DOI:
10.1039/D2NR06665C
(Paper)
Nanoscale, 2023,
15, 2756-2766
The bio-inspired heterogeneous single-cluster catalyst Ni100–Fe4S4 for enhanced electrochemical CO2 reduction to CH4†
Received
29th November 2022
, Accepted 26th December 2022
First published on 26th December 2022
Abstract
Electrochemical conversion of CO2-to-CH4 is a process of converting the inert greenhouse gas into energy molecules. It offers great promise for the transformation of carbon-neutral economy. However, achieving high CH4 activity and selectivity remains a major challenge because the electrochemical reduction of CO2-to-CH4 is accompanied by various C1 intermediates at the catalytic site, involving multiple proton-coupled electron transfer processes. Herein, different from the traditional designing strategy, we propose a bio-inspired theoretical design approach to construct a heterogeneous single-cluster catalyst Ni100–Fe4S4 at the atomic level, which may show high CO2 electroreduction performance. Combined with the crystallographic data and theoretical calculations, Ni100–Fe4S4 and CO dehydrogenase exhibit highly similar catalytic geometric active centers and CO2 binding modes. By exploring the origin of the catalytic activity of this biomimetic structure, we found that the activation of CO2 on Ni100–Fe4S4 theoretically exceeds that on natural CO dehydrogenase. Density functional theory calculations reveal that the dehydrogenase enzyme-liked Fe–Ni active site serves as an electron enrichment ‘electro-bridge’ (an electron-rich highly active catalytic site), which can activate CO2 molecules efficiently and stabilize various intermediates in multistep elementary reactions to selectively produce CH4 at a low overpotential (0.13 eV). The calculated CO2 electroreduction pathways are well consistent with the nickel-based catalytic materials reported in experimental studies. Our work showcases and highlights the rational design of high-performance catalytic materials via the biomimetic methodology at the atomic level.
1. Introduction
The global energy environmental crisis is particularly prominent in the 21st century. The excessive consumption of fossil fuels has not only brought about the energy crisis, but also caused global warming,1 water pollution,2 extreme climate change,3 and extinction of biological populations4 due to excessive CO2 emissions. Developing a carbon-neutral strategy provides breakthrough solutions to the global crisis including energy, food, and climate change. Electrochemical CO2 reduction (CO2RR) to useful fuels and value-added chemical feedstocks offers promising pathways for carbon neutrality. However, the rational design of low-cost, efficient, high-selectivity CO2 electrocatalysts remains a great challenge.5,6 Currently, the two main strategies for the design of highly active CO2RR electrocatalysts are to increase the number of active sites on a given electrode and increase the intrinsic activity of each active site.7 They may have transport problems and consume more expensive materials upon increasing the number of active sites on a given electrode.7 In addition, compared with the former strategy, the latter strategy improves from key origins of catalyst activity. However, significant challenges remain in increasing the intrinsic activity of each active site, which requires a dynamic understanding of the atomic structure of the catalyst at the electronic structure level.5 For example, Li et al. cleverly designed a metal–organic framework based bimetallic site catalyst with self-adaptive characteristics,8 which achieved selective CO2 photoreduction to CH4. Zhang et al. designed a carbon nanotube-based nickel phthalocyanine catalyst at the molecular level, achieving selective CO2 reduction.9 Wang et al. designed a Ni–N–C complex catalyst from the electronic structure level by combining theoretical calculation and experiment, achieving efficient CO2RR.10 The electronic structure of the catalyst surface can also be adjusted through surface modification, such as tailoring on the surface of copper-based catalysts for C–C coupling11 and the etching strategy of metal–organic framework materials.12 At the same time, the addition of artificial intelligence provides more ideas for catalyst design. Zhong and Tran et al. combined active machine learning with DFT calculations to develop a catalyst computational design framework.13,14 They further performed approximately 4000 DFT simulations, which combined with experimental testing finally revealed Cu–Al materials with highly efficient CO2-selective reduction properties.14 Therefore, the current design of excellent CO2RR catalysts is extremely challenging, requiring expensive high-throughput calculations, as well as a deep understanding of the microscopic geometry and electronic structure of materials.5,6 In other words, bionics has been gradually applied to the design of various catalysts in recent years.15–20 From the perspective of Shafaat et al., it is highly successful to design efficient CO2 reduction catalysts by combining biological and chemical strategies.15 It is fascinating and highly promising to propose a strategy for the bio-inspired design approach of CO2RR catalysts at the atomic level.
The biological redox conversion of CO2, N2, O2 and H2 is an important process in biogeochemical cycles. Abundant evidence suggests that both the existence of the current biosphere and the origin of the earliest life on the Earth rely on the biological capacity to fix CO2 and convert into living matter.21,22 To date, seven carbon fixation pathways in nature have been discovered. As the workhorses of large-scale CO2 fixation in nature, the Calvin cycle23 combined with photosynthesis was first recognized in 1948, followed by the reverse Krebs cycle24 (reverse TCA cycle) in 1966, the reductive acetyl-CoA pathway25 (Wood–Ljungdahl pathway) in 1972, the 3-hydroxypropionate bicycle26 (3-HP/malyl-CoA cycle) in 1993, the dicarboxylate/4-hydroxybutyrate cycle27 in 2007 (Di-4HB cycle), the 3-hydroxypropionate-4-hydroxybutyrate cycle28 in 2008 (3HP-4HB cycle), and the enoyl-CoA carboxylases/reductases29 in 2016. Among these CO2 fixation pathways in nature, the acetyl-CoA (Wood–Ljungdahl pathway) pathway is the most ancient and relies on metalloenzymes containing transition metal active centers for CO2 activation.30 This kind of ancient metalloenzyme is named Carbon monoxide dehydrogenase/acetyl-CoA synthase (CODHase/ACS). It may be the earliest biological carbon-fixing enzyme evolved by natural thermophilic anaerobic microorganisms in the autotrophic theory of the origin of life,31–33 endowing it with the ability to live in the CO2-rich atmosphere that existed during the Archean Eon.34,35
CO dehydrogenase has been proven to exhibit very good biological activity in CO2 reduction.15,36–38 Herein, inspired by the crystal structure of CO dehydrogenase II39 isolated from Carboxyothermus hydrogenoformans (CODHIICh), we designed a heterogeneous Fe4S4 single-cluster catalyst on the Ni (100) face (Ni100–Fe4S4) for the electrocatalytic reduction of CO2. Compared with gaseous CO2 and adsorbed CO2 in CODHIICh from crystallographic analysis measured in experimental studies,39,40 the adsorbed CO2 on Ni100–Fe4S4 exhibits longer bond lengths and smaller bond angles, which endows Ni100–Fe4S4 with better CO2 activation. Furthermore, charge difference and density of states (DOS) analyses indicated that the electrons of Ni atoms and the Fe4S4 iron–sulfur cluster on the Ni (100) surface aggregated to the heterojunction interface, forming a highly active electron-rich Fe–Ni ‘electro-bridge’ catalytic site, which should be one of the key origins of efficient electrochemical reduction of CO2-to-CH4. The calculation results can well correlate with the nickel-based and FeS-based catalytic materials reported in the recent experiments.41–45 This bio-inspired design of the Ni100–Fe4S4 catalyst well combines the biological advantages of natural enzymes with the concepts of modern catalytic science, opening an avenue for the rational development of efficient artificial carbon fixation catalysts.
2. Computational details
All spin-polarized DFT calculations for periodic material systems were performed with the Vienna Ab initio simulation package (VASP)46,47 using the projector-augmented wave (PAW) method.48 The exchange–correlation function was handled using the generalized gradient approximation (GGA) formulated by the Revised Perdew–Burke–Ernzerhof (RPBE).49 The van der Waals (vdW) interactions are described using the DFT-D3 method in Grimme's scheme.50,51 The interaction between the atomic core and electrons was described by the projector augmented wave method. The plane-wave basis set energy cutoff was set to 500 eV. The Brillouin zone was sampled with a 2 × 3 × 1 grid centered at the gamma (Γ) point for geometry relaxation. The perfect Ni bulk was fully optimized using 16 × 16 × 16 k-point Gamma (Γ) centered Monkhorst–Pack mesh sampling. The Ni (100) facets were modeled using a four-layer 4 × 3 slab containing 96 atoms with 13.91 Å × 10.43 Å in x and y directions, respectively, and a 15 Å vacuum region, ensuring the negligible lateral interaction of the adsorbates. The Fe4S4 cluster absorbs on the Ni (100) surface, with 13.91 Å × 10.43 Å × 20.22 Å simulation boxes containing 107 atoms, the bottom two layers were kept frozen at the lattice position. All structural models with a dynamic magnetic moment were fully relaxed to optimize without any restrictions until their total energy gradient converged to <1 × 10−5 eV, and the average residual forces were <0.02 eV Å−1. Moreover, the Gibbs free energy calculation is performed with the computational hydrogen electrode (CHE) model,52 and the solvent effect is considered with the implicit solvent model implemented in VASPsol.53,54 Position-specific charge values are obtained using Bader analysis.
3. Results and discussion
3.1 Designing catalysts by learning from nature
Nature provides a blueprint to efficiently activate CO2 molecules and selectively generate useful fuels and value-added chemical feedstocks using metalloenzymes. To accelerate high-performance CO2RR catalyst discovery, we propose a method for the rational design of catalysts assisted by ‘nature blueprints’. In this study, a dehydrogenase separated from Carboxyothermus hydrogenoformans (a bacterium isolated from a Russian hotspring55) was used as a ‘nature blueprint’ to aid in the structural design of Ni100–Fe4S4 (Fig. 1). CO dehydrogenases (CODHases) are the biological catalysts for the reversible oxidation of CO2 to CO:39 |  | (1) |
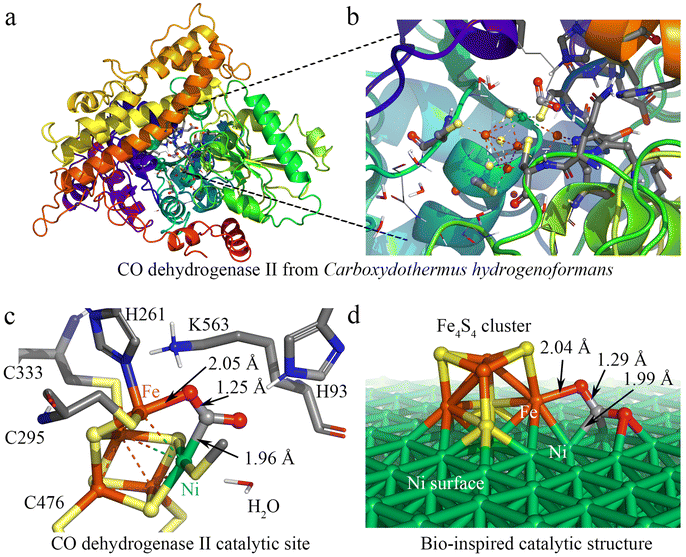 |
| Fig. 1 Design of the Ni100–Fe4S4 catalyst inspired by CO dehydrogenase II. (a) The crystal structure of CODHIICh. (b) The catalytic center of CODHIICh. (c) The structure of the CODHIICh catalytic center combined with CO2. (d) The structure of the bio-inspired heterogeneous Ni100–Fe4S4 catalyst. (In atomic coloring, white is H, gray is C, blue is N, red is O, yellow is S, orange is Fe, and green is Ni). | |
Fig. 1a shows the crystal structure of CO dehydrogenase II, which contains a special asymmetric [Ni-4Fe-5S] cluster. In the detailed crystal structure experimental data,39,56 the structure of CODHIICh with CO2 reveals a triatomic ligand bridging Fe and Ni (Fig. 1b and c). This Fe–Ni bimetallic factor often plays a synergistic role in the catalytic reaction,8,57 which is beneficial for the activation and catalytic conversion of CO2. Based on this special asymmetric [Ni–4Fe–5S] cluster enzymatic active center, we designed a heterogeneous catalyst by loading Fe4S4 single-clusters on the Ni surface. The calculation results show that the adsorption energies (the equation is given in ESI S1†) of the Fe4S4 cluster on the three most common surfaces of nickel58–62 Ni (100), Ni (111) and Ni (110) are −8.05 eV, −7.02 eV and −5.72 eV, respectively (Fig. S1†), indicating that the Fe4S4 cluster tends to adsorb on Ni (100) to form the most stable supported catalysts Ni100–Fe4S4. In addition, the Fe–O bonds (2.04 Å) and Ni–C bonds (1.99 Å) formed after the Ni100–Fe4S4 catalyst adsorbs CO2 are only 0.01 Å and 0.03 Å different from those experimentally measured in CODHIICh (Fe–O 2.05 Å, Ni–C 1.96 Å), respectively (Fig. 1c and d). The similar adsorption bond length means that Ni100–Fe4S4 and CODHIICh have the same CO2 binding mode, indicating that the Ni100–Fe4S4 catalyst may have enzyme-like catalytic activity.
3.2 Activating CO2 beyond nature
The electroreduction of carbon dioxide by the catalyst is preferentially determined by the ability and degree of CO2 activation. To activate CO2 molecules, the active site basically needs to have the ability to adsorb CO2. Since gaseous CO2 molecules exhibit a linear shape and have stable triple-center π bonds (Fig. 2), ordinary physical adsorption hardly changes their geometric and electronic structures. Therefore, the activation of CO2 molecules requires stronger chemisorption, and most forms of chemisorption will change the bond length and bond angle of CO2 molecules, resulting in the splitting of its three-center π bond. The geometric and electronic structures are subsequently changed, exhibiting a metastable activated CO2 molecule.
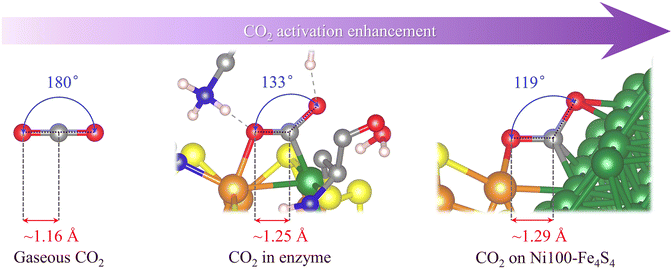 |
| Fig. 2 Schematic diagram of the geometric structure of CO2 in different adsorption states and CO2 activation enhancement. (In atomic coloring, white is H, gray is C, blue is N, red is O, yellow is S, orange is Fe, and green is Ni). | |
As shown in Fig. 2, the crystal structure obtained by rotating anode X-ray diffraction39 shows that the CO2 molecules adsorbed on the catalytically active site of CODHIICh are no longer linear in gaseous CO2. Its ∠O–C–O angle is 133°, and the C–O bond length elongates from 1.16 Å to 1.25 Å compared with gaseous CO2 molecules. Interestingly, in the structure of Ni100–Fe4S4 adsorbing CO2, its ∠O–C–O angle is smaller than that in CODHIICh, only 119°, and the C–O bond is also longer, reaching 1.29 Å. A smaller ∠O–C–O angle and longer C–O bonds indicate that the designed Ni100–Fe4S4, inspired by the active center structure of CODHIICh, exhibits stronger CO2 activation ability.
3.3 Fe–Ni ‘electro-bridge’ enhanced CO2 activation
Bio-inspired strategies to control the performance of CO2 activation focus on the geometric and electronic structures of the active site. We applied this methodology to the design of Ni100–Fe4S4. Fig. 3a shows the top view, front view and side view of the Ni100–Fe4S4 catalyst structure, which was obtained by loading Fe4S4 iron–sulfur clusters onto the Ni (100) surface. The geometric structure is the global energy minimum obtained by spin-polarized first-principles calculations. These iron–sulfur clusters, which are commonly used in biology to transfer electrons, are stabilized on the Ni (100) surface to form a special Ni100–Fe4S4 cluster heterojunction interface structure.
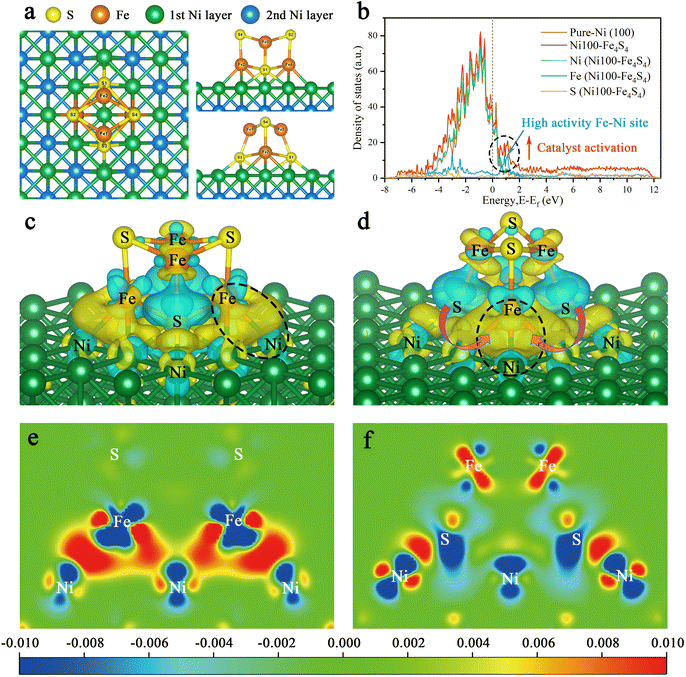 |
| Fig. 3 The geometric and electronic structures of Ni100–Fe4S4. (a) The geometric structure of Ni100–Fe4S4. (b) The projected density of states (PDOS) onto the p and d states of Ni100–Fe4S4. (c and d) The charge difference analysis of the Ni100–Fe4S4 catalyst. (Light blue and yellow stand for the outflow and inflow of electrons, respectively. The black dotted circle represents the Fe–Ni site ‘electro-bridge’. Magenta arrows represent electron flow from S to Fe–Ni sites.) (e and f) The tangent plane of charge difference in the Ni100–Fe4S4 catalyst. (Blue stands for the outflow and red for the inflow of electrons.). | |
To unveil the origin of the structural activity of the Ni100–Fe4S4 heterojunction, we further calculated the projected density of states (PDOS) onto the p and d states of Ni100–Fe4S4 (Fig. 3b). In the PDOS of Ni100–Fe4S4, the peak near the Fermi level is mainly composed of Fe and Ni, implying that Fe–Ni is a highly active site. The Fe–Ni site in Ni100–Fe4S4 mimics the Fe–Ni catalytic sites in CODHIICh, which are crucial for CO2 activation and reaction selectivity. Owing to the interaction of the heterojunction interface between Ni (100) and Fe4S4, the surface Ni atoms and Fe4S4 iron–sulfur clusters could transfer charge to the Fe–Ni site and an optimized polarization field is generated on its surface.
Furthermore, charge difference analysis results show that a large number of electrons are transferred from the Fe4S4 iron–sulfur clusters and the Ni (100) surface to the Fe–Ni site, forming a highly active electron-rich Fe–Ni ‘electro-bridge’ catalytic site on the heterojunction interface (Fig. 3c–f). Here, the role of S atoms is also very important. Through the charge difference calculation, we can observe that most of the charges on the Fe–Ni site come from S (Fig. 3d, magenta arrow), and the S atom acts as the charge supplier of the active site of the catalyst. Therefore, the Fe–Ni site can inject enough electrons into the CO2 2π* antibonding orbitals, disrupting the strong sp-hybridization symmetry of the carbon atom.
3.4 The mechanism of CO2 adsorption and activation
Based on the above designed structure by bio-inspired strategies, the adsorption models for theoretical calculations can be constructed to gain insights into the CO2 adsorption step on Ni100–Fe4S4 from an atomic/electronic-level standpoint. Specifically, the thermodynamic energy conversion, d-band center, work function and electron-density isosurfaces were used to theoretically and systematically reveal the mechanism of the CO2 adsorption step on Ni100–Fe4S4. To shed light on the characteristics and performance of Ni100–Fe4S4 adsorption of CO2, we first analyzed the adsorption energy (the equation is ESI S2†). As depicted in Fig. 4a, Ni100–Fe4S4 shows a much more significant thermodynamic energy conversion of the CO2 adsorption step (−0.56 eV) than pure Ni (100) (−0.44 eV). Pure Ni (100) has been widely proven to be a good CO2 adsorbent both theoretically and experimentally.41,63–65 This means Ni100–Fe4S4 tends to well adsorb CO2 in theory, which is well in line with the d-band center (Fig. 4d, the equation is ESI S3†). The favorable adsorption of CO2 on Ni100–Fe4S4 will accelerate its CO2RR processes and promote its CO2RR activity.
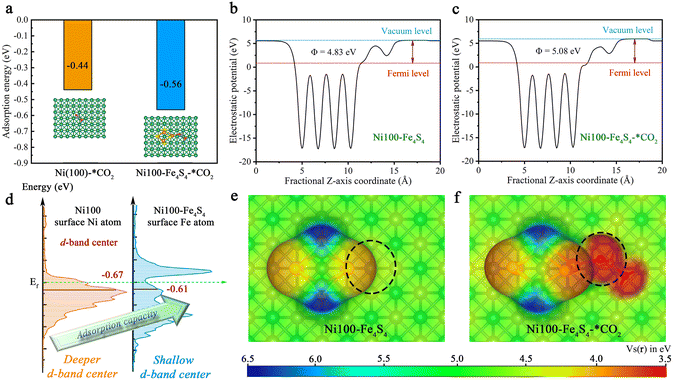 |
| Fig. 4 The adsorption energy, d-band center, surface electrostatic potential and work function analysis of CO2 adsorption. (a) Comparison of the adsorption energies between Ni (100) and Ni100–Fe4S4. (b) The work function of Ni100–Fe4S4. (c) The work function of Ni100–Fe4S4–*CO2. (d) Comparison of the d-band centers between the Ni atom on Ni (100) and the Fe atom on Ni100–Fe4S4. (e) Electron-density isosurface of Ni100–Fe4S4. (f) Electron-density isosurface of Ni100–Fe4S4*–CO2. The color bar stands for the scale of the electrostatic potential and the isosurfaces are plotted at the 0.002 e bohr−3 level. | |
To investigate the electronic structure and surface adsorption behavior on Ni100–Fe4S4, we calculated the electron-density isosurface (Fig. 4b and c) and the electronic work function (Fig. 4e and f) for Ni100–Fe4S4 and Ni100–Fe4S4–*CO2, respectively. The work function is an essential physical property of metallic materials,66 which has been widely used in the design and evaluation of various photo/electrocatalysts,67–69 can be obtained by calculating the difference between the electrostatic potential at the vacuum level Evac and the electrostatic potential at the Fermi level EF using ESI eqn (S4) and (S5).† The detailed electrostatic potential and work function calculation formula are given in ESI S3 and S4.† As shown in Fig. 4b and c, the work function of Ni100–Fe4S4 increases from 4.83 eV to 5.08 eV after CO2 adsorption, which means that the electrons on the surface of Ni100–Fe4S4 flow to the CO2 molecule, thereby increasing the work function of the system. According to previous reports, the surface energy of the system is inversely proportional to the work function.70 Therefore, the adsorption step of CO2 on Ni100–Fe4S4 simultaneously reduces the surface energy of the system and increases the stability of the material surface. Meanwhile, the electron-density isosurface demonstrates that the Fe–Ni sites with a lower electrostatic potential (Fig. 4e, black dotted circle area) on the Ni100–Fe4S4 surface are favorable for the nucleophilic attack on the electrophilic carbonyl carbon of CO2 molecules. In addition, the electrostatic potentials around the isosurface of the O atom of CO2 are at a low level (Fig. 4f, black dotted circle area), indicating a selective hydrogenation site.
Also, we calculated the charge distribution of Ni100–Fe4S4–*CO2. As presented in Fig. 5a, we can clearly observe the electron inflow into the two O atoms of the adsorbed CO2 in Ni100–Fe4S4–*CO2 by the charge density difference. Further Bader charge analysis showed that electrons flowed mainly from Fe atoms to CO2 molecules attributed to the large spin polarization on Fe (Fig. 5b), which is consistent with the electron-density isosurface and work function analysis. Remarkably, in this charge transfer mode, the C atom of the CO2 molecule has a highly positive charge (+2.21e), and the oxygen atoms have highly negative charges (−1.76e and −1.62e), which means that the most negatively charged oxygen atomic site (−1.76e) is more favorable for the subsequent electrocatalytic step to combine with H+. This selectivity of the hydrogenation site is consistent with subsequent Gibbs free energy calculations (Fig. 6, ESI Tables S1 and S4†).
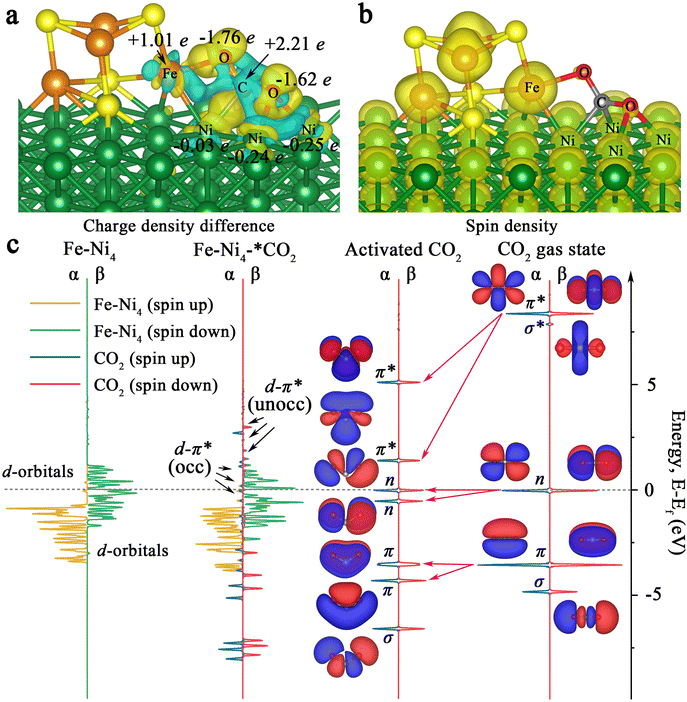 |
| Fig. 5 Associative and activation mechanism analysis. (a) Charge density difference of CO2 adsorption on Ni100–Fe4S4 (Light blue stands for the outflow and yellow for the inflow of electrons.). (b) Spin density of Ni100–Fe4S4 (Yellow stands for spin electrons.). (c) Projected electronic densities of states (PDOS) of FeNi4, CO2 and their interactive configuration FeNi4–*CO2. | |
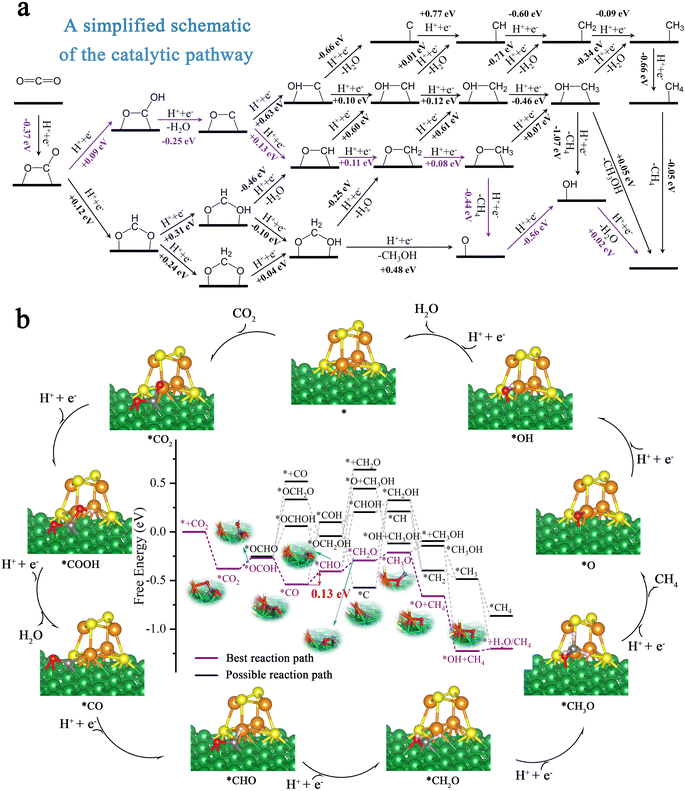 |
| Fig. 6 The CO2RR pathways on Ni100–Fe4S4. (a) A simplified schematic of the free energy changes (ΔG) in the entire possible CO2RR pathways; the energy optimal route is marked in purple. (b) The schematic diagram of the reaction mechanism of CO2 electroreduction to CH4 during the entire CO2 reduction catalytic cycles. | |
To elucidate the bonding nature of the CO2 molecules and Ni100–Fe4S4 involved in the associative mechanism, we further calculated the densities of states (DOS) of Ni100–Fe4S4–*CO2 and Fe–Ni4 sites (five atoms in Ni100–Fe4S4 directly affected by CO2 molecules) for comparison (Fig. 5c). By comparing the DOS and molecular orbitals of the gaseous linear CO2 molecule and the activated bent CO2 molecule, we found that the π, n and π* orbitals of the CO2 molecule were split after activation. The π* orbital splits into two empty orbitals with unequal energy levels in the activated CO2 molecule, which matched well with the Fe–Ni4 polarized β-spin d orbitals near the Fermi level, forming partially occupied d–π* hybrid orbitals. Although the energy levels of the majority α-spin d orbitals of Fe–Ni4 are about 2.0 eV lower than the π* orbitals of activated CO2, the results show that the interaction between the α orbitals and the π* orbitals is obvious. This α-orbital interaction is due to the fact that there are still minority α orbitals present near the Fermi level, matching the π* orbitals of the CO2 molecule. This CO2 activation mechanism reveals that the electrons from the d orbitals of Ni100–Fe4S4 partially transfer to the empty π* orbitals of adsorbed CO2, which is consistent with the charge density difference analysis (Fig. 5a) and DOS analysis (Fig. 5c).
3.5 Reaction mechanism of CO2 electroreduction to CH4
In order to further evaluate the activity and selectivity of Ni100–Fe4S4 to convert CO2* to hydrocarbon species, we studied the free energy changes (ΔG) of the entire CO2 reduction catalytic cycles. We first considered the adsorption pattern of CO2 molecules on the Ni100–Fe4S4 surface (ESI Fig. S2, Table S4†), DFT calculations showed that CO2 molecules tended to be in the C–O site adsorption pattern (ESI Fig. S2c†) rather than the O–O site (ESI Fig. S2a†) adsorption pattern. This adsorption pattern is consistent with previously reported Ni-based materials.63,64 Then, the free energy calculation results (Fig. 6a) confirmed that the first hydrogenation step of the CO2RR is preferentially on O to form COOH* rather than C (OCHO*) due to the low level of electrostatic potentials around the isosurface of the O atom of CO2 (Fig. 4f, black dotted circle area, ESI Table S4†). During the entire hydrogenation steps and reduction mechanism of CO2, the CO2RR on Ni100–Fe4S4 follows the circulation pathway of * → CO2* → COOH* → CO* → CHO* → CH2O* → CH3O* → O* → OH* → H2O* → *, with the final products of CH4 and H2O (Fig. 6b). With this Ni100–Fe4S4 catalyst, the potential determining step (PDS) is CO* → CHO*, and the entire catalytic cycle has a limiting potential of only 0.13 eV, which is much lower than those of most reported CO2RR catalysts in recent years (ESI Table S2†). Therefore, Ni100–Fe4S4 is potentially an efficient CO2RR catalyst producing CH4 at a low overpotential selectively.
3.6 Selectivity for CO2 electroreduction
In order to study the stability of the cluster and the selectivity of the catalyst, we considered side reactions involving the hydrogen evolution reaction (HER) and the H2S evolution reaction (H2SER). The HER is an important competing side reaction, which can constantly consume proton–electron pairs from the electrolyte solution, restraining the faradaic efficiency of the CO2RR significantly.14,71,72 To identify possible HER side-reaction sites, we first calculated Gibbs free energy changes (ΔG) of the HER (* + H+ + e− → H*) on the Fe–Ni, Fe, Ni and S sites (Fig. 7a, ESI Table S4†). According to the Brønsted–Evans–Polanyi relation,73,74 the result shows that the Fe–Ni site with a lower ΔG = −0.15 eV is kinetically more favored due to the smaller reaction barriers. Besides, the calculated results show that hydrogenation is preferentially on O to form COOH* rather than C (OCHO*) both thermodynamically and kinetically (ESI Table S4,†Fig. 4). So, we compare the HER with the first hydrogenation step of the CO2RR (* + CO2(g) + H+ + e− → COOH*). The heatmap diagram of the Gibbs free energy changes (Fig. 7c) indicates that Ni100–Fe4S4 has higher selectivity toward the CO2RR.
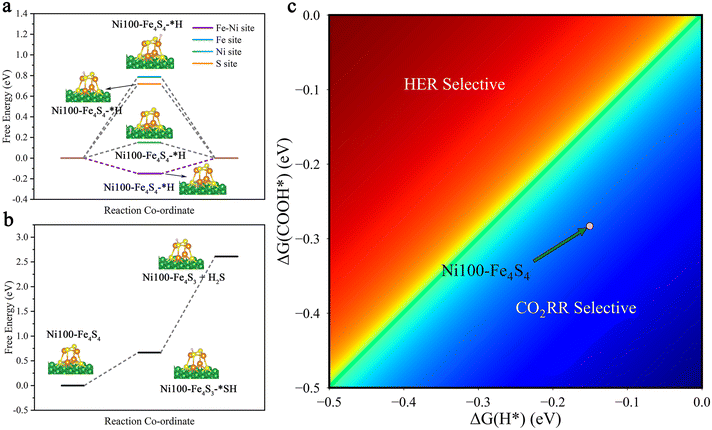 |
| Fig. 7 Selectivity between the CO2RR, the HER and the H2SER. (a) Free energy diagrams of the possible HER mechanisms on the Fe–Ni, Fe, Ni and S sites. (b) Free energy diagrams of the possible H2SER mechanisms on the cluster. (c) Selectivity for the CO2RR vs. HER; the light pink data points represent the Ni100–Fe4S4 catalyst, and the blue region represents higher selectivity to the CO2RR, while the red region to the HER. | |
The H2S evolution reaction is a possible dissociation event on Fe4S4 clusters.75 To test the stability of Ni100–Fe4S4, we calculated and plotted the free energy of the H2SER in Fig. 7b and ESI Table S4.† The calculated thermodynamic energy barrier is quite large ΔG = +1.94 eV, which suggests that the H2SER on Ni100–Fe4S4 is thermodynamically difficult and the reduction of Fe4S4 to Fe in metallic form is more difficult. Therefore, the analysis results of side reactions demonstrate that the Ni100–Fe4S4 catalyst is promising as a cathode material for the CO2RR with high selectivity and faradaic efficiency.
4. Conclusion
In conclusion, we propose a strategy for the bio-inspired design approach of CO2RR catalysts at the atomic level. Taking Ni100–Fe4S4 as an example, we designed this heterogeneous single-cluster catalyst by imitating the spatial structure of the Fe–Ni active center in CO dehydrogenase II from Carboxyothermus hydrogenoformans. We found that Ni100–Fe4S4 exhibits a stronger CO2 activation ability than natural CO dehydrogenase by analyzing the changes in the CO2 adsorption state, geometric structure and electronic structure. This excellent CO2 activation ability of Ni100–Fe4S4 is mainly attributed to the Fe–Ni site designed by the bio-inspired strategy, forming a Fe–Ni ‘electro-bridge’ enhanced CO2 activation. The detailed electronic structure analysis shows that the electrons from the Fe–Ni site's d orbitals partially transfer to the empty π* orbitals of CO2, leading to partial occupation of the formed d–π* orbitals. Moreover, the free energy changes (ΔG) of the entire CO2 reduction catalytic cycles were calculated. A detailed study on the reaction mechanism of CO2 electroreduction shows that CO2RR on Ni100–Fe4S4 follows the circulation pathway of * → CO2* → COOH* → CO* → CHO* → CH2O* → CH3O* → O* → OH* → H2O* → *, with the final products of CH4 and H2O. To be more rigorous, the side reactions involving the hydrogen evolution reaction (HER) and the H2S evolution reaction (H2SER) were also performed and proved to be thermodynamically unfavorable. These promising results show the feasibility of using a Ni100–Fe4S4 catalyst as the cathode for the CO2RR with high selectivity and faradaic efficiency. Therefore, bio-inspired strategies as a transformative approach can be directly applied to heterogeneous catalysts. The clever use of these bio-inspired strategies in modern catalyst design will lead our catalysts beyond their current limitations exploring the final frontier of catalysis.
Author contributions
Hengyue Xu: conceptualization, data curation, formal analysis, investigation, methodology, and writing – original draft. Daqin Guan: formal analysis and writing – review & editing. Lan Ma: project administration, resources, and supervision.
Conflicts of interest
The authors declare no competing interests.
Acknowledgements
This research was funded by the Institute of Biomedical Health Technology and Engineering, Shenzhen Bay Laboratory. We are grateful to the Shenzhen Bay Laboratory Supercomputing Center for providing the high performance computing platform.
References
- P. M. Cox, R. A. Betts, C. D. Jones, S. A. Spall and I. J. Totterdell, Nature, 2000, 408, 184–187 CrossRef CAS PubMed.
- V. Humphrey, J. Zscheischler, P. Ciais, L. Gudmundsson, S. Sitch and S. I. Seneviratne, Nature, 2018, 560, 628–631 CrossRef CAS PubMed.
- I. P. Montañez, N. J. Tabor, D. Niemeier, W. A. DiMichele, T. D. Frank, C. R. Fielding, J. L. Isbell, L. P. Birgenheier and M. C. Rygel, Science, 2007, 315, 87–91 CrossRef PubMed.
- C. D. Thomas, A. Cameron, R. E. Green, M. Bakkenes, L. J. Beaumont, Y. C. Collingham, B. F. Erasmus, M. F. De Siqueira, A. Grainger and L. Hannah, Nature, 2004, 427, 145–148 CrossRef CAS PubMed.
- Z. W. Seh, J. Kibsgaard, C. F. Dickens, I. Chorkendorff, J. K. Norskov and T. F. Jaramillo, Science, 2017, 355, 1 CrossRef PubMed.
- Y. Y. Birdja, E. Pérez-Gallent, M. C. Figueiredo, A. J. Göttle, F. Calle-Vallejo and M. T. M. Koper, Nat. Energy, 2019, 4, 732–745 CrossRef CAS.
- J. D. Benck, T. R. Hellstern, J. Kibsgaard, P. Chakthranont and T. F. Jaramillo, ACS Catal., 2014, 4, 3957–3971 CrossRef CAS.
- J. Li, H. Huang, W. Xue, K. Sun, X. Song, C. Wu, L. Nie, Y. Li, C. Liu, Y. Pan, H.-L. Jiang, D. Mei and C. Zhong, Nat. Catal., 2021, 4, 719–729 CrossRef CAS.
- X. Zhang, Y. Wang, M. Gu, M. Wang, Z. Zhang, W. Pan, Z. Jiang, H. Zheng, M. Lucero, H. Wang, G. E. Sterbinsky, Q. Ma, Y.-G. Wang, Z. Feng, J. Li, H. Dai and Y. Liang, Nat. Energy, 2020, 5, 684–692 CrossRef CAS.
- Z. L. Wang, J. Choi, M. Xu, X. Hao, H. Zhang, Z. Jiang, M. Zuo, J. Kim, W. Zhou, X. Meng, Q. Yu, Z. Sun, S. Wei, J. Ye, G. G. Wallace, D. L. Officer and Y. Yamauchi, ChemSusChem, 2020, 13, 929–937 CrossRef CAS PubMed.
- Z. Zhang, L. Bian, H. Tian, Y. Liu, Y. Bando, Y. Yamauchi and Z. L. Wang, Small, 2022, 18, 2107450 CrossRef CAS PubMed.
- Z. X. Cai, Z. L. Wang, Y. J. Xia, H. Lim, W. Zhou, A. Taniguchi, M. Ohtani, K. Kobiro, T. Fujita and Y. Yamauchi, Angew. Chem., Int. Ed., 2021, 60, 4747–4755 CrossRef CAS PubMed.
- K. Tran and Z. W. Ulissi, Nat. Catal., 2018, 1, 696–703 CrossRef CAS.
- M. Zhong, K. Tran, Y. Min, C. Wang, Z. Wang, C.-T. Dinh, P. De Luna, Z. Yu, A. S. Rasouli, P. Brodersen, S. Sun, O. Voznyy, C.-S. Tan, M. Askerka, F. Che, M. Liu, A. Seifitokaldani, Y. Pang, S.-C. Lo, A. Ip, Z. Ulissi and E. H. Sargent, Nature, 2020, 581, 178–183 CrossRef CAS PubMed.
- H. S. Shafaat and J. Y. Yang, Nat. Catal., 2021, 4, 928–933 CrossRef.
- H.-B. Wang, J.-Q. Wang, R. Zhang, C.-Q. Cheng, K.-W. Qiu, Y.-j. Yang, J. Mao, H. Liu, M. Du, C.-K. Dong and X.-W. Du, ACS Catal., 2020, 10, 4914–4921 CrossRef CAS.
- H. Zhang, L. Huang, J. Chen, L. Liu, X. Zhu, W. Wu and S. Dong, Nano Energy, 2021, 83, 105798 CrossRef CAS.
- P. T. Cesana, B. X. Li, S. G. Shepard, S. I. Ting, S. M. Hart, C. M. Olson, J. I. Martinez Alvarado, M. Son, T. J. Steiman, F. N. Castellano, A. G. Doyle, D. W. C. MacMillan and G. S. Schlau-Cohen, Chem, 2022, 8, 174–185 CAS.
- C. Ren, P. Yang, J. Sun, E. Y. Bi, J. Gao, J. Palmer, M. Zhu, Y. Wu and J. Liu, J. Am. Chem. Soc., 2021, 143, 7891–7896 CrossRef CAS PubMed.
- N. S. Weliwatte and S. D. Minteer, Joule, 2021, 5, 2564–2592 CrossRef CAS.
- R. Braakman and E. Smith, PLoS Comput. Biol., 2012, 8, e1002455 CrossRef CAS PubMed.
- I. A. Berg, D. Kockelkorn, W. H. Ramos-Vera, R. F. Say, J. Zarzycki, M. Hugler, B. E. Alber and G. Fuchs, Nat. Rev. Microbiol., 2010, 8, 447–460 CrossRef CAS PubMed.
- M. Calvin and A. A. Benson, Science, 1948, 107, 476–480 CrossRef CAS PubMed.
- M. Evans, B. B. Buchanan and D. I. Arnon, Proc. Natl. Acad. Sci. U. S. A., 1966, 55, 928 CrossRef CAS PubMed.
- M. Schulman, D. Parker, L. G. Ljungdahl and H. G. Wood, J. Bacteriol., 1972, 109, 633–644 CrossRef CAS PubMed.
- G. Strauss and G. Fuchs, Eur. J. Biochem., 1993, 215, 633–643 CrossRef CAS PubMed.
- I. A. Berg, D. Kockelkorn, W. Buckel and G. Fuchs, Science, 2007, 318, 1782–1786 CrossRef CAS PubMed.
- H. Huber, M. Gallenberger, U. Jahn, E. Eylert, I. A. Berg, D. Kockelkorn, W. Eisenreich and G. Fuchs, Proc. Natl. Acad. Sci. U. S. A., 2008, 105, 7851–7856 CrossRef CAS PubMed.
- T. Schwander, L. S. v. Borzyskowski, S. Burgener, N. S. Cortina and T. J. Erb, Science, 2016, 354, 900–904 CrossRef CAS PubMed.
- S. J. Varma, K. B. Muchowska, P. Chatelain and J. Moran, Nat. Ecol. Evol., 2018, 2, 1019–1024 CrossRef PubMed.
- M. Can, F. A. Armstrong and S. W. Ragsdale, Chem. Rev., 2014, 114, 4149–4174 CrossRef CAS PubMed.
- C. L. Drennan, T. I. Doukov and S. W. Ragsdale, J. Biol. Inorg. Chem., 2004, 9, 511–515 CrossRef CAS PubMed.
- P. S. Adam, G. Borrel and S. Gribaldo, Proc. Natl. Acad. Sci. U. S. A., 2018, 115, E1166–E1173 CrossRef CAS PubMed.
- D. C. Catling and K. J. Zahnle, Sci. Adv., 2020, 6, eaax1420 CrossRef CAS PubMed.
- G. P. Glasby, Episodes, 1998, 21, 252–256 CrossRef PubMed.
- J. Ruickoldt, Y. Basak, L. Domnik, J.-H. Jeoung and H. Dobbek, ACS Catal., 2022, 12, 13131–13142 CrossRef CAS.
- J. Fesseler, J.-H. Jeoung and H. Dobbek, Angew. Chem., Int. Ed., 2015, 54, 8560–8564 CrossRef CAS PubMed.
- D.-H. Nam, P. De Luna, A. Rosas-Hernández, A. Thevenon, F. Li, T. Agapie, J. C. Peters, O. Shekhah, M. Eddaoudi and E. H. Sargent, Nat. Mater., 2020, 19, 266–276 CrossRef CAS PubMed.
- J.-H. Jeoung and H. Dobbek, Science, 2007, 318, 1461–1464 CrossRef CAS PubMed.
- P. J. Ogren, J. Chem. Educ., 2002, 79, 117 CrossRef CAS.
- W. Gac, W. Zawadzki, M. Rotko, M. Greluk, G. Słowik and G. Kolb, Catal. Today, 2020, 357, 468–482 CrossRef CAS.
- M.-A. Serrer, A. Gaur, J. Jelic, S. Weber, C. Fritsch, A. H. Clark, E. Saraçi, F. Studt and J.-D. Grunwaldt, Catal. Sci. Technol., 2020, 10, 7542–7554 RSC.
- T. A. Le, M. S. Kim, S. H. Lee, T. W. Kim and E. D. Park, Catal. Today, 2017, 293–294, 89–96 CrossRef CAS.
- Y. Gao, L. Dou, S. Zhang, L. Zong, J. Pan, X. Hu, H. Sun, K. K. Ostrikov and T. Shao, Chem. Eng. J., 2021, 420, 127693 CrossRef CAS.
- T. G. Senthamaraikannan and D.-H. Lim, J. Phys. Chem. C, 2022, 126, 7012–7021 CrossRef CAS.
- G. Kresse and J. Furthmüller, Comput. Mater. Sci., 1996, 6, 15–50 CrossRef CAS.
- G. Kresse and J. Furthmüller, Phys. Rev. B: Condens. Matter Mater. Phys., 1996, 54, 11169 CrossRef CAS PubMed.
- P. E. Blöchl, Phys. Rev. B: Condens. Matter Mater. Phys., 1994, 50, 17953 CrossRef PubMed.
- B. Hammer, L. B. Hansen and J. K. Nørskov, Phys. Rev. B: Condens. Matter Mater. Phys., 1999, 59, 7413 CrossRef.
- S. Grimme, J. Comput. Chem., 2006, 27, 1787–1799 CrossRef CAS PubMed.
- S. Grimme, J. Antony, S. Ehrlich and H. Krieg, J. Chem. Phys., 2010, 132, 154104 CrossRef PubMed.
- J. K. Nørskov, J. Rossmeisl, A. Logadottir, L. Lindqvist, J. R. Kitchin, T. Bligaard and H. Jonsson, J. Phys. Chem. B, 2004, 108, 17886–17892 CrossRef.
- K. Mathew, R. Sundararaman, K. Letchworth-Weaver, T. Arias and R. G. Hennig, J. Chem. Phys., 2014, 140, 084106 CrossRef PubMed.
- K. Mathew, V. C. Kolluru, S. Mula, S. N. Steinmann and R. G. Hennig, J. Chem. Phys., 2019, 151, 234101 CrossRef PubMed.
- M. Wu, Q. Ren, A. S. Durkin, S. C. Daugherty, L. M. Brinkac, R. J. Dodson, R. Madupu, S. A. Sullivan, J. F. Kolonay, W. C. Nelson, L. J. Tallon, K. M. Jones, L. E. Ulrich, J. M. Gonzalez, I. B. Zhulin, F. T. Robb and J. A. Eisen, PLoS Genet., 2005, 1, e65 CrossRef PubMed.
- H. Dobbek, V. Svetlitchnyi, L. Gremer, R. Huber and O. Meyer, Science, 2001, 293, 1281–1285 CrossRef CAS PubMed.
- Y. Wang, L. Cao, N. J. Libretto, X. Li, C. Li, Y. Wan, C. He, J. Lee, J. Gregg, H. Zong, D. Su, J. T. Miller, T. Mueller and C. Wang, J. Am. Chem. Soc., 2019, 141, 16635–16642 CrossRef CAS PubMed.
- N. Al-Sarraf, J. T. Stuckless and D. A. King, Nature, 1992, 360, 243–245 CrossRef CAS.
- K. Y. Ma, L. Zhang, S. Jin, Y. Wang, S. I. Yoon, H. Hwang, J. Oh, D. S. Jeong, M. Wang, S. Chatterjee, G. Kim, A.-R. Jang, J. Yang, S. Ryu, H. Y. Jeong, R. S. Ruoff, M. Chhowalla, F. Ding and H. S. Shin, Nature, 2022, 606, 88–93 CrossRef CAS PubMed.
- X. Ding, L. De Rogatis, E. Vesselli, A. Baraldi, G. Comelli, R. Rosei, L. Savio, L. Vattuone, M. Rocca, P. Fornasiero, F. Ancilotto, A. Baldereschi and M. Peressi, Phys. Rev. B, 2007, 76, 195425 CrossRef.
- T. Suzuki, T. Yamada and K. Itaya, J. Phys. Chem., 1996, 100, 8954–8961 CrossRef CAS.
- J. Stuckless, C. Wartnaby, N. Al-Sarraf, S. J. Dixon-Warren, M. Kovar and D. King, J. Chem. Phys., 1997, 106, 2012–2030 CrossRef CAS.
- J. Cai, Y. Han, S. Chen, E. J. Crumlin, B. Yang, Y. Li and Z. Liu, J. Phys. Chem. C, 2019, 123, 12176–12182 CrossRef CAS.
- A. Farjamnia and B. Jackson, J. Chem. Phys., 2017, 146, 074704 CrossRef PubMed.
- X. Zhou, B. Kolb, X. Luo, H. Guo and B. Jiang, J. Phys. Chem. C, 2017, 121, 5594–5602 CrossRef CAS.
- N. D. Lang and W. Kohn, Phys. Rev. B: Solid State, 1971, 3, 1215–1223 CrossRef.
- D. Guan, J. Zhong, H. Xu, Y.-C. Huang, Z. Hu, B. Chen, Y. Zhang, M. Ni, X. Xu, W. Zhou and Z. Shao, Appl. Phys. Rev., 2022, 9, 011422 CAS.
- H. Xu and D. Guan, ACS Appl. Mater. Interfaces, 2022, 14, 51190–51199 CrossRef PubMed.
- X. Guo, H. Xu, W. Li, Y. Liu, Y. Shi, Q. Li and H. Pang, Adv. Sci., 2022, 2206084, DOI:10.1002/advs.202206084.
- J. Wang and S.-q. Wang, Appl. Surf. Sci., 2015, 357, 1046–1052 CrossRef CAS.
- X. Hong, K. Chan, C. Tsai and J. K. Nørskov, ACS Catal., 2016, 6, 4428–4437 CrossRef CAS.
- L. Ju, X. Tan, X. Mao, Y. Gu, S. Smith, A. Du, Z. Chen, C. Chen and L. Kou, Nat. Commun., 2021, 12, 5128 CrossRef CAS PubMed.
- J. Bronsted, Chem. Rev., 1928, 5, 231–338 CrossRef CAS.
- M. Evans and M. Polanyi, Trans. Faraday Soc., 1938, 34, 11–24 RSC.
- J. B. Varley, H. A. Hansen, N. L. Ammitzbøll, L. C. Grabow, A. A. Peterson, J. Rossmeisl and J. K. Nørskov, ACS Catal., 2013, 3, 2640–2643 CrossRef CAS.
|
This journal is © The Royal Society of Chemistry 2023 |