DOI:
10.1039/D3SC06573A
(Edge Article)
Chem. Sci., 2024,
15, 6314-6320
High-throughput single-cell mass spectrometry enables metabolic network analysis by resolving phospholipid C
C isomers†
Received
7th December 2023
, Accepted 2nd April 2024
First published on 4th April 2024
Abstract
Single-cell mass spectrometry (MS) is an essential technology for sensitive and multiplexed analysis of metabolites and lipids for cell phenotyping and pathway studies. However, the structural elucidation of lipids from single cells remains a challenge, especially in the high-throughput scenario. Technically, there is a contradiction between the inadequate sample amount (i.e. a single cell, 0.5–20 pL) for replicate or multiple analysis, on the one hand, and the high metabolite coverage and multidimensional structure analysis that needs to be performed for each single cell, on the other hand. Here, we have developed a high-throughput single-cell MS platform that can perform both lipid profiling and lipid carbon–carbon double bond (C
C) location isomer resolution analysis, aided by C
C activation in unsaturated lipids by the Paternò–Büchi (PB) reaction and tandem MS, termed single-cell structural lipidomics analysis. The method can achieve a single-cell analysis throughput of 51 cells per minute. A total of 145 lipids were structurally characterized at the subclass level, of which the relative abundance of 17 isomeric lipids differing in the location of C
C from 5 lipid precursors was determined. While cell-to-cell variations in MS1-based lipid profiling can be large, an advantage of quantifying lipid C
C location isomers is the significantly improved quantitation accuracy. For example, the relative standard deviations (RSDs) of the relative amounts of PC 34:1 C
C position isomers in MDA-MB-468 cells are half smaller than those measured for PC 34:1 as a whole by MS1 abundance profiling. Taken together, the developed method can be effectively used for in-depth structural lipid metabolism network analysis by high-throughput analysis of 142 MDA-MB-468 human breast cancer cells.
Introduction
Single-cell metabolomics and lipidomics are promising for cell biology, physiology, and medicine. Due to the extremely small volume of single cells, metabolites or lipids contained in them are typically pooled together through cell population analysis; such analysis, however, obscures the cell-to-cell heterogeneity.1 Conventional and novel single-cell analysis techniques have been proposed to reveal this heterogeneity, including fluorescence-based flow cytometry and inorganic heavy metal isotope-based mass cytometry (CyTOF).2,3 Besides these single cell analysis tools mainly targeted for protein analysis, organic MS-based single cell metabolomics and lipidomics have emerged and rapidly progressed to address important biological and biomedical questions.4,5 In the development of organic single cell MS analysis methods, various ionization/desorption approaches, such as electrospray ionization (ESI),6–9 matrix-assisted laser desorption ionization (MALDI),10,11 secondary ion mass spectrometry (SIMS),12,13 and desorption electrospray ionization (DESI)14,15 have been employed. Among these, static extraction-based single cell analysis methods such as live single-cell video MS, single probe MS, T-probe MS, pulsed direct current electrospray ionization (pico-ESI) MS, and induced nanoESI (InESI) MS have been reported as well.16–20 To leverage single cell analysis towards high-throughput analysis, automatic cell sorting and introduction methods have been developed, including Dean flow-induced cell sorting21,22 and CyESI-MS.23,24 These methods can detect a large number of small molecule metabolites, especially with good response to lipids. However, one accompanying problem is the structural characterization of lipids or metabolites detected, of which over 98% spectra in an untargeted metabolomics experiment cannot be annotated.25
Lipids, as major components of cell membranes, play essential roles in energy storage, signal transduction, and the regulation of membrane protein functions.26–29 Previous studies have shown that lipid alterations are closely related to type-II diabetes (T2D), cancers, and cytotoxicity of NK cells.30–33 Besides, a great number of isomers exist in the lipidome, including C
C location isomers and C
C geometry isomers, and in glycerolipids, fatty acyl sn-positional isomers, etc. The relative amounts of lipid isomers have been demonstrated to be correlated with various diseases including cancer, at the cell, animal model, and human levels.34–36 Over the past 20 years, innovative methods have emerged to tackle the structural diversity and complexity of lipids at the omics level towards structural lipidomics. Of note, novel ion activation methods or chemical reactions coupled with MS or MS/MS have become routine methods for detailed lipid structure analysis, e.g., ozone-induced dissociation (OzID), electron impact excitation of ions from organics (EIEIO), and ultraviolet photodissociation (UVPD).37–40 Since 2014, the PB reaction coupled with tandem MS has been reported and has now become an effective method for lipid analysis with C
C specificity.41–45 The PB-MSn method allows comprehensive analysis of lipids at the omics level in plasma, blood, tissues, and organs, in a shotgun approach or coupled with liquid chromatography (LC) for separation prior to MS analysis. Based on PB reactions, to our knowledge, we developed a single cell lipid analysis method with C
C specificity in 2021, by combining cell fixation, lipid derivatization, droplet-assisted electrospray ionization (DAESI), and MS/MS, capable of revealing new metabolic features of human breast cancer at the C
C level.35 However, similar to other static extraction methods, this approach suffers from a relatively low throughput for single-cell analysis, making it labor-intensive and difficult to accumulate a sufficient number of single cells for biological applications.
In this work, we aim to develop a high-throughput single-cell in-depth lipidomics workflow capable of detailed lipid structure elucidation and quantitative lipid isomer analysis with high accuracy, as depicted in Fig. 1 and S1.† In brief, freshly prepared single cells can be analyzed directly by MS/MS through a cell introduction device consisting of a three-layered capillary tubing (i). In addition, prior to cell introduction and MS analysis, we can also conduct batch cell fixation and PB photochemical derivatization that allow parallel C
C activation of a large number of single cells. The derivatized cells were then introduced into the MS for rapid sequential analysis (PB-MS/MS), at 51 cells per minute (ii). We used this single cell MS analysis system to analyze the in-depth lipidome of human breast cancer cells, revealing clear cellular heterogeneity and lipid composition differences with a particular dimension of detailed lipid structures, e.g., lipid C
C locations. We can simultaneously analyze six distinct lipid isomers from a single cancer cell through multiple MS2 analysis. With multiplexed lipid structure elucidation and quantitative analysis, an increased number of dimensions of lipid information can be acquired to enable more accurate cell phenotyping, reveal new metabolic features, and characterize cellular heterogeneity. Moreover, the in-depth structural lipid metabolic network can be mapped with unprecedented details to validate the co-regulation of distinct lipid species.
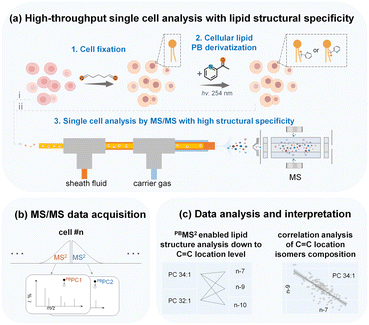 |
| Fig. 1 Illustration of the workflow for high-throughput single-cell lipid structure analysis. Single cells in suspension can be analyzed directly (i) and can also be analyzed after cell fixation followed by lipid PB derivatization (ii). Lipids of single cells can be ionized by ESI, which is accomplished through a custom-built platform with a three-layered capillary, and then analyzed via MS/MS (a). Additionally, multiple targeted MS/MS analyses can be conducted (b). Single-cell data can subsequently be used for lipid structure elucidation, correlation analysis of C C location isomers, or any other desired method of statistical analysis (c). | |
Results and discussion
Setup and performance of the single-cell lipid analysis platform
We combined single cell fixation, lipid derivatization, and ESI analysis to develop a high throughput single-cell lipid analysis platform with C
C specificity. Experimental parameters of the cell introduction device were optimized using MDA-MB-468 cells, including carrier gas pressure and sheath fluid flow rate, along with MS instrument parameters. Specifically, we found that (1) applying an appropriate high voltage (3 kV) and carrier gas pressure (6 bar) enhanced the ionization efficiency of single cell analysis (Fig. S2a–c†); (2) increasing the sheath fluid flow rate and adjusting the distance between the outlet of the middle and inner capillary initially led to an increase in signal intensity, followed by a subsequent decrease, which may be related to the extraction efficiency and dilution effect of metabolites (Fig. S2d and e†); (3) the cell concentration and the cell injection pressure were positively correlated with single cell analytical throughput (Fig. S3†). The number of single cell MS signals per minute matched well with the number of cells analyzed at different cell concentrations, indicating that most cells were detected as a single cell. A camera was used to record this process (Videos S1 and S2†). However, as the cell concentration was increased to >5 × 104 cells per mL, cell adhesion occurred and cell aggregates were observed as evidenced by the occurrence of increased proportion of consecutive MS peaks for cells (Fig. S4†). In addition, we conducted solvent evaluation for the sheath liquid for lipid extraction of single cells. Methanol yielded the highest signal intensity when used as the sheath liquid, while the addition of 1% formic acid suppressed lipid [M + Na]+ ions, thereby favoring [M + H]+ ions and yielding cleaner spectra (Fig. S5†). Consequently, methanol containing 1% formic acid was utilized as the sheath fluid.
Following method optimization, we set to evaluate the performance of the developed method for single cell analysis with lipid C
C specificity, at a cell analysis rate of 51 cells per minute (Fig. 2a). Representative mass spectra of MDA-MB-468 cells collected in positive ion mode using the developed method are shown in Fig. 2b. To improve lipid derivatization and MS detection by avoiding inter-cellular contamination, single cell fixation was performed in batch using glutaraldehyde. Fixed cells were then subjected to PB derivatization with 2-acetylpyridine (2-AP), resulting in a 121 Da mass shift (2-AP molecular weight, 121 Da) in derivatized lipids with 47.5% reaction yield (Fig. 2b and S6†). 2-AP is a versatile reagent for C
C modification in unsaturated lipids, with no reaction selectivity towards C
Cs at different locations in polyunsaturated lipids.43 Without the PB reaction, we utilized tandem MS to analyze 61 lipid precursors, leading to 145 lipids being identified, including 32 phosphatidylcholines (PCs), 35 phosphatidylethanolamines (PEs), 16 phosphatidylserines (PSs), 3 lysophosphatidylcholines (LPCs), 3 sphingomyelin (SM), and 56 triacylglycerols (TAGs) (Fig. 2c, S7 and Table S1†). With the PB reaction, we additionally identified 17 lipid C
C location isomers from 5 PC precursors in single cells (Fig. 2d and Table S2†). Of note, the fixation of glutaraldehyde on cells is based on cross-linking of amino residues in proteins. However, fixation also leads to the loss of amino lipids such as PEs and PSs, therefore no such lipid isomers were analyzed after fixation (Fig. S8†). By using PC 26:0 as the internal standard, it is estimated that the recovery rate of PCs after fixation is >95%, and after derivatization the recovery rate is >90% (Fig. S9†).
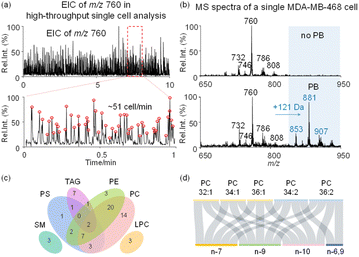 |
| Fig. 2 The analytical performance of the high-throughput single-cell lipid analysis method. (a) Single-cell analysis throughput reached approximately 51 cells per minute. (b) Mass spectra of lipids from a single cell without and with PB photochemical derivatization (the m/z values in blue: PB products of lipids). (c) Classification of identified lipids belonging to 61 parent ions in unfixed single cells. (d) C C location isomer identification in fixed and derivatized single cells. | |
Analytical performance of the high-throughput single-cell analysis platform
We first compared the analytical platform for single-cell analysis using MS1 (lipid species) and MS2 (lipid C
C location isomers) levels in analyzing MDA-MB-468 cells. Compared with the relatively large variations in MS1 lipid profiling (adding PC 26:0 to the sheath fluid as the internal standard), the relative amounts of lipid C
C location isomers offered increased quantitative stability in terms of the relative standard deviation (RSD). For PC 34:1, the precursor ions at m/z 760 among 100 single cells showed a wide distribution with a large RSD of ∼57.8% in intensity (Fig. 3a). By contrast, the variations in the relative amounts of PC 34:1 C
C location isomers were much smaller, i.e., RSDs of 48.7% for the n-10 isomers, 2.2% for the n-9 isomers and 13.8% for the n-7 isomer, with a 2.7-fold improvement compared with MS1 lipid profiling (Fig. 3a). The larger RSD for the n-10 isomers compared with the n-9 and n-7 counterparts was due to the low amount of the former. Similar results were observed for PC 34:2, where the RSD based on MS1 analysis compared to the internal standard was 62.4%, while the RSDs for the n-10, n-9, n-7, and n-6,9 isomers were 58.7%, 23.4%, 22.2%, and 10%, respectively, with an average of 2.2-fold improvement in RSD (Fig. 3b). The significantly lower variation in the quantification of lipid C
C positional isomers using the developed method can be rationalized by the tightly regulated lipid C
C positional isomer homeostasis in the biological system.34 This advantage of single cell structural lipidomics analysis with C
C specificity offers a new dimension for obtaining cellular information with added quantitative accuracy, avoiding the major difficulties of introducing lipid standards into a single cell.
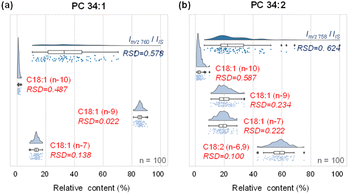 |
| Fig. 3 Comparison of analysis stability between the lipid species level and C C location isomer level for PC 34:1 (a) and PC 34:2 (b). “n” is the number of single cells analyzed. Internal standard (IS): PC 26:0 (m/z 650). | |
Inter-cellular heterogeneity between normal breast epithelial cells and breast cancer cells
With the capability to acquire detailed lipid structures and quantify lipid isomers by the developed method, additional levels of single-cell molecular information can be obtained to comprehensively characterize inter-cellular heterogeneity, enabling more accurate disease diagnostics. Specifically, we conducted a comparative analysis of human breast cancer MDA-MB-468 cells and normal breast epithelial MCF-10A cells. In these two cell lines, the C
C position isomers of PC 18:1_18:1 were structurally characterized and relatively quantified at the single-cell level. Interestingly, we observed that MCF-10A cells (n = 52) consistently showed higher amounts of n-9 isomers than MDA-MB-468 cancer cells (n = 385), as shown in Fig. 4a. Bivariate joint kernel density estimation showed significant differences in PC 18:1_18:1 isomer composition in MDA-MB-468 and MCF-10A cells. As shown in Fig. 4a, the two cell populations showed non-overlapping distributions, strongly suggesting PC 18:1_18:1 isomer composition to be a useful marker for discriminating breast cancer cells from normal ones. We also conducted an unsupervised t-distributed stochastic neighbour embedding (t-SNE) calculation for successful classification of these two cell lines based on the lipid isomer ratio (Fig. S12†). The corresponding chemical structures and MS/MS spectra of PC 18:1_18:1 isomers are shown in Fig. 4b and c. As expected, two-dimensional joint kernel density estimation of lipid isomer distribution has larger differences than one-dimensional composition distribution, highlighting the advantage of multidimensional cell classification enhanced with lipid structure information.
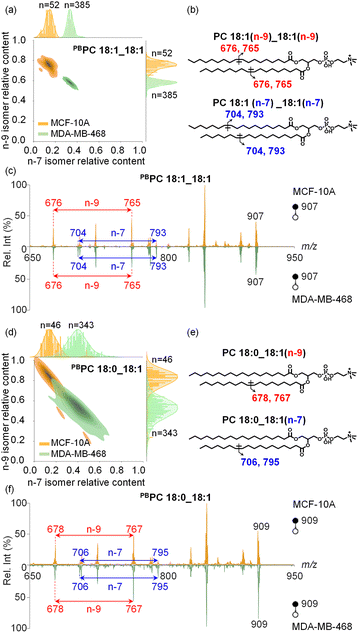 |
| Fig. 4 The heterogeneity analysis of lipid n-7 and n-9 C C location isomers in breast epithelial cells MCF-10A and cancer cells MDA-MB-468. (a) Two-dimensional kernel density estimation with a marginal plot of single cell lipid n-7 and n-9 C C location isomers in PC 18:1_18:1. The contour lines on the density map correspond to different density levels, with darker regions indicating a higher density of cells with similar metabolite levels. The marginal charts plotted on the top and right margin show the distribution of lipid isomers along both axes. “n” is the number of single cells collected. (b) Chemical structures of C C location and their specific diagnostic ions for PC 18:1_18:1, where the C C in C18:1 was located at n-9 and n-7. (c) MS/MS spectrum of PBPC 18:1_18:1 (m/z 907) from a single MDA-MB-468 cell. (d) Two-dimensional kernel density estimation with a marginal plot of single cell lipid n-7 and n-9 C C location isomers in PC 18:0_18:1. (e) Chemical structures of C C location and their specific diagnostic ions for PC 18:0_18:1, where the C C in C18:1 was located at n-9 and n-7. (f) MS/MS spectrum of PBPC 18:0_18:1 (m/z 909) from a single MDA-MB-468 cell. | |
In another abundant phospholipid PC 18:0_18:1, the relative proportions of n-9 and n-7 C
C location isomers differ significantly between MDA-MB-468 and MCF-10A cells, yet these distinct isomer amounts still show some overlap (Fig. 4d–f, n = 46 and 343 for MCF-10A cells and MDA-MB-468 cells, respectively). Nonetheless, the trend in lipid C
C isomer composition remains similar to that of PC 18:1_18:1, and for both lipids the relative amounts of n-7 isomers are higher in cancer cells.
Next, we conducted lipid C
C location isomer analysis besides n-7 and n-9 isomer analysis. As has been reported, lipid desaturation can be achieved via two biological pathways in humans, including the generation of C18:1 n-7 and n-9 isomers via stearoyl-CoA desaturase 1 (SCD1) or the generation of the C18:1 n-10 isomer through fatty acid desaturase 2 (FADS2).46 We detected elevated amounts of the C18:1 n-10 isomer in cancer cells compared to normal epithelial cells for both PC 18:1_18:1 and PC 18:0_18:1 (Fig. S11a and b†). This observation is consistent with the recently reported metabolic plasticity of lipid desaturation in cancer, where the otherwise less active FADS2 pathway in normal cells is activated in the corresponding cancer cells, leading to increased levels of phospholipid n-10 C
C isomers.46 Besides phospholipids containing only monounsaturated fatty acyls, we also analyzed the C
C isomer composition of polyunsaturated lipids. For instance, in PC 18:0_18:2, normal epithelial cells exhibited increased amounts of n-6,9 C
C location isomers (Fig. S11c†), while in PC 16:0_18:2, decreased amounts of n-6,9 C
C location isomers were detected with large isomer composition variations among the cell population analyzed (Fig. S11d†). The large variations of the amounts of n-6,9 isomers in polyunsaturated lipids are intriguing, as these lipids are exogenous and cannot be synthesized de novo in cells.
Single cell lipid analysis with C
C specificity enabling in-depth structural lipid metabolic network analysis
To demonstrate the advantages of single-cell lipid analysis with structural specificity, we focused on the relative distribution of lipid C
C location isomers in MDA-MB-468 cells to study their roles in lipid metabolic pathways. A metabolic network involving C18:1-containing lipids is shown in Fig. S13a,† where PC 18:1_18:1 containing two C18:1 fatty acyls was used as an example (Fig. S10a†). The composition of lipid isomers including n-7, n-9, and n-10 isomers in single cells was analyzed and used to evaluate their metabolic correlations. A strong negative correlation between the n-7 and n-9 isomers was observed (Fig. S13b†), which can be well explained by the fact that C18:1 n-7 and n-9 isomers are generated from the same SCD1 pathway, and more importantly the amounts of these two isomers may be more dominant than other possible isomers, e.g., the less abundant C18:1 n-10 isomer from the independent FADS2 pathway (Fig. S13a†).
We attempted to apply this method to more complex lipid pathways, which requires characterization and quantitation of more structurally distinct lipids from single cells. Such analysis requires the collection of multiple MS/MS spectra from a single cell for structural elucidation of lipids. In the shotgun analysis mode, we set up an MS1 full scan followed by multiple targeted MS/MS scans (Fig. S14†). To extend the cell analysis time without compromising detection sensitivity, we adjusted the distance between the middle/inner capillary outlets from 2 mm to 4 mm. MS/MS analysis of derivatized PC 16:0_18:1 (m/z 881) and PC 16:0_16:1 (m/z 853) was performed for structural elucidation and quantitation of their C
C isomers (Fig. S15†). The biosynthesis pathways of common lipid C
C isomers are illustrated in Fig. 5a. MS/MS analysis revealed 6 PC C
C location isomers and enabled their metabolic correlations. While the majority of lipid C
C isomers exhibited negative correlations, a few instances (3 out of 15 correlation analysis results) showed positive correlations (Fig. 5b and Table S3†). Specifically, positive correlations were observed between the n-7 location isomers of PC 16:0_16:1 and PC 16:0_18:1, as well as between their corresponding n-9 and n-10 location isomers. This finding is consistent with the generation of n-10, n-7, and n-9 fatty acyl-CoA species, which are then utilized for further synthesis of more complex phospholipids, such as PC 16:0_16:1 and PC 16:0_18:1. Within PC 16:0_16:1 or PC 16:0_18:1 (Fig. 5b), a strong negative correlation between their n-7 and n-9 isomers was also observed, as has been pointed out previously. These experimental results highlight the usefulness of lipid structural elucidation and quantitative analysis in lipid metabolic pathway analysis.
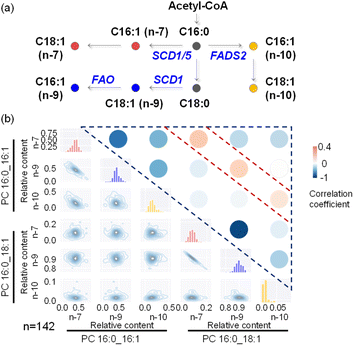 |
| Fig. 5 Multi-target lipid analysis enabled metabolic network analysis of lipid C C location isomers. (a) Biosynthetic pathways of monounsaturated FA 16:1 and FA 18:1. SCD1 and SCD5, stearoyl-CoA desaturase 1 and 5; FADS2, fatty acid desaturase 2; FAO, fatty acid oxidation. (b) Pairwise bivariate distributions of n-7, n-9, and n-10 C C locations within PC 16:0_16:1 and PC 16:0_18:1 molecules for lipid isomer co-regulation analysis. In the lower left part, a two-dimensional kernel density estimation visually represented the distribution of the specified metabolites along both axes, with contour lines indicating different density levels. The diagonal histograms provided insights into the marginal distribution of each individual compound. The correlation analysis results are displayed in the upper right part, and the specific results correspond to the accompanying color bar. “n” is the number of single cells collected. | |
Conclusions
Single-cell analysis has emerged as a research hotspot, primarily due to its ability to reveal detailed information that is often obscured in population analysis. In this work, we have successfully achieved high-throughput single-cell fine structure analysis by combining PB derivatization with the CyESI-MS system, resulting in an impressive throughput of nearly 51 cells per minute. By derivatizing the cells, we were able to characterize the precise lipid structures within individual cells and perform heterogeneity analysis of lipid C
C position isomers. As a result, 17 lipid C
C location isomers from 5 PC precursors can be identified at the single cell level using PB derivatization. This breakthrough in high-throughput single-cell lipidomics analysis allowed us to observe distinct distributions of lipid C
C location isomer composition between the breast cancer cell line MDA-MB-468 and the mammary epithelial cell line MCF-10A. Furthermore, correlation analysis of different lipid C
C position isomers showed a compelling agreement with metabolic pathways observed in living organisms. The good agreement with known pathways confirms the reliability and accuracy of our single-cell analysis approach, demonstrating its potential to provide deeper insights into cellular processes and offer new perspectives on cellular heterogeneity and the complex interactions within cellular systems. This innovative approach has the potential to revolutionize lipidomics research and contribute to a better understanding of cellular heterogeneity, disease mechanisms, and potential therapeutic targets.
Data availability
All experimental and characterization data and detailed experimental procedures are available in the published article and ESI.†
Author contributions
S. C., C. C., and X. M. designed the experiments. S. C. and C. C. prepared the materials, performed the experiments, and conducted data analysis. Y. Q., C. C., and H. Y. maintained the single cell analysis device. S. C., C. C., and X. M. wrote the manuscript. Y. Q., H. Y., X. G., X. D., and Z. O. modified the figures and the manuscript. X. D., Z. O., and X. M. supervised the project. All the authors discussed the results and contributed to editing the manuscript.
Conflicts of interest
There are no conflicts to declare.
Acknowledgements
This work was supported by the National Key R&D Program of China (2022YFC3401900 and 2022YFF0705001), the Plan for Leading Talents of Science and Technology Innovation (WR2202), and the National Natural Science Foundation of China (21974077, 21927812, and 22304169).
Notes and references
- L. Zhang and A. Vertes, Angew. Chem., Int. Ed., 2018, 57, 4466–4477 CrossRef CAS PubMed.
- S. C. Bendall, E. F. Simonds, P. Qiu, E. D. Amir, P. O. Krutzik, R. Finck, R. V. Bruggner, R. Melamed, A. Trejo and O. I. Ornatsky, Science, 2011, 332, 687–696 CrossRef CAS PubMed.
- G. Han, M. H. Spitzer, S. C. Bendall, W. J. Fantl and G. P. Nolan, Nat. Protoc., 2018, 13, 2121–2148 CrossRef CAS PubMed.
- R. Zenobi, Science, 2013, 342, 1243259 CrossRef CAS PubMed.
- B. Shrestha, Methods Mol. Biol., 2020, 2064, 1–8 CrossRef CAS PubMed.
- Z. Li, Z. Wang, J. Pan, X. Ma, W. Zhang and Z. Ouyang, Anal. Chem., 2020, 92, 10138–10144 CrossRef CAS PubMed.
- X.-C. Zhang, Q. Zang, H. Zhao, X. Ma, X. Pan, J. Feng, S. Zhang, R. Zhang, Z. Abliz and X. Zhang, Anal. Chem., 2018, 90, 9897–9903 CrossRef CAS PubMed.
- X. Gong, Y. Zhao, S. Cai, S. Fu, C. Yang, S. Zhang and X. Zhang, Anal. Chem., 2014, 86, 3809–3816 CrossRef CAS PubMed.
- H. Mizuno, N. Tsuyama, T. Harada and T. Masujima, J. Mass Spectrom., 2008, 43, 1692–1700 CrossRef CAS PubMed.
- A. J. Ibáñez, S. R. Fagerer, A. M. Schmidt, P. L. Urban, K. Jefimovs, P. Geiger, R. Dechant, M. Heinemann and R. Zenobi, Proc. Natl. Acad. Sci. U. S. A., 2013, 110, 8790–8794 CrossRef PubMed.
- A. Amantonico, P. L. Urban, S. R. Fagerer, R. M. Balabin and R. Zenobi, Anal. Chem., 2010, 82, 7394–7400 CrossRef CAS PubMed.
- Z. Yuan, Q. Zhou, L. Cai, L. Pan, W. Sun, S. Qumu, S. Yu, J. Feng, H. Zhao, Y. Zheng, M. Shi, S. Li, Y. Chen, X. Zhang and M. Q. Zhang, Nat. Methods, 2021, 18, 1223–1232 CrossRef CAS PubMed.
- I. Lanekoff, M. E. Kurczy, K. L. Adams, J. Malm, R. Karlsson, P. Sjövall and A. G. Ewing, Surf. Interface Anal., 2011, 43, 257–260 CrossRef CAS.
- H.-M. Bergman and I. Lanekoff, Analyst, 2017, 142, 3639–3647 RSC.
-
C. R. Ferreira, V. Pirro, A. K. Jarmusch, C. M. Alfaro and R. G. Cooks, Single Cell Metabolism: Methods and Protocols, 2020, pp. 159–179 Search PubMed.
- H. Mizuno, N. Tsuyama, T. Harada and T. Masujima, J. Mass Spectrom., 2008, 43, 1692–1700 CrossRef CAS PubMed.
- N. Pan, W. Rao, N. R. Kothapalli, R. Liu, A. W. G. Burgett and Z. Yang, Anal. Chem., 2014, 86, 9376–9380 CrossRef CAS PubMed.
- R. Liu, N. Pan, Y. Zhu and Z. Yang, Anal. Chem., 2018, 90, 11078–11085 CrossRef CAS PubMed.
- Z. Wei, X. Xiong, C. Guo, X. Si, Y. Zhao, M. He, C. Yang, W. Xu, F. Tang, X. Fang, S. Zhang and X. Zhang, Anal. Chem., 2015, 87, 11242–11248 CrossRef CAS PubMed.
- H. Zhu, G. Zou, N. Wang, M. Zhuang, W. Xiong and G. Huang, Proc. Natl. Acad. Sci. U. S. A., 2017, 114, 2586–2591 CrossRef CAS PubMed.
- Q. Huang, S. Mao, M. Khan, L. Zhou and J.-M. Lin, Chem. Commun., 2018, 54, 2595–2598 RSC.
- S. Xu, M. Liu, Y. Bai and H. Liu, Angew. Chem., Int. Ed., 2021, 60, 1806–1812 CrossRef CAS PubMed.
- H. Yao, H. Zhao, X. Zhao, X. Pan, J. Feng, F. Xu, S. Zhang and X. Zhang, Anal. Chem., 2019, 91, 9777–9783 CrossRef CAS PubMed.
- Q. Liu, W. Ge, T. Wang, J. Lan, S. Martínez-Jarquín, C. Wolfrum, M. Stoffel and R. Zenobi, Angew. Chem., Int. Ed., 2021, 60, 24534–24542 CrossRef CAS PubMed.
- R. R. da Silva, P. C. Dorrestein and R. A. Quinn, Proc. Natl. Acad. Sci. U. S. A., 2015, 112, 12549–12550 CrossRef CAS PubMed.
- U. Meier and A. M. Gressner, Clin. Chem., 2004, 50, 1511–1525 CrossRef CAS PubMed.
- D. Lingwood and K. Simons, Science, 2010, 327, 46–50 CrossRef CAS PubMed.
- M. P. Wymann and R. Schneiter, Nat. Rev. Mol. Cell Biol., 2008, 9, 162–176 CrossRef CAS PubMed.
- A. Shevchenko and K. Simons, Nat. Rev. Mol. Cell Biol., 2010, 11, 593–598 CrossRef CAS PubMed.
- Z. Wang, E. Klipfell, B. J. Bennett, R. Koeth, B. S. Levison, B. Dugar, A. E. Feldstein, E. B. Britt, X. Fu, Y.-M. Chung, Y. Wu, P. Schauer, J. D. Smith, H. Allayee, W. H. W. Tang, J. A. DiDonato, A. J. Lusis and S. L. Hazen, Nature, 2011, 472, 57–63 CrossRef CAS PubMed.
- F. Röhrig and A. Schulze, Nat. Rev. Cancer, 2016, 16, 732–749 CrossRef PubMed.
- C. B. Wood, N. A. Habib, A. Thompson, H. Bradpiece, C. Smadja, M. Hershman, W. Barker and K. Apostolov, BMJ, 1985, 291, 163–165 CrossRef CAS PubMed.
- J. Li, S. Condello, J. Thomes-Pepin, X. Ma, Y. Xia, T. D. Hurley, D. Matei and J.-X. Cheng, Cell Stem Cell, 2017, 20, 303–314.e5 CrossRef CAS PubMed.
- W. Zhang, D. Zhang, Q. Chen, J. Wu, Z. Ouyang and Y. Xia, Nat. Commun., 2019, 10, 79 CrossRef CAS PubMed.
- Z. Li, S. Cheng, Q. Lin, W. Cao, J. Yang, M. Zhang, A. Shen, W. Zhang, Y. Xia, X. Ma and Z. Ouyang, Nat. Commun., 2021, 12, 2869 CrossRef CAS PubMed.
- X. Guo, W. Cao, X. Fan, Z. Guo, D. Zhang, H. Zhang, X. Ma, J. Dong, Y. Wang, W. Zhang and Z. Ouyang, Angew. Chem., Int. Ed., 2023, 62, e202214804 CrossRef CAS PubMed.
- M. C. Thomas, T. W. Mitchell, D. G. Harman, J. M. Deeley, J. R. Nealon and S. J. Blanksby, Anal. Chem., 2008, 80, 303–311 CrossRef CAS PubMed.
- H. T. Pham, A. T. Maccarone, M. C. Thomas, J. L. Campbell, T. W. Mitchell and S. J. Blanksby, Analyst, 2014, 139, 204–214 RSC.
- T. Baba, J. L. Campbell, J. C. Y. Le Blanc and P. R. S. Baker, Anal. Chem., 2017, 89, 7307–7315 CrossRef CAS PubMed.
- J. S. Brodbelt, L. J. Morrison and I. Santos, Chem. Rev., 2020, 120, 3328–3380 CrossRef CAS PubMed.
- X. Ma and Y. Xia, Angew. Chem., Int. Ed., 2014, 53, 2592–2596 CrossRef CAS PubMed.
- X. Ma, L. Chong, R. Tian, R. Shi, T. Y. Hu, Z. Ouyang and Y. Xia, Proc. Natl. Acad. Sci. U. S. A., 2016, 113, 2573–2578 CrossRef CAS PubMed.
- W. Cao, S. Cheng, J. Yang, J. Feng, W. Zhang, Z. Li, Q. Chen, Y. Xia, Z. Ouyang and X. Ma, Nat. Commun., 2020, 11, 375 CrossRef CAS PubMed.
- T.-H. Kuo, H.-H. Chung, H.-Y. Chang, C.-W. Lin, M.-Y. Wang, T.-L. Shen and C.-C. Hsu, Anal. Chem., 2019, 91, 11905–11915 CrossRef CAS PubMed.
- W. Cao, X. Ma, Z. Li, X. Zhou and Z. Ouyang, Anal. Chem., 2018, 90, 10286–10292 CrossRef CAS PubMed.
- K. Vriens, S. Christen, S. Parik, D. Broekaert, K. Yoshinaga, A. Talebi, J. Dehairs, C. Escalona-Noguero, R. Schmieder, T. Cornfield, C. Charlton, L. Romero-Pérez, M. Rossi, G. Rinaldi, M. F. Orth, R. Boon, A. Kerstens, S. Y. Kwan, B. Faubert, A. Méndez-Lucas, C. C. Kopitz, T. Chen, J. Fernandez-Garcia, J. A. G. Duarte, A. A. Schmitz, P. Steigemann, M. Najimi, A. Hägebarth, J. A. Van Ginderachter, E. Sokal, N. Gotoh, K.-K. Wong, C. Verfaillie, R. Derua, S. Munck, M. Yuneva, L. Beretta, R. J. DeBerardinis, J. V. Swinnen, L. Hodson, D. Cassiman, C. Verslype, S. Christian, S. Grünewald, T. G. P. Grünewald and S.-M. Fendt, Nature, 2019, 566, 403–406 CrossRef PubMed.
|
This journal is © The Royal Society of Chemistry 2024 |