DOI:
10.1039/D4FO00721B
(Review Article)
Food Funct., 2024, Advance Article
Biomarkers of food intake and their relevance to metabolic syndrome
Received
13th February 2024
, Accepted 29th May 2024
First published on 7th June 2024
Abstract
Metabolic syndrome (MetS) constitutes a prevalent risk factor associated with non communicable diseases such as cardiovascular disease and type 2 diabetes. A major factor impacting the etiology of MetS is diet. Dietary patterns and several individual food constituents have been related to the risk of developing MetS or have been proposed as adjuvant treatment. However, traditional methods of dietary assessment such as 24 h recalls rely greatly on intensive user-interaction and are subject to bias. Hence, more objective methods are required for unbiased dietary assessment and efficient prevention. While it is accepted that some dietary-derived constituents in blood plasma are indicators for certain dietary patterns, these may be too unstable (such as vitamin C as a marker for fruits/vegetables) or too broad (e.g. polyphenols for plant-based diets) or reflect too short-term intake only to allow for strong associations with prolonged intake of individual food groups. In the present manuscript, commonly employed biomarkers of intake including those related to specific food items (e.g. genistein for soybean or astaxanthin and EPA for fish intake) and novel emerging ones (e.g. stable isotopes for meat intake or microRNA for plant foods) are emphasized and their suitability as biomarker for food intake discussed. Promising alternatives to plasma measures (e.g. ethyl glucuronide in hair for ethanol intake) are also emphasized. As many biomarkers (i.e. secondary plant metabolites) are not limited to dietary assessment but are also capable of regulating e.g. anti-inflammatory and antioxidant pathways, special attention will be given to biomarkers presenting a double function to assess both dietary patterns and MetS risk.
1 Introduction
Metabolic syndrome (MetS) is among the most common health conditions related to non-communicable diseases (NCDs) and has become increasingly predominant in Western societies, as well as in developing countries, over the last century.1 Typically, it is diagnosed when 3 out of 5 health-related components have manifested, i.e., elevated blood sugar, high triglycerides, low high-density lipoprotein cholesterol, large waist-circumference, and high blood pressure.2 Currently, it is estimated that approximately 25% of the world population has MetS, making it a prime healthcare issue that affects more people than diabetes.1 Among the risks associated with MetS, there is an increased chance of developing cardiometabolic diseases such as type 2 diabetes mellitus (T2DM) (3- to 7-fold elevated risk) and cardiovascular diseases (CVD) (2-fold increased risk), among other.2 Due to its strong relation to other comorbidities and the burden these may place on public healthcare systems, interest in the etiology and prevention of MetS has increased over the past decades. For example, the costs of medical treatment for MetS patients in the U.S. were estimated to be 1.6 times higher than those for non-MetS patients in 2009, with an average increase of 24% in the annual healthcare costs for each MetS component present.3 Therefore, monitoring risk factors that could affect the etiology of MetS is paramount.
An important aspect of MetS prevention are lifestyle factors, especially dietary patterns. Indeed, the association between dietary patterns and cardiometabolic disease including MetS is widely known and has been explored thoroughly.4–7 For example, fruit and vegetable consumption has been associated with reduced risk of CVD, reduced blood pressure, and overall mortality.8,9 These health effects are possibly linked to the intake of dietary fiber and secondary plant metabolites and their related anti-inflammatory aspects,4 or their impact on the gut microbiota.10,11 Secondary plant metabolites include metabolites such as polyphenols and carotenoids, which could further impact cellular signaling cascades by interacting with transcription factors and nuclear receptors.11 For similar reasons, the intake of whole grain cereals has been related to reduced risk of MetS.12 Contrarily, refined grains have been positively associated with MetS, as these can be a rich source of simple refined carbohydrates.12 Also positively associated with the risk of MetS has been meat consumption, especially processed meats,13 possibly due to their association with higher levels of processing, higher salt intake, and possibly less healthy fatty acid composition.13,14 The intake of food items with healthy fatty acid composition, such as omega-3 fatty acids via fish has however been related to reduced risk of developing MetS.15
Therefore, improving dietary habits promises to be one of the most effective tools to protect against MetS. To properly evaluate the role that dietary patterns have on health and their protection against certain chronic diseases, it is of the utmost importance that food intake can be properly measured.16 However, serious limitations exist in how dietary intake is assessed in both clinical practice and research.17 Traditionally, food intake has been assessed through either real-time recording of intake (e.g., food diaries) or self-reported methods that include food-frequency questionnaires (FFQ) and 24-hour dietary recalls, with the last two being the most popular methods in the literature for assessing dietary intake.17 If 24 h recalls are employed, which is the recommended way to assess food consumption patterns of adults by some organizations such as European Food Safety Authority (EFSA),18 at least two 24 h recalls should be obtained, preferably one from a weekday and one from the weekend.
However, regardless of their popularity, self-reporting methods have inherent errors, such as recall bias or over/under reporting.16,19,20 Using a trained nurse can help minimize said limitation, but this is a costly and lengthy process.21,22 Moreover, the assessment of these documents is often time-consuming and may delay the decision-making of healthcare professionals. In addition, such data captures only food items and has to be translated into nutrient intakes by means of combining the intake data with relevant food composition databases, such as the one published by the United States Department of Agriculture (USDA),23 introducing another potential source of error. Other limitations arise for these methods. For example, 24 h dietary recalls are prone to overlook non-frequently consumed food items, which may be of special interest when considering intake estimation of certain nutrients such as vitamin A from offals.21
Thus, there is a great interest in developing appropriate objective biomarkers of food intake (BFI) that accurately reflect the consumption of specific food groups or dietary constituents. Ideally, appropriate markers can be measured within biological samples such as blood or urine and reflect intake over at least mid-term periods. This requires the assumption that dietary intake translates directly and proportionally into measurable differences in concentrations of said markers. However, such biomarkers should ideally fulfill other criteria that would allow for reproducibility and practicability in real life scenarios.24
The purpose of the present review is to summarize the state-of-the-art of biological endpoints that can act as markers for mid-long term intake of either food constituents or groups that are related to the risk of MetS or its severity. Furthermore, the article will emphasize as to how these compounds may not only constitute suitable markers to assess dietary intake, but in some instances possess bioactive effects themselves, influencing mechanistic pathways involved in the etiology of MetS such as those related to reducing oxidative stress25 and inflammation.26
2 Biomarker definition and need
One way to overcome the limitation questionnaires pose in the assessment of dietary patterns and nutrient intake is to use biomarkers of food intake (BFI) as an alternative or complimentary tool for dietary assessment.27 Many definitions of biomarkers have been proposed through the years. For the purpose of this review, a biomarker is defined as a measurable characteristic in a biological system that indicates normal or pathological processes or exposure to environmental elements, here, nutrients and other dietary constituents.28
The definition of a biomarker poses an extra challenge to address the question of what constitutes a promising biomarker. Dragsted et al. have defined the following eight criteria for validating biomarkers of food intake: plausibility, dose–response, time–response, robustness, reliability, stability, analytical performance and inter-laboratory reproducibility,20 and other reviews in this domain have employed the same criteria.29 Similarly and in line with this proposition, we have outlined a set of requisites in Table 1, with the addition of practicability and the omission of robustness that refers to stability across different study designs and populations as this may not be always required when focusing on a very specific population.30,31 In the following, we will briefly describe said conditions.
Table 1 List of main criteria used to define characteristics of a promising biomarker of food intake (BFI)
Criteria of suitable BFI |
Explanations |
Adapted from ref. 20. Terms employed by Dragested et al.20 |
Selectivity/specificity (plausibilitya) |
Should be specific for the food group or ingredient in question, i.e. food item should be a major source |
Sensitivity (dose responsea) |
Should allow to differentiate between reasonably small differential intake and show a dose–response relationship |
Time responsea |
|
Short term marker |
Should indicate intake of the element over a short period of time (e.g. a single meal) |
Mid/long term marker |
Should indicate the average intake of a longer period (e.g. repeated intakes) |
Validity (reliabilitya) |
Should reliably capture food component of interest and has been tested to do so through FFQs or equivalent methods |
Stabilitya |
Should not degrade easily during collection, storage, and analysis |
Reproducibilitya |
Should have a low coefficient of variability upon repeated measures, it should be reproducible in different laboratories and between batch analyses |
Easy to measure/practicality |
Should not require highly trained personnel and does has a reasonable cost for the analyses, |
Analytical performancea |
Sufficient precision, accuracy and detection limits |
Specificity (or selectivity) is among the most important criteria. When discussing markers of dietary intake it refers to the fact that the marker should be specifically associated with the intake of either a dietary constituent or a specific group of food items. Thus, the presence and changes of a given biomarker can be clearly correlated with changes in the studied condition. For example, while vitamin C in plasma may be related to fruit intake, it is also found in many vegetables and is sensitive to food processing and storage conditions,32 and is thus not a very specific marker for fruit intake. Some nutrients are even originating from other sources besides dietary intake such as cultural habits and lifestyle factors in the case of vitamin D, formed under the skin.33 In addition, supplementation, food fortification and food additives may constitute further sources of nutrients.
Sensitivity is another important criteria, it implies that the biomarker levels should correlate well and in a predictable manner with intake, allowing the detection of small changes within typical dietary intake ranges.34 For example, plasma vitamin B12 could act as a biomarker of meat and meat products intake due to its specificity (it is exclusively present in meat and fish and its products).35 Nevertheless, it has limited sensitivity, as the plasma concentration of vitamin B12 is homeostatically regulated and kept constant for several months due to liver reserves.36 The same is true for many minerals that are homeostatically regulated such as Mg or Ca, which do generally (i.e. their blood concentration) not correlate well with dietary intake,37,38 and less so with individual food groups. In addition to homeostatic regulation, other individual factors such as the variability caused by age, sex, ethnicity, medication, smoking status, and alcohol consumption among other does influence nutrient metabolism and can affect the levels of biomarkers.16,39 Furthermore, genetic variability and gut microbiota are considered important host factors that can impact ADME (absorption, distribution, metabolism, and excretion) aspects within a population and should thus be considered as additional factors explaining inter-individual variability of circulating nutrients and non-nutrients. For instance, some persons have a gut microbiota suitable to produce equol from the soy isoflavone daidzein, while others do not.40 In addition, many SNPs are involved in the uptake or transport of nutrients from the gut. For example, a limited set of SNPs has been shown to explain a majority of inter-individual variability of carotenoid absorption.41,42 For more in-depth information, the reader is referred to more comprehensive reviews.43,44 However, such individual factors cannot be generally considered in more population-oriented research, and the marker may thus only work reasonably well for a certain – often general – population, similar as dietary intake recommendations.
Other challenges associated with biomarker research are the need for affordable markers that can easily be used in the clinical context, i.e. practicality. For instance, markers should be measurable by non-invasive or minimally invasive methods that can be incorporated into clinical practice. This may at present exclude expensive or time demanding analysis and extremely well trained personnel, such as required for various -omics measures.
Regarding stability, biomarkers should not be easily degraded after collection and during storage, and the results should be reproducible. Low stability of a biomarker obstructs its use, as complex protocols have to be employed for its preservation, which also decreases the affordability and reproducibility.45 It is generally recommended that samples are stored at either −80 °C or −20 °C or under protective atmosphere such as argon to improve the stability of the markers. This way, minimal changes in biomarker concentrations will occur;46 though even so, stability at −80 °C may be limited for several months. For instance, vitamin C may become difficult to measure after one year, even if kept at −80 °C and using stabilizers.45
Reproducibility can be compromised by many factors, such as variable extraction efficacy from the matrix or variable responses at detection, such as cross-reactivity when employing ELISA methods.47 Time-response is also an important criteria, as the half-life of many dietary constituent-derived markers in the bloodstream can vary broadly, depending on interactions with other tissues/organs. Said organs may act as targets or storage reservoirs lasting from a few hours to several days, and may therefore reflect either intake from a few individual food items or truly reflecting mid-long term dietary intake, with the latter being the preferred characteristic.
The growing accessibility of mass spectrophotometry (MS) and other techniques such as nuclear magnetic resonance (NMR), high throughput sequencing, and available gene array chips has led to the use of omics to identify biomarkers of food intake, e.g. within the Food Biomarkers Alliance (FOODBALL) consortia, which systematically explored and validated such markers in a European context and made a considerable effort towards harmonizing methodologies and validating markers. Unfortunately, after the end of the project in 2017, its webpage is no longer available.48 While FOODBALL used metabolomics to assess dietary intake, the MIRDIET project complemented the research by focusing on circulating microRNAs (miRNAs) and their link to dietary intake. In this project, two plasma miRNAs were linked to polyphenol intake (miR-22-3 and miR-378a-3p).48
Unfortunately, and although the use and development of BFI promises to revolutionize dietary data collection, few markers have been validated thus far, e.g., genistein for soy intake49 or tartaric acid for grapes,50 while the number of proposed markers continues to increase exponentially.19,39 Thus, it is of vital importance to review existing BFI for different food groups or even individual food items that are related to health outcomes and further validate the most promising ones so they can further be applied in research in clinical contexts. It is important to note that many of the molecules especially some BFI most recently added have typically only marginally been studied, and not all the criteria of BFI can be addressed. It also means that the role of host factors such as genetics/polymorphisms that may act upon their metabolism have not been studied. Of note, in the following, the focus rests on physiologically plausible intake, while high intakes that may be related to even adverse effects, including that from supplemental intake is rather not considered, also in sight of the focus on existing markers for food group intake and consumption by the background diet, rather than focusing on individual nutrients.
3 MetS, inflammation and oxidative stress
MetS has a complex etiology that includes, among the traditional components (in accordance with e.g. ATP III2) other components, such as oxidative stress,51 systemic inflammation,52 or gut microbiota dysbiosis.53 These aspects are of special importance as they can exert systemic effects that interact with traditional MetS components such as blood pressure or insulin resistance.54 Moreover, oxidative stress and systemic inflammation, together with traditional elements, especially visceral obesity, can activate positive feedback loops that trigger pro-inflammatory responses in various tissues, thereby e.g. aggravating vessel health and blood pressure.51 The main cause for low-grade chronic systemic inflammation in MetS seems to originate from increased visceral adiposity, a traditional component of MetS.55
In this regard, diet can play a twofold beneficial effect on MetS; by directly reducing the amount of visceral fat, and thus inflammation and blood pressure, and by providing antioxidants and anti-inflammatory molecules that may reduce oxidative stress and inflammation.51 The latter can be reduced directly such as via quenching reactive oxygen species (ROS)56 or via acting on cellular signaling cascades and nuclear receptors such as nuclear factor kappa B (NF-κB),56 peroxisome proliferator-activated receptor (PPAR),56 and nuclear factor-erythroid factor 2-related factor 2 (Nrf2),56 all of which are upstream of many further antioxidant and inflammatory agents.
Currently, a combination of oxidative stress and visceral adiposity are thought to initiate the chronic inflammatory response that is characteristic of MetS (Fig. 1).57 First, visceral adiposity leads to a dysregulation of cytokines, reducing the anti-inflammatory (IL-10, adiponectin) and increasing pro-inflammatory ones (leptin, Il-6, TNF-α).57 Low-grade systemic inflammation can aggravate MetS components, such as elevated blood pressure or elevated glucose levels. For instance, systemic inflammation is capable of inducing an increase in blood pressure through interaction with the renin–angiotensin system (RAS), responsible for regulating blood pressure and being capable of immune cell activation. This activation also promotes pro-inflammatory cytokine (Il-6, IFN-γ, TNF-α) production and release. Thus, RAS-mediated inflammation produces a positive feedback loop, further increasing blood pressure (Fig. 1).52 Blood pressure is further linked to inflammation through the cell adhesion molecules (CAM) ICAM-1 and VCAM-1. These molecules are recruited by TNF-α and IL-1β58 and are involved in inflammatory responses in the endothelium that lead to endothelial damage or atherosclerosis, among others. Overall, this decrease in cardiovascular health leads to a subsequent increase in blood pressure due to the vessel diameter reduction.58,59
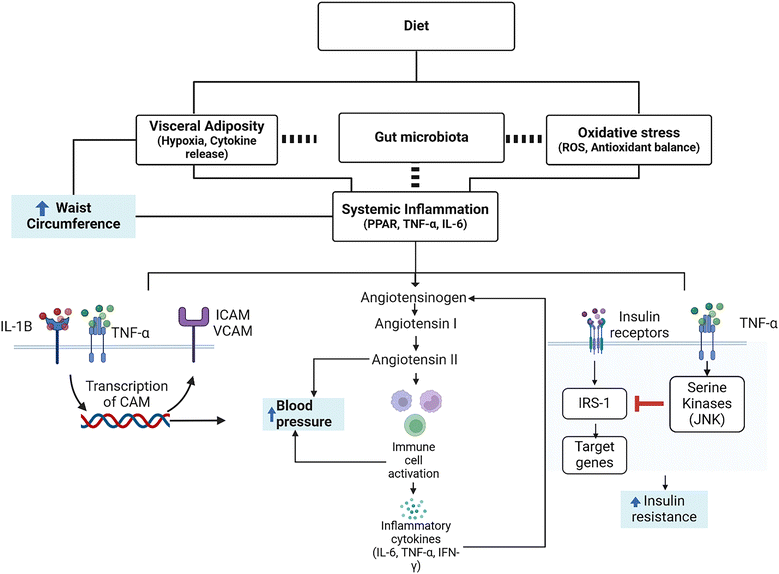 |
| Fig. 1 Effects of diet on metabolic syndrome components (in blue boxes) through systemic inflammation, oxidative stress, and visceral adiposity. The role of microbiota is shown as a dashed line as the links are not fully understood. | |
Additionally, inflammatory cytokines are also involved in the induction of insulin resistance that leads to hyperglycemia.51,52 Briefly, TNF-α and other cytokines promote the activity of serine kinases, mainly JNK, that phosphorylate the insulin receptor substrate 1 (IRS-1), which constitutes a key element of the signaling cascade of insulin in the cell. Phosphorylation of IRS-1 decreases the efficiency of insulin signal transduction and deficient insulin signal transduction decreases the response to insulin, leading to insulin resistance (Fig. 1).51,52 Therefore, the presence of pro-inflammatory cytokines is considered a hallmark of low-grade chronic inflammation that accompanies MetS, and such markers could be monitored to study the success of dietary intervention, in addition to the traditional endpoints (i.e. ATP III criteria). This could work either as early markers in humans or animal models and as fast-changing markers in cellular models that do not allow monitoring hard endpoints. To further understand the complex signaling network of adipocyte dependent inflammation, we refer the reader to additional reviews.56,60,61 In this regard, dietary components can exert a positive action on pathways of oxidative stress or inflammation. For example, polyphenols have been shown to increase Nrf-2 and thus the body's own antioxidant defense mechanisms, upregulating the enzymatic activity of, e.g., superoxide dismutase and catalase.62 For carotenoids, in addition to potentially acting as direct scavengers of ROS, they can also interact with transcription factors including Nf-κB and Nrf-2.11,63 Said interactions decrease circulating ROS or inflammatory cytokines (e.g., TNF-α), and may even influence adipose tissue differentiation via interacting with nuclear receptors.63 In this regard, the use of multiple food bioactive compounds in a combined portfolio may be the most promising for reducing the risk of MetS or even for adjuvant treatment, similar to the strategy proposed by Jenkins et al. in their portfolio dietary approach over two decades ago.64
4 Biomarkers of food intake and their relation to MetS status
Fruits and vegetables
Fruits and vegetables constitute a large array of products of plant origin. Thus, many of them differ substantially by their nutrient profile and phytochemical composition.65 Regardless of this variety, fruits and vegetables share some similarities in their nutritional profile, such as the presence of dietary fiber, certain micronutrients that are not found in animals (i.e., vitamin E, vitamin C, or D2), or the presence of secondary plant metabolites (e.g., polyphenols or carotenoids).65 For these reasons, meta-analyses have generally shown a positive association between the portions of fruits and vegetables consumed and the risk of MetS.6,7
Potential biomarkers of fruit and vegetable intake could thus encompass a large array of different compounds. The most common markers of fruit and vegetable intake proposed in the literature include serum carotenoids, vitamin C, or polyphenol sub-groups such as flavonoids.16 Vitamin C and total carotenoids are among the most widely studied markers for general fruit/vegetable consumption, again due to their wide distribution in the plant kingdom, while other plant bioactive compounds such as specific phenolic subgroups or individual carotenoids may be used to assess intake of specific food items.66
Although many compounds could be considered as biomarkers, the general challenge associated with the wide variability of nutrients and non-nutrients in plant food items persists. Even in the same species variability can be high, for example depending on exogenous conditions (e.g. provenience, harvest, storage, and processing).67,68 In addition, several compounds are not specific for fruits and vegetables but do also occur in other plant-dominated food groups such as cereals and grains, which also typically contain polyphenols, as well as vitamins C and E; though in part to a lesser extent compared to fruits and vegetables, due to the dominance of starch in these food groups.69
To overcome the lack of specificity/selectivity, authors have claimed the need for novel biomarkers of vegetable and fruit intake that have more specificity and correlate better with specific subgroups or even food items than traditional markers such as vitamin C.66,70,71 While many BFI have been proposed with the rise of -omics technologies, very few are validated, and even fewer are used in research studies or clinical practice, however, these will also be briefly discussed.
Vitamin C. Vitamin C or ascorbic acid is an essential nutrient and plant bioactive compound, with daily recommended dietary intakes of ca. 100 mg d−1.72 Vitamin C has a well-regarded antioxidant activity in which it acts synergistically with vitamin E in the prevention of lipid peroxidation.73 Regarding MetS risk, studies have shown a protective effect of vitamin C.74 Vitamin C allows for an increase of nitric oxide (NO) in plasma by preventing its degradation while also actively promoting NO synthesis as demonstrated in cultured human endothelium.75 NO is of particular relevance for MetS as it has vasodilator properties that may ameliorate blood pressure issues.75 This blood pressure lowering effect was shown in a recent meta-analysis including 8 RCT and 614 participants with hypertension that were supplemented vitamin C for 4–24 weeks. Supplementing 3 to 10 times the recommended dietary allowances (RDA) was inversely associated with reduced blood pressure (P < 0.05), with a weighted mean difference of −3.75 and −3.25 mm Hg for systolic and diastolic blood pressure, respectively. Indeed, supplementation with vitamin C has been proposed as a complementary tool to treat primary hypertension.76 Overall, these results are in line with the reduced risk of CVD associated in epidemiological studies with dietary intake of ascorbic acid.75It is usually measured through HPLC and UV detection, although LC-MS can also be employed.77 Plasma levels of ascorbic acid have been shown to correlate reasonably well with the dietary intake of vitamin C (as determined by questionnaires or food records), for instance a correlation coefficient of r = 0.41 (after adjusting for energy intake) was found in a meta-analysis encompassing over 26
000 participants.78 The intermediate correlation coefficient may indicate degradation during gastro-intestinal digestion, limited bio accessibility of the vitamin from the food matrix, and variably inter-individual ADME (absorption, distribution, metabolism, and excretion) patterns.77 It is important to state that the correlations in the above meta-analysis varied between populations and countries, ranging from 0.12 in India to 0.53 in Spain. Such population-based differences could be attributed to lifestyle factors, errors in food table compositions from which vitamin C content was derived, differences in handling of samples, or even human genetic variability due to polymorphisms that may affect pharmacokinetics.24,79 However, plasma vitamin C may serve as a proper biomarker of its intake,78 an important prerequisite prior to its assessment to act as a marker of food group intake.
Correlation coefficients between vitamin C plasma levels and dietary intake of fruits and vegetables as determined by FFQ or multiple 24 h recalls has been reported to vary in the range of 0.1–0.5.80,81 Regarding individual food items, strongest correlations have been reported for citrus fruits (0.24).81 Vitamin C is usually more abundant in fruits than vegetables (on average, common fruits, i.e., orange or berries, have 2–3 times higher concentrations than vegetables).79 In the latter group, green leafy vegetables and bell peppers have more predominant concentrations than any other vegetables (up to 4–5 times more, up to 150 mg per 100 g).16,82 Consequently, correlations appear typically higher for fruits than for vegetables. In a study with 200 adults, similar correlation coefficients of 0.2 for vegetables and 0.49 for fruits were found for men when comparing plasma vitamin C with dietary records,80 while for women correlation coefficients were lower. In addition to fruits and vegetables, wholegrain cereals do also contain some vitamin C, although its concentration is rather low and can be easily disregarded.83 Overall, the high variability of vitamin C concentrations across different food items may pose a challenge to accurately predict the amount of fruits and vegetables ingested.82
Furthermore, vitamin C is unstable and degrades under a wide array of conditions such as high pH, heat, oxygen (or other oxidizing agents), the presence of certain metallic ions, or with time in aqueous medium.32 For example, storage and processing of food items such as cooking can hugely impact its concentration, often diminishing it by as much as 50% from thermal processing alone.68,82,84 Upon sampling, degradation of vitamin C may occur in plasma samples over time if not properly handled. It has been reported that vitamin C degrades even at −80 °C, being oxidized into dehydroascorbic acid, although treatment with reducing (e.g., TCEP) or stabilizing agents (e.g., EDTA, metaphosporic acid) prior to analysis can make the samples viable for at least 1 year when kept at −80 °C and several hours if kept at 4 °C.45
Ingested vitamin C has a half-life of approximately 2 h in plasma, the time during which it is either excreted through urine79 or taken up by body tissues.77 When the daily intake dose is below 60 mg, urinary excretion is almost non-existing, indicating that most vitamin C will be absorbed and utilized by the body. When the recommended daily dose of 100 mg is taken, cells will reach a stable concentration of vitamin C that could indicate saturation.77,79 As a water-soluble vitamin, there is no specific target tissue for vitamin C that may serve as a marker for more long-term intake and that is easily accessible.79 However, vitamin C levels in plasma clearly drop upon low dietary intake, from a rather normal range of 55 μM in plasma when following the recommended daily dose to 8.7 μM when intake drops to 30 mg day−1.77 Therefore, while low levels of vitamin C intake can likely be detected in plasma, higher intakes are metabolically regulated (i.e., excreted in urine) and cannot be differentiated. As vitamin C daily needs are already covered by, e.g., 100 g leafy vegetable or citrus consumption,79 this marker is possibly not well suitable to predict whether someone is following the 5 a day (i.e., 5 times 80 g) recommendation of fruits and vegetables.
In summary, vitamin C may serve as a reasonable marker to assess whether a minimum daily requirement of overall fruit and vegetable intake is met, but not for differentiating higher amounts of intake or differentiation between fruit/vegetable subgroups, and its analytical determination requires stabilization of samples.
Carotenoids. Carotenoids are lipophilic organic pigments occurring in plants, algae, and certain types of fungi.85 Although there are >1100 compounds belonging to the group,86 only about a dozen (especially alpha-and beta-carotene, lycopene, phytoene, phytofluene, lutein, beta-cryptoxanthin, and zeaxanthin16,71,87) do play a relevant role in human nutrition. For instance, β-cryptoxanthin, α- and β-carotenes constitute a dietary source of pro-vitamin A.The intake of carotenoids may ameliorate MetS components due to their antioxidant and anti-inflammatory capacity.88 Studies have related higher carotenoid intake with reduced risk of MetS.89 For instance, carotenoids play a role in cardiovascular health by increasing the concentrations of NO in plasma, mainly through removal of O2˙−. This activity could prevent the degradation of circulating NO due to oxidation, therefore increasing its concentrations as shown through in vitro assays in different cell types.90 Moreover, carotenoid may protect endothelial health, though to date results have been inconclusive. For example, four months of lycopene supplementation (4–30 mg day−1) did not affect ICAM-1 in plasma as shown in a meta-analysis.91 Although other carotenoids (i.e., β-carotene) have been found to act similar regarding their effect on CAM molecules,92 to the best of our knowledge no meta-analyses on the relation of their dietary intake and epithelial markers have been published. While epidemiological and interventional studies in humans have shown a protective effect of carotenoids against CVD,90 the association between NO and carotenoids has so far mostly been researched in vitro on immune cells, also as animals often metabolize carotenoids in a different way than humans.90 Moreover, concentrations employed have often been rather high (in the mM range) and consequently not in the physiological range.90
The positive health effects of carotenoids are not limited to cardiovascular health alone. Epidemiological studies, such as the EPIC study, with over 37
846 participants, have shown a positive association between carotenoid intake (i.e., α and β-carotene) and the prevention and amelioration of T2D diabetes.93 While carotenoids seem to improve insulin sensitivity in humans,94 no significant reduction in fasting blood glucose could be observed in a meta-analysis when astaxanthin was supplemented (at physiological doses of 8–18 mg day−1 for 8–12 weeks).95 Similar non-significant results were obtained in another meta-analysis focusing on the natural dietary intake of lutein in longitudinal cohorts for over a decade; most studies did not find an association with insulin resistance (though a significant reduction of MetS was noted).96
Nonetheless, mechanistic models have contributed to the plausibility of carotenoids and aspects of the MetS. It was show that some carotenoids and their apocarotenoid metabolites, such as crocetin and astaxanthin, can inhibit or attenuate the JNK pathway responsible for IRS-1 phosphorylation, which is important for relaying the insulin signal to the interior of the cell (Fig. 1). Due to their increased electrophilicity, it appears plausible that this concerns especially the more polar apocarotenoids or xanthophylls.97 Similarly, fucoxanthin showed to increase GLUT4 and insulin receptors expression in the skeletal muscle of obese mice.94,98
Another pivotal aspect may be the ability of carotenoids to affect gene expression through interaction with PPARs, i.e., PPARα and PPARγ.63,99 PPARγ acts as a key regulator in insulin sensitivity, and its modulation may lead to reduced glucose plasma levels. PPARγ is involved in many other pathways, including inhibition of TNF-α or adipogenesis.94,99–101 Focusing on the latter, the association between carotenoids (i.e. β-carotene, xanthophylls) and their conversion products (following cleavage by BCO1 or BCO2) with adipose tissue and adipogenesis has been clearly described63,99 and has been confirmed in some small RCTs.102 For example, β-carotene supplementation to mice (150 mg kg−1 for 14 weeks) has shown to produce an adiposity reducing effect through its conversion to biologically active retinoids, including all-trans retinoic acid.99
The link between adipose tissue and carotenoids is further reinforced by studies in mice demonstrating shared pathways between adiposity, brown adipose tissue thermogenesis, and carotenoid metabolism.63 Paradoxically, other carotenoids and their metabolites (i.e. bixin and apo-12′-lycopenal) have shown opposite effects in mice, acting as promotors of adipogenesis through an agonistic effect on PPAR receptors (α and γ) as reviewed previously.99 The assays finding these associations were mostly performed in 3T3-L1 adipocytes and found an increased adiponectin expression as a consequence of e.g. bixin-PPAR interaction in differentiated adipocytes.99 In mice receiving bixin for four weeks (0.5% and 1% bixin), the intervention increased adiponectin without improving their obesity status.99,103 Therefore, the role of carotenoids towards PPAR is not straightforward as some could play a double-edged sword with respect to their impact on obesity and care should be taken regarding potential supplementation.
Regarding biomarkers of intake, their intake from animal food items, such as eggs for lutein and zeaxanthin, or astaxanthin for salmon and crustaceans, is rather small compared to the intake from plant based food items,87 making them appropriate biomarkers of overall plant food intake. Unfortunately, carotenoid concentrations can vary greatly, with differences in up to 20 times in amount per serving between different fruits and vegetables.104 A recent review has reported mean correlation coefficients between various carotenoid intakes and their plasma levels to vary between 0.17 (zeaxanthin) and 0.45 (beta-cryptoxanthin),87 possibly owing to the same reasons that affect vitamin C variability, including large variability of carotenoids in fruits/vegetables and relevant databases. In addition, extremely variable inter-individual bioavailability even in homogeneous populations has been reported, with values reaching 137% CV in the case of lutein.105 This variability is surely a limitation for employing carotenoids as biomarkers of their intake for the individual.106,107 Nevertheless, their plasma levels have been proposed as a suitable marker for overall fruit and vegetable intake with a mean correlation of r = 0.40,108,109 especially as their occurrence in grains, except for corn (up to 800 μg per 100 mg lutein/zeaxanthin) is rather low.110
Among carotenoids, intake of beta-carotene is highest in most countries, followed by lycopene and lutein.87 Lutein and zeaxanthin are stereoisomers, which may additionally hamper their separate identification. Lutein is usually the most abundant carotenoid in fruits and vegetables and is found in high concentrations in spinach, kale, broccoli, and other green leafy vegetables.111 β-Carotene can be found in orange vegetables such as carrots (also α-carotene in lower concentrations), sweet potato, or green leafy vegetables. β-Cryptoxantin is found rather specifically at high concentrations in certain fruits, including citrus fruits, peaches or papaya104,112 and may present a good marker for their intake. Nonetheless, pro-vitamin A carotenoids may be cleaved by β-carotene oxygenase 1 into vitamin A. Therefore, these carotenoids may present a less reliable marker of intake, given that bioconversion to vitamin A is depending on vitamin A status.113
Another special case constitutes lycopene and tomato products, as it is the most abundant carotenoid in tomato fruits. This makes lycopene a promising biomarker of tomato intake.112 Moreover, tomato-derived products such as ketchup or tomato sauce, which are predominant in Westernized dietary patterns, provide the biggest dietary source of lycopene (due also to their high lycopene bioavailability that is much higher compared to unprocessed tomatoes114) for many persons.112 This makes of lycopene not only a marker of tomato intake but also potentially of adherence to Westernized dietary patterns.87
In addition to plasma levels, novel non-invasive methods have been developed recently that show moderate correlations between intake and carotenoids in tissues. This is the case for skin carotenoids assessed through Raman spectroscopy, which have been correlated with plasma (r = 0.69) and total dietary carotenoid (r = 0.29) intake recently.108,115 Apart from the skin, other tissues can act as reservoirs of carotenoids and be used to measure fruit and vegetable intake. For example, lutein and zeaxanthin are the only dietary carotenoids to accumulate in the macula of the eye; their measurable optical pigment density (OMPD) has been proposed as a good marker, especially for vegetable intake. In a recent meta-analysis by Wilson and colleagues, a mean difference (CI 95%) of 0.11 units as per DerSimonian and Laird method between high and low intake of lutein/zeaxanthin from vegetable sources (>20 mg day−1), and OMPD has been found.116 Otherwise, carotenoids accumulate in the adipose tissue (lycopene and β-carotene),87 the liver (especially beta-carotene87), and in the brain (lutein),87 all tissues that are not normally accessible for allowing their use as a biomarker.
Regarding dietary factors, the bioavailability of carotenoids is mostly related to the lipid content of the food matrix and the cooking status, i.e., maceration of the plant cells. Given the lipophilic nature of carotenoids, these will be more easily available when high amounts of lipids are present in the food matrix.111 While cooking may increase the availability of carotenoids, they are also thermolabile. Therefore, there is a complex balance between these two components regarding the availability of carotenoids. Moreover, carotenoids are, due to their antioxidant nature, likely oxidized and metabolized into other products, such as shorter apo-carotenoids,11 which may somewhat limit their use for long-term markers of plant based intake.85,111 However, reasonably long plasma half-lives have been reported. In the case of depletion studies using labeled isotopes, half-lives of carotenoids in serum ranged between 27 days for lycopene and 66 days for lutein.117 Meanwhile, plasma half-lives after single dose studies were significantly lower, 2–7 days, with lycopene having the shortest half-live and β-carotene the longest.117 Both findings suggest that carotenoids could be a suitable marker regarding mid-term supply of dietary derived carotenoids from fruits and vegetables.
Though carotenoids are generally susceptible to heat, oxygen, and light, their stability during analyses and storage is sufficiently high, up to 10 years at −80 °C and one week at 4 °C.118 An outstanding criterion is their extremely high molecular absorption coefficient, making them suitable for detection by UV-Vis, even without prior separation by LC or LC-MS.119 Therefore, due to their reasonably long plasma half-life, their predominant occurrence in fruits and vegetables, as well as their good detectability by UV-Vis methods, these pigments appear to constitute a decent marker of choice of fruit and vegetable intake. However, rather than employing overall carotenoid concentrations, individual carotenoids are likely more promising markers for fruits/vegetables, such as β-cryptoxantin for fruit intake, lycopene for tomato products, and β-carotene for overall fruit/vegetable intake.
Vitamin E. Vitamin E constitutes a group of eight vitamers, 4 tocopherols and 4 tocotrienols, of which α-tocopherol is the main and most active form.120 With respect to MetS, much focus has been dedicated to reduction of hypertension and vitamin E and its protective effect.121 For example, a recent meta-analysis encompassing over 18 interventional studies, and 839 participants, studied the effects of high-dose vitamin E supplementation (amounts ranging between 80 and 1206 mg day−1 depending on the study) to reduce hypertension, much higher than the RDA for vitamin E of 15 mg day−1 for adults.122 The analysis only found significant effects of vitamin E for lowering systolic blood pressure but no effect on diastolic or mean arterial pressure.122 The effects were explained by the reduction of circulating ROS that protect NO against degradation. Vitamin E has also been shown to promote prostaglandin I2 levels, another vasodilator, at least in vitro using human cells. However, this association appeared tissue dependent and has not been studied in animal models.122,123Absorption of tocopherols has shown a high variability, ranging from 20 to 80% due to saturation of transporters, sequestration by the food matrix, or genetic factors.120 Most of the vitamin E in the body (90%) is accumulated in the adipose tissue for long periods, followed by the liver.120 After a depletion diet of 4 weeks in rats, no reduction in the levels of tocopherol was observed in the adipose tissue while they were depleted in the liver,120 suggesting that tocopherol stored in the liver is more readily available than from the adipose tissue. Therefore, plasma levels appear to be strongly homeostatically regulated, which would impede their use of a marker of dietary intake. Indeed, concentrations in plasma are usually low, around 27 nmol mL−1.124
Nevertheless, some studies have found good correlations between total vitamin E intake estimated by an FFQ in 300 adults and plasma concentrations of α-tocopherol, i.e. 0.51 and 0.41 for men and women respectively,125 correlations which were higher than for carotenoids, though those findings were likely driven by the intake of supplemental vitamin E.125 Other studies that have tried to draw correlations between dietary intake of vitamin E and circulating plasma levels of α-tocopherols have either been unsuccessful or found a correlation driven by the use of vitamin E supplements rather than by the background diet itself.126–128 However, another issue is that tocopherols can also be found in seeds, oils, and cereals,129,130 which interferes with their use as a marker for fruit and vegetable intake, making it necessary for it to be measured together with other biomarkers of food intake to gain specificity.131,132 Some bacteria, invertebrates, and fungi also synthesize vitamin E, though they are not relevant from a dietary perspective.
Analytically, vitamin E appears to be reasonably stable upon analyses, and storage at −20 °C resulted in 5 months of stability, while the samples remained viable for more than 28 months if stored at −70 °C.133 Tocopherols and tocotrienols can be detected at high sensitivity by fluorescence methods, given their separation form the matrix such as by chromatographic methods such as HPLC.134 Taken together, while some of its characteristics, such as its presence in plant matrices, good storability, and analytical detectability, make vitamin E a good candidate for a biomarker of food intake, it also has some major limitations, with the most notable being its homeostatic regulation.
Polyphenols. Polyphenols are secondary plant metabolites found mostly in plants though also in mushrooms and algae;135,136 they can be divided into five groups according to their chemistry: flavonoids, which are the most common and numerous group; phenolic acids; phenolic alcohols, stilbenes, and lignans.137 Overall, the >8000 polyphenols are the most abundant and frequently consumed phytochemicals in human diet, with dietary intakes of about 1–3 g d−1.138–140Dietary polyphenol intake has in general been associated with positive effects on human health, especially cardiometabolic health,137 mostly due to direct antioxidant/quenching effects141 and due to their influence on cellular signaling cascades, especially Nrf-2.142 Regarding their association with MetS, studies have shown that different types of polyphenols (e.g. anthocyanins) have a clear protective effect.143,144 Overall, a large number of polyphenols have been reported to exert a positive effect on blood pressure reduction, endothelial function improvement, increase of total antioxidant capacity in the body, reducing inflammatory responses, and glycemic control and insulin metabolism, among other benefits.144 Some of the most studied polyphenols such as hydroxytyrosol present in olive oil, or resveratrol present in red grapes and wine have been reported to increase NO production and endothelial NO synthase both in vitro and in vivo using mice models, which in turn would reduce blood pressure.144–146 Although some polyphenols seem to be capable of regulating hypertension components, conflicting data is reported in the literature.144 For instance, EGCG (a green tea polyphenol) has been found to inhibit the inducible NO synthase in macrophages by decreasing the activation of NF-κB, therefore reducing immune responses associated with this gene.145,147
Total urinary polyphenol excretion was linked in the PREDIMED study with a decreased concentration of VCAM-1 in plasma, a molecule related to cardiovascular health.148 Similar observations have been made focusing on polyphenol subgroups (i.e., anthocyanins) for both VCAM-1 and ICAM-1, as shown in a meta-analysis of RCTs.149 The anti-inflammatory properties of polyphenols have also been shown to interfere with cytokine signaling pathways in inflammation-mediated RAS, by downregulating NF-κB in activated B cells,146 reducing the circulating levels of IL-6 and TNF-α.
The protective effect of polyphenols on hyperglycemia control has been explained by two different mechanisms. First, by improving the regulation of postprandial glycemic control.144 Though the underlying mechanism is not understood, it is speculated that an insulin mimetic activity and/or the reduction of intestinal glycosidase by polyphenols may be involved in the process.150 Secondly, by regulating glucose and insulin metabolism through inhibition of α-amylases and α-glucosidases during digestion, hampering glucose release and thus intestinal uptake.144,151 Furthermore, certain polyphenols (i.e., naringenin found in grapefruits and oranges or tea polyphenols) can inhibit glucose absorption in the intestine through inhibition of sodium dependent glucose transporter 1 (SGLT-1), as shown in different intestinal epithelial cell models.152 Other polyphenols, such as quercetin, can interact with different signaling cascades (e.g., phosphoinositide 3-kinase (PIK3) and c-Jun N-terminal kinase (JNK)). These interactions can (at least in vitro) attenuate insulin resistance through increased GLUT 4 translocation.144,145 When these results from in vitro studies were tested in healthy volunteers, the effects were usually not as pronounced, mainly due to limited amounts of polyphenols found in diet and especially due to limited bioavailability compared to in vitro models, often employing supra-physiological doses of 10 μM or higher.153
As for the effect of polyphenols in adipogenesis there is insufficient available information in humans, although studies have been carried out in rodents as well as in vitro.154 Most polyphenol supplementation in vitro has been shown to interact with the PPAR-γ pathway, with some exceptions such as catechins and quercetin.155,156 In a small RCT with 25 participants supplementation with resveratrol and EGCG for 12 weeks reduced energy metabolism and cell turnover, although no effects could be noted regarding adiposity size or morphology.154 Furthermore, interactions with SIRT-1 an enzyme linked to the production of brown adipose tissue have been established.156 Nonetheless, the majority of these studies were done in vitro with often supra-physiological concentrations.156 Therefore, the actual effect of polyphenols in inducing brown adipose tissue differentiation remains unknown.
The main issue with polyphenols as biomarkers of intake is that they are largely metabolized and transformed by enterocytes and the liver through phase I and II enzymatic reactions and by the gut microbiota in the case of the non-absorbed fraction.157 In the gut epithelium, polyphenols can be conjugated into O-glucuronides, O-methyl ether, or sulfate esters, which can further metabolized in the liver.158 Those phenols that were not absorbed in the small intestine are transformed by the gut microbiota into phenolic acids, i.e., small aromatic compounds in the colon.158 Meanwhile the gut microbiota uses the sugar moiety as energy, cleaving the aglycon,158 and in part stimulating the growth of short chain fatty acid (SCFA) producing bacteria and therefore the concentrations of SCFA in the host organism.10 It is estimated that >95% of polyphenolic intake suffers some type of modification before reaching the circulatory system.157 Due to these extensive transformations, use of specific phenolic compounds to assess dietary intake may be complex as there are many unknown details regarding their metabolism,157 with a great inter-personal variability on their ADME.159 Due to their extensive metabolism, final plasma concentrations are typically not very high, e.g. 10–50 nmol L−1 in the case of anthocyanins,160 as most polyphenols, at least in their native form. Meanwhile, metabolites can reach higher concentrations of up to 4 μM.160
The use of non-specific methods to measure polyphenols, such as the Folin-Ciocalteau or the Lowry method has been widely applied in the past to identify total phenolics.161 Nevertheless, this technique may also give positive results with other reducing compounds, such as for EDTA (often used in blood vials as anti-clotting factor), ammonium sulfate (used as a food additive especially in some baking products), vitamin C, and even some reducing sugars.161,162 Therefore, such markers are too unspecific to be reliable. Recently, MS has become more affordable for researchers, which would allow for detecting polyphenols at low concentrations and give insights into the specific compounds in the sample, which can be useful for those related to specific food items. HPLC may constitute a compromise, but due to lower sensitivity and resolution, polyphenol metabolites such as glucuronides and sulfates may need to be cleaved enzymatically (e.g. as obtained from Helix pomatia) in order to reach enough sensitivity.163
Given their abundance and relatively high concentrations of polyphenols in vegetables and fruits (ca. 300 mg per 100 g (ref. 164)), total phenolics have been proposed to be a suitable marker for their intake. It has been stated to be a more reliable biomarker than carotenoids or vitamin C, as its concentrations are more stable across vegetables.16,82 Although it is viable in theory, the use of total phenols to asses fruit and vegetable intake has not been tested in detail in humans.16 Furthermore, whole grains also have high levels of polyphenols, mostly ferulic acid, up to 200 mg per 100 g.137 Thus, caution is advised when using them as biomarkers for fruit and vegetable consumption.
Looking into polyphenol subgroups, flavonoids have been proposed as complementary biomarkers to vitamin C and carotenoids for fruit and vegetable intake,16 as good correlations between their plasma levels (i.e., their glucuronide/sulfate metabolites) and their intake (r = 0.3–0.5) have been found.165,166 Nevertheless, polyphenols are usually rapidly metabolized, and thus, and their plasma half-life is typically short, around 1–3 hours (though some compounds such as epicatechins can reach half-lives close to 30 hours).167 Their short half-life limits their use as markers of short-term intake. Moreover, since there is no specific target tissue where they accumulate or are stored in the human body, no plasma half-life is related to compartmental exchanges with any deeper body pools, further limiting their use as more mid-long-term markers of intake. A good example are anthocyanins, a subgroup of flavonoids found in highly pigmented (i.e., blue, red, orange) vegetables and fruits, especially in berries.168
Certain anthocyanins or their combinations (also with other polyphenols, at least in pairs of two) have thus been proposed as biomarkers of various berry intake such as blueberries (hippuric acid and malvidin glycosides),169 while individual compounds were not regarded to constitute reliable biomarkers. These methods would thus require determination of polyphenols by MS techniques. While the parent compounds of anthocyanins have a very short half-life due to their fast metabolism, including already degradation under acidic environments such as in the stomach, urinary recovery of its metabolites may be measurable 96 hours of ingestion as determined by C13 labeled berries, thus showing the potential for using anthocyanins as a marker for berry consumption.170 However, some reports have emphasized the very low bioavailability of anthocyanins, as often <1% of ingested anthocyanins are recovered in plasma or urine.171
Anthocyanins are also contained in other vegetables, such as in some types of cabbages or beetroot, hampering their use of biomarkers of berry intake. In addition, their stability during sample processing171 and storage is also limited, with losses of 40% upon storage at −70 °C during 1 month, though with >90% for some compounds upon 3 freeze–thaw cycles.171
Naringenin and hesperetin are flavanones present exclusively in citrus fruits,172 though with some variability, ranging e.g. between 70 and 300 mg per 100 g in various citrus fruits.172 Urine measurements by HPLC and MS of these two phytochemicals have been shown to account for a large fraction of ingested flavonoids. At least within the first 4–8 hours, a time-frame after which more than 60% of ingested flavonones have been excreted via the urine,173 although a high variability between studies has been recorded regarding naringenin and hesperetin and total flavonoid urinary excretion. Both of these flavanones’ urinary concentrations had already been shown to correlate with fruit and, more specifically, citrus intake (r = 0.44).174
Of these two compounds, hesperetin showed more promising results, due to its dose–dependent correlation between intake and urinary excretion. Meanwhile, naringenin only showed differences between the lowest and highest intake groups.175 Regarding stability, both compounds were shown to be stable at room temperature for 24 h and three weeks at −20 °C. Moreover, they have also been shown to be resistant to up to three freeze–thaw cycles.176,177 Again, as with other polyphenol markers, their short plasma half-life and rapid excretion into urine impedes their use as a long-term marker of fruit/vegetable intake, while they could be well suited for measuring more short-term intake.
Additional markers. In the vegetable subgroup, sulforaphane, an organosulfur compound, and its derivatives have been related to broccoli consumption and other crucifers such as cabbage, cauliflower, and kale, through exploratory metabolomics analyses.31 Indeed, it is rather specific for these food items. Sulphoraphane is produced from glucoraphanin following cleavage by myrosinases,178 and concentrations of this precursor up to. 0.20 μg mg−1 in broccoli were reported.178 Sulphoraphane reaches its highest concentration in plasma 3 h after ingestion, being eliminated after 12 h, with a half-life of approximately 2 h.179 In urine, it can be measured as sulforaphane N-acetyl cysteine, with reported concentrations of up to 42 nmol mg−1,180 the major metabolite of sulforaphane in urine that acts as a good proxy of plasma levels and can be measured by MS. It was reported to show a half-life of 11 h in aqueous solution at room temperature and with no detectable degradation if stored at 4 °C.179,181 However, there is no apparent target tissue for sulforaphane in the human body, and the short half-life limits its use as a more long-term marker. In addition, cooking procedures can drastically reduce sulphoraphane for reasons that remained unclear.182Regarding biomarkers of fruit intake, the use of MS has also allowed for identifying many compounds that may be suitable as markers of intake for specific food items. For example, a recent review investigated 17 tropical fruits.183 Several compounds were highlighted for their promising prospects to be used as biomarkers, but only for avocados, bananas and watermelon candidates were recommended, while none was found for the other fruits.
Of especial interest are perseitol and mannoheptulose, carbohydrates made up of seven carbon atoms that have outstanding elevated concentrations in avocados and that were proposed to act as biomarkers of intake of said fruit.183,184 These molecules accumulated in urine in the hours following avocado consumption (between 3 and 12 h after intake), thus serving as a marker of short-term intake with good correlations (r = 0.77 perseitol and 0.87 mannoheptulose) to avocado intake.183,184
Regarding watermelon, the non-essential amino acid citrulline has been proposed as a candidate for watermelon consumption, but human studies are needed to study its robustness, as citrulline is formed also endogenously.185 A return to normal levels (20–40 μM) was found in humans following 24 h after watermelon consumption.185 A 16-day supplementation study with watermelon concentrated juice and an intake of 3.4 g citrulline per day resulted in a 3.5–4–6 times higher plasma concentration compared to apple juice intake,186 suggesting an interesting marker that deserves further investigation.
In conclusion, many compounds from different plant sources have been proposed to be used as markers of fruit and vegetable intake. While vitamin C remains the most popular marker, it is limited by its instability and variable content in fruits and vegetables, though it can constitute a marker of short-mid-term intake of fruits and vegetables if considering its relatively long life in tissue storage. Vitamin E has an interesting prospect, but lack of research and its presence in many food groups limit its suitability as a biomarker of intake for fruits and vegetables.
Other plant bioactive compounds such as polyphenols and carotenoids are becoming more popular as they suffer less from stability issues during food processing, intake, and storage and may at least equally well reflect overall fruit/vegetable intake compared to vitamin C. However, polyphenol half-life is short, and they are subject to extensive metabolization, which in turn poses a challenge in their measurement and usefulness, requiring typically LC-MS-MS for polyphenol analyses so that all metabolites can be taken into consideration. Carotenoids, as lipophilic compounds that are also stored in tissues, may constitute more promising long-term markers of fruit/vegetable intake, also in the sight of measuring their status by skin or macular measures as non-invasive approaches. The reasonable good correlations with carotenoids and fruit/vegetable intake are also promising, but individual carotenoids, not total carotenoids should be assessed, such as β-carotene for total fruit/vegetable intake or β-cryptoxanthin for total fruit intake.
Currently, no BFI on the whole group fulfills all the criteria to become a gold standard. However, lipophilic constituents with longer half-lives that are specifically found in fruits and vegetables, such as certain carotenoids, are likely the best measure for overall fruit/vegetable intake, also in sight of their detectability by affordable methods such as UV-Vis.
Legumes
Legumes or pulses refer to the family of plants Leguminosidae, which produces seeds used in traditional human diets. Some frequently consumed legumes include lentils, chickpeas, peanuts, peas, or soybeans.40 Of said pulses, soybeans have been the most widely studied, likely due to their high protein content and the comparably high biological value of soy proteins. Nonetheless, a growing interest in other legumes such as lentils and chickpeas has been detected in recent years.31,187 In general, legumes are marked by both a relatively high protein content (up to 50% seed dry weight, ref. 188), and a relatively high dietary fiber content (15–30% of dry weight, ref. 188). Although it was initially believed that legumes had a protective effect against MetS, a recent meta-analysis has found no correlation between legume intake and MetS.189 Despite their central role in many diets, no markers of overall legume intake with good specificity have been researched to date. Nevertheless, the alkaloid trigonelline has been proposed in multiple occasions to be a good reporter of overall legume intake.31
Isoflavones. Isoflavonoids, the parent group of isoflavones, are a subclass of flavonoids that occur in vegetables and are mostly restricted in their occurrence to legumes, especially soy.190 As Isoflavones are a subclass of polyphenols, most of the health outcome properties earlier discussed also apply here. Particularly intake of isoflavones has been linked to a reduced risk of MetS.191Two predominant isoflavones, genistein, and daidzein, have been proposed as biomarkers for pulses consumption, and again especially for soybeans, as their concentration in plasma and urine has shown a dose–dependent relationship with their intake, as reviewed by Sri Harsha et al. and Lampe et al.40,49 Soybeans contain approx. 2.5 mg per 100 g of isoflavonoids.192 As intermediate lipophilic compounds, they are directed to the liver after intake, where they are metabolized into glucuronide and sulphate forms that are excreted via entero-hepatic-recirculation and further metabolized by microbiota into other compounds (mostly equol and O-desmethylangolensin (O-DMA)). Following re-circulation, both the original compounds and the metabolites are excreted through urine in the form of sulfates and glucuronides.193 In the case of daidzein, the correlation between dietary intake (measured with FFQ) and urinary excretion (spot urine sample) (following cleavage of glucuronides/sulfates) was r = 0.50, and for genistein, the correlation was reported at r = 0.48, while for equol the correlation was only 0.11, likely related to the fact that some persons are not considered equol producers.40 The correlation for daidzein and genistein was more accurate when 24 h urine samples were investigated, with correlation factors of r = 0.72 and 0.67 respectively, while no significant correlation could be drawn for equol.40 The same – improved correlation with 24 h instead of spot urine sampling, is likely true for all correlations, as this may overcome issues related to the circadian rhythm, even when correcting spot urine samples for urinary creatinine.194 Urinary recovery postprandial is usually complete only after 36 h, which could indicate its usefulness as a marker for short-term intake,40 but likely not for habitual long-term intake, and there exists no target organ for isoflavonoids.
Measurements in plasma have also shown good correlations, although with higher variability between studies,40 perhaps due to the rapid excretion via urine. The plasma disappearance half-lives for these two compounds show variability between studies, although a good approximation would be 8 h and 6 h for genistein and daidzein, respectively, in line with the rapid and complete urinary excretion.40 Overall, these two isoflavones appear as suitable markers of short-term soybean intake, although further testing in larger and non-controlled settings would be required for further validation. Regarding their metabolites, equol and O-DMA, these have not received sufficient attention in their use as BFIs of soy, likely due to the expected larger variability in concentrations compared to their parental compounds.40 Although they may reach concentrations up to 40 times higher than the original (native) compounds in urine, the total excretion time (4 days) and high inter-individual variability does not make them a better fit as BFIs than either genistein or daidzein.40
Assessment of isoflavonoid in urine and plasma can be carried out via HPLC-UV-Vis detection,195 though due to lower sensitivity of HPLC compared to MS, cleavage of glucuronides and sulfates into the respective aglycons such as by Helix pomatia enzymes are typically carried out.195 Finally, both genistein and daidzein are quite stable after 3 months of storage at −20 °C, which would be a favorable attribute toward their use as biomarkers.40
As for other pulses, a recent metabolomics study focused on urinary BFI of chickpea, lentils, and white bean intake.196 This study revealed some compounds that could be used to assess short-term intake of different pulses. For lentil intake, associations with flavan-3-ol metabolites and arginine-related compounds were reported.196 Regarding white beans, jasmonic acid derivate compounds and pipecolic acid were reported. Finally, protocatechuic acid glucoside served as a marker for BFI for chickpeas. However, none of these markers has been more rigorously studied and the amount of data on these potential BFI is limited.
Grains
Cereals, the edible seeds of the Gramminae family, constitute one of the most important sources of dietary fiber and energy worldwide.197 Cereals can either be consumed as whole grains or as refined grains, in which the nutrients and non-nutrients in the germ and bran are removed, improving their shelf life, texture, and popularity.198 Regarding grain intake and MetS, meta-analyses have proposed a reduction in MetS in persons consuming preferably wholegrain cereals, including bread.12 The refinement of cereals also means that the outer layer of the seed, which contains most vitamins, minerals, polyphenols, and dietary fiber, is lost.132 Nevertheless, even though the concentration of phytochemicals is low in refined cereals,132 it may be important due to the high consumption of cereals throughout the day, having the same potential effect as that of vegetables or fruits, at least in high consumers.132 However, to date, no biomarker of refined grain intake has been established, possibly because most of the unique bioactive compounds in different grains are found in the outer layers of the seed. Moreover, in most studies where novel biomarkers have been assessed, refined grains were usually the control group, which further impeded the finding of biomarkers in refined grains.30 Interestingly, no study has focused on vitamin E, i.e., in the form of tocotrienols (since they are more abundant in cereals199), as markers of grain intake, even though it could be an interesting marker when used in combination with others.
Carotenoids and polyphenols. Carotenoids are abundant not only in fruits and vegetables but are found also in cereals, even though at marked lower concentrations (rather around 10 μg g−1 (ref. 200)), while concentrations of polyphenols are typically comparable to those encountered in fruits and vegetables, i.e. up to 900 mg per 100 g.201 The health promoting benefits of carotenoids and polyphenols discussed for the plants and vegetables section also apply for the secondary plant metabolite present in grains.In the carotenoid group, the most prominent ones are likely lutein and zeaxanthin, which have shown relatively high concentrations in corn (800 μg per 100 mg), constituting one of the main dietary sources.110,202 Therefore, zeaxanthin could be a good marker of corn and corn products (such as corn-bread intake), as shown in a recent study in which a moderate correlation of r = 0.181 was revealed between OMPD and sweet corn intake.203 However, analytically, zeaxanthin is often detected concomitantly with lutein, with the latter carotenoid also been found in many vegetables, thus impeding its use as a specific marker for grain intake. The content of the other carotenoids would be much lower than zeaxanthin and their predominant presence in fruits/vegetables would not make them a suitable marker for grain intake.
Regarding phenolic compounds in cereals, flavonoids and phenolic acids are the most common ones, with levels up to 2.2 mg per g dry matter.204 Some of the latter are rare and specific to certain grains, as it is the case of avenanthramides (AVA), which can be found in oat.205 AVAs are a family of N-cinnamoyl anthranilic acids unique to oat, and they have shown promising results in the short-term assessment of dietary intake.30 AVAs half-life however is short (2–5 hours), due to the extensive metabolism they undergo in humans, as for many other polyphenols. Moreover, the bioavailability of these compounds seems to be dependent on the gut microbiota and thus shows great variability between study participants.30 Nevertheless, AVA metabolites could be found in the urine after 8 h of consuming oat-enriched food products,30 although no correlation between them and dietary intake has been reported yet. Therefore, their suitability as at least short-term markers of oat intake awaits further investigation.
Alkylresorcinols and other. Alkylresorcinols (ARs) are 3,5-dihydroxy-phenolic compounds and, thus, polyphenols with an alkyl chain of varying length found in the outer parts of some cereals. These compounds have been linked in some studies with a decreased risk of MetS and T2DM.206,207 ARs with odd-length chains are only found in wheat, rye, and barley grains. Meanwhile, ARs with even-length chains have been reported in quinoa. Since ARs are exclusively present in cereals, they have been proposed as markers of their intake.30,208 Odd length ARs are the most widely studied BFIs of grain, and their half-life in plasma after intake of grains is about 5 h, which allows assessing short-term intake.30,208 Urinary levels of 1,3-dihydroxy-benzoic acid (DHBA) and 3-(1,3-dihydroxyphenyl)-1-propionic acid (DHPPA), metabolites of ARs have been positively correlated (r = 0.4–0.6), with wheat, rye, and barley consumption. Moreover, they have also been proposed as a marker of gluten consumption (since only gluten-containing grains have -odd-length ARs).209On the other hand, even length-chained ARs seem to be greatly present in quinoa, and thus they could be used as a biomarker of quinoa intake, although research has not been as extensive as for odd chain ARs.30 Interestingly, ARs accumulate in adipose tissue and thus may be used as a long-term BFI as its concentrations in adipose tissue correlate strongly (r = 0.6) with intake.210 Although a good correlation (r = 0.49–0.81) between plasma and adipose tissue ARs has been reported, the turnover rate and half-life of ARs in adipose tissue have not been studied in detail to our knowledge.211
Benzoxazinoids are another group of lipophilic, naturally produced secondary plant metabolites that are mostly found in wheat and rye grains that can be both identified in flours and bread products (2.3–3.3 mg per g dry weight). Although intake of products containing benzoxazinoids has been shown to increase their concentrations in plasma and urine, the correlation between intake and concentrations in humans is still poorly understood, with high variability between studies,30 possibly due to the high variability in their bioavailability. Benzoxazinoids are usually excreted either non-metabolized or as phase II metabolites conjugated with sulfate groups or glucuronides.212
Overall, and besides their ubiquitous presence in the human diet, not many BFIs of cereals have been researched. ARs seem to be a promising marker given both their short and long-term good correlations with intake and concentrations with adipose tissue. Moreover, AVAs and other polyphenols may act as promising markers, but not enough evidence to support this claim is available yet.
Nuts, seeds, and oils
Although nuts, oils, and seeds are (i.e. botanically) part of fruits and vegetables, these are usually considered as a separate food group, due to their high level of proteins, fats, and energy. The reason for grouping these three food components together is that they share a similar nutrient profile, being rich in FA, especially PUFAs and MUFAs, and this group has shown health benefits against the risk and treatment of MetS, as reported in several meta-analyses,213,214 despite obvious differences in protein content between the three. To the best of our knowledge, no BFI has been described that reflects the intake of the whole group, but rather, BFIs for individual food components have been mostly researched.
Fatty acids. Omega-n-3-PUFAs have been associated with improved insulin sensitivity for some time.62 The most accepted theory at present is that n-3-PUFAs are capable of improving β-oxidation in cells, which decreases both lipid accumulation (and its associated lipotoxicity) and ROS accumulation, both triggering inflammatory responses.62 In addition, n-3 PUFAs are generally ascribed to exerting an anti-inflammatory response.62 Their acting as precursors for resolvins, anti-inflammatory molecules capable of acting in very low doses downstream of TNF-α, and anti-inflammatory prostaglandins could be a contributing factor to the observed health benefits that has been observed in both in vitro and small clinical trials.215,216 Regarding MetS, several studies have proposed a reduction in MetS risk with increased consumption of PUFAS.217Oils and nuts are the main natural sources of PUFAs and MUFAs in the diet, and both α-linoleic acid (PUFA) and oleic acid (MUFA) have been proposed to be BFIs of certain types of oil and walnuts, which are especially rich in PUFAS.218–220 Unfortunately, these FAs are rapidly metabolized, transformed, or stored.221 Although approximately 1% of the total FA in adipose tissue accounts for α-linoleic acid, the fluxes between adipose tissue and blood have not been characterized,221 which limits their use as long term markers. Additionally, concentrations of FAs in food items may vary greatly in the same food according to season, country of production, or even variety, as it happens for olive oil.222 For these reasons, FAs are not recommended as standalone BFIs but rather to be used with other BFIs, such as urolithins in the case of walnuts,131 as described in the following section.
Polyphenols and metabolites. Urolithins are the result of the heavy metabolism of the hydrophilic polyphenols ellagitannins and ellagic acid in the gut microbiota.223 Urolithins have been shown to exert protective effects against certain cardiometabolic diseases such as type 2 diabetes and MetS.224 Cellular models have shown an immune suppressor capacity of urolithins inhibiting ROS production in white blood cells and suppression of inflammatory cytokines, such as IL-6 or TNF-α through inhibition of the upstream NF-κB pathway.224 Furthermore it has been shown to increase lifespan in the flatworm C. elegans through a protective stress against OS caused by mitochondrial ageing.225 Another classical component of MetS, dyslipidemia, has also been shown to be ameliorated through the actions of urolithins, mainly through an increase of fatty acid oxidation that reduces circulating tryglicerides, all being mediated by an AMPK pathway activation as shown in human adipocytes and hepatocytes.224Ellagic acid (urolithins parent compound) occurs in many vegetables but has its highest concentrations in berries and walnuts (up to 1900 mg per 100 g (ref. 226)), which constitute, in that order, their main dietary sources.226 Unfortunately, for that reason, urolithins alone cannot act as BFI of walnuts; thus, it has been proposed that the unique FA composition of nuts in combination with urolithin concentrations can be used to indicate nut consumption.66,131 Phase II metabolites of urolithins have shown to have a half-life in plasma of approximately 24 h,223 enabling their use for short-term intake markers. Nevertheless, the lack of dose–response correlation studies for walnut intake with urolithins may also impede the task of using them as adequate markers of intake. Especially as it has been hypothesized that ellagitanin bioavailability (and thus urolithins excretion) is greatly dependent on the food matrix composition,131 as non-absorbed ellagitanins will be transformed into urolithins in the gut, although this process is further dependent on the host gut microbiota.157
Additionally, the high selenium concentrations present in nuts, together with other minerals, can give an extra dimension to the biomarker analysis and improve the specificity to be employed as a multi-modal marker for nuts intake.131 Nevertheless, the strict homeostatic regulation of circulating trace elements may not allow for their use as reliable biomarkers.227 In addition, Se concentration is very variable in nuts, ranging between 0.2 and 512 μg g−1. Especially Brazil nuts can be an excellent source of Se, but as it is a ubiquitously present trace element, it cannot be used alone as a marker of the intake of nuts.228
Finally, the polyphenol hydroxytyrosol and its metabolites have also been proposed as BFIs of olive oil.131 Hydroxytyrosol is also regarded as a lead compound of olive oil to which a health claim by EFSA has been granted.229 The excretion of urinary hydroxytyrosol has been correlated with the intake of olive oil in multiple studies, although the exact correlation coefficients have not been disclosed. Hydroxytyrosol excretion usually occurs in the first 6 h post consumption, mostly in the form of different metabolites, as it is common for polyphenols.131 Therefore, it may constitute at best, a marker of short-term intake. Furthermore, hydroxytyrosol also occurs in other food items such as wine, which is, after olive oil, its second predominant dietary source, and it may be intimately linked (as olive oil) with Mediterranean diet styles.230
Meat and fish
Meat. Meat is a dietary component originating from animals that is rich in proteins and fat and that is predominant in Westernized dietary patterns. Although a nutrient-rich food, its excessive intake has also been associated with several health issues,231 including the increased risk of developing MetS.232 Some meta-analyses have related meat consumption with the risk of developing MetS.13 Two categories of meat are widely recognized according to their components and animal source: white and red meat. However, regarding the risk of disease, especially processed meat (RR = 1.35) has been associated with an increased risk of cardiometabolic diseases,13 including MetS, while white meat has even shown a protective effect for MetS (RR = 0.86).13Regarding total meat intake, histidine-derived compounds, i.e. dipeptides, have been proposed as markers. These include 3-methylhistidine (3-MH), a compound with very low endogenous formation in humans (3 μmol kg−1 day−1),233 but that can be found in meat in higher concentrations (>120 μg g−1).234 This high specificity to animal products has led some researchers to propose 3-MH as a BFI of total meat intake as measured by LC-MS. Unfortunately, 3-MH has poor stability even when frozen, resulting in significant losses during 5 months storage at −40 °C.235 Additionally, not much is known about its metabolism or kinetics in plasma or urine. Moreover, it has been shown that many factors, such as age, gender, or frailty, may also affect the levels of 3-MH.236 Finally, it may also be indicative of the intake of fish.35
Besides the apparent limitations, its levels in plasma and urine have been analyzed and correlated well (R = 0.6–0.7, depending on the population) with total meat intake, as assessed through FFQ and 24 h recalls.237,238 Moreover, the dipeptide anserine (3-MH + alanine) has also been linked with meat intake, although it is not clear whether it can be used for the whole group intake or only for poultry intake.237,239 Another BFI of total meat intake that has traditionally been used is total nitrogen in urine.240 Nevertheless, N concentrations in urine are rather linked with protein rich food intakes, which includes other groups such as legumes.240 Given the lack of specificity and the presence of more promising biomarkers, total N or urea concentrations are not further discussed in this review.
Fish intake. The protective effect of PUFAs and MUFAs (see also section nuts, seeds, and oils) as anti-inflammatory and antioxidant molecules is likely at least in part explaining the positive role that eating fish can have on risk of MetS.241,242 Dietary fish intake has traditionally been studied using the n-3-PUFAs docosahexaenoic acid (DHA) and eicosapentaenoic acid (EPA) concentrations in blood and serum.243 Even though α-linolenic acid (plasma half-life 1 h),244 a substrate for both DHA and EPA, is present in other food groups, the conversion rate to these FAs is very low in humans (0.2% for EPA and <1% for DHA).243 Thus, fish can be considered the predominant source of EPA and DHA,243 whose plasma half-lives have been reported as 2–3 days and 1 day, respectively, although a high variability between persons has been registered.244,245 Those half-lives would allow for a midterm (1 week) assessment of diet, especially in the case of EPA.However, other factors may affect n-3-PUFAs levels besides diet, such as age, sex, or smoking status.246 Although these factors affect the circulating levels of n-3-PUFAs, they can be accounted for in statistical models. Moreover, they do not change diet as the main source for changes in n-3-PUFAs concentrations in plasma.246 Some authors have claimed that cooking techniques (i.e., frying) of fish may also affect EPA and DHA concentrations,247 which remains controversial, as many studies have not found such an association.248,249 Intake of total seafood assessed through FFQ has been shown to correlate moderately (r = 0.2–0.4 depending on the ethnic group) with plasma levels of EPA + DHA.247 N-3-PUFAS are very susceptible to oxidation; thus, samples used for testing these biomarkers should be handled carefully to prevent lipid peroxidation.250 Storage at −75 °C of blood samples containing EPA and DHA keeps the concentrations without significant reduction even after 6 months. Moreover, treatment with antioxidants such as butylated hydroxytoluene may be a suitable alternative for storage at room temperature or 4 °C when −75 °C freezing may not be immediately possible.251
Besides the limitations they may face, n-3-PUFAs are widely regarded as strong biomarkers for fish intake.252 Their concentration is typically determined by GC-MS following methylation of the fatty acids, a method that has become reasonably available,253 though it is still not used in clinics.
Another molecule that has been linked with fish intake is trimethylamine-N-oxide (TMAO), which can be measured in both urine and plasma. TMAO intake has been further been discussed to be also a promising marker related to human health, as it has been associated with several obesity related diseases, including MetS.254 It is of remarkable interest as a mediator of inflammation.255 Although it has been linked to cholesterol metabolism and pro-inflammatory cytokines, the full underlying mechanism being not fully understood.256 Most TMAO (>95%) is excreted through urine with a peak after 24 h of intake.257 While plasma levels reach a peak around 12 hours after intake, it's plasma half-life has not been reported to the best of our knowledge.257
TMAO is formed in humans after the oxidation of trimethylamine (TMA), which also originates endogenously from the metabolism of choline.258 This metabolism of choline into TMA seems to be achieved by the gut microbiota after the small intestine transporters are saturated at concentrations of choline around 300 μM.259 L-Carnitine has also been reported to be metabolized into TMA.259 Seafood is high in TMAO.260 Thus, fish are direct dietary sources of this compound.259
Although fish intake had shown to increase plasma levels of TMAO significantly, a correlation between fish intake and plasma TMAO levels had not been drawn until recently.237 In a recent study analyzing two independent cohorts, TMAO plasma levels have been shown to correlate positively with n-3-PUFAs intake (r = 0.22) and fish intake (r = 0.215).261 Unfortunately, TMAO can be obtained from other dietary sources apart from fish, as its precursors (choline and L-carnitine) can also be found in vegetables, grains, and poultry.237 Moreover, the same study that drew a correlation between fish intake and TMAO also found correlations between TMAO plasma levels and vegetables, as well as whole-grain products (r = 0.27 and 0.20, respectively).261 Analysis of TMAO from plasma or urine samples is typically carried out via HPLC-MS, NMR, or UHPLC-UV.261–263 TMAO has been shown to be stable in plasma samples for up to 5 years if kept at −80 °C.262 Thus, although a promising short-term biomarker of fish intake, it should always be used in combination with other BFIs.
In conclusion, 3-MH is one of the most promising BFIs for assessing total meat intake, given its high specificity, its correlation with meat intake, and the cross validation with FFQs. While it fulfills many of the criteria to be considered a good BFI, it is still necessary to conduct more validation projects in larger populations before being able to be used in research and clinical practice. Additionally, more research on its metabolism and kinetics are needed. Moreover, it has poor stability upon storage. Overall, 3-MH may be a good tool for assessing total meat intake, but it is not selective as it can be taken up from fish, which does not allow contrasting meat and fish intake regarding their different associations with health outcomes. Thus, other markers may be used in conjunction to differentiate from fish and seafood intake, which could include circulating n-3-PUFAs (DHA and EPA) and TMAO. These two have shown promise in their correlations and stability, with the main difference being that TMAO is less specific for fish and seafood and thus it should be used in conjunction with DHA and EPA, which are possibly the most specific assessable short/mid-term markers related to fish intake.
Dairy products
Overall, besides the global importance of dairy foods in many diets and health benefits, not many markers for whole dairy consumption or for specific components have been identified.264 Overall, the relation of total dairy consumption and risk of MetS is not very strong,265 although meta-analyses have shown clear associations between milk and yogurt consumption and a protective effect against MetS.266 Some fatty acids found in dairy have been proposed as markers of total dairy. Furthermore, products of dairy fermentation, such as acylglycines in cheese, have also been suggested for selected dairy products.267
Fatty acids. The most popular markers to assess dairy intake are dairy-characteristic fatty acids. To this end, both pentadecanoic acid (C15:0) and heptadecanoic acid (C17:0) have remained popular markers of full fat dairy intake.264,268,269 These fatty acids originate from bacterial fermentation in the rumen of cows and are thus specific to the intake of ruminant derived food items.270 Nevertheless, the correlations between these FAs and dairy intake have shown high variability between studies, possibly due to the varying degree of the production of such fatty acids related to ruminal fermentation between dairy products.271 Moreover, they have also been associated with other dietary components besides dairy products (i.e., populations with a high intake of fish).264 These fatty acids are, like the even-numbered fatty acids, similarly distributed and metabolized in the body, and thus, their half-life in plasma is relatively short, with a peak in plasma concentrations after 4 h of consumption.272Other studies have shown associations between different sphingomyelins and dairy intake; nevertheless, the data is still very limited, and sphingomyelins are present in other foods as animal products or nuts.264,267,273 Thus, the use of FAs to assess total dairy intake is questionable, and better BFIs should be developed, not only in sight of their specificity but also regarding their short half-life.
Acylglycines and carotenoids. Acylglycines have been traditionally used to detect inborn metabolic defects through urinary analyses (i.e., β-oxidation defects), and until recently, they had never been related to the intake of any dietary component.264,274 The presence of some acylglycines (isovalerylglycine, isobutyrylglycine, and tiglylglycine) in urine after cheese intake was reported by MS-LC by Hjerpsted and colleagues over a decade ago and hypothesized to have an origin in bacterial fermentation that occurs during cheese ripening.275 Since acylglycines have not been associated with any other food component to date, they constitute a promising marker for cheese intake. Unfortunately, and although it was first reported over a decade ago, this correlation has not been further studied to the best of our knowledge. Some products may be very specific to the specific cultures used during cheese ripening. For instance, red smear ripened soft cheeses produce very specific carotenoids, allowing for unique identification of the consumption of these cheeses, at least in theory,276 as very little is known on the understanding of the ADME of these carotenoids.Overall, BFIs for dairy consumption remain elusive. In most cases, components derived from bacterial fermentation that originate from the different types of dairy have been the target for BFI development. Unfortunately, those compounds are either not specific enough (i.e., FAs), have a short half-life (i.e., FAs), or their metabolism is poorly understood (i.e., acylglycines and bacterial carotenoids). For this reason, no BFI has received enough validation to be further used in research, and the search for an appropriate marker continues.
Beverages and drinks
Total alcohol. Alcohol consumption has been reported in some instances to produce some positive health effects regarding cardiovascular diseases,277 however this results are generally discussed within the scientific community. Regarding its effect on MetS the effects are more discussed and while alcohol abuse is positively linked to MetS risk, moderate consumption has again been argued to have a protective effect.277,278 Total alcohol consumption can be measured by ethanol metabolites such as ethyl glucuronide and phosphatidylethanol.279 Both of these markers can be detected in serum and urine after a few hours of consumption and for several days after intake, thus being useful biomarkers of short and mid-term intake.279 They are also fairly specific as they are derived almost only from ethanol containing drinks. An interesting approach is studying their concentration in hair samples, which can reflect a much more long-term marker, up to 12 months.280 Detection of alcohol metabolites in hair has attracted some attention in the past decade, as it shows a good correlation with intake (r = 0.54–0.89)280 and can easily be detected by LC/MS and GC/MS.281 Moreover, the samples show good stability upon storage, although in controlled environments, alcohol metabolite stability has only been analyzed for a few months. Ethyl glucuronide has been employed for assessing drinking habits from XIX century mummies’ hair.281 While total alcohol BFIs can be very useful (e.g., forensic diagnostics), it would also be of interest to develop biomarkers that allow for further characterization between some of the most popular alcoholic drinks (i.e., wine and beer),282 as differences may exist regarding health related outcomes for some of them, such as for red wine. Disputes still exist on whether certain sources of alcohol may have less negative health consequences compared to other sources due to other components present in certain drinks, such as polyphenols (wine) or humolone from hops (beer).277,283
Wine. Wine, and especially red wine, due to the maceration process that extracts the contents of the grape skin into wine, is a fermented alcoholic drink rich in plant bioactive compounds. These compounds, most of which are polyphenols, have been proposed to be used as BFI of wine intake.50 The health promoting benefits of polyphenols (chapter fruits/vegetables) surely contribute to the reported health benefits of wine. Regarding tartaric acid, although some epidemiological analysis have found correlations with improved health status, a mechanistic link has not been validated to our knowledge.284Resveratrol is one of the most prominent polyphenols present in wine, in some cases reaching concentrations of up to 36 mg L−1,285 and has been extensively researched together with its most common phase II metabolite (cis-resveratrol-o-glucuronide), as a BFI of wine intake. These compounds can be detected in plasma within 1 h of intake and have a plasma disappearance half-life of approximately 9 hours.286 Resveratrol and its metabolites show an association with wine intake, although it is not known whether there is a clear dose–response relationship.50 Nevertheless, since resveratrol is present in grapes, the consumption of this fruit or its derivate (i.e. grape juice) may be related to the presence of resveratrol in plasma.230 However, the short half-life of most phenolics in general would again impede their use as a more long-term marker of wine intake. Other phenolics, such as hydroxytyrosol, have also been associated with wine intake but lack the specificity needed to be good biomarkers as they are also present in other foods such as cocoa and olive oil.131,271
Another compound that has been proposed as BFI of both wine and grapes is tartaric acid, which is naturally occurring in grapes. Tartaric acid has been fully validated as a marker for wine intake if corrected for grape and raisin consumption while also showing a dose–dependent relationship with urinary concentrations of 0.01 μg ml−1 and a usual background level in non-wine consumers of 68 ng ml−1.50,284 It can occur in concentrations of up to 1047 mg per 100 g of grapes, which are much higher levels than those encountered in other fruits (e.g., 0.04 mg per 100 g in lemon or 0.32 mg per 100 g of peach) or even vegetables, with relatively high concentrations such as lettuce (5 mg per 100 g).287 Its urinary excretion has been strongly associated with wine intake (r = 0.92).288 The metabolism of tartaric acid in humans is rather unknown, although high levels of tartaric acid can be found in urine shortly after intake of grapes, while the same is not true for plasma levels, which seems contradictory. Tartaric acid is quite stable ex vivo; it can be stored at −80 °C and detected, e.g., by MS or HPLC, although a maximum advisable storage time has not been reported to our knowledge.288
Beer. Beer is a complex drink with many compounds present in it, with three of them having been validated to different degrees as BFIs: isoxanthohumol, 8-prenylnaringenin, and iso-alpha-acids.50,271 As a polyphenol, isoxanthohumol has been linked with multiple health promoting properties. For instance, mechanistic studies in cell lines have shown its anti-inflammatory capacity through regulation of NF-κB and TNF-α. Furthermore, it has been associated with cardiovascular health as a regulator of angiogenesis.289 Although isoxanthohumol has been proposed to be a protective molecule against MetS,290 to the best of our knowledge no studies have been reported that have tested this hypothesis.These compounds typically originate during malting and fermentation.271 While iso-alpha-acids have reasonable specificity for beer, they are also easily degraded both during beer storage and in the human body, inducing a high variability in their concentrations, which limits their usefulness as a marker of intake.50 The flavonoid isoxanthohumol has its origin in the hops used in beer production and has no other dietary source known to date, although it is dependent on bacterial transformations by the gut microbiota that can affect its circulating levels, creating high inter-individual variability.50,271 These transformations can result in the production of 8-prenylnaringenin, which has also been validated as a biomarker of beer intake. The rate of biotransformation from isoxanthohumol to 8-prenylnaringenin is highly variable between individuals, ranging from 0 to 100% in the most extreme cases; thus, both isoxanthohumol and 8-prenylnaringenin should be employed together.271,291 The half-life of these compounds in blood is short to intermediate, around 20 to 25 h.292 Detection can be best carried out by LC-MS/MS methods.292
Coffee & tea. Coffee and tea constitute some of the most popular drinks worldwide and are subject to a natural process of fermentation after harvesting the beans and the leaves, respectively.50,271 Regarding coffee and tea consumption (green or black), both have been associated with reduced risk of developing MetS as shown by a recent meta-analysis.293Coffee is also a good example where the speed of metabolism, determined by genetic polymorphisms, is likely to influence the validity of a BFI within a population, as fast and slow coffee metabolizers have been reported related to cytochrome P450 polymorphism.294
The fermentation produces metabolites exclusive for these products, such as the brownins, which are the products of catechins and gallate fermentation. Although interesting compounds for BFI use, no research in this regard has been conducted.271 Other products such as caffeine and (epi)catechins, are produced in planta and could thus also function as markers of unfermented products such as white teas. While some candidate BFIs of both tea and coffee share some overlap, (i.e. caffeine and epicatechins), there are also some compounds exclusive to coffee and tea.271
Regarding coffee, some biomarkers have been associated with the unique furans originating through the Maillard reaction after roasting the beans.271 This is the case for 2-furoylglycine (2-FG), which is a product of coffee roasting.295 In a small clinical trial, 2-FG was detected in urine after coffee consumption but not after consuming a coffee substitute (tea, chicory) intake.296 2-FG was detected in both urine and plasma after coffee intake, with the peak in the former taking place within 2 h of intake and with levels in urine returning to baseline within 24 h of intake,296,297 indicative of a short half-life in the circulatory system. Another compound present in the coffee beans is the alkaloid trigonelline, with its concentration being negatively correlated with roasting.298 Trigonelline has been detected in both plasma and urine after coffee intake a direct correlation with it has been shown. Nevertheless, trigonelline has recently also been associated with legume intake, which reduces its specificity.29 It is needed to assess how these findings may affect the previous claims that trigonelline was among the most reliable markers of coffee intake.271 Additionally, it remains detectable longer than 2-FG since its urinary levels remain elevated after 48 h of coffee consumption.295,299 Nevertheless, its half-life in plasma is rather short (5 h).300 Analytically, it can be detected by HPLC, GC-MS, or LC-MS301 and showed good stability upon storage at room temperature for 9 h and 4 °C for 24 h.302
The use of MS also opens the door to other tissues to be used as biomarkers. This is the case of hair, which has been employed to detect caffeine in hair by LC-MS-MS analysis.303 Caffeine is rather fully absorbed and thus there is little variability regarding its absorption.304 Moreover, this hair matrix allowed discerning between high intake participants (above 5.5 ng per mg hair) and ordinary (below 5.5 ng mg−1) caffeine consumers.303 Although the use of hair offers a much better long-term marker of intake than caffeine detection in plasma with a relatively easy to follow protocol, some issues arise, such as partial validation of the correlation only with FFQs but not with blood/plasma samples. In this regard collecting further data to describe the quantitative relation between caffeine intake and accumulation in hair is needed. In an older MS study, the mineral concentration (i.e., higher Al, Ni, S, and Ti & Lower Pb) of hair was also related to coffee intake.305 This subject has not been further analyzed to our knowledge and, in some cases, could be linked to the cooking technique (i.e., Al in the coffee Brewer) rather than to the food item itself.305
In the case of tea, its phytochemical profile reveals a unique composition of catechins and their phase II metabolites. Thus, catechins have been the main biomarker of tea intake. The most common catechins associated with tea are epicatechin (EC), epicatechin gallate (ECG), epigallactocatechin (EGC), and epigallactocatechin gallate (EGCG). Of those, EGCG, EGC, and ECG are exclusively found in tea.295 Their plasma half-lives are close to 3 h following ingestion, and their urinary peak occurs 2 h after intake. There seems to be an agreement that urinary excretion of EGC and EC occurs within the first 8 to 9 h after ingestion, thus allowing them to constitute short-term markers of intake.306,307 Only EGC has been successfully used in cohort studies, also as EGCG and ECG have limited and variable absorption in the gut, which may impede their study outside of controlled environments.295 Other, more long-term markers of tea intake have to our knowledge, not been investigated. The study by Chojnacka et al.305 also studied the relation between the consumption of tea and the mineral composition of hair; decreased amounts of Hg were found in self-reported high consumers of tea, but such findings are rather considered circumstantial.
Sugar-sweetened beverages (SSB). SSB consumption has strongly been related to negative health outcomes, likely due to their high energy and sugar content, and low satiety value, resulting in likely over-consumption of such beverages. Interest in the development is mainly due to the numerous health concerns related to the intake of SSB, such as an increased risk of T2DM, CVD, and MetS.308Developing BFIs for sugar-sweetened beverages (SSB) is a big challenge, often because of the simplicity of the general matrix and the diversity of the group. In those regions where the main added sugar is originating from a C4 crop (i.e., sugarcane and corn in the USA), the ratio of the C isotopes C13/C12 has been utilized as a promising proxy of added sugar intake, and thus of SSB.295 This is possible due to the different metabolisms between C3 and C4 plants and how C3 plants discriminate C13 isotopes, resulting in a lower C13 to C12 proportion.295 Unfortunately, if the main source of added sugars is not a C4 vegetable (i.e., sugar beet in Europe), this method is not insightful. Many other sweeteners such as non-nutritive sweeteners (e.g. aspartame, acesulfame etc.) exist that are regularly added to beverages, especially sodas, instead of sugar, and that have also been proposed to be used as markers of these soft-drinks (Table 6 in ref. 295). Though some issues arise, for example, aspartame is degraded during digestion, and its products are no longer specific for SSB intake, other sweeteners can also originate from other products such as gums and other sweets or yogurts. Also, the variety of artificial sweeteners (around a dozen are employed in the EU309), does prevent the use of a single marker to be representative for the entire group.
In conclusion, BFIs for drinks are varied depending on the type of drink (alcoholic, fermented, SSB). In most cases, these BFIs have been identified in exploratory analyses and have not been further validated in additional studies or different setups. Moreover, the exploratory nature of these analyses usually means that many aspects of the metabolism or kinetics of the proposed BFIs are either not known or poorly understood. At present, the most promising markers include ethylglucuronide for total alcohol intake in short and long term intake, tartaric acid for wine intake, and 8-prenylnaringenin for beer. In the case of non-alcoholic beverages, trigonelline for coffee and EGC for tea remain promising markers for short-term intake (apart from caffeine in hair). Finally, SSB pose a great challenge for the development of BFIs, although isotopic methods can be used in certain regions to assess their intake.
5 Novel markers-nucleic acids
In addition to plant or animal metabolites present in the food matrix and that are detectable in human tissues, other components including nucleic acids, i.e. either DNA or RNA, may be surviving the gastro-intestinal passage, and may be absorbable.310 These exogenous sequences may later be detectable in e.g. plasma, and due to their unique composition could give an indication of their origin from specific food items. Moreover, these sequences may even play a regulatory function upon different signaling pathways in humans310 and thus could potentially be related to health outcomes.
As early as 2012, a group of researchers managed to detect an exogenous non-coding RNA, specifically a micro RNA (miRNA), in human plasma that is only expressed in vegetables, mir-168a.310 MiRNAs are relatively short sequences of 21–23 nucleotides, are highly conserved311 and though non-coding are involved in gene-expression.311 Although similar studies have also been conducted for animal miRNAs,312 which have also been shown to be absorbable, here we will focus on plant sequences, as these are usually non-existent in animals and thus their dietary origin is easier to prove.312
When surveyed in a cohort study, the expression of mir-168a in plasma was effectively linked to dietary intake of rice.310 Continuing this line of research, another group managed to detect another vegetable miRNA (mir-172) in mice plasma after dietary supplementation of cabbage RNA through gavage.313 This miRNA was not detectable in the rodents before the intervention. In yet a different set-up, researchers demonstrated the survival of synthetic vegetable miRNAs in simulated in vitro digestion. Said study proved that plant miRNAs were able to survive the initial steps of digestion during which the sequences are exposed to enzymes present in the saliva.314 The same observation was also made for different sequences during the gastric phase of digestion in a different study, which is typically more drastic due to the lower pH and longer time exposure and the high sheer forces.315
When studying the survival of exogenous DNA in the GI tract of mice, a recent study observed an approximate half-life of 71 minutes at the gastric phase;316 we could not find any studies reporting the gastric half-life of exogenous RNA although we estimate it to be lower due to the decreased stability of a single nucleotide strand.317 It may be assumed however that RNA may be stabilized in various food matrices given protection of plant cells that are not easily broken down during digestion, such as exosome-like nanoparticles of plant origin (ELN).314,318 There is consensus that part of the miRNAs are encapsulated in ELN.310,314 These nanoparticles, which range in size between 50 and 200 nm, have been shown to provide an excellent delivery method for therapeutic agents into animal tissues.318 Moreover, studies have shown how these particles are at least in part resistant to gastric and intestinal enzymes such as pepsin and pancreatin and can be absorbed by enterocytes,318 such as by endocytosis. Thus, it is suspected that ELNs may be involved in the transport and absorption of dietary miRNAs. However, detailed data regarding survival during in vitro intestinal digestion, arguably the most important step due to the release and absorption of dietary constituents is missing. Likewise, further data regarding their pathway of absorption and stability in human plasma or tissues is missing.
Taken together, these results suggest that miRNAs are capable of surviving gastrointestinal digestion and are absorbed by the gut epithelia. Furthermore, they may also be able to regulate pathways related to health outcomes in vivo. For instance, ELNs play a role in intestinal homeostasis, as they seem to participate in the epithelia renewal in healthy participants and increase the anti-inflammatory properties of intestinal macrophages.318,319 Unfortunately, and although the existing research points towards a promising research field, dietary miRNAs and their survival have been a largely overlooked after a period of initial interest. A good example in this regard is that of the Dietary microRNA Database (DMD).320 This database launched almost a decade ago with the promise to become “an archive database and analytic tool for food borne microRNAs”.320 Nonetheless, at some point between 2019 and 2023 its service was discontinued and the information it entailed (over 5865 dietary miRNAs from up to 16 different species, including both plants and animals) was lost.321
Apart from direct dietary regulation by absorption of miRNAs from the food matrix, specific endogenous miRNA profiles have been associated with different dietary patterns and/or supplementation. Mostly, studies have shown a correlation between polyphenol intake (i.e. resveratrol) and specific miRNA profiles.322 The same type of observations have also been made for vitamin D,322 although reproducibility has been inconsistent. Overall, the association between diet and miRNA signature is complex and is probably a mix between direct absorption of sequences from the food matrix and further indirect measurable changes by regulation of endogenous sequences through unknown pathways. Nonetheless, dietary regulation of miRNAs opens up the possibility of using their signature as a marker of dietary intake to be considered. However, much information is currently missing, such as regarding their variability of absorption depending on the food matrix and processing techniques, kinetic data on ADME such as half-life in plasma, as well as any host factors that could contribute to inter-individual variability of absorption, such as activity of digestion enzymes etc.
Finally, there other types of non-coding RNAs, for which there is no reason to believe they are not absorbed as well. Some of them, such as circular RNAs are more robust to degradation than other types of RNA, while they are also capable of post-transcriptional regulation.323 This could make circular RNAs interesting targets for dietary absorption research as it has already been speculated in regards to bovine milk.324 However, to the best of our knowledge, there are no reports on the bioavailability of circular RNAs. Moreover, genetic material originating in the chloroplast and the mitochondria could also provide a great resource for research given its specificity. In this regard, chloroplast DNA has already been in use for decades through chloroplast DNA metabarcoding. This technique has mainly been used in ecology, although it has also been used in humans to study diet composition from stool samples.325 If these sequences can be absorbed and would be detectable in plasma, the same principles used in stool samples could be extrapolated to plasma, enabling for an easier bio-specimen collection as blood is more frequently collected than stool.
6 Conclusions
Diet plays an important role in the risk of developing and the outcome of certain chronic diseases and metabolic disorders such as MetS. Although dietary assessment through questionnaire-based methods such as FFQ are frequently applied and constitute, in part, validated tools, their application is time-consuming and prone to inaccuracies due to bias. In addition, the relation of dietary intake of certain constituents and their circulating levels, or dietary status, depends on the variable bioavailability that many food constituents exhibit in dependence on the food matrix, food processing conditions, including kitchen preparation procedures, and also host factors, which can influence their bioavailability.87,106,247,326 Thus, more objective measures of dietary intake and status that also relate well to health outcomes have been sought after. In this context, the assessment of dietary intake with novel tools that allow for measurements that are more reliable has become a popular topic for research in recent years.
The use of MS has allowed for a multitude of exploratory analyses that ultimately has led to thousands of metabolites being detected and proposed for their use as biomarkers of dietary intake for different food groups or components. Nevertheless, most of these biomarkers are still poorly validated, and only a few fulfill the criteria for being considered appropriate BFIs, i.e. being selective, specific, usable as both short and long term markers, and being of sufficient validity, reliability, stability over long periods of time, allowing for reproducible studies, and ease of measure so they could be included in routine clinical settings (Table 2). In this regard, the lack of data regarding biomarkers of long-term intake poses an extreme limitation to the use of many proposed BFIs, where FFQ and other traditional methods may still be more adequate for tracking long-term dietary patterns. Moreover, there is a clear preference in the literature to develop BFIs that are perceived as healthy, such as a plant-based diet rich in fruits and vegetables. For this reason, most of the discussed BFIs in this review focused on compounds of vegetal origin, namely polyphenols, carotenoids, and vitamin E, among others. These compounds are widely regarded in research for their specificity to plant-based food groups but also for their health benefits.
Table 2 Proposed biomarkers of food intake (BFIs) for the different food groups or food items when applicable
Food group/item |
Compound |
Sample type |
Specific |
Sensitive |
Short term |
Mid–long term |
Validated |
Stable |
Practicality |
Y: yes, n: no, u: unsure. *: partially validated, MS: mass-spectrometry, Ars: alkylresorcinols, ava: avenanthramides, mh: methylhistidine, dha: docosahexaenoic acid, epa: eicosapentaenoic acid, fv: fruits and vegetables; tmao: trimethylamine-n-oxide, ompd: optical macula pigment density. The meaning of the columns is based upon the criteria listed in Table 1. Adapted from ref. 35. Stable when stabilized (i.e. by metaphosphoric acid). |
Total FV |
Vitamin C |
Plasma urine |
Y |
Y* |
Y |
N |
Y |
N* |
N |
Total FV |
Vitamin E |
Adipose tissue |
N |
U |
U |
Y |
N |
Y |
Y |
Total FV |
Carotenoids |
Plasma, skin |
Y |
Y* |
Y |
U |
Y |
U |
N (HPLC required) |
Citrus fruits |
β-Cryptoxantin |
Plasma |
Y |
U |
U |
U |
U |
U |
N (HPLC required) |
Orange vegetables |
α–β-Carotenes |
Plasma |
Y |
U |
U |
U |
U |
U |
N (HPLC required) |
Tomato |
Lycopene |
Plasma |
Y |
U |
U |
U |
U |
U |
Y (spectrophotometric) |
Total FV/cerals |
Lutein zeaxanthin |
Macula (OMPD) |
Y |
Y |
U |
Y* |
Y |
U |
N (HPLC required) |
Total FV |
Total phenolics |
Plasma |
Y |
U |
Y |
N |
U |
U |
Y (spectrophotometric) |
Total FV |
Flavonoids |
Plasma |
Y |
Y |
Y |
N |
Y |
U |
Y (spectrophotometric) |
Berries |
Anthocyanins |
Urine |
Y |
U |
Y* |
U |
N |
U |
Y (spectrophotometric) |
Citrics |
Narigenin |
Urine |
Y |
N |
Y |
N |
Y |
Y |
N (HPLC required) |
Citrics |
Hesperetin |
Urine |
Y |
Y |
Y |
N |
Y |
Y |
N (HPLC required) |
Broccoli |
Sulfuraphane-NAC |
Urine |
Y* |
U |
Y |
N |
U |
Y |
N (HPLC required) |
Avocado |
Perseitola |
Urine |
Y |
Y* |
Y |
U |
U |
U |
N (HPLC required) |
Avocado |
Manoheptulosea |
Urine |
Y |
Y* |
Y |
U |
U |
U |
N (HPLC required) |
Soybean/pulses |
Genistein |
Plasma urine |
Y |
Y |
Y |
Y* |
Y |
Y |
N (HPLC required) |
Soybean/pulses |
Daidzein |
Plasma urine |
Y |
Y |
Y |
Y* |
Y |
Y |
N (HPLC required) |
Wheat/rye/barley |
Odd Ars |
Plasma urine adipose tissue |
Y |
Y |
Y |
Y |
N |
U |
N (HPLC required) |
Quinoa |
Even Ars |
Plasma urine adipose tissue |
Y |
U |
Y |
Y |
N |
U |
N (HPLC required) |
Oat |
AVA |
Urine |
Y |
U |
Y |
N |
N |
U |
N (HPLC required) |
Wheat/rye |
Benzoxazinoids |
Plasma urine |
U |
U |
Y |
N |
N |
U |
Y (spectrophotometric) |
Nuts |
Urolithins |
Plasma urine |
N |
U |
Y |
U |
N |
U |
Y (spectrophotometric) |
Olive/walnuts |
α-Linoleic acid |
Plasma |
N |
U |
Y |
U |
N |
U |
N (HPLC required) |
Olive/walnuts |
Oleic acid |
Plasma |
N |
U |
Y |
U |
N |
U |
N (HPLC required) |
Olive oil |
Hydroxytyrosol |
Urine |
N |
Y* |
Y |
N |
N |
U |
N (HPLC required) |
Total meat |
3-MH |
Urine |
Y |
Y |
Y |
U |
Y |
U |
N (MS required) |
Fish and seafood |
DHA |
Plasma |
Y |
Y |
Y |
U |
Y |
Y |
N (GC required) |
Fish and seafood |
EPA |
Plasma |
Y |
Y |
Y |
U |
Y |
Y |
N (GC required) |
Fish and seafood |
TMAO |
Plasma |
N |
Y |
Y |
U |
Y |
U |
|
Total dairy |
C15:0 |
Plasma |
N |
N |
Y |
U |
U |
U |
N (GC required) |
Total dairy |
C17:0 |
Plasma |
N |
N |
Y |
U |
U |
U |
N (GC required) |
Total dairy |
Sphingomyelins |
Plasma |
N |
N |
Y |
U |
N |
U |
Y (spectrophotometric) |
Cheese |
Acylglycines |
Urine |
Y |
U |
U |
U |
N |
U |
N (GC-MS required) |
Total alcohol |
Ethyl glucuronide |
Plasma urine hair |
Y |
Y |
Y |
Y |
Y |
Y |
N (MS required) |
Wine |
Resveratrol |
Plasma |
Y |
Y |
Y |
N |
N |
U |
N (HPLC required) |
Wine |
Hydroxytyrosol |
Urine |
N |
U |
Y |
N |
N |
U |
N (HPLC required) |
Wine/grapes |
Tartaric acid |
Urine |
Y |
Y |
Y |
N |
N |
Y |
Y (spectrophotometry) |
Beer |
Isoxanthumol |
Plasma |
Y |
N |
Y |
N |
N |
U |
N (HPLC required) |
Beer |
8-Prenylnarigenin |
Plasma |
Y |
N |
Y |
N |
N |
U |
N (HPLC required) |
Beer |
Iso-alpha-acids |
Plasma |
Y |
U |
U |
U |
N |
N |
N (HPLC required) |
Cofee/pulses |
Trigonelline |
Plasma urine |
Y |
Y |
Y |
N |
N |
Y |
N (HPLC required) |
Cofee |
2-Furoglycine |
Plasma urine |
Y |
U |
Y |
N |
N |
U |
N (GC-MS required) |
Tea |
Epicatechin gallate |
Urine |
Y |
Y |
Y |
N |
Y |
U |
N (HPLC required) |
Sugar sweetened beverages (region dependent) |
C13/C12 ratio |
Plasma urine |
N |
Y |
Y |
U |
N |
Y |
N (MS required) |
Overall, BFIs such as vitamin C, total carotenoids, or certain polyphenols (i.e., flavonols) constitute good markers for fruit and vegetable intake, although none of them meets all the criteria for an ideal biomarker of food intake (Table 2). Therefore, most BFIs should preferably be used in combination. Other food groups contain potential BFIs that, although very specific only for certain food items, are very promising. This is the case, for instance, for genistein and daidzein for soybean intake, alkylresorcinols for some whole grains, DHA and EPA for fish and seafood consumption. A general limitation is that most nutrients/non-nutrients are not stored by the body, therefore only constituting short-term markers. For this reason, at present, the most accepted and suitable BFIs are usually limited to specific food items rather than whole food group intakes.
On the other hand, BFI related to poor health outcomes, such as processed meats, sugared sweetened beverages, and processed foods, are still elusive, with some exceptions. For example, alcohol intake, which can be assessed by ethyl glucuronide measurements through MS in, e.g., hair samples. Other methods still have many limitations but offer some promise, such as the C13/C12 ratio in sugar sweetened beverages, though are only suitable in areas where sugars are produced from C-4 plants. Therefore, although the use of BFI to assess dietary intake seems promising, complementation with FFQ or multiple 24 h recalls is still required in order to obtain a more global overview of dietary patterns.
As outlined, health beneficial plant compounds could play a twofold role as biomarkers. On the one hand, they can be used to assess with a certain degree of confidence dietary intake. On the other hand, many of these constituents have also biological activity, i.e., unique anti-inflammatory, antioxidant, and regulatory properties that can modulate components of cardiometabolic health such as blood pressure or insulin resistance. In this regard, the introduction of such biomarkers in the clinical practice may prove extremely useful as a dual active tool, allowing on the one hand to verify dietary intake recommendations without relying solely on more classic and sometimes imprecise tools (e.g. FFQs for dietary intake). On the other hand, they could allow understanding the status of cardiometabolic health and function as early markers before more manifest endpoints (e.g. ATPIII criteria for MetS) can be reliably diagnosed.
Finally, although biomarker research has focused on metabolomics for the past decade, other omics technologies such as transcriptomics, and the use of miRNAs for dietary assessment have received increasing attention in the past years. In this regard, the discovery of plant miRNAs in plasma over a decade ago sparked interest in the scientific community as an example of inter-kingdom regulation.310 Although the concentrations of such plant miRNAs may not be high enough to exert significant bioactive regulation, they may still prove useful as tools for dietary assessment. In the same regard, diet is known to modulate the gut microbiota composition and diversity.327 Nonetheless, many unknowns in the gut microbiota composition exist that must be understood (such as host influence on gut microbiota, and the role of specific bacteria in disease risks or progression) before species diversity or similar can be used as a marker of diet.328 Another novel area is the relationship between food items and epigenetics. For instance, oxidative stress has been reported to influence epigenetic drift, and early dietary intervention in life could aid in reducing diseases occurring later in life, including cancer329,330 and cardiometabolic diseases.331 Thus, dietary intervention with trackable food components that target oxidative stress may not only benefit individuals at risk of MetS, but also people that follow healthy diets thought prolonged periods.
In summary, the development of biomarkers of food intake from different sources may prove a useful tool that can provide further insights and, in turn, perhaps constitute a better marker of dietary intake than classical/questionnaire based dietary assessment methods such as FFQ. However, at present, many biomarkers lack the specificity that would ideally make them a standalone tool but rather need the support of other BFIs. Overall, the inclusion of biomarkers from varied sources and their integration into multi-modal biomarkers (e.g., combining traditional markers with metabolomics and transcriptomics) may pave the way to the development of novel dietary and cardiometabolic assessment strategies.
Author contributions
Conceptualization: TB and MC, writing-original draft: MC, writing review and editing: TB, FV and YD.
Conflicts of interest
The authors declare no conflict of interests.
Acknowledgements
The authors are grateful for the support of the Luxembourg National Research Fund (PRIDE21/16749720/NEXTIMMUNE2).
References
- M. G. Saklayen, The Global Epidemic of the Metabolic Syndrome, Curr. Hypertens. Rep., 2018, 20, 12 CrossRef PubMed.
- S. L. Samson and A. J. Garber, Metabolic syndrome, Endocrinol. Metab. Clin. North Am., 2014, 43, 1–23 CrossRef PubMed.
- D. M. Boudreau, D. C. Malone, M. A. Raebel, P. A. Fishman, G. A. Nichols, A. C. Feldstein, A. N. Boscoe, R. H. Ben-Joseph, D. J. Magid and L. J. Okamoto, Health care utilization and costs by metabolic syndrome risk factors, Metab. Syndr. Relat. Disord., 2009, 7, 305–314 CrossRef CAS.
- A. Esmaillzadeh, M. Kimiagar, Y. Mehrabi, L. Azadbakht, F. B. Hu and W. C. Willett, Fruit and vegetable intakes, C-reactive protein, and the metabolic syndrome, Am. J. Clin. Nutr., 2006, 84, 1489–1497 CrossRef CAS PubMed.
- M. Lee, M. Lim and J. Kim, Fruit and vegetable consumption and the metabolic syndrome: a systematic review and dose–response meta-analysis, Br. J. Nutr., 2019, 122, 723–733 CrossRef CAS PubMed.
- Y. Zhang and D. Z. Zhang, Associations of vegetable and fruit consumption with metabolic syndrome. A meta-analysis of observational studies, Public Health Nutr., 2018, 21, 1693–1703 CrossRef PubMed.
- Y. Tian, L. Su, J. Wang, X. Duan and X. Jiang, Fruit and vegetable consumption and risk of the metabolic syndrome: a meta-analysis, Public Health Nutr., 2018, 21, 756–765 CrossRef.
- A. Alonso, C. de la Fuente, A. M. Martin-Arnau, J. de Irala, J. A. Martinez and M. A. Martinez-Gonzalez, Fruit and vegetable consumption is inversely associated with blood pressure in a Mediterranean population with a high vegetable-fat intake: the Seguimiento Universidad de Navarra (SUN) Study, Br. J. Nutr., 2004, 92, 311–319 CrossRef CAS PubMed.
- A. Agudo, L. Cabrera, P. Amiano, E. Ardanaz, A. Barricarte, T. Berenguer, M. D. Chirlaque, M. Dorronsoro, P. Jakszyn, N. Larranaga, C. Martinez, C. Navarro, J. R. Quiros, M. J. Sanchez, M. J. Tormo and C. A. Gonzalez, Fruit and vegetable intakes, dietary antioxidant nutrients, and total mortality in Spanish adults: findings from the Spanish cohort of the European Prospective Investigation into Cancer and Nutrition (EPIC-Spain), Am. J. Clin. Nutr., 2007, 85, 1634–1642 CrossRef CAS PubMed.
- K. Kawabata, Y. Yoshioka and J. Terao, Role of Intestinal Microbiota in the Bioavailability and Physiological Functions of Dietary Polyphenols, Molecules, 2019, 24, 370 CrossRef PubMed.
- T. Bohn, A. R. de Lera, J.-F. Landrier and R. Rühl, Carotenoid metabolites, their tissue and blood concentrations in humans and further bioactivity via retinoid receptor-mediated signalling, Nutr. Res. Rev., 2022, 1–14, DOI:10.1017/S095442242200021X.
- H. Guo, J. Ding, J. Liang and Y. Zhang, Associations of Whole Grain and Refined Grain Consumption With Metabolic Syndrome. A Meta-Analysis of Observational Studies, Front. Nutr., 2021, 8, 695620 CrossRef PubMed.
- Y. Kim and Y. Je, Meat Consumption and Risk of Metabolic Syndrome: Results from the Korean Population and a Meta-Analysis of Observational Studies, Nutrients, 2018, 10, 390 CrossRef PubMed.
- S. R. Kim, K. Kim, S. A. Lee, S. O. Kwon, J. K. Lee, N. Keum and S. M. Park, Effect of Red, Processed, and White Meat Consumption on the Risk of Gastric Cancer: An Overall and Dose(-)Response Meta-Analysis, Nutrients, 2019, 11, 826 CrossRef.
- C. Torris, M. Molin and M. S. Cvancarova, Lean fish consumption is associated with lower risk of metabolic syndrome: a Norwegian cross sectional study, BMC Public Health, 2016, 16, 347 CrossRef CAS PubMed.
- J. V. Woodside, J. Draper, A. Lloyd and M. C. Mckinley, Use of biomarkers to assess fruit and vegetable intake, Proc. Nutr. Soc., 2017, 76, 308–315 CrossRef CAS PubMed.
- A. Naska, A. Lagiou and P. Lagiou, Dietary assessment methods in epidemiological
research: current state of the art and future prospects, F1000Research, 2017, 6, 926 Search PubMed.
- European Food Safety Authority (EFSA), General principles for the collection of national food consumption data in the view of a pan-European dietary survey, EFSA J., 2009, 7(12), 1435 CrossRef.
- G. Praticò, Q. Gao, A. Scalbert, G. Vergères, M. Kolehmainen, C. Manach, L. Brennan, S. H. Pedapati, L. A. Afman, D. S. Wishart, R. Vázquez-Fresno, C. Andres-Lacueva, M. Garcia-Aloy, H. Verhagen, E. J. M. Feskens and L. O. Dragsted, Guidelines for Biomarker of Food Intake Reviews (BFIRev): how to conduct an extensive literature search for biomarker of food intake discovery, Genes Nutr., 2018, 13, 3 CrossRef PubMed.
- L. O. Dragsted, Q. Gao, A. Scalbert, G. Vergères, M. Kolehmainen, C. Manach, L. Brennan, L. A. Afman, D. S. Wishart, C. A. Lacueva, M. Garcia-Aloy, H. Verhagen, E. J. M. Feskens and G. Praticò, Validation of biomarkers of food intake—critical assessment of candidate biomarkers, Genes Nutr., 2018, 13, 14 CrossRef CAS PubMed.
- J.-S. Shim, K. Oh and H. C. Kim, Dietary assessment methods in epidemiologic studies, Epidemiol. Health, 2014, 36, e2014009 CrossRef PubMed.
- C. Perez Rodrigo, J. Aranceta, G. Salvador and G. Varela-Moreiras, Food frequency questionnaires, Nutr. Hosp., 2015, 31(Suppl 3), 49–56 Search PubMed.
- S. McNutt, T. P. Zimmerman and S. G. Hull, Development of food composition databases for food frequency questionnaires (FFQ), J. Food Compos. Anal., 2008, 21, S20–S26 CrossRef.
- M. Jenab, N. Slimani, M. Bictash, P. Ferrari and S. A. Bingham, Biomarkers in nutritional epidemiology: applications, needs and new horizons, Hum. Genet., 2009, 125, 507–525 CrossRef PubMed.
- S. K. Masenga, L. S. Kabwe, M. Chakulya and A. Kirabo, Mechanisms of Oxidative Stress in Metabolic Syndrome, Int. J. Mol. Sci., 2023, 24, 7898 CrossRef CAS.
- T. Bohn, H. Samouda and A. a. Alkerwi, in Diet, Inflammation, and Health, ed. J. R. Hébert and L. J. Hofseth, Academic Press, 2022, pp. 261–366. DOI:10.1016/B978-0-12-822130-3.00014-4.
- N. Hidalgo-Liberona, T. Merono, R. Zamora-Ros, M. Rabassa, R. Semba, T. Tanaka, S. Bandinelli, L. Ferrucci, C. Andres-Lacueva and A. Cherubini, Adherence to the Mediterranean diet assessed by a novel dietary biomarker score and mortality in older adults: the InCHIANTI cohort study, BMC Med., 2021, 19, 280 CrossRef CAS PubMed.
- K. Strimbu and J. A. Tavel, What are biomarkers?, Curr. Opin. HIV AIDS, 2010, 5, 463–466 CrossRef PubMed.
- R. Landberg, P. Karra, R. Hoobler, E. Loftfield, I. Huybrechts, J. I. Rattner, S. Noerman, L. Claeys, V. Neveu, N. H. Vidkjaer, O. Savolainen, M. C. Playdon and A. Scalbert, Dietary biomarkers-an update on their validity and applicability in epidemiological studies, Nutr. Rev., 2023 DOI:10.1093/nutrit/nuad119.
- R. Landberg, K. Hanhineva, K. Tuohy, M. Garcia-Aloy, I. Biskup, R. Llorach, X. Yin, L. Brennan and M. Kolehmainen, Biomarkers of cereal food intake, Genes Nutr., 2019, 14, 28 CrossRef PubMed.
- T. Rafiq, S. M. Azab, K. K. Teo, L. Thabane, S. S. Anand, K. M. Morrison, R. J. de Souza and P. Britz-McKibbin, Nutritional Metabolomics and the Classification of Dietary Biomarker Candidates: A Critical Review, Adv. Nutr., 2021, 12, 2333–2357 CrossRef PubMed.
- A. C. Carita, B. Fonseca-Santos, J. D. Shultz, B. Michniak-Kohn, M. Chorilli and G. R. Leonardi, Vitamin C: One compound, several uses. Advances for delivery, efficiency and stability, Nanomedicine, 2020, 24, 102117 CrossRef CAS.
- T. C. Chen, F. Chimeh, Z. Lu, J. Mathieu, K. S. Person, A. Zhang, N. Kohn, S. Martinello, R. Berkowitz and M. F. Holick, Factors that influence the cutaneous synthesis and dietary sources of vitamin D, Arch. Biochem. Biophys., 2007, 460, 213–217 CrossRef CAS PubMed.
- D. Corella and J. M. Ordovas, Biomarkers: background, classification and guidelines for applications in nutritional epidemiology, Nutr. Hosp., 2015, 31(Suppl 3), 177–188 Search PubMed.
- C. Cuparencu, G. Praticó, L. Y. Hemeryck, P. S. C. Sri Harsha, S. Noerman, C. Rombouts, M. Xi, L. Vanhaecke, K. Hanhineva, L. Brennan and L. O. Dragsted, Biomarkers of meat and seafood intake: an extensive literature review, Genes Nutr., 2019, 14, 35 CrossRef CAS.
- K. Okuda, Discovery of vitamin B12in the liver and its absorption factor in the stomach: A historical review, J. Gastroenterol. Hepatol., 2002, 14, 301–308 CrossRef.
- M. Barbagallo, M. Belvedere and L. J. Dominguez, Magnesium homeostasis and aging, Magnesium Res., 2009, 22, 235–246 CAS.
- N. Matikainen, T. Pekkarinen, E. M. Ryhänen and C. Schalin-Jäntti, Physiology of Calcium Homeostasis: An Overview, Endocrinol. Metab. Clin. North Am., 2021, 50, 575–590 CrossRef PubMed.
- S. Gupta, A. Venkatesh, S. Ray and S. Srivastava, Challenges and prospects for biomarker research: a current perspective from the developing world, Biochim. Biophys. Acta, 2014, 1844, 899–908 CrossRef CAS PubMed.
- P. S. C. Sri Harsha, R. A. Wahab, M. Garcia-Aloy, F. Madrid-Gambin, S. Estruel-Amades, B. Watzl, C. Andres-Lacueva and L. Brennan, Biomarkers of legume intake in human intervention and observational studies: a systematic review, Genes Nutr., 2018, 13, 25 CrossRef PubMed.
- T. Bohn, C. Desmarchelier, L. O. Dragsted, C. S. Nielsen, W. Stahl, R. Ruhl, J. Keijer and P. Borel, Host-related factors explaining interindividual variability of carotenoid bioavailability and tissue concentrations in humans, Mol. Nutr. Food Res., 2017, 61, 1600685 CrossRef PubMed.
- P. Borel, C. Desmarchelier, M. Nowicki and R. Bott, A combination of single-nucleotide polymorphisms is associated with interindividual variability in dietary beta-carotene bioavailability in healthy men, J. Nutr., 2015, 145, 1740–1747 CrossRef CAS PubMed.
- G. R. Healey, R. Murphy, L. Brough, C. A. Butts and J. Coad, Interindividual variability in gut microbiota and host response to dietary interventions, Nutr. Rev., 2017, 75, 1059–1080 CrossRef.
- A. Niforou, V. Konstantinidou and A. Naska, Genetic Variants Shaping Inter-individual Differences in Response to Dietary Intakes-A Narrative Review of the Case of Vitamins, Front. Nutr., 2020, 7, 558598 CrossRef PubMed.
- J. Pullar, S. Bayer and A. Carr, Appropriate Handling, Processing and Analysis of Blood Samples Is Essential to Avoid Oxidation of Vitamin C to Dehydroascorbic Acid, Antioxidants, 2018, 7, 29 CrossRef PubMed.
- N. Dakappagari, H. Zhang, L. Stephen, L. Amaravadi and M. U. Khan, Recommendations for clinical biomarker specimen preservation and stability assessments, Bioanalysis, 2017, 9, 643–653 CrossRef CAS PubMed.
- F. W. Fidler and J. Wilcox, Reproducibility of Scientific Results, https://plato.stanford.edu/archives/sum2021/entries/scientific-reproducibility Search PubMed.
- J. P. I. A. H. D. f. a. H. Life, Results of FOODBALL and MIRDIET, https://healthydietforhealthylife.eu/index.php/news/497-results-of-foodball-and-mirdiet.
- J. W. Lampe, Isoflavonoid and lignan phytoestrogens as dietary biomarkers, J. Nutr., 2003, 133(Suppl 3), 956S–964S CrossRef CAS PubMed.
- M. Trius-Soler, G. Praticò, G. Gürdeniz, M. Garcia-Aloy, R. Canali, N. Fausta, E. M. Brouwer-Brolsma, C. Andrés-Lacueva and L. O. Dragsted, Biomarkers of moderate alcohol intake and alcoholic beverages: a systematic literature review, Genes Nutr., 2023, 18, 19 Search PubMed.
- M. Alicka and K. Marycz, The Effect of Chronic Inflammation and Oxidative and Endoplasmic Reticulum Stress in the Course of Metabolic Syndrome and Its Therapy, Stem Cells Int., 2018, 2018, 1–13 CrossRef PubMed.
- G. S. Hotamisligil, Inflammation and metabolic disorders, Nature, 2006, 444, 860–867 CrossRef CAS PubMed.
- H. Xu, X. Li, H. Adams, K. Kubena and S. Guo, Etiology of Metabolic Syndrome and Dietary Intervention, Int. J. Mol. Sci., 2018, 20, 128 CrossRef PubMed.
- R. Monteiro and I. Azevedo, Chronic Inflammation in Obesity and the Metabolic Syndrome, Mediators Inflammation, 2010, 2010, 1–10 CrossRef PubMed.
- J. E. Hall, A. J. Mouton, A. A. da Silva, A. C. M. Omoto, Z. Wang, X. Li and J. M. do Carmo, Obesity, kidney dysfunction, and inflammation: interactions in hypertension, Cardiovasc. Res., 2021, 117, 1859–1876 CrossRef CAS.
- G. Fahed, L. Aoun, M. Bou Zerdan, S. Allam, M. Bou Zerdan, Y. Bouferraa and H. I. Assi, Metabolic Syndrome: Updates on Pathophysiology and Management in 2021, Int. J. Mol. Sci., 2022, 23, 786 CrossRef CAS PubMed.
- J. C. Fernandez-Garcia, F. Cardona and F. J. Tinahones, Inflammation, oxidative stress and metabolic syndrome: dietary modulation, Curr. Vasc. Pharmacol., 2013, 11, 906–919 CrossRef CAS PubMed.
- M. F. Troncoso, J. Ortiz-Quintero, V. Garrido-Moreno, F. Sanhueza-Olivares, A. Guerrero-Moncayo, M. Chiong, P. F. Castro, L. Garcia, L. Gabrielli, R. Corbalan, L. Garrido-Olivares and S. Lavandero, VCAM-1 as a predictor biomarker in cardiovascular disease, Biochim. Biophys. Acta, Mol. Basis Dis., 2021, 1867, 166170 CrossRef CAS PubMed.
- V. Singh, R. Kaur, P. Kumari, C. Pasricha and R. Singh, ICAM-1 and VCAM-1: Gatekeepers in various inflammatory and cardiovascular disorders, Clin. Chim. Acta, 2023, 548, 117487 CrossRef CAS PubMed.
- A. Chait and L. J. den Hartigh, Adipose Tissue Distribution, Inflammation and Its Metabolic Consequences, Including Diabetes and Cardiovascular Disease, Front. Cardiovasc. Med., 2020, 7, 22 CrossRef CAS PubMed.
- F. P. De Heredia, S. Gómez-Martínez and A. Marcos, Obesity, inflammation and the immune system, Proc. Nutr. Soc., 2012, 71, 332–338 CrossRef CAS.
- M. Lepretti, S. Martucciello, M. Burgos Aceves, R. Putti and L. Lionetti, Omega-3 Fatty Acids and Insulin Resistance: Focus on the Regulation of Mitochondria and Endoplasmic Reticulum Stress, Nutrients, 2018, 10, 350 CrossRef PubMed.
- T. Bohn, M. L. Bonet, P. Borel, J. Keijer, J.-F. Landrier, I. Milisav, J. Ribot, P. Riso, B. Winklhofer-Roob, Y. Sharoni, J. Corte-Real, Y. Van Helden, M. R. Loizzo, B. Poljšak, M. Porrini, J. Roob, P. Trebše, R. Tundis, A. Wawrzyniak, R. Rühl and J. Dulińska-Litewka, Mechanistic aspects of carotenoid health benefits – where are we now?, Nutr. Res. Rev., 2021, 34, 276–302 CrossRef CAS PubMed.
- D. J. Jenkins, C. W. Kendall, A. Marchie, D. A. Faulkner, J. M. Wong, R. de Souza, A. Emam, T. L. Parker, E. Vidgen, K. G. Lapsley, E. A. Trautwein, R. G. Josse, L. A. Leiter and P. W. Connelly, Effects of a dietary portfolio of cholesterol-lowering foods vs lovastatin on serum lipids and C-reactive protein, J. Am. Med. Assoc., 2003, 290, 502–510 CrossRef CAS.
- W. P. Luo, Y. J. Fang, M. S. Lu, X. Zhong, Y. M. Chen and C. X. Zhang, High consumption of vegetable and fruit colour groups is inversely associated with the risk of colorectal cancer: a case-control study, Br. J. Nutr., 2015, 113, 1129–1138 CrossRef CAS PubMed.
- J. V. Woodside, I. S. Young and M. C. Mckinley, Fruits and vegetables: measuring intake and encouraging increased consumption, Proc. Nutr. Soc., 2013, 72, 236–245 CrossRef PubMed.
- C. Nicolle, A. Carnat, D. Fraisse, J. L. Lamaison, E. Rock, H. Michel, P. Amouroux and C. Remesy, Characterisation and variation of antioxidant micronutrients in lettuce (Lactuca sativa folium), J. Sci. Food Agric., 2004, 84, 2061–2069 CrossRef CAS.
- J. Wang, C.-L. Law, A. Mujumdar and H.-W. Xiao, The degradation mechanism and kinetics of vitamin C in fruits and vegetables during thermal processing, in Fundamentals & applications (Part III), ed. P.K Nema, B.P Kaur and A.S Mujumdar, 2017, pp. 227–253 Search PubMed.
- A. Evers, L. O'brien and A. Blakeney, Cereal structure and composition, Aust. J. Agric. Res., 1999, 50, 629–650 CrossRef.
- J. V. Woodside, J. Draper, A. Lloyd and M. C. McKinley, Use of biomarkers to assess fruit and vegetable intake, Proc. Nutr. Soc., 2017, 76, 308–315 CrossRef CAS.
- E. M. Brouwer-Brolsma, B. Brandl, M. E. C. Buso, T. Skurk and C. Manach, Food intake biomarkers for green leafy vegetables, bulb vegetables, and stem vegetables: a review, Genes Nutr., 2020, 15, 7 CrossRef PubMed.
- H. Aysun, An overview of ascorbic acid biochemistry, Ankara Univ. Eczacilik Fak. Derg., 2009, 38, 233–255 CrossRef.
- F. E. Pehlivan, in Vitamin C, InTech, 2017, ch. 2. DOI:10.5772/intechopen.69660.
- H. Guo, J. Ding, Q. Liu, Y. Li, J. Liang and Y. Zhang, Vitamin C and Metabolic Syndrome: A Meta-Analysis of Observational Studies, Front. Nutr., 2021, 8, 728880 CrossRef.
- M. B. Morelli, J. Gambardella, V. Castellanos, V. Trimarco and G. Santulli, Vitamin C and Cardiovascular Disease: An Update, Antioxidants, 2020, 9, 1227 CrossRef CAS PubMed.
- Y. Guan, P. Dai and H. Wang, Effects of vitamin C supplementation on essential hypertension: A systematic review and meta-analysis, Medicine, 2020, 99, e19274 CrossRef PubMed.
- S. Padayatty and M. Levine, Vitamin C: the known and the unknown and Goldilocks, Oral Dis., 2016, 22, 463–493 CrossRef CAS.
- M. Dehghan, N. Akhtar-Danesh, C. R. Mcmillan and L. Thabane, Is plasma vitamin C an appropriate biomarker of vitamin C intake? A systematic review and meta-analysis, Nutr. J., 2007, 6, 41 CrossRef PubMed.
- M. Doseděl, E. Jirkovský, K. Macáková, L. Krčmová, L. Javorská, J. Pourová, L. Mercolini, F. Remião, L. Nováková and P. Mladěnka, Vitamin C—Sources, Physiological Role, Kinetics, Deficiency, Use, Toxicity, and Determination, Nutrients, 2021, 13, 615 CrossRef.
- C. Lassale, K. Castetbon, F. Laporte, V. Deschamps, M. Vernay, G. M. Camilleri, P. Faure, S. Hercberg, P. Galan and E. Kesse-Guyot, Correlations between Fruit, Vegetables, Fish, Vitamins, and Fatty Acids Estimated by Web-Based Nonconsecutive Dietary Records and Respective Biomarkers of Nutritional Status, J. Acad. Nutr. Diet., 2016, 116, 427–438 CrossRef PubMed.
- R. P. Bogers, P. C. Dagnelie, K. R. Westerterp, A. D. Kester, J. D. van Klaveren, A. Bast and P. A. van den Brandt, Using a correction factor to correct for overreporting in a food-frequency questionnaire does not improve biomarker-assessed validity of estimates for fruit and vegetable consumption, J. Nutr., 2003, 133, 1213–1219 CrossRef CAS PubMed.
- G. G. Kuhnle, Nutritional biomarkers for objective dietary assessment, J. Sci. Food Agric., 2012, 92, 1145–1149 CrossRef CAS PubMed.
- M. Garg, A. Sharma, S. Vats, V. Tiwari, A. Kumari, V. Mishra and M. Krishania, Vitamins in Cereals: A Critical Review of Content, Health Effects, Processing Losses, Bioaccessibility, Fortification, and Biofortification Strategies for Their Improvement, Front. Nutr., 2021, 8, 586815 CrossRef PubMed.
- K. M. Phillips, M. T. Tarragó-Trani, S. E. Gebhardt, J. Exler, K. Y. Patterson, D. B. Haytowitz, P. R. Pehrsson and J. M. Holden, Stability of vitamin C in frozen raw fruit and vegetable homogenates, J. Food Compos. Anal., 2010, 23, 253–259 CrossRef CAS.
- E. M. Yahia, J. de Jesús Ornelas-Paz, T. Emanuelli, E. Jacob-Lopes, L. Q. Zepka and B. Cervantes-Paz, in Fruit and Vegetable Phytochemicals, 2017, pp. 285–346. DOI:10.1002/9781119158042.ch15.
- T. Bohn, Carotenoids and Markers of Oxidative Stress in Human Observational Studies and Intervention Trials: Implications for Chronic Diseases, Antioxidants, 2019, 8, 179 CrossRef CAS PubMed.
- V. Bohm, G. Lietz, B. Olmedilla-Alonso, D. Phelan, E. Reboul, D. Banati, P. Borel, J. Corte-Real, A. R. de Lera, C. Desmarchelier, J. Dulinska-Litewka, J. F. Landrier, I. Milisav, J. Nolan, M. Porrini, P. Riso, J. M. Roob, E. Valanou, A. Wawrzyniak, B. M. Winklhofer-Roob, R. Ruhl and T. Bohn, From carotenoid intake to carotenoid blood and tissue concentrations - implications for dietary intake recommendations, Nutr. Rev., 2021, 79, 544–573 CrossRef PubMed.
- A. G. Maria, R. Graziano and D. O. Nicolantonio, Carotenoids: potential allies of cardiovascular health?, Food Nutr. Res., 2015, 59, 26762 CrossRef PubMed.
- M. A. Beydoun, X. Chen, K. Jha, H. A. Beydoun, A. B. Zonderman and J. A. Canas, Carotenoids, vitamin A, and their association with the metabolic syndrome: a systematic review and meta-analysis, Nutr. Rev., 2018, 77, 32–45 CrossRef PubMed.
- N. Di Pietro, P. D. Tomo and A. Pandolfi, Carotenoids in cardiovascular disease prevention, JSM Atheroscler, 2016, 1, 1002 Search PubMed.
- H. M. Cheng, G. Koutsidis, J. K. Lodge, A. Ashor, M. Siervo and J. Lara, Tomato and lycopene supplementation and cardiovascular risk factors: A systematic review and meta-analysis, Atherosclerosis, 2017, 257, 100–108 CrossRef CAS PubMed.
- M. Ucci, P. D. Tomo, F. Tritschler, V. G. P. Cordone, P. Lanuti, G. Bologna, S. D. Silvestre, N. D. Pietro, C. Pipino, D. Mandatori, G. Formoso and A. Pandolfi, Anti-inflammatory Role of Carotenoids in Endothelial Cells Derived from Umbilical Cord of Women Affected by Gestational Diabetes Mellitus, Oxid. Med. Cell. Longevity, 2019, 2019, 8184656 Search PubMed.
- I. Sluijs, E. Cadier, J. W. Beulens, A. D. van der, A. M. Spijkerman and Y. T. van der Schouw, Dietary intake of carotenoids and risk of type 2 diabetes, Nutr., Metab. Cardiovasc. Dis., 2015, 25, 376–381 CrossRef CAS PubMed.
- A. Roohbakhsh, G. Karimi and M. Iranshahi, Carotenoids in the treatment of diabetes mellitus and its complications: A mechanistic review, Biomed. Pharmacother., 2017, 91, 31–42 CrossRef CAS PubMed.
- L. Y.-L. Leung, S. M.-N. Chan, H.-L. Tam and E. S.-W. Wong, Astaxanthin Influence on Health Outcomes of Adults at Risk of Metabolic Syndrome: A Systematic Review and Meta-Analysis, Nutrients, 2022, 14, 2050 CrossRef CAS PubMed.
- E. T. Leermakers, S. K. Darweesh, C. P. Baena, E. M. Moreira, D. Melo van Lent, M. J. Tielemans, T. Muka, A. Vitezova, R. Chowdhury, W. M. Bramer, J. C. Kiefte-de Jong, J. F. Felix and O. H. Franco, The effects of lutein on cardiometabolic health across the life course: a systematic review and meta-analysis, Am. J. Clin. Nutr., 2016, 103, 481–494 CrossRef CAS PubMed.
- K. Linnewiel-Hermoni, Y. Motro, Y. Miller, J. Levy and Y. Sharoni, Carotenoid derivatives inhibit nuclear factor kappa B activity in bone and cancer cells by targeting key thiol groups, Free Radicals Biol. Med., 2014, 75, 105–120 CrossRef CAS PubMed.
- S. Nishikawa, M. Hosokawa and K. Miyashita, Fucoxanthin promotes translocation and induction of glucose transporter 4 in skeletal muscles of diabetic/obese KK-A(y) mice, Phytomedicine, 2012, 19, 389–394 CrossRef CAS PubMed.
- M. L. Bonet, J. Ribot, S. Galmes, F. Serra and A. Palou, Carotenoids and carotenoid conversion products in adipose tissue biology and obesity: Pre-clinical and human studies, Biochim. Biophys. Acta, Mol. Cell Biol. Lipids, 2020, 1865, 158676 CrossRef CAS PubMed.
- A. Leonardini, L. Laviola, S. Perrini, A. Natalicchio and F. Giorgino, Cross-Talk between PPARgamma and Insulin Signaling and Modulation of Insulin Sensitivity, PPAR Res., 2009, 2009, 818945 CrossRef PubMed.
- M. Le Goff, E. L. Ferrec, C. Mayer, V. Mimouni, D. Lagadic-Gossmann, B. Schoefs and L. Ulmann, Microalgal carotenoids and phytosterols regulate biochemical mechanisms involved in human health and disease prevention, Biochimie, 2019, 167, 106–118 CrossRef CAS PubMed.
- J. A. Canas, Mixed carotenoid supplementation and dysmetabolic obesity: gaps in knowledge, Int. J. Food Sci. Nutr., 2021, 72, 653–659 CrossRef CAS PubMed.
- T. Goto, N. Takahashi, S. Kato, Y. I. Kim, T. Kusudo, A. Taimatsu, K. Egawa, M. S. Kang, T. Hiramatsu, T. Sakamoto, T. Uemura, S. Hirai, M. Kobayashi, F. Horio and T. Kawada, Bixin activates PPARalpha and improves obesity-induced abnormalities of carbohydrate and lipid metabolism in mice, J. Agric. Food Chem., 2012, 60, 11952–11958 CrossRef CAS PubMed.
- G. Maiani, M. J. Caston, G. Catasta, E. Toti, I. G. Cambrodon, A. Bysted, F. Granado-Lorencio, B. Olmedilla-Alonso, P. Knuthsen, M. Valoti, V. Bohm, E. Mayer-Miebach, D. Behsnilian and U. Schlemmer, Carotenoids: actual knowledge on food sources, intakes, stability and bioavailability and their protective role in humans, Mol. Nutr. Food Res., 2009, 53(Suppl 2), S194–S218 Search PubMed.
- P. Borel, C. Desmarchelier, M. Nowicki, R. Bott, S. Morange and N. Lesavre, Interindividual variability of lutein bioavailability in healthy men: characterization, genetic variants involved, and relation with fasting plasma lutein concentration, Am. J. Clin. Nutr., 2014, 100, 168–175 CrossRef CAS PubMed.
- T. Bohn, C. Desmarchelier, L. O. Dragsted, C. S. Nielsen, W. Stahl, R. Rühl, J. Keijer and P. Borel, Host-related factors explaining interindividual variability of carotenoid bioavailability and tissue concentrations in humans, Mol. Nutr. Food Res., 2017, 61, 1600685 CrossRef PubMed.
- C. Desmarchelier and P. Borel, Overview of carotenoid bioavailability determinants: From dietary factors to host genetic variations, Trends Food Sci. Technol., 2017, 69, 270–280 CrossRef CAS.
- L. Jahns, L. K. Johnson, Z. Conrad, M. Bukowski, S. K. Raatz, S. Jilcott Pitts, Y. Wang, I. V. Ermakov and W. Gellermann, Concurrent validity of skin carotenoid status as a concentration biomarker of vegetable and fruit intake compared to multiple 24 h recalls and plasma carotenoid concentrations across one year: a cohort study, Nutr. J., 2019, 18, 78 CrossRef PubMed.
- L. Notario-Barandiaran, E.-M. Navarrete-Muñoz, D. Valera-Gran, E. Hernández-Álvarez, E. Donoso-Navarro, S. González-Palacios, M. García-De-La-Hera, M. F. Fernández, C. Freire and J. Vioque, Biochemical Validation of a Self-Administered Food Frequency Questionnaire to Assess Diet Using Carotenoids and Vitamins E and D in Male Adolescents in Spain, Antioxidants, 2021, 10, 750 CrossRef CAS PubMed.
- B. Eisenhauer, S. Natoli, G. Liew and V. Flood, Lutein and Zeaxanthin—Food Sources, Bioavailability and Dietary Variety in Age–Related Macular Degeneration Protection, Nutrients, 2017, 9, 120 CrossRef PubMed.
- C. S. Boon, D. J. McClements, J. Weiss and E. A. Decker, Factors influencing the chemical stability of carotenoids in foods, Crit. Rev. Food Sci. Nutr., 2010, 50, 515–532 CrossRef CAS PubMed.
- R. K. Saini, P. Prasad, V. Lokesh, X. Shang, J. Shin, Y.-S. Keum and J.-H. Lee, Carotenoids: Dietary Sources, Extraction, Encapsulation, Bioavailability, and Health Benefits—A Review of Recent Advancements, Antioxidants, 2022, 11, 795 CrossRef CAS PubMed.
- J. Von Lintig, J. Moon, J. Lee and S. Ramkumar, Carotenoid metabolism at the intestinal barrier, Biochim. Biophys. Acta, Mol. Cell Biol. Lipids, 2020, 1865, 158580 CrossRef CAS PubMed.
- T. Bohn, Bioavailability of non-provitamin A carotenoids, Curr. Nutr. Food Sci., 2008, 4, 240–258 CrossRef CAS.
- S. B. Jilcott Pitts, N. S. Johnson, Q. Wu, G. C. Firnhaber, A. Preet Kaur and J. Obasohan, A meta-analysis of studies examining associations between resonance Raman spectroscopy-assessed skin carotenoids and plasma carotenoids among adults and children, Nutr. Rev., 2022, 80, 230–241 CrossRef PubMed.
- L. M. Wilson, S. Tharmarajah, Y. Jia, R. D. Semba, D. A. Schaumberg and K. A. Robinson, The Effect of Lutein/Zeaxanthin Intake on Human Macular Pigment Optical Density: A Systematic Review and Meta-Analysis, Adv. Nutr., 2021, 12, 2244–2254 CrossRef CAS PubMed.
- E. Schwedhelm, R. Maas, R. Troost and R. H. Böger, Clinical Pharmacokinetics of Antioxidants and Their Impact on Systemic Oxidative Stress, Clin. Pharmacokinet., 2003, 42, 437–459 CrossRef CAS PubMed.
- S. S. Tworoger and S. E. Hankinson, Collection, processing, and storage of biological samples in epidemiologic studies: sex hormones, carotenoids, inflammatory markers, and proteomics as examples, Cancer Epidemiol. Biomarkers Prev., 2006, 15, 1578–1581 CrossRef CAS PubMed.
- M. Donaldson, Development of a rapid, simple assay of plasma total carotenoids, BMC Res. Notes, 2012, 5, 521 CrossRef CAS PubMed.
- K. Szewczyk, A. Chojnacka and M. Górnicka, Tocopherols and Tocotrienols—Bioactive Dietary Compounds; What Is Certain, What Is Doubt?, Int. J. Mol. Sci., 2021, 22, 6222 CrossRef CAS PubMed.
- Y. Zhang, J. Ding, H. Guo, Z. Liu, Q. Liu, Y. Li, D. Zhang and J. Liang, Associations of Dietary and Circulating Vitamin E Level With Metabolic Syndrome. A Meta-Analysis of Observational Studies, Front. Nutr., 2021, 8, 783990 CrossRef PubMed.
- M. R. Emami, M. Safabakhsh, S. Alizadeh, O. Asbaghi and M. Z. Khosroshahi, Effect of vitamin E supplementation on blood pressure: a systematic review and meta-analysis, J. Hum. Hypertens., 2019, 33, 499–507 CAS.
- R. Brigelius-Flohe, Vitamin E research: Past, now and future, Free Radicals Biol. Med., 2021, 177, 381–390 CrossRef CAS PubMed.
- P. Borel, M. Moussa, E. Reboul, B. Lyan, C. Defoort, S. p. Vincent-Baudry, M. Maillot, M. Gastaldi, M. Darmon, H. Portugal, R. Planells and D. Lairon, Human Plasma Levels of Vitamin E and Carotenoids Are Associated with Genetic Polymorphisms in Genes Involved in Lipid Metabolism, J. Nutr., 2007, 137, 2653–2659 CrossRef CAS PubMed.
- A. Ascherio, M. J. Stampfer, G. A. Colditz, E. B. Rimm, L. Litin and W. C. Willett, Correlations of vitamin A and E intakes with the plasma concentrations of carotenoids and tocopherols among American men and women, J. Nutr., 1992, 122, 1792–1801 CrossRef CAS PubMed.
- S. A. Mcnaughton, G. C. Marks, P. Gaffney, G. Williams and A. Green, Validation of a food-frequency questionnaire assessment of carotenoid and vitamin E intake using weighed food records and plasma biomarkers: The method of triads model, Eur. J. Clin. Nutr., 2005, 59, 211–218 CrossRef CAS PubMed.
- A. M. Hodge, J. A. Simpson, M. Fridman, K. Rowley, D. R. English, G. G. Giles, Q. Su and K. O'Dea, Evaluation of an FFQ for assessment of antioxidant intake using plasma biomarkers in an ethnically diverse population, Public Health Nutr., 2009, 12, 2438–2447 CrossRef PubMed.
- W. C. Willett, M. J. Stampfer, B. A. Underwood, F. E. Speizer, B. Rosner and C. H. Hennekens, Validation of a dietary questionnaire with plasma carotenoid and α-tocopherol levels, Am. J. Clin. Nutr., 1983, 38, 631–639 CrossRef CAS PubMed.
- H. Zielinski, E. Ciska and H. Kozlowska, The cereal grains: Focus on vitamin E, Czech J. Food Sci., 2001, 19, 182–188 CrossRef CAS.
- F. Shahidi, A. C. C. Pinaffi-Langley, J. Fuentes, H. Speisky and A. C. de Camargo, Vitamin E as an essential micronutrient for human health: Common, novel, and unexplored dietary sources, Free Radicals Biol. Med., 2021, 176, 312–321 CrossRef CAS PubMed.
- M. Garcia-Aloy, P. J. M. Hulshof, S. Estruel-Amades, M. C. J. Osté, M. Lankinen, J. M. Geleijnse, J. De Goede, M. Ulaszewska, F. Mattivi, S. J. L. Bakker, U. Schwab and C. Andres-Lacueva, Biomarkers of food intake for nuts and vegetable oils: an extensive literature search, Genes Nutr., 2019, 14, 7 CrossRef PubMed.
- B. McKevith, Nutritional aspects of cereals, Nutr. Bull., 2004, 29, 111–142 CrossRef.
- N. E. Craft, E. D. Brown and J. C. Smith Jr., Effects of storage and handling conditions on concentrations of individual carotenoids, retinol, and tocopherol in plasma, Clin. Chem., 1988, 34, 44–48 CrossRef CAS.
- W. M. Stöggl, C. W. Huck, H. Scherz, M. Popp and G. K. Bonn, Analysis of vitamin E in food and phytopharmaceutical preparations by HPLC and HPLC-APCI-MS-MS, Chromatographia, 2001, 54, 179–185 CrossRef.
- H. El Gharras, Polyphenols: food sources, properties and applications – a review, Int. J. Food Sci. Technol., 2009, 44, 2512–2518 CrossRef CAS.
- J. Y. Jung, I. K. Lee, S. J. Seok, H. J. Lee, Y. H. Kim and B. S. Yun, Antioxidant polyphenols from the mycelial culture of the medicinal fungi Inonotus xeranticus and Phellinus linteus, J. Appl. Microbiol., 2008, 104, 1824–1832 CrossRef CAS PubMed.
- C. Filesi, R. Di Benedetto, R. Gargiulo, C. Giovannini and R. Masella, Polyphenols, dietary sources and bioavailability, 2007 Search PubMed.
- A. Scalbert and G. Williamson, Dietary Intake and Bioavailability of Polyphenols, J. Nutr., 2000, 130, 2073S–2085S CrossRef CAS PubMed.
- F. Saura-Calixto, J. Serrano and I. Goñi, Intake and bioaccessibility of total polyphenols in a whole diet, Food Chem., 2007, 101, 492–501 CrossRef CAS.
- R. Tsao, Chemistry and Biochemistry of Dietary Polyphenols, Nutrients, 2010, 2, 1231–1246 CrossRef CAS PubMed.
- M. A. Carluccio, L. Siculella, M. A. Ancora, M. Massaro, E. Scoditti, C. Storelli, F. Visioli, A. Distante and R. D. Caterina, Olive Oil
and Red Wine Antioxidant Polyphenols Inhibit Endothelial Activation, Arterioscler., Thromb., Vasc. Biol., 2003, 23, 622–629 CrossRef CAS PubMed.
- S. Asgary, R. Karimi, S. Momtaz, R. Naseri and M. H. Farzaei, Effect of resveratrol on metabolic syndrome components: A systematic review and meta-analysis, Rev. Endocr. Metab. Disord., 2019, 20, 173–186 CrossRef CAS PubMed.
- R. Naseri, F. Farzaei, P. Haratipour, S. F. Nabavi, S. Habtemariam, M. H. Farzaei, R. Khodarahmi, D. Tewari and S. Momtaz, Anthocyanins in the Management of Metabolic Syndrome: A Pharmacological and Biopharmaceutical Review, Front. Pharmacol., 2018, 9, 1310 CrossRef CAS PubMed.
- C. Costa, A. Tsatsakis, C. Mamoulakis, M. Teodoro, G. Briguglio, E. Caruso, D. Tsoukalas, D. Margina, E. Dardiotis, D. Kouretas and C. Fenga, Current evidence on the effect of dietary polyphenols intake on chronic diseases, Food Chem. Toxicol., 2017, 110, 286–299 CrossRef CAS PubMed.
- N. Yahfoufi, N. Alsadi, M. Jambi and C. Matar, The Immunomodulatory and Anti-Inflammatory Role of Polyphenols, Nutrients, 2018, 10, 1618 CrossRef PubMed.
- C. C. Tangney and H. E. Rasmussen, Polyphenols, Inflammation, and Cardiovascular Disease, Curr. Atheroscler. Rep., 2013, 15, 324 CrossRef PubMed.
- B. N. Singh, S. Shankar and R. K. Srivastava, Green tea catechin, epigallocatechin-3-gallate (EGCG): Mechanisms, perspectives and clinical applications, Biochem. Pharmacol., 2011, 82, 1807–1821 CrossRef CAS PubMed.
- A. Medina-Remón, R. Casas, A. Tressserra-Rimbau, E. Ros, M. A. Martínez-González, M. Fitó, D. Corella, J. Salas-Salvadó, R. M. Lamuela-Raventos and R. Estruch, Polyphenol intake from a Mediterranean diet decreases inflammatory biomarkers related to atherosclerosis: a substudy of the PREDIMED trial, Br. J. Clin. Pharmacol., 2017, 83, 114–128 CrossRef PubMed.
- A. A. Fallah, E. Sarmast, P. Fatehi and T. Jafari, Impact of dietary anthocyanins on systemic and vascular inflammation: Systematic review and meta-analysis on randomised clinical trials, Food Chem. Toxicol., 2020, 135, 110922 CrossRef CAS PubMed.
- M. A. Bernardo, M. L. Silva, E. Santos, M. M. Moncada, J. Brito, L. Proença, J. Singh and M. F. De Mesquita, Effect of Cinnamon Tea on Postprandial Glucose Concentration, J. Diabetes Res., 2015, 2015, 1–6 CrossRef PubMed.
- J. B. Xiao and P. Hogger, Dietary polyphenols and type 2 diabetes: current insights and future perspectives, Curr. Med. Chem., 2015, 22, 23–38 CrossRef CAS PubMed.
- M. Shahwan, F. Alhumaydhi, G. M. Ashraf, P. M. Z. Hasan and A. Shamsi, Role of polyphenols in combating Type 2 Diabetes and insulin resistance, Int. J. Biol. Macromol., 2022, 206, 567–579 CrossRef CAS PubMed.
- G. Williamson, Effects of Polyphenols on Glucose–Induced Metabolic Changes in Healthy Human Subjects and on Glucose Transporters, Mol. Nutr. Food Res., 2022, 66, 2101113 CrossRef CAS PubMed.
- J. Most, I. Warnke, M. V. Boekschoten, J. W. E. Jocken, P. D. Groot, A. Friedel, I. Bendik, G. H. Goossens and E. E. Blaak, The effects of polyphenol supplementation on adipose tissue morphology and gene expression in overweight and obese humans, Adipocyte, 2018, 7, 190–196 CrossRef CAS PubMed.
- J. Domínguez-Avila, G. González-Aguilar, E. Alvarez-Parrilla and L. D. L. Rosa, Modulation of PPAR Expression and Activity in Response to Polyphenolic Compounds in High Fat Diets, Int. J. Mol. Sci., 2016, 17, 1002 CrossRef PubMed.
- L. Flori, E. Piragine, J. Spezzini, V. Citi, V. Calderone and A. Martelli, Influence of Polyphenols on Adipose Tissue: Sirtuins as Pivotal Players in the Browning Process, Int. J. Mol. Sci., 2023, 24, 9276 CrossRef CAS PubMed.
- S. V. Luca, I. Macovei, A. Bujor, A. Miron, K. Skalicka-Wozniak, A. C. Aprotosoaie and A. Trifan, Bioactivity of dietary polyphenols: The role of metabolites, Crit. Rev. Food Sci. Nutr., 2020, 60, 626–659 CrossRef CAS PubMed.
- A. Scalbert, C. Morand, C. Manach and C. Remesy, Absorption and metabolism of polyphenols in the gut and impact on health, Biomed. Pharmacother., 2002, 56, 276–282 CrossRef CAS PubMed.
- R. Landberg, C. Manach, F.-M. Kerckhof, A.-M. Minihane, R. N. M. Saleh, B. De Roos, F. Tomas-Barberan, C. Morand and T. Van De Wiele, Future prospects for dissecting inter-individual variability in the absorption, distribution and elimination of plant bioactives of relevance for cardiometabolic endpoints, Eur. J. Nutr., 2019, 58, 21–36 CrossRef PubMed.
- C. Manach, G. Williamson, C. Morand, A. Scalbert and C. Rémésy, Bioavailability and bioefficacy of polyphenols in humans. I. Review of 97 bioavailability studies, Am. J. Clin. Nutr., 2005, 81, 230S–242S CrossRef CAS PubMed.
- S. Kupina, C. Fields, M. C. Roman and S. L. Brunelle, Determination of Total Phenolic Content Using the Folin-C Assay: Single-Laboratory Validation, First Action 2017.13, J. AOAC Int., 2018, 101, 1466–1472 CrossRef CAS PubMed.
- A. Rodger and K. Sanders, in Encyclopedia of Spectroscopy and Spectrometry, 2017, pp. 495–502. DOI:10.1016/b978-0-12-803224-4.00108-4.
- J.-J. Yuan, J.-L. Tu, F. G. F. Qin, Y.-J. Xu and B. Li, Phenolic composition of oleuropein extract after enzymatic process by HPLC-MS and their antioxidant and antibacterial activities, J. Food Biochem., 2018, 42, e12517 CrossRef.
- E. Cieślik, A. Gręda and W. Adamus, Contents of polyphenols in fruit and vegetables, Food Chem., 2006, 94, 135–142 CrossRef.
- J. Radtke, J. Linseisen and G. Wolfram, Fasting plasma concentrations of selected flavonoids as markers of their ordinary dietary intake, Eur. J. Nutr., 2002, 41, 203–209 CrossRef CAS PubMed.
- J. Cao, Y. Zhang, W. Chen and X. Zhao, The relationship between fasting plasma concentrations of selected flavonoids and their ordinary dietary intake, Br. J. Nutr., 2010, 103, 249–255 CrossRef CAS PubMed.
- C. Manach and J. L. Donovan, Pharmacokinetics and metabolism of dietary flavonoids in humans, Free Radical Res., 2004, 38, 771–785 CrossRef CAS PubMed.
- J. A. T. Pennington and R. A. Fisher, Food component profiles for fruit and vegetable subgroups, J. Food Compos. Anal., 2010, 23, 411–418 CrossRef CAS.
- H. Mostafa, A. Cheok, T. Meroño, C. Andres-Lacueva and A. Rodriguez-Mateos, Biomarkers of Berry Intake: Systematic Review Update, J. Agric. Food Chem., 2023, 71, 11789–11805 CrossRef CAS PubMed.
- R. M. De Ferrars, C. Czank, Q. Zhang, N. P. Botting, P. A. Kroon, A. Cassidy and C. D. Kay, The pharmacokinetics of anthocyanins and their metabolites in humans, Br. J. Pharmacol., 2014, 171, 3268–3282 CrossRef CAS PubMed.
- M. K. Samota, M. Sharma, K. Kaur, Sarita, D. K. Yadav, A. K. Pandey, Y. Tak, M. Rawat, J. Thakur and H. Rani, Onion anthocyanins: Extraction, stability, bioavailability, dietary effect, and health implications, Front. Nutr., 2022, 9, 917617 CrossRef PubMed.
- I. Erlund, Review of the flavonoids quercetin, hesperetin, and naringenin. Dietary sources, bioactivities, bioavailability, and epidemiology, Nutr. Res., 2004, 24, 851–874 CrossRef CAS.
- A. Brevik, S. E. Rasmussen, C. A. Drevon and L. F. Andersen, Urinary Excretion of Flavonoids Reflects Even Small Changes in the Dietary Intake of Fruits and Vegetables, Cancer Epidemiol., Biomarkers Prev., 2004, 13, 843–849 CrossRef CAS.
- A. L. Brantsæter, M. Haugen, S. E. Rasmussen, J. Alexander, S. O. Samuelsen and H. M. Meltzer, Urine flavonoids and plasma carotenoids in the validation of fruit, vegetable and tea intake during pregnancy in the Norwegian Mother and Child Cohort Study (MoBa), Public Health Nutr., 2007, 10, 838–847 CrossRef PubMed.
- S. E. Nielsen, R. Freese, P. i. Kleemola and M. Mutanen, Flavonoids in Human Urine as Biomarkers for Intake of Fruits and Vegetables1, Cancer Epidemiol., Biomarkers Prev., 2002, 11, 459–466 CAS.
- Y. Ma, P. Li, D. Chen, T. Fang, H. Li and W. Su, LC/MS/MS quantitation assay for pharmacokinetics of naringenin and double peaks phenomenon in rats plasma, Int. J. Pharm., 2006, 307, 292–299 CrossRef CAS PubMed.
- F. I. Kanaze, E. Kokkalou, M. Georgarakis and I. Niopas, A validated solid-phase extraction HPLC method for the simultaneous determination of the citrus flavanone aglycones hesperetin and naringenin in urine, J. Pharm. Biomed. Anal., 2004, 36, 175–181 CrossRef CAS PubMed.
- Y. Yagishita, J. W. Fahey, A. T. Dinkova-Kostova and T. W. Kensler, Broccoli or Sulforaphane: Is It the Source or Dose That Matters?, Molecules, 2019, 24, 3593 CrossRef PubMed.
- R. Santin-Marquez, A. Alarcon-Aguilar, N. E. Lopez-Diazguerrero, N. Chondrogianni and M. Konigsberg, Sulforaphane - role in aging and neurodegeneration, GeroScience, 2019, 41, 655–670 CrossRef PubMed.
- P. A. Egner, T. W. Kensler, J.-G. Chen, S. J. Gange, J. D. Groopman and M. D. Friesen, Quantification of Sulforaphane Mercapturic Acid Pathway Conjugates in Human Urine by High-Performance Liquid Chromatography and Isotope-Dilution Tandem Mass Spectrometry, Chem. Res. Toxicol., 2008, 21, 1991–1996 Search PubMed.
- A. A. Al Janobi, R. F. Mithen, A. V. Gasper, P. N. Shaw, R. J. Middleton, C. A. Ortori and D. A. Barrett, Quantitative measurement of sulforaphane, iberin and their mercapturic acid pathway metabolites in human plasma and urine using liquid chromatography-tandem electrospray ionisation mass spectrometry, J. Chromatogr. B: Anal. Technol. Biomed. Life Sci., 2006, 844, 223–234 CrossRef CAS PubMed.
- M. Vermeulen, I. W. Klopping-Ketelaars, R. van den Berg and W. H. Vaes, Bioavailability and kinetics of sulforaphane in humans after consumption of cooked versus raw broccoli, J. Agric. Food Chem., 2008, 56, 10505–10509 CrossRef CAS PubMed.
- N. Vazquez-Manjarrez, M. Ulaszewska, M. Garcia-Aloy, F. Mattivi, G. Pratico, L. O. Dragsted and C. Manach, Biomarkers of intake for tropical fruits, Genes Nutr., 2020, 15, 11 CrossRef CAS PubMed.
- X. Liu, J. Sievert, M. L. Arpaia and M. A. Madore, Postulated Physiological Roles of the Seven-carbon Sugars, Mannoheptulose, and Perseitol in Avocado, J. Am. Soc. Hortic. Sci., 2002, 127, 108–114 CAS.
- H. Mandel, N. Levy, S. Izkovitch and S. H. Korman, Elevated plasma citrulline and arginine due to consumption of Citrullus vulgaris (watermelon), J. Inherited Metab. Dis., 2005, 28, 467–472 CrossRef CAS PubMed.
- S. J. Bailey, J. R. Blackwell, E. Williams, A. Vanhatalo, L. J. Wylie, P. G. Winyard and A. M. Jones, Two weeks of watermelon juice supplementation improves nitric oxide bioavailability but not endurance exercise performance in humans, Nitric Oxide, 2016, 59, 10–20 CrossRef CAS PubMed.
- M. Garcia-Aloy, M. Ulaszewska, P. Franceschi, S. Estruel-Amades, C. H. Weinert, A. Tor-Roca, M. Urpi-Sarda, F. Mattivi and C. Andres-Lacueva, Discovery of Intake Biomarkers of Lentils, Chickpeas, and White Beans by Untargeted LC-MS Metabolomics in Serum and Urine, Mol. Nutr. Food Res., 2020, 64, e1901137 CrossRef PubMed.
- F. Sparvoli, R. Bollini and E. Cominelli, in Grain Legumes, Springer New York, 2015, pp. 291–325. DOI:10.1007/978-1-4939-2797-5_10.
- Y. T. Jiang, J. Y. Zhang, Y. S. Liu, Q. Chang, Y. H. Zhao and Q. J. Wu, Relationship between legume consumption and metabolic syndrome: A systematic review and meta-analysis
of observational studies, Nutr., Metab. Cardiovasc. Dis., 2020, 30, 384–392 CrossRef PubMed.
- R. A. Dixon and D. Ferreira, Genistein, Phytochemistry, 2002, 60, 205–211 CrossRef CAS PubMed.
- H. W. Woo, M. K. Kim, Y.-H. Lee, D. H. Shin, M.-H. Shin and B. Y. Choi, Habitual consumption of soy protein and isoflavones and risk of metabolic syndrome in adults ≥40 years old: a prospective analysis of the Korean Multi-Rural Communities Cohort Study (MRCohort), Eur. J. Nutr., 2019, 58, 2835–2850 CrossRef CAS PubMed.
- D. Zhu, N. S. Hettiarachchy, R. Horax and P. Chen, Isoflavone Contents in Germinated Soybean Seeds, Plant Foods Hum. Nutr., 2005, 60, 147–151 CrossRef CAS PubMed.
- I.-S. Kim, Current Perspectives on the Beneficial Effects of Soybean Isoflavones and Their Metabolites for Humans, Antioxidants, 2021, 10, 1064 CrossRef CAS PubMed.
- W. De Keyzer, I. Huybrechts, A. L. M. Dekkers, A. Geelen, S. Crispim, P. J. M. Hulshof, L. F. Andersen, I. Řehůřková, J. Ruprich, J.-L. Volatier, G. Van Maele, N. Slimani, P. Van'T Veer, E. D. Boer and S. D. Henauw, Predicting urinary creatinine excretion and its usefulness to identify incomplete 24 h urine collections, Br. J. Nutr., 2012, 108, 1118–1125 CrossRef CAS PubMed.
- T. Bohn, M. Blackwood, D. Francis, Q. Tian, S. J. Schwartz and S. K. Clinton, Bioavailability of Phytochemical Constituents From a Novel Soy Fortified Lycopene Rich Tomato Juice Developed for Targeted Cancer Prevention Trials, Nutr. Cancer, 2013, 65, 919–929 CrossRef CAS PubMed.
- A. E. McNamara and L. Brennan, Potential of food intake biomarkers in nutrition research, Proc. Nutr. Soc., 2020, 1–11, DOI:10.1017/S0029665120007053.
- D. Lafiandra, G. Riccardi and P. R. Shewry, Improving cereal grain carbohydrates for diet and health, J. Cereal Sci., 2014, 59, 312–326 CrossRef CAS PubMed.
- . A. H. Association, Whole Grains, Refined Grains, and Dietary Fiber, https://www.heart.org/en/healthy-living/healthy-eating/eat-smart/nutrition-basics/whole-grains-refined-grains-and-dietary-fiber, (accessed 24/02/2023, 2023).
- P. Sookwong, K. Nakagawa, Y. Yamaguchi, T. Miyazawa, S. Kato, F. Kimura and T. Miyazawa, Tocotrienol distribution in foods: estimation of daily tocotrienol intake of Japanese population, J. Agric. Food Chem., 2010, 58, 3350–3355 CrossRef CAS PubMed.
- D. Trono, Carotenoids in Cereal Food Crops: Composition and Retention throughout Grain Storage and Food Processing, Plants, 2019, 8, 551 CrossRef CAS PubMed.
- S. Gorinstein, O. J. M. Vargas, N. O. Jaramillo, I. A. Salas, A. L. M. Ayala, P. Arancibia-Avila, F. Toledo, E. Katrich and S. Trakhtenberg, The total polyphenols and the antioxidant potentials of some selected cereals and pseudocereals, Eur. Food Res. Technol., 2007, 225, 321–328 CrossRef CAS.
- E.-S. Abdel-Aal, H. Akhtar, K. Zaheer and R. Ali, Dietary Sources of Lutein and Zeaxanthin Carotenoids and Their Role in Eye Health, Nutrients, 2013, 5, 1169–1185 CrossRef CAS PubMed.
- W. Sae-Lao, K. Wunjuntuk, T. Techakriengkrai and P. P. Sirichakwal, Consumption of Lutein and Zeaxanthin and Its Relation to the Level of Macular Pigment Optical Density in Thai Subjects, J. Nutr. Metab., 2022, 2022, 1–8 CrossRef PubMed.
- D. Horvat, G. Šimić, G. Drezner, A. Lalić, T. Ledenčan, M. Tucak, H. Plavšić, L. Andrić and Z. Zdunić, Phenolic Acid Profiles and Antioxidant Activity of Major Cereal Crops, Antioxidants, 2020, 9, 527 CrossRef CAS PubMed.
- B. Ed Nignpense, N. Francis, C. Blanchard and A. B. Santhakumar, Bioaccessibility and Bioactivity of Cereal Polyphenols: A Review, Foods, 2021, 10, 1595 CrossRef CAS PubMed.
- L. Zhou, X. Mo, Q. Wang, H. Liu, Y. Liu, Q. Luo, J. Yin, X. Peng, Y. Huang, X. Li, Z. Shan, G. Liu, C. Xia, J. Cheng, W. Yang and T. Sun, Plasma Alkylresorcinol Metabolite, a Biomarker of Whole-Grain Wheat and Rye Intake, and Risk of Type 2 Diabetes and Impaired Glucose Regulation in a Chinese Population, Diabetes Care, 2017, 41(3), dc171570 Search PubMed.
- I. Biskup, C. Kyro, M. Marklund, A. Olsen, R. M. van Dam, A. Tjonneland, K. Overvad, B. Lindahl, I. Johansson and R. Landberg, Plasma alkylresorcinols, biomarkers of whole-grain wheat and rye intake, and risk of type 2 diabetes in Scandinavian men and women, Am. J. Clin. Nutr., 2016, 104, 88–96 CrossRef CAS PubMed.
- R. Landberg, A. Kamal-Eldin, A. Andersson, B. Vessby and P. Aman, Alkylresorcinols as biomarkers of whole-grain wheat and rye intake: plasma concentration and intake estimated from dietary records, Am. J. Clin. Nutr., 2008, 87, 832–838 CrossRef CAS PubMed.
- A. B. Ross, Present Status and Perspectives on the Use of Alkylresorcinols as Biomarkers of Wholegrain Wheat and Rye Intake, J. Nutr. Metab., 2012, 2012, 1–12 CrossRef PubMed.
- M. Jawhara, S. B. Sørensen, B. L. Heitmann and V. Andersen, Biomarkers of Whole-Grain and Cereal-Fiber Intake in Human Studies: A Systematic Review of the Available Evidence and Perspectives, Nutrients, 2019, 11, 2994 CrossRef CAS PubMed.
- H. Wu, N. A. Mhd Omar, N. Håkansson, A. Wolk, K. Michaëlsson and R. Landberg, Evaluation of alkylresorcinols in adipose tissue biopsies as a long-term biomarker of whole-grain wheat and rye intake in free-living Swedish men and women, Public Health Nutr., 2018, 21, 1933–1942 CrossRef PubMed.
- K. B. Adhikari, B. B. Laursen, P. L. Gregersen, H. J. Schnoor, M. Witten, L. K. Poulsen, B. M. Jensen and I. S. Fomsgaard, Absorption and metabolic fate of bioactive dietary benzoxazinoids in humans, Mol. Nutr. Food Res., 2013, 57, 1847–1858 CrossRef CAS PubMed.
- Y. Kim, J. Keogh and P. Clifton, Nuts and Cardio-Metabolic Disease: A Review of Meta-Analyses, Nutrients, 2018, 10, 1935 CrossRef PubMed.
- R. Pastor, C. Bouzas and J. A. Tur, Beneficial effects of dietary supplementation with olive oil, oleic acid, or hydroxytyrosol in metabolic syndrome: Systematic review and meta-analysis, Free Radicals Biol. Med., 2021, 172, 372–385 CrossRef CAS PubMed.
- K. H. Weylandt, C. Y. Chiu, B. Gomolka, S. F. Waechter and B. Wiedenmann, Omega-3 fatty acids and their lipid mediators: towards an understanding of resolvin and protectin formation, Prostaglandins Other Lipid Mediators, 2012, 97, 73–82 CrossRef CAS PubMed.
- P. C. Calder, Omega-3 fatty acids and inflammatory processes: from molecules to man, Biochem. Soc. Trans., 2017, 45, 1105–1115 CrossRef CAS PubMed.
- B. Kapoor, D. Kapoor, S. Gautam, R. Singh and S. Bhardwaj, Dietary Polyunsaturated Fatty Acids (PUFAs): Uses and Potential Health Benefits, Curr. Nutr. Rep., 2021, 10, 232–242 CrossRef PubMed.
- G. Cubas-Basterrechea, I. Elio, S. Sumalla-Cano, S. Aparicio-Obregon, C. T. Gonzalez-Anton and P. Munoz-Cacho, The Regular Consumption of Nuts Is Associated with a Lower Prevalence of Abdominal Obesity and Metabolic Syndrome in Older People from the North of Spain, Int. J. Environ. Res. Public Health, 2022, 19, 1256 CrossRef PubMed.
- E. Ros and J. Mataix, Fatty acid composition of nuts – implications for cardiovascular health, Br. J. Nutr., 2006, 96, S29–S35 CrossRef CAS PubMed.
- E. M. Yubero-Serrano, J. Lopez-Moreno, F. Gomez-Delgado and J. Lopez-Miranda, Extra virgin olive oil: More than a healthy fat, Eur. J. Clin. Nutr., 2019, 72, 8–17 CrossRef PubMed.
- G. C. Burdge, Metabolism of α-linolenic acid in humans, Prostaglandins, Leukotrienes Essent. Fatty Acids, 2006, 75, 161–168 CrossRef CAS PubMed.
- D. Boskou, G. Blekas and M. Tsimidou, in Olive Oil, ed. D. Boskou, AOCS Press, 2nd edn, 2006, pp. 41–72. DOI:10.1016/B978-1-893997-88-2.50008-0.
- R. García-Villalba, J. A. Giménez-Bastida, A. Cortés-Martín, M.Á Ávila-Gálvez, F. A. Tomás-Barberán, M. V. Selma, J. C. Espín and A. González-Sarrías, Urolithins: a Comprehensive Update on their Metabolism, Bioactivity, and Associated Gut Microbiota, Mol. Nutr. Food Res., 2022, 66, 2101019 CrossRef PubMed.
- A. M. Toney, D. Fox, V. Chaidez, A. E. Ramer-Tait and S. Chung, Immunomodulatory Role of Urolithin A on Metabolic Diseases, Biomedicines, 2021, 9, 192 CrossRef CAS PubMed.
- D. Ryu, L. Mouchiroud, P. A. Andreux, E. Katsyuba, N. Moullan, A. A. Nicolet-Dit-Félix, E. G. Williams, P. Jha, G. Lo Sasso, D. Huzard, P. Aebischer, C. Sandi, C. Rinsch and J. Auwerx, Urolithin A induces mitophagy and prolongs lifespan in C. elegans and increases muscle function in rodents, Nat. Med., 2016, 22, 879–888 CrossRef CAS PubMed.
- J. M. Landete, Ellagitannins, ellagic acid and their derived metabolites: A review about source, metabolism, functions and health, Food Res. Int., 2011, 44, 1150–1160 CrossRef CAS.
- S. R. Hennigar and J. P. McClung, Homeostatic regulation of trace mineral transport by ubiquitination of membrane transporters, Nutr. Rev., 2016, 74, 59–67 CrossRef PubMed.
- M. Kieliszek, Selenium–Fascinating Microelement, Properties and Sources in Food, Molecules, 2019, 24, 1298 CrossRef CAS PubMed.
- V. Mancebo-Campos, M. D. Salvador and G. Fregapane, EFSA Health Claims-Based Virgin Olive Oil Shelf-Life, Antioxidants, 2023, 12, 1563 CrossRef CAS PubMed.
- M. I. Fernández-Mar, R. Mateos, M. C. García-Parrilla, B. Puertas and E. Cantos-Villar, Bioactive compounds in wine: Resveratrol, hydroxytyrosol and melatonin: A review, Food Chem., 2012, 130, 797–813 CrossRef.
- J. Kim and I. Jo, Grains, vegetables, and fish dietary pattern is inversely associated with the risk of metabolic syndrome in South korean adults, J. Am. Diet. Assoc., 2011, 111, 1141–1149 CrossRef PubMed.
- S. Castro-Barquero, A. M. Ruiz-León, M. Sierra-Pérez, R. Estruch and R. Casas, Dietary Strategies for Metabolic Syndrome: A Comprehensive Review, Nutrients, 2020, 12, 2983 CrossRef CAS PubMed.
- J. A. Rathmacher, P. J. Flakoll and S. L. Nissen, A compartmental model of 3-methylhistidine metabolism in humans, Am. J. Physiol., 1995, 269, E193–E198 CAS.
- A. D. Jones, A. C. Homan, D. J. Favell, C. H. Hitchcock, P. M. Berryman, N. M. Griffiths and M. J. Billington, Investigation of the levels of N(tau)-Methylhistidine in a range of beef cuts and offals, Meat Sci., 1985, 15, 137–147 CrossRef CAS PubMed.
- N. Hashizume, H. Ihara, M. Ohtsuka and H. Kadowaki, Blood amino acid levels in sarin poisoning patients, Rinsho Byori, 1997, 45, 785–789 CAS.
- B. Kochlik, W. Stuetz, K. Pérès, C. Féart, J. Tegner, L. Rodriguez-Mañas, T. Grune and D. Weber, Associations of Plasma 3-Methylhistidine with Frailty Status in French Cohorts of the FRAILOMIC Initiative, J. Clin. Med., 2019, 8, 1010 CrossRef CAS PubMed.
- C. Cuparencu, G. Pratico, L. Y. Hemeryck, P. S. C. Sri Harsha, S. Noerman, C. Rombouts, M. Xi, L. Vanhaecke, K. Hanhineva, L. Brennan and L. O. Dragsted, Biomarkers of meat and seafood intake: an extensive literature review, Genes Nutr., 2019, 14, 35 CrossRef CAS PubMed.
- G. E. Fraser, K. Jaceldo-Siegl, S. M. Henning, J. Fan, S. F. Knutsen, E. H. Haddad, J. Sabate, W. L. Beeson and H. Bennett, Biomarkers of Dietary Intake Are Correlated with Corresponding Measures from Repeated Dietary Recalls and Food-Frequency Questionnaires in the Adventist Health Study-2, J. Nutr., 2016, 146, 586–594 CrossRef CAS PubMed.
- W. Cheung, P. Keski-Rahkonen, N. Assi, P. Ferrari, H. Freisling, S. Rinaldi, N. Slimani, R. Zamora-Ros, M. Rundle, G. Frost, H. Gibbons, E. Carr, L. Brennan, A. J. Cross, V. Pala, S. Panico, C. Sacerdote, D. Palli, R. Tumino, T. Kuhn, R. Kaaks, H. Boeing, A. Floegel, F. Mancini, M. C. Boutron-Ruault, L. Baglietto, A. Trichopoulou, A. Naska, P. Orfanos and A. Scalbert, A metabolomic study of biomarkers of meat and fish intake, Am. J. Clin. Nutr., 2017, 105, 600–608 CrossRef CAS PubMed.
- B. Jakse, B. Jakse, M. Pajek and J. Pajek, Uric Acid and Plant-Based Nutrition, Nutrients, 2019, 11, 1736 CrossRef CAS PubMed.
- D. Venturini, A. N. Simao, M. R. Urbano and I. Dichi, Effects of extra virgin olive oil and fish oil on lipid profile and oxidative stress in patients with metabolic syndrome, Nutrition, 2015, 31, 834–840 CrossRef CAS PubMed.
- C. Torris, M. Molin and M. Cvancarova Smastuen, Fish consumption and its possible preventive role on the development and prevalence of metabolic syndrome - a systematic review, Diabetol. Metab. Syndr., 2014, 6, 112 CrossRef PubMed.
- W. Shen, A. M. Weaver, C. Salazar, J. M. Samet, D. Diaz-Sanchez and H. Tong, Validation of a Dietary Questionnaire to Screen Omega-3 Fatty Acids Levels in Healthy Adults, Nutrients, 2019, 11, 1470 CrossRef CAS PubMed.
- R. J. Pawlosky, J. R. Hibbeln, J. A. Novotny and N. Salem, Physiological compartmental analysis of α-linolenic acid metabolism in adult humans, J. Lipid Res., 2001, 42, 1257–1265 CrossRef CAS.
- S. D. Zuijdgeest-Van Leeuwen, P. C. Dagnelie, T. Rietveld, J. W. O. Van Den Berg and J. H. Paul Wilson, Incorporation and washout of orally administeredn-3 fatty acid ethyl esters in different plasma lipid fractions, Br. J. Nutr., 1999, 82, 481–488 CrossRef CAS PubMed.
- R. H. M. de Groot, R. Emmett and B. J. Meyer, Non-dietary factors associated with n-3 long-chain PUFA levels in humans - a systematic literature review, Br. J. Nutr., 2019, 121, 793–808 CrossRef CAS PubMed.
- H. Chung, J. A. Nettleton, R. N. Lemaitre, R. G. Barr, M. Y. Tsai, R. P. Tracy and D. S. Siscovick, Frequency and type of seafood consumed influence plasma (n-3) fatty acid concentrations, J. Nutr., 2008, 138, 2422–2427 CrossRef CAS PubMed.
- M. I. Gladyshev, N. N. Sushchik, G. A. Gubanenko, S. M. Demirchieva and G. S. Kalachova, Effect of boiling and frying on the content of essential polyunsaturated fatty acids in muscle tissue of four fish species, Food Chem., 2007, 101, 1694–1700 CrossRef CAS.
- S. Costa, C. Afonso, N. M. Bandarra, S. Gueifao, I. Castanheira, M. L. Carvalho, C. Cardoso and M. L. Nunes, The emerging farmed fish species meagre (Argyrosomus regius): how culinary treatment affects nutrients and contaminants concentration and associated benefit-risk balance, Food Chem. Toxicol., 2013, 60, 277–285 CrossRef CAS PubMed.
- A. Kamal-Eldin and N. V. Yanishlieva, N-3 fatty acids for human nutrition: stability considerations, Eur. J. Lipid Sci. Technol., 2002, 104, 825–836 CrossRef CAS.
- A. H. Metherel, J. J. Aristizabal Henao and K. D. Stark, EPA and DHA levels in whole blood decrease more rapidly when stored at −20 degrees C as compared with room temperature, 4 and −75 degrees C, Lipids, 2013, 48, 1079–1091 CrossRef CAS PubMed.
- B. S. Solvik, J. Oyen, I. Kvestad, M.
W. Markhus, P. M. Ueland, A. McCann and T. A. Strand, Biomarkers and Fatty Fish Intake: A Randomized Controlled Trial in Norwegian Preschool Children, J. Nutr., 2021, 151, 2134–2141 CrossRef PubMed.
- J. Ren, E. L. Mozurkewich, A. Sen, A. M. Vahratian, T. G. Ferreri, A. N. Morse and Z. Djuric, Total Serum Fatty Acid Analysis by GC-MS: Assay Validation and Serum Sample Stability, Curr. Pharm. Anal., 2013, 9, 331–339 CrossRef CAS PubMed.
- L. Barrea, G. Annunziata, G. Muscogiuri, C. D. Somma, D. Laudisio, M. Maisto, G. De Alteriis, G. Tenore, A. Colao and S. Savastano, Trimethylamine-N-oxide (TMAO) as Novel Potential Biomarker of Early Predictors of Metabolic Syndrome, Nutrients, 2018, 10, 1971 CrossRef PubMed.
- P. Gatarek and J. Kaluzna-Czaplinska, Trimethylamine N-oxide (TMAO) in human health, EXCLI J., 2021, 20, 301–319 Search PubMed.
- S. Yang, X. Li, F. Yang, R. Zhao, X. Pan, J. Liang, L. Tian, X. Li, L. Liu, Y. Xing and M. Wu, Gut Microbiota-Dependent Marker TMAO in Promoting Cardiovascular Disease: Inflammation Mechanism, Clinical Prognostic, and Potential as a Therapeutic Target, Front. Pharmacol., 2019, 10, 1360 CrossRef CAS PubMed.
- W. Stremmel, K. V. Schmidt, V. Schuhmann, F. Kratzer, S. F. Garbade, C.-D. Langhans, G. Fricker and J. G. Okun, Blood Trimethylamine-N-Oxide Originates from Microbiota Mediated Breakdown of Phosphatidylcholine and Absorption from Small Intestine, PLoS One, 2017, 12, e0170742 CrossRef PubMed.
- J. R. Baker and S. Chaykin, The Biosynthesis of Trimethylamine-N-Oxide, J. Biol. Chem., 1962, 237, 1309–1313 CrossRef CAS PubMed.
- M. Ufnal, A. Zadlo and R. Ostaszewski, TMAO: A small molecule of great expectations, Nutrition, 2015, 31, 1317–1323 CrossRef CAS PubMed.
- R. Jalandra, N. Dalal, A. K. Yadav, D. Verma, M. Sharma, R. Singh, A. Khosla, A. Kumar and P. R. Solanki, Emerging role of trimethylamine-N-oxide (TMAO) in colorectal cancer, Appl. Microbiol. Biotechnol., 2021, 105, 7651–7660 CrossRef CAS PubMed.
- G. Costabile, C. Vetrani, L. Bozzetto, R. Giacco, L. Bresciani, D. Del Rio, M. Vitale, G. Della Pepa, F. Brighenti, G. Riccardi, A. A. Rivellese and G. Annuzzi, Plasma TMAO increase after healthy diets: results from 2 randomized controlled trials with dietary fish, polyphenols, and whole-grain cereals, Am. J. Clin. Nutr., 2021, 114, 1342–1350 CrossRef PubMed.
- Z. Wang, B. S. Levison, J. E. Hazen, L. Donahue, X.-M. Li and S. L. Hazen, Measurement of trimethylamine-N-oxide by stable isotope dilution liquid chromatography tandem mass spectrometry, Anal. Biochem., 2014, 455, 35–40 CrossRef CAS PubMed.
- E. Garcia, J. Wolak-Dinsmore, Z. Wang, X. S. Li, D. W. Bennett, M. A. Connelly, J. D. Otvos, S. L. Hazen and E. J. Jeyarajah, NMR quantification of trimethylamine- N -oxide in human serum and plasma in the clinical laboratory setting, Clin. Biochem., 2017, 50, 947–955 CrossRef CAS PubMed.
- L. H. Münger, M. Garcia-Aloy, R. Vázquez-Fresno, D. Gille, A. R. R. Rosana, A. Passerini, M.-T. Soria-Florido, G. Pimentel, T. Sajed, D. S. Wishart, C. A. Lacueva, G. Vergères and G. Praticò, Biomarker of food intake for assessing the consumption of dairy and egg products, Genes Nutr., 2018, 13, 26 CrossRef PubMed.
- G. E. Crichton, J. Bryan, J. Buckley and K. J. Murphy, Dairy consumption and metabolic syndrome: a systematic review of findings and methodological issues, Obes. Rev., 2011, 12, e190–e201 CAS.
- S. Jin and Y. Je, Dairy Consumption and Risk of Metabolic Syndrome: Results from Korean Population and Meta-Analysis, Nutrients, 2021, 13, 1574 CrossRef CAS PubMed.
- A. Hruby, C. Dennis and P. F. Jacques, Dairy Intake in 2 American Adult Cohorts Associates with Novel and Known Targeted and Nontargeted Circulating Metabolites, J. Nutr., 2020, 150, 1272–1283 CrossRef PubMed.
- K. Trieu, S. Bhat, Z. Dai, K. Leander, B. Gigante, F. Qian, A. V. A. Korat, Q. Sun, X. F. Pan, F. Laguzzi, T. Cederholm, U. de Faire, M. L. Hellenius, J. H. Y. Wu, U. Riserus and M. Marklund, Biomarkers of dairy fat intake, incident cardiovascular disease, and all-cause mortality: A cohort study, systematic review, and meta-analysis, PLoS Med., 2021, 18, e1003763 CrossRef CAS PubMed.
- A. Sofie Biong, P. Berstad and J. I. Pedersen, Biomarkers for intake of dairy fat and dairy products, Eur. J. Lipid Sci. Technol., 2006, 108, 827–834 CrossRef.
- B. Jenkins, J. West and A. Koulman, A Review of Odd-Chain Fatty Acid Metabolism and the Role of Pentadecanoic Acid (C15:0) and Heptadecanoic Acid (C17:0) in Health and Disease, Molecules, 2015, 20, 2425–2444 CrossRef PubMed.
- K. J. Li, E. M. Brouwer-Brolsma, K. J. Burton-Pimentel, G. Vergères and E. J. M. Feskens, A systematic review to identify biomarkers of intake for fermented food products, Genes Nutr., 2021, 16, 5 CrossRef PubMed.
- V. A. Stallings, J. T. Mondick, J. I. Schall, J. S. Barrett, M. Wilson and M. R. Mascarenhas, Diagnosing malabsorption with systemic lipid profiling: pharmacokinetics of pentadecanoic acid and triheptadecanoic acid following oral administration in healthy subjects and subjects with cystic fibrosis, Int. J. Clin. Pharmacol. Ther., 2013, 51, 263–273 CrossRef CAS PubMed.
- M. C. Playdon, S. C. Moore, A. Derkach, J. Reedy, A. F. Subar, J. N. Sampson, D. Albanes, F. Gu, J. Kontto, C. Lassale, L. M. Liao, S. Mannisto, A. M. Mondul, S. J. Weinstein, M. L. Irwin, S. T. Mayne and R. Stolzenberg-Solomon, Identifying biomarkers of dietary patterns by using metabolomics, Am. J. Clin. Nutr., 2017, 105, 450–465 CrossRef CAS PubMed.
- C. G. Costa, W. S. Guerand, E. A. Struys, U. Holwerda, H. J. ten Brink, I. Tavares de Almeida, M. Duran and C. Jakobs, Quantitative analysis of urinary acylglycines for the diagnosis of beta-oxidation defects using GC-NCI-MS, J. Pharm. Biomed. Anal., 2000, 21, 1215–1224 CrossRef CAS PubMed.
- J. B. Hjerpsted, C. Ritz, S. S. Schou, T. Tholstrup and L. O. Dragsted, Effect of cheese and butter intake on metabolites in urine using an untargeted metabolomics approach, Metabolomics, 2014, 10, 1176–1185 CrossRef CAS.
- P. Galaup, A. Gautier, Y. Piriou, A. d. Villeblanche, A. Valla and L. Dufossé, First pigment fingerprints from the rind of French PDO red-smear ripened soft cheeses Epoisses, Mont d'Or and Maroilles, Innovative Food Sci. Emerging Technol., 2007, 8, 373–378 CrossRef CAS.
- S. Minzer, R. A. Losno and R. Casas, The Effect of Alcohol on Cardiovascular Risk Factors: Is There New Information?, Nutrients, 2020, 12, 912 CrossRef CAS PubMed.
- D. Vancampfort, M. Hallgren, J. Mugisha, M. D. Hert, M. Probst, D. Monsieur and B. Stubbs, The Prevalence of Metabolic Syndrome in Alcohol Use Disorders: A Systematic Review and Meta-analysis, Alcohol Alcohol., 2016, 51, 515–521 CrossRef PubMed.
- F. M. Wurst, N. Thon, M. Yegles, A. Schruck, U. W. Preuss and W. Weinmann, Ethanol metabolites:
their role in the assessment of alcohol intake, Alcohol: Clin. Exp. Res., 2015, 39, 2060–2072 CrossRef CAS PubMed.
- C. L. Crunelle, M. Yegles, A. L. N. V. Nuijs, A. Covaci, M. De Doncker, K. E. Maudens, B. Sabbe, G. Dom, W. E. Lambert, P. Michielsen and H. Neels, Hair ethyl glucuronide levels as a marker for alcohol use and abuse: A review of the current state of the art, Drug Alcohol Depend., 2014, 134, 1–11 CrossRef CAS PubMed.
- F. Pragst, in Hair Analysis in Clinical and Forensic Toxicology, 2015, pp. 71–139. DOI:10.1016/b978-0-12-801700-5.00004-2.
- A. Macedo, S. Gouveia and J. Rebelo, The Global Demand for Alcoholic Beverages, 2010–2015: Price and Expenditure Elasticities, J. Int. Food Agribus. Mark., 2021, 33, 398–422 CrossRef.
- H. Tobe, in Beer in Health and Disease Prevention, ed. V. R. Preedy, Academic Press, San Diego, 2009, pp. 695–702. DOI:10.1016/B978-0-12-373891-2.00070-5.
- I. Domínguez-López, I. Parilli-Moser, C. Arancibia-Riveros, A. Tresserra-Rimbau, M. A. Martínez-González, C. Ortega-Azorín, J. Salas-Salvadó, O. Castañer, J. Lapetra, F. Arós, M. Fiol, L. Serra-Majem, X. Pintó, E. Gómez-Gracia, E. Ros, R. M. Lamuela-Raventós and R. Estruch, Urinary Tartaric Acid, a Biomarker of Wine Intake, Correlates with Lower Total and LDL Cholesterol, Nutrients, 2021, 13, 2883 CrossRef PubMed.
- L. Bavaresco, L. Lucini, M. Busconi, R. Flamini and M. De Rosso, Wine Resveratrol: From the Ground Up, Nutrients, 2016, 8, 222 CrossRef PubMed.
- P. Wang and S. Sang, Metabolism and pharmacokinetics of resveratrol and pterostilbene, BioFactors, 2018, 44, 16–25 CrossRef CAS PubMed.
- P. Flores, P. Hellín and J. Fenoll, Determination of organic acids in fruits and vegetables by liquid chromatography with tandem-mass spectrometry, Food Chem., 2012, 132, 1049–1054 CrossRef CAS.
- J. Regueiro, A. Vallverdú-Queralt, J. Simal-Gándara, R. Estruch and R. M. Lamuela-Raventós, Urinary tartaric acid as a potential biomarker for the dietary assessment of moderate wine consumption: a randomised controlled trial, Br. J. Nutr., 2014, 111, 1680–1685 CrossRef CAS PubMed.
- R. Negrão, D. Duarte, R. Costa and R. Soares, Isoxanthohumol modulates angiogenesis and inflammation via vascular endothelial growth factor receptor, tumor necrosis factor alpha and nuclear factor kappa B pathways, BioFactors, 2013, 39, 608–622 CrossRef PubMed.
- P. Dostálek, M. Karabín and L. Jelínek, Hop Phytochemicals and Their Potential Role in Metabolic Syndrome Prevention and Therapy, Molecules, 2017, 22, 1761 CrossRef PubMed.
- S. Possemiers, S. Bolca, C. Grootaert, A. Heyerick, K. Decroos, W. Dhooge, D. De Keukeleire, S. Rabot, W. Verstraete and T. Van de Wiele, The prenylflavonoid isoxanthohumol from hops (Humulus lupulus L.) is activated into the potent phytoestrogen 8-prenylnaringenin in vitro and in the human intestine, J. Nutr., 2006, 136, 1862–1867 CrossRef CAS PubMed.
- R. B. Van Breemen, Y. Yuan, S. Banuvar, L. P. Shulman, X. Qiu, R. F. R. Alvarenga, S.-N. Chen, B. M. Dietz, J. L. Bolton, G. F. Pauli, E. Krause, M. Viana and D. Nikolic, Pharmacokinetics of prenylated hop phenols in women following oral administration of a standardized extract of hops, Mol. Nutr. Food Res., 2014, 58, 1962–1969 CrossRef CAS PubMed.
- W. Liu, C. Wan, Y. Huang and M. Li, Effects of tea consumption on metabolic syndrome: A systematic review and meta-analysis of randomized clinical trials, Phytother. Res., 2020, 34, 2857–2866 CrossRef CAS PubMed.
- A. Nehlig, Interindividual Differences in Caffeine Metabolism and Factors Driving Caffeine Consumption, Pharmacol. Rev., 2018, 70, 384–411 CrossRef CAS PubMed.
- J. A. Rothwell, F. Madrid-Gambin, M. Garcia-Aloy, C. Andres-Lacueva, C. Logue, A. M. Gallagher, C. Mack, S. E. Kulling, Q. Gao, G. Praticò, L. O. Dragsted and A. Scalbert, Biomarkers of intake for coffee, tea, and sweetened beverages, Genes Nutr., 2018, 13, 15 CrossRef PubMed.
- S. S. Heinzmann, E. Holmes, S. Kochhar, J. K. Nicholson and P. Schmitt-Kopplin, 2-Furoylglycine as a Candidate Biomarker of Coffee Consumption, J. Agric. Food Chem., 2015, 63, 8615–8621 CrossRef CAS PubMed.
- K. A. Guertin, E. Loftfield, S. M. Boca, J. N. Sampson, S. C. Moore, Q. Xiao, W. Y. Huang, X. Xiong, N. D. Freedman, A. J. Cross and R. Sinha, Serum biomarkers of habitual coffee consumption may provide insight into the mechanism underlying the association between coffee consumption and colorectal cancer, Am. J. Clin. Nutr., 2015, 101, 1000–1011 CrossRef CAS PubMed.
- R. Lang, E. F. Yagar, A. Wahl, A. Beusch, A. Dunkel, N. Dieminger, R. Eggers, G. Bytof, H. Stiebitz, I. Lantz and T. Hofmann, Quantitative Studies on Roast Kinetics for Bioactives in Coffee, J. Agric. Food Chem., 2013, 61, 12123–12128 CrossRef CAS PubMed.
- O. Midttun, A. Ulvik, O. Nygard and P. M. Ueland, Performance of plasma trigonelline as a marker of coffee consumption in an epidemiologic setting, Am. J. Clin. Nutr., 2018, 107, 941–947 CrossRef.
- K. Chandrasekaran and D. Karunasagar, Determination of trace elements in the Pb–Bi-eutectic system by inductively coupled plasma-quadrupole mass spectrometry after sequential removal of the matrix by precipitation, J. Anal. At. Spectrom., 2014, 29, 1720–1725 RSC.
- N. Mohamadi, F. Sharififar, M. Pournamdari and M. Ansari, A Review on Biosynthesis, Analytical Techniques, and Pharmacological Activities of Trigonelline as a Plant Alkaloid, J. Diet. Suppl., 2018, 15, 207–222 CrossRef CAS PubMed.
- G. Wadhwa, K. V. Krishna, R. Taliyan, N. Tandon, S. S. Yadav, D. Banerjee, A. Narwaria, C. K. Katiyar and S. K. Dubey, Preclinical pharmacokinetics of trigonelline using ultra–performance liquid chromatography–tandem mass spectrometry and pharmacological studies targeting type 2 diabetes, Sep. Sci. Plus, 2021, 4, 185–194 CrossRef CAS.
- S. Jiang, Y. Zhong, H. Qiao, B. Di, J. Chen and M. Su, UPLC-MS/MS method for the simultaneous quantification of caffeine and illicit psychoactive drugs in hair using a single-step high-speed grinding extraction - Insights into a cut-off value for caffeine abuse, J. Pharm. Biomed. Anal., 2022, 209, 114489 CrossRef CAS PubMed.
- J. Blanchard and S. J. A. Sawers, The absolute bioavailability of caffeine in man, Eur. J. Clin. Pharmacol., 1983, 24, 93–98 CrossRef CAS PubMed.
- K. Chojnacka, A. Zielinska, I. Michalak and H. Gorecki, The effect of dietary habits on mineral composition of human scalp hair, Environ. Toxicol. Pharmacol., 2010, 30, 188–194 CrossRef CAS PubMed.
- C. S. Yang, L. Chen, M. J. Lee, D. Balentine, M. C. Kuo and S. P. Schantz, Blood and urine levels of tea catechins after ingestion of different amounts of green tea by human volunteers, Cancer Epidemiol., Biomarkers Prev., 1998, 7, 351–354 CAS.
- M. J. Lee, Z. Y. Wang, H. Li, L. Chen, Y. Sun, S. Gobbo, D. A. Balentine and C. S. Yang, Analysis of plasma and urinary tea polyphenols in human subjects, Cancer Epidemiol., Biomarkers Prev., 1995, 4(4), 393–399 CAS.
- V. S. Malik and F. B. Hu, Sugar-Sweetened Beverages and Cardiometabolic Health: An Update of the Evidence, Nutrients, 2019, 11, 1840 CrossRef CAS PubMed.
- A. Mortensen, Sweeteners permitted in the European Union: safety aspects, Scand. J. Food Nutr., 2006, 50, 104–116 CrossRef.
- L. Zhang, D. Hou, X. Chen, D. Li, L. Zhu, Y. Zhang, J. Li, Z. Bian, X. Liang, X. Cai, Y. Yin, C. Wang, T. Zhang, D. Zhu, D. Zhang, J. Xu, Q. Chen, Y. Ba, J. Liu, Q. Wang, J. Chen, J. Wang, M. Wang, Q. Zhang, J. Zhang, K. Zen and C. Y. Zhang, Exogenous plant MIR168a specifically targets mammalian LDLRAP1: evidence of cross-kingdom regulation by microRNA, Cell Res., 2012, 22, 107–126 CrossRef CAS PubMed.
- A. A. Millar and P. M. Waterhouse, Plant and animal microRNAs: similarities and differences, Funct. Integr. Genomics, 2005, 5, 129–135 CrossRef CAS PubMed.
- L. Zhang, T. Chen, Y. Yin, C. Y. Zhang and Y. L. Zhang, Dietary microRNA-A Novel Functional Component of Food, Adv. Nutr., 2019, 10, 711–721 CrossRef PubMed.
- G. Liang, Y. Zhu, B. Sun, Y. Shao, A. Jing, J. Wang and Z. Xiao, Assessing the survival of exogenous plant microRNA in mice, Food Sci. Nutr., 2014, 2, 380–388 CrossRef CAS PubMed.
- X. Qin, X. Wang, K. Xu, Y. Zhang, X. Ren, B. Qi, Q. Liang, X. Yang, L. Li and S. Li, Digestion of Plant Dietary miRNAs Starts in the Mouth under the Protection of Coingested Food Components and Plant-Derived Exosome-like Nanoparticles, J. Agric. Food Chem., 2022, 70, 4316–4327 CrossRef CAS PubMed.
- A. Philip, V. A. Ferro and R. J. Tate, Determination of the potential bioavailability of plant microRNAs using a simulated human digestion process, Mol. Nutr. Food Res., 2015, 59, 1962–1972 CrossRef CAS PubMed.
- R. Xing, H. Liu, X. Qi and L. Pan, Measuring the process and rate of exogenous DNA degradation during digestion in mice, Sci. Rep., 2022, 12, 6463 CrossRef CAS PubMed.
- A. Wada and A. Suyama, Local stability of DNA and RNA secondary structure and its relation to biological functions, Prog. Biophys. Mol. Biol., 1986, 47, 113–157 CrossRef CAS PubMed.
- M. Nemati, B. Singh, R. A. Mir, M. Nemati, A. Babaei, M. Ahmadi, Y. Rasmi, A. G. Golezani and J. Rezaie, Plant-derived extracellular vesicles: a novel nanomedicine approach with advantages and challenges, Cell Commun. Signaling, 2022, 20, 69 CrossRef CAS PubMed.
- S. Rome, Biological properties of plant-derived extracellular vesicles, Food Funct., 2019, 10, 529–538 RSC.
- K. Chiang, J. Shu, J. Zempleni and J. Cui, Dietary MicroRNA Database (DMD): An Archive Database and Analytic Tool for Food-Borne microRNAs, PLoS One, 2015, 10, e0128089 CrossRef PubMed.
- J. Cui, in Handbook of Nutrition, Diet, and Epigenetics, 2019, ch. 90, pp. 2219–2232. DOI:10.1007/978-3-319-55530-0_90.
- S. Rome, Use of miRNAs in biofluids as biomarkers in dietary and lifestyle intervention studies, Genes Nutr., 2015, 10, 483 CrossRef PubMed.
- R. A. Wesselhoeft, P. S. Kowalski and D. G. Anderson, Engineering circular RNA for potent and stable translation in eukaryotic cells, Nat. Commun., 2018, 9, 2629 CrossRef PubMed.
- Y. Wang, D. Li, Y. Wang, M. Li, X. Fang, H. Chen and C. Zhang, The landscape of circular RNAs and mRNAs in bovine milk exosomes, J. Food Compos. Anal., 2019, 76, 33–38 CrossRef CAS.
- B. L. Petrone, A. Aqeel, S. Jiang, H. K. Durand, E. P. Dallow, J. R. Mccann, H. K. Dressman, Z. Hu, C. B. Tenekjian, W. S. Yancy, P.-H. Lin, J. J. Scialla, P. C. Seed, J. F. Rawls, S. C. Armstrong, J. Stevens and L. A. David, Diversity of plant DNA in stool is linked to dietary quality, age, and household income, Proc. Natl. Acad. Sci. U. S. A., 2023, 120, e2304441120 CrossRef CAS PubMed.
- C. Manach, D. Milenkovic, T. Van De Wiele, A. Rodriguez-Mateos, B. De Roos, M. T. Garcia-Conesa, R. Landberg, E. R. Gibney, M. Heinonen, F. Tomás-Barberán and C. Morand, Addressing the inter-individual variation in response to consumption of plant food bioactives: Towards a better understanding of their role in healthy aging and cardiometabolic risk reduction, Mol. Nutr. Food Res., 2017, 61, 1600557 CrossRef PubMed.
- K. P. Scott, S. W. Gratz, P. O. Sheridan, H. J. Flint and S. H. Duncan, The influence of diet on the gut microbiota, Pharmacol. Res., 2013, 69, 52–60 CrossRef CAS.
- P. Illiano, R. Brambilla and C. Parolini, The mutual interplay of gut microbiota, diet and human disease, FEBS J., 2020, 287, 833–855 CrossRef CAS PubMed.
- M. A. J. Hullar and B. C. Fu, Diet, the Gut Microbiome, and Epigenetics, Cancer J., 2014, 20, 170–175 CrossRef CAS PubMed.
- S. Raghubeer, The influence of epigenetics and inflammation on cardiometabolic risks, Semin. Cell Dev. Biol., 2023, 154, 175–184 CrossRef PubMed.
- P. Agarwal, T. S. Morriseau, S. M. Kereliuk, C. A. Doucette, B. A. Wicklow and V. W. Dolinsky, Maternal obesity, diabetes during pregnancy and epigenetic mechanisms that influence the developmental origins of cardiometabolic disease in the offspring, Crit. Rev. Clin. Lab. Sci., 2018, 55, 71–101 CrossRef CAS PubMed.
|
This journal is © The Royal Society of Chemistry 2024 |