DOI:
10.1039/D3MA00390F
(Review Article)
Mater. Adv., 2023,
4, 5094-5125
Response surface methodology: a powerful tool for optimizing the synthesis of metal sulfide nanoparticles for dye degradation
Received
10th July 2023
, Accepted 2nd September 2023
First published on 8th September 2023
Abstract
Metal sulfide nanoparticles (MSNs) have attracted significant attention due to their unique optical, electronic, and catalytic properties. These nanomaterials have great potential for many applications, such as solar cells, sensors, and environmental remediation. In particular, MSNs have demonstrated promising results in the degradation of dyes, which are hazardous pollutants commonly released by industries (textiles, leather, paper, and plastics). This review article provides a comprehensive overview of recent advancements in the synthesis of MSNs and their application in dye degradation. Two optimization approaches, namely, conventional and response surface methodology (RSM), are discussed in detail, highlighting their advantages and limitations. The conventional approach involves varying one parameter at a time, while the RSM approach uses statistical and mathematical tools to model and analyze the relationship between multiple variables and their effects on the desired response. This article also highlights recent research on optimizing the synthesis conditions for various MSNs, such as zinc sulfide (ZnS), copper sulfide, and cadmium sulfide (CdS) using both conventional and RSM approaches. Additionally, this article presents a discussion on the photocatalytic performance of MSNs in the degradation of various organic dyes, including azo, triphenylmethane, and anthraquinone dyes. Overall, this review serves as a valuable resource for researchers working in the field of nanotechnology and environmental remediation.
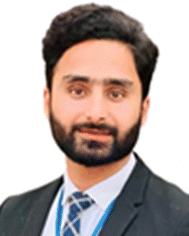
Zeshan Ali Sandhu
| Zeshan Ali Sandhu is a distinguished scientific researcher and scholar in the field of chemistry holding an MPhil degree in chemistry from the University of Gujrat. His academic journey has been marked by exceptional dedication and a passion for advancing the frontiers of scientific knowledge. Zeshan exhibited a keen aptitude for practical application of the theoretical concepts. He has expertise spanning a variety of domains, including photocatalysis, electrocatalysis, energy storage devices, and environment remediation. His interdisciplinary approach and holistic understanding of these areas showcase a unique ability to connect diverse scientific principles. |
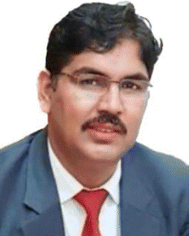
Muhammad Asam Raza
| Dr Muhammad Asam Raza is a distinguished researcher and academician with a strong background in the field of chemistry. He earned his PhD in chemistry from GC University Lahore, Currently, Dr Raza holds the esteemed position of Associate Professor at the University of Gujrat in Pakistan. His research journey has been marked by a profound focus on synthesis of new molecules and their application related to medicinal chemistry. Driven by a commitment to advancing scientific knowledge, he is working on the synthesis of nanomaterials for industrial and environmental applications. Dr Raza's contributions to the academic community are exemplified by his impressive publication record, which boasts more than 100 articles in reputed journals. Beyond his research endeavor, Dr Raza has generously shared his expertise by serving as reviewers for numerous esteemed scientific journals. |
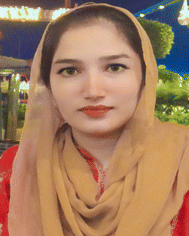
Umme Farwa
| Umme Farwa received her Master's of Philosophy degree from the Department of Chemistry, University of Gujrat, Pakistan in 2021. She is currently doing her PhD in chemistry. Her present research interests are synthesis of heterocyclic organic compounds, nanomaterials, treatment of wastewater, bioenergy production, microbial community analysis, phytotoxity studies, computational studies, and pharmaceutical applications. |
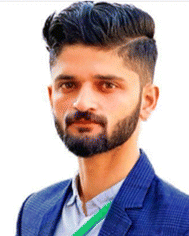
Sufyan Ashraf
| Sufyan Ashraf is a dedicated young researcher pursuing an MPhil in chemistry at the University of Gujrat, Pakistan. His research focuses on the fascinating realm of nanomaterials and their versatile applications in energy storage, biological systems, and photocatalysis. Sufyan's passion for pushing the boundaries of knowledge in these domains drives his commitment to scientific exploration and innovation. Sufyan Ashraf's dedication to research and his promising contributions make him a rising star in the world of chemistry and nanotechnology |
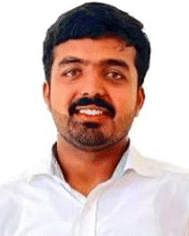
Haseeb Ashraf
| Haseeb Ashraf is an MPhil chemistry scholar at the University of Gujrat, Pakistan. Haseeb's expertise encompasses nanoparticle synthesis, cyclic voltammetry, microbial community analysis, microbial fuel cells and phytotoxicity studies. With a commitment to bridging theory and application, he is poised to make meaningful contributions to the fields of electrochemistry and interdisciplinary research. |
1. Introduction
In recent years, the ever-increasing demand for advanced materials has led to the development of metal sulfide nanoparticles (MSNs) due to their unique optical, electronic, and catalytic properties.1,2 These materials are being used in solar cells, sensors, and environmental remediation.3 There are many ways to synthesize MSNs, such as chemical precipitation, hydrothermal, sol–gel, and solvothermal4–9 methods. Each method has its advantages and limitations which affect the morphology, particle size, and overall performance of the synthesized nanoparticles.10 Among the numerous categories of sulfide photocatalysts,11 NiS2, a semiconductor, has unique electrical, optical, and magnetic characteristics that make it useful in a variety of fields.12 NiS2 has a small band gap of approximately 0.3 eV, which allows it to capture light in the visual range and be used in photocatalytic processes.13,14 Chen et al. (2020) synthesized facile NiS2, which serves as an effective catalyst, enhancing the photocatalytic efficiency of g-C3N4 in H2 production. A simple, low-temperature (80 °C) impregnation technique was devised for NiS2/g-C3N4 photocatalyst creation. In recycling tests, NiS2/g-C3N4(3 wt%) demonstrated consistent, high-performance photocatalytic H2 generation under visible light.15 The activity of metal sulfides can sometimes be limited due to factors such as poor conductivity, insufficient surface area, and inadequate stability. Various approaches have been developed to enhance the activity of metal sulfides.16Fig. 1 illustrates the optimization strategies for enhancing metal sulfide system activity.
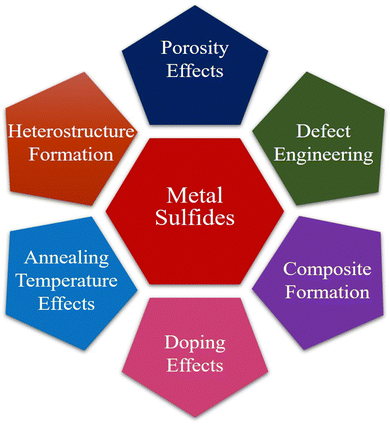 |
| Fig. 1 Systematic methods for boosting performance in metal sulfide systems. | |
Similarly, the preferred substance for photocatalytic and hydrogen evolution has been cobalt sulfide. This material can be artificially tailored for the elimination of organic pigments via visible light photocatalysis and adsorption by shrinking its size to the nanodomain. This material's band gap, phase, and shape can all be adjusted in this way.17 Efficient CoS2, Co3S4, and Co9S8 hollow nanospheres (HNSs) were synthesized by Li et al. (2020) for use as peroxymonosulfate (PMS) activators in ciprofloxacin18 degradation. The CoS2 HNS/PMS system's performance was investigated by varying operating parameters. CoS2 HNSs demonstrated strong catalytic activity across a pH range of 3–10. At an initial pH of 8.0, 10 mg L−1 CIP was entirely removed by CoS2 HNSs within 3 minutes, achieving 62.6% CIP mineralization.19 Comparably, as a member of the family of metal sulfides, cadmium sulfide (CdS) is an intrinsic n-type semiconductor substance. With its narrow band gap energy (2.42 eV), CdS is a perfect visible photocatalyst in the visible region.20,21Fig. 2 presents the hydrothermal treatment for the synthesis of CdS nanoflowers. The energy bands of some of the most extensively researched metal sulfides are presented in Fig. 3.
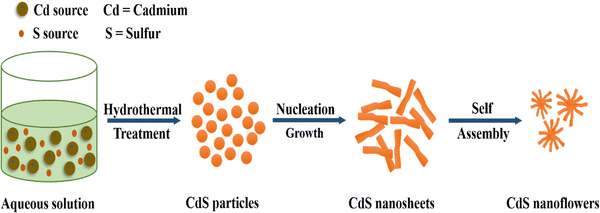 |
| Fig. 2 Exploring CdS nanoflower formation through the hydrothermal treatment technique. | |
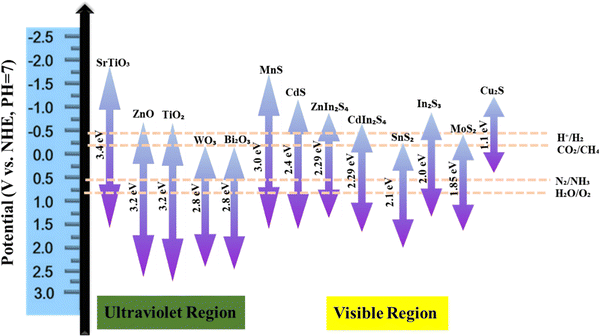 |
| Fig. 3 Energy band gap values of key metal sulfide nanoparticles. | |
The introduction of large amounts of dyes into both natural water and waste water treatment systems results from the discharge of various dyes from various sources, such as the paper and pulp, textile and intermediate industries.22,23 One of the critical applications of MSNs is in the degradation of dyes, which are hazardous pollutants. The presence of dyes in aquatic ecosystems can cause severe damage to the environment and aquatic life, making it crucial to explore effective techniques for dye removal.24 Physical, chemical, and biological approaches have been developed to minimize the amount of toxins in the environment.25 However, these methods were ineffective because the toxins had not been broken down. Instead, they were just turned into other species, which led to more pollution. In recent years, MSNs have gained interest as photocatalysts for the removal of different organic dyes.26 The use of nanoscale catalysis and photocatalysis with nanoparticles (NPs), nanowires (NWs), or nanotubes (NTs) in wastewater treatment processes has recently shown promise in addressing some of the key issues related to heavy metals, pigments, and contaminants. The size and shape of nanoparticles play an important role in photocatalytic applications.27,28
Due to heavy water contamination from different industries, scholars and scientists have redirected their attention towards the exploration and advancement of effective and environmentally friendly approaches to eliminate pollutants.29 Mostly, the photocatalytic reaction is optimized using the conventional kinetic approach, which investigates the effect of reaction parameters (including photocatalyst concentration, dye molecule concentration, irradiation time, and pH) on the response by varying one reaction parameter and measuring its effect on degradation while keeping all other reaction parameters constant.30 Using traditional trial-and-error methods is often a long process that uses a lot of resources and may not lead to the desired results. Recently, an innovative technique for the optimization of these photocatalytic processes called response surface methodology (RSM) has evolved. This method makes use of quantitative and statistical models. This optimization method assumes that all response factors are independent of each other and have no connection.31 As it includes interaction factors that take the connection between response parameters into consideration, this method is better than the traditional optimization method. Because of this sophisticated method, the reaction is further optimized.32 The present discourse aims to elucidate the profound importance of response surface methodology (RSM), a statistical and mathematical approach that facilitates the methodical enhancement of intricate processes.33 The utilization of RSM facilitates the systematic manipulation of various factors that impact the synthesis process, thereby enabling a comprehensive examination of their interrelationships. This approach proves invaluable in the identification of optimal conditions that yield improved properties of nanoparticles for the purpose of dye degradation.34
Recent research has focused on adjusting the nanostructure of metal sulfide nanoparticles to improve their photocatalytic activity. The surface area and light absorption characteristics are influenced by size, shape, and morphology. One option is to create hierarchical structures, such as core–shell or heterostructure topologies, which encourage charge separation and the use of a wider spectrum of light.35,36 Doping metal sulfide nanoparticles with different elements may improve their catalytic capabilities significantly. Incorporating transition metals or nonmetals, for example, may enhance charge carrier mobility and increase light absorption. Metal sulfides with varied compositions may also be allowed to control their bandgap, resulting in increased visible light utilization and photocatalytic activity.37 Surface engineering holds significance in enhancing catalytic activity. Surface modifications such as co-catalysts, organic ligands, or plasmonic nanoparticles may improve charge transfer kinetics while decreasing recombination rates. These changes also improve the nanoparticles' durability under strong reaction conditions.38 The creation of heterojunctions between metal sulfide nanoparticles and other semiconductors or materials, such as graphene, improves charge carrier separation and migration. This material synergy results in excellent electron–hole separation and enhanced photocatalytic activity.39
The review offers a thorough understanding of metal sulfide nanoparticles, their synthesis methods, the mechanisms involved in dye degradation, and the effectiveness of RSM as a powerful statistical tool for optimizing the parameters affecting metal sulfide-based dye degradation processes. To our best knowledge, this review is the first detailed report on the RMS regarding the synthesis of nanomaterials and their application in photocatalytic processes. This study will be the new horizon for the people working on the synthesis of such materials and their uses.
2. Metal sulfide nanoparticles
Metal sulfide nanoparticles refer to nanoscale particles composed of metal and sulfur atoms.40,41 These nanoparticles exhibit unique optical, electrical, and chemical properties. Their small size and high surface area-to-volume ratio make them excellent candidates for catalytic reactions and energy storage applications.42 Metal sulfides are of three main types: (a) pure metal sulfides, (b) doped metal sulfides, and (c) composite metal sulfides.
Those purely composed of a single metal exhibit catalytic, magnetic and electrochemical properties.43,44 The structural, electrochemical, magnetic, photo-catalytic and catalytic properties of metal sulfides can be enhanced, when doped metal chalcogenides are used.45 In this process, binary metals are used, with one present in high concentration and other in a small concentration.46 Different metals like Ce, Sr, Cu, Co, etc. can be used as a dopant. A metal sulfide nanocomposite refers to a material composed of two or more distinct components, with one of the components being a metal sulfide.47
3. Synthetic approaches for metal sulfide nanoparticles
Two common methods for synthesizing metallic nanoparticles are top–down and bottom–up approaches. These methods differ primarily in the type of precursor used for nanoparticle preparation.48 The top–down approach employs bulk materials as precursors,49 while the bottom–up approach utilizes atoms or molecules.50 Understanding these differences is essential for tailoring nanoparticle synthesis and properties for specific applications. Fig. 4 represents the synthetic approaches for metal sulfide nanoparticles.
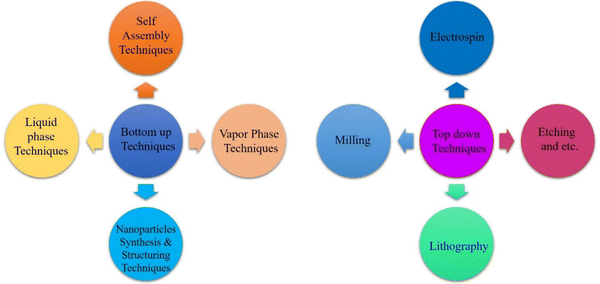 |
| Fig. 4 Systematic techniques for the synthesis of metal sulfide nanoparticles. | |
3.1. Top–down method
The preparation of small nanosized particles involves the breakdown of bulk precursor material using various physical methods, such as sputtering, grinding, milling, thermal or laser ablation.51–55 These processes result in the formation of nanoparticles with reduced dimensions compared to the original bulk material.56 Top–down methods are categorized into two type of methods:
Scotch tape method.
In this method, there is no chemical reaction involved and it is therefore called a non-destructive method.57 This is a simple process that results in the production of nanoflake-like particles.58
Physical method.
Various physical methods like mechanical milling,59 ultra-sonication or liquid phase exfoliation60 and electron spray pyrolysis61 are used.
3.1.1. Mechanical milling.
It is an inexpensive method for producing NPs from bulk and kinetic energy is transferred from the grinding medium to the material during reduction.62 Reduction in particle size with high energy ball mining is the working principle involved in this method (Fig. 5).63 NPs are produced by adding bulk powder and heavy balls that rotate at high speed in a container, resulting in the production of small size particles. Meng et al. (2021) reported the synthesis and optimization of a photocatalyst with a high-efficiency MoS2 modification; this catalyst was composed of metal-free graphitic carbon nitride (g-C3N4), black phosphorus (BP), and MoS2 (MCN/BP/MS). The optimized MCN/BP/MS photocatalyst exhibited excellent performance in hydrogen evolution, with a degradation rate of 100% in 25 min.64
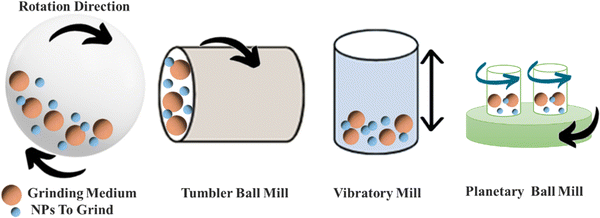 |
| Fig. 5 Different mechanisms for mechanical ball milling. | |
3.1.2. Liquid-phase exfoliation.
Exfoliation methods are efficient, low cost, result in fewer by-products and are convenient.58 It is also one of the top–down methods in which solvent is scattered with a bulk of material. By means of mechanical processing techniques, such as ultra-sonication, 2D flake-like particles are generated through the exfoliation of the material. DMF is used as a solvent in this method. This approach is used for the fabrication of monolayer nanosheets with a thickness of <1 nm.65 Hadi et al. (2018) explored a new method to increase the output of liquid-phase exfoliation of graphite to graphene sheets. The technique involves utilizing magnetic Fe3O4 nanoparticles as a “particle wedge” to aid in delaminating graphitic layers (Fig. 6). The use of Fe3O4 particles and ultrasonic waves generates strong shear forces, leading to improved exfoliation of graphite. This approach provides high-quality graphene sheets, and the separation of Fe3O4 particles from the graphene solution is easily accomplished due to the magnetic properties of Fe3O4 nanoparticles.66
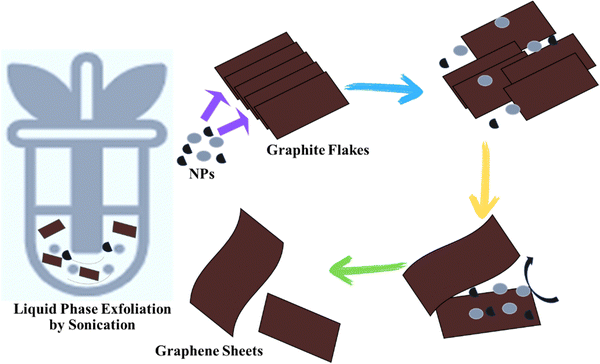 |
| Fig. 6 Nanomaterials for delaminating graphitic layers via the liquid phase exfoliation method. | |
3.1.3. Electrospray pyrolysis.
This method involves the delivery of precursors in the gaseous form into a heated reactor.67 The precursor is facilitated using a nebulizer system, which comprises a fluid nebulizer, a vertical tubular reactor, and a precipitator.56 Lee et al. (2021) studied Au/ZnO to control the electron–hole recombination of the photocatalyst. The ultrasonic spray pyrolysis method was employed to generate Au/ZnO particles under different conditions (Fig. 7). The photocatalytic decomposition rate of rhodamine-B was faster than that of ZnO in all Au/ZnO particles, with the best photocatalytic performance observed in particles containing 0.1 mass% Au supported on ZnO. Additionally, optimal photolysis activity occurred when 10 mg of Au/ZnO particles was added to 100 mL of a 5 mg L−1 RhB aqueous solution.68
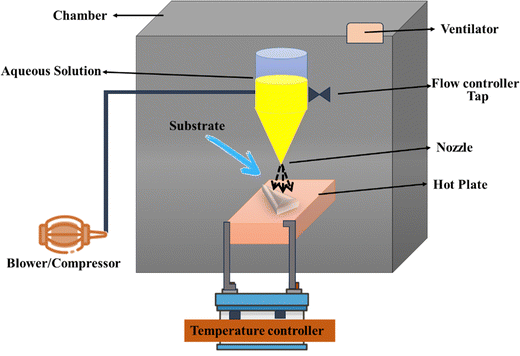 |
| Fig. 7 A complete setup for electrospray pyrolysis. | |
3.2. Bottom–up method
In this approach, small particles, such as atoms or molecules, are merged to prepare nanoparticles (NPs). NPs are synthesized using chemical and biological techniques.69Fig. 8 illustrates the general mechanism of bottom–up and top–down approaches for metal sulfide nanoparticles.
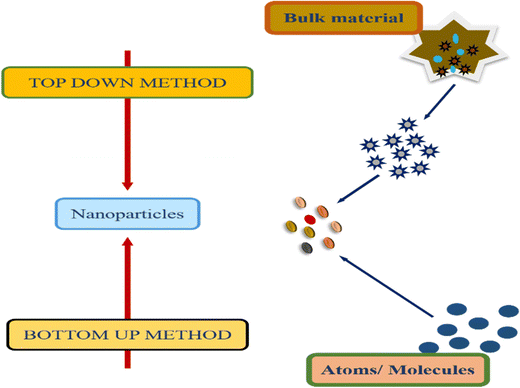 |
| Fig. 8 General mechanism of bottom–up and top–down approaches. | |
3.2.1. Chemical methods for nanoparticle synthesis.
Chemical methods are superior in terms of the rate of production and the production of nanoparticles with specific and controllable sizes. Metallic precursors, stabilizing agents and reducing agents are mainly used in chemical methods.70
Hydrothermal method.
The synthesis of metal sulphides through the hydrothermal method needs a closed system with regulated temperature and pressure.71 This is a low cost method for the synthesis of MoS2 NPs. Sodium molybdate is used as the Mo precursor while thiourea and thioacetamide are used as sulphur sources.72 This eco-friendly approach eliminates the need for pH control and complexing agents. Through this method, FeS2,73 CuS,74 Ag2S,75 MoS,76 and CdS77 are prepared. NPs are synthesized by heating a mixture of metal salts and sulfide sources. The commonly used metal sources include chlorides or nitrates, while organic sulfides serve as sulfide sources. This technique primarily generates nanoparticles in an autoclave. The synthesis of Ag2S nanoflowers via hydrothermal treatment methodology is depicted in Fig. 9.
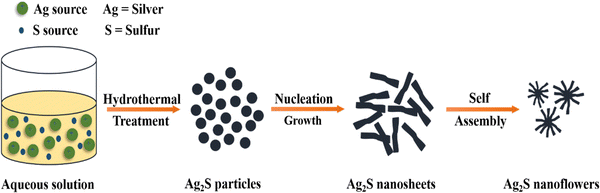 |
| Fig. 9 Synthetic representation of Ag2S nanoflowers by the hydrothermal method. | |
Amral et al. (2022) synthesized MoS2 nanoflower-like nanoparticles by setting the autoclave temperature at 180 °C for 20 hours and utilizing molybdenum oxide and potassium thiocyanate as precursors. Temperature and duration have a significant influence nanoparticle architecture, with coral-like structures observed at 150 °C for 25 hours and nanosheet structures with extensive surface areas obtained at 240 °C for 47 hours.58 Wang et al. (2023) prepared ZnS/TiO2 heterojunction photocatalysts by hydrothermally depositing ZnS nanoparticles on TiO2 nanofibers. Fig. 10 illustrates the synthesis of MoS2 nanoflowers by using a hydrothermal method.
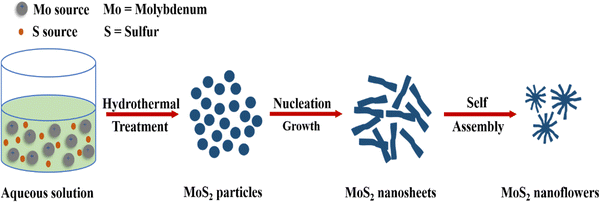 |
| Fig. 10 Systematic representation of hydrothermal technique for the synthesis of MoS2 nanoflowers. | |
Microwave-assisted method.
In this approach, there is an interplay between the material and microwaves, transmitting heat energy from the exterior to the interior, resulting in a thermal response. Internal heat generation within the reaction chamber, rather than external induction, is observed (Fig. 11). Microwave reactors offer a safer, greener alternative to conventional oil bath heating.78 Thermally decomposing metal acetates produce PbS79 and CdS nanoparticles.80 Iravani et al. (2014) synthesized CdS through a preparation method that involves dissolving cadmium acetate and thiourea in ethylene glycol, adding NaOH as a capping agent, and evaporating the solvent through microwave irradiation. The resulting NPs are collected after cooling, washing, and drying.81
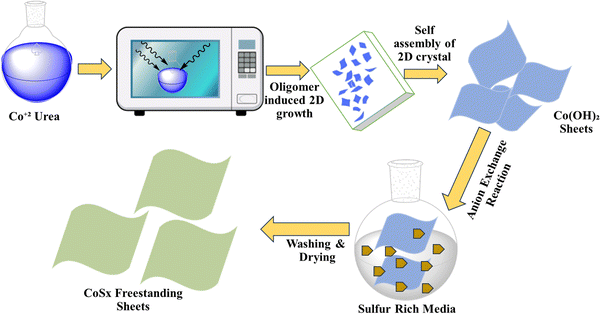 |
| Fig. 11 Synthesis of CoSx using a microwave-assisted approach. | |
Solvothermal method.
In this procedure, metal sulfide nanoparticles are synthesized within a sealed system, involving chemical reactions between precursors in a solvent environment.41,71 This technique necessitates the use of solvents in precursor reactions, with DMF and water being the primary choices for this purpose. The key factors influencing reaction control and product size include temperature, solvent, duration, and precursors.82 The solvothermal approach, a simple technique for synthesizing NPs with various shapes, is employed to produce MoS2/rGO NPs. This cost-effective method, featuring a brief processing time, is ideal for creating metal sulfides with minimal crystallite size. Sambathkumar et al. (2021) studied the Bi[DTC]3 complex and hexadecylamine (HDA), which were combined in a 1
:
1 weight ratio and dispersed in 100 mL of dimethylformamide (DMF). The Bi2S3 product was collected, dried overnight in an oven at 60 °C, and analyzed using various analytical techniques (Fig. 12).83
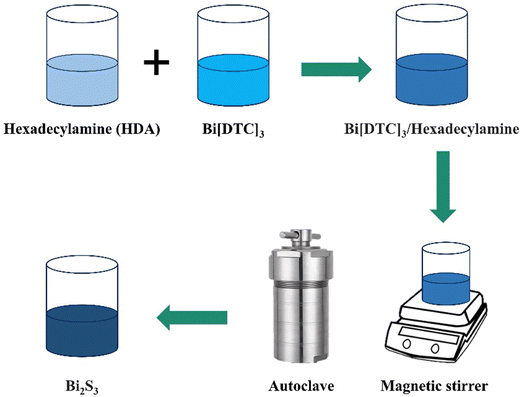 |
| Fig. 12 Synthesis of Bi2S3 by a solvothermal method. | |
Precipitation method.
This is a simple synthetic method for the preparation of metal sulphide NPs and does not need extreme conditions. In2S3 has been synthesized by this method using Na2S·3H2O as a precipitating agent.84 Afsheen et al. (2020) prepared CuS nanoparticles (NPs) through a method that employed Na2S as the sulfur donor and Cu(OAc)2 as the metallic precursor. Various metal sulfide NPs, such as AgS,85 PbS,86 Bi2S,87 and Au/Ag2S,88 were generated using this approach. For the preparation of Ag2S, AgNO3 was dissolved in toluene and oleyamine, with stirring for 60 min at room temperature, resulting in the formation of Ag2S NPs.10
Chemical vapour deposition method.
In this approach, film generation occurs via the deposition of gaseous molecules through chemical reactions, which is essential for film creation. Film growth in CVD systems relies on substrate characteristics and surface processing (Fig. 13).89 A number of methods are used for depositions, including photo-initiated chemical vapour deposition,90 plasma enhanced chemical vapour deposition91 and thermally active chemical vapour deposition method.92 In the CVD technique, precursors amass to create nanosheets, generating a significant nanoparticle yield at minimal cost. High-purity materials are deposited, and CVD necessitates a lower vacuum than PVD methods.93 CVD is a very effective approach for the preparation of monolayer transition metal chalcogenides.94
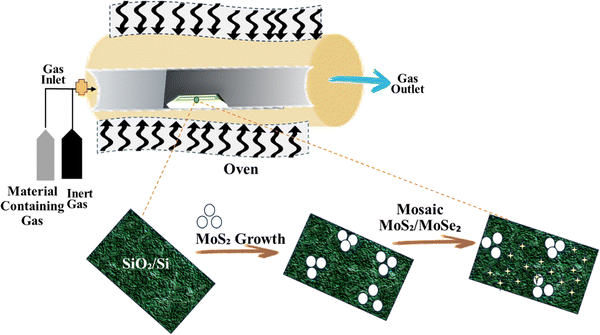 |
| Fig. 13 Synthesis of MoS2/MoSe2 by chemical vapor deposition. | |
3.2.2. Biosynthesis approach.
Biosynthesis of metal sulfides by bacteria, fungi, algae and actinomycetes has garnered immense attention in the field of nanotechnology.95 Bacteria possess the capability to reduce metallic ions into nanoparticles and are one of the most suitable candidates for metal sulfide nanoparticles because of their manageable nature and high growth rate. Metal complexation typically involves bacterial cell wall functional groups like hydroxyl, amine, and carbonyl groups.71 Fungal synthesis presents challenges compared to bacterial methods due to difficulties in manipulation and increased intracellular absorption.96 However, fungi exhibit superior productivity and metal tolerance.97 Economical and efficient nanoparticle production (Fig. 14), such as CdS98 and ZnS,99 is achievable through Fusarium oxysporum's extracellular process, owing to its enhanced bioaccumulation of metal ions.69 Recent studies reveal that besides fungi, yeast is utilized for biogenic synthesis due to its innate ability to assimilate toxic metal ions from the environment. Yeast cells can adapt to metal toxicity through detoxification processes. A marine ascomycetous yeast, Yarrowia lipolytica, produces silver nanoparticles.100 The advantages and disadvantages of the different methods have been listed in Table 1. The various properties of metal sulfide nanoparticles have been listed in Table 2.
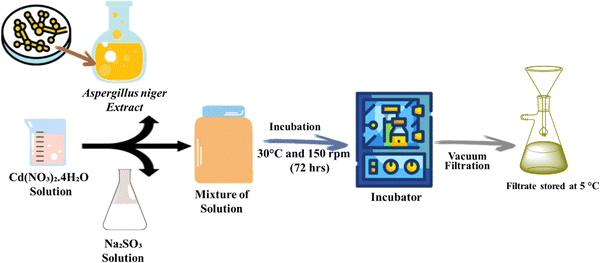 |
| Fig. 14 Synthesis of CdS NPs using the extract of Aspergillus niger. | |
Table 1 Advantages and disadvantages of different nanoparticle synthesis methods
Method |
Advantages |
Disadvantages |
Ref. |
Mechanical milling |
Scalable and relatively simple process |
High energy consumption |
56
|
Suitable for brittle materials |
Potential contamination from milling media |
Liquid phase exfoliation |
Scalable and versatile |
Yield can vary depending on material |
101
|
Suitable for 2D materials |
May require surfactants for stability |
Electro spray pyrolysis |
Precise control over particle size |
Complex equipment and operation |
102
|
High uniformity |
Limited to certain materials |
Hydrothermal method |
Mild conditions |
Limited to certain compositions |
103
|
Narrow size distribution |
Long processing times |
Microwave assisted method |
Rapid heating and synthesis |
Limited to specific materials |
104
|
Enhanced reaction rates |
Potential hotspots and uneven heating |
Solvothermal method |
High purity and crystallinity |
High pressure and temperature required |
105
|
Controlled morphology |
Long synthesis times |
Precipitation method |
Simple and cost-effective |
Limited control over particle size |
106
|
Suitable for bulk production |
May require additional purification |
Chemical vapor deposition |
Precise control over film thickness |
Complex setup and operation |
107
|
High purity and uniformity |
Limited to thin films |
Biosynthesis approach |
Environmentally friendly |
Variable product quality |
108
|
Biocompatible nanoparticles |
Limited scalability |
Table 2 Comparative analysis of metal sulfide properties
Metal sulfide |
Crystal structure |
Color |
Melting point (°C) |
Band gap (eV) |
Electrical conductivity |
Solubility |
Ref. |
FeS2 |
Cubic |
Pale yellow |
1065 |
0.95–1.2 |
Semiconductor |
Insoluble |
109
|
PbS |
Cubic |
Gray |
1123 |
0.36 |
Poor conductor |
Insoluble |
10
|
HgS |
Hexagonal |
Red |
580 |
∼1.6 |
Insulator |
Insoluble |
110
|
Cu2S |
Monoclinic |
Gray-black |
1035 |
∼0.9 |
Semiconductor |
Insoluble |
111
|
Sb2S3 |
Orthorhombic |
Gray |
550 |
∼1.1 |
Poor conductor |
Slightly soluble |
112
|
CuS |
Hexagonal |
Indigo blue |
∼500 |
∼1.5 |
Semiconductor |
Insoluble |
113
|
ZnS |
Hexagonal |
White |
1182 |
3.5–3.8 |
Semiconductor |
Insoluble |
114
|
NiS |
Hexagonal |
Greenish |
∼797 |
∼1.3 |
Semiconductor |
Slightly soluble |
115
|
MoS2 |
Hexagonal |
Dark gray |
1850 |
∼1.2 |
Semiconductor |
Insoluble |
116
|
4. Synthetic parameters of metal sulfide nanoparticles
Metal sulfide nanoparticles refer to nanoscale particles composed of metal and sulfur atoms.40 These nanoparticles exhibit unique optical, electrical, and chemical properties.117 Huerta et al. (2018) synthesized PVP capped CdS nanoparticles via hydrothermal method. The synthesized nanoparticles exhibit flower-like morphology.1Table 3 presents synthetic characteristics of metal sulfide nanoparticles.
Table 3 Key synthetic parameters and physicochemical properties of metal sulfide nanoparticles
Sr. no. |
Metal sulfide NPs |
Method |
Metal precursor |
Morphology |
Size (nm) |
Ref. |
01. |
PVP capped-CdS |
Hydrothermal |
Cd(NO3)2·4H2O, CH4N2S |
Flower-like |
— |
1
|
02. |
CdS/Ag |
Hydrothermal |
Cd(O2CCh3)2(H2O)2, AgNO3 |
Spherical |
28.3 |
118
|
03. |
CdS-Ag2S |
Co-precipitation |
CdCl2, Na2S2O3 |
— |
— |
119
|
04. |
ODA-CdS |
Hydrothermal & chemical |
Cd(S2CNR2)2 |
Sphere |
1.92–5 |
120
|
05. |
CdS/PVA |
Precipitation deposition |
CdAc |
Nematic |
4–8 |
121
|
06. |
CdS |
Hydrothermal |
Cd(NO3)2·4H2O, Na2S·9H2O |
Hexagonal |
— |
122
|
07. |
CuS–TiO2 |
Photochemical |
CuSO4,Na2S2O3 |
— |
2.75 ± 0.8 |
123
|
08. |
Cu2SnS3 |
Hydrothermal |
H2N–CH(SH)–CH2–COOH |
Pin-like |
∼40 |
27
|
09. |
CuS |
— |
Cu(C5H10NS2)2 |
Hexagonal covellite |
31.47 |
124
|
10. |
CuS-QDs/ZnO |
Hydrothermal |
Cu(CH3COO)2 |
Sphere like |
4 |
125
|
11. |
CdS/ZnO |
Deposition |
C4H6O2Cd.H2O |
Hexagonal crystal |
400–200 |
126
|
12. |
Ge–Ce–CuS |
Hydrothermal |
Cu(C2H3O2)2·H2O, Ce(NH4)2(NO3)6 |
Hexagonal |
100–200 |
127
|
13. |
Ni-Cd-S/rGO |
Hydrothermal |
Cd(NO3)2, Ni(NO3)2·6H2O |
Cauliflower |
18 nm |
128
|
14. |
CdS |
Hydrothermal |
C2H5NS, Cd(NO3)2·5H2O |
Hexagonal |
66.28 ± 12.95 |
129
|
15. |
CdS |
Mechanochemical |
Cd(CH3COO)2, Na2S |
Hexagonal |
10 |
130
|
16. |
CuS |
Solvothermal |
CuCl2,CH4N2S |
Spherical |
10–12 |
131
|
17. |
CNT/CuS |
Coprecipitation |
Cu(NO3)2 |
Flake like & tubular |
10–25 |
132
|
18. |
CuS/In2S3 |
Solvothermal |
Cu(C5H7O2)2 |
— |
6.34 ± 0.31 |
78
|
19. |
CdS |
Green synthesis |
CdCl2,Na2S |
Hexagonal |
50–180 |
133
|
20. |
CdS |
Hydrothermal |
CdCl2 |
Nanopetals |
|
134
|
21. |
ZnFe/CuS |
Hydrothermal |
CuSO4·5H2O, Fe(NO3)·9H2O |
Cubic shaped |
26–64 |
135
|
22. |
CdS |
Hydrothermal |
Cd(NO3)2·4H2O, Na2S·9H2O |
Hexagonal |
500 |
136
|
23. |
RE-CdS |
— |
CdCl2 |
Wurtzite |
10 ± 2 |
137
|
24. |
CdS/SnO2 |
Hydrothermal & co-precipitation |
Cd(NO3)2, Na2S |
— |
18 |
138
|
25. |
CdS/TiO2 |
Melt quench |
— |
Stone-like |
5.33 |
139
|
26. |
CdS/g- C3N4/MOF |
Solvothermal |
DMF, Cd(CH3COO)2·2H2O, CH4N2S |
— |
— |
140
|
27. |
CdS/ZnO |
Hydrothermal |
ZnO, Cd(CH3COO)2·2H2O |
Spherical |
71.09 ± 16.66 |
141
|
28. |
CdS/ZnO |
Hydrothermal |
Zn(OAC)2·2H2O |
— |
— |
142
|
29. |
CdS/BiOBr |
Precipitation |
Cd(NO3)2·2H2O |
Rod like |
400 |
143
|
30. |
Fe/CdS |
Chemical precipitation |
CdCl2·5H2O, Na2S·5H2O, Fe(NO3)3·9H2O |
Spherical |
11.6–4.1 |
144
|
31. |
BiOCl-Au/CdS |
Chemical bath deposition |
HAuCl4, NH2CSNH2, Cd (CH3COOH)2 |
— |
1000 |
145
|
32. |
ZnO/CdS/CuS |
— |
Cu(NO3)2.3H2O, CH4N2S |
— |
10–15 |
146
|
33. |
CuS |
— |
CH4N2S, Cu(C12H25SO4)(OH)2 |
Hexagonal covellite |
∼10 |
147
|
34. |
NiS2 |
Co-precipitation |
Ni(dtc)2, C2H4(NH2)2 |
Spherical |
62 |
148
|
35. |
NiS2 |
Wet impregnation ultrasonic assisted |
H12N2NiO12, C2H5NS |
Crystalline |
30.47 |
149
|
36. |
NiS2 |
Co-precipitation |
[Ni(dtc)2], C6H18N4 |
Orthorhombic |
5–200 |
150
|
37. |
NiS2 |
Hydrothermal |
Ni(NO3)2·6H2O |
Cubic crystalline |
22 |
1
|
38. |
NiS2 |
Chemical precipitation |
NiSO4,Na2S |
Hexagonal |
0.26 |
151
|
39. |
NiS2 |
Hydrothermal |
NiCl2·6H2O, C3H7NO2S |
Crystalline |
9.5 |
14
|
40. |
NiS2 |
Coprecipitation |
NiCl2·6H2O, Na2S |
Cubic crystalline |
22.11 |
152
|
41. |
NiS2 |
Hydrothermal |
NiSO4 |
Spherical crystalline |
12.1 |
153
|
42. |
NiS2 |
Solution combustion |
(Ni(NO3)2·6H2O), (CS(NH2)2) |
Spherical |
200–300 |
154
|
43. |
NiS2 |
Hypothermal |
Ni(NO3)2·6H2O, SC(NH2)2 |
Rhombohedral |
0.25 |
155
|
44. |
NiS2 |
Wet chemical |
(NiCl2.6H2O) |
Crystalline |
51 |
156
|
45. |
NiS2 |
Solvothermal |
C3N4/Ni-MOF |
Spherical |
0.24–0.55 |
157
|
46. |
NiS2 |
Hydrothermal |
NiCl2·6H2O |
Plane crystal |
200 |
158
|
47. |
NiS2 |
Solution combustion |
Ni(NO3)2·6H2O |
Sponge crystalline |
100–200 |
159
|
48. |
CoS2 |
Precipitation |
C4H6CoO4·4H2O |
Hexagonal |
24.37 |
17
|
49. |
CoS2 |
Solvothermal |
CoH12N2O12 |
Crystalline |
33.97 |
160
|
50. |
NiCo2S4 |
Precipitation |
Ni2N2O6·6H2O, CoN2O6·6H2O |
Spherical |
500 |
161
|
51. |
CoS2 |
Precipitation |
[Ni(S2CNMe2)(C5H4FeC5H4)(C2H5C6H5)]2 |
Spherical and ring |
20 |
162
|
52. |
CoS2 |
Precipitation |
[Ni(S2CNMe2)(C5H4FeC5H4)(C2H5C6H5)]2 |
Spherical |
15–20 |
163
|
53. |
Co5S2 |
Reverse emulsion |
Co (NO3)2⋯6H2O |
Cubic |
230 |
164
|
54. |
NiS2-rGO |
Hydrothermal |
NiCl2 |
Plane crystalline |
0.20 |
13
|
55. |
CoS2 |
Electrochemical deposition |
Na2S |
Nanocrystalline |
14 |
165
|
56. |
Co9S8 |
Solvothermal |
CoSO4·7H2O |
Flower-like crystalline |
230 |
166
|
57. |
Co-ZnS |
Chemical-precipitation |
Zn(CH3COO)2·2H2O, Co(NO3)2·6H2O |
Spherical particles |
3.832 |
167
|
58. |
Ni–Co–S |
Coprecipitation |
Co(CH3COO)2·4H2O, Ni(CH3COO)2·4H2O |
Crystalline |
40–50 |
168
|
59. |
NiS2 |
Precipitation |
NiBr2.3H2O |
Rhombohedral |
50 |
169
|
60. |
NiS-In2O3/GO |
Hydrothermal |
In2O3 and NiS |
— |
66 |
170
|
61. |
NiS-SiO2 |
Ultrasound microwave |
Ni(CH3CO2)2·4H2O |
Hexagonal crystalline |
7.2 |
171
|
62. |
CoS2 |
Chemical precipitation |
Co(NO3)2·6H2O |
Flower-like crystalline |
500 |
172
|
63. |
NiS2 |
Hydrothermal |
Ni(NO3)2·6H2O |
Crystalline |
2–50 |
173
|
64. |
NiS2 |
Precipitation |
NiSO4 |
Powder |
26.75 |
174
|
65. |
Ni–Co–S |
Precipitation |
Ni(OAc)2.4H2O, Co(OAc)2.4H2O |
Cubic crystalline |
30–60 |
175
|
66. |
CoS2 |
Ultrasonication |
CoSO4 and Na2S |
Hexagonal plane |
2.2 |
176
|
67. |
NaFeS2 |
Hydrothermal |
Na2S.9H2O & Fe(NO3)3 |
Monoclinic |
25–30 |
177
|
68. |
ZnS |
Heating |
(ZnSo4.H2O), C2H5NO2, MnSO4.H2O, Na2S |
Crystalline |
— |
178
|
69. |
MoS2-ZnS |
Hydrothermal |
MoS2, ZnS film |
Crystalline |
20–25 |
179
|
70. |
FeS2 |
Hydrothermal |
— |
Nanostructures |
— |
180
|
71. |
Cu2SnS3 |
Hydrothermal |
— |
— |
— |
181
|
72. |
CoS/Ag2WO4 |
Chemical precipitation |
— |
— |
— |
182
|
73. |
CdS |
— |
— |
Hexagonal nanostructures |
— |
183
|
74. |
NiS2-rGO |
Hydrothermal |
— |
Nanospheres |
10 ± 0.317 |
184
|
75. |
(CoS) |
Precipitation |
— |
Nanostructure |
— |
185
|
76. |
NiS/CNTs |
Hydrothermal |
— |
— |
— |
186
|
77. |
ZnS |
Biomolecule-assisted |
— |
— |
— |
187
|
78. |
CuS/CdS |
Hydrothermal |
— |
— |
— |
188
|
79. |
CuS/CuO |
Thermal oxidation |
— |
— |
— |
189
|
80. |
CoFe2O4/MoS2 |
Hydrothermal |
Fe(NO3)3/Co(NO3)2/PVP |
Flower-like |
— |
190
|
81. |
FeS2 |
Hydrothermal |
— |
Crystalline |
50 |
191
|
82. |
pC/ZnS/CuS |
Adsorption and deposition |
— |
— |
|
192
|
83. |
Fe3O4/CdS–ZnS |
Brunauer–Emmett–Teller |
— |
Spherical shape |
25–45 |
193
|
84. |
ZnS QDs |
Chemical precipitation |
— |
— |
— |
194
|
85. |
ZnS/chitosan |
Gelation |
|
— |
100 |
195
|
86. |
CoS/Ag2WO4 |
Co-precipitation |
CoSO4 and Na2S |
Heterojunction |
200 |
196
|
87. |
ZnS-rGO |
Solvothermal |
— |
Sphere |
5–12 |
197
|
88. |
CuS-ZnS-graphene |
Hydrothermal |
— |
Spherical |
5–20 |
198
|
89. |
MoS2 |
Hydrothermal |
— |
Crystalline |
∼20–30 |
123
|
90. |
Ag-ZnS |
Sonochemically |
— |
Crystalline |
|
199
|
91. |
Mn-ZnS |
|
— |
Crystalline |
1–10 |
200
|
92. |
CuO-FeS2 |
Hydrothermal |
— |
Heterostructure |
— |
201
|
93. |
CTS + GO |
Precipitation |
|
Crystalline |
— |
202
|
94. |
Sb2S3 |
Sol–gel |
SbCl3 and TAA |
Spherical shape |
∼180–500 |
203
|
95. |
MoS2/SnO2 |
Hydrothermal |
— |
Heterostructure crystalline |
12 |
204
|
96. |
Bi2S3 |
One pot hydrothermal |
— |
Crystalline |
— |
205
|
97. |
Ag-SnS |
Hot injection |
Ag and SnS |
Nano crystalline |
— |
206
|
98. |
Ag2WO4-MoS2-GO |
Hydrothermal |
— |
— |
— |
207
|
5. Photo catalysis mechanisms: understanding the science behind the light-driven reactions
Photocatalysis is a promising green technology that harnesses the power of light to drive chemical reactions typically by employing semiconductor materials as photocatalysts to convert solar energy into chemical energy.208 This process has gained considerable attention in recent years due to its potential applications in various fields, such as environmental remediation, energy production, and organic synthesis. The photocatalytic removal of pollutants possesses several advantages209,210 including utilization of renewable resources (such as sunlight), a simple operation scheme, efficient removal and the complete mineralization of dyes, etc.170 Organic dyes not only damage the aesthetical aspects of water but are also inappropriate for the ecosystem; they can damage the irrigational land, pose health hazards, and disturb the food chain.
Photocatalysis serves as a remarkable solution for various environmental challenges.14,211 The conventional semiconductors employed as photocatalysts include ZnO,212,213 TiO2,214,215 CdS,216,217etc., which play a significant role in the degradation of environmental contaminants. Photocatalysis is a cost-effective, fast and straightforward method for the cleansing of organic and inorganic contaminants from wastewater.165 In photocatalytic degradation, the commonly involved active species are electrons, holes, hydroxyl (˙OH) and superoxide (O2˙−) radicals. Metal oxides have confined 2p orbitals for oxygen due to the high effective mass of hole carriers. In contrast, metal sulfides exhibit 3p orbitals with a smaller band gap and a greater capacity to capture visible light and facilitate charge transfer. However, a higher recombination rate of electron–hole pairs may impede photocatalysis.176 The unique properties of semiconductor materials make them particularly interesting for photocatalytic reactions for environmental applications.172Fig. 15 illustrates advanced TiO2-based photocatalytic systems for methylene blue degradation.
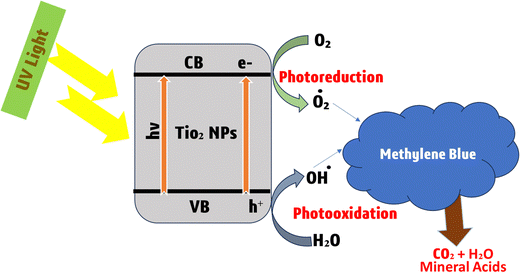 |
| Fig. 15 Representation of enhanced photocatalytic breakdown of methylene blue dye with TiO2 nanoparticles. | |
Although photocatalysis is an effective technology but photocatalyst materials have some issues, such as the bandgap of catalysts, source of light, nature of dye, the concentration of dye and some other factors that may limit the photocatalytic action of photocatalysts.167 Methylene blue (MB) dye is a common industrial pollutant that poses serious environmental and health risks when released into water bodies. Fig. 16 illustrates the photocatalytic degradation mechanism of MB dye using liquid chromatography–mass spectrometry (LC-MS) to identify the intermediates and products formed during the process.31
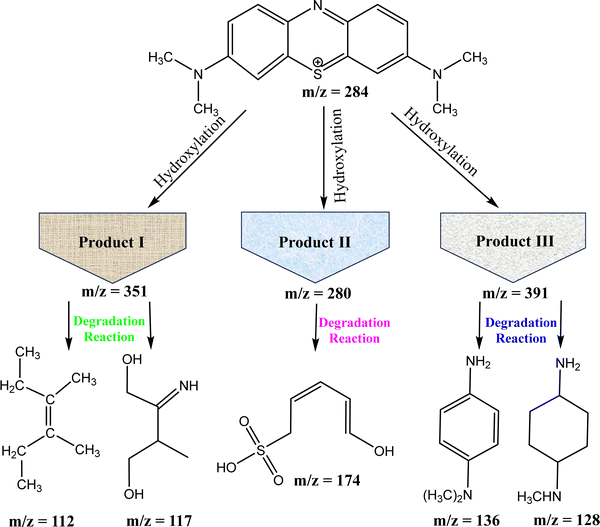 |
| Fig. 16 Degradation pathway of MB dye from molecular breakdown to primary intermediates and the final product. | |
5.1. Photocatalytic degradation of methylene blue (MB) using NiS
In the photocatalytic degradation of MB using a UV-visible spectrophotometer, the λmax of 10 mg L−1 of MB dye was observed at 670 nm.151 Visible light photons with energy equal to or exceeding the bandgap excite the photocatalyst valence band electrons, initiating redox reactions. Photogenerated electrons on the photocatalyst surface create superoxide radicals by reacting with oxygen, while photogenerated holes generate hydroxyl radicals through water interaction.14 The photocatalyst absorbs photons from the irradiation source, which lights the sample solution. This absorption excites semiconductor electrons from the valence band to the conduction band. The photocatalyst generates electron–hole pairs from electron excitation. Positively charged holes form in the valence band and electrons are promoted to the conduction band, forming mobile charge carriers. These photogenerated electrons and holes react. Electrons form oxygen radicals (O2˙−) and hydroxyl radicals (˙OH) from oxygen molecules in the environment, while holes generate positively charged holes. The degradation process uses sample solution water molecules as intermediates. Electrons from the conduction band react with oxygen to make oxygen radicals, which react with water molecules to form hydroxyl radicals and protons. Hydroxyl ions (OH−) in the solution combine with water molecules to create hydrogen peroxide. This reactive species can participate in degradation activities as a powerful oxidant. Hydrogen peroxide from the preceding step can interact with oxygen radicals to produce ˙OH and hydroxyl ions. These very reactive hydroxyl radicals degrade organic substances, including colours. In one situation, hydroxyl radicals immediately react with dye molecules, starting degradation pathways that break down the colour into safer components. Alternatively, hydroxyl radicals might adsorb and degrade dye molecules on the photocatalyst. Parallel to the valence band, positively charged holes (h+) can combine with hydroxyl ions to form hydroxyl radicals (˙OH). These hydroxyl radicals may degrade dye molecules or engage in oxidation events. The mechanism for the degradation of methylene blue is shown in Fig. 17.
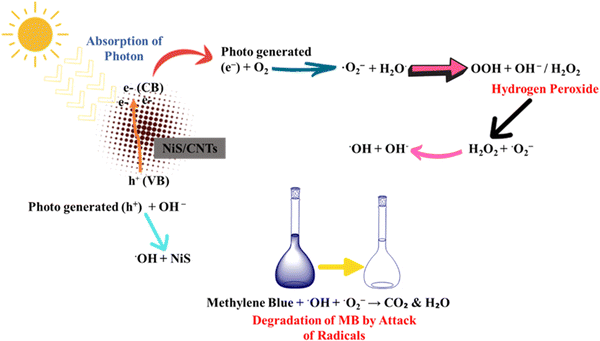 |
| Fig. 17 Mechanism of photocatalytic degradation of methylene blue (MB) using NiS. | |
5.2. Photocatalytic degradation of crystal violet using Nis
The degradation of CV (crystal violet) dye at various degradation times and catalyst doses (5–30 mg) was observed under sunlight irradiation followed by, magnetic stirring. The photocatalytic breakdown of crystal violet dye includes photo-induced processes assisted by the photoactive catalyst. The photocatalyst absorbs irradiation. This energy absorption causes an electron to shift from the photocatalyst's valence band (VB) to its conduction band (CB). An electron moves from the VB to the photocatalyst CB after energy absorption. This produces a h+ in the VB and an e− in the CB. The excited electron interacts with ambient molecular oxygen (O2) in the CB. This interaction generates superoxide radicals. These extremely reactive superoxide radicals have oxidative potential. In the photo catalyst's VB, H2O adsorbed on the surface is oxidized. Hydrogen ions and hydroxyl radicals (˙OH) result from this oxidation. Strong oxidizers are hydroxyl radicals. VB hydroxyl radicals (˙OH) and CB superoxide radicals (O2˙−) react with water and color molecules. These reactions produce water and CO2 as intermediates. The crystal violet dye molecules adsorbed on the photocatalyst interact with radical production and electron transfer intermediary species. These interactions degrade dye molecules into nontoxic components. Photocatalytic degradation of crystal violet dye uses photon energy to trigger electron transfers, reactive radical production, and dye molecule chemical processes. The dye degrades into water and carbon dioxide. The mechanistic aspects of this photodegradation are given in Fig. 18.152
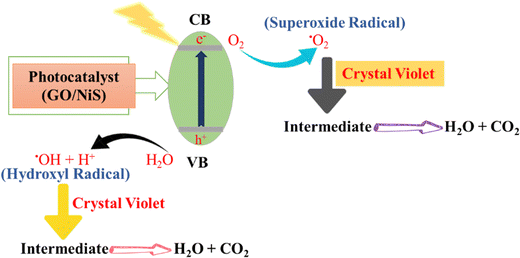 |
| Fig. 18 Photocatalytic degradation through a free radical mechanism. | |
5.3. Photocatalytic degradation of rhodamine B dye using NiS
The catalyst molecule may directly interact with the dye molecule and actively participate in the photocatalytic dye degradation. Also, the standard redox potential of OH−/˙OH (+1.9 V vs. NHE) was found to be less positive than the valence band position of nickel sulfide.156 The mechanism for the degradation of rhodamine is given in Fig. 19. Light absorption from an irradiation source propels the MoS2/NiS2 composite photocatalyst to degrade rhodamine B (RhB) dye through a series of complex stages. The MoS2/NiS2 composite photocatalyst absorbs photons, exciting electrons from the valence band to the conduction band. This excitation creates reactive species with high redox potential by generating electron–hole pairs. A multi-faceted cascade begins when photocatalyst conduction band electrons are activated. Water molecules near the conduction band electrons generate hydroxyl radicals (˙OH) and hydrogen ions. Hydroxyl radicals are very reactive and break down organic dye molecules. Water and oxygen react in the photo catalyst's valence band to form hydrogen peroxide. The valence band holes facilitate hydrogen peroxide production in these processes. Additional reactive oxygen species promote decomposition. Hydroxyl radicals (˙OH) from the conduction band and hydrogen peroxide (H2O2) from the valence band target rhodamine B dye synergistically. These reactive organisms break down dye molecules into simpler, ecologically friendly chemicals. The breakdown of RhB dye produces a mixture of compounds. Carbon dioxide, water, nitrate ions, and ammonium chloride are these products. These degradation products are quickly incorporated into the ecosystem and have no environmental effect.
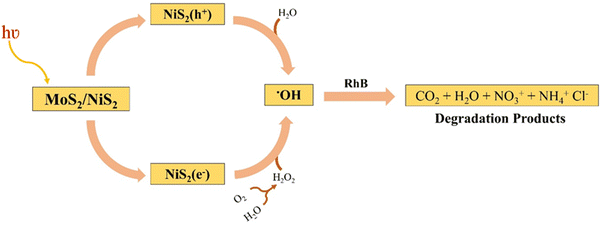 |
| Fig. 19 Degradation of rhodamine B dye by NiS. | |
5.4. Photocatalytic degradation of CR dye CoS-rGO
The hydrophobic nature of metal sulfide–rGO nanocomposites results in exceptional catalytic activity for water and organic pollutant decomposition. Sunlight exposure excites electrons in the valence band, causing them to move to the conduction band.13 The CoSrGO (cobalt-sulfur reduced graphene oxide) photocatalyst degrades CR (Congo red) dye molecules via light-induced reactions. Electrons in CoSrGO are excited by light from the irradiation source. Electrons move to the conduction band (CB) and leave holes in the valence band (VB) after this stimulation. The conduction band of CoSrGO reacts with environmental hydroxyl radicals (*OH). Reactive species that interact with CR dye molecules result from this process. The *OH radicals break the CR dye's molecular structure. In the valence band of CoSrGO, reduced graphene oxide (rGO) interacts with H2O, O2, and H+ to collectively generate hydrogen peroxide. Hydrogen peroxide boosts the environment's oxidative potential, promoting the breakdown of organic molecules. In the valence band of CoSrGO, reduced graphene oxide (rGO) interacts with H2O, O2, and H+ to collectively generate hydrogen peroxide. Hydrogen peroxide boosts the environment's oxidative potential, promoting organic molecule breakdown. Hydrogen peroxide (H2O2) reacts to form hydroxyl radicals (*OH). *OH radicals are potent oxidizers that break down CR dye molecules into safer components. CR dye degradation is caused by the combined activities of *OH radicals generated from CoS in the conduction band and hydrogen peroxide conversion. Radicals attack dye molecules, modifying their structure and cleaving molecular bonds, disintegrating them into non-toxic by-products. A schematic representation of the mechanism of degradation of CR in the presence of the sulfide–rGO nanocomposite is given in Fig. 20.
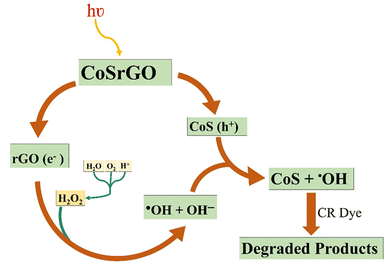 |
| Fig. 20 CR dye degradation using CoS-rGO. | |
5.5. Photocatalysis of indigo carmine dye using NiS2/NP
NiS2/NP, and NiS2/NP (1
:
10, 2
:
10, and 4
:
10) uptake were tested under identical conditions (pH 11, 20 mg L−1 indigo carmine, 0.2 g L−1 photo catalyst). The NiS2/NP catalyst's activity increased, removing over 98% of the IC (indigo carmine) dye (20 mg L−1) within 180 min of visible light exposure.149 The reaction of conversion of indigo carmine in the presence of O2 into isatin and then the conversion of isatin in the presence of O2 into CO2 and H2O is given in Fig. 21.
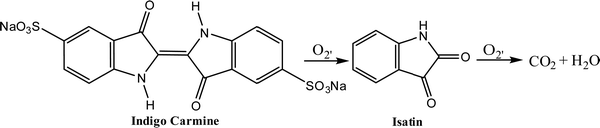 |
| Fig. 21 Degradation of indigo carmine dye via NiS2/NP photocatalysis. | |
5.6. Photocatalysis of crystal violet (CV), Congo red (CR) and methylene red (MR) using CoS
CoS catalyzes the reduction of dyes CV, CR and MR, which exhibit colors like purple, yellow and red. After reduction, their solutions turn colorless. Reduction experiments were conducted at room temperature with the same dye concentration, NaBH4, and catalyst dosage. After reduction, their solutions turned colorless. In the absence of a catalyst, no change in absorbance was observed for hours, indicating the catalyst's crucial role in reducing organic compounds.164Fig. 22 presents degradation mechanism of crystal violet, methylene red and crystal red.
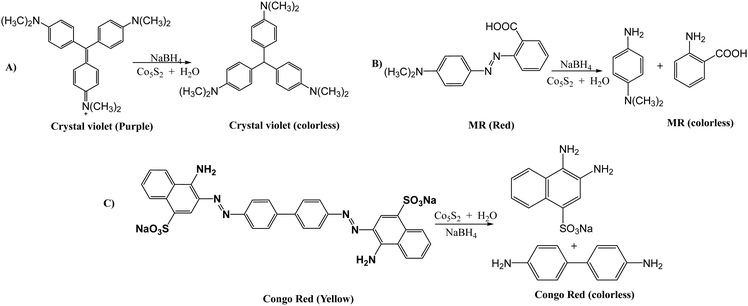 |
| Fig. 22 Photocatalytic degradation of dyes: (A) crystal violet, (B) methylene red, and (C) Congo red. | |
5.7. Science of color: an in-depth look at the features of important dyes
There are a number of significant dyes that relate to different classes of chemicals having different λmax values and applications. For example, Poly R-478 is a member of the polycyclic class of dyes, showing a λmax (nm) value of 520, and it is used in dye-sensitized solar cells. Table 4 presents the characteristic features of some important dyes while Fig. 23 illustrates the chemical structures.
Table 4 Comparative analysis of key characteristics for selected important dyes
Dye name |
Chemical class |
λ
max (nm) |
Applications |
Ref. |
Poly R-478 |
Polycyclic |
520 |
Dye-sensitized solar cells |
218
|
Remazol Brilliant Blue R |
Anthraquinone |
595 |
Textile and paper industries |
219
|
Crystal violet |
Triarylmethane |
590 |
Microbiology, textile dyes |
220
|
Rhodamine B |
Xanthene |
554 |
Fluorescent dyes and tracers |
221
|
Congo red |
Azo |
497 |
Textile dyes, pH indicators |
222
|
Indigo carmine |
Indigoid |
610 |
Food coloring, pH indicators |
223
|
Acid orange 7 |
Monoazo |
484 |
Textile dyes, food coloring |
224
|
Sudan 1 |
Azo |
480 |
Oil-soluble dye, histology |
225
|
Reactive Black 5 |
Azo |
595 |
Textile dyes |
226
|
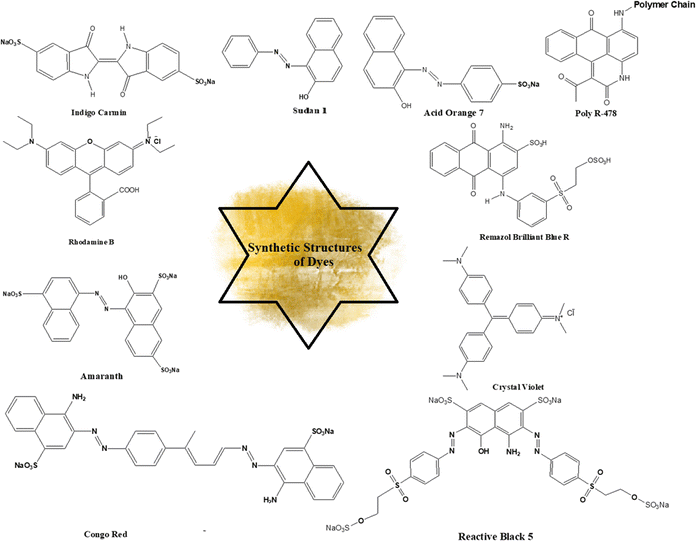 |
| Fig. 23 Comparative analysis of chemical structures for key synthetic dyes. | |
6. Dye degradation: a visual analysis of the process and results
Dye degradation is the breakdown of colored compounds in dyes, which can occur through a variety of chemical, physical, and biological processes. In a visual analysis of the process and results of dye degradation, key characteristics can be observed. Ravikumar et al. (2023) performed degradation of Reactive red 120 dye under a UV irradiation source. After 120 min, 93% degradation of dye was observed.118Table 5 represents the visual analysis of photocatalysis.
Table 5 A visual guide for understanding dye degradation characteristics and features
Sr. no. |
Dye used |
Reaction conditions |
Degradation (%) |
Ref. |
Dose (mg) |
pH |
Time (min) |
01. |
Indigo carmine |
— |
— |
240 |
78 |
1
|
02. |
Reactive red 120 |
50 |
5 |
120 |
93 |
118
|
03. |
Methyl orange |
— |
— |
90 |
87 |
227
|
04. |
Methylene blue |
0.211 |
— |
180 |
62 |
120
|
05. |
Methylene blue |
100 |
— |
60 |
100 |
121
|
06 |
Eosin B |
0.03 |
— |
160 |
95 |
122
|
07. |
Methyl blue |
— |
— |
10 |
99.97 |
123
|
08. |
Rhodamine B |
— |
— |
180 |
92 |
27
|
09. |
Methylene blue |
20 |
— |
120 |
100 |
124
|
10. |
Methylene blue |
0.2 |
— |
45 |
100 |
125
|
11. |
Rhodamine B |
0.1 |
— |
90 |
98.8 |
126
|
12. |
Malachite green oxalate |
1.5 |
9 |
120 |
90.7 |
127
|
13. |
Methyl orange |
25 |
— |
150 |
97.1 |
128
|
14. |
Reactive red 141 |
50 |
|
180 |
98 |
129
|
15. |
Orange red |
20 |
10 |
180 |
93 |
130
|
16. |
Methylene blue |
0.5–2 |
— |
30 |
98.26 |
131
|
17. |
Methyl violet |
— |
— |
90 |
91.8 |
131
|
18. |
Rhodamine B |
— |
|
120 |
64.1 |
132
|
19. |
Methylene blue |
10 |
— |
30 |
100 |
78
|
20. |
Cationic methylene blue |
100 |
— |
270 |
99.04 |
133
|
21. |
Rhodamine B |
10 |
— |
240 |
63 |
134
|
22. |
Methyl orange |
10 |
— |
240 |
78 |
134
|
23. |
Rhodamine B |
— |
— |
— |
93 |
135
|
24. |
Rhodamine B |
10 |
— |
120 |
88 |
136
|
25. |
Methylene blue |
100 |
— |
90 |
99.0 ± 0.4 |
137
|
26. |
Methylene blue |
— |
— |
180 |
90 |
138
|
27. |
Indigo carmine |
100 |
— |
240 |
85.30 |
139
|
28. |
Rhodamine B |
238.43 |
— |
90 |
90.2 |
140
|
29. |
Reactive red 141 |
50 |
— |
240 |
73 |
141
|
30. |
Rhodamine B |
— |
— |
15 |
85 |
142
|
31. |
Rhodamine B |
50 |
— |
40 |
90 |
143
|
32. |
Methylene blue |
10 |
— |
25 |
7 |
144
|
33. |
Rhodamine B |
50 |
— |
40 |
100 |
145
|
34. |
Rhodamine B |
5 |
— |
120 |
82 |
146
|
35. |
Methylene blue |
— |
— |
60 |
71 |
147
|
36. |
Rhodamine B |
— |
— |
180 |
71 |
148
|
Methylene blue |
59 |
37. |
Indigo carmine |
1000 |
— |
180 |
98 |
149
|
38. |
Methylene blue |
— |
— |
180 |
91 |
150
|
Rhodamine-B |
92 |
39. |
Indigo carmine |
100 |
7 |
240 |
51 |
1
|
40. |
Methylene blue |
3 |
— |
140 |
56.3 |
151
|
41. |
Methylene blue |
5 |
— |
70 |
89 |
14
|
42. |
Crystal violet |
20 |
— |
60–80 |
91 |
152
|
43. |
Amido black |
10 |
2–10 |
300–420 |
98 |
153
|
44. |
Methylene blue |
5 |
— |
20 |
56 |
154
|
45. |
Methyl orange |
10 |
2 |
90 |
7.9 |
155
|
46. |
Rhodamine-B |
— |
— |
120 |
87 |
156
|
47. |
Eosin yellow |
— |
10 |
540 |
— |
157
|
48. |
Methylene blue |
— |
3–7 |
30–100 |
87 |
158
|
49. |
Methylene blue |
— |
— |
10 |
99 |
159
|
50. |
Methyl blue |
50 |
8.5 |
300 |
93.6 |
17
|
51. |
Cr(VI) |
1000 |
2–10 |
200 |
57.05 |
160
|
52. |
Eosin yellow |
50 |
10 |
360 |
100 |
161
|
53. |
Rhodamine-B |
100 |
— |
30 |
— |
162
|
54. |
Methylene blue |
100 |
— |
180 |
94 |
163
|
55. |
Crystal violet |
— |
— |
120 |
76 |
164
|
56. |
Congo red |
1000 |
3–11 |
120 |
57.89 |
13
|
57. |
Methyl orange |
100 |
— |
50 |
98 |
165
|
58. |
Methylene blue |
200 |
— |
80 |
5 |
166
|
59. |
Rhodamine-B |
1000 |
— |
75 |
96 |
167
|
60. |
Congo red |
5 |
7 |
— |
90 |
168
|
61. |
Methylene blue |
5 |
— |
90 |
88 |
169
|
62. |
Methylene blue |
20 |
12 |
120 |
76–78 |
228
|
63. |
Methylene blue |
0.01 |
— |
240 |
81 |
229
|
64. |
Methylene blue |
10 |
— |
40 |
98.25 |
170
|
65. |
Methyl red |
— |
10 |
50 |
95.01 |
171
|
66. |
Methylene blue |
10 |
— |
— |
54.4 |
172
|
67. |
Methylene blue |
— |
4–7 |
— |
— |
173
|
68. |
Azure B |
100 |
8 |
240 |
90 |
174
|
69. |
Congo red |
5 |
12 |
10 |
50–60 |
175
|
70. |
Methylene blue |
114 |
— |
220 |
91.8 |
176
|
71. |
Methylene blue |
60 |
— |
105 |
9 |
230
|
72. |
Brilliant green |
500 |
— |
30 |
— |
178
|
73. |
Crystal violet |
— |
— |
40 |
98.5 |
231
|
74. |
Indigo carmine |
— |
— |
— |
88 |
1
|
75. |
Rhodamine B |
— |
— |
180 |
92 |
27
|
76. |
Methylene blue |
— |
— |
220 |
91.8 |
176
|
77. |
Methyl green |
10 |
2 |
120 |
91 |
232
|
78. |
Congo red |
— |
— |
40 |
97.03 |
13
|
79. |
Methylene blue |
20 |
6.8 |
10 |
99.8 |
17
|
80. |
Methylene blue |
— |
— |
50 |
96 |
14
|
81. |
Rhodamine B |
— |
— |
150 |
85 |
28
|
82. |
Methylene blue |
250 |
— |
10 |
99.97 |
233
|
83. |
Congo red |
20 |
— |
60 |
94.9 |
234
|
84. |
Indigo carmine |
200 |
— |
— |
88 |
1
|
85. |
Brilliant green |
10 |
7 |
60 |
95% |
235
|
86. |
Crystalline violet |
5.0 |
11 |
90 |
98.5 |
236
|
87. |
A-2bng |
40 |
7.5 |
120 |
98.17 |
237
|
88. |
Methylene blue |
5 |
— |
220 |
91.8 |
176
|
89. |
Methylene blue |
— |
— |
180 |
75.02 |
238
|
90. |
MO-Rhb |
— |
4.45–10.38 |
60 |
98 |
239
|
96 |
91. |
Rhodamine B |
— |
— |
25 |
96 |
240
|
92. |
Rhodamine B |
0.00125 |
9 |
116 |
87.53 |
241
|
93. |
4,5-Dibromofluorescein |
— |
— |
<30 |
97 |
242
|
94. |
Brilliant green |
— |
— |
90 |
95 |
243
|
95. |
Azo dye |
— |
— |
— |
88 |
244
|
96. |
Rhodamine B |
— |
2.9 |
150 |
∼89 |
245
|
97. |
Methylene blue |
— |
— |
240 |
70 |
246
|
98. |
Rhodamine B |
— |
— |
— |
— |
247
|
99. |
Congo red |
— |
— |
100 |
100 |
206
|
100. |
Methyl orange |
— |
2–9 |
90 |
93 |
207
|
7. Advantages and disadvantages of recent advancements in electric field-assisted photocatalytic dry reforming of metals
The electric field-assisted photocatalytic dry reforming of metals is a new technology with the potential to convert carbon dioxide (CO2) and methane (CH4) into useful syngas (a combination of hydrogen and carbon monoxide). Although this new technique has some benefits, it also has multiple challenges that must be solved before it can be used successfully.
7.1. Advantages
Enhanced reaction kinetics.
During photocatalytic dry reforming, the use of an electric field can accelerate charge separation, improve electron mobility, and stimulate catalytic activity. Because of this, reaction kinetics are enhanced, resulting in better conversion rates and a larger syngas yield.248
Improved selectivity.
The reaction's selectivity may be influenced by an electric field aid, favoring the formation of syngas over undesirable by-products. The improved charge separation leads to a more selective and cleaner conversion process by preventing side reactions that may otherwise result in the formation of coke or carbon deposition.249
Lower activation energy.
An electric field may reduce the activation energy needed for the reaction, allowing it to occur at lower temperatures. This minimizes the amount of energy needed and may improve the catalyst's stability under more comfortable operating conditions.250
Reduced catalyst deactivation.
An electric field aid may minimize catalyst deactivation caused by carbon deposition because improved charge separation lowers the possibility of carbon adhesion on the catalyst surface. This results in increased catalyst lifespan and stability.251
7.2. Disadvantages
Complex engineering.
Complex engineering and design concerns need to be considered for implementing electric field-assisted photocatalytic devices. The incorporation of electrodes, power supplies, and reaction chambers complicates the setup and may present difficulties in scaling up the process.252
Energy consumption.
Reaction kinetics can be improved by electric fields, but they also need energy to operate. Especially, in the context of the process's overall energy efficiency, the energy used to generate and sustain the electric field should be carefully evaluated.253
Electrode fouling.
Electric fields may cause electrode fouling or deterioration over time owing to electrode material corrosion or reaction byproduct buildup. This may have an effect on the stability and efficiency of electric field generation.254
Cost considerations.
The use of an electric field aid can result in extra expenditures for equipment, power sources, and upkeep. To determine the economic viability of the technology, a complete cost-benefit analysis is required.255
8. Density functional theory (DFT) studies on metal sulfide nanoparticles for dye degradation
The adoption of density functional theory (DFT) has become increasingly prevalent as a powerful computational instrument in the investigation of the electronic configuration, optical characteristics, and adsorption tendencies of nanomaterials. Metal sulfide nanoparticles have been extensively studied due to their unique properties and potential applications in various fields.256 One of the key aspects of their characterization involves understanding the energetics of dye adsorption, reaction pathways, and reaction mechanisms. In this regard, density functional theory calculations have emerged as a powerful tool for gaining insights into these fundamental aspects. By employing DFT, researchers are able to computationally investigate the interactions between metal sulphide nanoparticles and dyes, thereby providing valuable information regarding the thermodynamics and kinetics of the adsorption process.257 Furthermore, DFT calculations enable the exploration of different reaction pathways and mechanisms, shedding light on the underlying chemical transformations occurring on the nanoparticle surface. Consequently, the utilization of DFT in the study of metal sulphide nanoparticles has significantly contributed to our understanding of their behavior and has paved the way for the development of novel applications in fields such as catalysis, energy storage, and sensing.258
8.1. Adsorption of dye molecules on metal sulfide nanoparticles
The use of DFT has provided us with the ability to make predictions about the adsorption behavior of dye molecules on metal sulphide nanoparticles. The interaction strength, preferred adsorption sites, and charge transfer pathways may all be clarified via the use of these simulations. The adsorption energies that are predicted using DFT give vital information that is necessary for understanding the stability of the dye-nanoparticle complexes and the possibility for effective dye degradation.259
8.2. Reaction pathways and mechanisms
The incorporation of density functional theory (DFT) studies provides a pathway for the investigation and examination of the complex reaction pathways and underlying mechanisms implicated in the process of dye degradation on metal sulphide nanoparticles. Through careful examination and investigation of the transition states and reaction intermediates, valuable insights can be obtained regarding the pivotal steps that dictate the overall rate of the reaction and the possible pathways that the reaction may follow.260 The discovery of these aforementioned insights holds paramount importance in the realm of designing and optimizing metal sulphide nanoparticles with the ultimate objective of achieving optimal efficacy in the degradation of dyes.261
8.3. Role of metal sulfide composition and morphology
DFT calculations may help us understand how the composition and form of metal sulphide nanoparticles impact the catalytic activity of these particles in the process of dye degradation. We can detect patterns and connections between the electronic structure and catalytic performance by conducting in-depth research on a variety of metal sulphide morphologies and compositions.262
9. Optimization strategies for the degradation of metal sulfides
The optimization strategies for degrading metal sulfides can be categorized into two primary classes. The first class involves a conventional kinetic approach, while the second utilizes the more advanced response surface methodology (RSM).
9.1. Conventional optimization approach
Most photocatalytic reactions are optimized using the traditional kinetic approach, in which the influence of reaction parameters (including photocatalyst concentration, dye molecule concentration, irradiation time, and pH) on the response (percentage degradation; %D) is investigated by varying one reaction parameter and measuring its influence on %D while keeping all other reaction parameters constant.263–267 This optimization method assumes that all reaction parameters are independent of one another and that there is no connection between these reaction parameters. Danish et al. (2021) employed the conventional kinetic approach for the degradation of MB dye. The MB concentration ranged from 15 to 65 ppm, with the highest degradation efficiency, 93.53%, occurring at 45 ppm. Beyond this point, the degradation rate declined quickly. This is expected since, initially, NCs' active sites can absorb extra MB, leading to rapid degradation until the optimal 45 ppm level is attained.32
Ebrahimi et al. (2022) examined the factors influencing the photocatalytic degradation of MC-LR. Key aspects, such as pH, contact duration, and catalyst quantity, were considered in the conventional method. The breakdown of MC-LR by BiVO4 was predominantly influenced by pH, exposure time, and catalyst dosage, as indicated by high mean square and F-value metrics. The most crucial factor was the catalyst amount, with mean square and F-value readings of 608.58 and 181.98, respectively. pH and contact time were of lesser importance. The study aimed to investigate MC-LR removal at varying pH levels, ranging from 5 to 9, and found that pH had a negative impact on MC-LR elimination.268 The novel photocatalyst tBiPO4/Bi2S3-HKUST-1-MOF was synthesized by Mosleh et al. (2016) and photocatalysis optimization of parameters like exposure duration, pH, catalyst quantity, spin rate, liquid flow, air supply, toluidine blue and auramine-O dye concentrations was performed. Ideal values are: 65 min, 6, 0.25 g L−1, 1300 rpm, 0.40 L min−1, 35 L min−1, 25, and 25 mg L−1, respectively. Under these conditions, TB and AO degradation reached 99.37% and 98.44%, with a desirability of 1.0.269Fig. 24 illustrates the impact of reaction parameters on %D values.
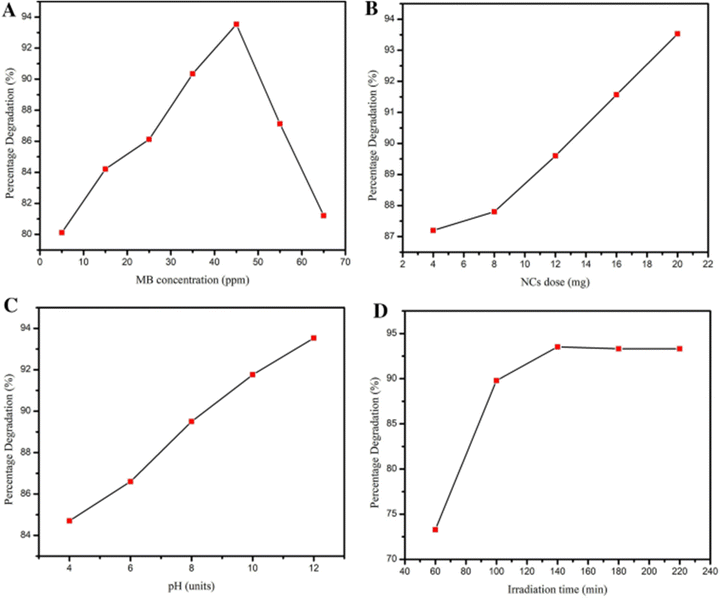 |
| Fig. 24 Impact of reaction parameters (A) concentration of dye, (B) pH values, (C) dose of catalyst, and (D) irradiation time on %D values. Reproduced with permission32 Copyright 2021 Springer Nature. | |
9.2. Response surface methodology (RSM) optimization approach
Response surface methodology (RSM) is a tool that is used in photocatalysis to optimize and model the relationship between experimental variables and photocatalytic performance. It involves setting up a series of experiments with different combinations of independent variables to find the best conditions for getting the desired result.270 RSM is particularly useful for studying complex reaction mechanisms and identifying the key factors that influence photocatalytic performance (Fig. 25).271 RSM helps researchers to find out the best conditions for maximizing the effectiveness of photocatalysis by giving them a mathematical model of the relationship between the input variables and the output response.272 Furthermore, RSM can also be used to optimize the synthesis and preparation of photocatalysts as well as the operating parameters of the photocatalytic process.271 Overall, RSM is a valuable tool for designing and optimizing photocatalytic processes, and its application is expected to continue to grow in the field of photocatalysis.
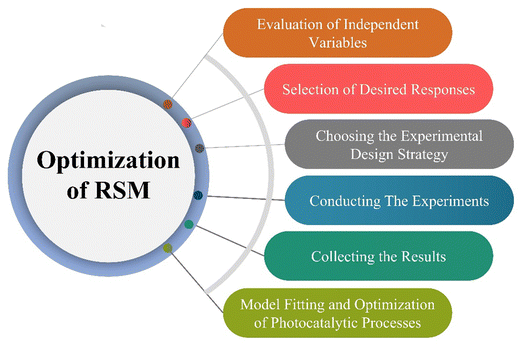 |
| Fig. 25 The optimization of physicochemical processes using RSM consists of six sequential steps. | |
9.2.1. Evaluation of independent variables and selection of desired responses.
The first and the most important stage in the procedure for employing RSM in the dye removal process is to recognize the independent variables that influence the procedure.273 It is crucial to select responses that have a significant impact on the pigment removal procedure and are independent of one another. Moreover, the ranges of these selected factors must be determined based on the results of previous evaluations.274 Several environmental parameters, such as reaction time, pH, and initial dye concentration, have a significant impact on the physicochemical removal of dyes.275 These parameters may vary based on the specified coloring agent removal method.275
9.2.2. Choosing the experimental design strategy.
The choice of a suitable design strategy has a significant impact on the creation of a response surface and the accuracy of the predicted model. For physicochemical removal of dyes, the main design strategies are full factorial design (FFD),276 central composite design (CCD),277–279 Box–Behnken design (BBD),280–282 and Doehlert design (DD) (Fig. 26).283 There are a number of computer programs that facilitate the use of these procedures. Minitab,284 Design Expert,285 Statistica and MATLAB are popular software applications for employing DOE for physicochemical removal of pigments.286
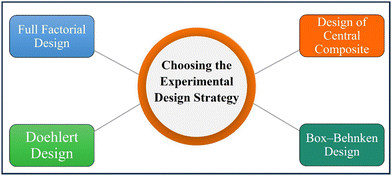 |
| Fig. 26 Choosing the experimental design strategy for degradation of dye. | |
Full factorial design.
The full factorial design method is a standard experimental design with 2–3 input variable levels.287 The two-level FFD generates 2K designs, accounting for low and high values for each factor.288 The three-level FFD creates 3K patterns with factors having low, middle, or high values.289 For fewer than five variables, the 3-k FFD is more useful.290,291
Design of central composites.
CCD is the most commonly used design technique in environmental systems for creating second-order reaction surface models.292 The CCD gives the same information as the three-level FFD but with far fewer trial tests.293 Furthermore, CCD predicts the linear and quadratic interaction impacts of variables affecting the chosen procedure.294Fig. 27 represents the CCD-based %D values of MB dye obtained from thirty experiments. Roya Tangsiri et al. (2020) synthesized CdS nanoparticles and utilized them to photodegrade ranitidine. Design studies utilizing a central composite design with response surface methodology investigated the simultaneous impacts of the influencing factors. The best RD (ranitidine degradation) was achieved at pH 6.2, a catalyst dosage of 0.6 g L−1, a ranitidine concentration of 3.4 mg L−1, and an irradiation period of 97.5 min. The quadratic polynomial model's strong correlation coefficient (R2 = 0.9739) demonstrated that RSM data matched experimental data.295 Arash et al. (2016) prepared manganese-impregnated zinc sulfide nanoparticles on activated carbon (ZnS: Mn-NPs-AC) and evaluated to remove malachite green and methylene blue in binary combination. Their removal efficiency was evaluated using the central composite design. Results from multiple regression analyses and 3-D response surface and contour plots indicated that a pH of 7.0, 3 min sonication time, 0.025 g Mn: ZnS-NPs-AC, and 15 mg L−1 MB and MG result in 99.87% and 98.56% removal efficiencies, respectively.296
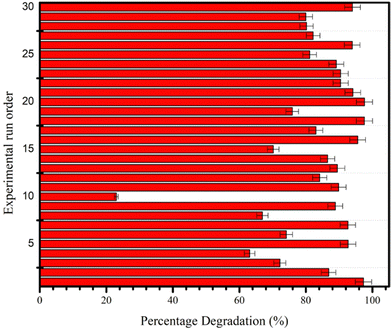 |
| Fig. 27 CCD-based %D values obtained from thirty experimental runs conducted to study the photocatalytic degradation reaction of MB. Reproduced with permission32 Copyright 2021 Springer Nature. | |
Box–Behnken design.
This concept was created by Box and Behnken297 and is a useful tool for conducting experiments as it provides three levels (−1, 0, +1) for each variable, and N = 2k(k1) +C0 represents the total number of experiments required, where k is the number of variables and C0 is the number of central points.280 This design is depicted as a cube, with all points located on a sphere with a radius of 2, and does not include any points at the vertices in the region formed by the upper and lower limits of each variable. The gold nanoparticle-loaded mesoporous TiO2 photocatalysts for ibuprofen (IBP) mineralization in an aqueous heterogeneous suspension were synthesized by Alaa et al. (2022). The photocatalytic process was optimized using a Box–Behnken design using response surface methodology (RSM) with three variables and three stages. An optimal IBP mineralization efficiency of 98.50% was achieved with 366 nm wavelength irradiation, 29.46 mg L−1 IBP concentration, and 0.5 wt% metal concentration.297 Hassan et al. (2023) studied response surface methodology and the Box–Behnken model to determine the synergistic effects of three independent experimental parameters (hormone concentration, solution pH, and photocatalyst dosage) on silica-supported g-C3N4/WO3 nanocomposites with enhanced estrone hormone degradation. These testing conditions resulted in 100% and 96% hormone photodegradation after 3 h of UV and visible light irradiation.282
Doehlert design.
Doehlert invented the symmetrical shell shape to support different levels of variation in variables, like major and minor levels. It is useful when studying variables with limitations, such as cost or time constraints.298 The Doehlert design has a regular distribution between each degree of each component. To determine the number of trials needed, the equation N = k2 + k + C0 is used. For two factors, the design is a regular hexagon with six hexagonal points surrounding a center point.299 For three variables, a cuboctahedron is used, which has high productivity and needs fewer trial points.300
9.2.3. Conducting the experiments and collecting the results.
After choosing the right design strategy and setting up the design level values, computer software can be used to set up a series of experiments in a certain order.301 After the experiments are done, the results are analyzed using the design strategy, which leads to a mathematical model. To prove that the whole process works, this mathematical model needs to be changed to fit the experimental data.302
9.2.4. Model fitting and optimization of photocatalytic processes.
In conventional methods for determining this relationship, experiments are conducted by systematically modifying the studied parameter while keeping all other parameters constant.303 In addition, this extensive procedure cannot identify the combined effect of the effective parameters. Thus, empirical evidence regarding the relationship between factors and response must be gathered. Moztahida and Lee et al. (2020) optimized PGP-0.5 hydrogels’ photocatalytic MB elimination. pH, temperature, and MB concentration were key factors. A 3K Box–Behnken model improved critical parameters for high efficiency in water-rich liquids. Measurements were represented by numbers (1, 0, or 1) indicating low, middle, or high values, respectively.31 Fathinia et al. (2010) optimized the photocatalytic decolorization of two dyes (AB92 and BB3) using a central composite design of response surface methodology. Four variables (starting dye content, UV light strength, flow rate, and reaction time) were tested, and a total of 31 trials were conducted for each dye. Minitab 15 was used to analyze the experimental data.304 Sahoo and Gupta et al. (2012) utilized a single factor study to identify significant factors affecting the photocatalytic degradation of MB, selecting the catalyst dosage, starting pigment content, and pH as variables. Using RSM, these factors were optimized as independent variables with COD and color elimination as response variables and evaluated through 15 sets of Box–Behnken trials.305
9.3. Distinctive perspectives on RSM optimization
Integration of machine learning.
The combination of response surface methodology with machine learning algorithms has the potential to improve the precision of predicting optimal conditions. This integration facilitates the expeditious and precise determination of synthesis parameters.306
Consideration of environmental impact assessment.
In addition to the catalytic efficiency, it is imperative for RSM optimization to also take into account the environmental implications associated with the synthesis methods employed. The implementation of strategies aimed at reducing waste, energy consumption, and the generation of hazardous by-products has the potential to foster the development of more sustainable processes for the synthesis of metal sulfide nanoparticles.307
9.4. Modification strategies for optimizing response surface methodology of metal sulfide nanoparticles in dye degradation
The utilization of response surface methodology (RSM) in the optimization of the synthesis process for metal sulfide nanoparticles has been demonstrated to be highly advantageous in attaining improved efficiency in dye degradation. In order to enhance the efficacy of this methodology, it is crucial to contemplate a multitude of modification strategies that have the potential to optimize photocatalytic performance and improve environmental impacts.32
9.4.1. Modification strategies for RSM optimization.
The subsequent section of this paper shall undertake a comprehensive exploration of diverse modification strategies that can be effectively implemented to enhance the efficiency and efficacy of the response surface methodology in the synthesis of metal sulfide nanoparticles.
Amorphous single atoms and diatomic research.
The incredible catalytic capabilities of amorphous single atom and diatomic structures have drawn attention lately. The integration of these configurations into metal sulfide nanoparticles has been shown to enhance the efficiency of dye degradation. This is achieved by facilitating a greater number of active sites and improving the surface area available for catalytic reactions.308 The subsequent section of this paper shall undertake a comprehensive exploration of diverse modification strategies that can be effectively implemented to enhance the efficiency and effectiveness of the response surface methodology in the synthesis of metal sulfide nanoparticles.
van der Waals heterojunctions.
The concept of van der Waals heterojunctions revolves around the deliberate manipulation and organization of distinct materials possessing complementary characteristics, thereby leading to the emergence of synergistic phenomena.309 The integration of heterojunctions within metal sulfide nanoparticles has been shown to enhance their charge separation capabilities and expand their light absorption range. Consequently, this integration has the potential to significantly enhance the photocatalytic performance of these nanoparticles in the context of dye degradation.310
Microenvironment regulation.
The optimization of photocatalytic activity in metal sulfide nanoparticles is heavily influenced by the meticulous adjustment of their microenvironment. The manipulation of various parameters, such as pH, temperature, and gas atmosphere, during the synthesis process has been found to have a significant impact on the surface states and band positions of catalysts. Consequently, these alterations in surface properties and electronic band structures have been observed to induce changes in the catalytic behavior of the materials under investigation.311
Amorphous 2D layered material coupling.
The combination of amorphous two-dimensional (2D) layered materials with metal sulfide nanoparticles has the potential to enhance photocatalytic reactions. The utilization of composite structures in various applications has drawn significant attention due to their ability to offer a high surface area, thereby facilitating efficient charge transport. In the context of dye degradation, the incorporation of composite structures has been found to yield notable improvements.312
9.5. Advantages of the RSM
1. Through RSM, the catalytic effectiveness and specificity may be increased by adjusting the nanoparticles' size, shape, and surface characteristics.313
2. It is possible to synthesize nanoparticles with unique and advantageous characteristics using sustainable and biologically friendly reagents and circumstances.314
3. Better dye degradation performance may be achieved via the production of nanoparticles with multifunctional properties like magnetic responsiveness or tailored distribution.315
4. More precise control over synthesis may be achieved by using sophisticated real-time monitoring tools, which guarantee that the required features are attained.316
9.6. Addressing stability challenges in optimizing response surface methodology for metal sulfide nanoparticle synthesis for dye degradation
The utilization of response surface methodology (RSM) has garnered significant attention as a potent instrument for optimizing a diverse range of chemical processes. One such application involves the synthesis of metal sulfide nanoparticles, specifically for the purpose of dye degradation.275 The utilization of response surface methodology provides a significant explanation of the connections between various factors and the desired result. However, it is crucial to set priorities for the establishment of stability within the optimization process.
Challenges in stability.
The synthesis of metal sulfide nanoparticles necessitates the optimization of various parameters simultaneously, a practice that may potentially introduce instability into the optimization procedure. The reproducibility of the optimized synthesis may be prevented by the presence of inconsistent results, which can arise from fluctuations in environmental conditions, reagent concentrations, and reaction kinetics.317 The presence of instability within the research not only undermines its credibility but also imposes constraints on its potential for practical implementation in real-world contexts.
Leveraging control variables.
In order to tackle the issues of stability, scientists might deliberately choose and manage factors that have a substantial influence on the synthesis process. Through the utilization of methodologies such as design of experiments,318 the identification of the most influential variables can be achieved, thereby enabling the refinement of the optimization process to yield outcomes that are both consistent and reproducible. Furthermore, the incorporation of process control mechanisms that possess the capability to autonomously adapt variables in accordance with alterations in the surrounding environment can effectively alleviate the adverse effects caused by fluctuations.319
Incorporating in situ monitoring.
Monitoring the real-time synthesis process can yield crucial information regarding its stability. By employing techniques such as electron microscopy, chromatography, and spectroscopy, scientists can track alterations in nanoparticles and identify deviations from the optimal pathway. In addition to facilitating the detection of stability issues, the practice of in situ monitoring enables swift implementation of corrective measures320
Validation and sensitivity analysis.
In order to ascertain the stability of the optimized synthesis, it is imperative to undertake a series of repeated experiments under diverse conditions. The assessment of the optimization process's robustness and its ability to withstand minor fluctuations is facilitated by this approach. The utilization of sensitivity analysis facilitates the identification of variables that exhibit heightened sensitivity towards stability issues, thereby empowering researchers to focus their efforts on the prioritization of control measures.321
10. Challenges and future prospects
This paper discusses the challenges and future trends in the synthesis of metal sulfide nanoparticles and their application in the degradation of dyes using conventional and response surface methodology (RSM) approaches. It also discusses the limitations of current methods, identifies research gaps, and proposes potential strategies to overcome existing challenges and enhance the performance of metal sulfide nanoparticles in dye degradation.
Challenges
1. Developing synthetic methods that can precisely control the size, shape, and crystal structure of MSNPs to enhance their performance in dye degradation.
2. Ensuring that the synthetic methods are both reproducible and scalable to enable industrial-scale synthesis of metal sulfide nanoparticles.
3. Developing environmentally friendly and cost-effective synthetic approaches to reduce the environmental impact and improve the economic viability of metal sulfide nanoparticles.
4. Improving the efficiency, stability, and reusability of metal sulfide nanoparticles in dye degradation applications to ensure their long-term effectiveness and sustainability.
5. Enhancing the selectivity and specificity of MNPs for different types of dyes.
6. Assessing and mitigating the potential toxicity and environmental impact of metal sulfide nanoparticles.
7. Addressing the limitations of conventional approaches in optimizing metal sulfide-based dye degradation systems.
8. Overcoming the challenges in implementing RSM for metal sulfide nanoparticle-based dye degradation.
Future prospects
1. Developing and investigating novel, eco-friendly, and cost-effective metal sulfide nanoparticles that allow precise control over their properties.
2. New strategies should be adopted to enhance the photocatalytic, adsorption, and redox capabilities.
3. Exploring the integration of conventional and RSM approaches with other optimization techniques, such as machine learning and artificial intelligence.
4. Encouraging collaborations among material scientists, environmental engineers, and industry experts to accelerate the development and implementation of innovative metal sulfide nanoparticles.
The recently suggested strategies for modification exhibit considerable potential for advancements in the area of photocatalysis. The utilization of doors in the context of innovative designs and tailored photocatalysts has been observed to provide opportunities for optimization in specific dye degradation applications. This comprehensive overview aims to provide researchers with strategies that can effectively guide their efforts. By seeking the information presented herein, researchers embarking on new studies can gain valuable insights and guidance to inform their research attempts.
11. Conclusions
In conclusion, the synthesis of metal sulfide nanoparticles and their use in the degradation of dyes using both traditional and response surface methodology approaches hold significant potential to help in solving problems caused by synthetic dyes that cause environmental remediation. Due to the high surface area, tunable bandgap, and strong redox capabilities, metal sulfide nanoparticles have become promising materials for the efficient degradation of environmental polluted dyes. This review provides insights into a comprehensive understanding of the diverse synthetic approaches. By tailoring the properties of these nanoparticles, researchers can optimize their performance in dye degradation applications. Moreover, the discussion of the different mechanisms involved in dye degradation, such as photocatalytic, adsorption, and redox processes, has shed light on the different factors that affect the efficiency of MSNPs. The conventional approaches have demonstrated promising results in the degradation of dyes using nanoparticles. As a powerful statistical tool, RSM has been shown to be an upright mode to improve the performance of nanomaterials in dye degradation systems to optimize the process parameters. By studying both the traditional and RSM approaches together, researchers can be more efficient and can gain a better understanding of the synthesis and photocatalytic processes. This study sheds light on the use of MSNPs in the real world for breaking down the effluents. The problems like stability, reusability, and selectivity of the NPs need further research and development in this area. Researchers can find ways to improve the performance of metal sulfide nanoparticles in environmental applications by identifying future research opportunities.
Conflicts of interest
There are no conflicts to declare.
Acknowledgements
The authors extend their appreciation to the research center for Advanced Materials Science (RCAMS)” at King Khalid University, Saudi Arabia, for funding this work under the grant number RCAMS/KKU/016-22.
References
- A. M. Huerta-Flores, L. M. Torres-Martínez, E. Moctezuma, A. P. Singh and B. Wickman, J. Mater. Sci.: Mater. Electron., 2018, 29, 11613–11626 CrossRef CAS.
- H. Zhang, Z. Wang, J. Zhang and K. Dai, Chin. J. Catal., 2023, 49, 42–67 CrossRef CAS.
- A. Ghorbani-Choghamarani and Z. Taherinia, Mol. Catal., 2023, 535, 112829 CrossRef CAS.
- H.-Q. Chen, J.-G. Hao, Y. Wei, W.-Y. Huang, J.-L. Zhang, T. Deng, K. Yang and K.-Q. Lu, Catalysts, 2023, 13, 544 CrossRef CAS.
- Z. Li, Y. Wang, K. Chen, J. Tang, L. Liu and W. Huang, J. Alloys Compd., 2023, 943, 169170 CrossRef CAS.
- J. Wang, J. Yang, H. Yang, H. Huang, X. Yang and L. Wei, Opt. Mater., 2023, 135, 113303 CrossRef CAS.
- S.-l Zhou, L.-g Gong, X.-y Zhao, Q.-l Liang, W.-j Zhang, L.-y Wang, K. Yu and B.-b Zhou, Chem. Phys. Lett., 2020, 759, 138034 CrossRef CAS.
- A. Singh, A. Ahmed, A. Sharma, C. Sharma, S. Paul, A. Khosla, V. Gupta and S. Arya, Phys. B, 2021, 616, 413121 CrossRef CAS.
- M. Sankar, M. Jothibas, A. Muthuvel, A. Rajeshwari and S. J. Jeyakumar, Surf. Interfaces, 2020, 21, 100775 CrossRef CAS.
- C. Balischewski, H. S. Choi, K. Behrens, A. Beqiraj, T. Körzdörfer, A. Geßner, A. Wedel and A. Taubert, ChemistryOpen, 2021, 10, 272–295 CrossRef PubMed.
- H. Peng, Y. Du, J. Yong, C. Huang, X. Zheng and J. Wen, Chem. Eng. J., 2023, 452, 139386 CrossRef CAS.
- C. Zhang, L. Fu, B. Yao, J. Zhu, W. Yang, D. Li and L. Zhou, ACS Appl. Mater. Interfaces, 2023, 15, 8022–8032 CrossRef CAS PubMed.
- P. Borthakur and M. R. Das, J. Colloid Interface Sci., 2018, 516, 342–354 CrossRef CAS PubMed.
- S. Haider, S. S. Shar, I. Shakir and P. O. Agboola, Ceram. Int., 2021, 47, 34269–34277 CrossRef CAS.
- F. Chen, H. Yang, X. Wang and H. Yu, Chin. J. Catal., 2017, 38, 296–304 CrossRef CAS.
- J. Bai, W. Chen, R. Shen, Z. Jiang, P. Zhang, W. Liu and X. Li, J. Mater. Sci. Technol., 2022, 112, 85–95 CrossRef CAS.
- A. A. Khan, S. Kumari, A. Chowdhury and S. Hussain, ACS Appl. Nano Mater., 2018, 1, 3474–3485 CrossRef CAS.
- E. Horozić, Z. Ademović, J. Suljagić, A. Cipurković, E. Roša, A. Sejfić, D. Husejnagić and S. Hodžić, Technol. Acta: Sci. Prof. J. Chem. Technol., 2019, 12, 27–30 Search PubMed.
- W. Li, S. Li, Y. Tang, X. Yang, W. Zhang, X. Zhang, H. Chai and Y. Huang, J. Hazard. Mater., 2020, 389, 121856 CrossRef CAS PubMed.
- C. Deng, X. Ling, L. Peng, T. Wang, R. Xu, Y. Zhu, W. Zhang, P. Sun, Y. Wu and H. Hu, Appl. Surf. Sci., 2023, 157065 CrossRef CAS.
- Z. Yuan, J. Zou, X. Zhao, J. Shi, C. Guo and M. Yan, Journal of Materials Science & Technology, 2023, 166(10), 86–97 Search PubMed.
- A. A. Yaqoob, C. Guerrero–Barajas, A. Ahmad, M. N. M. Ibrahim and M. B. Alshammari, Green Chem. Sustainable Water Purif., 2023, 179–202 Search PubMed.
- W.-K. Jo and R. J. Tayade, Chin. J. Catal., 2014, 35, 1781–1792 CrossRef CAS.
- E. Issaka, S. Yakubu, H. Sulemana, A. Kerkula and O. Nyame-do Aniagyei, Chem. Eng. J. Adv., 2023, 100449 CrossRef CAS.
-
V. Devra, Fungal Cell Factories for Sustainable Nanomaterials Productions and Agricultural Applications, Elsevier, 2023, pp. 257–288 Search PubMed.
- Q. Jiang, C. Ji, D. J. Riley and F. Xie, Nanomaterials, 2018, 9, 1 CrossRef CAS PubMed.
- M. B. Zaman and R. Poolla, Opt. Mater., 2020, 104, 109853 CrossRef CAS.
- D. Samanta, P. Basnet, T. I. Chanu and S. Chatterjee, J. Alloys Compd., 2020, 844, 155810 CrossRef CAS.
- N. Thomas, D. D. Dionysiou and S. C. Pillai, J. Hazard. Mater., 2021, 404, 124082 CrossRef CAS PubMed.
- P. W. Koh, L. Yuliati and S. L. Lee, Iran. J. Sci. Technol., Trans. A: Sci., 2019, 43, 95–103 CrossRef.
- M. Moztahida and D. S. Lee, J. Hazard. Mater., 2020, 400, 123314 CrossRef CAS PubMed.
- M. Danish, H. Ayub, Z. A. Sandhu, A. Shoaib, S. Akram, J. Najeeb and S. Naeem, Appl. Nanosci., 2021, 11, 2503–2515 CrossRef CAS.
- L. G. De Oliveira, A. P. de Paiva, P. P. Balestrassi, J. R. Ferreira, S. C. da Costa and P. H. da Silva Campos, Int. J. Adv. Des. Manuf. Technol., 2019, 104, 1785–1837 CrossRef.
- I. Veza, M. Spraggon, I. R. Fattah and M. Idris, Results Eng., 2023, 101213 CrossRef CAS.
- W.-K. Chong, B.-J. Ng, L.-L. Tan and S.-P. Chai, Energy Fuels, 2022, 36, 4250–4267 CrossRef CAS.
- J. Low, C. Zhang, F. Karadas and Y. Xiong, Chin. J. Catal., 2023, 50, 1–5 CrossRef CAS.
- T. Takayama, I. Tsuji, N. Aono, M. Harada, T. Okuda, A. Iwase, H. Kato and A. Kudo, Chem. Lett., 2017, 46, 616–619 CrossRef CAS.
- Y. Ren, D. Zeng and W.-J. Ong, Chin. J. Catal., 2019, 40, 289–319 CrossRef CAS.
- P. Guo, D. Zhang, X. Liu, W. Liu, R. Wang, Z. Zhang and S. Qiu, J. Alloys Compd., 2022, 921, 166066 CrossRef CAS.
- Z. Wu, Z. Xiong and B. Lai, Environ. Funct. Mater., 2022, 1(3), 298–315 Search PubMed.
- B. Li, F. Wang, K. Wang, J. Qiao, D. Xu, Y. Yang, X. Zhang, L. Lyu, W. Liu and J. Liu, J. Mater. Sci. Technol., 2022, 104, 244–268 CrossRef CAS.
- T. Eisa, M. A. Abdelkareem, D. A. Jadhav, H. O. Mohamed, E. T. Sayed, A. G. Olabi, P. Castaño and K.-J. Chae, Prog. Energy Combust. Sci., 2023, 94, 101044 CrossRef.
- X.-X. Ji, L. Yang, Y.-L. Liu, Y. Fu and F. Ye, Inorg. Chim. Acta, 2023, 545, 121244 CrossRef CAS.
- K. Ao, Q. Wei and W. A. Daoud, ACS Appl. Mater. Interfaces, 2020, 12, 33595–33602 CrossRef CAS PubMed.
- G. de Souza Dias, J. M. Costa and A. F. de Almeida Neto, Adv. Colloid Interface Sci., 2023, 102891 CrossRef PubMed.
- S. Wang, X. He, S. Wang, X. Huang, M. Wu and D. Xiang, Electrochim. Acta, 2023, 441, 141790 CrossRef CAS.
- G. Murugadoss, S. Salla, M. R. Kumar, N. Kandhasamy, H. Al Garalleh, M. Garaleh, K. Brindhadevi and A. Pugazhendhi, Environ. Res., 2023, 115171 CrossRef CAS PubMed.
- N. Abid, A. M. Khan, S. Shujait, K. Chaudhary, M. Ikram, M. Imran, J. Haider, M. Khan, Q. Khan and M. Maqbool, Adv. Colloid Interface Sci., 2022, 300, 102597 CrossRef CAS PubMed.
-
L. Krishnia, P. Thakur and A. Thakur, Synthesis and Applications of Nanoparticles, Springer, 2022, pp. 45–59 Search PubMed.
- P. F. de Oliveira, R. M. Torresi, F. Emmerling and P. H. Camargo, J. Mater. Chem. A, 2020, 8, 16114–16141 RSC.
- A. M. El-Khawaga, A. Zidan and A. I. Abd El-Mageed, J. Mol. Struct., 2023, 135148 CrossRef CAS.
- M. Aydin, A. S. Ahsen and R. Demir-Cakan, J. Solid State Electrochem., 2023, 1–10 Search PubMed.
- W. Zhong, J. Hong, C. Wang, Z. Li, J. Chen and S. Dmytro, Ionics, 2023, 29, 917–930 CrossRef CAS.
- P. R. Nair, C. R. S. Ramirez, M. A. G. Pinilla, B. Krishnan, D. A. Avellaneda, R. F. C. Pelaes and S. Shaji, Appl. Surf. Sci., 2023, 157096 CrossRef.
- A. Nyabadza, M. Vazquez and D. Brabazon, Crystals, 2023, 13, 253 CrossRef CAS.
- P. G. Jamkhande, N. W. Ghule, A. H. Bamer and M. G. Kalaskar, J. Drug Delivery Sci. Technol., 2019, 53, 101174 CrossRef CAS.
- B. Kanrar, K. Sanyal and R. V. Pai, J. Anal. At. Spectrom., 2022, 37, 741–749 RSC.
- L. O. Amaral and A. L. Daniel-da-Silva, Molecules, 2022, 27, 6782 CrossRef CAS PubMed.
- S. Wang, T. Wang, X. Kong, X. Zhao, H. Gan, X. Wang, Q. Meng, F. He, P. Yang and Z. Liu, J. Colloid Interface Sci., 2023, 630, 204–211 CrossRef CAS PubMed.
- S. S. Hossain, A. F. A. Rahman, A. Arsad, A. Basu, A. L. Pang, Z. Harun, M. M. A. Alwi and S. S. Ali, Polymers, 2023, 15, 1528 CrossRef CAS PubMed.
- V. Ganesh, T. AlAbdulaal and I. Yahia, Phys. Scr., 2023, 98, 045908 CrossRef.
- I. Ijaz, E. Gilani, A. Nazir and A. Bukhari, Green Chem. Lett. Rev., 2020, 13, 223–245 CrossRef CAS.
- A. Fathy, A. Wagih and A. Abu-Oqail, Ceram. Int., 2019, 45, 2319–2329 CrossRef CAS.
- A. Meng, W. Tian, H. Yang, X. Wang, X. Wang and Z. Li, J. Hazard. Mater., 2021, 413, 125400 CrossRef CAS PubMed.
- H. Yu, H. Huang, K. Xu, W. Hao, Y. Guo, S. Wang, X. Shen, S. Pan and Y. Zhang, ACS Sustainable Chem. Eng., 2017, 5, 10499–10508 CrossRef CAS.
- A. Hadi, J. Zahirifar, J. Karimi-Sabet and A. Dastbaz, Ultrason. Sonochem., 2018, 44, 204–214 CrossRef CAS PubMed.
- A. B. Workie, H. S. Ningsih and S.-J. Shih, J. Anal. Appl. Pyrolysis, 2023, 105915 CrossRef CAS.
- Y. Lee, T. Fujimoto, S. Yamanaka and Y. Kuga, Adv. Powder Technol., 2021, 32, 1619–1626 CrossRef CAS.
- G. Gahlawat and A. R. Choudhury, RSC Adv., 2019, 9, 12944–12967 RSC.
- D. Devi, N. M. Julkapli, S. Sagadevan and M. R. Johan, Inorg. Chem. Commun., 2023, 110700 Search PubMed.
- S. Munyai and N. Hintsho-Mbita, Curr. Res. Green Sustainable Chem., 2021, 4, 100163 CrossRef CAS.
- M. Ahmaruzzaman and V. Gadore, J. Environ. Chem. Eng., 2021, 9, 105836 CrossRef CAS.
- M. M. Gul, K. S. Ahmad, A. G. Thomas and S. M. Ibrahim, Opt. Mater., 2023, 138, 113682 CrossRef CAS.
- M. Abdullah, P. John, M. N. Ashiq, S. Manzoor, M. I. Ghori, M. U. Nisa, A. G. Abid, K. Y. Butt and S. Ahmed, Nanotechnol. Environ. Eng., 2023, 8, 63–73 CrossRef CAS.
- G. M. Neelgund, S. F. Aguilar, E. A. Jimenez and R. L. Ray, Catalysts, 2023, 13, 476 CrossRef CAS.
- Z. Ali, Q. Hussain, M. A. Yawer, M. Mehmood, R. Hussain, A. Shah, H. Kanwal, A. Yawer, S. Ahmad and S. Zahid, J. Mol. Struct., 2023, 134929 CrossRef CAS.
- K. H. Do, D. P. Kumar, P. Rangappa, J. Lee, S. Yun and T. K. Kim, J. Mater. Chem. A, 2023, 11, 8392–8403 RSC.
- M. Liu, A. Sheardy, G. Pathiraja, F. Tukur, A. Jayapalan and J. Wei, Cleaner Chem. Eng., 2023, 100093 CrossRef.
-
C. Ameta, Y. Vyas and P. Chundawat, Quantum Dots, Elsevier, 2023, pp. 115–145 Search PubMed.
- M. Şaylan, B. Metin, H. Akbıyık, F. Turak, G. Çetin and S. Bakırdere, J. Food Compos. Anal., 2023, 115, 104965 CrossRef.
- S. Iravani, H. Korbekandi, S. V. Mirmohammadi and B. Zolfaghari, Res. Pharm. Sci., 2014, 9, 385 CAS.
- W. Zhu, Y. Cheng, C. Wang, N. Pinna and X. Lu, Nanoscale, 2021, 13, 9112–9146 RSC.
- C. Sambathkumar, V. Manirathinam, A. Manikandan, M. Krishna Kumar, S. Sudhahar and P. Devendran, J. Mater. Sci.: Mater. Electron., 2021, 32, 20827–20843 CrossRef CAS.
- P. Rao, S. Kumar, N. Raje, R. Tokas and N. Sahoo, Mater. Res. Bull., 2016, 79, 105–114 CrossRef CAS.
- S. Sheikhi, M. Aliannezhadi and F. S. Tehrani, Mater. Today Commun., 2023, 34, 105103 CrossRef CAS.
- P. Jeya, S. Keerthana, L. Kungumadevi, R. Yuvakkumar, G. Ravi, A. Kandasami and T. Senthil, Environ. Res., 2023, 226, 115651 CrossRef CAS PubMed.
- Z. H. Jabbar, B. H. Graimed, A. A. Okab, M. M. Alsunbuli and R. A. Al-husseiny, J. Photochem. Photobiol., A, 2023, 114734 CrossRef CAS.
- M. Q. He, Y. Ai, W. Hu, L. Guan, M. Ding and Q. Liang, Adv. Mater., 2023, 2211915 CrossRef PubMed.
- S. V. P. Vattikuti and C. Byon, Nanoscaled Films Layers, 2017, 239 DOI:10.5772/67825.
- C. S. Torres-Castillo and J. R. Tavares, Can. J. Chem. Eng., 2023, 101, 1410–1420 CrossRef CAS.
- J. H. Chung, N. Baek, H. Lim, C. Seo, Y. K. Cho, D. Jung and D. H. Han, Invest. Clin. Urol., 2023, 64, 175–181 CrossRef PubMed.
- Z. Luo, X. Lin, L. Tang, Y. Feng, Y. Gui, J. Zhu, W. Yang, D. Li, L. Zhou and L. Fu, ACS Appl. Mater. Interfaces, 2020, 12, 34755–34762 CrossRef CAS PubMed.
- Y. Wu, S. Wang and K. Komvopoulos, J. Mater. Res., 2020, 35, 76–89 CrossRef CAS.
- S. Luo, C. P. Cullen, G. Guo, J. Zhong and G. S. Duesberg, Appl. Surf. Sci., 2020, 508, 145126 CrossRef CAS.
- S. S. Salem, Arch. Microbiol., 2023, 205, 128 CrossRef CAS PubMed.
-
P. Narayanan, S. Divijendra Natha Reddy and P. Rao, Modern Approaches in Waste Bioremediation: Environmental Microbiology, Springer, 2023, pp. 251–263 Search PubMed.
- G. K. Sodhi and S. Saxena, Environ. Exp. Bot., 2023, 209, 105312 CrossRef CAS.
- J. Loa, I. Cruz-Rodríguez and N. Rojas-Avelizapa, Appl. Biochem. Biotechnol., 2023, 1–16 Search PubMed.
- J. L. Lopez-Miranda, G. A. Molina, M. A. González-Reyna, B. L. España-Sánchez, R. Esparza, R. Silva and M. Estévez, Int. J. Mol. Sci., 2023, 24, 1474 CrossRef CAS PubMed.
- S. S. Salem and A. Fouda, Biol. Trace Elem. Res., 2021, 199, 344–370 CrossRef CAS PubMed.
- V. Bianchi, T. Carey, L. Viti, L. Li, E. H. Linfield, A. G. Davies, A. Tredicucci, D. Yoon, P. G. Karagiannidis and L. Lombardi, Nat. Commun., 2017, 8, 15763 CrossRef CAS PubMed.
- S. Mohammadi, J. Poostforooshan, M. F. Stodt, V. Olszok, J. Kiefer, U. Fritsching and A. P. Weber, Appl. Energy Combust. Sci., 2023, 100151 Search PubMed.
- G. Yang and S.-J. Park, Materials, 2019, 12, 1177 CrossRef CAS PubMed.
- S. Mondal, G. Hoang, P. Manivasagan, M. S. Moorthy, T. T. V. Phan, H. H. Kim, T. P. Nguyen and J. Oh, Ceram. Int., 2019, 45, 2977–2988 CrossRef CAS.
- M. d P. Yeste, C. Fernández-Ponce, E. Félix, M. Tinoco, R. Fernández-Cisnal, C. García-Villar, C. Pfaff, J. Kriwet, E. Natividad and M. Cauqui, Ceram. Int., 2022, 48, 31191–31202 CrossRef CAS.
- L. L. Félix, J. M. Porcel, F. F. H. Aragón, D. G. Pacheco-Salazar and M. H. Sousa, SN Appl. Sci., 2021, 3, 1–10 Search PubMed.
- M. Yaseen, M. Humayun, A. Khan, M. Usman, H. Ullah, A. A. Tahir and H. Ullah, Energies, 2021, 14, 1278 CrossRef CAS.
- H. Chopra, S. Bibi, I. Singh, M. M. Hasan, M. S. Khan, Q. Yousafi, A. A. Baig, M. M. Rahman, F. Islam and T. B. Emran, Front. Bioeng. Biotechnol., 2022, 10, 548 Search PubMed.
- Y. Yuan, L. Wang and L. Gao, Front. Chem., 2020, 8, 818 CrossRef CAS PubMed.
- F. Qi, K.-J. Jeong, J. Gong and Z. Tang, Acc. Chem. Res., 2022, 55, 2425–2438 CrossRef CAS PubMed.
- L. Li, J. Su, J. Lu and Q. Shao, Chem. - Asian J., 2023, 18, e202201044 CrossRef CAS PubMed.
- M. A. Farhana, A. Manjceevan and J. Bandara, J. Sci.: Adv. Mater. Devices, 2023, 100533 CAS.
- Y. Liu, M. Ji and P. Wang, Mol. Pharmaceutics, 2019, 16, 3322–3332 CrossRef CAS PubMed.
- B. Poornaprakash, U. Chalapathi, M. Kumar, S. P. Vattikuti, B. Rajitha, P. Poojitha and S.-H. Park, Mater. Sci. Semicond. Process., 2021, 121, 105395 CrossRef CAS.
- A. Sharma, P. R. Makgwane, E. Lichtfouse, N. Kumar, A. H. Bandegharaei and M. Tahir, Environ. Sci. Pollut. Res., 2023, 1–17 Search PubMed.
- S. Krithika and J. Balavijayalakshmi, Inorg. Chem. Commun., 2022, 110324 Search PubMed.
- R. Zhang, F. Kiessling, T. Lammers and R. M. Pallares, Drug Delivery Transl. Res., 2023, 13, 378–385 CrossRef PubMed.
- S. Ravikumar, D. Mani, E. Chicardi, R. Sepúlveda, K. Balu, V. Pandiyan and Y.-H. Ahn, Ceram. Int., 2023, 49, 9551–9559 CrossRef CAS.
- M. N. Kaka, N. Borah, A. K. Guha and C. Tamuly, Inorg. Chem. Commun., 2023, 110868 CrossRef.
- P. Ajibade, N. Mkhwanazi and L. Mphahlele, Chalcogenide Lett., 2021, 18, 339–349 CrossRef CAS.
- R. Afeesh, N. A. Barakat, S. S. Al-Deyab, A. Yousef and H. Y. Kim, Colloids Surf., A, 2012, 409, 21–29 CrossRef CAS.
- S. Ali, F. Akbar Jan, R. Ullah and N. Ullah, Water Sci. Technol., 2022, 85, 1040–1052 CrossRef CAS PubMed.
- A. Puhan, B. Bhushan, S. Satpathy, S. Meena, A. Nayak and D. Rout, Appl. Surf. Sci., 2019, 493, 593–604 CrossRef CAS.
- P. A. Ajibade and A. E. Oluwalana, Nanomaterials, 2021, 11, 2000 CrossRef CAS PubMed.
- R. Mohammed, M. E. M. Ali, E. Gomaa and M. Mohsen, Sci. Rep., 2022, 12, 18153 CrossRef CAS PubMed.
- X. Li, J. Wang, J. Zhang, C. Zhao, Y. Wu and Y. He, J. Colloid Interface Sci., 2022, 607, 412–422 CrossRef CAS PubMed.
- K. Meena and M. Shanthi, Sustainability, 2022, 14, 15325 CrossRef CAS.
- N. Kandhasamy, G. Murugadoss, T. Kannappan, K. Kirubaharan and R. Kumar Manavalan, Sustainability, 2022, 14, 16184 CrossRef CAS.
- T. Senasu, N. Ruengchai, S. Khamdon, N. Lorwanishpaisarn and S. Nanan, Molecules, 2022, 27, 7944 CrossRef CAS PubMed.
- Z. Shalabayev, M. Baláž, N. Khan, Y. Nurlan, A. Augustyniak, N. Daneu, B. Tatykayev, E. Dutková, G. Burashev and M. Casas-Luna, Nanomaterials, 2022, 12, 1250 CrossRef CAS PubMed.
- L. Isac, C. Cazan, L. Andronic and A. Enesca, Catalysts, 2022, 12, 1135 CrossRef CAS.
- Y. Wang, F. Jiang, J. Chen, X. Sun, T. Xian and H. Yang, Nanomaterials, 2020, 10, 178 CrossRef CAS PubMed.
- P. K. Boruah, B. Sharma, I. Karbhal, M. V. Shelke and M. R. Das, J. Hazard. Mater., 2017, 325, 90–100 CrossRef CAS PubMed.
- M. Iqbal, A. Ali, N. A. Nahyoon, A. Majeed, R. Pothu, S. Phulpoto and K. H. Thebo, Mater. Sci. Energy Technol., 2019, 2, 41–45 Search PubMed.
- H. Sabeeh, S. Musaddiq, M. Shahid, M. A. Khan, M. Sher and M. F. Warsi, Mater. Res. Express, 2018, 5, 065062 CrossRef.
- M. Rani and U. J. Shanker, Chem. Eng. J., 2018, 348, 754–764 CrossRef.
- C.-Y. Zou, W.-C. Ji, S.-Q. Liu, Z. Shen, Y. Zhang and N.-S. Jiang, Chin. J. Catal., 2018, 39, 1051–1059 CrossRef CAS.
- E. E. El-Katori, M. Ahmed, A. El-Bindary and A. M. Oraby, J. Photochem. Photobiol., A, 2020, 392, 112403 CrossRef CAS.
- S. Janbandhu, A. Joshi, S. Munishwar and R. Gedam, Appl. Surf. Sci., 2019, 497, 143758 CrossRef CAS.
- Y. Chen, B. Zhai, Y. Liang, Y. Li and J. Li, J. Solid State Chem., 2019, 274, 32–39 CrossRef CAS.
- T. Senasu, T. Chankhanittha, K. Hemavibool and S. Nanan, Mater. Sci. Semicond. Process., 2021, 123, 105558 CrossRef CAS.
- K. A. Adegoke, M. Iqbal, H. Louis and O. S. Bello, Mater. Sci. Energy Technol., 2019, 2, 329–336 Search PubMed.
- J. You, L. Wang, W. Bao, A. Yan and R. Guo, J. Mater. Sci., 2021, 56, 6732–6744 CrossRef CAS.
- M. Junaid, M. Imran, M. Ikram, M. Naz, M. Aqeel, H. Afzal, H. Majeed and S. Ali, Appl. Nanosci., 2019, 9, 1593–1602 CrossRef CAS.
- M. Zhang, H.-f Yin, J.-c Yao, M. Arif, B. Qiu, P.-f Li and X.-h Liu, Colloids Surf., A, 2020, 602, 124778 CrossRef CAS.
- P. Nandi and D. Das, J. Phys. Chem. Solids, 2022, 160, 110344 CrossRef CAS.
- C. Nethravathi, J. T. Rajamathi and M. Rajamathi, ACS Omega, 2019, 4, 4825–4831 CrossRef CAS PubMed.
- P. Lakshmanan, S. Thirumaran and S. Ciattini, J. Mol. Struct., 2020, 1220, 128704 CrossRef CAS.
- H. Hafdi, J. Mouldar, M. Joudi, B. Hatimi, H. Nasrellah, M. A. E. Mhammedi and M. Bakasse, Opt. Quantum Electron., 2021, 53, 1–13 CrossRef.
- S. Thirumaran, G. Gurumoorthy, R. Arulmozhi and S. Ciattini, Appl. Organomet. Chem., 2020, 34, e5761 CrossRef CAS.
- S. Kokilavani, A. A. Al-Kheraif, A. M. Thomas, A. Syed, A. M. Elgorban, L. L. Raju, A. Das and S. S. Khan, Phys. E, 2021, 133, 114767 CrossRef CAS.
- V. Manikandan, R. Elancheran, P. Revathi, P. Suganya and K. Krishnasamy, Bull. Mater. Sci., 2020, 43, 1–10 CrossRef.
- S. Muninathan and S. Arumugam, Int. J. Hydrogen Energy, 2021, 46, 6532–6546 CrossRef CAS.
- N. A. Marand, S. Masoudpanah and M. S. Bafghi, Ceram. Int., 2018, 44, 17277–17282 CrossRef.
- Y. Li, X. Li, X.-T. Wang, L.-J. Jian, N. I. M. Abdallah, X.-F. Dong and C.-W. Wang, Colloids Surf., A, 2021, 608, 125565 CrossRef CAS.
- R. Khan, N. S. Das, B. Das, B. Das and K. K. Chattopadhyay, J. Photochem. Photobiol., A, 2021, 412, 113212 CrossRef CAS.
- Y. Qi, J. Xu, M. Zhang, H. Lin and L. Wang, Int. J. Hydrogen Energy, 2019, 44, 16336–16347 CrossRef CAS.
- P. Arumugam, P. Sengodan, N. Duraisamy, R. Rajendran and V. Vasudevan, Ionics, 2020, 26, 4201–4212 CrossRef CAS.
- N. Ardebilchi Marand, S. Masoudpanah, M. S. Bafghi and S. Alamolhoda, J. Electron. Mater., 2020, 49, 1266–1272 CrossRef CAS.
- K. Arathi, T. Ravishankar, K. Raj and K. Nagashree, Chem. Pap., 2021, 75, 4707–4718 CrossRef CAS.
- S. Zhao, J. Xu, Z. Li, Z. Liu and Y. Li, J. Colloid Interface Sci., 2019, 555, 689–701 CrossRef CAS PubMed.
-
G. Gurumoorthy, R. Hema and M. Sundararajan, Annals of the Romanian Society for Cell Biology, 2021, 2086–2090 Search PubMed.
-
G. Gurumoorthy, R. Hema and M. Sundararajan, Annals of the Romanian Society for Cell Biology, 2021, 2096–2099 Search PubMed.
- I. Mukhtar, S. Ali, S. Jamil and S. R. Khan, Chem. Phys. Lett., 2020, 754, 137649 CrossRef CAS.
- S. M. Pourmortazavi, M. Rahimi-Nasrabadi, B. Larijani, M. S. Karimi and S. Mirsadeghi, J. Mater. Sci.: Mater. Electron., 2018, 29, 13833–13841 CrossRef CAS.
- M. Sohail, J. Huang, Z. Lai, Y. Cao, S. Ruan, M. N. Shah, F. U. Khan, H. I. A. Qazi and B. Ullah, J. Inorg. Organomet. Polym. Mater., 2020, 30, 5168–5179 CrossRef CAS.
- M. Danish and M. Muneer, Ceram. Int., 2021, 47, 13043–13056 CrossRef CAS.
- A. Chowdhury, S. Kumari, A. A. Khan and S. Hussain, J. Hazard. Mater., 2020, 385, 121602 CrossRef CAS PubMed.
- M. A. Khan, W. Hussain, K. Tufail, M. Sulaman, A. R. Ayub, W. A. Khan and H. Li, Energy Rep., 2021, 7, 7615–7627 CrossRef.
- M. I. Din, R. Khalid, J. Najeeb and Z. Hussain, J. Cleaner Prod., 2021, 298, 126567 CrossRef CAS.
- M. Hosseini, N. Fazelian, A. Fakhri, H. Kamyab, K. K. Yadav and S. Chelliapan, J. Photochem. Photobiol., B, 2019, 194, 128–134 CrossRef CAS PubMed.
- D. Ayodhya and G. Veerabhadram, Mater. Today Energy, 2018, 9, 83–113 CrossRef.
- X. Zheng, Y. Dong and T. Liu, Colloids Surf., A, 2020, 598, 124854 CrossRef CAS.
- R. Chaudhary, S. C. Ameta and R. Ameta, Maced. J. Chem. Chem. Eng., 2019, 38, 107–114 CrossRef CAS.
- A. Chowdhury, A. A. Khan, S. Kumari and S. Hussain, ACS Sustainable Chem. Eng., 2019, 7, 4165–4176 CrossRef CAS.
- S. Kokilavani, A. Syed, H. A. AL-Shwaiman, M. M. Alkhulaifi, F. N. Almajdhi, A. M. Elgorban and S. S. Khan, Colloid Interface Sci. Commun., 2021, 42, 100415 CrossRef CAS.
- M. A. Abid, D. A. Abid, W. J. Aziz and T. M. Rashid, Phys. B, 2021, 622, 413277 CrossRef CAS.
- J. Li and W. B. Ko, Elastomers Compos., 2018, 53, 75–79 CAS.
- S. B. Atla, W.-R. Lin, T.-C. Chien, M.-J. Tseng, J.-C. Shu, C.-C. Chen and C.-Y. Chen, Mater. Chem. Phys., 2018, 216, 380–386 CrossRef CAS.
- S. Baek, Y. Ghaffari and J. Bae, Catalysts, 2022, 12, 1045 CrossRef CAS.
- P. K. Boruah, B. Sharma, I. Karbhal, M. V. Shelke and M. R. Das, J. Hazard. Mater., 2017, 325, 90–100 CrossRef CAS PubMed.
- S. Das, A. Samanta and S. Jana, ACS Sustainable Chem. Eng., 2017, 5, 9086–9094 CrossRef CAS.
- F. Davar, A. Majedi and A. Abbasi, J. Mater. Sci.: Mater. Electron., 2017, 28, 4871–4878 CrossRef CAS.
- O. Długosz, K. Szostak, M. Krupiński and M. Banach, Int. J. Environ. Sci. Technol., 2021, 18, 561–574 CrossRef.
- S. Douafer, H. Lahmar, M. Benamira, G. Rekhila and M. Trari, J. Phys. Chem. Solids, 2018, 118, 62–67 CrossRef CAS.
- M. Dubey, N. V. Challagulla, S. Wadhwa and R. Kumar, Colloids Surf., A, 2021, 609, 125720 CrossRef CAS.
- D. P. Dutta, M. Ramakrishnan, M. Roy and A. Kumar, J. Photochem. Photobiol., A, 2017, 335, 102–111 CrossRef CAS.
- Z.-T. Hu, J. Liu, X. Yan, W.-D. Oh and T.-T. Lim, Chem. Eng. J., 2015, 262, 1022–1032 CrossRef CAS.
- F. Iazdani and A. Nezamzadeh-Ejhieh, Chem. Phys., 2021, 550, 111305 CrossRef CAS.
- K. Kirchberg, A. Becker, A. Bloesser, T. Weller, J. Timm, C. Suchomski and R. Marschall, J. Phys. Chem. C, 2017, 121, 27126–27138 CrossRef CAS.
- M. A. J. Kouhbanani, N. Beheshtkhoo, A. M. Amani, S. Taghizadeh, V. Beigi, A. Z. Bazmandeh and N. Khalaf, Mater. Res. Express, 2018, 5, 115013 CrossRef.
- Y. Li, X.-T. Wang, X.-Q. Zhang, X. Li, J. Wang and C.-W. Wang, Phys. E, 2020, 118, 113865 CrossRef CAS.
- Y. Liu, L. Zong, C. Zhang, W. Liu, A. Fakhri and V. K. Gupta, Surf. Interfaces, 2021, 26, 101292 CrossRef CAS.
- S. Lohrasbi, M. A. J. Kouhbanani, N. Beheshtkhoo, Y. Ghasemi, A. M. Amani and S. Taghizadeh, Bionanoscience, 2019, 9, 317–322 CrossRef.
- N. Madubuonu, S. O. Aisida, A. Ali, I. Ahmad, T.-K. Zhao, S. Botha, M. Maaza and F. I. Ezema, J. Photochem. Photobiol., B, 2019, 199, 111601 CrossRef CAS PubMed.
- S. Mortazavi-Derazkola, M. Salavati-Niasari, O. Amiri and A. Abbasi, J. Energy Chem., 2017, 26, 17–23 CrossRef.
- H. Muthukumar and M. Matheswaran, ACS Sustainable Chem. Eng., 2015, 3, 3149–3156 CrossRef CAS.
- A. C. Pradhan, B. Nanda, K. Parida and G. R. Rao, J. Phys. Chem. C, 2015, 119, 14145–14159 CrossRef CAS.
- S. Qasim, A. Zafar, M. S. Saif, Z. Ali, M. Nazar, M. Waqas, A. U. Haq, T. Tariq, S. G. Hassan and F. Iqbal, J. Photochem. Photobiol., B, 2020, 204, 111784 CrossRef CAS PubMed.
- M. Rani and U. Shanker, Chem. Eng. J., 2018, 348, 754–764 CrossRef.
- H. Sabeeh, S. Musaddiq, M. Shahid, M. A. Khan, M. Sher and M. F. Warsi, Mater. Res. Exp., 2018, 5, 065062 CrossRef.
- N. Shaheen, M. A. Yousuf, I. Shakir, S. Zulfiqar, P. O. Agboola and M. F. Warsi, Phys. B, 2020, 580, 411820 CrossRef CAS.
- S. Vignesh, M. Sivakami, P. Muniyappan and J. K. Sundar, Int. Res. J. Eng. Technol., 2017, 4, 70 Search PubMed.
- G. Zhao, Z. Mo, P. Zhang, B. Wang, X. Zhu and R. Guo, J. Porous Mater., 2015, 22, 1245–1253 CrossRef CAS.
- C.-Y. Zou, W.-C. Ji, S.-Q. Liu, Z. Shen, Y. Zhang and N.-S. Jiang, Chin. J. Catal., 2018, 39, 1051–1059 CrossRef CAS.
- C. Behera, S. P. Ghosh, J. P. Kar and S. L. Samal, New J. Chem., 2020, 44, 11684–11693 RSC.
- N. Tahir, M. Zahid, A. Jillani, M. Yaseen and Q. Abbas, J. Photochem. Photobiol., A, 2023, 436, 114376 CrossRef CAS.
- G. Luna-López, M. Del Barrio, J. Fize, V. Artero, A. M. Coito, I. A. Pereira, J. C. Conesa, A. Iglesias-Juez, A. L. De Lacey and M. Pita, Bioelectrochemistry, 2023, 150, 108361 CrossRef PubMed.
- M. A. Al-Nuaim, A. A. Alwasiti and Z. Y. Shnain, Chem. Pap., 2023, 77, 677–701 CrossRef CAS PubMed.
- W. Fu, J. Fan and Q. Xiang, Chin. J. Struct. Chem., 2022, 41, 2206039–2206047 CAS.
- S. Han, B. Li, L. Huang, H. Xi, Z. Ding and J. Long, Chin. J. Struct. Chem., 2022, 41, 2201007–2201013 CAS.
- H. Leelavathi, R. Muralidharan, N. Abirami, S. Tamizharasan, S. Sankeetha, A. Kumarasamy and R. Arulmozhi, Colloids Surf., A, 2023, 656, 130449 CrossRef CAS.
- L.-H. Kao, K.-S. Chuang, H. N. Catherine, J.-H. Huang, H.-J. Hsu, Y.-C. Shen and C. Hu, J. Taiwan Inst. Chem. Eng., 2023, 142, 104638 CrossRef CAS.
- M. Lyulyukin, N. Kovalevskiy, A. Bukhtiyarov, D. Kozlov and D. Selishchev, Int. J. Mol. Sci., 2023, 24, 5693 CrossRef CAS PubMed.
- R. Sinha and P. S. Ghosal, J. Environ. Manage., 2023, 328, 117011 CrossRef PubMed.
- C. Yuan, H. Lv, Y. Zhang, Q. Fei, D. Xiao, H. Yin, Z. Lu and Y. Zhang, Carbon, 2023, 206, 237–245 CrossRef CAS.
- W. Jin, L. Jing, X. Wu, R. Ying-Yi and W. Feng, Acta Phys.-Chim. Sin., 2021, 37 DOI:10.3866/PKU.WHXB202008043.
- Q. Husain, Crit. Rev. Biotechnol., 2006, 26, 201–221 CrossRef CAS PubMed.
- E. Forgacs, T. Cserháti and G. Oros, Environ. Int., 2004, 30, 953–971 CrossRef CAS PubMed.
- N. Patel Jay, K. Vaishnav Darshit, R. Joshi Pavan and R. Tipre Devayani, Res. J. Chem. Environ., 2023, 27, 4 Search PubMed.
- M. Sukumar, J. R. Rajabathar, H. Al-Lohedan, S. Suresh, C. S. Dash, M. Sundararajan, P. S. Subudhi, S. Arokiyaraj, E. Yanmaz and S. Yuvaraj, J. Alloys Compd., 2023, 169902 CrossRef CAS.
- E. Turcu, C. G. Coromelci, V. Harabagiu and M. Ignat, Catalysts, 2023, 13, 345 CrossRef CAS.
- T. Islam, M. R. Repon, T. Islam, Z. Sarwar and M. M. Rahman, Environ. Sci. Pollut. Res., 2023, 30, 9207–9242 CrossRef CAS PubMed.
- D. Sannino, N. Morante, O. Sacco, A. Mancuso, L. De Guglielmo, G. Di Capua, N. Femia and V. Vaiano, Photochem. Photobiol. Sci., 2023, 22, 185–193 CrossRef CAS PubMed.
- Z. Ma, Y. Han, F. Xu and X. Wang, Int. J. Biol. Macromol., 2023, 124265 CrossRef CAS PubMed.
- T. Amber, S. Nosheen, R. Kamran, S. Kiran, M. I. Yousaf, N. Sharifi and S. Riazi, Pak. J. Sci. Ind. Res., Ser. A, 2023, 66, 16–22 Search PubMed.
- C. Han, C. Cheng, F. Liu, X. Li, G. Wang and J. Li, Nanotechnol. Rev., 2023, 12, 20220503 CrossRef CAS.
-
A. Qayoom, I. Ashraf, A. Rashid, M. Ayoub and D. Kumar, 2022.
-
R. Mugumoa, E. O. Ichipi, S. M. Tichapondwa and E. MN, 2023.
- V. Dileepkumar, P. Surya, C. Pratapkumar, R. Viswanatha, C. Ravikumar, M. A. Kumar, H. Muralidhara, I. M. Al-Akraa, A. M. Mohammad and Z. Chen, J. Environ. Chem. Eng., 2020, 8, 104005 CrossRef CAS.
- T. T. Xuan, L. N. Long and T. Van Khai, Vietnam J. Chem., 2020, 58, 92–100 CrossRef CAS.
- S. Munyai, Z. Tetana, M. Mathipa, B. Ntsendwana and N. Hintsho-Mbita, Optik, 2021, 247, 167851 CrossRef CAS.
- M. Mahanthappa, N. Kottam and S. Yellappa, Appl. Surf. Sci., 2019, 475, 828–838 CrossRef CAS.
- B. Ren, W. Shen, L. Li, S. Wu and W. Wang, Appl. Surf. Sci., 2018, 447, 711–723 CrossRef CAS.
- F. Soltani-Nezhad, A. Saljooqi, A. Mostafavi and T. Shamspur, Ecotoxicol. Environ. Saf., 2020, 189, 109886 CrossRef CAS PubMed.
- F. Karimi, H. R. Rajabi and L. Kavoshi, Ultrason. Sonochem., 2019, 57, 139–146 CrossRef CAS PubMed.
- A. Alharbi, R. K. Shah, A. Sayqal, A. Subaihi, A. A. Alluhaybi, F. K. Algethami, A. M. Naglah, A. A. Almehizia, H. A. Katouah and H. M. Youssef, Alexandria Eng. J., 2021, 60, 2167–2178 CrossRef.
- A. M. Palve and D. N. Kokil, Mater. Res. Express, 2019, 6, 105536 CrossRef CAS.
- J. Varghese, J. Phys. Chem. Solids, 2021, 156, 109911 CrossRef CAS.
- N. Tavker and M. Sharma, J. Environ. Manage., 2020, 255, 109906 CrossRef CAS PubMed.
- E. B. Yazdani and A. Mehrizad, J. Mol. Liq., 2018, 255, 102–112 CrossRef CAS.
- Z. Ahmadi, H. Ramezani, S. N. Azizi and M. J. Chaichi, Environ. Sci. Pollut. Res., 2020, 27, 9707–9717 CrossRef CAS PubMed.
- A. G. Raju, B. D. Rao, G. Himabindu and S. M. Botsa, J. Mater. Res. Technol., 2022, 17, 2648–2656 CrossRef CAS.
- H. D. Shelke, A. R. Machale, A. A. Surwase, S. F. Shaikh, A. U. H. S. Rana and H. M. Pathan, Coatings, 2023, 13, 522 CrossRef CAS.
- O. F. Er, A. Caglar, B. Ulas, H. Kivrak and A. Kivrak, Mater. Chem. Phys., 2020, 241, 122422 CrossRef CAS.
- P. O. Agboola and I. Shakir, J. Mater. Res. Technol., 2022, 18, 4303–4313 CrossRef CAS.
- H. Shen, Z. Shao, Q. Zhao, M. Jin, C. Shen, M. Deng, G. Zhong, F. Huang, H. Zhu and F. Chen, J. Colloid Interface Sci., 2020, 573, 115–122 CrossRef CAS PubMed.
- Q. Lei, S. Yang, D. Ding, J. Tan, J. Liu and R. Chen, J. Mater. Chem. A, 2021, 9, 2491–2525 RSC.
- X. Zhang, W. Huang, L. Yu, M. García-Melchor, D. Wang, L. Zhi and H. Zhang, Carbon Energy, 2022, e362 Search PubMed.
- Y. Zhang, Y. Shen, J. Liu, L. Lv, M. Zhou, X. Yang, X. Meng and Z. Zhou, Appl. Phys. Lett., 2023, 122 DOI:10.1063/5.0127150.
- C. Hu, S. Tu, N. Tian, T. Ma, Y. Zhang and H. Huang, Angew. Chem., Int. Ed., 2021, 60, 16309–16328 CrossRef CAS PubMed.
- Y. Su, L. Zhang, W. Wang and D. Shao, ACS Sustainable Chem. Eng., 2018, 6, 8704–8710 CrossRef CAS.
- T.-Y. Chen, Y. W. Hsiao, M. Baker-Fales, F. Cameli, P. Dimitrakellis and D. G. Vlachos, Chem. Sci., 2022, 13, 10644–10685 RSC.
- R. Wang, J. Shen, W. Zhang, Q. Liu, M. Zhang and H. Tang, Ceram. Int., 2020, 46, 23–30 CrossRef CAS.
- G. Zhang, L. Li, Z.-J. Zhao, T. Wang and J. Gong, Acc.Mater. Res., 2022, 4, 212–222 CrossRef.
- X. Tu, H. Xu, C. Li, X. Liu, G. Fan and W. Sun, Comput. Theor. Chem., 2021, 1203, 113360 CrossRef CAS.
- J. Su, D. Luise, I. Ciofini and F. Labat, J. Phys. Chem. C, 2023, 127, 5968–5981 CrossRef CAS.
- J. Tang, B. Gao, J. Pan, L. Chen, Z. Zhao, S. Shen, J.-K. Guo, C.-T. Au and S.-F. Yin, Appl. Catal., A, 2019, 588, 117281 CrossRef CAS.
- P. Lakshmanan, R. Arulmozhi, S. Thirumaran and S. Ciattini, Polyhedron, 2022, 218, 115766 CrossRef CAS.
- S. Abdpour, E. Kowsari, B. Bazri, M. R. A. Moghaddam, S. S. Tafreshi, N. H. de Leeuw, I. Simon, L. Schmolke, D. Dietrich and S. Ramakrishna, J. Mol. Liq., 2020, 319, 114341 CrossRef CAS.
- P. Lakshmanan, E. Gayathri, S. Thirumaran and S. Ciattini, J. Mol. Struct., 2023, 1273, 134306 CrossRef CAS.
- H. Wang, J. Xu, Q. Zhang, S. Hu, W. Zhou, H. Liu and X. Wang, Adv. Funct. Mater., 2022, 32, 2112362 CrossRef CAS.
- S. Awais, H. Munir, J. Najeeb, F. Anjum, K. Naseem, N. Kausar, M. Shahid, M. Irfan and N. Najeeb, J. Cleaner Prod., 2023, 136916 CrossRef CAS.
- N. Tiwari, S. Chakrabortty, K. Samal, S. Moulick, B. G. Mohapatra, S. Samanta, P. Mohapatra, K. Sanjay, J. Nayak and S. Banerjee, J. Taiwan Inst. Chem. Eng., 2023, 104800 CrossRef CAS.
- P. Kowsalya, S. Uma Bharathi, S. Sivasankar and M. Chamundeeswari, J. Iran. Chem. Soc., 2023, 1–13 Search PubMed.
- S. Rastgar, H. Rezaei, H. Younesi, H. Abyar and A. Kordrostami, Biomass Convers. Biorefin., 2023, 1–12 Search PubMed.
- R. Shakunthala and M. Matheswaran, Opt. Mater., 2023, 138, 113719 CrossRef.
- A. Ebrahimi, K. Ebrahim, A. Abdolahnejad, N. Jafari, M. Karimi, A. Mohammadi and A. Nikoonahad, Int. J. Environ. Anal. Chem., 2022, 102, 7015–7032 CrossRef CAS.
- S. Mosleh, M. Rahimi, M. Ghaedi, K. Dashtian and S. Hajati, RSC Adv., 2016, 6, 63667–63680 RSC.
- V. Mahmoodi and J. Sargolzaei, Desalin. Water Treat., 2014, 52, 6664–6672 CrossRef CAS.
- M. Lal, P. Sharma, L. Singh and C. Ram, Results Eng., 2023, 100890 CrossRef CAS.
- F. Elfghi, Chem. Eng. Res. Des., 2016, 113, 264–272 CrossRef CAS.
- P. Pakravan, A. Akhbari, H. Moradi, A. H. Azandaryani, A. M. Mansouri and M. Safari, Appl. Petrochem. Res., 2015, 5, 47–59 CrossRef CAS.
- S. J. M. Breig and K. J. K. Luti, Mater. Today: Proc., 2021, 42, 2277–2284 CrossRef.
- S. Karimifard and M. R. A. Moghaddam, Sci. Total Environ, 2018, 640, 772–797 CrossRef PubMed.
- K. Kighuta, A.-I. Gopalan, D.-E. Lee, G. Saianand, Y.-L. Hou, S.-S. Park, K.-P. Lee, J.-C. Lee and W.-J. Kim, J. Environ. Chem. Eng., 2021, 9, 106417 CrossRef CAS.
- H. Chaker, A. E. Attar, M. Djennas and S. Fourmentin, Chem. Eng. Res. Des., 2021, 171, 198–212 CrossRef CAS.
- M. H. Mahmoudian, A. Mesdaghinia, A. H. Mahvi, S. Nasseri, R. Nabizadeh and M. H. Dehghani, J. Environ. Health Sci. Eng., 2022, 1–12 Search PubMed.
- K. Rasouli, A. Alamdari and S. Sabbaghi, Sep. Purif. Technol., 2023, 307, 122799 CrossRef CAS.
- H. Chaker, N. Ameur, K. Saidi-Bendahou, M. Djennas and S. Fourmentin, J. Environ. Chem. Eng., 2021, 9, 104584 CrossRef CAS.
- N. Mohammed, P. Palaniandy, F. Shaik, H. Mewada and D. Balakrishnan, Chemosphere, 2023, 314, 137665 CrossRef CAS PubMed.
- H. Ali, M. Yasir, F. A. Ngwabebhoh, T. Sopik, O. Zandraa, J. Sevcik, M. Masar, M. Machovsky and I. Kuritka, J. Photochem. Photobiol., A, 2023, 114733 CrossRef CAS.
- R. Tabaraki and O. Abdi, J. Iran. Chem. Soc., 2020, 17, 839–846 CrossRef CAS.
- M. Berkani, M. K. Bouchareb, M. Bouhelassa and Y. Kadmi, Top. Catal., 2020, 63, 964–975 CrossRef CAS.
- N. Mohammed, P. Palaniandy, F. Shaik, B. Deepanraj and H. Mewada, Environ. Res., 2023, 115696 CrossRef CAS PubMed.
- S. K. Kassahun, Z. Kiflie, H. Kim and A. F. Baye, Environ. Technol. Innovation, 2021, 23, 101761 CrossRef CAS.
- M. Rostami, H. Mazaheri, A. Hassani Joshaghani and A. Shokri, Int. J. Eng., 2019, 32, 1074–1081 CAS.
- B. Lee, M. Mahtab, T. Neo, I. Farooqi and A. Khursheed, J. Water Process Eng., 2022, 47, 102673 CrossRef.
- A. Oulebsir, T. Chaabane, S. Zaidi, K. Omine, V. Alonzo, A. Darchen, T. Msagati and V. Sivasankar, Arabian J. Chem., 2020, 13, 271–289 CrossRef CAS.
- M. S. A. Wahab, S. Abd Rahman and R. A. Samah, Heliyon, 2020, 6, e05610 CrossRef PubMed.
- M. T. Luiz, J. S. R. Viegas, J. P. Abriata, F. Viegas, F. T. M. de Carvalho Vicentini, M. V. L. B. Bentley, M. Chorilli, J. M. Marchetti and D. R. Tapia-Blacido, Eur. J. Pharm. Biopharm., 2021, 165, 127–148 CrossRef PubMed.
- M. Eftekhari, M. Akrami, M. Gheibi, H. Azizi-Toupkanloo, A. M. Fathollahi-Fard and G. Tian, Environ. Sci. Pollut. Res., 2020, 27, 43999–44021 CrossRef CAS PubMed.
- A. Raychaudhuri and M. Behera, J. Hazard., Toxic Radioact. Waste, 2020, 24, 04020013 CrossRef.
- N. Parmar and J. K. Srivastava, J. Indian Chem. Soc., 2022, 99, 100584 CrossRef CAS.
- R. Tangsiri and A. Nezamzadeh-Ejhieh, Chem. Phys. Lett., 2020, 758, 137919 CrossRef CAS.
- A. Asfaram, M. Ghaedi, F. Yousefi and M. Dastkhoon, Ultrason. Sonochem., 2016, 33, 77–89 CrossRef CAS PubMed.
- A. E. Attar, H. Chaker, M. Djennas and S. Fourmentin, Inorg. Chem. Commun., 2022, 110210 CrossRef CAS.
- L. A. Meira and F. de Souza Dias, Microchem. J., 2017, 130, 56–63 CrossRef CAS.
- T. Sudha, G. Divya, J. Sujaritha and P. Duraimurugan, J. Pharm. Res., 2017, 7(8), 550–565 Search PubMed.
- A. Khataee, M. Kasiri and L. Alidokht, Environ. Technol., 2011, 32, 1669–1684 CrossRef CAS PubMed.
- C. Song, X. Li, L. Wang and W. Shi, Sci. Rep., 2016, 6, 1–12 CrossRef PubMed.
- S. Sohrabi and F. Akhlaghian, Process Saf. Environ. Prot., 2016, 99, 120–128 CrossRef CAS.
- E. Nascimben Santos, Z. László, C. Hodúr, G. Arthanareeswaran and G. Veréb, Asia-Pac. J. Chem. Eng., 2020, 15, e2533 CrossRef CAS.
- M. Fathinia, A. Khataee, M. Zarei and S. Aber, J. Mol. Catal. A: Chem., 2010, 333, 73–84 CrossRef CAS.
- C. Sahoo and A. Gupta, J. Hazard. Mater., 2012, 215, 302–310 CrossRef PubMed.
- R. Hasanzadeh, P. Mojaver, T. Azdast, S. Khalilarya and A. Chitsaz, Int. J. Hydrogen Energy, 2023, 48, 5873–5886 CrossRef CAS.
- V. Shrivastava, I. Ali, M. M. Marjub, E. R. Rene and A. M. F. Soto, Chemosphere, 2022, 293, 133553 CrossRef CAS PubMed.
- J. Xi, H. Sun, D. Wang, Z. Zhang, X. Duan, J. Xiao, F. Xiao, L. Liu and S. Wang, Appl. Catal., B, 2018, 225, 291–297 CrossRef CAS.
- S. Chu, Y. Pan, Y. Wang, H. Zhang, R. Xiao and Z. Zou, J. Mater. Chem. A, 2020, 8, 14441–14462 RSC.
- X. Zhao, J. Feng, J. Liu, J. Lu, W. Shi, G. Yang, G. Wang, P. Feng and P. Cheng, Adv. Sci., 2018, 5, 1700590 CrossRef PubMed.
- H. Zhou, X. Sheng, J. Xiao, Z. Ding, D. Wang, X. Zhang, J. Liu, R. Wu, X. Feng and L. Jiang, J. Am. Chem. Soc., 2020, 142, 2738–2743 CrossRef CAS PubMed.
- J. Wang and Z. Liu, TrAC, Trends Anal. Chem., 2020, 133, 116089 CrossRef CAS.
- Z. Qin, Y. Li and N. Gu, Adv. Healthcare Mater., 2018, 7, 1800347 CrossRef PubMed.
- A. A. Barzinjy, S. M. Hamad, S. Aydın, M. H. Ahmed and F. H. Hussain, J. Mater. Sci.: Mater. Electron., 2020, 31, 11303–11316 CrossRef CAS.
- Y. Zou, B. Huang, L. Cao, Y. Deng and J. Su, Adv. Mater., 2021, 33, 2005215 CrossRef CAS PubMed.
- J. M. Aguirre-Cortés, A. I. Moral-Rodríguez, E. Bailón-García, A. Davó-Quiñonero, A. F. Pérez-Cadenas and F. Carrasco-Marín, Appl. Mater. Today, 2023, 32, 101831 CrossRef.
- F. Lamberti, C. Mazzariol, F. Spolaore, R. Ceccato, L. Salmaso and S. Gross, Sustainable Chem., 2022, 3, 114–130 CrossRef CAS.
- D. Tetzlaff, S. K. Carey, J. P. McNamara, H. Laudon and C. Soulsby, Water Resour. Res., 2017, 53, 2598–2604 CrossRef.
- F.-Y. Chen, Z.-Y. Wu, Z. Adler and H. Wang, Joule, 2021, 5, 1704–1731 CrossRef CAS.
- J. Annamalai, S. B. Ummalyma, A. Pandey and T. Bhaskar, Environ. Sci. Pollut. Res., 2021, 28, 49362–49382 CrossRef CAS PubMed.
- I. M. Fukuda, C. F. F. Pinto, C. D. S. Moreira, A. M. Saviano and F. R. Lourenço, Braz. J. Pharm. Sci., 2018, 54 DOI:10.1590/s2175-97902018000001006.
|
This journal is © The Royal Society of Chemistry 2023 |