DOI:
10.1039/D4MH00053F
(Communication)
Mater. Horiz., 2024,
11, 2106-2114
Ovonic threshold switching-based artificial afferent neurons for thermal in-sensor computing†
Received
16th January 2024
, Accepted 19th March 2024
First published on 21st March 2024
Abstract
Artificial afferent neurons in the sensory nervous system inspired by biology have enormous potential for efficiently perceiving and processing environmental information. However, the previously reported artificial afferent neurons suffer from two prominent challenges: considerable power consumption and limited scalability efficiency. Herein, addressing these challenges, a bioinspired artificial thermal afferent neuron based on a N-doped SiTe ovonic threshold switching (OTS) device is presented for the first time. The engineered OTS device shows remarkable uniformity and robust endurance, ensuring the reliability and efficacy of the artificial afferent neurons. A substantially decreased leakage current of the SiTe OTS device by nitrogen doping results in ultra-low power consumption less than 0.3 nJ per spike for artificial afferent neurons. The inherent temperature response exhibited by N-doped SiTe OTS materials allows us to construct a highly compact artificial thermal afferent neuron over a wide temperature range. An edge detection task is performed to further verify its thermal perceptual computing function. Our work provides an insight into OTS-based artificial afferent neurons for electronic skin and sensory neurorobotics.
New concepts
Recent literature on afferent neurons focuses mostly on metal–insulator transition (MIT) materials, which suffer from two prominent challenges: considerable power consumption and limited scalability efficiency. From the perspective of core material design, this research demonstrates a temperature-sensing artificial afferent neuron based on an ovonic threshold switching (OTS) material that features low power consumption and a compact circuit structure. These advantages are due to the decreased leakage current by nitrogen doping and the inherent temperature response of OTS. An edge detection task is performed to further verify the thermal perceptual computing function of artificial afferent neurons. The finding of this study provides a new material approach for the study of electronic skin and sensory neurorobotics.
|
1. Introduction
With the emergence of artificial intelligence and internet of things (AIoT), an immense volume of data from ubiquitous edge devices necessitates real-time processing with high energy efficiency.1,2 To overcome the von Neumann bottleneck prevalent in the traditional computational paradigm, neuromorphic computing architecture at the edge has emerged as a compelling contender for its advantageous energy and area efficiency.3,4 Drawing inspiration from the human capacity for perceiving and processing environmental information with remarkable parallelism and minimal power consumption,5–7 the artificial afferent neurons in the sensory nervous system play a pivotal role in neuromorphic computing hardware, implementing the function of converting analog signals into electrical neural spikes.8,9 Moreover, these synthetically perceptive artificial afferent neurons can be applied to abundant scenarios at the edge, such as intelligent sensors, image recognizers and nociceptors, which provides potential for bio-electronic interfaces and the input of the spiking neural network (SNN).10–16 However, the conventional CMOS-based artificial afferent neurons require intricate peripheral circuits including analog-to-digital converters (ADCs) and voltage-to-spike converters (VSCs),17,18 resulting in inefficiencies in energy and overall area utilization.19
Recently, emerging devices exhibiting metal–insulator transition (MIT) behavior represented by VOx and NbOx have been favorable for constructing artificial afferent neurons in extensive research studies.20–23 These novel artificial neurons with a simple circuit structure show better scalability compared with CMOS-based ones. Nevertheless, they usually suffer from considerable power consumption due to the high leakage current.24 On the other hand, various sensors have to be introduced into the neuron circuit as MIT devices are insensitive to external signals, such as light or heat. Additional sensors result in the physical separation of sensing and computing functions for artificial afferent neurons, which will increase the area overhead inevitably. To tackle these problems, here, a novel device with low leakage current and direct response to external signals is urgently required to replace the MIT devices and sensors in the above afferent neuron circuits to achieve both low power consumption and high scalability for the integration of sensing and computing. As a chalcogenide threshold device, OTS is more suitable for building afferent neurons owing to its typical semiconductor characteristics.25,26 Te-based OTS devices exhibit good comprehensive performance and simple composition in the family of OTS materials,27 yet binary Te-based OTS devices have a relatively large leakage current.28,29
In this work, we propose an N-doped SiTe OTS device used as a temperature-sensing artificial afferent neuron with low power consumption. Nitrogen doping enables the SiTe OTS device to have an extremely low leakage current of 5.5 nA; hence, ultra-low power consumption less than 0.3 nJ per spike for this artificial afferent neuron is acquired. At the same time, the superior cycle-to-cycle uniformity of the N-doped SiTe device ensures a stable spike frequency for afferent neurons. Furthermore, taking advantage of the inherent temperature response of our OTS device, a highly compact artificial thermal afferent neuron was put forward, which is capable of sensing temperatures ranging from 25 °C to 100 °C without extra sensors and then converting them into spike signals with different frequencies. Finally, we simulate a sensory array based on a frequency-encoded artificial thermal afferent neuron to accomplish an edge detection task. The experimental results prove that our OTS-based artificial afferent neuron shows great potential for use in highly efficient and compact neuromorphic perception systems.
2. Results and discussion
2.1. Electrical characteristics of OTS devices
We fabricated N-doped SiTe OTS devices with a via-hole structure, as well as SiTe OTS control samples with the same feature size. Fig. 1a shows an SEM image of the via-hole array and an enlarged view of the via-hole with a diameter of 250 nm. Fig. 1b depicts the schematic and cross-sectional TEM images of the OTS devices with avia-hole structure. Pristine OTS devices require a large voltage to be applied during the initial electroforming process (Fig. S1, ESI†). After forming, the typical DC I–V characteristics of the SiTe OTS devices with and without nitrogen doping are shown in Fig. 1c. The current in the subthreshold region increases exponentially with the applied voltage mainly due to Poole–Frenkel (PF) emission.30 Both OTS devices exhibit threshold switching behavior with an abrupt current increasing to 50 μA (compliance current) at the threshold voltage (Vth). OTS devices remain in the ON state until the applied voltage decreases below the hold voltage (Vh). The leakage current at half of the Vth drops more than one order of magnitude from 86.8 nA to 5.5 nA by nitrogen doping. The extremely low leakage current of N-doped SiTe is crucial for the realization of artificial afferent neurons with low power consumption.20 Besides the voltage sweep mode, the current sweep was tested to further observe device characteristics (Fig. S2, ESI†). Variations in the I–V characteristics with the same scanning parameters of all 30 N-doped SiTe devices are compared in Fig. 1d, which presents a distinct switching window in the DC sweep. The temperature-dependent I–V characteristics of the N-doped SiTe OTS device were measured, as presented in Fig. 1e. The threshold voltage decreases from about 1 V to 0.5 V as the temperature increases from 25 °C to 100 °C. Therefore, the inherent response of the OTS device to the temperature can be exploited to build a highly compact afferent neuron for thermal perceptual computing.
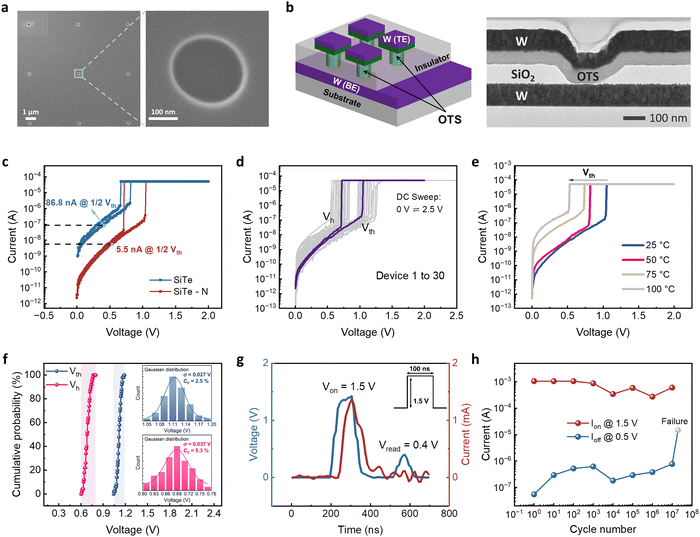 |
| Fig. 1 Structure and electrical characteristics of the OTS devices. (a) SEM image of the via-hole array (left) and an enlarged view of the via-hole (right). (b) Schematic and cross-sectional TEM images of OTS devices with a via-hole structure. (c) The typical DC I–V sweep of SiTe and N-doped SiTe devices with a compliance current of 50 μA. (d) I–V curves of the DC sweep on 30 N-doped SiTe devices. (e) I–V characteristics of the N-doped SiTe OTS device at different temperatures. (f) Statistical distributions of Vth and Vh of the N-doped SiTe device under 100 consecutive triangular voltage pulses (2.5 V amplitude and 10 μs rising/falling edges). The normal distributions of Vth and Vh are shown in insets. (g) Switching behavior of the N-doped SiTe device at a square pulse. (h) Endurance test of the N-doped SiTe device. | |
Fig. 1f displays the distributions of Vth and Vh of the N-doped SiTe OTS device under 100 consecutive triangular voltage pulses (2.5 V amplitude and 10 μs rising/falling edges). Vth and Vh are extracted from each dynamical transient response of threshold switching (Fig. S3, ESI†). Vth and Vh distribute tightly around 1.12 V and 0.69 V with a very small coefficient of variation (CV = σ/μ, where σ is standard deviation and μ is mean value) of 2.5% and 5.3%, respectively. Both Vth and Vh conform to a normal distribution, as illustrated in the insets of Fig. 1f. The excellent uniformity in Vth and Vh of the N-doped SiTe OTS device would be conducive for achieving a steady electrical oscillation behavior in afferent neurons. Fig. 1g confirms that this device can be turned on by a square pulse with a 1.5 V amplitude and turned off when the pulse is removed. Next, the above pulses were applied to the device to test its cycle endurance. According to Fig. 1h, the N-doped SiTe device can withstand square pulses for up to ∼107 cycles with a stable on/off current, which is ten times larger than that of a memory cell, about 106 cycles in general.31 It should be noted that the endurance of OTS is of critical importance for artificial neurons in the training and inference of hardware neural networks. The switching speed was also tested, and the device was turned on within ∼15 ns at a voltage of 1.68 V, which was collected by an oscilloscope (Fig. S4, ESI†). This switching speed is faster than that of MIT devices (>100 ns) and fully meets the requirement for electrical spikes emitted by afferent neurons.22,23
2.2. Photoelectron spectroscopy analysis and the band structure of OTS
Photoelectron spectroscopy measurements were performed to clarify the role of nitrogen doping in the leakage current reduction, and the results are shown in Fig. 2. XPS was performed to examine the elemental composition and chemical valence states of N-doped SiTe. Fig. 2a displays the typical XPS spectra of the as-deposited N-doped SiTe thin film, where the peak located at 103.91 eV is associated with the Si2p state and two spin–orbit coupling splitting peaks observed at 573.81 eV and 584.21 eV correspond to the 3d5/2 and 3d3/2 states of Te, respectively.32 The peak located at 398.96 eV of N1s indicates the existence of a certain amount of Si–N bonds,33 and the atomic percentage of the N element in N-doped SiTe is 2.4% by comparing the peak area. To further understand the effect of nitrogen doping on the band structure and electron transport behavior of the OTS, UV-vis-NIR absorption spectra and UPS were measured to determine the energy band structure of the SiTe-based OTS with and without nitrogen doping. As shown in Fig. 2b, the band gap of amorphous N-doped SiTe and SiTe is quite close, around 0.79 eV by linear fitting of the near absorption edge according to the Tauc plot: (αhν)1/2 = B (hν − Eg), where α is the absorption coefficient, hν is the photon energy, B is a constant and Eg is the band gap. The ultraviolet photoelectron spectra shown in Fig. 2c were used to examine the work functions of the thin films. The Fermi energy (EF) of N-doped SiTe combined with the cutoff edges of the low binding energy region was estimated to be −4.83 eV, which shifted to a lower energy position compared to that of the SiTe thin film. According to the inset of Fig. 2c, the valence band maximum (VBM, EV) values of N-doped SiTe and SiTe were calculated to be −5.24 eV and −5.21 eV, respectively.
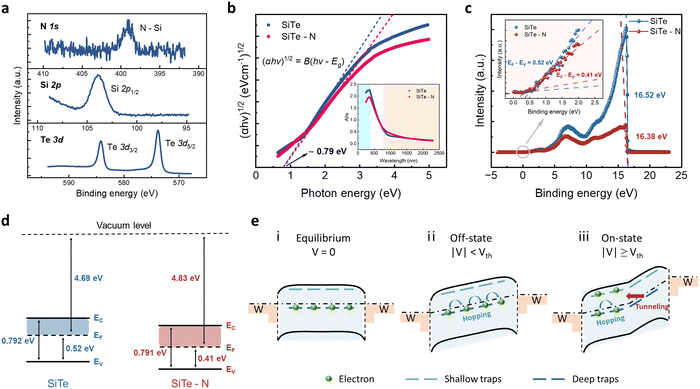 |
| Fig. 2 Photoelectron spectroscopy analysis of the OTS film. (a) XPS spectra of the N-doped SiTe film. (b) UV-vis-NIR absorption spectra (inset: absorbance versus wavelength of light) and (c) UPS (inset: enlarged view near the zero binding energy) of SiTe-based films with and without nitrogen doping indicating the band gap and VBM, respectively. (d) The energy band structures of the SiTe-based OTS with and without nitrogen doping. (e) Schematic energy band diagrams of the W/OTS/W device at the equilibrium, off state and on state. | |
Based on the above measurement and calculation results, the energy band structures of the SiTe-based OTS with and without nitrogen doping are shown in Fig. 2d. Both devices show symmetrical ohmic contact at the metal–semiconductor interface as the work function of tungsten is 4.55 eV and the SiTe-based OTS exhibits n-type conductance. Therefore, it is necessary to consider only the effect of nitrogen doping on the intrinsic trap of the OTS material. For the SiTe-based OTS with a narrow bandgap, structural distortion caused by Si–Si bonds is considered to be a dominant source of shallow traps, which creates adjacent band tail localized states close to the extended states in the conduction band.34 Some of the Si–Si bonds are replaced by stronger Si–N bonds in the case of nitrogen doping, so the band tail states are decreased. At the same time, Si–N bonds can reduce deep traps by saturating some dangling bonds of the silicon atoms caused by the disorder.35 The energy barrier (EC − EF) is increased by 0.1 eV due to the reduction of both shallow and deep traps in N-doped SiTe. It is more difficult for electrons in the deep traps to be excited into the extended states of the conduction band. The leakage current accordingly decreases more than one order of magnitude by nitrogen doping. Fig. 2e illustrates the threshold switching (TS) process of the SiTe-based devices, which conforms to the trap-limited transport model based on PF emission.30 The device is in equilibrium at zero bias. Once the voltage is applied, it turns to a non-equilibrium state. When the bias is below Vth, most electrons hop only among the deep traps. As the voltage increases, the energy band will bend due to unbalanced carriers and an inhomogeneous electric field, resulting in electrons in deep traps tunneling to shallow traps. When the bias exceeds Vth, shallow traps are filled, and the device is turned on.
2.3. Analysis of the subthreshold region of the OTS device
According to the PF model, temperature-dependent subthreshold currents can assist in the analysis of the relationship between the threshold voltage and temperature. We adopted the subthreshold I–V data of the N-doped SiTe device shown in Fig. 1b, as shown in the inset of Fig. 3a. The current increases as the temperature increases from 25 °C to 100 °C, which originates from the increased emission probability of hot carriers at higher temperatures.36,37 The derived formula for conduction activation energy (EA) is given by30 | 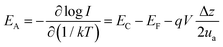 | (1) |
where I is the current, k is the Boltzmann constant, T is the absolute temperature (K), q is the electron charge, V is the voltage, Δz is the inter-trap distance and ua is the thickness of the OTS film. By calculating the middle term in eqn (1), we obtain the EA values at different voltages. Then, by extrapolating the linear relationship, EA is 0.408 eV at zero field, which is close to the energy barrier measured by spectral analysis (0.38 eV).
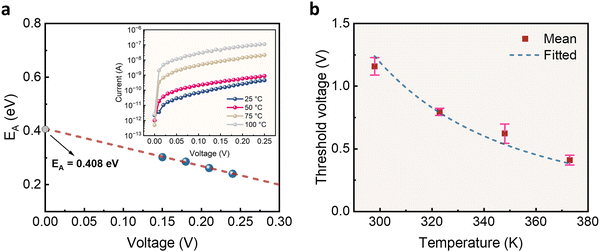 |
| Fig. 3 Analysis of the subthreshold region of the N-doped SiTe OTS device. (a) Plot of the conduction activation energy versus applied voltage. The inset shows the subthreshold I–V characteristics of the N-doped SiTe device. (b) Temperature dependence of the threshold voltage under experimental and fitting conditions. | |
Based on the obtained EA, we approximated the correlation between the threshold voltage and temperature of the N-doped SiTe device in the hot-carrier trap-limited transport model by the following formula:37
|  | (2) |
where
V0 is a material-dependent pre-exponential factor determined by the transfer time (
τ). For the same device, five DC sweeps were performed at each temperature to analyze the variation in the threshold voltage. In
Fig. 3b, it can be observed that the threshold voltage extracted from the experiment shows a diminishing trend consistent with this fitting formula, which confirms the temperature response characteristics of the semiconductor chalcogenide materials in the subthreshold region.
2.4. OTS-based artificial thermal afferent neurons
The perception and cognition of external thermal information is one of the basic abilities of the human sensory nervous system, which follows a unique strategy to perceive and process the information almost simultaneously with femtojoule-level ultra-low power consumption.7 Taking advantage of the low leakage current and inherent temperature response, the N-doped SiTe OTS device, compared with MIT devices, has greater potential for achieving thermal sensing and computing integration with lower power consumption. Fig. 4a elaborates on the perception, encoding and processing of thermal stimuli from the outside, which are implemented by a combination of thermal receptors, afferent neurons and the cerebral cortex. In this system, afferent neurons are very critical to achieving the function of collecting information from receptors and then converting them into electrical spikes.
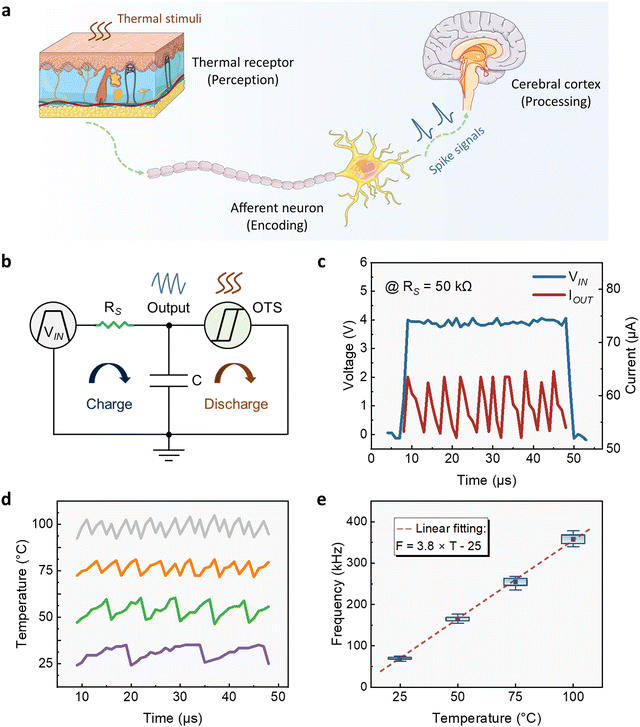 |
| Fig. 4 Construction of artificial thermal afferent neurons. (a) Schematic of the human sensory nervous system. Thermal stimuli from external objects are converted into electrical spikes by receptors and afferent neurons and then transported to the cortex for further processing. (b) Schematic of the artificial thermal afferent neuron circuit. (c) Oscillation behavior of afferent neurons under a constant voltage input. (d) Robust spiking frequency dynamic response of afferent neurons at different temperatures. (e) Dependence of spiking frequency on temperature under the experimental and fitting conditions. | |
In order to construct an energy-efficient artificial sensory nervous system, first, a compact thermal afferent neuron with low power consumption based on N-doped SiTe OTS is demonstrated. As schematically illustrated in Fig. 4b, the afferent neuron comprises a fixed resistor (RS), a capacitor (C) and an OTS device, which has an identical circuit model as a leaky integrate-and-fire (LIF) neuron. The RS used here is 50 kΩ to provide a suitable partial voltage. When a voltage (VIN) is applied, the capacitor charges through the charging loop as the resistance of the OTS is larger than that of the RS. The OTS device switches from a high resistance state (HRS) to a low resistance state (LRS) once the voltage surpasses Vth, and then the capacitor discharges through the discharging loop. When the voltage on the capacitor drops below Vh, the OTS device spontaneously returns to its HRS, and the capacitor charges again. Under a constant voltage input, a steady electrical oscillation behavior of the afferent neuron accompanied by continuous switching between the HRS and LRS of the OTS device can be produced, as shown in Fig. 4c. For the sake of simplicity, the current flowing through the OTS device is measured as the output response.
Frequency encoding of information is widely used in the human sensory nervous system and SNN. Fig. 4d shows that the oscillation frequency of afferent neurons can be modulated by temperature. The corresponding decrease in the threshold voltage with increasing temperature results in faster oscillation behavior. It is also worth noting that our thermal afferent neuron is capable of spiking frequency encoding over a wide temperature range from 25 °C to 100 °C without additional temperature sensors. As a result, artificial afferent neurons based on N-doped SiTe OTS can emulate neuromorphic temperature perception by converting thermal signals into frequency-encoded spikes. Therefore, the sensory nervous system built from OTS-based artificial afferent neurons is able to perceive and process external thermal information simultaneously. The relationship between the spiking frequency and temperature is summarized in Fig. 4e. Here, the frequency was measured five times at each temperature for the same device, and the results showed that the spiking frequencies were tightly distributed for the corresponding temperature. Through fitting analysis, there is a quasi-linear relationship between the spiking frequency and temperature. The frequency-encoded artificial afferent neuron also features a bio-frequency adaptation of hundreds of kilohertz, which provides potential for bio-electronic interface applications.
To verify the feasibility of constructing an energy-efficient artificial sensory nervous system with our artificial afferent neurons, we further calculate the energy consumption of the artificial afferent neurons (Fig. S5, ESI†). The transient power is calculated by multiplying the input voltage by the output current, and the energy consumption for each spike is determined by dividing the total energy consumption by the number of spikes within a period of time. An ultra-low power consumption of less than 0.3 nJ per spike for this artificial afferent neuron over the perceived temperature range is acquired (Fig. S6, ESI†). The low power consumption originates from the extremely low leakage current and the relatively low threshold voltage of N-doped SiTe OTS. Table S1 (ESI†) benchmarks our OTS-based artificial afferent neurons with other state-of-the-art afferent neurons. Compared with existing works in the literature, the artificial afferent neuron we propose effectively reduces power consumption by an order of magnitude by utilizing an N-doped SiTe OTS device with an extremely low leakage current.
2.5. Edge detection based on artificial thermal afferent neurons
In order to mimic the function of perception and cognition in the biological sensory nervous system, which enables us to recognize the most significant information from complex thermal stimuli, an edge detection task of thermal perception and processing was built based on N-doped SiTe OTS artificial thermal afferent neurons, utilizing its capability of temperature sensing with low power consumption. The output spikes from artificial afferent neurons can be subjected to a pulse coupled neural network (PCNN) for edge detection, even with complex image backgrounds. The PCNN, as a neural network model based on experimental observations in the animal visual cortex, is constructed by a single layer two-dimensional simplified neural network.38,39 Compared with traditional neural network models, PCNN is capable of performing edge detection tasks efficiently without training.40,41
Edge detection of the thermography of the PCNN was demonstrated by simulation. Input thermography can be detected and encoded into spikes by an array of artificial afferent neurons pixel-by-pixel and, in turn, the output spikes are fed into the PCNN, as shown in Fig. 5a. The thermography was set within a temperature range of 20–45 °C according to the actual situation. As aforementioned, an increase in temperature results in an increase in the frequency of the spikes (51–146 kHz). Fig. 5b provides a brief description of the edge detection process via the PCNN. In order to detect more accurately, three frequency values (58, 98.5 and 139 kHz) in the encoded image were set as thresholds to search for edge information at different levels.16 Each pixel was traversed iteratively. The pulse generator of the PCNN would fire when the frequency value of the pixel is greater than the threshold or close to that of neighboring pixels. After several iterations, the image edges generated by the PCNN at each threshold level were recorded. The edge details of the background, person and kettle were determined by iterations of the three thresholds. Finally, as shown in Fig. 5c, we obtained the complete edge detection results by combining the edge information under the three thresholds. Here, PCNN-based edge detection is taken as an example to demonstrate the great potential of our N-doped SiTe OTS artificial thermal afferent neurons for realizing a high-power and area-efficient thermal imaging and identification system.
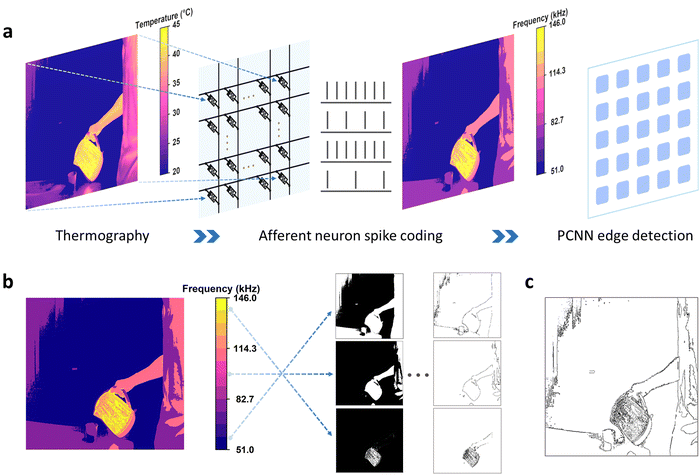 |
| Fig. 5 Edge detection based on artificial thermal afferent neurons using the PCNN. (a) Schematic of the thermography edge detection process in a PCNN with artificial thermal afferent neurons. (b) Schematic of mapping and iteration in the edge detection process. (c) The results of edge detection using the PCNN. | |
3. Conclusions
In summary, an artificial thermal afferent neuron based on the N-doped SiTe OTS has been experimentally implemented for perceiving and processing environmental information by converting thermal signals into frequency-encoded spikes. We remarkably decreased the leakage current of the SiTe OTS by more than one order of magnitude from 86.8 nA to 5.5 nA by nitrogen doping. Meanwhile, the inherent temperature response of our OTS device assists the artificial thermal afferent neurons in performing in situ sensing and computing, leading to a simplified circuit structure. As a result, we have successfully demonstrated the realization of a highly energy-efficient and compact artificial thermal afferent neuron, characterized by an impressively low power consumption less than 0.3 nJ per spike and the absence of a thermal sensor component. Further, our simulation results show that a combination of our N-doped SiTe OTS artificial thermal afferent neurons with a PCNN can accomplish edge detection. From the viewpoint of the core material design, this study paves the way for the implementation of in-sensor computing in a highly energy and area-efficient neuromorphic perception system.
4. Experimental
Film synthesis and characterization
N-doped Si2Te3 thin films were deposited via co-sputtering of pure Si and Te targets with a mixed argon and nitrogen pressure of 0.5 Pa and a 40
:
5 argon–nitrogen ratio. Si2Te3 thin films were prepared as control samples under the same conditions without nitrogen being introduced into the sputtering chamber. Ultraviolet-visible-near infrared (UV-vis-NIR) absorption spectra were measured to evaluate the optical band gap of the thin films using a Lambda 1050+ UV-vis-NIR spectrophotometer (PerkinElmer, USA). X-ray photoelectron spectroscopy (XPS) and ultraviolet photoelectron spectroscopy (UPS) analyses of the thin films were performed using a 50 AXIS SUPRA+ instrument (Kratos, Japan) to measure the composition of the films and estimate the Fermi level and valence band maximum (VBM), respectively. Argon ion etching was performed to avoid contamination before photoelectron spectroscopy measurements.
Device fabrication, characterization and electrical measurements
OTS devices with via-hole structures were fabricated on silicon wafers. A ∼100 nm tungsten bottom electrode (BE) layer and a ∼100 nm SiO2 insulating layer were deposited first. Electron beam lithography (EBL) was used to pattern via-holes in the SiO2 insulating layer after deposition. The top electrode region was then defined using ultraviolet lithography. Finally, a ∼50 nm OTS layer and ∼100 nm tungsten top electrode (TE) layer were deposited by magnetron sputtering, followed by a lift-off process. Scanning electron microscopy (SEM) images of the via-hole array were obtained using a ZEISS Gemini300. Transmission electron microscopy (TEM) characterization of the OTS devices was performed on an FEI Titan G2 60-300. All the electrical measurements were performed using a B1500A semiconductor parameter analyzer, an Agilent B1530A waveform generator/fast measurement unit (WGFMU) and an Agilent DSO7104B oscilloscope.
Author contributions
Kai Li: conceptualization, investigation, data curation, formal analysis, methodology, writing – original draft, and writing – review & editing. Jiaping Yao: software, data curation, and visualization. Peng Zhao: investigation and formal analysis. Yunhao Luo: methodology. Xiang Ge: validation. Rui Yang: supervision, project administration, and resources. Xiaomin Cheng: supervision, project administration, resources, funding acquisition, and writing – review & editing. Xiangshui Miao: supervision, project administration, resources, funding acquisition, and writing – review & editing.
Conflicts of interest
There are no conflicts to declare.
Acknowledgements
This work was supported by the National Key Research and Development Program of China (No. 2023YFB4502200), Major Program (JD) of Hubei Province (2023BAA008-3), Hubei Key Laboratory of Advanced Memories, and Hubei Engineering Research Center on Microelectronics.
References
- D. Silver, A. Huang, C. J. Maddison, A. Guez, L. Sifre, G. Van Den Driessche, J. Schrittwieser, I. Antonoglou, V. Panneershelvam, M. Lanctot, S. Dieleman, D. Grewe, J. Nham, N. Kalchbrenner, I. Sutskever, T. Lillicrap, M. Leach, K. Kavukcuoglu, T. Graepel and D. Hassabis, Nature, 2016, 529, 484–489 CrossRef CAS PubMed
.
- M. Satyanarayanan, Computer, 2017, 50, 30–39 Search PubMed
.
- P. A. Merolla, J. V. Arthur, R. Alvarez-Icaza, A. S. Cassidy, J. Sawada, F. Akopyan, B. L. Jackson, N. Imam, C. Guo, Y. Nakamura, B. Brezzo, I. Vo, S. K. Esser, R. Appuswamy, B. Taba, A. Amir, M. D. Flickner, W. P. Risk, R. Manohar and D. S. Modha, Science, 2014, 345, 668–673 CrossRef CAS PubMed
.
- M. Davies, N. Srinivasa, T.-H. Lin, G. Chinya, Y. Cao, S. H. Choday, G. Dimou, P. Joshi, N. Imam, S. Jain, Y. Liao, C.-K. Lin, A. Lines, R. Liu, D. Mathaikutty, S. McCoy, A. Paul, J. Tse, G. Venkataramanan, Y.-H. Weng, A. Wild, Y. Yang and H. Wang, IEEE Micro., 2018, 38, 82–99 Search PubMed
.
- C. Wan, P. Cai, M. Wang, Y. Qian, W. Huang and X. Chen, Adv. Mater., 2020, 32, 1902434 CrossRef CAS PubMed
.
- G. W. Burr, R. M. Shelby, A. Sebastian, S. Kim, S. Kim, S. Sidler, K. Virwani, M. Ishii, P. Narayanan, A. Fumarola, L. L. Sanches, I. Boybat, M. Le Gallo, K. Moon, J. Woo, H. Hwang and Y. Leblebici, Adv. Phys.: X, 2017, 2, 89–124 Search PubMed
.
- K. Boahen, Comput. Sci. Eng., 2017, 19, 14–28 Search PubMed
.
- G. Indiveri, B. Linares-Barranco, T. J. Hamilton, A. V. Schaik, R. Etienne-Cummings, T. Delbruck, S.-C. Liu, P. Dudek, P. Häfliger, S. Renaud, J. Schemmel, G. Cauwenberghs, J. Arthur, K. Hynna, F. Folowosele, S. Saighi, T. Serrano-Gotarredona, J. Wijekoon, Y. Wang and K. Boahen, Front. Neurosci., 2011, 5 DOI:10.3389/fnins.2011.00073
.
- H. Kim, S. Hwang, J. Park, S. Yun, J.-H. Lee and B.-G. Park, IEEE Electron Device Lett., 2018, 39, 630–633 CAS
.
- F. Wang, F. Hu, M. Dai, S. Zhu, F. Sun, R. Duan, C. Wang, J. Han, W. Deng, W. Chen, M. Ye, S. Han, B. Qiang, Y. Jin, Y. Chua, N. Chi, S. Yu, D. Nam, S. H. Chae, Z. Liu and Q. J. Wang, Nat. Commun., 2023, 14, 1938 CrossRef CAS PubMed
.
- J. H. Yoon, Z. Wang, K. M. Kim, H. Wu, V. Ravichandran, Q. Xia, C. S. Hwang and J. J. Yang, Nat. Commun., 2018, 9, 417 CrossRef PubMed
.
- C. Chen, Y. He, H. Mao, L. Zhu, X. Wang, Y. Zhu, Y. Zhu, Y. Shi, C. Wan and Q. Wan, Adv. Mater., 2022, 34, 2201895 CrossRef CAS PubMed
.
- K.-Y. Chun, Y. J. Son, E.-S. Jeon, S. Lee and C.-S. Han, Adv. Mater., 2018, 30, 1706299 CrossRef PubMed
.
- J.-K. Han, S.-C. Park, J.-M. Yu, J.-H. Ahn and Y.-K. Choi, Nano Lett., 2022, 22, 5244–5251 CrossRef CAS PubMed
.
- L. Yang, Z. Wang, S. Zhang, Y. Li, C. Jiang, L. Sun and W. Xu, Nano Lett., 2023, 23, 8–16 CrossRef CAS PubMed
.
- K. Shi, S. Heng, X. Wang, S. Liu, H. Cui, C. Chen, Y. Zhu, W. Xu, C. Wan and Q. Wan, IEEE Electron Device Lett., 2022, 43, 2196–2199 CAS
.
- S. L. Fang, C. Y. Han, Z. R. Han, B. Ma, Y. L. Cui, W. Liu, S. Q. Fan, X. Li, X. L. Wang, G. H. Zhang, X. D. Huang and L. Geng, IEEE Trans. Electron Devices, 2022, 69, 2346–2352 Search PubMed
.
- J. Shamsi, K. Mohammadi and S. B. Shokouhi, IEEE Trans. VLSI Syst., 2018, 26, 2795–2805 Search PubMed
.
- C. Bartolozzi and G. Indiveri, Neural Comput., 2007, 19, 2581–2603 CrossRef PubMed
.
- X. Zhang, Y. Zhuo, Q. Luo, Z. Wu, R. Midya, Z. Wang, W. Song, R. Wang, N. K. Upadhyay, Y. Fang, F. Kiani, M. Rao, Y. Yang, Q. Xia, Q. Liu, M. Liu and J. J. Yang, Nat. Commun., 2020, 11, 51 CrossRef CAS PubMed
.
- Q. Wu, B. Dang, C. Lu, G. Xu, G. Yang, J. Wang, X. Chuai, N. Lu, D. Geng, H. Wang and L. Li, Nano Lett., 2020, 20, 8015–8023 CrossRef CAS PubMed
.
- F. Li, R. Wang, C. Song, M. Zhao, H. Ren, S. Wang, K. Liang, D. Li, X. Ma, B. Zhu, H. Wang and Y. Hao, ACS Nano, 2021, 15, 16422–16431 CrossRef CAS PubMed
.
- R. Yuan, Q. Duan, P. J. Tiw, G. Li, Z. Xiao, Z. Jing, K. Yang, C. Liu, C. Ge, R. Huang and Y. Yang, Nat. Commun., 2022, 13, 3973 CrossRef CAS PubMed
.
- D. Lee, M. Kwak, K. Moon, W. Choi, J. Park, J. Yoo, J. Song, S. Lim, C. Sung, W. Banerjee and H. Hwang, Adv. Electron. Mater., 2019, 5, 1800866 CrossRef
.
- R. R. Shanks, J. Non-Cryst. Solids, 1970, 2, 504–514 CrossRef
.
- H. Fritzsche and S. R. Ovshinsky, J. Non-Cryst. Solids, 1970, 2, 393–405 CrossRef CAS
.
- J. Yoo, S. H. Kim, S. A. Chekol, J. Park, C. Sung, J. Song, D. Lee and H. Hwang, Adv. Electron. Mater., 2019, 5, 1900196 CrossRef
.
- T. Gao, J. Feng, H. Ma, X. Zhu and Z. Ma, Appl. Phys. Lett., 2019, 114, 163505 CrossRef
.
- Y. Koo and H. Hwang, Sci. Rep., 2018, 8, 11822 CrossRef PubMed
.
- D. Ielmini and Y. Zhang, J. Appl. Phys., 2007, 102, 054517 CrossRef
.
- M. Zhu, W. Song, P. M. Konze, T. Li, B. Gault, X. Chen, J. Shen, S. Lv, Z. Song, M. Wuttig and R. Dronskowski, Nat. Commun., 2019, 10, 3525 CrossRef PubMed
.
- C.-Y. Lin, R. K. Ulaganathan, R. Sankar, R. C. Murugesan, A. Subramanian, A. Rozhin and S. Firdoz, J. Mater. Chem. C, 2021, 9, 10478–10486 RSC
.
- G. M. Ingo and N. Zacchetti, High Temp. Sci., 1988, 28, 137–151 CAS
.
- E. A. Davis and N. F. Mott, Philos. Mag., 1970, 22, 0903–0922 CrossRef CAS
.
- W. E. Spear and P. G. Le Comber, J. Non-Cryst. Solids, 1972, 8–10, 727–738 CrossRef CAS
.
- P. Fantini, N. Polino, A. Ghetti and D. Ielmini, Adv. Elect. Mater., 2023, 2300037 CrossRef CAS
.
- E. Piccinini, A. Cappelli, F. Buscemi, R. Brunetti, D. Ielmini, M. Rudan and C. Jacoboni, J. Appl. Phys., 2012, 112, 083722 CrossRef
.
- Z. Wang, Y. Ma, F. Cheng and L. Yang, Image Vision Comput., 2010, 28, 5–13 CrossRef
.
- P. Ganasala and V. Kumar, J. Digit Imaging, 2016, 29, 73–85 CrossRef PubMed
.
- J. L. Johnson and M. L. Padgett, IEEE Trans. Neural Network Learn. Syst., 1999, 10, 480–498 CrossRef CAS PubMed
.
- X. Gu, Neural Process Lett., 2008, 27, 25–41 CrossRef
.
|
This journal is © The Royal Society of Chemistry 2024 |