DOI:
10.1039/D3QM01315D
(Research Article)
Mater. Chem. Front., 2024,
8, 1958-1970
Boosting the catalytic performance of metalloporphyrin-based covalent organic frameworks via coordination engineering for CO2 and O2 reduction†
Received
21st December 2023
, Accepted 27th February 2024
First published on 28th February 2024
Abstract
Metalloporphyrin-based covalent organic frameworks (Por-COFs) are emerging as electrocatalysts; however, so far experiments have primarily focused on M–N4-coordinated Por-COFs. A wealth of coordination modified systems with potential catalytic capability remains to be explored. Herein, we report a proof-of-concept computational study on the coordination engineering of Por-COFs as electrocatalysts for reducing CO2 and O2. Systematic density functional theory calculations were performed on 15 types of heteroatomic Por-COFs, featuring M–NxOySz (M = Fe, Co, and Ni; x + y + z = 4) centers. Calculations predicted that the Co–N2O2-Por-COF is an optimal CO2-to-CO catalyst candidate (limiting potential
) and the Ni–N2S2-Por-COF is an optimal O2-to-H2O catalyst candidate (overpotential ηORR = 0.46 V); both heteroatomic-Por-COFs display better catalytic activity and selectivity than their corresponding parent M–N4-Por-COFs. Electronic structure analysis attributes the enhanced catalytic performance to the additional stabilization, endowed by the heteroatoms, of critical reaction intermediates. Furthermore, feature importance analysis based on machine learning models confirmed that the interplay between the central metal and the coordinated atoms is crucial for catalytic performance. This work predicts new Por-COFs as electrocatalysts for CO2 and O2 reduction and showcases the great potential of coordination regulation strategies in designing high-performance COF-based electrocatalysts.
1 Introduction
Covalent organic frameworks (COFs) are emerging electrocatalysts for energy conversion and storage, primarily due to their well-defined active sites, tunable coordination and topology, and accessible pore surfaces.1–5 COFs are organized by organic knots and covalently connected linkers. Recently, the incorporation of metallo-porphyrin (Por) or phthalocyanine (Pc) knots into COFs has resulted in highly active, selective, and stable catalysts for electrochemical reduction of CO2 and/or O2.6–28 In 2015, the Yaghi group developed the first cobalt porphyrin-based COF (i.e., COF-366-Co), which enables a 26-fold increase in electrochemical CO2 reduction reaction (CO2RR) activity compared to the molecular porphyrin catalyst.6 The Jiang group synthesized the CoPc-PDQ-COF, which enables a 32-fold increase in CO2-to-CO activity compared to the molecular phthalocyanine catalyst.25 In addition, the Co-TEPP-COF/C composite and FePc-BBL-COF displayed high electrocatalytic activity and selectivity for the reduction of O2 to water.26,27
Of note, the abovementioned Por/Pc-based COFs share a M–N4-coordination centre. Coordination engineering, which alters the N4-environment to heteroatoms, is an effective strategy to improve the catalytic properties of two-dimensional atomic dispersed transition metal catalysts in N-doped carbon, i.e. M–N–C single atom catalysts (SACs).29–33 For example, Zhao et al. designed Ni-based carbon nanosheet SACs with NiN3S sites and Song et al. designed Cu-based carbon support SACs with CuN3O or CuCO3 sites; all of these catalysts showed high catalytic activity and selectivity for CO production.34,35 Li et al. synthesized an ortho-coordinated FeN2O2@graphene catalyst and achieved enhanced oxygen reduction reaction (ORR) performance in zinc–air batteries.36 Wang et al. synthesized a carbon-supported Ni-based SAC with N2O2 coordination for high-efficiency O2 reduction to H2O2.37 Theoretically, the performance of graphene-based MSxNy (M = Fe, Co, Ni, and Cu) and FeXYiN3−i (X, Y = B, C, O, P, and S) SACs was investigated, leading to promising catalysts for electrochemical CO2RR or water electrolysis, respectively.38,39 However, to our knowledge, in experiments, coordination engineering has not been applied in Por/Pc-based COFs yet.
As a matter of fact, molecular heteroatomic porphyrins and metallo-heteroatomic porphyrins complexes have already been successfully synthesized.40–49 These include singly-substituted cores, such as N3O41–43 and N3S,44–47 as well as doubly-substituted cores, such as N2O2,41 N2S2,47,48 and N2OS.49 The embedded central metals include Fe, Co, Ni, Cu, Re, and Ru. In addition, H. Kim et al. have shown that Ni coordinated with 21-oxatetraphenylporphyrin (N3O-TPP) becomes highly active toward electrochemical CO2-to-CO reduction, unlike the barely active NiN4–TPP complex.50 These achievements make us believe that it is possible to synthesize heteroatomic-Por-COFs in the future. Accordingly, we anticipate that the incorporation of O and/or S atoms into the porphyrin unit will change the catalytic properties of the COFs, thus affording a series of new catalysts. Hence, a priori theoretical computation is important.
Herein, we present a proof-of-concept computational study on the coordination engineering of Por-COFs for catalysing the reduction of CO2 and O2, highlighting their importance in carbon neutrality and fuel cells. Through systematic periodic density functional theory calculations on M–NxOySz coordinated Por-COFs (Fig. 1), where M = Fe, Co, and Ni, we confirmed that coordination engineering is an effective strategy to boost the catalytic performance of Por-COFs. Calculations predict the Co–N2O2-Por-COF to be the optimal catalyst candidate for CO2RR while the Ni–N2O2-Por-COF is optimal for ORR. The essence of enhanced catalytic performance was further investigated through electronic structure analysis and machine learning models. This study provides a fundamental mechanistic understanding of a new group of Por-COF electrocatalysts and identifies several candidates for CO2RR/ORR, thus paving the way toward computation-guided design of potential electrocatalysts.
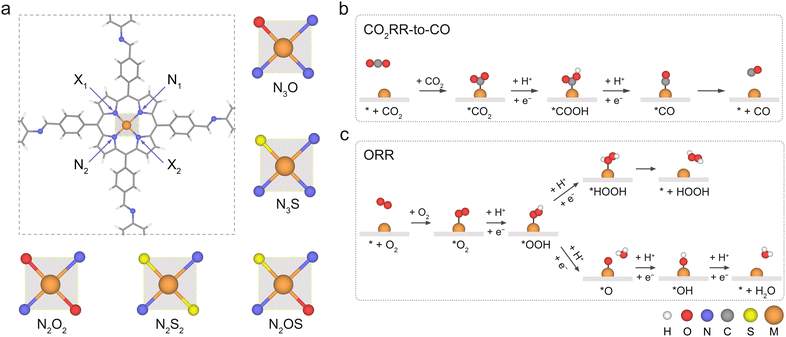 |
| Fig. 1 (a) Unit cell of the M–N4-Por-COF (M = Fe/Co/Ni) considered in this work and the constructed NxOySz coordination models. Reaction pathways for (b) CO2RR-to-CO and (c) ORR-to-H2O2 (2e−)/H2O (4e−). | |
2 Computational details
2.1 Calculation model setup
The metal-anchored Por-COF shown in Fig. 1a was used as the parent model for coordination engineering. It has been readily synthesized with 5,10,15,20-tetrakis(4-benzaldhyde)porphyrin and p-phenylenediamine (PPDA).17,51 A periodic model of the Por-COF was used for calculations throughout this work. The unit cell of monolayer M–NxOySz-Por-COFs, containing 60 carbon atoms, 8 nitrogen atoms (or one or two to be substituted with oxygen and/or sulphur atoms), 36 hydrogen atoms, and 1 metal atom, was used. Furthermore, a 20 Å vacuum layer was placed on top of the surface to prevent mirror interactions.
2.2 Density functional theory (DFT) calculation methods
Spin polarized periodic DFT calculations were performed using the Vienna Ab initio Simulation Package (VASP).52,53 Electron exchange and correction were described using the Perdew–Burke–Ernzerhof (PBE) functional within the generalized gradient approximation (GGA).54,55 Grimmes's D3 scheme of dispersion correction was included to describe the van der Waals (vdW) interaction.56 The plane wave basis was employed using the projector augmented wave (PAW) method with a cutoff energy of 520 eV.57,58 The DFT+U calculations were performed and the corresponding U–J values are listed in Table S1 (ESI†).59,60 Brillouin zone was sampled using 3 × 3 × 1 and 5 × 5 × 1 k-point grids for geometry optimization and electronic structure calculations, respectively. All atoms were allowed to relax during geometry optimization, and the convergence criteria for force and energy were set to be 10−2 eV Å−1 and 10−5 eV, respectively. A vacuum layer of 20 Å was placed between adjacent layers to prevent interaction. For M = Fe/Co/Ni, different spin states were tested and the discussed data correspond to the most stable structures (Tables S2–S4, ESI†). The implicit solvent model implemented in VASPsol with a dielectric constant of 78.4 was used for the aqueous solution.61,62
2.3 Reaction free energy calculation
The computational hydrogen electrode (CHE) model developed by Nørskov et al.63 was applied to obtain the thermodynamic Gibbs free energy change (ΔG) of all elementary reactions: | ΔG = ΔE + ΔEZPE − TΔS + ΔGU + ΔGpH | (1) |
where ΔE, ΔEZPE and ΔS are the difference in electronic energy, zero-point energy correction and entropy between the product and the reactant of each elementary reaction, respectively, and T is set as 298.15 K. EZPE and ΔS were obtained by calculating the vibrational frequencies for the adsorption intermediates (Tables S5 and S6, ESI†). ΔGU and ΔGpH represent the free energy corrections caused by the electrode potential (U) and the H+ concentration, respectively. Specifically, ΔGU = −neU, where n is the number of transferred electrons and U is the applied potential; ΔGpH = kBT × ln
10 × pH, where kB is the Boltzmann constant. The pH is set as 0 in this work for convenience, as has been done in most works.18,38,39,64 The stability of the designed COFs under highly acidic conditions requires further experimental examination, but existing literature supports that certain M-Por-COFs are stable in acidic solutions.65–67 In a few cases, energy values were computed at pH = 7 for comparison. It is important to note that the relative catalytic capability of the designed COF catalysts remains the same under different pH conditions.
The potential-determining step (PDS) is the step with the largest reaction Gibbs free energy value (ΔGmax) among all elementary steps. The limiting potential
of CO2RR is defined as UL = −ΔGmax/e. The overpotential (ηORR) of ORR is defined as η = U0 − UL, where U0 is the equilibrium potential.
2.4 Stability evaluations
The thermodynamic and electrochemical stabilities of Por-COFs were investigated using binding energy (Eb), the difference between adsorption energy and cohesive energy (Eb − Ecoh) and dissolution potential (Udiss). Eb measures the binding energy between the metal atom and the substrate,68Ecoh measures the cohesion tendency of isolated metal atom to form the bulk metal,69,70Udiss measures the ability of active metal atoms to resist dissolution under electrochemical conditions;71 the related equations are as follows | Eb = EPor-COF+M − EPor-COF − Esingle-M | (2) |
| Ecoh = Ebulk − n × Esingle-M | (3) |
| 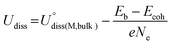 | (4) |
where ECOF+M, ECOF, Esingle-M and Ebulk are the total energy of the Por-COF with M, the Por-COF without M, the single M atom and the total energy of metal atoms in the most stable bulk structure, respectively;
and Ne are the standard dissolution potential of bulk metal and the number of electrons involved in dissolution, respectively. Eb < 0, Eb − Ecoh < 0, and Udiss > 0 indicate that the catalyst has excellent thermodynamic and electrochemical stability. These criteria have been used to evaluate the stability of single atom catalysts in several works.60,72,73
The dynamic stability of Co–N2O2, Ni–N2S2-, and Fe–N2OS-Por-COFs was further tested through ab initio molecular dynamics (AIMD) simulations using the VASP package. A constant volume/temperature (NVT) ensemble and a Nose–Hoover thermostat were used to maintain the temperature at 300 K. The equation of motion is integrated using the Verlet algorithm method with a timestep of 2 fs, and the total simulation time is 10 ps for each system.
2.5 Machine learning (ML)
All machine learning (ML) predictions were conducted using an open-source Scikit-learn library within the Python3 environment.74 The optimum parameter values for the three different ML algorithms used in this work, i.e., random forest regression (RFR),75 gradient boosting regression (GBR),76,77 and extreme gradient boosting regression (XGBoost),78 are given in Table S7 (ESI†). Twenty widely recognized features relevant to active center properties were selected as input features for training ML models. All data sources were obtained either from existing databases or through single-step optimization calculations.79 The input dataset was randomly divided into a training set and a test set in a 4
:
1 ratio. Given the constrained dataset size and potential errors stemming from the segmentation process, a fourfold cross-validation approach was implemented to ensure the model's generalizability. This approach entails the creation of four distinct training and test sets for ML model training and evaluation over four iterations. The optimal algorithm for
and ηORR prediction was determined by comparing the average values of root mean square error (RMSE) and coefficient of determination value (R2 score) across four iterations; then, the best prediction on the test set was recorded for discussion.
3 Results and discussion
As shown in Fig. 1a, the metal-anchored Por-COF with PPDA as a linker was used as the parent model for coordination engineering. Three non-noble transition metal centers were considered: M = Fe, Co, and Ni. The four coordinated N atoms were partially substituted with either O or S atoms, leading to 5 different coordination models: N3O, N3S, ortho-N2O2, ortho-N2S2, and ortho-N2OS. Taking together, a total of 15 types of M–NxOySz-Por-COFs were designed. The optimized structures (Fig. S1, ESI†) of the porphyrin macrocycles of M–N3O- and M–N2O2-Por-COFs maintain the square-planar geometry, as observed in M–N4-Por-COFs, whereas the substituted S atoms are positioned out of the plane due to their bulkier atom size. The observation of plane distortion caused by the bulkier S atom has been noted in molecular N3S-, N2S2- and N2OS-porphyrins,40,46,49 as well as in M–N–C graphene-based SACs.80,81
3.1 Stability and conductivity
The thermodynamic and electrochemical stabilities of heteroatomic Por-COFs were evaluated using binding energy (Eb), the difference between binding energy and cohesive energy (Eb − Ecoh) and dissolution potential (Udiss). Calculated values (Fig. 2a–c) suggest that the N3O-, N3S and N2O2-coordinated Por-COFs, similar to the experimentally synthesized M–N4-Por-COFs, meet all the stability criteria, i.e., Eb < 0, Eb − Ecoh < 0 and Udiss > 0, meaning that the materials are able to avoid migration and aggregation of metal atoms under actual electrochemical work conditions. However, for the N2S2- and N2OS-coordinated Por-COFs, the values of (Eb − Ecoh) are slightly positive and Udiss values are slightly negative, which is likely attributed to the structure deformation introduced by S atoms. Hence, we further tested their dynamic stability using ab initio molecular dynamics (AIMD) simulations at 300 K. Throughout the 10 ps simulation, the systems maintain their integrity with the metal atoms sitting in the center position, confirming their structural stability (Fig. S2, ESI†).
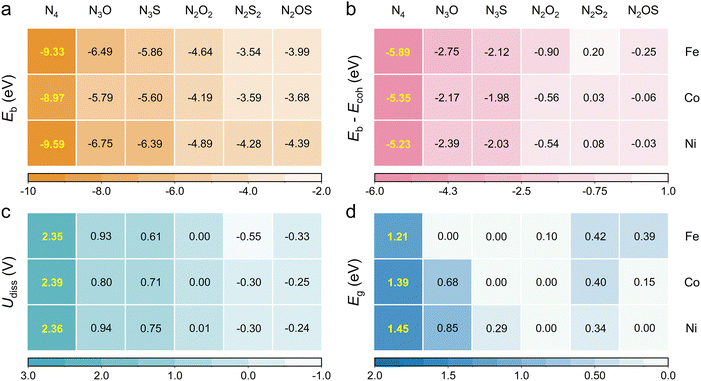 |
| Fig. 2 (a) The binding energy, (b) the difference between binding energy and cohesive energy, (c) the dissolution potential and (d) the band gap of Fe/Co/Ni–NxOySz-Por-COFs. | |
Then, the electrical conductivity of the designed materials was evaluated based on their band gaps (Eg). The Eg values of parent M–N4-Por-COFs are 1.21, 1.39 and 1.45 eV for M = Fe, Co, and Ni, respectively (Fig. 2d and Fig. S3, ESI†). Such large gaps indicate their poor electron transfer ability, consistent with the limited current densities observed in experiments.17 Encouragingly, the calculated Eg of heteroatomic Por-COFs decreases greatly, suggesting an improved conductivity. Especially, the calculated band gap of Fe–N3O-, Fe–N3S-, Co–N3S-, Co–N2O2-, Ni–N2O2- and Ni–N2OS-Por-COFs vanishes, thus displaying conductor characteristics.
Accordingly, substituting the N-atom with O/S-atoms in the metal coordination of M–N4-Por-COFs largely maintains stability and improves conductivity, serving as the foundation of further exploration of catalytic performance.
3.2 Catalytic performance
To investigate the effect of the coordination environment on the catalytic performance of Por-COFs, we studied the electrocatalytic reduction reaction of CO2 and O2 in sequence.
3.2.1 Reduction of CO2 to CO.
The conversion of CO2 to CO is the dominant product of CO2RR catalysed by Por-COFs,6–11,15,17–19,22,28,82 so it is the focus of this study. As illustrate in Fig. 1b, the pathway of converting CO2 to CO catalysed by Por-COFs follows these steps: (1) adsorption of CO2, (2) formation of carboxylic acid intermediate (*COOH) via the 1st proton-coupled electron transfer (PCET) step, (3) dehydration of *COOH intermediates to form *CO intermediates via the 2nd PCET step, and (4) desorption of CO to regenerate the catalyst.
The first step is the adsorption of CO2. The absorption strength was slightly changed by substituting the N4-coordination with NxOySz-coordination, with ΔEads-CO2 ranging from 0.02 to −0.34 eV (Table S8, ESI†). This is consistent with previous reports.64 Meanwhile, depending on the coordination type the adsorbed shape of CO2 on Por-COFs (Fig. S4 and S5, ESI†) can either be linear (for the case of N4, N3S, and N2S2) or bent (for the case of N2O2, N3O, and N2OS). For the bent case, the M–C*CO2 bond is as short as 1.96 to 2.17 Å, along with a stretching of the C–O bond by 0.05 to 0.10 Å (ca. 4.3–8.6% elongation).
Then, the following catalytic steps for CO2-to-CO conversion were computed and are presented in the free energy diagrams (Fig. S6–S8, ESI†). As calculated, the potential determining step (PDS) for all the target M–NxOySz-Por-COFs is the formation of the *COOH intermediate (Fig. 3a and Fig. S6, ESI†). Fig. 4a summarizes the corresponding theoretical limiting potentials UL. In general, changing the coordination from N4 to NxOySz shifts the ULCO2RR towards the positive direction, meaning an easier reduction and thus higher CO2-to-CO catalytic activity. This is largely due to the stabilization of the *COOH intermediate, thus lowering the free energy of the PDS (ΔG*→*COOH). In comparison, the UL values of the Co-system are generally less negative than those of the Fe-/Ni-counterparts (Fig. 4a). Among all the designed Fe/Co/Ni–NxOySz-Por-COFs, the Co–N2O2-Por-COF (UL = −0.58 V) is predicted to be the best CO2-to-CO catalyst, followed by Fe–N2OS (UL = −0.64 V), Fe–N2O2 (UL = −0.66 V) and Co–N3O-Por-COFs (UL = −0.67 V). The above results evidenced that modifying the M–N4 coordination via introducing O or/and S atoms is an effective strategy to enhance the CO2RR catalytic activity of Por-COFs.
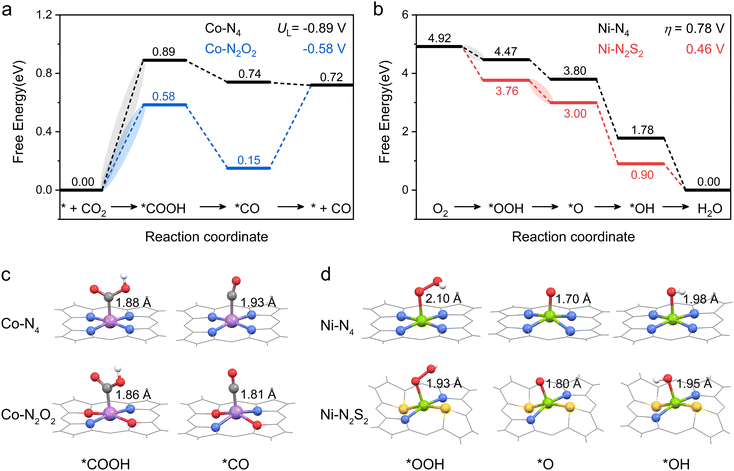 |
| Fig. 3 Free energy diagrams of (a) CO2RR on Co–N4-and Co–N2O2-Por-COFs and (b) ORR on Ni–N4-and Ni–N2S2-Por-COFs. Optimized configurations of (c) *COOH, *CO, and (d) *OOH, *O, *OH intermediates. Color code: Co, purple; Ni, green; C, gray; N, blue; O, red; S, yellow; and H, white. For clarity, the tetraphenyl and p-phenylenediamine (PPDA) moieties of M–NxOySz-Por-COFs are not shown. Note: the corresponding free energy diagrams computed at pH = 7 are presented in Fig. S18 (ESI†) for comparison. | |
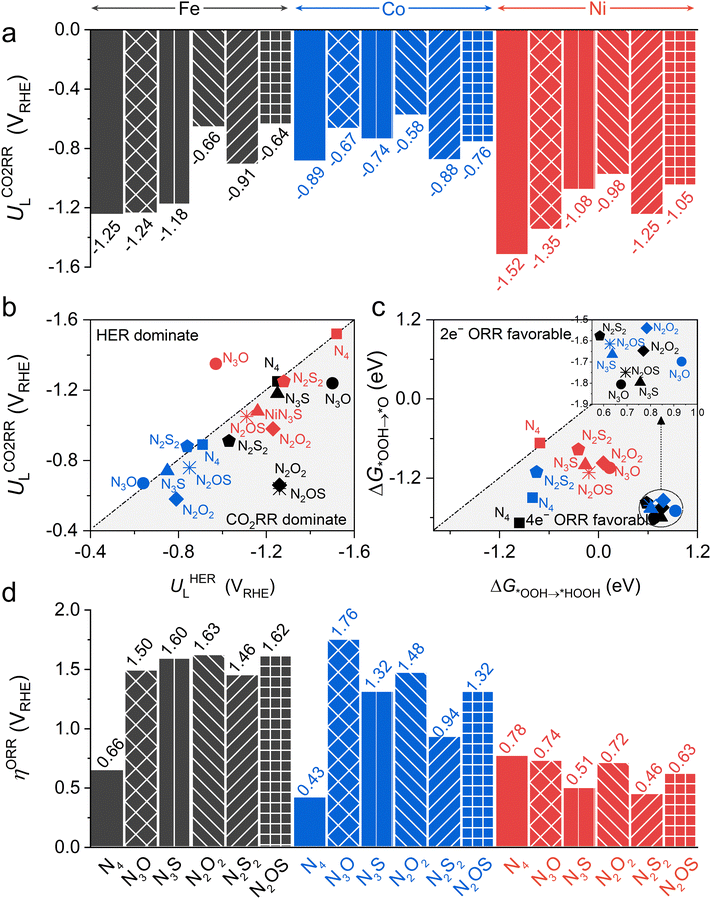 |
| Fig. 4 (a) Limiting potential of CO2RR-to-CO of Fe/Co/Ni–NxOySz-Por-COFs. (b) Limiting potential of CO2RR-to-CO vs. limiting potential of HER. (c) Gibbs free energy changes (ΔG*OOH → *O) of *O formation vs. Gibbs free energy changes (ΔG*OOH → HOOH) of HOOH formation. (d) Overpotential of ORR-to-H2O of Fe/Co/Ni–NxOySz-Por-COFs. | |
In addition, modifying the N4 coordination also enhances the selectivity of CO2RR over HER. Unlike the original three M–N4-Por-COFs which exhibit a strong competition between CO2RR and HER (Fig. 4b and Fig. S9, S10, ESI†), the majority of the designed M–NxOySz-Por-COFs display a distinct preference for CO2RR. Exceptions are Ni–N3O, Co–N3O, Co–N3S and Co–N2S2 systems, which a show slight preference for HER. Such undesired preference can be reversed in practice by adjusting the pH of the electrolyte or tuning the composition of the electrochemical double layer.83–85
Although not the focus of this study, a great portion of spin distribution was observed on the porphyrin ligands, as shown in Co–N2O2–COOH intermediates (Fig S11, ESI†). This indicates the non-innocent role of the porphyrin ligands, which has been suggested by Liao et al.86–89 This observation gives us a hint that modifying the porphyrin ligand could further tune the catalytic ability of the Por-COFs.
Based on the screening process considering intrinsic stability, catalytic activity, and selectivity, four CO2-to-CO catalyst candidates are predicted: Co–N2O2, Fe–N2OS, Fe–N2O2 and Co–N3O-Por-COFs. Remarkably, all of them display performance comparable to available Por-COFs synthesized through experiments (Fig. S12, ESI†). It is worth noting that these newly designed Por-COFs may yield deep-reduction C1 products (CH3OH and CH4), especially when combined with photosensitizers, sacrificial electron donors, or supporting materials.22,82,87,89–91 These aspects merit further exploration, but we have limited this work to CO2-to-CO reduction.
In brief, incorporating O/S atoms to modulate the coordination environment of active metals in Fe/Co/Ni-Por-COFs has been proven to be useful for CO2-to-CO catalyst design. To explore the generality of this coordination modulation strategy, we now redirect our attention to another crucial electroreduction reaction, ORR.
3.2.2 Oxygen reduction reaction.
Typically, the catalyzed ORR under acidic conditions can proceed through either a two-electron (2e−) pathway, resulting in the production of H2O2, or a four-electron (4e−) pathway, resulting in the production of H2O. Fig. 1c shows the specific reaction pathways.
The adsorption of O2 is the first step of ORR. In comparison, all the O/S coordinated Por-COFs show stronger adsorption of O2 than the parent M–N4-systems, with the exception of the Ni–N2S2-system. Observing the most stable O2-adsorped configurations on the M–NxOySz-Por-COF (Fig. S13–S15, ESI†) shows that Fe-systems favour a side-on configuration (resembling the Griffiths-type) while Co/Ni-systems, except the Co–N2OS case, favour an end-on configuration (resembling the Pauling-type). The side-on *O2 structures exhibit a greater O–O bond stretch relative to the free O2 (over 14% elongation for Fe-systems) than that of end-on *O2 (roughly 10% elongation for Co/Ni-systems), indicating stronger activation of O2 in the former (Fig. S16 and Table S9, ESI†). The calculated O2 adsorption energy (ΔEads-O2) ranges from −0.18 to −1.58 eV, indicating chemisorption of O2.
Since the 2e− and 4e− ORR pathways share the first two steps (* + O2 → *O2 and *O2 → *OOH) and diverge at the *OOH intermediate, the selectivity is determined by the competition between the free energy difference of ΔG*OOH→*HOOH and ΔG*OOH→*O. Fig. 4c shows that all the considered catalysts favour the 4e− ORR pathway over the 2e− ORR pathway, except for the Ni–N4-system shows similar preference, whose ΔG*OOH→*O is −0.67 eV and ΔG*OOH→*HOOH is −0.71 eV. Hence, we will focus on the 4e− ORR pathway, which converts O2 to H2O, in the following discussion.
The effect of changing coordination from N4 to NxOySz on 4e− ORR activity is central-metal-dependent: the ORR activity is enhanced when M = Ni, however it is inhibited when M = Fe or Co (Fig. 4d). An ideal 4e− ORR catalyst requires the ΔG of each elementary step to be ∼−1.23 eV at zero potential, but normally the differential adsorption/desorption behavior of the intermediates will disrupt this balance and results in overpotentials (ηORR). Specifically, each catalyst has a different PDS for the 4e− ORR (Fig. 3b and Fig. S17, ESI†): 1st step (* + O2 → *OOH) for Ni–N4, Co–N4, and Co–N2S2 system; 2nd step (*OOH → *O) for the Ni–N2S2 system, and 4th step (*OH → *H2O) for the rest. Although the parent M–N4 catalysts have lower ηORR values for Fe–N4 (0.66 V) and Co–N4 (0.43 V) than for Ni–N4 (0.78 V), changing the coordinated environment to NxOySz only lowers the ηORR of Ni-systems. These heteroatom-coordinated Ni-systems are Ni–N3O (ηORR = 0.74 V), Ni–N2O2 (0.72), Ni–N2OS (0.63 V), Ni–N3S (0.51 V) and Ni–N2S2 (0.46 V). The increase in ηORR for heteroatom-coordinated Fe/Co-systems is attributed to the excessive strong adsorption of some intermediates (Fig. S19–S21, ESI†). Of note, the parent Co–N4-catalysts exhibits the lowest ηORR of 0.43 V, followed by the Ni–N2S2 (0.46) and Ni–N3S (0.51) systems. All of them are promising ORR electrocatalyst candidates as compared to the commercial Pt (111) catalyst (ηORR = 0.45 V).63
3.3 Activity origin
The above calculations predict that, among the modified Por-COFs, the Co–N2O2-Por-COF is the best catalyst candidate for CO2 reduction and the Ni–N2S2-Por-COF is the best candidate for O2 reduction. To understand the reason behind the improved catalytic performance compared to parent Co–N4/Ni–N4-Por-COFs, electronic structure analyses were performed. It has been found that by changing the coordination from N4 to N2O2/N2S2, the key intermediates associated with the PDSs were stabilized due to a better match between the orbitals of the central metal and the adsorbed species, thus improving catalytic activity.
In terms of comparison between Co–N2O2 and Co–N4, *COOH serves as the key intermediate for CO2-to-CO conversion (Fig. 3a). Changing the coordination from N4 to N2O2 increases the orbital overlap between Co's 3d orbitals and the *COOH’ 2p orbitals, as depicted in Fig. 5a and b. The charge transferred from Co to the COOH-moiety (Fig. 5c and d) increases from 0.15 e− (Co–N4) to 0.32 e− (Co–N2O2), and the respective Co−C*COOH bond length decreases from 1.88 to 1.86 Å (Fig. 3c). Hence, the improved matching of critical orbitals leads to the stabilization of the *COOH intermediate, thus lowering the ΔG of step * + CO2 → *COOH (Fig. 3a).
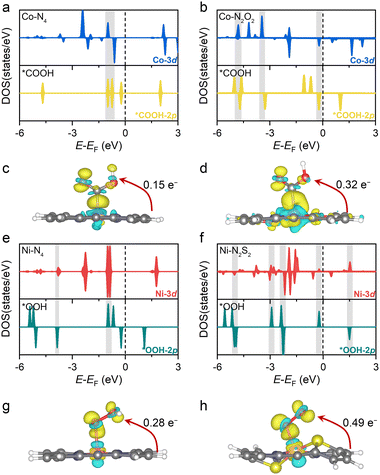 |
| Fig. 5 Projected electronic densities of states (PDOS) and charge density difference (CDD) of (a)–(d) *COOH intermediates adsorbed on Co–N4- and Co–N2O2-Por-COFs, and (e)–(h) *OOH intermediates adsorbed on Ni–N4- and Ni–N2S2-Por-COFs. The cyan/yellow colors indicate the regions of electron loss/gain. Isosurfaces of charge density are set to 0.005 e Å −3. For clarity, the tetraphenyl and the p-phenylenediamine (PPDA) moieties of M–NxOySz-Por-COFs are not shown. | |
Similarly, when comparing Ni–N4 and Ni–N2S2 for the 4e− ORR, the key intermediate *OOH was stabilized (Fig. 3b). A greater orbital overlap between Ni 3d orbitals and the 2p orbital of the adsorbed species was found as the coordination of Ni shifted from N4 to N2S2 (Fig. 5e, f and Fig. S22, ESI†). The charge transferred from the substrate to intermediates increases from 0.28 e− to 0.49 e− for *OOH (Fig. 5g and h), from 0.33 e− to 0.43 e− for *O (Fig. S22, ESI†), and from 0.46 e− to 0.57 e− for *OH. The differential stabilization of these species collectively contributes to the adsorption–desorption equilibrium of reaction intermediates, thus decreasing the overpotential of the Ni–N2S2-Por-COF (Fig. 3b).
Overall, the increased catalytic performance of Co–N2O2-and Ni–N2S2-Por-COFs is attributed to the stabilization of critical intermediates via the introduction of heteroatoms into the coordination. Such enhanced effects due to heteroatoms have also been observed in multiple electrocatalysts.92–94
3.4 Machine learning (ML)
Furthermore, we used ML to find the intrinsic characteristics that contribute to the differential CO2RR or ORR performance of the above Por-COFs. As listed in Table 1, twenty widely recognized features were selected as input features for training the ML models. Numeric values of the features are present in Table S10 (ESI†). Since the size of the sample set is small, 18 in our case (14 in the training set and 4 in the test set), we tried three algorithms: the random forest regression (RFR), gradient boosting regression (GBR), and extreme gradient boosting regression (XGBoost) algorithms. Then, GBR was selected for predicting
and XGBoost was selected for predicting ηORR, as these models yield the lowest root mean square error (RMSE) and the highest coefficient of determination value (R2 score) for the training set (Fig. 6 and Tables S11, 12, ESI†). A good linear correlation was achieved between the DFT-calculated and the ML-predicted values (Table S13, ESI†) of both
(Fig. 6a) and ηORR (Fig. 6b). The data of the test set are distributed near the diagonal line, indicating that the trained ML models are capable of predicting the catalytic activity of M-NxOySz-Por-COFs for CO2RR and ORR, where M = Fe/Co/Ni.
Table 1 Features used for training the ML models
Category |
Features |
Abbreviation |
Properties of central metal |
Atomic number |
N
atom
|
Number of valence electron |
n
e
|
Number of d electron |
n
d
|
Covalent radius |
r
atom
|
van der Waals radius |
r
vdW
|
Relative mass |
m
|
Pauling electronegativity |
EN |
Electron affinity |
EA |
First ionization energy |
IE |
Properties of coordinated atoms |
Number of coordinated N atoms |
n
N
|
Sum of valence electron count |
∑ne |
Sum of p electron |
∑np |
Sum of covalent radius |
∑ratom |
Sum of van der Waals radius |
∑rvdw |
Sum of Pauling electronegativity |
∑EN |
The sum of electron affinity |
∑EA |
DFT calculated bond length |
M–X1 |
d
M–X1
|
M–X2 |
d
M–X2
|
M–N1 |
d
M–N1
|
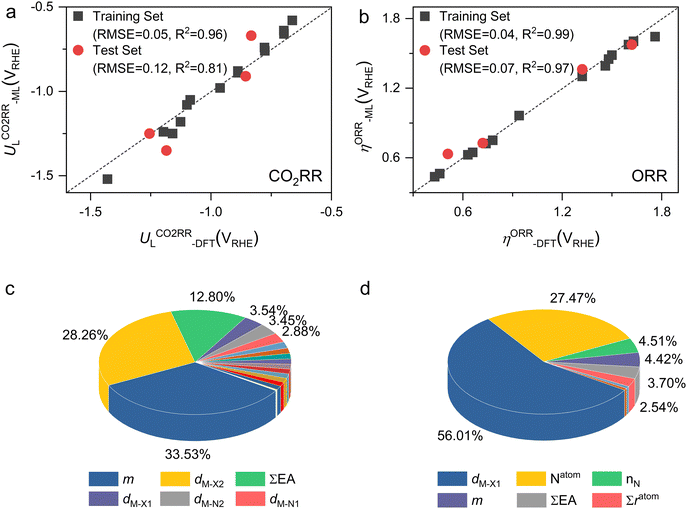 |
| Fig. 6 Comparison between DFT-calculated and ML-predicted values of (a) and (b) ηORR. Feature importance for (c) in the GBR model and (d) ηORR in the XGBoost model. | |
Then, feature importance analysis was performed based on the selected models to evaluate the significance of the features. For
, the most important features (Fig. 6c) are the relative mass of central metals, m (33.53%); the M–X2 bond length, dM–X2 (28.26%); and the sum of electron affinity of coordination environments, ∑EA (12.80%). Other bond length features, including dM–X1, dM–N2, and dM–N1 (each accounts for ∼3%), also have some importance. For ηORR, the most crucial features (Fig. 6d) are the M–X1 bond length, dM–X1 (56.01%), and the atomic number of central metals, Natom (27.47%); followed by the number of coordinated N atoms, nN, (4.51%), the relative mass of central metals, m (4.42%), the sum of electron affinity of coordination environments, ∑EA (3.70%) and the sum of covalent radius of coordination environments, ∑ratom (2.54%). The remaining features contribute insignificantly (Table S14, ESI†). The fact that the important features of CO2RR and ORR are different is not unexpected, because their inherent mechanisms are different. As a matter of fact, one can see that no single feature overwhelmingly dominates the prediction. Hence the interplay between the central metal and the coordinated atoms is very important for determining the catalytic capability.
We further tested the applicability of the trained model in predicting CO2RR or ORR catalytic performance of systems beyond the training set, i.e., central metal being Cu or Pt, and coordination atoms including C (refer to ESI† Note S1 for further details). There is a broad agreement on the catalytic order, which is inspiring; nevertheless, the match between ML-predicted and DFT-calculated values is not satisfactory (∼0.3 V error). We anticipate that increasing the sample size and type in the training set would improve the accuracy of the model, and this is currently underway in our group.
4 Conclusions
In summary, by adopting the strategy of substituting N-atoms with O/S-atoms in the metallo-porphyrin unit, our calculations have identified several types of NxOySx-coordinated metallo-Por-COFs as catalyst candidates for CO2 or O2 electroreduction. Since current efforts to enhance the catalytic performance of Por-COFs mainly focus on modifying the metal or the length or type of the linker, this study offers an alternative promising strategy: coordination engineering.
Changing the N4-coordination to NxOySz-coordination (1) improved the conductivity of the M-Por-COFs (M= Fe/Co/Ni), while maintaining their stability; (2) enhanced the CO2-to-CO reduction catalytic capability and selectivity against the HER in general; and (3) did not alter the preference for the 4e− ORR in comparison to the 2e− path, and increased the ORR activity for M = Ni, while hindering it for M = Fe/Co. We predict that the Co–N2O2-Por-COF
is the best catalyst candidate for CO2-to-CO reduction and the Ni–N2S2-Por-COF (ηORR = 0.46 V) is the best candidate for ORR-to-H2O reduction. The improved catalytic performance, as compared to their parent M–N4-system, is attributed to a better orbital match between the central metal and the adsorbed species, leading to the stabilization of the critical intermediate of the potential determining step. Feature importance analysis based on machine leaning models showed that the relative mass of central metals and the bond length between metal and coordinated heteroatoms are the most important intrinsic characteristics for activity prediction.
This study showcases that the coordination engineering is an effective strategy for improving the catalytic performance of Por-based COFs. We envision that this strategy will have broader applications beyond Por-COFs. Although this study presents valuable findings, the mechanisms were solely based on the energetics of stationary points and neglected the kinetics. Further exploration of the detailed mechanisms of the best catalyst candidates is warranted and the impact of pH and explicit solvent molecules shall be taken into account. Besides, although the machine learning model provides information on the weight of important features, the predictability of the model is unsatisfactory. To improve the predictability and transferability of the model, it is necessary to expand the size and diversity of the dataset. Furthermore, the combination of changing the coordination environment and the linkers is also worth exploring.
Author contributions
Zhixin Ren: conceptualization, investigation, methodology, formal analysis, visualization, and writing – original draft. Ke Gong: formal analysis. Bo Zhao: visualization. Shi-Lu Chen: methodology and writing – review and editing. Jing Xie: conceptualization, visualization, supervision, resources, project administration, and writing – review and editing.
Conflicts of interest
The authors declare that they have no conflict of interest.
Acknowledgements
This work is supported by the National Natural Science Foundation of China (no. 22273004 and 22173007), the Beijing Natural Science Foundation (no. 2222028), the Teli Fellowship, the Innovation Foundation (no. 2021CX01026) from the Beijing Institute of Technology (BIT), China, and the BIT Research and Innovation Promoting Project (no. 2023YCXY043).
Notes and references
- A. P. Côté, A. I. Benin, N. W. Ockwig, M. O'Keeffe, A. J. Matzger and O. M. Yaghi, Porous, crystalline, covalent organic frameworks, Science, 2005, 310, 1166–1170 CrossRef PubMed.
- P. J. Waller, F. Gándara and O. M. Yaghi, Chemistry of Covalent Organic Frameworks, Acc. Chem. Res., 2015, 48, 3053–3063 CrossRef CAS PubMed.
- C. S. Diercks and O. M. Yaghi, The atom, the molecule, and the covalent organic framework, Science, 2017, 355, eaal1585 CrossRef PubMed.
- J. Li, X. Jing, Q. Li, S. Li, X. Gao, X. Feng and B. Wang, Bulk COFs and COF nanosheets for electrochemical energy storage and conversion, Chem. Soc. Rev., 2020, 49, 3565–3604 RSC.
- Z. Alsudairy, N. Brown, A. Campbell, A. Ambus, B. Brown, K. Smith-Petty and X. Li, Covalent organic frameworks in heterogeneous catalysis: recent advances and future perspective, Mater. Chem. Front., 2023, 7, 3298–3331 RSC.
- S. Lin, C. S. Diercks, Y.-B. Zhang, N. Kornienko, E. M. Nichols, Y. Zhao, A. R. Paris, D. Kim, P. Yang, O. M. Yaghi and C. J. Chang, Covalent organic frameworks comprising cobalt porphyrins for catalytic CO2 reduction in water, Science, 2015, 349, 1208–1213 CrossRef CAS PubMed.
- C. S. Diercks, S. Lin, N. Kornienko, E. A. Kapustin, E. M. Nichols, C. Zhu, Y. Zhao, C. J. Chang and O. M. Yaghi, Reticular Electronic Tuning of Porphyrin Active Sites in Covalent Organic Frameworks for Electrocatalytic Carbon Dioxide Reduction, J. Am. Chem. Soc., 2018, 140, 1116–1122 CrossRef CAS PubMed.
- P. L. Cheung, S. K. Lee and C. P. Kubiak, Facile Solvent-Free Synthesis of Thin Iron Porphyrin COFs on Carbon Cloth Electrodes for CO2 Reduction, Chem. Mater., 2019, 31, 1908–1919 CrossRef CAS.
- Q. Wu, R.-K. Xie, M.-J. Mao, G.-L. Chai, J.-D. Yi, S.-S. Zhao, Y.-B. Huang and R. Cao, Integration of Strong Electron Transporter Tetrathiafulvalene into Metalloporphyrin-Based Covalent Organic Framework for Highly Efficient Electroreduction of CO2, ACS Energy Lett., 2020, 5, 1005–1012 CrossRef CAS.
- H.-J. Zhu, M. Lu, Y.-R. Wang, S.-J. Yao, M. Zhang, Y.-H. Kan, J. Liu, Y. Chen, S.-L. Li and Y.-Q. Lan, Efficient electron transmission in covalent organic framework nanosheets for highly active electrocatalytic carbon dioxide reduction, Nat. Commun., 2020, 11, 497 CrossRef CAS PubMed.
- S. An, C. Lu, Q. Xu, C. Lian, C. Peng, J. Hu, X. Zhuang and H. Liu, Constructing Catalytic Crown Ether-Based Covalent Organic Frameworks for Electroreduction of CO2, ACS Energy Lett., 2021, 6, 3496–3502 CrossRef CAS.
- M. Chen, H. Li, C. Liu, J. Liu, Y. Feng, A. G. H. Wee and B. Zhang, Porphyrin- and porphyrinoid-based covalent organic frameworks (COFs): From design, synthesis to applications, Coord. Chem. Rev., 2021, 435, 213778 CrossRef CAS.
- W. Ji, T.-X. Wang, X. Ding, S. Lei and B.-H. Han, Porphyrin- and phthalocyanine-based porous organic polymers: From synthesis to application, Coord. Chem. Rev., 2021, 439, 213875 CrossRef CAS.
- Z. Liang, H.-Y. Wang, H. Zheng, W. Zhang and R. Cao, Porphyrin-based frameworks for oxygen electrocatalysis and catalytic reduction of carbon dioxide, Chem. Soc. Rev., 2021, 50, 2540–2581 RSC.
- Q. Wu, M.-J. Mao, Q.-J. Wu, J. Liang, Y.-B. Huang and R. Cao, Construction of Donor–Acceptor Heterojunctions in Covalent Organic Framework for Enhanced CO2 Electroreduction, Small, 2021, 17, 2004933 CrossRef CAS PubMed.
- J.-Y. Yue, Y.-T. Wang, X. Wu, P. Yang, Y. Ma, X.-H. Liu and B. Tang, Two-dimensional porphyrin covalent organic frameworks with tunable catalytic active sites for the oxygen reduction reaction, Chem. Commun., 2021, 57, 12619–12622 RSC.
- H. Dong, M. Lu, Y. Wang, H.-L. Tang, D. Wu, X. Sun and F.-M. Zhang, Covalently anchoring covalent organic framework on carbon nanotubes for highly efficient electrocatalytic CO2 reduction, Appl. Catal., B, 2022, 303, 120897 CrossRef CAS.
- M. Fang, L. Xu, H. Zhang, Y. Zhu and W.-Y. Wong, Metalloporphyrin-Linked Mercurated Graphynes for Ultrastable CO2 Electroreduction to CO with Nearly 100% Selectivity at a Current Density of 1.2 A cm−2, J. Am. Chem. Soc., 2022, 144, 15143–15154 CrossRef CAS PubMed.
- T. He, C. Yang, Y. Chen, N. Huang, S. Duan, Z. Zhang, W. Hu and D. Jiang, Bottom-Up Interfacial Design of Covalent Organic Frameworks for Highly Efficient and Selective Electrocatalysis of CO2, Adv. Mater., 2022, 34, 2205186 CrossRef CAS PubMed.
- S. Huang, K. Chen and T.-T. Li, Porphyrin and phthalocyanine based covalent organic frameworks for electrocatalysis, Coord. Chem. Rev., 2022, 464, 214563 CrossRef CAS.
- M. Liu, S. Liu, C.-X. Cui, Q. Miao, Y. He, X. Li, Q. Xu and G. Zeng, Construction of Catalytic Covalent Organic Frameworks with Redox-Active Sites for the Oxygen Reduction and the Oxygen Evolution Reaction, Angew. Chem., Int. Ed., 2022, 61, e202213522 CrossRef CAS PubMed.
- Y.-R. Wang, H.-M. Ding, X.-Y. Ma, M. Liu, Y.-L. Yang, Y. Chen, S.-L. Li and Y.-Q. Lan, Imparting CO2 Electroreduction Auxiliary for Integrated Morphology Tuning and Performance Boosting in a Porphyrin-based Covalent Organic Framework, Angew. Chem., Int. Ed., 2022, 61, e202114648 CrossRef CAS PubMed.
- S. Bhunia, A. Peña-Duarte, H. Li, H. Li, M. F. Sanad, P. Saha, M. A. Addicoat, K. Sasaki, T. A. Strom, M. J. Yacamán, C. R. Cabrera, R. Seshadri, S. Bhattacharya, J.-L. Brédas and L. Echegoyen, [2,1,3]-Benzothiadiazole-Spaced Co-Porphyrin-Based Covalent Organic Frameworks for O2 Reduction, ACS Nano, 2023, 17, 3492–3505 CrossRef CAS PubMed.
- Y. Yuan, K.-T. Bang, R. Wang and Y. Kim, Macrocycle-Based Covalent Organic Frameworks, Adv. Mater., 2023, 35, 2210952 CrossRef CAS PubMed.
- N. Huang, K. H. Lee, Y. Yue, X. Xu, S. Irle, Q. Jiang and D. Jiang, A Stable and Conductive Metallophthalocyanine Framework for Electrocatalytic Carbon Dioxide Reduction in Water, Angew. Chem., Int. Ed., 2020, 59, 16587–16593 CrossRef CAS PubMed.
- G. Lu, H. Yang, Y. Zhu, T. Huggins, Z. J. Ren, Z. Liu and W. Zhang, Synthesis of a conjugated porous Co(II) porphyrinylene–ethynylene framework through alkyne metathesis and its catalytic activity study, J. Mater. Chem. A, 2015, 3, 4954–4959 RSC.
- Z. Zhang, W. Wang, X. Wang, L. Zhang, C. Cheng and X. Liu, Ladder-type π-conjugated metallophthalocyanine covalent organic frameworks with boosted oxygen reduction reaction activity and durability for zinc-air batteries, Chem. Eng. J., 2022, 435, 133872 CrossRef CAS.
- X. Zhang, Y. Yuan, H. Li, Q. Wu, H. Zhu, Y. Dong, Q. Wu, Y. Huang and R. Cao, Viologen linker as a strong electron-transfer mediator in the covalent organic framework to enhance electrocatalytic CO2 reduction, Mater. Chem. Front., 2023, 7, 2661–2670 RSC.
- X. Li, H. Rong, J. Zhang, D. Wang and Y. Li, Modulating the local coordination environment of single-atom catalysts for enhanced catalytic performance, Nano Res., 2020, 13, 1842–1855 CrossRef CAS.
- Y. Zhu, J. Sokolowski, X. Song, Y. He, Y. Mei and G. Wu, Engineering Local Coordination Environments of Atomically Dispersed and Heteroatom-Coordinated Single Metal Site Electrocatalysts for Clean Energy-Conversion, Adv. Energy Mater., 2020, 10, 1902844 CrossRef CAS.
- W. Xu, H. Tang, H. Gu, H. Xi, P. Wu, B. Liang, Q. Liu and W. Chen, Research progress of asymmetrically coordinated single-atom catalysts for electrocatalytic reactions, J. Mater. Chem. A, 2022, 10, 14732–14746 RSC.
- K. Liu, P. Chen, Z. Sun, W. Chen, Q. Zhou and X. Gao, The atomic interface effect of single atom catalysts for electrochemical hydrogen peroxide production, Nano Res., 2023, 16, 10724–10741 CrossRef CAS.
- X. Xie, H. Peng, G. Ma, Z. Lei and Y. Xu, Recent progress in heteroatom doping to modulate the coordination environment of M–N–C catalysts for the oxygen reduction reaction, Mater. Chem. Front., 2023, 7, 2595–2619 RSC.
- X. Zhao, S. Huang, Z. Chen, C. Lu, S. Han, C. Ke, J. Zhu, J. Zhang, D. Tranca and X. Zhuang, Carbon nanosheets supporting Ni–N3S single-atom sites for efficient electrocatalytic CO2 reduction, Carbon, 2021, 178, 488–496 CrossRef CAS.
- P. Song, B. Hu, D. Zhao, J. Fu, X. Su, W. Feng, K. Yu, S. Liu, J. Zhang and C. Chen, Modulating the Asymmetric Atomic Interface of Copper Single Atoms for Efficient CO2 Electroreduction, ACS Nano, 2023, 17, 4619–4628 CrossRef CAS PubMed.
- Y. Li, Y. Ding, B. Zhang, Y. Huang, H. Qi, P. Das, L. Zhang, X. Wang, Z.-S. Wu and X. Bao, N,O symmetric double coordination of an unsaturated Fe single-atom confined within a graphene framework for extraordinarily boosting oxygen reduction in Zn–air batteries, Energy Environ. Sci., 2023, 16, 2629–2636 RSC.
- Y. Wang, R. Shi, L. Shang, G. I. N. Waterhouse, J. Zhao, Q. Zhang, L. Gu and T. Zhang, High-Efficiency Oxygen Reduction to Hydrogen Peroxide Catalyzed by Nickel Single-Atom Catalysts with Tetradentate N2O2 Coordination in a Three-Phase Flow Cell, Angew. Chem., Int. Ed., 2020, 59, 13057–13062 CrossRef CAS.
- P. Hou, Y. Huang, F. Ma, X. Wei, R. Du, G. Zhu, J. Zhang and M. Wang, S and N coordinated single-atom catalysts for electrochemical CO2 reduction with superior activity and selectivity, Appl. Surf. Sci., 2023, 619, 156747 CrossRef CAS.
- S. Wang, B. Huang, Y. Dai and W. Wei, Tuning the Coordination Microenvironment of Central Fe Active Site to Boost Water Electrolysis and Oxygen Reduction Activity, Small, 2023, 19, 2205111 CrossRef CAS.
- T. Chatterjee, V. S. Shetti, R. Sharma and M. Ravikanth, Heteroatom-Containing Porphyrin Analogues, Chem. Rev., 2017, 117, 3254–3328 CrossRef CAS PubMed.
- P. J. Chmielewski, L. Latos-Grażyński, M. M. Olmstead and A. L. Balch, Nickel Complexes of 21-Oxaporphyrin and 21, 23-Dioxaporphyrin, Chem. – Eur. J., 1997, 3, 268–278 CrossRef CAS PubMed.
- M. Pawlicki and L. Latos-Grazyński, Iron complexes of 5,10,15,20-tetraphenyl-21-oxaporphyrin, Inorg. Chem., 2002, 41, 5866–5873 CrossRef CAS PubMed.
- S. Stute, L. Götzke, D. Meyer, M. L. Merroun, P. Rapta, O. Kataeva, W. Seichter, K. Gloe, L. Dunsch and K. Gloe, Molecular Structure, UV/Vis Spectra, and Cyclic Voltammograms of Mn(II), Co(II), and Zn(II) 5,10,15,20-Tetraphenyl-21-oxaporphyrins, Inorg. Chem., 2013, 52, 1515–1524 CrossRef CAS PubMed.
- J. Lisowski, L. Latos-Grazynski and L. Szterenberg, Nuclear magnetic resonance study of the molecular and electronic structure of nickel(II) tetraphenyl-21-thiaporphyrins, Inorg. Chem., 1992, 31, 1933–1940 CrossRef CAS.
- L. Latos-Grazynski, J. Lisowski, P. Chmielewski, M. Grzeszczuk, M. M. Olmstead and A. L. Balch, Palladium complexes of 21-thiaporphyrin: syntheses and characterization, Inorg. Chem., 1994, 33, 192–197 CrossRef CAS.
- I. Gupta and M. Ravikanth, Synthesis of meso-furyl porphyrins with N4, N3S, N2S2 and N3O porphyrin cores, Tetrahedron, 2003, 59, 6131–6139 CrossRef CAS.
- T. Kaur, A. Ghosh, P. Rajakannu and M. Ravikanth, Synthesis and crystal structure of the rhenium(I) tricarbonyl complex of 5,10,15,20-tetra-p-tolyl-21,23-dithiaporphyrin, Inorg. Chem., 2014, 53, 2355–2357 CrossRef CAS PubMed.
- T. Kaur, W.-Z. Lee and M. Ravikanth, Rhenium(I) Tricarbonyl Complexes of meso-Tetraaryl-21,23-diheteroporphyrins, Inorg. Chem., 2016, 55, 5305–5311 CrossRef CAS PubMed.
- S. Punidha, N. Agarwal, R. Burai and M. Ravikanth, Synthesis of N3S, N3O, N2S2, N2O2, N2SO and N2OS Porphyrins with One meso-Unsubstituted Carbon, Eur. J. Org. Chem., 2004, 2223–2230 CrossRef CAS.
- H. Kim, D. Shin, W. Yang, D. H. Won, H.-S. Oh, M. W. Chung, D. Jeong, S. H. Kim, K. H. Chae, J. Y. Ryu, J. Lee, S. J. Cho, J. Seo, H. Kim and C. H. Choi, Identification of Single-Atom Ni Site Active toward Electrochemical CO2 Conversion to CO, J. Am. Chem. Soc., 2021, 143, 925–933 CrossRef CAS PubMed.
- Y. Meng, Y. Luo, J.-L. Shi, H. Ding, X. Lang, W. Chen, A. Zheng, J. Sun and C. Wang, 2D and 3D Porphyrinic Covalent Organic Frameworks: The Influence of Dimensionality on Functionality, Angew. Chem., Int. Ed., 2020, 59, 3624–3629 CrossRef CAS PubMed.
- G. Kresse and J. Hafner, Ab initio molecular dynamics for open-shell transition metals, Phys. Rev. B: Condens. Matter Mater. Phys., 1993, 48, 13115–13118 CrossRef CAS PubMed.
- G. Kresse and J. Furthmüller, Efficient iterative schemes for ab initio total-energy calculations using a plane-wave basis set, Phys. Rev. B: Condens. Matter Mater. Phys., 1996, 54, 11169–11186 CrossRef CAS PubMed.
- J. P. Perdew, K. Burke and M. Ernzerhof, Generalized Gradient Approximation Made Simple, Phys. Rev. Lett., 1996, 77, 3865–3868 CrossRef CAS PubMed.
- S. Grimme, J. Antony, S. Ehrlich and H. Krieg, A consistent and accurate ab initio parametrization of density functional dispersion correction (DFT-D) for the 94 elements H-Pu, J. Chem. Phys., 2010, 132, 154104 CrossRef PubMed.
- S. Grimme, S. Ehrlich and L. Goerigk, Effect of the damping function in dispersion corrected density functional theory, J. Comput. Chem., 2011, 32, 1456–1465 CrossRef CAS PubMed.
- P. E. Blöchl, Projector augmented-wave method, Phys. Rev. B: Condens. Matter Mater. Phys., 1994, 50, 17953–17979 CrossRef.
- G. Kresse and D. Joubert, From ultrasoft pseudopotentials to the projector augmented-wave method, Phys. Rev. B: Condens. Matter Mater. Phys., 1999, 59, 1758–1775 CrossRef CAS.
- S. L. Dudarev, G. A. Botton, S. Y. Savrasov, C. J. Humphreys and A. P. Sutton, Electron-energy-loss spectra and the structural stability of nickel oxide: An LSDA + U study, Phys. Rev. B: Condens. Matter Mater. Phys., 1998, 57, 1505–1509 CrossRef CAS.
- H. Xu, D. Cheng, D. Cao and X. C. Zeng, A universal principle for a rational design of single-atom electrocatalysts, Nat. Catal., 2018, 1, 339–348 CrossRef CAS.
- K. Mathew, R. Sundararaman, K. Letchworth-Weaver, T. A. Arias and R. G. Hennig, Implicit solvation model for density-functional study of nanocrystal surfaces and reaction pathways, J. Chem. Phys., 2014, 140, 084106 CrossRef PubMed.
- K. Mathew, V. S. C. Kolluru, S. Mula, S. N. Steinmann and R. G. Hennig, Implicit self-consistent electrolyte model in plane-wave density-functional theory, J. Chem. Phys., 2019, 151, 234101 CrossRef PubMed.
- J. K. Nørskov, J. Rossmeisl, A. Logadottir, L. Lindqvist, J. R. Kitchin, T. Bligaard and H. Jónsson, Origin of the Overpotential for Oxygen Reduction at a Fuel-Cell Cathode, J. Phys. Chem. B, 2004, 108, 17886–17892 CrossRef.
- S. Cao, S. Wei, X. Wei, S. Zhou, H. Chen, Y. Hu, Z. Wang, S. Liu, W. Guo and X. Lu, Can N, S Cocoordination Promote Single Atom Catalyst Performance in CO2RR? Fe–N2S2 Porphyrin versus Fe–N4 Porphyrin, Small, 2021, 17, 2100949 CrossRef CAS PubMed.
- A. Nagai, X. Chen, X. Feng, X. Ding, Z. Guo and D. Jiang, A Squaraine-Linked Mesoporous Covalent Organic Framework, Angew. Chem., Int. Ed., 2013, 52, 3770–3774 CrossRef CAS PubMed.
- C. Liu, H. Li, F. Liu, J. Chen, Z. Yu, Z. Yuan, C. Wang, H. Zheng, G. Henkelman, L. Wei and Y. Chen, Intrinsic Activity of Metal Centers in Metal-Nitrogen-Carbon Single-Atom Catalysts for Hydrogen Peroxide Synthesis, J. Am. Chem. Soc., 2020, 142, 21861–21871 CrossRef CAS.
- J.-D. Yi, R. Xu, G.-L. Chai, T. Zhang, K. Zang, B. Nan, H. Lin, Y.-L. Liang, J. Lv, J. Luo, R. Si, Y.-B. Huang and R. Cao, Cobalt single-atoms anchored on porphyrinic triazine-based frameworks as bifunctional electrocatalysts for oxygen reduction and hydrogen evolution reactions, J. Mater. Chem. A, 2019, 7, 1252 RSC.
- S. Back, J. Lim, N. Kim, Y. Kim and Y. Jung, Single-atom catalysts for CO2 electroreduction with significant activity and selectivity improvements, Chem. Sci., 2017, 8, 1090–1096 RSC.
- Y. Su, L. Zhang, Y. Wang, J. Liu, V. Muravev, K. Alexopoulos, I. A. W. Filot, D. G. Vlachos and E. J. M. Hensen, Stability of heterogeneous single-atom catalysts: a scaling law mapping thermodynamics to kinetics, npj Comput. Mater., 2020, 6, 144 CrossRef CAS.
- M. Ha, D. Y. Kim, M. Umer, V. Gladkikh, C. W. Myung and K. S. Kim, Tuning metal single atoms embedded in NxCy moieties toward high-performance electrocatalysis, Energy Environ. Sci., 2021, 14, 3455–3468 RSC.
- J. Greeley and J. K. Nørskov, Electrochemical dissolution of surface alloys in acids: Thermodynamic trends from first-principles calculations, Electrochim. Acta, 2007, 52, 5829–5836 CrossRef CAS.
- C. Fang, J. Zhou, L. Zhang, W. Wan, Y. Ding and X. Sun, Synergy of dual-atom catalysts deviated from the scaling relationship for oxygen evolution reaction, Nat. Commun., 2023, 14, 4449 CrossRef CAS PubMed.
- X. Guo, S. Zhang, L. Kou, C. Yam, T. Frauenheim, Z. Chen and S. Huang, Data-driven pursuit of electrochemically stable 2D materials with basal plane activity toward oxygen electrocatalysis, Energy Environ. Sci., 2023, 16, 5003–5018 RSC.
- F. Pedregosa, G. Varoquaux, A. Gramfort, V. Michel, B. Thirion, O. Grisel, M. Blondel, P. Prettenhofer, R. Weiss, V. Dubourg, J. Vanderplas, A. Passos, D. Cournapeau, M. Brucher, M. Perrot and É. Duchesnay, Scikit-learn: Machine Learning in Python, J. Mach. Learn. Res., 2011, 12, 2825–2830 Search PubMed.
- L. Breiman, Random Forests, Mach. Learn., 2001, 45, 5–32 CrossRef.
- J. H. Friedman, Greedy function approximation: A gradient boosting machine, Ann. Stat., 2001, 29, 1189–1232 Search PubMed.
- J. H. Friedman, Stochastic gradient boosting, Comput. Stat. Data Anal., 2002, 38, 367–378 CrossRef.
- T. G. Dietterich, Approximate Statistical Tests for Comparing Supervised Classification Learning Algorithms, Neural Comput., 1998, 10, 1895–1923 CrossRef PubMed.
- H. Mai, T. C. Le, D. Chen, D. A. Winkler and R. A. Caruso, Machine Learning for Electrocatalyst and Photocatalyst Design and Discovery, Chem. Rev., 2022, 122, 13478–13515 CrossRef CAS PubMed.
- Z. Jin, D. Jiao, Y. Dong, L. Liu, J. Fan, M. Gong, X. Ma, Y. Wang, W. Zhang, L. Zhang, Z. G. Yu, D. Voiry, W. Zheng and X. Cui, Boosting Electrocatalytic Carbon Dioxide Reduction via Self-Relaxation of Asymmetric Coordination in Fe-based Single Atom Catalyst, Angew. Chem., Int. Ed., 2023, e202318246 Search PubMed.
- H. Jia, A. Nandy, M. Liu and H. J. Kulik, Modeling the roles of rigidity and dopants in single-atom methane-to-methanol catalysts, J. Mater. Chem. A, 2022, 10, 6193–6203 RSC.
- Z. Ren, B. Zhao and J. Xie, Designing N-Confused Metalloporphyrin-Based Covalent Organic Frameworks for Enhanced Electrocatalytic Carbon Dioxide Reduction, Small, 2023, 19, 2301818 CrossRef CAS PubMed.
- A. S. Hall, Y. Yoon, A. Wuttig and Y. Surendranath, Mesostructure-Induced Selectivity in CO2 Reduction Catalysis, J. Am. Chem. Soc., 2015, 137, 14834–14837 CrossRef CAS PubMed.
- X.-Q. Li, G.-Y. Duan, J.-W. Chen, L.-J. Han, S.-J. Zhang and B.-H. Xu, Regulating electrochemical CO2RR selectivity at industrial current densities by structuring copper@poly(ionic liquid) interface, Appl. Catal., B, 2021, 297, 120471 CrossRef CAS.
- S. Banerjee, C. S. Gerke and V. S. Thoi, Guiding CO2RR Selectivity by Compositional Tuning in the Electrochemical Double Layer, Acc. Chem. Res., 2022, 55, 504–515 CrossRef CAS PubMed.
- Y.-Q. Zhang, J.-Y. Chen, P. E. M. Siegbahn and R.-Z. Liao, Harnessing Noninnocent Porphyrin Ligand to Circumvent Fe-Hydride Formation in the Selective Fe-Catalyzed CO2 Reduction in Aqueous Solution, ACS Catal., 2020, 10, 6332–6345 CrossRef CAS.
- L.-L. Shi, M. Li, B. You and R.-Z. Liao, Theoretical Study on the Electro-Reduction of Carbon Dioxide to Methanol Catalyzed by Cobalt Phthalocyanine, Inorg. Chem., 2022, 61, 16549–16564 CrossRef CAS PubMed.
- Y.-C. Cao, L.-L. Shi, M. Li, B. You and R.-Z. Liao, Deciphering the Selectivity of the Electrochemical CO2 Reduction to CO by a Cobalt Porphyrin Catalyst in Neutral Aqueous Solution: Insights from DFT Calculations, ChemistryOpen, 2023, 12, e202200254 CrossRef CAS PubMed.
- J.-Y. Chen, M. Li and R.-Z. Liao, Mechanistic Insights into Photochemical CO2 Reduction to CH4 by a Molecular Iron−Porphyrin Catalyst, Inorg. Chem., 2023, 62, 9400–9417 CrossRef CAS PubMed.
- H. Rao, L. C. Schmidt, J. Bonin and M. Robert, Visible-light-driven methane formation from CO2 with a molecular iron catalyst, Nature, 2017, 548, 74–77 CrossRef CAS PubMed.
- Y. Wu, Z. Jiang, X. Lu, Y. Liang and H. Wang, Domino electroreduction of CO2 to methanol on a molecular catalyst, Nature, 2019, 575, 639–642 CrossRef CAS PubMed.
- X. Li, H. Zhang, Q. Hu, W. Zhou, J. Shao, X. Jiang, C. Feng, H. Yang and C. He, Unlocking the Transition of Electrochemical Water Oxidation Mechanism Induced by Heteroatom Doping, Angew. Chem., Int. Ed., 2023, 62, e202309732 CrossRef CAS PubMed.
- C. Feng, M. Lv, J. Shao, H. Wu, W. Zhou, S. Qi, C. Deng, X. Chai, H. Yang, Q. Hu and C. He, Lattice Strain Engineering of Ni2P Enables Efficient Catalytic Hydrazine Oxidation-Assisted Hydrogen Production, Adv. Mater., 2023, 35, 2305598 CrossRef CAS PubMed.
- Q. Hu, K. Gao, X. Wang, H. Zheng, J. Cao, L. Mi, Q. Huo, H. Yang, J. Liu and C. He, Subnanometric Ru clusters with upshifted D band center improve performance for alkaline hydrogen evolution reaction, Nat. Commun., 2022, 13, 3958 CrossRef CAS PubMed.
|
This journal is © the Partner Organisations 2024 |