DOI:
10.1039/D2EN00469K
(Communication)
Environ. Sci.: Nano, 2023,
10, 424-439
Nanoparticles in bodily tissues: predicting their equilibrium distributions
Received
17th May 2022
, Accepted 3rd December 2022
First published on 12th December 2022
Abstract
Nanoparticles (NPs) interact within organisms via various biochemical interactions which can bring benefits to society. Classically, fate/distribution of substances is assessed via phase (octanol–water) based partitioning. A decade ago, Praetorius famously stated that phase-based partitioning for NPs is “a road to nowhere”. While (in vivo) experiments are cumbersome, reliable partitioning values are of utmost importance given a wealth of medicinal/toxicological and environmental exposure assessments. In this communication, we describe calculus for distribution in human tissues. We applied surface free energy components for NPs, cell membranes/vesicles, plasma and protein describing (van de Waals and Lewis acid–base) interactions amongst tissue and blood constituents. We considered neutral and charged NPs, and various tissues for statistical evaluation. Comparison to experiments showed that predictions are acceptable (R2 ≥ 0.7). Depending on surface functionality, phagocyte-rich and cancerous tissues accumulate NPs distinctly from ‘normal’ tissue, via e.g., receptor (lectin/cadherin) binding. Our modeling study aids and supplements experiments to quantify the interactions, tissues concentrations and transport of NPs with(in) organs, to unravel mechanisms of human exposures. It provides a reference for partitioning to benchmark upcoming medical applications (e.g., PBPK) and human/ecological risk assessments, enabling experimentalists more efficient monitoring, data interpretation, and reduces cost/time-intensive medicinal and toxicological campaigns.
Environmental significance
Current study in our research group deals with the prediction of distribution of nanoparticles in humans. This is crucial, but not adequately covered by current fate models. In this study surface-driven models were developed capable of predicting partitioning of structurally diverse nanoparticles. The developed models can be used to predict distribution in various tissues. The methods developed in our study are the first of its kind that allow for robust predictions that were not possible previously. We believe Environmental Science: Nano readers will benefit from the results outlined in this study as it aids their further research and policy decisions.
|
1. Introduction
Nanoparticles (NPs)1,2 have a wide range of applications in chemical industry and in medicine.3,4 NPs are, e.g., used therapeutically to target tumor cells. NPs however, also come with environmental risks5,6 depending on non-targeted biochemical interactions.7,8 As NPs come in different materials and sizes, quantifying the impact of surface coating9/functionalization on NPs cellular transport has important implications in toxicology.
For decades, fate and accumulation of small organic compounds have been benchmarked using phase-behavior/partitioning.10 Oil–water11,12 and octanol–water partition coefficient (Kow) have been used to predict NP accumulation/transformation in environments13,14 and organisms.10,15 However, NPs interact with bio-membrane surfaces,16 preventing dispersion.17,18 Interactions between NPs and biological matrices are difficult to characterize due to adsorption and (irreversible) agglomeration.
Pauli, markedly said, “God made the bulk; the surface was invented by the devil”.19 In a bulk phase, elements are surrounded by other similar elements. Surface elements interact either with elements from the same surface, or with elements located just below, above or beyond it. Therefore, properties of a phase and its energies differ depending upon location, making phase-partitioning inadequate to describe exposure. As NP interactions are surface-driven, Praetorius stated that “assessing NP fate via Kow is a road to nowhere”.17
Fully empirical (i.e., ‘black box’)20 methods, evaluate cellular equilibria of NPs without regard for mechanism and have confined applicability due to lack of understanding. Instead, mechanistic insights are needed to describe NP-biological interactions semi-empirically. Current semi-empirical methods21,22 apply mechanisms but still have limited applicability to other exposure regimes.
Interaction with tissue components is at the basis of (NP) accumulation.23,24 Transport of NPs in(to) cells25 depend on uptake pathway, possible via passive diffusion into/through the cytoplasm, adhesion to endoplasmic reticulum26 or Golgi apparatus27 to be encapsulated by membranes and vesicles,28–30 (e.g., non-endocytic pathways for red blood cells31). NP can agglomerate in vesicles, to be excreted by cells.29 NPs transport and accumulation (agglomeration) in/to lysosomes17 enables acid-catalyzed degradation,7,30,32,33 altering their surfaces.34
Transport by vesicles35 drives NP exo/endocytosis. Upon cytosis, cell membranes and vesicles deform to fuse and release/trap NPs.36 Therefore, past research predicted transport based on membrane energies like crossing, deformation,37,38 encapsulation and combination.39 Recent work40 linked NP properties to traits of cells to assess interaction energy and predict cellular uptake and elimination. Properties of NPs, e.g., charge (density41) and cell traits influence NP transport, but it remains difficult to characterize binding to vesicles. Identifying the probability/frequency of binding and transport42 enable assessing NP exposure.
Relationships between surface physico-chemical properties and cell behavior at the interface have been hypothesized.43–46 We specify this hypothesis by considering NP properties and tissue/cells traits to assess partitioning in organs, Fig. 1. In this communication, we quantify exposure by using interaction energies between NPs and membranes. We consider the fraction/frequency of NPs bound/encapsulated by/in organ(elles). We focus on polar (Lewis acid–base) and Van de Waals forces. We assessed our model with experimental data for various tissues and explored the effect of NP properties on partitioning.
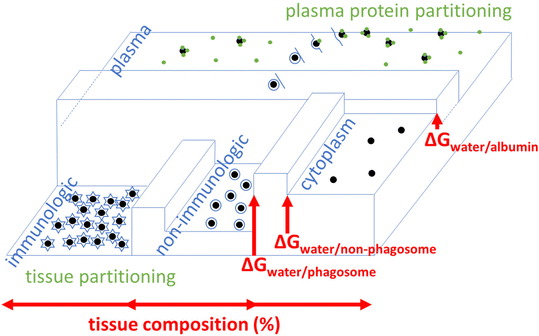 |
| Fig. 1 Example distribution of NPs throughout/around tissues, influenced by energy G. Plasma = extracellular serum (saline water + protein). Difference between dividing beams (barriers) are ΔG = ΔG‡on − ΔG‡off, i.e., denotes equilibrium which is attained after long-term exposure. Intracellular vesicle-free NPs exist.47 Low G means high NP concentration: [NP] across compartments i (horizontal) [NP]j/[NP]total = e−ΔGnp-j/RT; depending on properties, accumulation in phagocytes/lysosomes. | |
2. Methods
2.1. Tissue compositions
Tissue partitioning (K) is affected by the amount of membrane in a cell, and how many cells of a type an organ tissue contains. E.g., cancerous cells express enhanced intracellular signaling via vesicles.48–52 As the concentration of cells and their membranes is in excess to NP concentration, we take that sorption is linear in NP concentration, and that K is a summation function over Boltzmann partitioning (e−ΔGi/RT) among cell types (i), weighted by their proportion in the tissue: | 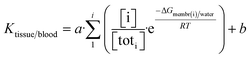 | (1) |
We take proportions of cell types from Table 1. We calculate binding energy changes ΔGi from surface energies γ, section 2.3. Apart from membranes, proteins influence distribution of NPs. We take serum protein concentration independent of tissue type (equal among capillary bloods), and describe its influence in section 2.2. Knowing how much water organs contain, we extrapolate e−ΔG(tissue/water)/RT to e−ΔG(organ/water)/RT, Table 1.
Table 1 Simple representative composition (%) of healthy human organ tissues by generic celltype. Colors denote dominant contribution to energy (red = hydrophobic, blue = Lewis basic, green = Lewis acidic). We combined compositions with surface energy data (Table 2). Membrane types have varying degrees of immunological (Fig. 1) relevance53–70
Representative functional cell;
excluding bone marrow;
assuming the majority of immunological cells is phagocytosic,
BBB consists of tightly packed endothelial cells.
White pulp (25% of splenictissue) structurally similar to lymph.71
|
|
Table 2 Energy components of membrane surfaces in cell types (mJ m−2). Ranges are variabilities across exp. setups. Colors denote dominant contribution to γ (red = hydrophobic, blue = basic polar, green = acidic polar). Octanol is a reference to phase partitioning44,116–131
Values represent untreated keratinous skin, keratin <85% of differentiated keratinocytes.132
Human endothelial cellline HUVEC.
Values unknown, γLW taken for a generic cell,78,80γ+ taken as range for non-immune cells, γ− taken corresponding to maximum binding.120
For bone/osteocytes, membranes surface reflects hydroxyapatite, values represent untreated (hydrophilic) hydroxyapatite (>70% crystalline) and collagen.
Phagocytic cell lines THP-1, HL-60.
Local tissue/organelle pH enhances γ: linear/exponential extrapolations82,102 imply γ+ = 1.2–6.0 mJ m−2, substantiating values reported.
Breast cancer cell line MCF-7.
|
|
2.2. Membrane–protein–water partitioning
We calculate Kivia Boltzmann, via ΔG: values for free energy of binding. Fig. 1 depicts the influence of G (vertical) across different surfaces/compartments (horizontal). The lower G, the higher the partitioning therein/on. The ability of NPs to partition onto membranes depend on their bio-availability, i.e., interaction with endemic serum proteins,46,72,73Fig. 1. We define partitioning of NPs between water (w) and membranes (m) as function of serum plasma protein coating in two additive terms: |  | (2) |
where we take that a NP is either covered or uncovered by serum proteins (p), analogous to small organic molecules. α is a dimensionless frequency of NP-encounters, proportional to plasma protein amount (7%); inversely proportional to the NPs (surface area) acting as a plasma protein scavenger,
. RT is gas constant; temp.
Serum contains 60–80 g L−1 plasma protein (35–50 g L−1 albumin), with MW of ∼150 kg mol−1, thus (70/150000) × 6.02 × 1023 = 2.8 × 1020 proteins per L (size dserum = 6 nm), of which (1000/(6 × 6) = ) 28 can adsorb on 1000 nm2 NP surface. Thus, protein concentration is in excess to NPs dosing concentration [NP] in any practical scenario (106–1012 NPs L−1 (ref. 74)). We thus disregard NP homo-/heteroaggregation/agglomeration, taking α = 0.07.
2.3. Free energy changes
We obtained different binding free energy changes ΔG via:
ΔGwater→membrane(serum) = A·(γABserum–plasma–membr + γLWserum–plasma–membr) |
ΔGwater→membrane(NP) = A·(γABNP–plasma–membr + γLWNP–plasma–membr) |
ΔGwater→serum(NP) = A·(γABNP–plasma–protein + γLWNP–plasma–serum) |
| ΔGserum→water(NP) = −A·(γABNP–plasma–serum + γLWNP–plasma–serum) | (3) |
In ‘classical phase partitioning’ (for small organic compounds) A is the solvent accessible molecular surface area. NP partitioning is geometry-driven;75 we assume only partial wrapping of surface area (e.g., bending/deformation negligible to AB/LW forces or compensated by receptor–ligand binding76) estimating the interaction area (m2) A from molar volume77 (here, the ethylene glycol monomer of PEG). We involved Vande Waals (LW)/polar Lewis acid–base (AB) forces40,78 taking distances in equilibrium by born repulsion, 0.157 nm.78
We calculated γAB and γLW (mJ m−2) from effective surface energy components γLW, γ+ and γ− (electron acceptor and donor) for each species: NPs, membranes, serum plasma protein and water; details in ref. 78 and 79, substantiated by multiple cell lines (macrophage, endothelial cancer, fibroblast, etc.).80,81 We take for γ+protein, γ−protein, γLWprotein of serum protein 0.002, 20, and 41 mJ m−2, taken to resemble dry albumin at pH 7,79,82 and for γ+plasma, γ−plasma, γLWplasma of plasma 25.5, 25.5 and 21.8, mJ m−2, taken to resemble water. For NP γ+NP, γ−NP, γLWNP we took 0, 45, and 43 mJ m−2, taken to be polyethylene glycol.
2.4. Cell membrane types
While carbohydrate contents in membrane surfaces do not (greatly) differ between cell types,83–87 differentiation involves glycosylation:88–90 phagocyte have glycosylated protein receptors (lectins90,91) with binding motifs specific to (β-)glucan-chitin copolymers92–94 recognizing foreign particles. Liver (Kupffer)92,95,96 and cancer cell97,98 membranes are lectin-rich. Immunological (mucus/phagocytic/cancerous) cells have enhanced metabolism over ‘tranquil’/‘sluggish’, e.g., endothelial cells.7,81,99,100
Via abnormal metabolism cancer cells produce e.g., lactate acidifying tissues,101 affecting bio-adsorption.102,103 Metastatic cancer cells migrate/proliferate to tissues via the blood,104,105 depending on hydrophilicity (i.e., γAB). Cells contain many surfaces: Golgi apparatus/vesicles/lysosomes/endoplasmic reticulum. Liver macrophages internalize NPs106–108 and entail acid-rich lysosomes, attacking particles.109,110 pH can alter/affect surface activity, tension (γ)111 and ‘biocollisions’.112
Membranes thereby differ in characteristic ‘surface acidity’,113 analogous to pKa/pH functionalities among organic compounds (pKa's on surfaces).114 We characterize cell membrane type by energy of surfaces γ. Adipocytes contain more lipid (with specific γ).85,115Table 2 lists γ+membrane, γ−membrane, γLWmembrane values that we used to effectuate aforementioned factors, substantiated by relationships between phagocytosis/contact angle (i.e., γ).43
2.5. Testing using experimental tissue partitioning.
We evaluate accuracies of K from eqn (1)–(3) by comparison with experimentally-derived K from in vivo concentration data133,134 (open literature). We neglect biotransformation, and disregard elimination via faeces/urine. We focus on large exposure times t, e.g., months,133 so organs continuously take in/eliminate NPs (4, 13, 100 nm, coated with PEG) with equal rates. By analogy, barriers in Fig. 1 are sufficiently low. Then, dividing uptake and elimination gives K for organ tissues: | 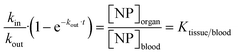 | (4) |
assuming that experiments reflect equilibrium. Section 4.2–4.4 discusses accuracy of the assumption.
3. Results
3.1. Interaction between NPs, membranes and serum
Table 2 shows energies for interaction between PEG-NPs and membrane biomaterial membrane surfaces (γ and ΔG). Interaction energies are either positive or negative. The larger the hydrophobicity and Lewis acidic character of the membrane, the more negative the energy values for interaction. The ΔG did not differ between differently sized PEG-NPs (4–100 nm) as the PEG coating groups are similarly sized.
The ΔGNP range from −4.4 to +5.1 kJ mol−1, which is an energy range of 9.5 kJ mol−1. If ΔGalbumin were taken into account also, the summed ΔG is a range of −11.5 to +7.8 kJ mol−1. This shows that albumin has a differentiating effect on cell type. Based on these values, viaeqn (1)–(3), ratios were calculated for partitioning of PEG-NPs between serum and membranes. Predicted K was highest for non-polar lipocyte surfaces, and lowest for endothelial/epithelial cells. Again, the larger the hydrophobicity/Lewis acidic character of the membrane surface, the larger the predicted K.
3.2. Partitioning of NPs in organ tissues
Experimentally derived (eqn (4)) Ktissue/blood for PEG-NPs range from 0.044 to 2600;133 these K's are independent of time within 7 days to 6 months. Other data for starch/dextran coated NPs135 and eqn (4) imply a 3 day apparent tissue/blood/K is ≥7 for phagocyte-rich tissues (e.g., liver/spleen); for phagocyte-poorer tissues, Ktissue/blood ≥ 0.3.135 These values appear low compared to longer exposure times >7 days–6 months. This indicates absence of equilibrium or steady state. We did not see a statistically significant effect of NP size on the Kexp,133Fig. 1. Surface functionality does influence partitioning, with 6 day Kliver/blood > Kspleen/blood for cationic CTAB-NPs, but Kliver/blood < Kspleen/blood for neutral PEG-NPs.134
3.3. Prediction of NP partitioning
Fig. 2 depicts predicted K, eqn (1)–(3) and experimentally derived K (eqn (4)) for PEG-NP partitioning in different organ tissues, with reference to blood (Ktissue/blood). For 4 nm PEG-NPS, the Pearson correlation coefficient R2 = 0.69 and p = 0.0004 (2SD). For 13 nm, R2 = 0.75, p = 0.0001 (2SD) and for 100 nm R2 = 0.70, p = 0.0004 (2SD). For 4, 13 and 100 nm grouped together, R2 = 0.68, p < 0.00001 (2SD). The p values of these four linear regressions are all lower than 0.05 (SD), denoting statistically significant relationships. R2 values are all higher than 0.6, which is often considered the minimally accepted prediction precision for (environmental) risk assessment.136
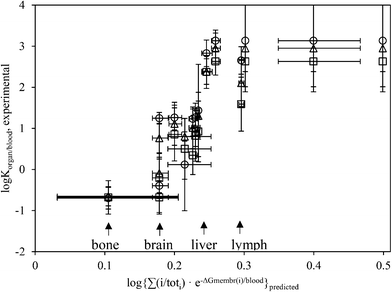 |
| Fig. 2 Predicted (x-axis, eqn (1)–(3)) vs. experiment-derived partitioning between organ tissue (Table 1) and blood of PEG-NPs (Cho et al.133 data, N = 42, eqn (4)). Circle ○ = 4 nm, triangle △ = 13 nm, square □ = 100 nm. Variance between same symbols due to difference in organ tissue composition (Table 1). Adipose tissue (log Kpred = 1.3) shown as 0.5. Horizontal error bars propagate variabilities in γ−membrane (Table 2); vertical error bars are 1SD based on 4 datapoints. ΔG in 2.303 RT. | |
The slope a of the linear regression (i.e., log
Korgan/blood,exp = a·log
Kpred + b) is approximately 15 ± 3 (2SD), significantly larger than 1. The offset b is −2 ± 1 (2SD). Regression fits (R2) were slightly higher for a log-logistic fit, as compared to a linear fit. As adipose tissue appears out of domain, it was not taken into account in regressions. Values for predicted K for partitioning of PEG-NPs from blood into skin and adipose tissue were relatively high, ≥0.4. Values for Kpred and Kexp for bone and brain were lowest. We did not observe any apparent outliers. Though we took data in Fig. 2 from 1 literature source,133 other sources134,137–139 show similar trends for PEG. eqn (1)–(3) correctly predict that albumin adsorption for cationic NPs is higher than for anionic NPs.134
log Korgan/blood,experimental = 15(±3)·log{∑(i/toti)·e−ΔGmembrane(i)/blood}predicted − (2 ± 1) |
4. Discussion
4.1. Energy considerations
Eqn (1)–(3) have a mechanistic basis. High/low K can be explained by many experimental phenomena. Cationic NPs (high γ+) are cleared from blood (hence, organs) faster than neutral or anionic NPs,137 presumably via enhanced binding to serum protein (high γ−). Eqn (3) predicts this, which constitutes a basis for tissue partitioning. Organs rich in phagocytes show enhanced K; indeed, NPs accumulate in lymph nodes.140 Instead of polar headgroups (AB interaction), NPs may interact with lipid tails (micelle-like system, involving differing γ (ref. 126 and 141)), as enhancing concentrations in adipose tissues (Fig. 2).
Across tissues, ΔG ranges from −4.4 to +5.1 kJ mol−1, a range of 9.5 kJ mol−1, equal to around 5–10 hydrogen (H) bonds. H-bonds need to be broken in order for surface molecules to interact. This number, ∼10 kJ mol−1, was associated to the difference between active and passive uptake mechanisms:142,143 cells with high positive ΔG (Table 3) take up PEG-NPs passively; cells with lower ΔG also take up PEG-NPs actively. The number of PEG chains on the (4 nm) NP surface would be ∼40,144–146 but a limited number need interact with biomembranes. Molecular initiating interaction events (MIE) between substance and biomolecule/system (e.g., ∼7 kJ mol−1 (ref. 147)) lead to outcome pathways. The MIE involves a limited/single functional group on the NP surface.
Table 3 Surface energies changes for adsorption interaction of albumin and PEG-NPs onto membranes biomaterials i, and for sorption of albumin onto PEG-NPs. Corresponding membrane-serum partitioning ratio K (eqn (2)) also shown. γwater→albuminNP = 4.2 mJ m−2
Though (e.g., lung) tissue contains only ∼1% phagocytes, these contain up to 83% of all (PEG) NPs in tissue.148 This implies a NP macrophage/tissue partitioning K = (100/[pha])·([NPtot]/[NPpha] − 1), i.e. (100/[1])·([100]/[83] − 1) = 20. This 20-fold enhancement matches higher receptor densities149 and activities150 of macrophages. Moving 1 mol of a substance across a 20-fold gradient at 25 °C is ΔG = (8.315 J mol−1 K−1)·(298 K)·ln(20/1) = 7.4 kJ mol−1.151 It is therefore unlikely that slope = 15, larger than 1 (Fig. 2), stems from inaccurate γ (eqn (1)–(3), Table 2). If our ΔG is fully precise and exact, slope (Fig. 2) should be 1 (according to Boltzmann). The difference between expected (1) and observed (∼15) may relate (partially) to unanticipated wrapping/bending or (geometry-)specific ligand–receptor energies152–154 contributing to γ, not reflected by Table 2, which may refine K. After phagocytosis, a cell minimizes its surface tension (γ) by smoothening.155
4.2. Cell signaling
Not the full NP surface area interacts with the biomembrane surface. Indeed, Kexp does not differ between NP sizes133(Fig. 2). Log-logistic fits are slightly better than linear fits between Kexp and Kpred (levelling off in Fig. 2), implying a crowding/shielding/saturation. This may refer to interaction area A (eqn (3)), which varies depending on strength of interaction (ΔG). ΔG depends on polymer size, but approaches (per monomer unit) zero at higher MW.156 Chemical potential of an atom/molecule depends on its surrounding, larger on convex surfaces than on flat surfaces, in turn larger than under concaves.157 While size/geometry can affect γ,158,159 interaction with serum/cytoplasmic constituents and geometric restrictions may offset the effect. The slope (∼15) is thus not a size-effect per se.
The slope (Fig. 2) may entail information on frequency, α in eqn (2) or i in eqn (1). Under steady state, it implies higher phagocytic activity. By analogy, in (eco)toxicology, IC50/EC50 values (in log-logistic curves) describe induction of biological response. Indeed, high (toxic) pressures instigate aggrupation of phagocytes (granuloma) at sites of NPs (increasing i/toti for phagocytes, eqn (1)). We assumed that one NP binds to/within one vesicle42,160 ignoring (intracellular) aggregation.33 This is sometimes not true: spleen phacocytes cluster (bioconcentrate) PEG-NPs in lysosomes;133 Kupffer macrophages engorge NP-aggregates.161 Aggregation changes the properties of the NP cluster.
A 10-fold increase in the average number of NPs per lysosome, implies an effective ‘bioconcentration factor’ of 10. Bioaccumulation (in organisms), rather, involves multiple uptake steps by different signaling pathways. Detecting bioactive substances enhances local internal concentrations.162–164 BAFs along (cell signaling94,165) pathways may be ∼100 times higher than BCF for the same substance.166 Bioaccumulation KBAF may be described as KBCF·KBCF·KBCF… etc., involving multiple concentrating steps (after the MIE).
Such steps may involve Ca2+, affecting lectin binding capacity,91,165,167 which γ (Table 2) not captures. Cancer cells lack Ca2+-dependent cadherin168–170 enhancing repulsion. Saline solutions effectuate different γ than pure water75,129 (eqn (3)). Amine-binding is key to pathogen detection and immune response,171–174 with electron-acceptor/donor interactions central to lectin binding to chitin (γLW = 41, γ+ = 1.3, γ− = 17.1 mJ m−2 (ref. 175)) via the N-acetyl group.176 Ca2+ complexation (bridging) affects its ΔG.177–180 This explains the high slope (Fig. 2) because Ca2+ is only relevant in those (i.e., immunological) tissues.181 For phagocytes, a decrease by Ca2+ in ΔGwater→membraneNP from 0 (Table 3) by a representative −25 kJ mol−1 (ref. 182 and 183) increases predicted log
K for e.g., the liver to ∼2.9, agreeing with experiment (2.4–2.8, Fig. 2).
4.3. Tissue inhomogeneities
Stronger correlations may imply a more homogeneous tissues or uniform binding mechanism. Inhomogeneities (e.g., layering) in tissues affect K (hence, R2, Fig. 2) via local increased exposures. Penetration of PEG-NPs through skin depends on hydration status.75,184,185 Mucus epithelial tissue (mouth/stomach) cells produce (N-)glycosylated proteins186 protecting organisms by binding (trapping) foreign material.187,188 This explains marked accumulation of NPs in (Ca2+-augmented) mucin (Table 3),189,190 having distinct γ (Table 1).
We cannot always assume the barriers in Fig. 1 are sufficiently low; inhibition of transport limits tissue partitioning.81 Macrophages (microglia) account for 10–15% of brain cells,191 and would readily take up NPs.192 However, the brain's blood vessels are lined with endothelial cells wedged tightly together, creating a boundary. Likewise, microvascular endothelial cells form the blood–spinal cord barrier; Sertoli cells constitute the blood–testis barrier. Pores sizes of ∼5 nm may complicate measuring a K in kidney.
We characterized each individual membrane surface (and serum protein) by a single γ set, implying that e.g., vesicles share the characteristics of cell surfaces, which combine during cytosis. γmembrane characterizes weighted averages of membrane components: lipids, receptors/proteins, counterions, etc. However, generic description of γ may not apply. Inhomogeneity in tissues is apparent from e.g. markedly different γ for bile in the liver (γLW = 23–26, γ+ = 36–46, γ− = 8–15 mJ m−2,193 and γ+ = 10–13, γ− = 35–41, γLW = 25–27 mJ m−2 (ref. 194)) and hydroxyapatite (γLW = 2.2, γ+ = 19.8, γ− = 73.2 mJ m−2 (ref. 195)), differing from Table 2. In reality, γmembrane differs across cell membranes. Pending (experimental) data, implementing distributions of γmembrane for inhomogeneous surfaces renders predictions more precise.
NP distribution depend on interaction with (intra)cellular/tissue compartments, other than in Table 1.196,197 A diversity of proteins in biological media198,199 may differentially functionalize NPs (and membranes) affecting γ, but was ignored. Tissue composition (Table 1) may be dependent on NP concentration, characterizable by healthy/affected tissues, in terms of phagocytes.200 Differences in body/organ weights and composition exist.163,201 We presume that inter-202 and intraspecies86 differences in lectin contribute to variance in NP distribution. Human physiology is not an exact science; assessments need customization. Standardization helps to benchmark exposures and tailor assessments.
4.4. Outlook and conclusion
Performance of eqn (1)–(3) is appreciable, R2 = 0.68, and statistically significant p < 0.00001 (2SD). In comparison, different labs monitoring in vivo concentration routinely yield a variability scatter of >20–40%.138 Variance in experimental log
K (e.g., 7 days or 6 months) is 20–100% depending on tissue.133 ∼70% of white blood cells are phagocytes, hence, representability of Table 1 entries introduces errors of ∼30%. Whether K reflects equilibrium (section 2.5) is uncertain due to experimental limits (colloids preventing dispersion). Barriers/inhomogeneities complicate in vivo measuring (local equilibria). K may increase/decrease via size-exclusion or immune response by aggrupation (clustering) of macrophages. Uncertain geometry of protein/vesicle/cell/tissue surfaces impacts γ, within 5%.203 Additional experiments on (de/at)tachment NPs aid assessment of tissue trafficking.
Depending on chemistry,103,204,205 lysosomes/autophagosomes206 degrade ionizable/polarizable NPs,26,207 affecting γ, but does not affect model results for inert PEG-NPs (Fig. 2). γ is a function of surface morphology (shape),155,208 but applicability to non-spherical NPs remains speculation, needing further study. Long-term exposures to various NPs/coatings (PbO, TiO2, QDs, C60, citrate61,209–214) show distributions similar to Fig. 2 and values, e.g. Kliver/blood ≈ 103,212 are comparable. Moreover, Fig. 3 ascertains flexibility of our ‘generic’ model by showing applicability to other NPs/biomolecules:
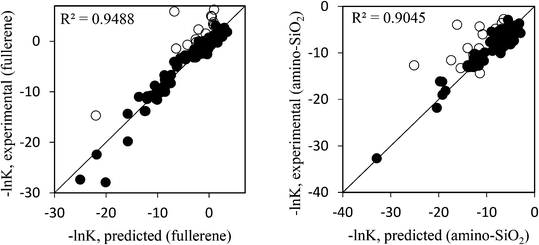 |
| Fig. 3 Experimental vs. predicted partitioning for 155 biomolecules between water and fullerene C60 (left) and amino-functionalized SiO2 NPs (right). Experimental values characterize partitioning of NPs onto (within) biomolecules and water. Data from ref. 215. Open symbols are (incompletely characterized mixtures of large) flexible molecules that minimize energy by molecular reorientation (oligonucleotides, small proteins). Interaction with C60 (γLW, γ+, γ− = 25, 2, 17 mJ m−2) via polarization and electron donation; with (cationic) SiO2–NH3 (γLW, γ+, γ− = 0, 50, 0.1 mJ m−2) via electron accepting. | |
Eqn (3) uses γ of NP and cell membrane (biomolecules), either experimental (if available) or predicted (i.e., computed80). We used γ (ref. 216 and 217) to describe adhesion of surfaces, avoiding shortcomings of Kow.17 Relationships (Fig. 2) depend on standardizing doses/exposures and sample processing (tissue-specific digestion/fixation/drying rates). γ (Table 2) is subject to test liquids (analogous to octanol). Surface energy components (γLW, γAB) describe small organic compounds (e.g., polar surface area); cations are electron-poor Lewis acids, anions are electron-rich Lewis donors. For NP surfaces with limited/uniform polarizability, we can obtain γLWNP, γ+NP and γ−NP from partial charged surface area/density.40,80 This unifies descriptions for NPs and small organic molecules:218 both γ for NPs and charged surface areas of small molecules drive their partitioning; both find use in risk assessment.214,219,220
Our calculus for various NP types successfully yields partitioning in(to) many tissues/organs, by cells40,81 and biomolecules. These data find use in PBPK modeling with extensions via scaling.201,214,221 Existing PBPK models parametrize partitioning without (much) regard for mechanism:222 higher K in non-phagocytotic organ tissues.223 Ideally, all parameters ought be mechanism-based to allow extrapolation across NPs/tissues. To our knowledge, a theoretical framework did not yet exist for tissue partitioning of NPs. We are happy to attribute complex behaviors to simple properties and traits and expand concepts for small molecules to NPs. Regressions between predicted/experimental K are useful to obtain tissue partitioning without experiments. We instigated future research for implementing biodistributions of particles in medical and toxicological applications.
Conflicts of interest
There are no conflicts to declare.
References
- K. McNamara and S. A. M. Tofail, Nanoparticles in biomedical applications, Adv. Phys.: X, 2017, 2, 54–88 CAS.
- S. Ko and C. Huh, Use of nanoparticles for oil production applications, J. Pet. Sci. Eng., 2019, 172, 97–114 CrossRef CAS.
- S. L. Zhang, H. J. Gao and G. Bao, Physical Principles of Nanoparticle Cellular Endocytosis, ACS Nano, 2015, 9, 8655–8671 CrossRef CAS PubMed.
- G. Baidya, R. Tiwary, M. Mudassir, N. Singh, S. Saha, K. Chosdol, S. Sinha and P. Chattopadhyay, Passive internalization and active extrusion determines PLGA-nanoparticle concentration in cancer cell lines, Nanomedicine, 2020, 15, 2229–2240 CrossRef CAS PubMed.
- R. Deng, D. H. Lin, L. Z. Zhu, S. Majumdar, J. C. White, J. L. Gardea-Torresdey and B. S. Xing, Nanoparticle interactions with co-existing contaminants: joint toxicity, bioaccumulation and risk, Nanotoxicology, 2017, 11, 591–612 CrossRef CAS PubMed.
- A. M. Malvandi, S. Shahba, A. Mohammadipour, S. H. Rastegar-Moghaddam and M. Abudayyak, Cell and molecular toxicity of lanthanum nanoparticles: are there possible risks to humans?, Nanotoxicology, 2021, 15, 951–972 Search PubMed.
- W. Poon, Y. N. Zhang, B. Ouyang, B. R. Kingston, J. L. Y. Wu, S. Wilhelm and W. C. W. Chan, Elimination Pathways of Nanoparticles, ACS Nano, 2019, 13, 5785–5798 CrossRef CAS PubMed.
- N. Oh and J. H. Park, Endocytosis and exocytosis of nanoparticles in mammalian cells, Int. J. Nanomed., 2014, 9, 51–63 Search PubMed.
- M. T. Zhu, G. J. Nie, H. Meng, T. Xia, A. Nel and Y. L. Zhao, Physicochemical Properties Determine Nanomaterial Cellular Uptake, Transport, and Fate, Acc. Chem. Res., 2013, 46, 622–631 CrossRef CAS PubMed.
- A. J. Hendriks, A. van der Linde, G. Cornelissen and D. Sijm, The power of size. 1. Rate constants and equilibrium ratios for accumulation of organic substances related to octanol-water partition ratio and species weight, Environ. Toxicol. Chem., 2001, 20, 1399–1420 CrossRef CAS PubMed.
- Q. Y. Bao, A. Y. Liu, Y. Ma, H. Chen, J. Hong, W. B. Shen, C. Zhang and Y. Ding, The effect of oil-water partition coefficient on the distribution and cellular uptake of liposome-encapsulated gold nanoparticles, Colloids Surf., B, 2016, 146, 475–481 CrossRef CAS PubMed.
- Y. F. Gao, Z. C. Xie, J. F. Feng, W. Q. Ma and L. Zhu, Different factors determined the toxicokinetics of organic chemicals and nanomaterials exposure to zebrafish (Danio Rerio), Ecotoxicol. Environ. Saf., 2019, 186, 109810 CrossRef CAS PubMed.
- J. Q. Wang, R. P. J. Hoondert, N. W. Thunnissen, D. van de Meent and A. J. Hendriks, Chemical fate of persistent organic pollutants in the arctic: Evaluation of simplebox, Sci. Total Environ., 2020, 720, 137579 CrossRef CAS PubMed.
- B. A. M. Bandowe, M. Bigalke, J. Kobza and W. Wilcke, Sources and fate of polycyclic aromatic compounds (PAHs, oxygenated PAHs and azaarenes) in forest soil profiles opposite of an aluminium plant, Sci. Total Environ., 2018, 630, 83–95 CrossRef CAS PubMed.
- Y. Zhang, X. J. Luo, L. Mo, J. P. Wu, B. X. Mai and Y. H. Peng, Bioaccumulation and translocation of polyhalogenated compounds in rice (Oryza sativa L.) planted in paddy soil collected from an electronic waste recycling site, South China, Chemosphere, 2015, 137, 25–32 CrossRef CAS PubMed.
- E. Price and A. J. Gesquiere, An in vitro assay and artificial intelligence approach to determine rate constants of nanomaterial-cell interactions, Sci. Rep., 2019, 9, 1394 CrossRef PubMed.
- A. Praetorius, N. Tufenkji, K. U. Goss, M. Scheringer, F. von der Kammer and M. Elimelech, The road to nowhere: equilibrium partition coefficients for nanoparticles, Environ. Sci.: Nano, 2014, 1, 317–323 RSC.
- M. Crane, R. D. Handy, J. Garrod and R. Owen, Ecotoxicity test methods and environmental hazard assessment for engineered nanoparticles, Ecotoxicology, 2008, 17, 421–437 CrossRef CAS PubMed.
-
W. Pauli, God made the bulk; surfaces were invented by the devil, As quoted in Growth, Dissolution, and Pattern Formation in Geosystems, ed. B. Jamtveit and P. Meakin, 1999, p. 291 Search PubMed.
- A. I. Khan, Q. Lu, D. Du, Y. H. Lin and P. Dutta, Quantification of kinetic rate constants for transcytosis of polymeric nanoparticle through blood-brain barrier, Biochim. Biophys. Acta, Gen. Subj., 2018, 1862, 2779–2787 CrossRef CAS PubMed.
- S. Ohta, S. Inasawa and Y. Yamaguchi, Real time observation and kinetic modeling of the cellular uptake and removal of silicon quantum dots, Biomaterials, 2012, 33, 4639–4645 CrossRef CAS PubMed.
- W. B. Chen, D. Z. D'Argenio, A. Sipos, K. J. Kim and E. D. Crandall, Biokinetic modeling of nanoparticle interactions with lung alveolar epithelial cells: uptake, intracellular processing, and egress, Am. J. Physiol. Regul. Integr. Comp. Physiol., 2021, 320, R36–R43 CrossRef CAS PubMed.
- N. W. van den Brink, A. Jemec Kokalj, P. V. Silva, E. Lahive, K. Norrfors, M. Baccaro, Z. Khodaparast, S. Loureiro, D. Drobne, G. Cornelis, S. Lofts, R. D. Handy, C. Svendsen, D. Spurgeon and C. A. M. van Gestel, Tools and rules for modelling uptake and bioaccumulation of nanomaterials in invertebrate organisms, Environ. Sci.: Nano, 2019, 6, 1985 RSC.
- J. Mosquera, I. Garcia and L. M. Liz-Marzan, Cellular Uptake of Nanoparticles versus Small Molecules: A Matter of Size, Acc. Chem. Res., 2018, 51, 2305–2313 CrossRef CAS PubMed.
- P. Ruenraroengsak, P. Novak, D. Berhanu, A. J. Thorley, E. Valsami-Jones, J. Gorelik, Y. E. Korchev and T. D. Tetley, Respiratory epithelial cytotoxicity and membrane damage (holes) caused by amine-modified nanoparticles, Nanotoxicology, 2012, 6, 94–108 CrossRef CAS PubMed.
- E. Frohlich, Cellular elimination of nanoparticles, Environ. Toxicol. Pharmacol., 2016, 46, 90–94 CrossRef PubMed.
- E. Silva, L. Barreiros, M. A. Segundo, S. A. C. Lima and S. Reis, Cellular interactions of a lipid-based nanocarrier model with human keratinocytes: Unravelling transport mechanisms, Acta Biomater., 2017, 53, 439–449 CrossRef CAS PubMed.
- P. Nativo, I. A. Prior and M. Brust, Uptake and intracellular fate of surface-modified gold nanoparticles, ACS Nano, 2008, 2, 1639–1644 CrossRef CAS PubMed.
- V. Marchesano, Y. Hernandez, W. Salvenmoser, A. Ambrosone, A. Tino, B. Hobmayer, J. M. de la Fuente and C. Tortiglione, Imaging Inward and Outward Trafficking of Gold Nanoparticles in Whole Animals, ACS Nano, 2013, 7, 2431–2442 CrossRef CAS PubMed.
- H. E. Chung, D. H. Park, J. H. Choy and S. J. Choi, Intracellular trafficking pathway of layered double hydroxide nanoparticles in human cells: Size-dependent cellular delivery, Appl. Clay Sci., 2012, 65–66, 24–30 CrossRef CAS.
- M. Geiser, B. Rothen-Rutishauser, N. Kapp, S. Schurch, W. Kreyling, H. Schulz, M. Semmler, V. I. Hof, J. Heyder and P. Gehr, Ultrafine particles cross cellular membranes by nonphagocytic mechanisms in lungs and in cultured cells, Environ. Health Perspect., 2005, 113, 1555–1560 CrossRef PubMed.
- X. W. Wang, Y. H. Qiu, M. Y. Wang, C. H. Zhang, T. S. Zhang, H. M. Zhou, W. X. Zhao, W. L. Zhao, G. M. Xia and R. G. Shao, Endocytosis and Organelle Targeting of Nanomedicines in Cancer Therapy, Int. J. Nanomed., 2020, 15, 9447–9467 CrossRef CAS PubMed.
- B. D. Chithrani and W. C. W. Chan, Elucidating the mechanism of cellular uptake and removal of protein-coated gold nanoparticles of different sizes and shapes, Nano Lett., 2007, 7, 1542–1550 CrossRef CAS PubMed.
- A. Sipos, K. J. Kim, R. H. Chow, P. Flodby, Z. Borok and E. D. Crandall, Alveolar epithelial cell processing of nanoparticles activates autophagy and lysosomal exocytosis, Am. J. Physiol. Lung Cell. Mol. Physiol., 2018, 315, L286–L300 CrossRef CAS PubMed.
- P. Foroozandeh and A. A. Aziz, Insight into Cellular Uptake and Intracellular Trafficking of Nanoparticles, Nanoscale Res. Lett., 2018, 13, 33 CrossRef PubMed.
- H. Deng, P. Dutta and J. Liu, Stochastic modeling of nanoparticle internalization and expulsion through receptor-mediated transcytosis, Nanoscale, 2019, 11, 11227–11235 RSC.
- H. Y. Tang, H. F. Ye, H. W. Zhang and Y. G. Zheng, Aggregation of nanoparticles regulated by mechanical properties of nanoparticle- membrane system, Nanotechnology, 2018, 29(40), 405102 CrossRef PubMed.
- A. Dey, J. Stenberg, P. Dandekar and R. Jain, A combinatorial study of experimental analysis and mathematical modeling: How do chitosan nanoparticles deliver therapeutics into cells?, Carbohydr. Polym., 2020, 229, 115437 CrossRef CAS PubMed.
- V. P. Zhdanov, Interpretation of amperometric kinetics of content release during contacts of vesicles with a lipid membrane, Eur. Biophys. J., 2017, 46, 461–470 CrossRef CAS PubMed.
- B. Q. Lu, A. J. Hendriks and T. M. Nolte, A generic model based on the properties of nanoparticles and cells for predicting cellular uptake, Colloids Surf., B, 2022, 209(1), 112155 CrossRef CAS PubMed.
- A. Bigdeli, M. R. Hormozi-Nezhad and H. Parastar, Using nano-QSAR to determine the most responsible factor(s) in gold nanoparticle exocytosis, RSC Adv., 2015, 5, 57030–57037 RSC.
- P. Rees, J. W. Wills, M. R. Brown, C. M. Barnes and H. D. Summers, The origin of heterogeneous nanoparticle uptake by cells, Nat. Commun., 2019, 10, 2341 CrossRef PubMed.
- J. Vitte, A. M. Benoliel, A. Pierres and P. Bongrand, Is there a predictable relationship between surface physical-chemical properties and cell behavious at the interface?, Eur. Cells Mater., 2004, 7, 52–63 CrossRef CAS PubMed.
-
J. Da Silva Domingues, Macrophage-mediated phagocytosis of bacteria adhering on biomaterial surfaces, PhD, University of Groningen, 2014, ch. III. Phagocytosis of bacteria adhering to a biomaterial surface in a surface thermodynamic perspective Search PubMed.
- C. H. Achebe, S. Iweriolor and J. L. Chukwuneke, Surface Energetics Study and Determination of the Combined Negative Hamaker Coefficient for Hepatitis C Virus Infected Human Blood Cells, J. Biomed. Sci. Eng., 2018, 11, 307–319 CrossRef CAS.
- I. Lynch, A. Ahluwalia, D. Boraschi, H. J. Byrne, B. Fadeel, P. Gehr, A. C. Gutleb, M. Kendall and M. G. Papadopoulos, The bio-nano-interface in predicting nanoparticle fate and behaviour in living organisms: towards grouping and categorising nanomaterials and ensuring nanosafety by design, BioNanoMat, 2013, 14, 195–216 Search PubMed.
- Y. Y. Liu, Q. Chang, Z. X. Sun, J. Liu, X. Y. Deng, Y. F. Liu, A. N. Cao and H. F. Wang, Fate of CdSe/ZnS quantum dots in cells: Endocytosis, translocation and exocytosis, Colloids Surf., B, 2021, 208, 112140 CrossRef CAS PubMed.
- A. Becker, B. K. Thakur, J. M. Weiss, H. S. Kim, H. Peinado and D. Lyden, Extracellular Vesicles in Cancer: Cell-to-Cell Mediators of Metastasis, Cancer Cell, 2016, 30, 836–848 CrossRef CAS PubMed.
- R. Szatanek and M. Baj-Krzyworzeka, CD44 and Tumor-Derived Extracellular Vesicles (TEVs). Possible Gateway to Cancer Metastasis, Int. J. Mol. Sci., 2021, 22(3), 1463 CrossRef CAS PubMed.
- E. M. Guerreiro, R. Ovstebo, B. Thiede, D. E. Costea, T. M. Soland and H. Kanli Galtung, Cancer cell line-specific protein profiles in extracellular vesicles identified by proteomics, PLoS One, 2020, 15, e0238591 CrossRef CAS PubMed.
- W. Tian, N. Lei, J. Zhou, M. Chen, R. Guo, B. Qin, Y. Li and L. Chang, Extracellular vesicles in ovarian cancer chemoresistance, metastasis, and immune evasion, Cell Death Dis., 2022, 13, 64 CrossRef CAS PubMed.
- K. Wu, F. Xing, S. Y. Wu and K. Watabe, Extracellular vesicles as emerging targets in cancer: Recent development from bench to bedside, Biochim. Biophys. Acta, Rev. Cancer, 2017, 1868, 538–563 CrossRef CAS PubMed.
- R. Pethig and D. B. Kell, The passive electrical properties of biological systems: their significance in physiology, biophysics and biotechnology, Phys. Med. Biol., 1987, 32, 933–970 CrossRef CAS PubMed.
- E. Pandey, A. S. Nour and E. N. Harris, Prominent Receptors of Liver Sinusoidal Endothelial Cells in Liver Homeostasis and Disease, Front. Physiol., 2020, 11, 873 CrossRef PubMed.
- D. V. Haute and J. M. Berlin, Challenges in realizing selectivity for nanoparticle biodistribution and clearance: lessons from gold nanoparticles, Ther. Delivery, 2017, 8, 763–774 CrossRef PubMed.
- C. I. Colino, J. M. Lanao and C. Gutierrez-Millan, Targeting of Hepatic Macrophages by Therapeutic Nanoparticles, Front. Immunol., 2020, 11, 218 CrossRef CAS PubMed.
- M. Bilzer, F. Roggel and A. L. Gerbes, Role of Kupffer cells in host defense and liver disease, Liver Int., 2006, 26, 1175–1186 CrossRef CAS PubMed.
- L. Bouwens, M. Baekeland, R. De Zanger and E. Wisse, Quantitation, tissue distribution and proliferation kinetics of Kupffer cells in normal rat liver, Hepatology, 1986, 6, 718–722 CrossRef CAS PubMed.
- A. K. Sohlenius-Sternbeck, Determination of the hepatocellularity number for human, dog, rabbit, rat and mouse livers from protein concentration measurements, Toxicol. In Vitro, 2006, 20, 1582–1586 CrossRef CAS PubMed.
- Z. E. Wilson, A. Rostami-Hodjegan, J. L. Burn, A. Tooley, J. Boyle, S. W. Ellis and G. T. Tucker, Inter-individual variability in levels of human microsomal protein and hepatocellularity per gram of liver, Br. J. Clin. Pharmacol., 2003, 56, 433–440 CrossRef CAS PubMed.
- M. Aborig, P. R. V. Malik, S. Nambiar, P. Chelle, J. Darko, A. Mutsaers, A. N. Edginton, A. Fleck, E. Osei and S. Wettig, Biodistribution and Physiologically-Based Pharmacokinetic Modeling of Gold Nanoparticles in Mice with Interspecies Extrapolation, Pharmaceutics, 2019, 11(4), 179 CrossRef CAS PubMed.
- Q. Gu, H. Yang and Q. Shi, Macrophages and bone inflammation, J. Orthop. Translat., 2017, 10, 86–93 CrossRef PubMed.
- E. L. Orr, J. E. Aschenbrenner, L. X. Oakford, F. L. Jackson and N. C. Stanley, Changes in brain and spinal cord water content during recurrent encephalomyelitis in female lewis rats, Mol. Chem. Neuropathol., 1994, 22, 185 CrossRef CAS PubMed.
- A. V. Leonard, E. Thornton and R. Vink, NK1 Receptor Blockade Is Ineffective in Improving Outcome following a Balloon Compression Model of Spinal Cord Injury, PLoS One, 2014, 9, e98364 CrossRef PubMed.
-
C. E. Pochedly, Disorders of the Spleen: Pathophysiology and Management, Informa Health Care, 1989, pp. 7–15, ISBN 0–8247–7933-9 Search PubMed.
- M. Gualdron-Lopez, M. Diaz-Varela, H. Toda, I. Aparici-Herraiz, L. Pedro-Cos, R. Lauzurica, M. V. G. Lacerda, M. A. Fernandez-Sanmartin, C. Fernandez-Becerra and H. A. Del Portillo, Multiparameter Flow Cytometry Analysis of the Human Spleen Applied to Studies of Plasma-Derived EVs From Plasmodium vivax Patients, Front. Cell. Infect. Microbiol., 2021, 11, 596104 CrossRef CAS PubMed.
- K. Lee, K. Jeoung, S. H. Kim, Y. B. Ji, H. Son, Y. Choi, Y. M. Huh, J. S. Suh and S. J. Oh, Measuring water contents in animal organ tissues using terahertz spectroscopic imaging, Biomed, Opt. Express, 2018, 9, 1582–1589 CrossRef CAS PubMed.
- G. Attina, S. Triarico, A. Romano, P. Maurizi, S. Mastrangelo and A. Ruggiero, Role of Partial Splenectomy in Hematologic Childhood Disorders, Pathogens, 2021, 10(11), 1436 CrossRef PubMed.
- B. Button, H. P. Goodell, E. Atieh, Y. C. Chen, R. Williams, S. Shenoy, E. Lackey, N. T. Shenkute, L. H. Cai, R. G. Dennis, R. C. Boucher and M. Rubinstein, Roles of mucus adhesion and cohesion in cough clearance, Proc. Natl. Acad. Sci. U. S. A., 2018, 115, 12501–12506 CrossRef CAS PubMed.
-
L. Dean, Blood Groups and Red Cell Antigens [Internet], National Center for Biotechnology Information (US), Bethesda (MD), 2005, Table 1, Complete blood count, Available from: https://www.ncbi.nlm.nih.gov/books/NBK2263/table/ch1.T1/ Search PubMed.
- V. Bronte and M. J. Pittet, The spleen in local and systemic regulation of immunity, Immunity, 2014, 39, 806–818 CrossRef PubMed.
- J. van de Wouw and J. A. Joles, Albumin is an interface between blood plasma and cell membrane, and not just a sponge, Clin. Kidney J., 2022, 15, 624–634 CrossRef CAS PubMed.
- P. Aggarwal, J. B. Hall, C. B. McLeland, M. A. Dobrovolskaia and S. E. McNeil, Nanoparticle interaction with plasma proteins as it relates to particle biodistribution, biocompatibility and therapeutic efficacy, Adv. Drug Delivery Rev., 2009, 61, 428–437 CrossRef CAS PubMed.
- P. L. Michael, Y. T. Lam, J. Hung, R. P. Tan, M. Santos and S. G. Wise, Comprehensive Evaluation of the Toxicity and Biosafety of Plasma Polymerized Nanoparticles, Nanomaterials, 2021, 11(5), 1176 CrossRef CAS PubMed.
- M. Zobel, Observing structural reorientations at solvent–nanoparticle interfaces by X-ray diffraction – putting water in the spotlight, Acta Crystallogr., Sect. A: Found. Adv., 2016, 72, 621–631 CrossRef CAS PubMed.
- A. H. Bahrami, R. Lipowsky and T. R. Weikl, The role of membrane curvature for the wrapping of nanoparticles, Soft Matter, 2016, 12, 581–587 RSC.
- R. T. Myers, True molar surface energy and alignment of surface molecules, J. Colloid Interface Sci., 2004, 274, 229–236 CrossRef CAS PubMed.
- T. M. Nolte, K. Kettler, J. A. J. Meesters, A. J. Hendriks and D. van de Meent, A Semi-Empirical Model for Transport of Inorganic Nanoparticles across a Lipid Bilayer: Implications for Uptake by Living Cells, Environ. Toxicol. Chem., 2015, 34, 488–496 CrossRef CAS PubMed.
- C. J. Vanoss, M. K. Chaudhury and R. J. Good, Monopolar Surfaces, Adv. Colloid Interface Sci., 1987, 28, 35–64 CrossRef CAS PubMed.
- B. Lu, A. Jan Hendriks and T. M. Nolte, A generic model based on the properties of nanoparticles and cells for predicting cellular uptake, Colloids Surf., B, 2022, 209, 112155 CrossRef CAS PubMed.
- B. Lu, J. Wang, P. T. J. Scheepers, A. J. Hendriks and T. M. Nolte, Generic prediction of exocytosis rate constants by size-based surface energies of nanoparticles and cells, Sci. Rep., 2022, 12, 17813 CrossRef CAS PubMed.
- C. J. van Oss, Long-range and short-range mechanisms of hydrophobic attraction and hydrophilic repulsion in specific and aspecific interactions, J. Mol. Recognit., 2003, 16, 177–190 CrossRef CAS PubMed.
-
G. Cooper, The Cell: A Molecular Approach, Structure of the Plasma Membrane, Sinauer Associates, Sunderland (MA), 2nd edn, 2000 Search PubMed.
- D. Snary, A. K. Allen, R. A. Faulkes and M. J. Crumpton, Carbohydrate composition of lymphocyte plasma membrane from pig mesenteric lymph node, Biochem. J., 1976, 153, 75–78 CrossRef CAS PubMed.
- P. J. Quinn and D. Chapman, The dynamics of membrane structure, Crit. Rev. Biochem., 1980, 8, 1–117 CrossRef CAS PubMed.
- D. Bladier, G. Perret, J. Baudelot and P. Cornillot, Carbohydrate content of human erythrocyte membrane, Variations with ABO-blood group, Hoppe-Seyler's Z. Physiol. Chem., 1979, 360, 501–506 CrossRef CAS PubMed.
- S. R. Pasricha, The red cell membrane, part 1: the role of the red cell membrane, Clin. Adv. Hematol. Oncol., 2014, 12, 533–535 Search PubMed.
- R. Tazhitdinova and A. V. Timoshenko, The Emerging Role of Galectins and O-GlcNAc Homeostasis in Processes of Cellular Differentiation, Cell, 2020, 9(8), 1792 CrossRef CAS PubMed.
- R. Uematsu, Y. Shinohara, H. Nakagawa, M. Kurogochi, J. Furukawa, Y. Miura, M. Akiyama, H. Shimizu and S. Nishimura, Glycosylation specific for adhesion molecules in epidermis and its receptor revealed by glycoform-focused reverse genomics, Mol. Cell. Proteomics, 2009, 8, 232–244 CrossRef CAS PubMed.
- C. D. Raposo, A. B. Canelas and M. T. Barros, Human Lectins, Their Carbohydrate Affinities and Where to Find Them, Biomolecules, 2021, 11(2), 188 CrossRef CAS PubMed.
- E. J. Veldhuizen, M. van Eijk and H. P. Haagsman, The carbohydrate recognition domain of collectins, FEBS J., 2011, 278, 3930–3941 CrossRef CAS PubMed.
- R. S. Haltiwanger, M. A. Lehrman, A. E. Eckhardt and R. L. Hill, The distribution and localization of the fucose-binding lectin in rat tissues and the identification of a high affinity form of the mannose/N-acetylglucosamine-binding lectin in rat liver, J. Biol. Chem., 1986, 261, 7433–7439 CrossRef CAS PubMed.
- Y. Zhang, X. Liu, J. Zhao, J. Wang, Q. Song and C. Zhao, The phagocytic receptors of beta-glucan, Int. J. Biol. Macromol., 2022, 205, 430–441 CrossRef CAS PubMed.
- A. M. Kerrigan and G. D. Brown, Phagocytes: Fussy about Carbs, Curr. Biol., 2011, 21, R500–R502 CrossRef CAS PubMed.
- M. Lech, H. E. Susanti, C. Rommele, R. Grobmayr, R. Gunthner and H. J. Anders, Quantitative expression of C-type lectin receptors in humans and mice, Int. J. Mol. Sci., 2012, 13, 10113–10131 CrossRef CAS PubMed.
- A. Dominguez-Soto, L. Aragoneses-Fenoll, F. Gomez-Aguado, M. T. Corcuera, J. Claria, C. Garcia-Monzon, M. Bustos and A. L. Corbi, The pathogen receptor liver and lymph node sinusoidal endotelial cell C-type lectin is expressed in human Kupffer cells and regulated by PU.1, Hepatology, 2009, 49, 287–296 CrossRef CAS PubMed.
- R. Lotan and A. Raz, Lectins in cancer cells, Ann. N. Y. Acad. Sci., 1988, 551, 385–396 CrossRef CAS PubMed , discussion 396-388.
- S. C. Tao, Y. Li, J. Zhou, J. Qian, R. L. Schnaar, Y. Zhang, I. J. Goldstein, H. Zhu and J. P. Schneck, Lectin microarrays identify cell-specific and functionally significant cell surface glycan markers, Glycobiology, 2008, 18, 761–769 CrossRef CAS PubMed.
- Y. K. Jeon, Y. H. Jang, D. R. Yoo, S. N. Kim, S. K. Lee and M. J. Nam, Mesenchymal stem cells' interaction with skin: Wound-healing effect on fibroblast cells and skin tissue, Wound Repair Regen., 2010, 18, 655–661 CrossRef PubMed.
- Y. Wang, M. Nakagawa, I. Garitaonandia, I. Slavin, G. Altun, R. M. Lacharite, K. L. Nazor, H. T. Tran, C. L. Lynch, T. R. Leonardo, Y. Liu, S. E. Peterson, L. C. Laurent, S. Yamanaka and J. F. Loring, Specific lectin biomarkers for isolation of human pluripotent stem cells identified through array-based glycomic analysis, Cell Res., 2011, 21(11), 1551–1563 CrossRef CAS PubMed.
- Y. Kato, S. Ozawa, C. Miyamoto, Y. Maehata, A. Suzuki, T. Maeda and Y. Baba, Acidic extracellular microenvironment and cancer, Cancer Cell Int., 2013, 13, 89 CrossRef CAS PubMed.
- M. Gindl and S. Tscheg, Significance of the Acidity of Wood to the Surface Free Energy Components of Different Wood Species, Langmuir, 2002, 18, 3209–3212 CrossRef CAS.
- J. P. Li, Y. S. Wang, C. Q. Xu, Q. W. Yu, X. H. Wang, H. B. Xie, L. F. Tian, Y. Qiu, R. Guo, Z. Z. Lu, M. Li and Q. He, Rapid pH-responsive self-disintegrating nanoassemblies balance tumor accumulation and penetration for enhanced anti-breast cancer therapy, Acta Biomater., 2021, 134, 546–558 CrossRef CAS PubMed.
- Z. W. Liu, F. M. Wang, X. P. Liu, Y. J. Sang, L. Zhang, J. S. Ren and X. G. Qu, Cell membrane-camouflaged liposomes for tumor cell-selective glycans engineering and imaging in vivo, Proc. Natl. Acad. Sci. U. S. A., 2021, 118(30), e2022769118 CrossRef CAS PubMed.
- R. Minnes, M. Nissinmann, Y. Maizels, G. Gerlitz, A. Katzir and Y. Raichlin, Using Attenuated Total Reflection-Fourier Transform Infra-Red (ATR-FTIR) spectroscopy to distinguish between melanoma cells with a different metastatic potential, Sci. Rep., 2017, 7(1), 4381 CrossRef PubMed.
- L. Q. Jiang, T. Y. Wang, T. J. Webster, H. J. Duan, J. Y. Qiu, Z. M. Zhao, X. X. Yin and C. L. Zheng, Intracellular disposition of chitosan nanoparticles in macrophages: intracellular uptake, exocytosis, and intercellular transport, Int. J. Nanomed., 2017, 12, 6383–6398 CrossRef CAS PubMed.
- L. Y. T. Chou, K. Zagorovsky and W. C. W. Chan, DNA assembly of nanoparticle superstructures for controlled biological delivery and elimination, Nat. Nanotechnol., 2014, 9, 148–155 CrossRef CAS PubMed.
- M. S. Dukhinova, A. Y. Prilepskii, A. A. Shtil and V. V. Vinogradov, Metal Oxide Nanoparticles in Therapeutic Regulation of Macrophage Functions, Nanomaterials, 2019, 9(11), 1631 CrossRef CAS PubMed.
- A. S. Haka, I. Grosheva, E. Chiang, A. R. Buxbaum, B. A. Baird, L. M. Pierini and F. R. Maxfield, Macrophages create an acidic extracellular hydrolytic compartment to digest aggregated lipoproteins, Mol. Biol. Cell, 2009, 20, 4932–4940 CrossRef CAS PubMed.
- G. L. Lukacs, O. D. Rotstein and S. Grinstein, Determinants of the Phagosornal pH in Macrophages, J. Biol. Chem., 1991, 266, 24540–24548 CrossRef CAS PubMed.
- J. F. Danielli, The relations between surface pH, ion concentrations and interfacial tension, Proc. R. Soc. B, 1937, 122, 155–174 CAS.
- H. Wu, Y. Yin, X. Hu, C. Peng, Y. Liu, Q. Li, W. Huang and Q. Huang, Effects of Environmental pH on Macrophage Polarization and Osteoimmunomodulation, ACS Biomater. Sci. Eng., 2019, 5, 5548–5557 CrossRef CAS PubMed.
-
H. G. Karge, 3.2.4.1 Concepts and Analysis of Surface Acidity and Basicity, in Handbook of Heterogeneous Catalysis: Online, 2008 Search PubMed.
- Z. Pawlak, A. D. Petelska, W. Urbaniak, K. Q. Yusuf and A. Oloyede, Relationship Between Wettability and Lubrication Characteristics of the Surfaces of Contacting Phospholipid-Based Membranes, Cell Biochem. Biophys., 2013, 65, 335–345 CrossRef CAS PubMed.
- C. A. Mattacks, D. Sadler and C. M. Pond, The cellular structure and lipid/protein composition of adipose tissue surrounding chronically stimulated lymph nodes in rats, J. Anat., 2003, 202, 551–561 CrossRef CAS PubMed.
- S. Murdan, C. Poojary, D. R. Patel, J. Fernandes, A. Haman, P. S. Saundh and Z. Sheikh, In vivo measurement of the surface energy of human fingernail plates, Int. J. Cosmet. Sci., 2012, 34, 257–262 CrossRef CAS PubMed.
- F. Eudier, G. Savary, M. Grisel and C. Picard, Skin surface physico-chemistry: Characteristics, methods of measurement, influencing factors and future developments, Adv. Colloid Interface Sci., 2019, 264, 11–27 CrossRef CAS PubMed.
-
M. J. Hill, Surface and Colloidal Approach to Endothelial Cell-Segmental Polyurethane Interactions, Doctor of Philosophy, The University at Buffalo, State University of New York, 2017 Search PubMed.
- D. Yongabi, M. Khorshid, A. Gennaro, S. Jooken, S. Duwe, O. Deschaume, P. Losada-Perez, P. Dedecker, C. Bartic, M. Wubbenhorst and P. Wagner, QCM-D Study of Time-Resolved Cell Adhesion and Detachment: Effect of Surface Free Energy
on Eukaryotes and Prokaryotes, ACS Appl. Mater. Interfaces, 2020, 12, 18258–18272 CrossRef CAS PubMed.
- L. De Bartolo, S. Morelli, A. Bader and E. Drioli, Evaluation of cell behaviour related to physico-chemical properties of polymeric membranes to be used in bioartificial organs, Biomaterials, 2002, 23, 2485–2497 CrossRef CAS PubMed.
- D. Aronov, R. Rosen, E. Z. Ron and G. Rosenman, Electron-induced surface modification of hydroxyapatite-coated implant, Surf. Coat. Technol., 2008, 202, 2093–2102 CrossRef CAS.
- E. M. Harnett, J. Alderman and T. Wood, The surface energy of various biomaterials coated with adhesion molecules used in cell culture, Colloids Surf., B, 2007, 55, 90–97 CrossRef CAS PubMed.
- B. B. Hole, D. S. Keller, W. M. Burry and J. A. Schwarz, Surface energetics of bone mineral and synthetic hydroxyapatite using inverse gas chromatography, J. Chromatogr., B, 2011, 879, 1847–1850 CrossRef CAS PubMed.
- J. Biggemann, P. Muller, D. Kollner, S. Simon, P. Hoffmann, P. Heik, J. H. Lee and T. Fey, Hierarchical Surface Texturing of Hydroxyapatite Ceramics: Influence on the Adhesive Bonding Strength of Polymeric Polycaprolactone, J. Funct. Biomater., 2020, 11(4), 73 CrossRef CAS PubMed.
-
H. J. J. Cho, Surface Tension and Electroporation of Lipid Bilayers, Master of Science, Massachusetts Institute of Technology, 2011 Search PubMed.
- E. Chibowski and M. Jurak, Interaction energy of model lipid membranes with water and diiodomethane, Colloids Surf., A, 2011, 383, 56–60 CrossRef CAS.
-
I. Yildirim, Surface Free Energy Characterization of Powders, Doctor of Philosophy, Virginia Polytechnic Institute and State University, 2001, ch. 1 Search PubMed.
- Z. Lin, D. Ba and C. M. Liu, Surface Energy and Work of Adhesion of Titania-related Materials, Phys. Procedia, 2012, 32, 580–589 CrossRef CAS.
- M. Rillosi and G. Buckton, Modelling mucoadhesion by use of surface energy terms obtained by the Lewis acid-Lewis base approach, Int. J. Pharm., 1995, 117, 75–84 CrossRef CAS.
- A. Alhalaweh, A. Vilinska, E. Gavini, G. Rassu and S. P. Velaga, Surface thermodynamics of mucoadhesive dry powder formulation of zolmitriptan, AAPS PharmSciTech, 2011, 12, 1186–1192 CrossRef CAS PubMed.
-
C. J. van Oss, Interfacial Forces in Aqueous Media, Marcel Dekker, New York, 1994 Search PubMed.
- E. Fuchs, Keratins and the skin, Annu. Rev. Cell Dev. Biol., 1995, 11, 123–153 CrossRef CAS PubMed.
- W. S. Cho, M. Cho, J. Jeong, M. Choi, B. S. Han, H. S. Shin, J. Hong, B. H. Chung, J. Jeong and M. H. Cho, Size-dependent tissue kinetics of PEG-coated gold nanoparticles, Toxicol. Appl. Pharmacol., 2010, 245, 116–123 CrossRef CAS PubMed.
- D. P. Lankveld, R. G. Rayavarapu, P. Krystek, A. G. Oomen, H. W. Verharen, T. G. van Leeuwen, W. H. De Jong and S. Manohar, Blood clearance and tissue distribution of PEGylated and non-PEGylated gold nanorods after intravenous administration in rats, Nanomedicine, 2011, 6, 339–349 CrossRef CAS PubMed.
- J. A. Tate, A. A. Petryk, A. J. Giustini and P. J. Hoopes, In vivo biodistribution of iron oxide nanoparticles: an overview, Proc. SPIE, 2011, 7901, 790117 CrossRef PubMed.
- A. Golbraikh and A. Tropsha, Predictive QSAR modeling based on diversity sampling of experimental datasets for the training and test set selection, J. Comput.-Aided Mol. Des., 2002, 16, 357–369 CrossRef CAS PubMed.
- X. Li, B. Wang, S. Zhou, W. Chen, H. Chen, S. Liang, L. Zheng, H. Yu, R. Chu, M. Wang, Z. Chai and W. Feng, Surface chemistry governs the sub-organ transfer, clearance and toxicity of functional gold nanoparticles in the liver and kidney, J. Nanobiotechnol., 2020, 18, 45 CrossRef CAS PubMed.
- T. Dubaj, K. Kozics, M. Sramkova, A. Manova, N. G. Bastus, O. H. Moriones, Y. Kohl, M. Dusinska, E. Runden-Pran, V. Puntes, A. Nelson, A. Gabelova and P. Simon, Pharmacokinetics of PEGylated Gold Nanoparticles: In Vitro-In Vivo Correlation, Nanomaterials, 2022, 12(3), 511 CrossRef CAS PubMed.
- C. M. Lavelle, J. H. Bisesi, M. H. Hahn, K. J. Kroll, T. Sabo-Attwood and N. D. Denslow, Oral bioavailability and sex specific tissue partitioning of quantum dots in fathead minnows Pimephales promelas, Environ. Sci.: Nano, 2015, 2, 583 RSC.
- C. Kuroda, K. Ajima, K. Ueda, A. Sobajima, A. Sobajima, K. Yoshida, T. Kamanaka, J. Sasaki, H. Ishida, H. Haniu, M. Okamoto and K. Aoki, Isolated lymphatic vessel lumen perfusion system for assessing nanomaterial movements and nanomaterial-induced responses in lymphatic vessels, Nano Today, 2021, 36, 101018 CrossRef CAS.
-
H. J. Cho, S. C. Maroo and E. N. Wang, Characterization of Lipid Membrane Properties for Tunable Electroporation, ASME 2012 Third International Conference on Micro/Nanoscale Heat and Mass Transfer, 3–6 March 3, 2012, ASME, Atlanta, Georgia, 2012 Search PubMed.
- E. Canepa, S. Salassi, F. Simonelli, R. Ferrando, R. Rolandi, C. Lambruschini, F. Canepa, S. Dante, A. Relini and G. Rossi, Non-disruptive uptake of anionic and cationic gold nanoparticles in neutral zwitterionic membranes, Sci. Rep., 2021, 11, 1256 CrossRef CAS PubMed.
- A. Ghavami, E. van der Giessen and P. R. Onck, Energetics of Transport through the Nuclear Pore Complex, PLoS One, 2016, 11, e014887 CrossRef PubMed.
- L. Shi, J. Zhang, M. Zhao, S. Tang, X. Cheng, W. Zhang, W. Li, X. Liu, H. Peng and Q. Wang, Effects of polyethylene glycol on the surface of nanoparticles for targeted drug delivery, Nanoscale, 2021, 13, 10748–10764 RSC.
- X. Xia, M. Yang, Y. Wang, Y. Zheng, Q. Li, J. Chen and Y. Xia, Quantifying the coverage density of poly(ethylene glycol) chains on the surface of gold nanostructures, ACS Nano, 2012, 6, 512–522 CrossRef CAS PubMed.
- J. Manson, D. Kumar, B. J. Meenan and D. Dixon, Polyethylene glycol functionalized gold nanoparticles: the influence of capping density on stability in various media, Gold Bull., 2011, 44, 99–105 CrossRef CAS.
- P. M. Paarakh, D. C. Sreeram, S. S. D and S. P. Ganapathy, In vitro cytotoxic and in silico activity of piperine isolated from Piper nigrum fruits Linn, In Silico Pharmacol., 2015, 3, 9 CrossRef PubMed.
- M. Geiser, O. Quaile, A. Wenk, C. Wigge, S. Eigeldinger-Berthou, S. Hirn, M. Schaffler, C. Schleh, W. Moller, M. A. Mall and W. G. Kreyling, Cellular uptake and localization of inhaled gold nanoparticles in lungs of mice with chronic obstructive pulmonary disease, Part. Fibre Toxicol., 2013, 10, 19 CrossRef CAS PubMed.
- R. L. Griffith, G. T. Virella, H. C. Stevenson and M. F. Lopes-Virella, Low density lipoprotein metabolism by human macrophages activated with low density lipoprotein immune complexes, A possible mechanism of foam cell formation, J. Exp. Med., 1988, 168, 1041–1059 CrossRef CAS PubMed.
- N. Im, T. D. Yang, K. Park, J. Lee, J. Lee, Y. H. Kim, J. Lee, B. Kim, K. Jung, Y. Choi and S. Baek, Application of M1 macrophage as a live vector in delivering nanoparticles for in vivo photothermal treatment, J. Adv. Res., 2021, 31, 155–163 CrossRef CAS PubMed.
-
A. L. Lehninger, D. L. Nelson and M. M. Cox, Principles of Biochemistry, Worth, New York, 2nd edn, 1993 Search PubMed.
- X. Liu, H. Yang, Y. Liu, X. Gong and H. Huang, Numerical study of clathrin-mediated endocytosis of nanoparticles by cells under tension, Acta Mech. Sin., 2018, 35(3), 691–701 CrossRef.
- S. Farokhirad, R. P. Bradley and R. Radhakrishnan, Thermodynamic analysis of multivalent binding of functionalized nanoparticles to membrane surface reveals the importance of membrane entropy and nanoparticle entropy in adhesion of flexible nanoparticles, Soft Matter, 2019, 15, 9271–9286 RSC.
- D. Bochicchio and L. Monticelli, Chapter Five - The Membrane Bending Modulus in Experiments and Simulations: A Puzzling Picture, Adv. Biomembr. Lipid Self-Assem., 2016, 23, 117–143 CAS.
- H. R. Petty, D. G. Hafeman and H. M. McConnell, Disappearance of macrophage surface folds after antibody-dependent phagocytosis, J. Cell Biol., 1981, 89, 223–229 CrossRef CAS PubMed.
- L. V. Mohite, V. A. Juvekar and J. Sahu, Quantification of Polymer−Surface Interaction Using Microcalorimetry, Ind. Eng. Chem. Res., 2019, 58, 7495–7510 CrossRef CAS.
-
D. C. Agrawal, in Introduction to Nanoscience and Nanomaterials, World Scientific, 2013, ch. 2: Surfaces Search PubMed.
-
H. Amara, J. Nelayah, J. Creuze, A. Chmielewski, D. Alloyeau, C. Ricolleau and B. Legrand, Is There Really a Size effect on the Surface Energy?, HAL Open Science, l-03310351, 2021 Search PubMed.
-
B. Molleman, Skimming the surface : A surface structural approach to understanding silver ion release from silver nanoparticles, PhD, Wageningen University, 2019 Search PubMed.
- N. Tlotleng, M. A. Vetten, F. K. Keter, A. Skepu, R. Tshikhudo and M. Gulumian, Cytotoxicity, intracellular localization and exocytosis of citrate capped and PEG functionalized gold nanoparticles in human hepatocyte and kidney cells, Cell Biol. Toxicol., 2016, 32, 305–321 CrossRef CAS PubMed.
- D. Li, G. Johanson, C. Emond, U. Carlander, M. Philbert and O. Jolliet, Physiologically based pharmacokinetic modeling of polyethylene glycol-coated polyacrylamide nanoparticles in rats, Nanotoxicology, 2014, 8(Suppl 1), 128–137 CrossRef CAS PubMed.
- T. M. Nolte, W. De Cooman, J. P. M. Vink, R. Elst, E. Ryken, A. M. J. Ragas and A. J. Hendriks, Bioconcentration of Organotin Cations during Molting Inhibits Heterocypris incongruens Growth, Environ. Sci. Technol., 2020, 54, 14288–14301 CrossRef CAS PubMed.
- A. J. Hendriks, A. van der Linde, G. Cornelissen and D. T. H. M. Sijm, The power of size. 1. Rate constants and equilibrium ratios for accumulation of organic substances related to octanol-water partition ratio and species weight, Environ. Toxicol. Chem., 2001, 20, 1399–1420 CrossRef CAS PubMed.
- I. A. O'Connor, K. Veltman, M. A. Huijbregts, A. M. Ragas, F. G. Russel and A. J. Hendriks, Including carrier-mediated transport in oral uptake prediction of nutrients and pharmaceuticals in humans, Environ. Toxicol. Pharmacol., 2014, 38, 938–947 CrossRef PubMed.
- M. A. Gronski, J. M. Kinchen, I. J. Juncadella, N. C. Franc and K. S. Ravichandran, An essential role for calcium flux in phagocytes for apoptotic cell engulfment and the anti-inflammatory response, Cell Death Differ., 2009, 16, 1323–1331 CrossRef CAS PubMed.
- J. A. Arnot and F. A. P. C. Gobas, A review of bioconcentration factor (BCF) and bioaccumulation factor (BAF) assessments for organic chemicals in aquatic organisms, Environ. Rev., 2006, 14, 257–297 CrossRef CAS.
- S. Beulaja Manikandan, R. Manikandan, M. Arumugam and P. Mullainadhan, An overview on human serum lectins, Heliyon, 2020, 6, e04623 CrossRef CAS PubMed.
- J. Batson, L. MacCarthy-Morrogh, A. Archer, H. Tanton and C. D. Nobes, EphA receptors regulate prostate cancer cell dissemination through Vav2-RhoA mediated cell-cell repulsion, Biol. Open, 2014, 3, 453–462 CrossRef PubMed.
- J. M. Halbleib and W. J. Nelson, Cadherins in development: cell adhesion, sorting,
and tissue morphogenesis, Genes Dev., 2006, 20, 3199–3214 CrossRef CAS PubMed.
- C. Y. Loh, J. Y. Chai, T. F. Tang, W. F. Wong, G. Sethi, M. K. Shanmugam, P. P. Chong and C. Y. Looi, The E-Cadherin and N-Cadherin Switch in Epithelial-to-Mesenchymal Transition: Signaling, Therapeutic Implications, and Challenges, Cells, 2019, 8(10), 1118 CrossRef CAS PubMed.
- S. M. Furst, P. Sukhai, R. A. Mcclelland and J. P. Uetrecht, Covalent Binding of Carbamazepine Oxidative Metabolites to Neutrophils, Drug Metab. Dispos., 1995, 23, 590–594 CAS.
- M. W. Panas, Z. Xie, H. N. Panas, M. C. Hoener, E. J. Vallender and G. M. Miller, Trace amine associated receptor 1 signaling in activated lymphocytes, J. Neuroimmune Pharmacol., 2012, 7, 866–876 CrossRef PubMed.
- R. Toy, P. Pradhan, V. Ramesh, N. C. Di Paolo, B. Lash, J. Liu, E. L. Blanchard, C. J. Pinelli, P. J. Santangelo, D. M. Shayakhmetov and K. Roy, Modification of primary amines to higher order amines reduces in vivo hematological and immunotoxicity of cationic nanocarriers through TLR4 and complement pathways, Biomaterials, 2019, 225, 119512 CrossRef CAS PubMed.
- S. L. Christian and M. D. Berry, Trace Amine-Associated Receptors as Novel Therapeutic Targets for Immunomodulatory Disorders, Front. Pharmacol., 2018, 9, 680 CrossRef PubMed.
- J. Wu, H. Lin and J. C. Meredith, Poly(ethylene oxide) bionanocomposites reinforced with chitin nanofiber networks, Polymer, 2016, 84, 267–274 CrossRef CAS.
- O. S. Avdeeva, Z. V. Kuropteva, M. K. Pulatova and N. A. Kravchenko, Localization of unpaired electrons in molecules of the substrate inhibitors of lysozyme. II. Oligosaccharides, Biofizika, 1977, 22, 576–581 CAS.
- Z. Ma, L. Zhang, Y. Liu, X. Ji, Y. Xu, Q. Wang, Y. Sun, X. Wang, J. Wang, J. Xue and X. Gao, Influential Mechanism of Natural Organic Matters with Calcium Ion on the Anion Exchange Membrane Fouling Behavior via xDLVO Theory, Membranes, 2021, 11(12), 968 CrossRef CAS PubMed.
- Q. Li and M. Elimelech, Organic fouling and chemical cleaning of nanofiltration membranes: measurements and mechanisms, Environ. Sci. Technol., 2004, 38, 4683–4693 CrossRef CAS PubMed.
- S. Tokura, S. Nishimura and N. Nishi, Studies on Chitin IX, Specific Binding of Calcium Ion, Polym. J., 1983, 15, 597–602 CrossRef CAS.
- R. Bos and H. J. Busscher, Role of acid–base interactions on the adhesion of oral streptococci and actinomyces to hexadecane and chloroform—influence of divalent cations and comparison between free energies of partitioning and free energies obtained by extended DLVO analysis, Colloids Surf., B, 1999, 14, 169–177 CrossRef CAS.
- M. Vig and J. P. Kinet, Calcium signaling in immune cells, Nat. Immunol., 2009, 10, 21–27 CrossRef CAS PubMed.
- C. Ràfols, E. Bosch, R. Barbas and R. Prohens, The Ca2+–EDTA chelation as standard reaction to validate Isothermal Titration Calorimeter measurements (ITC), Talanta, 2016, 154, 354–359 CrossRef PubMed.
- J. O. Joswig, J. Anders, H. Zhang, C. Rademacher and B. G. Keller, The molecular basis for the pH-dependent calcium affinity of the pattern recognition receptor langerin, J. Biol. Chem., 2021, 296, 100718 CrossRef CAS PubMed.
- N. Tiwari, E. R. Blanco, A. Sonzogni, D. Ubieto, H. Wang and M. Calderón, Nanocarriers for Skin Applications: Where Do We Stand?, Angew. Chem., Int. Ed., 2021, 61, e202107960 Search PubMed.
- S. Barua and S. Mitragotri, Challenges associated with Penetration of Nanoparticles across Cell and Tissue Barriers: A Review of Current Status and Future Prospects, Nano Today, 2014, 9, 223–243 CrossRef CAS PubMed.
-
Y. Huang, N. A. Peppa, Nanoscale Analysis of Mucus–Carrier Interactions for Improved Drug Absorption, in Nanotechnology in Therapeutics. Current Technology and Applications, horizon
bioscience, Wymondham, 2006 Search PubMed.
- C. Liu, X. Jiang, Y. Gan and M. Yu, Engineering nanoparticles to overcome the mucus barrier for drug delivery: Design, evaluation and state-of-the-art, Med. Drug Discovery, 2021, 12, 100110 CrossRef CAS.
-
N. A. Peppas, J. Z. Hilt and J. B. Thomas, Nanotechnology in Therapeutics, Current Technology and Applications, horizon bioscience, Wymondham, 2006 Search PubMed.
- R. Bansil and B. S. Turner, The biology of mucus: Composition, synthesis and organization, Adv. Drug Delivery Rev., 2018, 124, 3–15 CrossRef CAS PubMed.
-
S. M. Jafari, Release and Bioavailability of Nanoencapsulated Food Ingredients, in Nanoencapsulation in the Food Industry, Charlotte Cockle, 2020, ch. 2.2.1 Mucus layer transport, p. 148 Search PubMed.
- L. J. Lawson, V. H. Perry and S. Gordon, Turnover of resident microglia in the normal adult mouse brain, Neuroscience, 1992, 48, 405–415 CrossRef CAS PubMed.
- J. Choi, Q. Zheng, H. E. Katz and T. R. Guilarte, Silica-based nanoparticle uptake and cellular response by primary microglia, Environ. Health Perspect., 2010, 118, 589–595 CrossRef CAS PubMed.
- M. L. Kerkeb, F. Gonzhlez-Caballero, B. Jahczukb and T. Biatopiotrowiczb, Components of surface free energy of cholesterol in the presence of bile salts, Colloids Surf., 1992, 62, 263–272 CrossRef CAS.
- B. Janczuk, M. L. Kerkeb, T. Bialopiotrowicz and F. Gonzalez-Caballero, Surface Free Energy of Cholesterol and Bile Salts from Contact Angle, J. Colloid Interface Sci., 1991, 151(2), 333–342 CrossRef.
- K. Vijayanand, D. K. Pattanayak, T. R. Ram Mohan and R. Banerjee, Interpreting Blood-Biomaterial Interactions from Surface Free Energy and Work of Adhesion, Trends Biomater. Artif. Organs, 2005, 18(2), 73 Search PubMed.
- M. S. Balzer, T. Rohacs and K. Susztak, How Many Cell Types Are in the Kidney and What Do, Annu. Rev. Physiol., 2021, 84, 507–531 CrossRef PubMed.
- A. L. Doiron, B. Clark and K. D. Rinker, Endothelial nanoparticle binding kinetics are matrix and size dependent, Biotechnol. Bioeng., 2011, 108, 2988–2998 CrossRef CAS PubMed.
- J. Ashby, S. Q. Pan and W. W. Zhong, Size and Surface Functionalization of Iron Oxide Nanoparticles Influence the Composition and Dynamic Nature of Their Protein Corona, ACS Appl. Mater. Interfaces, 2014, 6, 15412–15419 CrossRef CAS PubMed.
- A. Tomak, S. Cesmeli, B. D. Hanoglu, D. Winkler and C. O. Karakus, Nanoparticle-protein corona complex: understanding multiple interactions between environmental factors, corona formation, and biological activity, Nanotoxicology, 2021, 15, 1331–1357 CrossRef CAS PubMed.
- T. Marquardt, T. Brune, K. Luhn, K. P. Zimmer, C. Korner, L. Fabritz, N. van der Werft, J. Vormoor, H. H. Freeze, F. Louwen, B. Biermann, E. Harms, K. von Figura, D. Vestweber and H. G. Koch, Leukocyte adhesion deficiency II syndrome, a generalized defect in fucose metabolism, J. Pediatr., 1999, 134, 681–688 CrossRef CAS PubMed.
- K. C. Stone, R. R. Mercer, P. Gehr, B. Stockstill and J. D. Crapo, Allometric relationships of cell numbers and size in the mammalian lung, Am. J. Respir. Cell Mol. Biol., 1992, 6, 235–243 CrossRef CAS PubMed.
- F. Roussel and J. Dalion, Lectins as markers of endothelial cells: comparative study between human and animal cells, Lab. Anim., 1988, 22, 135–140 CrossRef CAS PubMed.
- D. Wang, Z. Hu, G. Peng and Y. Yin, Surface Energy of Curved Surface Based on Lennard-Jones Potential, Nanomaterials, 2021, 11(3), 686 CrossRef CAS PubMed.
- M. I. Setyawati, X. Yuan, J. P. Xie and D. T. Leong, The influence of lysosomal stability of silver nanomaterials on their toxicity to human cells, Biomaterials, 2014, 35, 6707–6715 CrossRef CAS PubMed.
- X. W. Ma, Y. Y. Wu, S. B. Jin, Y. Tian, X. N. Zhang, Y. L. Zhao, L. Yu and X. J. Liang, Gold Nanoparticles Induce Autophagosome Accumulation through Size-Dependent Nanoparticle Uptake and Lysosome Impairment, ACS Nano, 2011, 5, 8629–8639 CrossRef CAS PubMed.
- J. X. Zhang, X. D. Zhang, G. Liu, D. F. Chang, X. Liang, X. B. Zhu, W. Tao and L. Mei, Intracellular Trafficking Network of Protein Nanocapsules: Endocytosis, Exocytosis and Autophagy, Theranostics, 2016, 6, 2099–2113 CrossRef CAS PubMed.
- O. Lunov, T. Syrovets, C. Rocker, K. Tron, G. U. Nienhaus, V. Rasche, V. Mailander, K. Landfester and T. Simmet, Lysosomal degradation of the carboxydextran shell of coated superparamagnetic iron oxide nanoparticles and the fate of professional phagocytes, Biomaterials, 2010, 31, 9015–9022 CrossRef CAS PubMed.
- R. Gupta, Y. Badhe, S. Mitragotri and B. Rai, Permeation of nanoparticles across the intestinal lipid membrane: dependence on shape and surface chemistry studied through molecular simulations, Nanoscale, 2020, 12, 6318–6333 RSC.
- J. Dumková, T. Smutná, L. Vrlíková, P. Le Coustumer, Z. Večeřa, B. Dočekal, P. Mikuška, L. Čapka, P. Fictum, A. Hampl and M. Buchtová, Sub-chronic inhalation of lead oxide nanoparticles revealed their broad distribution and tissue-specific subcellular localization in target organs, Part. Fibre Toxicol., 2017, 14, 55 CrossRef PubMed.
- W. C. Chou, Y. H. Cheng, J. E. Riviere, N. A. Monteiro-Riviere, W. G. Kreyling and Z. Lin, Development of a multi-route physiologically based pharmacokinetic (PBPK) model for nanomaterials: a comparison between a traditional versus a new route-specific approach using gold nanoparticles in rats, Part. Fibre Toxicol., 2022, 19, 47 CrossRef CAS PubMed.
- N. Hoshyar, S. Gray, H. Han and G. Bao, The effect of nanoparticle size on in vivo pharmacokinetics and cellular interaction, Nanomedicine, 2016, 11, 673–692 CrossRef CAS PubMed.
- E. Yaghini, E. Tacconi, A. Pilling, P. Rahman, J. Broughton, I. Naasani, M. R. S. Keshtgar, A. J. MacRobert and O. Della Pasqua, Population pharmacokinetic modelling of indium-based quantum dot nanoparticles: preclinical in vivo studies, Eur. J. Pharm. Sci., 2021, 157, 105639 CrossRef CAS PubMed.
- L. Geraets, A. G. Oomen, P. Krystek, N. R. Jacobsen, H. Wallin, M. Laurentie, H. W. Verharen, E. F. Brandon and W. H. de Jong, Tissue distribution and elimination after oral and intravenous administration of different titanium dioxide nanoparticles in rats, Part. Fibre Toxicol., 2014, 11, 30 CrossRef PubMed.
- Y. Zheng and B. Nowack, Meta-analysis of Bioaccumulation Data for Nondissolvable Engineered Nanomaterials in Freshwater Aquatic Organisms, Environ. Toxicol. Chem., 2022, 41, 1202–1214 CrossRef CAS PubMed.
- X. R. Xia, N. A. Monteiro-Riviere and J. E. Riviere, An index for characterization of nanomaterials in biological systems, Nat. Nanotechnol., 2010, 5, 671–675 CrossRef CAS PubMed.
- L. Ponsonnet, K. Reybier, N. Jaffrezic, V. Comte, C. Lagneau, M. Lissac and C. Martelet, Relationship between surface properties (roughness, wettability) of titanium and titanium alloys and cell behaviour, Mater. Sci. Eng., C, 2003, 23, 551–560 CrossRef.
-
R. Bos, H. J. Busscher, G. I. Geertsema-Doornbusch and H. C. Van Der Mei, Acid-base interactions in microbial adhesion to hexadecane and chloroform, in 2nd International Symposium on Acid-Base Interactions - Relevance to Adhesion Science and Technology, Newark, NJ, 1998, pp. 513–524 Search PubMed.
- Y. Xiao and M. R. Wiesner, Characterization of surface hydrophobicity of engineered nanoparticles, J. Hazard. Mater., 2012, 215, 146–151 CrossRef PubMed.
- A. Valsesia, C. Desmet, I. Ojea-Jiménez, A. Oddo, R. Capomaccio, F. Rossi and P. Colpo, Direct quantification of nanoparticle surface hydrophobicity, Commun. Chem., 2018, 1, 53 CrossRef.
- J. A. J. Meesters, A. A. Koelmans, J. T. K. Quik, A. J. Hendriks and D. van de Meentt, Multimedia Modeling of Engineered Nanoparticles with SimpleBox4nano: Model Definition and Evaluation, Environ. Sci. Technol., 2014, 48, 5726–5736 CrossRef CAS PubMed.
- J. D. Crapo, B. E. Barry, P. Gehr, M. Bachofen and E. R. Weibel, Cell number and cell characteristics of the normal human lung, Am. Rev. Respir. Dis., 1982, 126, 332–337 CAS.
- L. M. Sweeney, L. MacCalman, L. T. Haber, E. D. Kuempel and C. L. Tran, Bayesian evaluation of a physiologically-based pharmacokinetic (PBPK) model of long-term kinetics of metal nanoparticles in rats, Regul. Toxicol. Pharmacol., 2015, 73, 151–163 CrossRef CAS PubMed.
- Z. Lin, N. A. Monteiro-Riviere and J. E. Riviere, A physiologically based pharmacokinetic model for polyethylene glycol-coated gold nanoparticles of different sizes in adult mice, Nanotoxicology, 2016, 10, 162–172 CAS.
|
This journal is © The Royal Society of Chemistry 2023 |
Click here to see how this site uses Cookies. View our privacy policy here.