DOI:
10.1039/D4SC00157E
(Edge Article)
Chem. Sci., 2024,
15, 3901-3906
In situ SERS imaging of protein-specific glycan oxidation on living cells to quantitatively visualize pathogen–cell interactions †
Received
9th January 2024
, Accepted 30th January 2024
First published on 2nd February 2024
Abstract
Glycan oxidation on the cell surface occurs in many specific life processes including pathogen–cell interactions. This work develops a surface-enhanced Raman scattering (SERS) imaging strategy for in situ quantitative monitoring of protein-specific glycan oxidation mediated pathogen–cell interactions by utilizing Raman reporter DTNB and aptamer co-assembled platinum shelled gold nanoparticles (Au@Pt-DTNB/Apt). Using Fusarium graminearum (FG) and MCF-7 cells as models, Au@Pt-DTNB/Apt can specifically bind to MUC1 protein on the cell surface containing heavy galactose (Gal) and N-acetylgalactosamine (GalNAc) modification. When FG interacts with cells, the secreted galactose oxidase (GO) can oxidize Gal/GalNAc, and the generated reactive oxygen species (ROS) further oxidizes DTNB to produce TNB for greatly enhancing the SERS signal. This strategy can quantitatively visualize for the first time both the protein-specific glycan oxidation and the mediated pathogen–cell interactions, thus providing key quantitative information to distinguish and explore the pathogen-resistance and pharmacological mechanisms of different drugs.
Introduction
Proteins on the eukaryote surface are generally modified with various glycans, which regulate the structure and function of proteins and further mediate a large number of physiological and pathological processes.1,2 In many specific life processes such as bacterium infections3 and pathogen–cell interactions,4 these glycans can be oxidized by various biological enzymes secreted from bacteria or pathogens to initiate the subsequent biological pathways. Despite broad applications of these glycan-oxidase enzymes in the recognition and labeling of glycans,5,6 the in situ quantitative visualization technique of the protein-specific glycan oxidation process in pathogen–cell interactions is still an urgent demand. The solution of this methodology will greatly facilitate the exploration of the related pathogenesis and the development of corresponding anti-resistance methods.
Fusarium graminearum (FG) is a fungus that causes Fusarium head blight through crop infections.7 It is one of the most destructive plant pathogens in the world, and the spores it produces can spread widely through the air. It can cause a wide range of human infections,8,9 especially the characteristic skin lesions caused by disseminated Fusarium, which is a most common manifestation of disease. In other cases, it can cause neutropenia and death.10FG can secrete galactose oxidase (GO) to oxidize galactose (Gal) or N-acetylgalactosamine (GalNAc), one of the most common glycans decorating on human epithelia. While oxidizing the terminal C6 hydroxy group of Gal or GalNAc to the corresponding aldehyde, hydrogen peroxide (H2O2) is generated through the reduction of oxygen,11,12 which coexists with the superoxide anion (˙O2−), hydroxyl radical (˙OH) and singlet oxygen (1O2) in biological systems, collectively referred to as reactive oxygen species (ROS).13 Thus, quantitative visualization of the generation of ROS during the oxidation of Gal and GalNAc, especially on specific protein, can provide the key information to reveal and deal with the FG induced human skin infection.
Fluorescence methods have been widely used to detect ROS in vitro and vivo.14–16 Most of these methods involve complex synthesis processes for obtaining the fluorescent probes, and usually suffer from the defects of high biological background fluorescence, low sensitivity, photobleaching, and cytotoxicity. As an alternative technique, the surface-enhanced Raman scattering (SERS) method is highly sensitive for the detection of small molecules,17 and is resistant to photobleaching and photodegradation in long-term monitoring.18 However, current SERS-based ROS probes are mainly used for intra-package detection and monitoring.19–22
In response to the lack of highly sensitive imaging methods for in situ quantitative monitoring of ROS generation and glycan oxidation on living cells, this work developed a SERS imaging strategy with a newly designed Au@Pt-DTNB/Apt nanoprobe to achieve quantitative visualization of protein-specific glycan oxidation and pathogen–cell interactions. This nanoprobe was constructed by co-assembling 5,5′-dithiobis (2-nitrobenzoic acid) (DTNB) as a Raman reporter, thiol polyethylene glycol (PEG) as the stabilizer, and thiol DNA aptamer (Apt-SH) (Table S1†) to specifically recognize the target protein of interest (POI) on platinum shell encapsulated 50 nm gold nanoparticles (Au@PtNPs) (Fig. 1A). When exposing the Au@Pt-DTNB/Apt nanoprobe bound cells to FG, the GO secreted from FG could catalyze the oxidation of the Gal/GalNAc on the cell surface to generate ROS (Fig. 1B). Due to the restricted diffusion, only the ROS generated from the oxidation of Gal/GalNAc around POI could be captured by the Au@Pt-DTNB/Apt, which converted DTNB to thiobis (2-nitrobenzoic acid) (TNB),21 thus greatly enhancing the SERS signal due to the much tighter binding of TNB to the Pt surface.23 As a proof-of-concept, MUC1 was chosen as the protein model, which is a common transmembrane glycoprotein with heavy Gal/GalNAc modification on the apical surface of most simple secretory epithelia,24–26 and abundant MUC1 expressed MCF-7 cells were used as the cell model.27 Using the commercial GO as the quantitative criterion, the proposed imaging strategy successfully achieved quantitative visualization of MUC1-specific Gal/GalNAc oxidation on living cells, and provided for the first time the quantitative information of the pathogen–cell interactions for distinguishing and exploring the different pathogen–resistance and pharmacological mechanisms of different drugs.
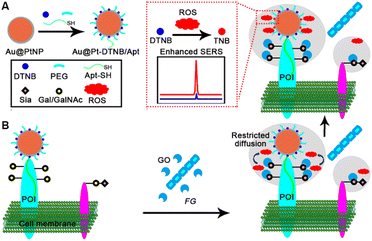 |
| Fig. 1 Schematic illustration. (A) Preparation of Au@Pt-DTNB/Apt. (B) In situ SERS imaging of MUC1-specific Gal/GalNAc oxidation for quantitative visualization of FG–cell interactions. | |
Results and discussion
Characterization of the Au@Pt-DTNB/Apt probe
The thickness of the Pt shell on Au@PtNPs was first optimized by cyclic voltammetry to check the integrity of the shell.28 By applying ascorbic acid (AA) to reduce different doses of H2PtCl6 on 50 nm Au cores, Pt shells with a thickness of 0.35 nm (Au@0.35PtNPs), 0.7 nm (Au@0.7PtNPs) and 1.4 nm (Au@1.4PtNPs) could be formed, respectively.29 After immobilizing these Au@PtNPs on glassy carbon electrodes, the cyclic voltammograms of Au@0.35PtNPs and Au@0.7PtNPs modified electrodes in 0.5 M H2SO4 showed the characteristic reduction peaks of both oxidized Au cores at +1.0 V and oxidized Pt shells around +0.45 V, while the Au@1.4PtNP modified electrode only exhibited the characteristic reduction peak of oxidized Pt shells (Fig. S1†), which indicated that the 1.4 nm Pt shell could completely coat the Au core. Thus Au@1.4PtNPs were chosen for all the following experiments.
The Au@Pt NPs were further characterized with UV/Vis absorption spectra, which showed a red shift of the maximum absorption peak upon coating of Pt shells on Au cores (Fig. S2†). The dynamic light scattering diameter also slightly increased after depositing Pt shells on Au cores (Fig. S3†), though the encapsulation of Au cores in Pt shells did not exhibit obvious morphology change (Fig. S4†). As desired, the EDS elemental mapping and drift corrected spectrum of Au@Pt NPs exhibited obvious Pt element distribution compared with those of AuNPs (Fig. S4B–E and G–K†), further demonstrating the successful construction of Au@Pt NPs.
To demonstrate the successful assembly of Apt-SH on Au@Pt NPs, FAM-Apt-SH and FAM-Apt (FAM is 5-carboxyfluorescein) were incubated with Au@Pt NPs for 30 min, respectively, which resulted in almost disappearance of FAM fluorescence in the incubated supernatant of FAM-Apt-SH, while the FAM fluorescence in the incubated supernatant of FAM-Apt slightly decreased (Fig. S5†). These results demonstrated that Apt-SH was successfully assembled into Au@PtNPs via the Pt–SH bond and the nonspecific adsorption of Apt on Au@PtNPs was negligible. The successful co-assembly of PEG, Apt-SH, and DTNB was further verified by Zeta potential analysis (Fig. S6†), which exhibited obviously more negative potential due to the presence of the DNA strand and was consistent with a previous report.30
The stability of the Au@Pt-DTNB/Apt nanoprobe was examined by in vitro Raman scanning. The Au@Pt-DTNB/Apt dispersion exhibited an obvious characteristic Raman peak of DTNB at 1337 cm−1 (Fig. S7†). Moreover, the dispersion was uniform, which led to the same Raman spectra and peak intensity at different locations, even after it was laid up for 3 days (Fig. S7†), indicating good stability of the Au@Pt-DTNB/Apt dispersion. The optical stability of the Au@Pt-DTNB/Apt dispersion was also demonstrated with the Raman spectra upon continuous laser irradiation for 600 s (Fig. S8†).
In vitro response of Au@Pt-DTNB/Apt to ROS
The feasibility of the Au@Pt-DTNB/Apt nanoprobe responding to ROS generation was in vitro verified by recording the Raman spectra in a Cu+-catalyzed Fenton reaction system.31 After adding different concentrations of ROS into the Au@Pt-DTNB/Apt dispersion, the characteristic Raman peak of DTNB at 1337 cm−1 increased with the increasing concentration of ROS (Fig. 2A), which led to a linear plot of peak intensity vs. the logarithm of ROS concentration (Fig. 2B). The limit of detection (LOD) for ROS was calculated to be 0.29 pM. The enhancement mechanism of the Raman signal could be attributed to the oxidation of DTNB in the Au@Pt-DTNB/Apt nanoprobe by ROS to produce TNB,32 which led to much tighter binding ability of the Raman reporter to the Pt surface due to the formation of the free –SH group (Fig. S9†).33 Moreover, the Au@Pt-DTNB/Apt kept stable in the 1 mM ROS solution for 3 h (Fig. S10†), which was time sufficient for the SERS imaging experiments. Thus, the designed Au@Pt-DTNB/Apt probe could be used to sensitively detect ROS in vitro, which shows promising application in bioanalysis.
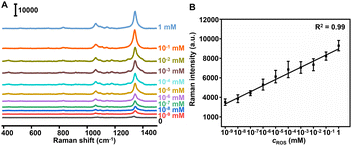 |
| Fig. 2
In vitro response of Au@Pt-DTNB/Apt to ROS. (A) Raman spectra of Au@Pt-DTNB/Apt incubated with different concentrations of ROS. (B) Plot of Raman intensity at 1337 cm−1vs. ROS concentration. The error bars indicate means ± SD (n = 5). | |
SERS imaging of protein-specific glycan oxidation on living cells
The specific binding of Au@Pt-DTNB/Apt to MUC1 protein was demonstrated by confocal laser scanning microscopy (CLSM) using FAM-Apt and Raman imaging. After MCF-7 and MCF-10A cells were treated with FAM-Apt or FAM modified random-sequenced DNA (FAM-Ran), only FAM-Apt treated cells showed obvious FAM fluorescence (Fig. S11†), indicating the specific binding of FAM-Apt to cell surface MUC1. Similarly, after incubating the cells with Au@Pt-DTNB/Apt for 40 min, a weak Raman signal of DTNB could be observed on the surface of MCF-7 cells, while the Au@Pt-DTNB/Ran treated cells did not show any Raman signal (Fig. S12†), thus Au@Pt-DTNB/Apt could specifically bind to cell surface MUC1, and the interference of the background signal and nonspecific adsorption on the Raman response was negligible. Upon the further incubation of the Au@Pt-DTNB/Apt treated cells with different concentrations of GO, the Raman signal continuously increased (Fig. 3A and B), while the Raman signal kept at the constant minimum value in the absence of GO (Fig. S12B and D†). Moreover, at different incubation times, the plots of Raman intensity vs. GO concentration showed good linearity, indicating the feasibility of using Au@Pt-DTNB/Apt to monitor the concentration of GO around cell surface MUC1.
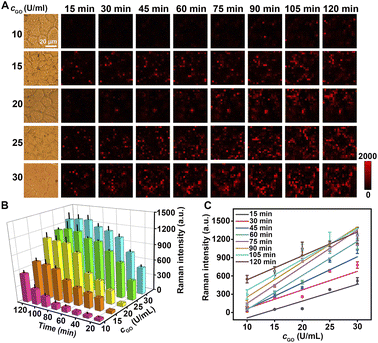 |
| Fig. 3 SERS imaging of protein-specific glycan oxidation on living cells. (A) Raman images of MCF-7 cells treated with 0.5 nM Au@Pt-DTNB/Apt for 40 min and then different concentrations of GO at different interaction times. (B) 3D histogram of Raman intensity to GO concentration at different interaction times. (C) Plots of Raman intensity vs. GO concentration at different interaction times. The error bars indicate means ± SD (n = 3). | |
The enhancement of the Raman signal on living cells in the presence of GO resulted from the formation of ROS during GO-catalyzed oxidation of Gal or GalNAc on cell surface MUC1, which diffused restrictively to bound Au@Pt-DTNB/Apt to oxidize DTNB. Thus, the Raman signal showed similar changes to those of MCF-7 cells treated with different concentrations of ROS, which were generated with a Cu+-catalyzed Fenton reaction system (Fig. S13†). To further investigate the source of ROS in the presence of GO, MCF-10A cells and 2-acetamido-2-deoxy-α-D-galactopyranoside (BAG) treated MCF-7 cells were incubated with 100 μg mL−1 of FITC-SBA (SBA is a lectin specific to Gal/GalNAc) at 37 °C for 40 min to collect their CLSM images, which did not show obvious fluorescence of FITC, while FITC-SBA stained MCF-7 cells showed strong FITC fluorescence (Fig. S14†), indicating that both MCF-10A and BAG treated MCF-7 cells could be used as Gal/GalNAc negative cells. After these negative controls were incubated with Au@Pt-DTNB/Apt and then GO, a tiny Raman signal at 1337 cm−1 was observed within 120 min (Fig. S15†), which demonstrated that ROS came from GO catalyzed Gal/GalNAc oxidation on the cell surface by dissolved oxygen.
Quantitative visualization of pathogen–cell interactions
Since Au@Pt-DTNB/Apt could quantitatively image MUC1-specific Gal/GalNAc oxidation on living cells, it was further applied to quantitatively visualize FG–cell interactions. The Au@Pt-DTNB/Apt treated MCF-7 cells were incubated with different concentrations of FG and subjected to Raman imaging in point review mode at 1337 cm−1 at different interaction times (Fig. S16A†). The Raman intensity increased with the increasing FG concentration and interaction time (Fig. S16B†). At different interaction times, all plots of Raman intensity vs. FG concentration exhibited a good linear relationship (Fig. S16C†). Combined with the linear equations of Raman intensity to GO concentration (Table S2†), the MUC1-specific Gal/GalNAc oxidation (MSGO) activities of FG at different interaction times could be calculated, which exhibited an obvious rise from 2.41 × 10−6 U per pcs to 2.54 × 10−5 U per pcs along with the increasing FG–cell interaction time (Table S2†). Thus, the designed strategy successfully achieved the quantitative visualization of FG–cell interactions through MUC1-specific Gal/GalNAc oxidation.
Pathogen-resistance and pharmacological mechanisms of different drugs
To verify the practicality of the designed strategy, it was applied to distinguish the pathogen-resistance mechanisms of different drugs. Three kinds of drugs including quercetin (QCT), carbendazim (CBZ) and cefoperazone (CFP) were selected to treat FG in view of their different application scenarios (Fig. S17†). QTC is a plant flavonoid that exerts antibacterial effects by causing cell membrane damage, altering membrane permeability, inhibiting nucleic acid and protein synthesis, reducing virulence factor expression, mitochondrial dysfunction, and preventing biofilm formation.34,35 CBZ is widely used in agriculture for crop disease control. It can be used for foliar spraying and soil treatment of plants, by changing the cell membrane and enzyme activity of pathogenic microorganisms, inhibiting their growth and reproduction, thereby protecting plants from disease damage and improving yield and quality.36 CFP is a third-generation broad-spectrum semi synthetic cephalosporin, which can combat various types of degradation effect of lactamases with a wide antibacterial spectrum.37 After FG (1 × 106 pcs per mL) was incubated with different doses of QCT (56 and 240 μg mL−1), CBZ (5 and 15 μg mL−1) and CFP (5 and 15 μg mL−1) for 6, 12 and 24 h, respectively, the drug treated FG was incubated with Au@Pt-DTNB/Apt treated MCF-7 cells for 120 min and then subjected to Raman imaging (Fig. 4A). Using the linear equations of Raman intensity to GO and FG concentrations at 120 min (Table S2†), the MSGO activities of FG treated with different doses of drugs were obtained (Fig. 4B), which demonstrated obviously different pathogen-resistance mechanisms. The MSGO activity of QCT treated FG showed abnormal QCT-dose dependence within 12 hours. The higher QCT dose conversely exhibited weaker inhibition to MSGO activity of FG, which even exhibited a tiny enhancement within 6 hours. A longer QCT-treatment time lowered the difference resulting from the dose. It could be inferred that 240 μg mL−1 of QCT led to quick structural destruction of FG to massively spill GO within a short time, while 56 μg mL−1 of QCT slowly decomposed the structure of FG without the spill of GO. Similar phenomena were observed for CBZ treated FG within 6 hours, which exhibited weaker inhibition and tiny enhancement of MSGO activity under a higher dose. Different to QTC, the CBZ exhibited tiny difference of the inhibition to MSGO activity just after 12 hours, which might be due to its stronger structural destruction ability to FG. Interestingly, the CFP exhibited the strongest inhibition to MSGO activity of FG at both doses and different treatment times, which could be attributed to the completely different antibiotic-resistance mechanism to FG. Thus, the designed strategy successfully provided key quantitative information to distinguish the different pathogen-resistance mechanisms of different drugs.
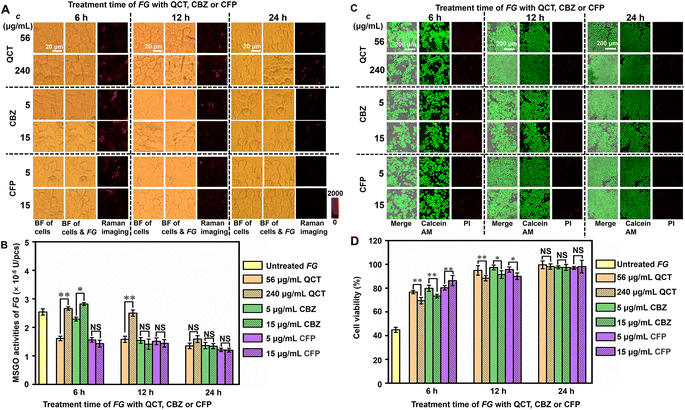 |
| Fig. 4 Pathogen-resistance and pharmacological mechanisms of different drugs. (A) Raman images of MCF-7 cells treated with 0.5 nM Au@Pt-DTNB/Apt for 40 min and then drug-treated FG for 120 min. (B) Histogram of MSGO activities of FG after treatment with drugs for different times. (C) CLSM images of calcein AM and PI stained MCF-7 cells after treatment with 0.5 nM Au@Pt-DTNB/Apt for 40 min and then drug-treated FG for 120 min. (D) Histogram of cell viability to drug treatment time of FG. The data were analyzed using a student's test at a significance level of p > 0.05 (NS), p < 0.05 (*) and p < 0.01 (**). The error bars indicate means ± SD (n = 5). | |
The states of cells during pathogen–cell interactions were critical for the further pharmacological exploration of pathogen-resistance drugs. To investigate the cell states after pathogen–cell interactions, the viability of Au@Pt-DTNB/Apt treated cells after incubation with drug-treated FG was investigated by staining these cells with calcein AM/propidium iodide (PI) to collect CLSM images (Fig. 4C, S18A and B†). Au@Pt-DTNB/Apt incubation did not obviously change the CLSM images of MCF-7 cells both after and before FG treatment (Fig. S18A†), indicating negligible influence of Au@Pt-DTNB/Apt on cell viability. By calculating the relative calcein AM/PI fluorescence values of the FG incubated groups to control groups without FG treatment, the cell viability of each group was obtained (Fig. 4D). The cells incubated with the normal FG exhibited a viability around 47% (Fig. S18A, C† and 4D), indicating the obvious toxicity of FG during the pathogen–cell interaction. After the FG was treated with three drugs respectively, the cells incubated with the treated FG showed higher viability than those incubated with untreated FG, moreover, Au@Pt-DTNB/Apt incubation did not affect the change, and the drug treatment of FG showed obvious dose dependence within 6 hours, which weakened with the increasing treatment time (Fig. 4D and S18C†). The cells incubated with CFP treated FG exhibited the highest maintaining of viability compared to those with QCT and CBZ treated FG, which was corresponding to the strongest inhibition of CFP to MSGO activity. Besides, FG treated with a higher dose of QCT and CBZ exhibited lower maintenance of cell viability within a short time of drug treatment, while the dose effect of CFP was opposite, indicating that a high dose of QCT and CBZ could quickly destroy the structure of FG, and the highly spilled GO was harmful to cells. The results of both FG MSGO activity and cell viability showed the different dynamic variations of the three drugs, indicating their different pharmacological mechanisms at the initial period of the pathogen–cell interactions. Thus, the designed strategy provided a powerful tool for the exploration of pharmacological mechanisms of pathogen-resistance drugs.
Conclusions
In summary, this work develops a SERS imaging strategy to in situ quantitatively visualize the protein-specific glycan oxidation during the pathogen–cell interaction. The proposed Au@Pt-DTNB/Apt nanoprobe can be conveniently prepared by co-assembling different components on Pt shelled AuNPs, and has been used for specifically and sensitively monitoring the generation of ROS in the oxidation process of MUC1-specific Gal/GalNAc on the cell surface during the FG–cell interactions, which oxidizes DTNB on protein-bound Au@Pt-DTNB/Apt to produce TNB for greatly enhancing the SERS signal due to the much tighter binding ability of TNB to the Pt surface. Using this quantitively SERS imaging platform, the FG-resistance phenomena of different drugs are visually monitored, which provides key quantitative information to distinguish and explore different pathogen-resistance and pharmacological mechanisms. By simple replacement of the responsive components, this quantitively SERS imaging platform can be easily expanded to other key molecules in biological systems, which provides a powerful tool for exploring the life processes related to pathogen–cell interactions.
Data availability
All relevant data are presented in the paper and ESI.† Raw data are available upon request by email to the corresponding author.
Author contributions
Y. R. W., Y. L. C., and H. X. J. proposed the idea and designed the experiments. Y. R. W., Y. L. C., and H. X. J. wrote the manuscript. Y. R. W. performed material synthesis, and cellular experiments. S. W., Y. J. Y. helped in Raman imaging. Y. H. Y., H. P. L. helped in collecting CLSM images. Y. L. C. and H. X. J. supervised and coordinated all investigators for this project.
Conflicts of interest
The authors declare no competing financial interests.
Acknowledgements
This work was supported by the National Natural Science Foundation of China (21974063, 21827812 and 21890741).
Notes and references
- E. Meezan, H. C. Wu, P. H. Black and P. W. Robbins, Biochemistry, 1969, 8, 2518–2524 CrossRef CAS PubMed.
- J. S. Axford, Biochim. Biophys. Acta, 1999, 1455, 219–229 CrossRef CAS PubMed.
- S. Hong, Y. Shi, N. C. Wu, S. Hong, Y. Shi, N. C. Wu, G. Grande, L. Douthit, H. Wang, W. Zhou, K. B. Sharpless, I. A. Wilson, J. Xie and P. Wu, Nat. Commun., 2019, 10, 1799 CrossRef PubMed.
- C. Horn, A. Namane, P. Pescher, M. Riviere, F. Romain, G. Puzo, O. Bârzu and G. Marchal, J. Biol. Chem., 1999, 274, 32023–32030 CrossRef CAS PubMed.
- Y. Guo, N. Wang, Y. Zhong, W. Li, Y. Li, G. Wang, Y. Yao, Y. Shi, L. Chen, X. Wang, L. Ding and H. Ju, J. Am. Chem. Soc., 2023, 145, 5092–5104 CrossRef CAS PubMed.
- J. Hui, L. Bao, S. Li, Y. Zhang, Y. Feng, L. Ding and H. Ju, Angew. Chem., Int. Ed., 2017, 56, 8139–8143 CrossRef CAS PubMed.
- M. P. McMullen, R. Jones and D. Gallenberg, Plant Dis., 1997, 81, 1340–1348 CrossRef PubMed.
- E. I. Boutati and E. J. Anaissie, Blood, 1997, 90, 999–1008 CrossRef CAS PubMed.
- T. J. Walsh and A. H. Groll, Transplant Infect. Dis., 1999, 1, 247–261 CrossRef CAS PubMed.
- M. Nucci and E. Anaissie, Clin. Microbiol. Rev., 2007, 20, 695–704 CrossRef CAS PubMed.
- J. W. Whittaker, Chem. Rev., 2003, 103, 2347–2363 CrossRef CAS PubMed.
- A. M. Pereira and C. Kemmelmeier, Braz. J. Microbiol., 2000, 31, 129–134 CAS.
- C. C. Winterbourn, Nat. Chem. Biol., 2008, 4, 278–286 CrossRef CAS PubMed.
- J. Xu, Y. Zhang, H. Yu, X. Gao and S. Shao, Anal. Chem., 2016, 88, 1455–1461 CrossRef CAS PubMed.
- Q. Ding, Y. Tian, X. Wang, P. Li, D. Su, C. Wu, W. Zhang and B. Tang, J. Am. Chem. Soc., 2020, 142, 20735–20743 CrossRef CAS PubMed.
- F. Liu, L. Yang, X. Yin, X. Liu, L. Ge and F. Li, Biosens. Bioelectron., 2019, 141, 111446 CrossRef CAS PubMed.
- S. Nie and S. R. Emory, Science, 1997, 275, 1102–1106 CrossRef CAS PubMed.
- Q. Xiong, C. Y. Lim, J. Ren, J. Zhou, K. Pu, M. B. Chan-Park, H. Mao, Y. C. Lam and H. Duan, Nat. Commun., 2018, 9, 1743 CrossRef PubMed.
- Q. Li, X. Ge, J. Ye, Z. Li, L. Su, Y. Wu, H. Yang and J. Song, Angew. Chem., Int. Ed., 2021, 60, 7323–7332 CrossRef CAS PubMed.
- X. Gu, H. Wang, Z. D. Schultz and J. P. Camden, Anal. Chem., 2016, 88, 7191–7197 CrossRef CAS PubMed.
- K. R. Williams, J. Chem. Educ., 2000, 77, 626–628 CrossRef CAS.
- X. Li, X. Duan, P. Yang, L. Li and B. Tang, Anal. Chem., 2021, 93, 4059–4065 CrossRef CAS PubMed.
- Y. L. Li, L. Jiang, Y. Q. Zou, Z. B. Song and S. Z. Jin, Appl. Surf. Sci., 2021, 540, 148381 CrossRef CAS.
- C. L. Hattrup and S. J. Gendler, Annu. Rev. Physiol., 2008, 70, 431–457 CrossRef CAS PubMed.
- S. R. Stowell, T. Ju and R. D. Cummings, Annu. Rev. Biochem., 1982, 51, 531–554 CrossRef PubMed.
- G. Ashwell and J. Harford, Annu. Rev. Biochem., 1982, 51, 531–554 CrossRef CAS PubMed.
- S. Staubach, H. Razawi and F. G. Hanisch, Proteomics, 2009, 9, 2820–2835 CrossRef CAS PubMed.
- S. Z. Zou and M. J. Weaver, Anal. Chem., 1998, 70, 2387 CrossRef CAS PubMed.
- S. Lin, H. Ze, X. G. Zhang, Y. J. Zhang, J. Song, H. Zhang, H. Zhang, Z. Yang, C. Yang, J. Li and Z. Zhu, Angew. Chem., Int. Ed., 2022, 61, e202203511 CrossRef CAS PubMed.
- Z. Wang, Y. Yan, C. Li, Y. Yu, S. Cheng, S. Chen, X. Zhu, L. Sun, W. Tao, J. Liu and F. Wang, ACS Nano, 2022, 16, 9019–9030 CrossRef CAS PubMed.
- A. D. Bokare and W. Choi, J. Hazard. Mater., 2014, 275, 121–135 CrossRef CAS PubMed.
- B. Sun, C. Luo, Yu Han, X. Zhang, Q. Chen, W. Yang, M. Wang, Q. Kan, H. Zhang, Y. Wang, Z. He and J. Sun, Nano Lett., 2018, 18, 3643–3650 CrossRef CAS PubMed.
- B. Yang and A. G. Agrios, J. Colloid Interface Sci., 2018, 513, 464–469 CrossRef CAS PubMed.
- K. Mansi, R. Kumar, J. Kaur, S. Devi and S. K. Mehta, J. Mol. Liq., 2021, 332, 115834 CrossRef CAS.
- T. L. A. Nguyen and D. Bhattacharya, Molecules, 2022, 27, 2494 CrossRef CAS PubMed.
- J. Shi, M. Zhao, K. Li, Y. Zhao, W. Li, Y. Peng and J. Zheng, J. Agric. Food Chem., 2022, 70, 4092–4101 CrossRef CAS PubMed.
- Y. W. Lam, M. H. Duroux, J. G. Gambertoglio, S. L. Barriere and B. J. Guglielmo, Antimicrob. Agents Chemother., 1988, 32, 298–302 CrossRef CAS PubMed.
|
This journal is © The Royal Society of Chemistry 2024 |