2–10 for ∼20 randomly selected small molecules that were detected in our previous single-cell studies.15 As shown in Fig. S1,† the coefficients were highly pH-dependent for several metabolites. For example, D varied by 3-log-orders for arginine, lysine, methionine, aspartic acid, and glutamic acid between pH 4 and 9. This information supported the notion that extraction may be enhanced by solvents with complementary polar–apolar characteristics and pH. For this study, we selected 50% methanol containing 0.5% acetic acid with pH 3.80 (denoted as “polarpH4”) as it performed well for polar compounds in our earlier work.15 To help extract apolar metabolites, we increased the apolar characteristic of this solvent formulation to 40% acetonitrile and 40% methanol with pH titrated to 4.70 (“apolarpH5”) and pH 8.30 (“apolarpH8”). These three solvents were anticipated to extract polar and apolar compounds under basic and acidic conditions with complementary performance.
Next, the solvent systems were applied to single blastomeres. Single midline animal–dorsal (D1) cells were identified in the right hemisphere of 8-cell Xenopus embryos (Fig. 1), and these cells (D1R) were microdissected following established cell-fate maps and isolation protocols.45,46 The separated cells were immediately transferred into a microvial containing chilled methanol (4 °C) to rapidly quench enzyme activity. While cells appeared physically intact in the solvent, exposure to methanol likely caused extraction for some compounds (e.g., polar metabolites). To control extraction across different solvent polarity–pH domains, contents of the microvial (cell and methanol) were lyophilized at 4 °C, causing methanol-extracted metabolites to precipitate on the surfaces of the cells and the vial. Ultimately, metabolites were extracted by adding polarpH4, apolarpH5, or apolarpH8 solvents to the contents of each microvial (cell and precipitated metabolites), followed by sonication in ice-cold water to lyse the cells and facilitate extraction. A total of n = 3–4 different D1R blastomeres (biological replicates) were measured for each solvent to account for technical and biological variability and empower statistical data analysis. The resulting extracts were centrifuged to pellet cell debris and precipitated proteins. A 10 nL portion of the aliquot was analyzed using our custom-built single-cell CE-ESI-HRMS platform (Fig. 1).
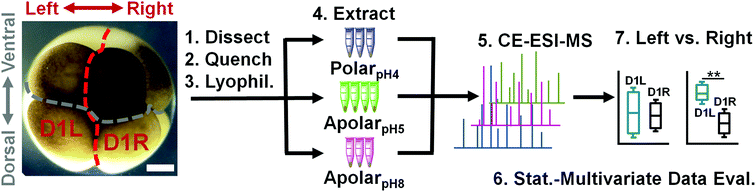 |
| Fig. 1 Experimental strategy to identify and quantify small molecules in D1 blastomeres in the 8-cell Xenopus embryo. Different D1 blastomeres were extracted using solvents with complementary physicochemical properties to enhance the detectable portion of the single-cell metabolome and assess metabolic differences between blastomeres on the left and right sides of the embryo. Scale bar = 200 μm. | |
The metabolic compositions of the resulting extracts were qualitatively compared. The primary mass spectrometric data were manually surveyed to identify molecular features, defined here as distinct accurate mass (m/z value) vs. separation time domains. The resulting molecular features were manually revised to disregard background signals from the solvents (e.g., common contaminants in ESI-HRMS) as well as isotopes, adducts, or noncovalent clusters. As a result, 156–232 different molecular features were detected using the multiple solvents, 25–35% of which were exclusive to each solvent (Fig. 2A) and 92 were detected in at least 3 biological replicates (see list in Table S1†). We identified 55 different molecular features by comparing their accurate mass, migration time, and fragmentation behavior via collision-induced dissociation using mass spectrometric databases (e.g., Metlin,56 Human Metabolome Database,57 and mzCloud), computational prediction (Mass Frontier), chemical standards, as well as our in house-built CE-ESI-MS/MS2 metabolomic database for Xenopus laevis.15 Identified metabolites are tabulated in Table S2† and include amino acids, energy carriers, bases, small organic acids, osmolites, and dipeptides. These metabolite identifications correspond to a ∼40% enhancement by multi-solvent extraction compared to our previous single-solvent approach.15
 |
| Fig. 2 Enhancing small-molecule detection from single blastomeres using multi-solvent extraction. (A) The polarpH4, apolarpH5, and apolarpH8 solvents allowed us to detect a complementary set of small molecules in the cells. Numbers indicate different compounds. (B) These solvents also provided complementary performance in electrophoretic separation; 28 select small molecules are shown. Higher signal-to-noise ratio and separation power was achieved using apolarpH5, and apolarpH8 solvents. Theoretical plate numbers (N) are provided for arginine. Key: CR, creatine; GSH, glutathione; GSSG, oxidized glutathione; HPX, hypoxanthine; S-adenosylmethionine ( ); ornithine ( ); lysine ( ). Identified compounds are listed in Table S2.† | |
Performance was quantified on the basis of CE separation power and signal-to-noise (S/N) ratio. The single-cell analysis workflow benefited from high separation power to simplify the complex metabolome for detection, provide separation time as compound-dependent information to help metabolite identification, and minimized spectral interferences to aid quantification. Representative separation of compounds is shown in Fig. 2B. Based on the separation of arginine, creatine, glutamic acid, and GSH, apolarpH5 produced ∼4-times higher S/N than the counterparts, and apolar solvents yielded 16–37% higher theoretical plate numbers. We attribute these enhancements in CE to on-column sample preconcentration by field-amplified sample stacking. This resulted from lower electrical conductivity in the acetonitrile-containing solvents and a dynamic pH-junction58 at the interfacing of the acidic background electrolyte (∼pH 3) with the sample prepared in basic apolarpH8.
The cell extracts were compared using multivariate and statistical tools. Selected-ion electropherograms were generated for the 80 most repeatedly quantified small molecules (Table S1†) between the blastomeres and their under-the-curve peak areas were integrated to serve as a quantitative proxy for metabolite abundance. The median quantitative error for these 80 features based on technical duplicates was ∼12% relative standard deviation (RSD) for the polarpH4, ∼18% RSD for the apolarpH5, and 23% RSD for the apolarpH8 extracts, providing sufficient repeatability to interpret biological significance with a fold change ≥1.5. To account for naturally variable cell sizes between embryos, we normalized the metadata to total signal abundance (see also Experimental); indeed, the normalized areas followed a normal distribution and were centered at zero (0) counts (data not shown). These normalized metadata were evaluated using analysis of variance (ANOVA) and hierarchical cluster analysis (HCA). Fig. 3 presents the HCA-heatmap calculated for the 70 most statistically significant molecular features of the metadata. Differential clustering between the molecular features and extraction conditions (see dendrogram branches) highlight groups of small molecules that were differentially extracted by the respective solvents (see grey squares). Fisher's least significant difference (LSD) analysis found about a dozen of these differences to be statistically significant (see Table S3†). Complementary metabolite extraction raised the potential to enhance the quantification of the single-cell metabolome.
 |
| Fig. 3 HCA-heat map identifying differential metabolite extraction from single D1R blastomeres using the 3 solvents. The plot was calculated for the 70 most statistically significant differences. Individual blastomeres with unique cell identifiers (Cell ID) are shown on the horizontal axis (see bottom axis). Different molecular features are shown on the vertical axis (see right axis). Dashed boxes exemplify quantitative chemical differences between the extracts. Metabolites with statistically significant differences in abundance based on Fisher's LSD are labeled (see also Table S3†). Metabolites are shown in three-letter codes. Key: Cit, citrulline; GB, glycine betaine. Molecular features that have yet to be identified are not shown (see all labels in Fig. S2†). | |
Assessing metabolic differences between left–right D1 blastomeres
Last, we asked whether blastomeres harbor different metabolomes on the left versus right side of the Xenopus embryo (see Fig. 1). This question is of importance because there are conflicting reports regarding left–right asymmetries in ion and neurotransmitter flow at cleavage stages.9,12,14 We selected the D1 blastomere in the 8-cell embryo for study because: (i) it already exhibits left–right asymmetry in H+/K+-ATPase alpha subunit mRNA and protein;59,60 (ii) blastomeres in the 8-cell embryo are considerably large, ∼180 nL in volume, facilitating manual cell dissection; (iii) D1 blastomeres are precursors to organs that show laterality in the tadpole (e.g., heart and hindgut61). To enhance the success of finding metabolic differences between the blastomeres, we minimized biological variability by using only embryos derived from a single set of parents, thus ensuring a common genetic background. We manually dissected left D1 (D1L) and right D1 (D1R) blastomeres and extracted each with the polarpH4, apolarpH5, or apolarpH8 solvents. To enhance the power of statistical data analysis, n = 3–5 different blastomeres (biological replicates) were processed using each solvent for the left or the right D1 cells, amounting to a total of 24 different blastomeres collected. With each blastomere measured in 1–3 technical replicates, a total of 36 different single-cell CE-ESI-HRMS measurements were performed in this portion of the study.
Small-molecule composition was compared between D1L and D1R blastomeres. The peak areas were determined for the 80 most repeatedly extracted compounds (Table S1†). The data were normalized (see Experimental) to account for potential differences in cell sizes, and these metadata on left vs. right cell content were analyzed for statistical significance (Student's t-test) and biological importance (fold change, FC, between D1L/D1R). This correlation is presented as a volcano plot in Fig. 4. Most small molecules were comparably produced in the D1L and D1R blastomeres, indicating that the main metabolic activities of the left and right blastomeres are similar in the 8-cell Xenopus embryo. Other metabolites had significantly different abundances between the left and right blastomeres. With complementary performance in separation, sensitivity, and quantitative repeatability, the different extraction solvents were able to uncover metabolic differences between cells to varying extent of statistical and biological significance (p value/FC). For example, significantly different amounts of GABA were extracted from D1L and D1R using the apolar (<0.05/≥1.5) but not the polarpH4 (0.585/1.4) solvent. Trolamine enrichment was revealed in D1L based on polarpH4 extracts, which was also captured to a biological, but not statistical significance during apolar extraction (0.07/2.41 for apolarpH5 and 0.257/1.72 for apolarpH8). Likewise, leucine accumulation in D1L was evidenced based on apolarpH5 to a biological significance in apolarpH8 (0.055/1.59), but not polarpH4 (0.626/1.271). Combined, metabolites that were differentially enriched included leucine, isoleucine, ethanolamine, GABA, and trolamine with higher abundance in D1L, and creatine, acetylcarnitine, spermidine, S-adenosylmethionine, and putrescine with higher abundance in D1R (Table 1). Combined, these findings suggest slight but detectable asymmetry in metabolic activity along the left–right axis in the 8-cell Xenopus embryo.
 |
| Fig. 4 Differential metabolite enrichment between D1 blastomeres on the left (D1L) and right (D1R) sides of the 8-cell Xenopus embryo. (A) Volcano plot compares signal differences between D1L/D1R cell extracts prepared using the three solvents. Dashed lines (grey) mark thresholds for statistical significance (p < 0.05) and biological importance (fold change ≥1.5). (B) Relative comparison of differentially enriched, identified metabolites (see Table 1 and all data in Table S4†). | |
Table 1 Quantification of metabolite differences between left and right D1 blastomeres of the 8-cell Xenopus embryo
Metabolite |
Left/right fold changea |
p value |
Extraction system |
Negative fold change values indicate down-production and were calculated by inverting ratios lower than 1.
|
Spermidine |
−28.88 |
0.0007 |
ApolarpH5 |
Creatine |
−2.58 |
0.0012 |
ApolarpH5 |
Putrescine |
−2.05 |
0.0171 |
ApolarpH5 |
Acetylcarnitine |
−1.85 |
0.0021 |
ApolarpH5 |
SAM |
−1.66 |
0.0006 |
PolarpH4 |
Ethanolamine |
1.52 |
0.0098 |
ApolarpH5 |
Trolamine |
1.99 |
0.0008 |
PolarpH4 |
Isoleucine |
2.08 |
0.0232 |
ApolarpH5 |
Leucine |
2.41 |
0.0296 |
ApolarpH5 |
GABA |
2.97; 3.10 |
0.0124; 0.0072 |
ApolarpH8; apolarpH5 |
These differentially enriched metabolites were mapped against known metabolic pathways. Using MetaboAnalyst as the search engine and Danio rerio (zebrafish) as the model, the 10 differentially quantified and identified metabolites were compared to the Kyoto Encyclopedia of Genes and Genomes (KEGG) metabolomic database. As shown in Fig. 5, the analysis suggested enrichment for arginine–proline metabolism with high statistical significance (p = 1.69 × 10−4) and pathway impact (0.154) and glutathione metabolism with moderate statistical significance (p = 0.015) and low pathway impact (0.022). Differentially accumulated metabolites were enriched in the arginine–proline pathway in D1R, but not in the D1L blastomeres.
 |
| Fig. 5 Pathway analysis for metabolites with asymmetric distribution between left and right D1 blastomeres. Correlation between p value from pathway enrichment analysis and pathway impact from pathway topology analysis in MetaboAnalyst identified arginine–proline as the most represented pathway (left panel). Identified metabolites underlying this pathway were mapped against KEGG using Danio rerio (zebrafish) as the model organism (right panel). Key: numbers correspond to KEGG metabolite identifiers. | |
Conclusions
In the presented work, we addressed the analytical sensitivity of discovery single-cell HRMS measurements to ask a cell biological question, whether blastomeres have different metabolomes along the left–right body axis in the early developing embryo. We enhanced the detectable coverage of the single-cell metabolome by designing solvent systems with complementary physicochemical properties (polarity and pH). Multi-solvent extraction also facilitated metabolite detection during single-cell CE-ESI-HRMS. On-column enrichment led to higher signal-to-noise ratios, which in turn improved small-molecule identifications and quantification. Complementary analytical performance by multiple extraction solvents enabled the detection of statistically and biologically significant enrichment differences for 10 different metabolites between D1L and D1R blastomeres, which would have been limited to fewer metabolites during a classical approach based on single-solvent extraction. Comparison with KEGG metabolic database found these metabolites to be enriched in the arginine–proline pathway in D1R, but not in D1L blastomeres, suggesting altered metabolism between the cells. Overall, these data captured asymmetry in the metabolic activity of D1 blastomeres on the left–right sides of the 8-cell Xenopus embryo.
Although the biological significance of the observed metabolic activity differences between left–right D1 blastomeres is unknown to us at present, these results demonstrate the potential of HRMS to aid cell and developmental biology studies. Continuing advances in cell sampling and treatment (e.g., sampling by microcapillaries35–37,42,62) enable the analysis of progressively smaller cells. To offset lower signal-to-noise ratios resulting from smaller materials measured in these studies, new technologies in sample handling, ionization, and HRMS detection sensitivity are required. Advancing tandem and multistage (MSn) high-resolution mass spectrometric databases and related search engines, such as Metlin,56 Human Metabolome Database,57 and mzCloud (based on precursor ion fingerprinting63 and fragmentation trees64), are essential to improving the confidence of metabolite identifications. Equally important is the measurement of a higher number of single cells to empower statistical analysis. By enabling the assaying of a large number of small molecules with deeper coverage of the metabolome, HRMS measurements of single cells provide new molecular insights into cell-to-cell differences that may not be detectable in classical cell-population averaging experiments, raising the ability to help better design hypothesis-driven studies for health research.
Author contributions
P. N., R. M. O., and S. A. M. designed the research; S. A. M. isolated the Xenopus blastomeres; R. M. O., S. E. M., and P. N. analyzed the cells; P. N. and R. M. O. interpreted the data and prepared the manuscript.
Acknowledgements
This research was supported by the National Institutes of Health Grant R21 GM114854 (to P. N.), The George Washington University Department of Chemistry Start-Up Funds (to P. N.), and the COSMOS Club Foundation award (to R. M. O).
References
- M. J. Sutherland and S. M. Ware, Am. J. Med. Genet., Part C, 2009, 151, 307–317 CrossRef PubMed
.
- M. Blum, A. Schweickert, P. Vick, C. V. E. Wright and M. V. Danilchik, Dev. Biol., 2014, 393, 109–123 CrossRef CAS PubMed
.
- E. H. Davidson, Development, 1990, 108, 365–389 CAS
.
- M. L. King, T. J. Messitt and K. L. Mowry, Biol. Cell, 2005, 97, 19–33 CrossRef CAS PubMed
.
- J. Heasman, Development, 2006, 133, 1205–1217 CrossRef CAS PubMed
.
- E. W. Abrams and M. C. Mullins, Curr. Opin. Genet. Dev., 2009, 19, 396–403 CrossRef CAS PubMed
.
- E. De Domenico, N. D. L. Owens, I. M. Grant, R. Gomes-Faria and M. J. Gilchrist, Dev. Biol., 2015, 408, 252–268 CrossRef CAS PubMed
.
- T. N. Cuykendall and D. W. Houston, Dev. Dyn., 2010, 239, 1838–1848 CrossRef CAS PubMed
.
- L. N. Vandenberg, J. M. Lemire and M. Levin, Commun. Integr. Biol., 2013, 6, e27155 CrossRef PubMed
.
- E. K. O. Namigai, N. J. Kenny and S. M. Shimeld, Genesis, 2014, 52, 458–470 CrossRef CAS PubMed
.
- M. Tingler, T. Ott, J. Tozser, S. Kurz, M. Getwan, M. Tisler, A. Schweickert and M. Blum, Genesis, 2014, 52, 588–599 CrossRef CAS PubMed
.
- J. B. Coutelis, N. Gonzalez-Morales, C. Geminard and S. Noselli, EMBO Rep., 2014, 15, 926–937 CrossRef CAS PubMed
.
- T. Fukumoto, I. P. Kema and M. Levin, Curr. Biol., 2005, 15, 794–803 CrossRef CAS PubMed
.
- C. Lombard-Banek, S. A. Moody and P. Nemes, Angew. Chem., Int. Ed., 2016, 55, 2454–2458 CrossRef CAS PubMed
.
- R. M. Onjiko, S. A. Moody and P. Nemes, Proc. Natl. Acad. Sci. U. S. A., 2015, 112, 6545–6550 CrossRef CAS PubMed
.
- R. Zenobi, Science, 2013, 342, 1243259 CrossRef CAS PubMed
.
- R. Trouillon, M. K. Passarelli, J. Wang, M. E. Kurczy and A. G. Ewing, Anal. Chem., 2013, 85, 522–542 CrossRef CAS PubMed
.
- S. S. Rubakhin, E. V. Romanova, P. Nemes and J. V. Sweedler, Nat. Methods, 2011, 8, S20–S29 CrossRef CAS PubMed
.
-
L. M. Borland, S. Kottegoda, K. S. Phillips and N. L. Allbritton, in Annu Rev Anal Chem (Palo Alto Calif), Palo Alto, 2008, vol. 1, pp. 191–227, annual reviews Search PubMed
.
- P. Dittrich and A. J. Ibanez, Electrophoresis, 2015, 36, 2196–2206 CrossRef CAS PubMed
.
- M. E. Kurczy, P. D. Piehowski, C. T. Van Bell, M. L. Heien, N. Winograd and A. G. Ewing, Proc. Natl. Acad. Sci. U. S. A., 2010, 107, 2751–2756 CrossRef CAS PubMed
.
- H. Tian, J. S. Fletcher, R. Thuret, A. Henderson, N. Papalopulu, J. C. Vickerman and N. P. Lockyer, J. Lipid Res., 2014, 55, 1970–1980 CrossRef CAS PubMed
.
- A. J. Ibanez, S. R. Fagerer, A. M. Schmidt, P. L. Urban, K. Jefimovs, P. Geiger, R. Dechant, M. Heinemann and R. Zenobi, Proc. Natl. Acad. Sci. U. S. A., 2013, 110, 8790–8794 CrossRef CAS PubMed
.
- W. Bouschen, O. Schulz, D. Eikel and B. Spengler, Rapid Commun. Mass Spectrom., 2010, 24, 355–364 CrossRef CAS PubMed
.
- X. Liu and A. B. Hummon, Anal. Chem., 2015, 87, 9508–9519 CrossRef CAS PubMed
.
- B. N. Walker, C. Antonakos, S. T. Retterer and A. Vertes, Angew. Chem., Int. Ed., 2013, 52, 3650–3653 CrossRef CAS PubMed
.
- P. J. O'Brien, M. Lee, M. E. Spilker, C. C. Zhang, Z. Yan, T. C. Nichols, W. Li, C. H. Johnson, G. J. Patti and G. Siuzdak, Cancer Metab., 2013, 1, 4 CrossRef PubMed
.
- A. F. Gonzalez-Serrano, V. Pirro, C. R. Ferreira, P. Oliveri, L. S. Eberlin, J. Heinzmann, A. Lucas-Hahn, H. Niemann and R. G. Cooks, PLoS One, 2013, 8 Search PubMed
.
- V. Pirro, P. Oliveri, C. R. Ferreira, A. F. Gonzalez-Serrano, Z. Machaty and R. G. Cooks, Anal. Chim. Acta, 2014, 848, 51–60 CrossRef CAS PubMed
.
- C. R. Ferreira, V. Pirro, L. S. Eberlin, J. E. Hallett and R. G. Cooks, Anal. Bioanal. Chem., 2012, 404, 2915–2926 CrossRef CAS PubMed
.
- B. Shrestha, P. Sripadi, B. R. Reschke, H. D. Henderson, M. J. Powell, S. A. Moody and A. Vertes, PLoS One, 2014, 9, e115173 Search PubMed
.
- Y. Coello, A. D. Jones, T. C. Gunaratne and M. Dantus, Anal. Chem., 2010, 82, 2753–2758 CrossRef CAS PubMed
.
- J. A. Stolee, B. Shrestha, G. Mengistu and A. Vertes, Angew. Chem., Int. Ed., 2012, 51, 10386–10389 CrossRef CAS PubMed
.
-
B. Shrestha and A. Vertes, in Plant Metabolism, ed. G. Sriram, Humana Press, 2014, vol. 1083, ch. 3, pp. 31–39 Search PubMed
.
- N. Pan, W. Rao, N. R. Kothapalli, R. Liu, A. W. Burgett and Z. Yang, Anal. Chem., 2014, 86, 9376–9380 CrossRef CAS PubMed
.
- T. Fujii, S. Matsuda, M. L. Tejedor, T. Esaki, I. Sakane, H. Mizuno, N. Tsuyama and T. Masujima, Nat. Protocols, 2015, 10, 1445–1456 CAS
.
- L. Zhang, D. P. Foreman, P. A. Grant, B. Shrestha, S. A. Moody, F. Villiers, J. M. Kwake and A. Vertes, Analyst, 2014, 139, 5079–5085 RSC
.
-
A. Buchberger, Q. Yu and L. Li, in Annual Review of Analytical Chemistry, Vol 8, ed. R. G. Cooks and J. E. Pemberton, Palo Alto, 2015, vol. 8, pp. 485–509, annual reviews Search PubMed
.
- W. Cheng, N. Klauke, H. Sedgwick, G. L. Smith and J. M. Cooper, Lab Chip, 2006, 6, 1424–1431 RSC
.
- T. Lapainis, S. S. Rubakhin and J. V. Sweedler, Anal. Chem., 2009, 81, 5858–5864 CrossRef CAS PubMed
.
- P. Nemes, A. M. Knolhoff, S. S. Rubakhin and J. V. Sweedler, Anal. Chem., 2011, 83, 6810–6817 CrossRef CAS PubMed
.
- J. T. Aerts, K. R. Louis, S. R. Crandall, G. Govindaiah, C. L. Cox and J. V. Sweedler, Anal. Chem., 2014, 86, 3203–3208 CrossRef CAS PubMed
.
- S. A. Moody, Methods Mol. Biol., 2000, 135, 331–347 CAS
.
- S. L. Klein, Dev. Biol., 1987, 120, 299–304 CrossRef CAS PubMed
.
- S. A. Moody and M. J. Kline, Anat. Embryol., 1990, 182, 347–362 CrossRef CAS PubMed
.
- P. A. Grant, M. B. Herold and S. A. Moody, J. Visualized Exp., 2013, 71, 4458 Search PubMed
.
- A. M. Knolhoff, K. M. Nautiyal, P. Nemes, S. Kalachikov, I. Morozova, R. Silver and J. V. Sweedler, Anal. Chem., 2013, 85, 3136–3143 CrossRef CAS PubMed
.
- P. Nemes, A. M. Knolhoff, S. S. Rubakhin and J. V. Sweedler, ACS Chem. Neurosci., 2012, 3, 782–792 CrossRef CAS PubMed
.
- P. Nemes, S. S. Rubakhin, J. T. Aerts and J. V. Sweedler, Nat. Protocols, 2013, 8, 783–799 Search PubMed
.
- J. Xia, I. V. Sinelnikov, B. Han and D. S. Wishart, Nucleic Acids Res., 2015, 43, W251–W257 CrossRef PubMed
.
- E. J. Want, G. O'Maille, C. A. Smith, T. R. Brandon, W. Uritboonthai, C. Qin, S. A. Trauger and G. Siuzdak, Anal. Chem., 2006, 78, 743–752 CrossRef CAS PubMed
.
- P. Masson, A. C. Alves, T. M. Ebbels, J. K. Nicholson and E. J. Want, Anal. Chem., 2010, 82, 7779–7786 CrossRef CAS PubMed
.
- J. D. Rabinowitz and E. Kimball, Anal. Chem., 2007, 79, 6167–6173 CrossRef CAS PubMed
.
- L. Vastag, P. Jorgensen, L. Peshkin, R. Wei, J. D. Rabinowitz and M. W. Kirschner, PLoS One, 2011, 6, e16881 CAS
.
- B. D. Bennett, J. Yuan, E. H. Kimball and J. D. Rabinowitz, Nat. Protocols, 2008, 3, 1299–1311 CAS
.
- Z. J. Zhu, A. W. Schultz, J. H. Wang, C. H. Johnson, S. M. Yannone, G. J. Patti and G. Siuzdak, Nat. Protocols, 2013, 8, 451–460 CAS
.
- D. S. Wishart, T. Jewison, A. C. Guo, M. Wilson, C. Knox, Y. F. Liu, Y. Djoumbou, R. Mandal, F. Aziat, E. Dong, S. Bouatra, I. Sinelnikov, D. Arndt, J. G. Xia, P. Liu, F. Yallou, T. Bjorndahl, R. Perez-Pineiro, R. Eisner, F. Allen, V. Neveu, R. Greiner and A. Scalbert, Nucleic Acids Res., 2013, 41, D801–D807 CrossRef CAS PubMed
.
- A. A. Kazarian, E. F. Hilder and M. C. Breadmore, J. Sep. Sci., 2011, 34, 2800–2821 CrossRef CAS PubMed
.
- M. Levin, T. Thorlin, K. R. Robinson, T. Nogi and M. Mercola, Cell, 2002, 111, 77–89 CrossRef CAS PubMed
.
- D. S. Adams, K. R. Robinson, T. Fukumoto, S. P. Yuan, R. C. Albertson, P. Yelick, L. Kuo, M. McSweeney and M. Levin, Development, 2006, 133, 1657–1671 CrossRef CAS PubMed
.
- E. H. Jaffe, A. Marty, A. Schulte and R. H. Chow, J. Neurosci., 1998, 18, 3548–3553 CAS
.
- J. W. Deng, Y. Y. Yang, M. Z. Xu, X. W. Wang, L. Lin, Z. P. Yao and T. G. Luan, Anal. Chem., 2015, 87, 9923–9930 CrossRef CAS PubMed
.
- M. T. Sheldon, R. Mistrik and T. R. Croley, J. Am. Soc. Mass Spectrom., 2009, 20, 370–376 CrossRef CAS PubMed
.
- A. Vaniya and O. Fiehn, TrAC, Trends Anal. Chem., 2015, 69, 52–61 CrossRef CAS PubMed
.
Footnote |
† Electronic supplementary information (ESI) available. See DOI: 10.1039/c6an00200e |
|
This journal is © The Royal Society of Chemistry 2016 |