DOI:
10.1039/D1TC02030G
(Perspective)
J. Mater. Chem. C, 2021,
9, 11619-11639
Colorimetric metasurfaces shed light on fibrous biological tissue
Received
2nd May 2021
, Accepted 5th August 2021
First published on 6th August 2021
Abstract
Fiber-affecting diseases – encompassing fibrosis, heart disease, neurological disease and cancer – are directly linked to the density and reorganization of fibrous media in biological tissue. Polarized light has unique capabilities to probe the structural reorganization of fibrous biological media, yet naturally occurring anisotropic light–matter interactions lie below thresholds relevant for disease visualization. Nano-optical metasurfaces have demonstrated immense capabilities to enhance light–matter interactions. However, beyond the initial focus of the field on energetic enhancement, the study of nano-optical materials which selectively enhance anisotropic light–matter interactions is at its infancy. This perspective highlights how colorimetric metasurfaces, with abilities to quantitatively map the polarization state of light onto structural color, hold great potential to visualize disease in fibrous biological tissue. We first discuss a range of major fiber-affecting diseases, where the role of tissue microstructure in disease progression has been characterized with polarized light, with a focus on Mueller Matrix polarimetry. Subsequently, an overview of state-of-the-art polarization-sensitive colorimetric metasurfaces in plasmonic, dielectric, hybrid and tunable platforms is presented. The insights provided forge a path to bridge the fields of tissue polarimetry and colorimetric metasurfaces for miniaturized, all-optical and label-free disease visualization enabled by the nanoscale science of light.
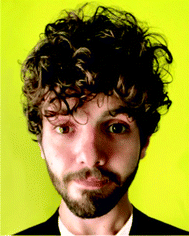
Zaid Haddadin
| Zaid Haddadin is a graduate student in Electrical Engineering at the University of California, San Diego. He holds a BSc degree in Computer Science and Biology from Purdue University. As part of the Poulikakos Lab, Zaid is working towards his interests in medical diagnostics by designing, optimizing, and fabricating nanophotonic surfaces for the purposes of detecting fiber-affecting diseases and discriminating between diseased & healthy tissue states. |
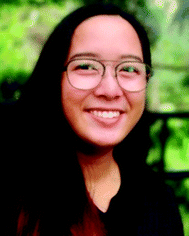
Trinity Pike
| Trinity Pike holds a BSc degree in Mechanical Engineering from the University of California, San Diego. She has previously served as an undergraduate research assistant for Project Lim[b]itless, an initiative that aims to make prosthetic limbs more accessible and affordable. Her work with the Poulikakos Lab investigates the optics and spectroscopy of nanostructures and organic materials. |
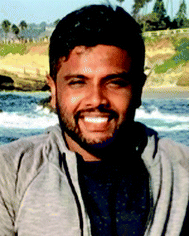
Jebin J. Moses
| Jebin J. Moses holds a MSc degree in Nanoengineering from the University of California, San Diego. He previously earned his BTech degree in Chemical Engineering from Anna University. In the Poulikakos Lab, his research interests include the design and nanofabrication of polarization-tunable, colorimetric, dielectric metasurfaces for tissue imaging applications. |
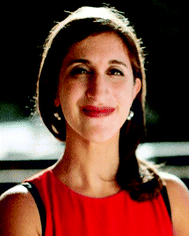
Lisa V. Poulikakos
| Lisa V. Poulikakos is an Assistant Professor in Mechanical and Aerospace Engineering at UC San Diego. Her research furthers the science of nanophotonic materials to controllably enhance, probe, and influence nanoscale light–matter interactions. She received her PhD from ETH Zürich, where she introduced an original theoretical and experimental technique enabling rational design of chiral nanophotonic systems. Her postdoctoral research at Stanford University focused on developing functional nanophotonic surfaces for cancer tissue diagnostics. She received the ETH Medal for outstanding doctoral theses, the L’Oréal USA For Women in Science Postdoctoral Fellowship, the Swiss National Science Foundation Early Postdoc Mobility Fellowship and she is a Fellow of the RCSA Scialog on Advancing BioImaging. |
Introduction
The presence and orientation of fibrous media in biological tissue is indicative of the origin and progression of a range of serious diseases, affecting organs across the human body from fibrosis1,2 to heart disease3,4 and neurological disease5,6 or various cancers.7–11 While current practice in the clinic and biomedical industry has established technologies to understand and characterize disease on the cellular, genetic and molecular level,12 the visualization of tissue microstructure – a critical indicator of fiber-affecting diseases – remains challenging.13,14
Polarized light interacts selectively with anisotropic matter, including fibrous biological tissue, where the presence and organization of fibrous media, e.g. ordered structural proteins in the extracellular matrix, distinctly alters the polarization state of light.15,16 Polarized light thus presents a unique opportunity to selectively visualize the microstructural properties of fibrous biological tissue, with potential to elucidate the origin and progression of fiber-affecting diseases in a label-free, non-invasive manner.
However, the inherent weakness of anisotropic optical properties in fibrous biological tissue has necessitated sophisticated optical configurations to probe and analyze the selective interaction with polarized light, as simpler methods such as polarized light microscopy face challenges in sensitive and quantitative readout.17 Examples of existing technologies include confocal microscopy,18–20 second harmonic generation (SHG) microscopy,21,22 polarization-sensitive optical coherence tomography (PS-OCT),23–25 or Stokes and Mueller Matrix polarimetry;15,26,27 the last of which is discussed in detail in this work, as it fully quantifies the interaction of a medium of interest with polarized light. While these and other methodologies have made significant strides toward revealing the role of tissue microstructure in disease, the cost and complexity of the required optical components and configurations, including modulated optoelectronics, lasers and extensive data post-processing, pose significant barriers to clinical and industrial applications.21,28 Indeed, hand-held devices in PS-OCT29 and Mueller Matrix polarimetry30–32 have made steps toward clinical translation, yet their underlying experimental design faces the same challenges as their benchtop counterparts.28
Here we provide a perspective on how the nanoscale science of light delivers uniquely suitable tools to address the challenge of polarization-sensitive imaging in fibrous biological tissue. In particular, nano-optical metasurfaces, composed of sub-wavelength-periodically arranged nanostructures with versatile optical properties, have been demonstrated to scale down and enhance the complex manipulation of light on a single optical surface, thus enabling a host of miniaturized, mobile and translatable applications.33–35
The concentrated electromagnetic near fields generated by optical nanostructures exhibit high sensitivity to changes in the refractive index environment, which has been leveraged for a variety of biosensing applications,36–38 while at infrared frequencies surface-enhanced spectroscopy enabled by optical nanoantennas has achieved ultrasensitive monitoring of molecular composition.39–41
Nano-optical metasurfaces have additional abilities to selectively detect the structural properties of biological matter. The chirality of biomolecules, embodied in the left- and right-handed arrangement of their molecular components, has been detected with enhanced sensitivity and selectivity by means of metasurfaces which enhance chiral light–matter interactions either by their chiral geometric configuration,42–53 or their distinct interaction with chiral light.54–58
Expanding on this concept, metasurfaces have been designed to exhibit material anisotropy in the geometry or assembly of their nanoresonator components, resulting in full phase and polarization control of incident light,59,60 while further work demonstrated full Stokes polarimetry on a single optical metasurface,61–64 paving the way toward a host of miniaturized bioimaging applications.65–67 While many of the abovementioned metasurfaces showcase abilities to probe light polarization with high sensitivity and selectivity, their readout remains encoded in the physics of polarimetry, posing barriers to the extraction of the material properties of interest and subsequently to widespread clinical and industrial applications.
To address this challenge, this perspective focuses on a further, formative property of nano-optical metasurfaces: their ability to generate structural color, which to date has been proposed for applications in display technology,68,69 miniaturized color printing70,71 or refractive-index sensing.68,72 In the context of biomedical imaging, colorimetry is arguably the most intuitive and translatable optical sensing metric, circumventing the need for spectroscopic analysis.
Here, we outline why structural color in metasurfaces has great potential for polarization-sensitive imaging of fibrous biological tissue. We first introduce the basic quantitative principles of polarized light–matter interactions, focusing on Mueller Matrix formalism. Subsequently, we discuss a range of representative fiber-affecting diseases, applicable to organs across the human body (Fig. 1). We highlight how the tissue microstructural properties of various disease states have been characterized in terms of their interaction with polarized light by Mueller Matrix polarimetry, thus showcasing how polarization-sensitive analysis provides critical information on disease state and progression. An overview of polarization-sensitive colorimetric metasurfaces is then presented, highlighting progress in this field for metallic, dielectric, hybrid and tunable material platforms. Finally, we discuss the opportunities and challenges of bridging these two fields where colorimetric, polarization-sensitive metasurfaces provide an accessible, miniaturized and translatable platform to visualize fiber-affecting disease.
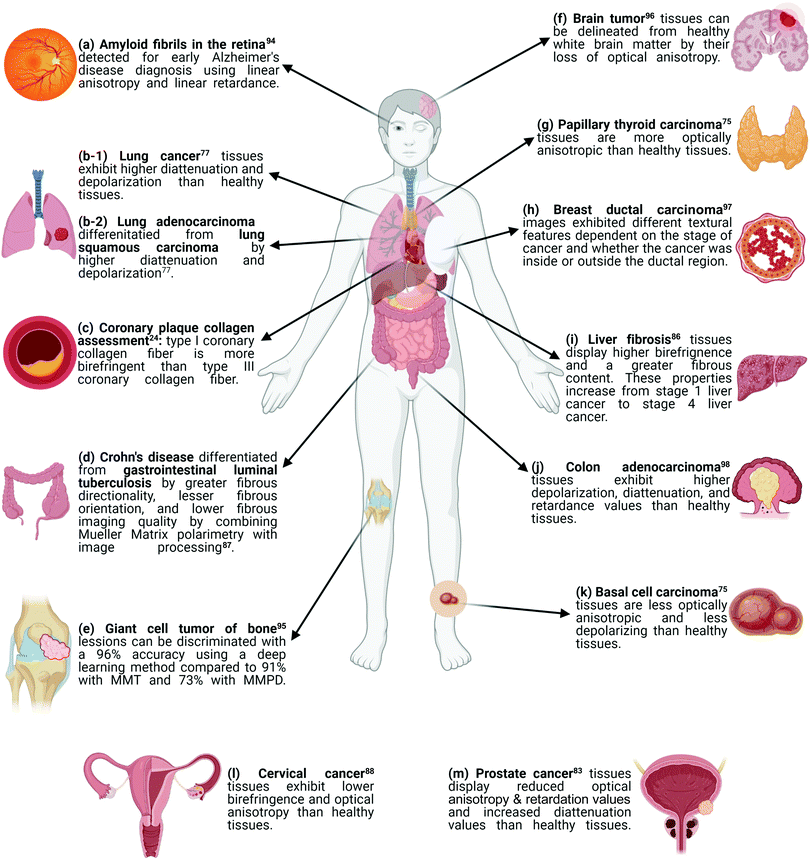 |
| Fig. 1 A visual summary of how disease states can be differentiated and determined using polarimetric methods. Parts (a) through (m) correspond to data entries in Table 1, which summarizes the experimental setup specifications for the following diseases: (a) amyloid fibrils in the retina,90 (b-1) lung cancer,77 (b-2) lung adenocarcinoma and lung squamous carcinoma,77 (c) coronary plaque collagen,24 (d) Crohn's disease and gastrointestinal luminal tuberculosis,86 (e) giant cell tumor of the bone,91 (f) brain tumor,92 (g) papillary thyroid carcinoma,75 (h) breast ductal carcinoma,93 (i) liver fibrosis,85 (j) colon adenocarcinoma,94 (k) basal cell carcinoma,75 (l) cervical cancer,87 (m) prostate cancer.83 Created with BioRender.com. | |
Quantifying polarized light–matter interactions
Stokes parameters
The polarization state of light can be quantified by the Stokes parameters, written in vector form as | 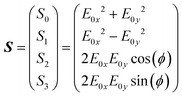 | (1) |
where E0x(y) represents the amplitude of the incident electric field in x and y orientation, and ϕ = ϕx − ϕy represents the phase difference between x and y components of the electric field. The zeroth Stokes parameter, S0, describes the total intensity of the optical beam and the first Stokes parameter, S1, represents the differential intensity of horizontally vs. vertically polarized light. The differential intensity of linearly polarized light at ±45° is quantified by the second Stokes parameter, S2. Finally, the third Stokes parameter, S3, describes the differential intensity of left- and right-handed circularly polarized light.26,73
Mueller matrix
The linear interaction between polarized light and a medium of interest is fully quantified by means of the Mueller Matrix | 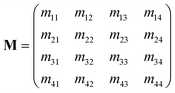 | (2) |
a 4 × 4 matrix which relates the input and output polarization state of light, represented by the Stokes’ vectors Sin and Sout, as26,27,74In complex, optically anisotropic media such as biological tissue, the Mueller Matrix elements simultaneously embody a variety of phenomena which can pose challenges to their interpretation.27 Thus, Mueller Matrix decomposition methods have been successfully implemented to facilitate physical interpretation, as described below.
Mueller Matrix Polar Decomposition (MMPD)15 describes the breakdown of the Mueller Matrix into three constituent matrices that describe the physical phenomena of depolarization (MΔ), diattenuation (MD), and retardance (MR)15,75–77 as
all of which have relevant occurrence in biological tissue. Depolarization represents a decrease in the degree of polarization of light upon traversing a medium, quantified by the net depolarization factor
Δ ∈ [0,1], where
Δ = 1 corresponds to fully polarized light.
78 In biological tissue, depolarization commonly occurs due to multiple scattering within the turbid medium, an effect which increases with penetration depth, becoming particularly significant for thicknesses above 0.5 mm.
79,80
Decreases in the degree of linear and circular polarization, can be directly obtained from the Mueller Matrix elements as77,81
| 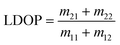 | (5) |
| 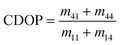 | (6) |
where LDOP and CDOP denote the linear and circular degree of depolarization, respectively. Accordingly, the linear depolarization is written as 1 − LDOP, while 1 − CDOP is the circular depolarization.
Diattenuation occurs when orthogonal polarization states exhibit a differential attenuation upon interaction with a medium, which can be defined accordingly for orthogonal linear or circular polarization states. From the first row of the Mueller Matrix (eqn (2)) the diattenuation value can be obtained as77
| 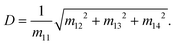 | (7) |
Retardance describes a phase shift occurring between orthogonal polarization states of light, where
δ denotes linear retardance, also known as birefringence, and
ψ denotes circular retardance, also known as optical rotation, while the optical axis orientation angle of the medium is written as
θ.
26,76 The total retardance
R ∈ [0,π] is obtained from the Mueller Matrix and allows for further material classification.
26,76
Mueller Matrix Transformation (MMT)82,83 is a further method by which a physically-relevant set of parameters, which provide information about the microstructural properties of anisotropic media, are extracted from the Mueller Matrix. The MMT parameters A, b, t and x are written as75,82,84–87
|  | (8) |
|  | (9) |
| 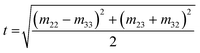 | (10) |
| 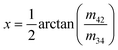 | (11) |
wherein
A represents the degree of anisotropy and characterizes the order of alignment of fibrous media. Isotropic and anisotropic media yield
A values of 0 and 1, respectively. The parameter
b is related to the depolarization ability of bulk tissues while
t is sensitive to the degree of anisotropy in scattering matter. Finally,
x is related to orientation directions of aligned fibrous structures.
Measurement of the Mueller Matrix can be achieved with Mueller Matrix polarimetry. This has been realized in transmission and backscattering configurations and, in certain cases, has been integrated in commercial microscopes (Fig. 2(a)),86,88 where the polarization state of the incident light is controlled by a polarization state generator (PSG) and the output polarization state is measured by a polarization state analyzer (PSA), both of which are composed of a frequency-modulated element, e.g. a photoelastic modulator (PEM), and polarizer arranged sequentially. The 16 Mueller Matrix elements are obtained in a series of measurements, where the input polarization state of light is varied between four polarization states and the four Stokes parameters are obtained by analysis of the output polarization state in each case.26,89–91
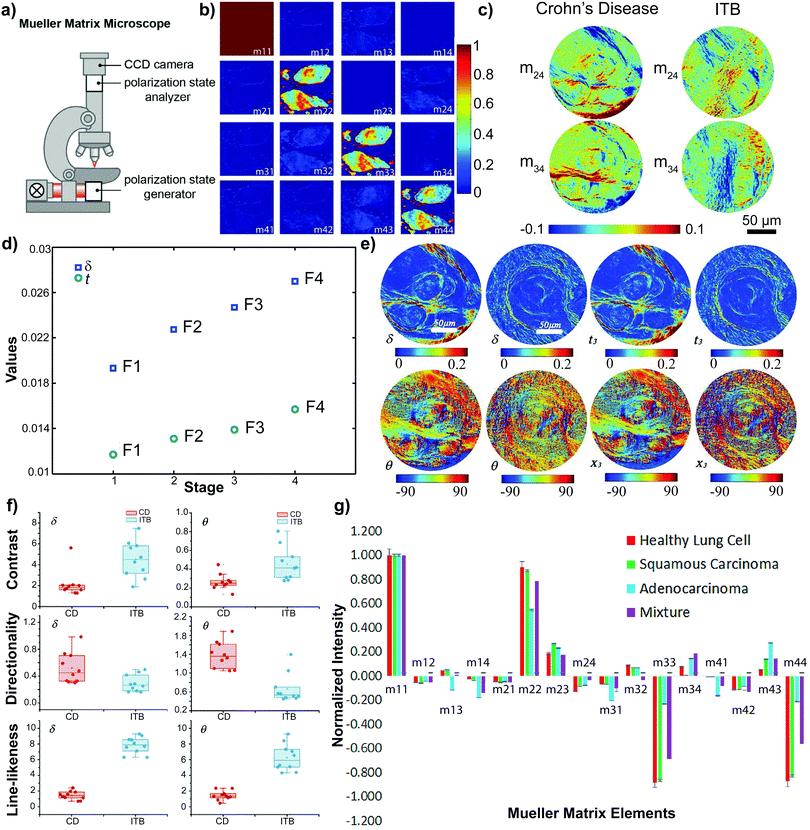 |
| Fig. 2 (a) A Mueller Matrix microscope created by adding a polarization state generator (PSG) and analyzer (PSA) to a commercially available light microscope. (b) The normalized Mueller Matrix of basal cell carcinoma tissues.75 (c) The m24 and m34 elements of the normalized Mueller Matrix of Crohn's disease and gastrointestinal luminal tuberculosis (ITB).87 (d) The average experimental values of MMPD δ (blue squares) and MMT t (green circles) parameters in different stages of liver cancer.86 (e) MMPD δ & θ and MMT t & x parameters of Crohn's disease and gastrointestinal luminal tuberculosis.87 The left image of any parameter is of a Crohn's disease sample. The right image of any parameter is a gastrointestinal luminal tuberculosis sample. (f) Box plots of an imaging analysis technique performed on 10 Crohn's disease (CD) samples (red dots) and 10 gastrointestinal luminal tuberculosis (ITB) samples (blue dots).87 (g) Mueller Matrix plot for healthy lung cells (red), lung squamous carcinoma cells (green), lung adenocarcinoma cells (blue) and a 50/50 mixture of squamous carcinoma & adenocarcinoma cells (purple).77 (b–d) Reprinted (adapted) from ref. 75, 86 and 87 with permission from SPIE Digital Library. (g) Reprinted/adapted from ref. 77 with permission from Elsevier. | |
Characterizing fibrous tissue with polarized light
This section highlights applications of polarimetric imaging methods for the detection of diseased states in fibrous biological tissue. The first section titled Characterizing Individual Disease States focuses on research that attempts to differentiate a diseased state from its healthy counterpart. The second section titled Differentiating Among Disease Stages highlights how Muller Matrix polarimetry has been used to describe the progression of a single diseased state. The third section titled Discriminating Between Disease States features polarimetric applications for discerning between different diseases that display similar diagnostic characteristics. Finally, the Miscellaneous Applications section showcases some unique applications of polarimetry beyond the categories of the previous sections. Relevant equations and variables used to quantify polarized light–matter interactions have been defined in the previous section. Fig. 1 summarizes the diseases discussed in the context of their polarizing optical properties, while Table 1 provides an overview of the experimental specifications required for the presented research.
Table 1 The experimental polarimetric setup specifications for different disease states
|
Tissue sample |
Thickness (mm) |
Light source |
Wavelength (nm) |
Power (mW) |
Experimental geometry |
Available data in literature |
The letters in the leftmost column correspond to the disease state described in Fig. 1. Abbreviations: LDOP: linear degree of polarization; MM: Mueller Matrix; MMPD: Mueller Matrix polar decomposition; MMT: Mueller Matrix transformation; NMM: normalized Mueller Matrix. |
a |
Retina94 |
— |
— |
— |
— |
— |
Intensity map: linear retardance & linear anisotropy |
b |
Normal lung fibroblast77 |
0.005 |
Laser diode |
1065 |
— |
Backscattering |
Numerical values: NMM |
b |
Lung squamous cell carcinoma (LUSC)77 |
0.005 |
Laser diode |
1065 |
— |
Backscattering |
Numerical values: NMM |
b |
Lung adenocarcinoma (LUAC)77 |
0.005 |
Laser diode |
1065 |
— |
Backscattering |
Numerical values: NMM |
b |
Mixture of LUSC & LUAC77 |
0.005 |
Laser diode |
1065 |
— |
Backscattering |
Numerical values: NMM |
d |
Crohn's disease87 |
0.012 |
LED |
633 |
3000 |
— |
Intensity map: MM, MMPD, & MMT |
d |
Gastrointestinal luminal tuberculosis87 |
0.012 |
LED |
633 |
3000 |
— |
Intensity map: MM, MMPD, & MMT |
e |
Giant cell tumor of bone95 |
— |
Halogen lamp |
— |
— |
— |
Intensity map: MM |
f |
Human brain96 |
60 × 90 × 10 |
— |
550 |
— |
Reflection |
Intensity map: depolarization & retardance |
f |
Calf brain96 |
90 × 70 × 10 |
— |
550 |
— |
Reflection |
Intensity map: depolarization & retardance |
g |
Papillary thyroid carcinoma75 |
0.028 |
LED |
650 |
3000 |
Backscattering |
Intensity map: NMM, LDOP, MMPD, & MMT |
h |
Breast ductal carcinoma97 |
0.012 |
LED |
632 |
3000 |
Backscattering |
Intensity map: MMPD & MMT |
i |
Liver fibrosis86 |
0.008 |
LED |
632 |
3000 |
Backscattering |
Intensity map: MMPD & MMT |
j |
Colon adenocarcinoma98 |
0.003 |
Xenon lamp |
425 |
150 |
— |
Numerical values: MM & MMPD |
k |
Basal cell carcinoma75 |
0.028 |
LED |
650 |
3000 |
Backscattering |
Intensity map: NMM, LDOP, MMPD, & MMT |
l |
Cervical cancer88 |
0.02 |
LED |
632 |
3000 |
Backscattering |
Intensity map: MMPD & MMT |
m |
Prostate cancer83 |
— |
Laser diode |
632.8 |
— |
Backscattering |
Intensity map: NMM & MMPD |
Characterizing individual disease states
Basal cell carcinoma (BCC) Fig. 1(k).
Du et al. differentiated healthy and cancerous tissues affected by basal cell carcinoma (BCC) by using parameters from three different polarization imaging techniques: linear degree of polarization (LDOP), Mueller Matrix polar decomposition (MMPD), and Mueller Matrix transformation (MMT).75
The backscattering Mueller Matrix of unstained BCC samples (Fig. 2(b)) measured nondiagonal elements to be close to zero, indicating isotropic optical properties which may be attributed to an absence of well-aligned fibers in cancerous regions.75,84,92 This indication was confirmed with measurements approaching zero for the linear retardance (δ) and the anisotropic MMT parameter A. Moreover, cancerous regions presented larger values of LDOP, D, and b than those in healthy regions, while the Δ value was smaller than in healthy regions.75 These findings imply that cancerous regions of BCC tissues exhibit lower depolarization values than healthy regions.75 As such, cancerous regions of BCC tissue samples can be characterized as less anisotropic and less depolarizing than their healthy tissue counterparts.
Cervical cancer (CEC) Fig. 1(l).
An examination by Wang et al. of human cervical cancerous tissues (CEC) using an ordinary, commercially available light microscope with a polarization state generator (PSG) and analyzer (PSA) (Fig. 2(a)) showed that while cancerous regions are more birefringent, they exhibit a lower uniform directionality in their fibers than their healthy counterparts.88 The purpose of this study was two-fold: (1) to show how a clinically-available microscope can be altered to measure the Mueller Matrix elements of a tissue sample, and (2) to differentiate the characteristic microstructural features of the examined cancerous tissues through measurements of the Mueller Matrix polar decomposition (MMPD) and Mueller Matrix transformation (MMT) parameters.88
The δ and t values, obtained from MMPD and MMT, respectively, were observed to be smaller in cancerous regions of CEC tissue samples than in healthy regions, indicative of decreased birefringence.88,92 Moreover, parameters characteristic of the direction of aligned fibers were lower in cancerous regions than in healthy regions, implying that CEC tissues exhibit lower optical anisotropy than their healthy counterparts.84,88,93
Colon adenocarcinoma (COA) Fig. 1(j).
Ahmad et al. aimed to study whether Mueller Matrix polarimetry can be used to differentiate between ex vivo normal and adenocarcinoma colon tissue samples in the 425–725 nm visible spectral range by characterizing the Mueller Matrix polar decomposition (MMPD).98 It was found that total (Δ) and linear depolarization (δ), diattenuation (D), and retardance (R) of the MMPD parameters were significantly higher for the adenocarcinoma colon samples than the healthy colon samples.98
These results are in accordance with previous studies showing how alterations to the adenocarcinoma tissue architecture result in malignant glands of varying sizes and disorganized fibrous stroma. These physical changes in turn increased the depolarization and affected the anisotropic properties of diseased colon tissues.93,98–100 Using statistical and algorithmic analysis,98,101–103 depolarization and retardance were determined as good candidates for the discrimination of the normal and adenocarcinoma colon tissues.98 Normal and adenocarcinoma colon samples were algorithmically characterized with 100% accuracy, sensitivity, and specificity for total (Δ) and linear (δ) depolarization as well as retardance (R).98 Through this work, Ahmad and colleagues showed how the Mueller Matrix can be used in combination with statistical and algorithmic analysis for the identification and classification of normal and adenocarcinoma colon tissue samples, permitting for future research to utilize this application toward automated and non-invasive cancer diagnosis.98
Giant cell tumor of bone (GCTB) Fig. 1(e).
Giant cell tumor of bone (GCTB) diagnosis has low efficiency as it depends on experience of histopathologists and requires a triple diagnosis with input from orthopedic surgeons, radiologists, and pathologists.95,104,105 To combat this drawback, Zhao et al. developed a deep learning model106 that jointly uses histopathological samples, Mueller Matrix polar decomposition (MMPD) parameters and Mueller Matrix transformation (MMT) parameters to predict whether a histological bone sample is healthy or contains a cancerous lesion.95
When characterizing images of cancerous lesions in histological bone samples, the deep-learning model showed the highest accuracy of ∼96% when compared to characterization through MMT (∼91% accuracy) or MMPD (∼73% accuracy).95 Through this research effort, Zhang and colleagues demonstrated a proof-of-concept for the use of deep learning models in the detection of cancerous versus healthy regions of tissues.95 Moreover, their ability to quantify how well MMT and MMPD characterize the differences in diseased and healthy states elucidates how, despite the abundance of information contained in both types of Muller Matrix decomposition, one polarization imaging technique may be more sensitive and accurate to a certain disease state than the other.95 For example, MMT is said to be orientation-insensitive, potentially explaining its improved performance with respect to MMPD in the study of Zhang et al.84,88,95
Papillary thyroid carcinoma (PTC) Fig. 1(g).
Polarimetric parameters obtained from the linear degree of polarization (LDOP), Mueller Matrix polar decomposition (MMPD), and Mueller Matrix transformation (MMT) were used to characterize and differentiate papillary thyroid carcinoma (PTC) tissues from their healthy tissue counterparts.75 Du et al. utilized the backscattering configuration of Mueller Matrix polarimetry to obtain their results.75
The Mueller Matrix of unstained PTC samples measured nondiagonal elements to be non-negligible, indicative that the PTC tissue samples are optically anisotropic.75,84 This conclusion was further confirmed by Mueller Matrix analysis where LDOP, the MMT parameter b and the MMPD parameters did not provide an indication of the cancerous region. However, the MMT parameter A revealed the cancerous region.75 This finding suggested the existence of well-aligned structures at the cancerous regions of the tissues, a result that is in conjunction with previous research showing that a morphological feature of PTC is fibroplasia, the development of fibrous structures.75,107 Moreover, it highlights that the MMT parameter A may be used as a discriminatory variable to differentiate between cancerous and healthy regions in tissues afflicted by papillary thyroid carcinoma.75
Prostate cancer (PRC) Fig. 1(m).
Backscattered Mueller Matrix imaging was utilized by Badieyan et al. to extract the characteristic optical features of bulk prostate tissues retrieved immediately after surgery. The aim of this study was to quantify a difference between prostate cancer (PRC) tissues and healthy prostate tissues through measurements of the Mueller Matrix polar decomposition (MMPD) parameters and the Mueller Matrix transformation (MMT) parameters, alongside statistical analysis. The obtained MMPD and MMT values can be seen in Table 2.
Table 2 MMPD & MMT values of cancerous prostate tissues and healthy prostate tissues
|
MMPD |
MMT |
Sample |
Diattenuation |
Retardance |
Depolarization |
A
|
b
|
t
|
x
|
Cancerous prostate tissues have higher MMPD diattenuation and depolarization values. Healthy prostate tissues have higher MMPD retardance and MMT A & b values. MMT values x and t were not provided in the literature. |
Cancerous prostate tissue |
0.7419 |
0.1648 |
0.8864 |
0.2050 |
0.0955 |
— |
— |
Healthy prostate tissue |
0.7176 |
0.5094 |
0.8636 |
0.3140 |
0.1565 |
— |
— |
The cancerous prostate tissues displayed higher values for the MMPD diattenuation (D) and depolarization (Δ) parameters than their healthy counterpart. Lower values were obtained for the MMPD retardance (R) and the MMT A & b parameters of cancerous samples than healthy prostate tissue.83 These values are in accordance with the rapid growth of cancerous tissues leading to a reduction in the order of alignment of the fibers. The subsequent lowering of the optical anisotropy is seen in the reduced MMPD retardance (R) and MMT A values. An increased absorption of light, leading to higher diattenuation, then follows with an increased cell density.83,108 Overall, these results indicated how changes in optical anisotropy can be powerful differentiators of healthy and cancerous prostate tissues, providing distinct information on the role of microstructure in disease progression.83
Differentiating among disease stages
Breast ductal carcinoma (BDC) Fig. 1(h).
The gold standard for breast cancer diagnosis has relied on histopathological observations that depend on the experience of pathologists.105,109 However, previous studies have shown that the progression of breast cancer affects the collagen structure around ducts and tumors in intact, unfixed, and non-sectioned mammary glands.8 In their study, Dong et al. characterized the progression of invasive breast ductal carcinoma (BDC) across disease stages 1 through 3 by segmenting the ductal areas in images of the Mueller Matrix polar decomposition (MMPD) and measuring their textural features.97,110
Dong and colleagues found, when compared to stage 1 cancerous tissues, stage 2 cancerous tissues showed MMPD linear retardance (δ) images with higher texture contrast and lower texture uniformity outside the ductal region. Inside and outside the ductal areas, images of the MMPD orientation (θ) in stage 2 tissue showed decreased texture contrast and increased linear dependence of grey tones across neighboring pixel areas.97 For stage 3 tissue, the MMPD δ parameter exhibited an increase in texture contrast inside the duct and an increase in texture uniformity outside the duct, in comparison to stage 2. Additionally, images of the MMPD θ parameter for stage 3 tissue showed an increase in texture contrast and a decrease in the linear dependence of grey tones outside the ducts.97 This study thus demonstrated how the Mueller Matrix can be assessed with an image textural analysis technique. The resulting quantitative characteristics aid pathologists to better characterize stages of progression in ductal breast cancer.97
Liver fibrosis (LVF) Fig. 1(i).
An examination of human liver cancer tissues by Wang et al. showed that cancerous regions were more birefringent with higher fibrous density than their healthy counterparts.88 Their measurements of the Mueller Matrix polar decomposition (MMPD) and the Mueller Matrix transformation (MMT) parameters exhibited higher values in cancerous regions for the MMPD retardation (R) and orientation (θ) parameters as well as the MMT parameters t and x.86,88 The values of R and t characterize the linear retardance of the media while θ and x characterize the direction of the aligned fibers; thus implying the presence of anisotropic properties, such as birefringence, in the cancerous tissue.84,88,92
The progression of liver cancer is accompanied by an increased development of fibrosis resulting from inflammatory reactions.86,111 As such, Wang et al. investigated the effects of this heightened fibrotic accumulation on the MMPD and MMT parameters across the four stages of liver cancer (F1, F2, F3, & F4): the orientation of the fibers, as described by the MMPD θ and MMT x values, remained relatively consistent across the four stages. In contrast, the R and t parameters showed a marked increase from F1 to F486 (Fig. 2(d)). The R parameter of MMPD changed more prominently than the t parameter of MMT, implying that MMPD may be more sensitive to the birefringence of a sample.86 Nonetheless, through their study, Wang et al. showed that liver fibrosis disease stages can be differentiated by Mueller Matrix polarimetry.
Discriminating between disease states
Crohn's disease (CRD) vs. gastrointestinal luminal tuberculosis (ITB) Fig. 1(d).
There remains a need to find a clinically distinguishing factor for the diagnosis of Crohn's disease and intestinal tuberculosis, which exhibit similar radiological, endoscopic, and histological features.112 As no single pathognomonic trait exists for either disease, misdiagnosis can result in inappropriate treatments with side effects that risk toxicity to the patient and a delay in proper treatment. Thus, it becomes imperative to find a standard by which to discriminate between both diseases.87,112 Liu et al. applied Mueller Matrix polar decomposition (MMPD) and Muller Matrix transformation (MMT) imaging techniques to Crohn's disease (CRD) and gastrointestinal luminal tuberculosis (ITB) tissue samples. The normalized Mueller Matrix of their samples is shown in Fig. 2(c). They determined that birefringence is a dominant feature of both diseases by their retardance (MMPD parameter R and MMT parameter t) and orientation (MMPD parameter θ and MMT parameter x)87 (Fig. 2(e)). It was previously shown that the MMPD parameters are more sensitive to birefringence than MMT parameters.113
As such, Liu and colleagues applied an image processing method to the MMPD linear retardance δ and orientation θ to quantitatively describe the samples across six physical and textural features (Fig. 2(f)): coarseness, contrast, directionality, line-likeness, regularity, and roughness.87,114 These indicated that fibrous structures in ITB tissues exhibit approximately the same orientation (line-likeness) and are of higher imaging quality (contrast) than CRD tissues. In contrast, fibrous structures in CRD tissues showed a larger tendency towards a certain direction (directionality). This supported the finding that ITB tissues have smaller, more aligned fibers while CRD tissues contain longer non-uniformly distributed fibers.87,112,114 These preliminary results indicate how the Mueller Matrix can be used to discriminate between two pathologically similar diseases with potential to provide quantitative information to clinical diagnosis.87
Lung squamous carcinoma (LUSC) vs. lung adenocarcinoma (LUAC) Fig. 1(b).
Shrestha et al. aimed to incorporate the Mueller Matrix in a label-free, polarimetric imaging technique at near-infrared wavelengths for the classification of different types of histopathological lung cancer samples.77 Specifically, they utilized an imaging modality technique known as Whole Slide Imaging, wherein remote scanning of conventional histopathological samples on glass slides produces digital images for clinical examination. Whole Slide Imaging is believed to lead to increased efficiency and improved patient outcomes if implemented across the healthcare industry.77,115 The authors introduced new optical polarimetric quantities to existing digital data analysis techniques.77,116–118 The four examined histopathological samples included (1) healthy fetal lung fibroblast epithelial cells, (2) human stage II squamous carcinoma cells (LUSC), (3) human stage II adenocarcinoma cells (LUAC), and (4) a 50%:50% mixture of the human stage II squamous carcinoma cells and of the human stage II adenocarcinoma cells.77
A normalized intensity plot of the Mueller Matrix elements of histopathological samples (1)–(4) establishes the diagonal matrix elements as the best discriminators77 (Fig. 2(g)). Overall, the diattenuation (D) and depolarization (Δ) values of LUAC cells were higher than LUSC cells. Further, the D and Δ values of LUSC cells were higher than for healthy cells.77 Additional polarimetric analysis showed an increase in backscattered polarized intensities from healthy cells to LUSC cells to LUAC cells.77 This increase in polarized intensity is correlated to an increase in ratio of nucleus-to-cytoplasmic sizes of the cells – from healthy to LUSC and from LUSC to LUAC. A larger ratio contributes an overall more complex morphology. This trend correlates with the fact that cancerous cells exhibit a higher depolarization of light than healthy cells.77,93,119,120 This study indicates how the Mueller Matrix along with other optical polarimetric tools can be utilized for both the discrimination of healthy and cancerous lung tissues as well as the differentiation between types of lung cancers.77
Miscellaneous applications
Amyloid detection in the retina Fig. 1(a).
The accumulation of amyloid plaques and neurofibrillary tangles are hallmark symptoms of Alzheimer's disease. However, although their accumulation begins before symptoms of cognitive impairment appear, a definitive diagnosis based on these two symptoms can only be obtained post-mortem.94,121 Early detection of these hallmark symptoms may be needed for the development of novel treatments. To date, no seemingly effective cure exists for patients who have developed cognitive deficiencies due to Alzheimer's disease.94,122,123 Qiu et al. demonstrated a promising route toward early detection of Alzheimer's disease by utilizing machine learning methods to detect amyloid presence in the retina as a biomarker for amyloid build-up in the brain.94,124 They utilized three different machine learning algorithms for this purpose,94,125–127 where Mueller Matrix polarimetry was incorporated to distinguish the presence of amyloid deposits.
Qiu and colleagues performed post-mortem analyses to determine whether detection of amyloid deposits required the fluorescent marker thioflavin.94 Their findings indicated that it may be possible to distinguish between fluorescent and non-fluorescent retinas by their polarizing optical properties (i.e., linear anisotropy and linear retardance), which implies that the ordered arrangement of the fibrils may be key to distinguishing between them.84,93,94 Moreover, a previous study has shown that in vivo detection of retinal amyloid deposits stained with curcumin is possible as a proof-of-concept imaging trial in Alzheimer's disease.128 The data presented by Qiu et al. provide a step towards the development of non-invasive, dye-free clinical methods of imaging retinal amyloid deposits as a predictor of amyloid build-up in the brain.94,121
Assessment of coronary plaque collagen for acute coronary syndromes Fig. 1(c).
Optical coherence tomography (OCT) operates analogously to ultrasound imaging but uses light, instead of sound, to obtain higher image resolution by measuring the back reflection of near-infrared light at the micron scale.24,129 When expanded to polarization sensitive OCT (PS-OCT), the birefringence of tissues can be measured.23,24,129 Giattina et al. used this imaging technique for in vivo assessment of coronary plaque collagen to detect vulnerable artery plaques that may lead to acute coronary syndromes.24,130
Giattina and colleagues showed that PS-OCT has significant correlation in identifying birefringent properties of collagen in comparison to Picrosirius staining, a dye used for assessment of organized collagen.24,131 Moreover, it was shown that PS-OCT images can correlate the concentration of fibers with the type of fibers: type I fibers, which are denser & larger, show higher birefringence than the smaller type III fibers.24,132 The difference in birefringence and resultant appearance of type I and type III fibers was also observed when examining cardiac tissue under polarized light.3 Although only 60% of the light had diagnostic value due to partially polarized illumination,24 a relation between PS-OCT images and Picrosirius stained images was identified regarding the build-up and presence of collagen in the coronary arteries. This demonstrated the capability of PS-OCT images to assess plaque collagen content, thus making an argument for its potential in the clinic.24
White matter visualization during neurosurgical procedures Fig. 1(f).
The extent of surgical brain tumor removal is an important factor in determining the post-operative prognosis a patient may receive.96,133 However, no more than 36% of patients achieve full resection of their brain tumors.134 Challenges lie in the difficulty of delineating the boundary between solid brain tumor and white brain matter during surgery, even with intraoperative microscopes. Incomplete removal can result when too little resection occurs, while too much resection can give rise to neurological deficits.96,134 Cancer invasion leads to substantial tissue remodeling that destroys the ordered structures of fibrillary tracts, characteristic of healthy white brain matter, resulting in a loss of optical anisotropy that can be used as a delineating factor.96,135 In their 2020 paper, Schucht et al. utilized wide-field Mueller polarimetry to detect healthy white matter by its anisotropic properties, thus revealing the location of tumor tissue.96 The visualization of white brain matter was performed by deriving the azimuth of the tissue optical axis from Mueller Matrix polar decomposition (MMPD) images of thick specimens measured in reflection.96
In fixed human brain tissue and fresh animal tissue, it was found that the orientation of fiber tracts in healthy brain white matter could be visualized by the azimuth of their optical axis, obtained from backscattered Mueller Matrix images.96 This proof-of-concept study illustrates how Mueller Matrix polarimetry can be used in real time during surgical procedures for the detection of the tissue–tumor interface. Though Schucht et al. emphasized its use in neurosurgery, this technique could potentially be applicable to any fiber-affecting disease where a boundary in the tissue can be delineated with polarized light, such as those highlighted earlier in this perspective.
Polarization-sensitive colorimetric metasurfaces
Structural color is a phenomenon that occurs when nanostructures selectively scatter and absorb incident light due to variations in the index of refraction. Prime examples from nature include wings of the morpho butterfly136–138 and bird of paradise,139 feathers of hummingbirds138 and peacocks,138 or the scales of jewel beetles140 and peacock spiders.141 Compared to color arising from the absorption of light in chemical pigments, structural color offers vivid saturation, improved resolutions, and increased resistance to high temperatures.142
Recent advances in nanotechnology have enabled researchers to emulate the effects observed in nature by realizing artificial structural color. A first glimpse into this world was demonstrated by colloidal assembly of so-called photonic pigments.142 The disordered arrangement of these colloidal particles can constitute photonic glasses which generate angle-independent, non-iridescent structural color.143–145 In contrast, when optical nanostructures are arranged in ordered, periodic assemblies to form photonic crystals146,147 or metasurfaces, the symmetry or asymmetry of their geometric configuration enables additional degrees of tunability in the generation of structural color.148 For example, structural colors enable inkless color printing through controlled crazing149,150 which leverages miniature material patterns in transparent polymers to produce color, and photonic crystals, which contain periodic structures that manipulate light to generate bright, tunable colors.151
In the following sections, we provide a broad overview of structural color response in nanostructures composed of plasmonic, dielectric and hybrid material platforms. Plasmonic metasurfaces generate color response from the resonant properties of surface plasmons in metallic nanostructures.148,152,153 Dielectric metasurfaces leverage the electric and magnetic dipolar resonances of high-refractive-index dielectric nanostructures or guided-mode-resonant properties for optical output.148,154–157 Hybrid systems utilize and combine the strengths of purely plasmonic and dielectric counterparts. After introducing each material platform, we highlight examples of corresponding polarization-tunable metasurfaces. For a comprehensive review of structural color in metasurfaces we refer the reader to prior articles.148,153,158,159
Structural color in plasmonic nanostructures
Surface plasmons arise from the collective oscillation of the free-electron cloud at the surface of conductive materials.160 Noble metal nanoparticles, with surface plasmon resonances (SPRs) in the visible wavelength range, are effective generators of structural color.139 A diverse set of plasmonic nanostructures including nanoholes, nanogratings and metal–insulator–metal systems have been arranged in periodic lattices to form metasurfaces.141,161–164 The optical properties of plasmonic nanostructures exhibit a high sensitivity to their refractive index environment.152,165 The resulting tunability of SPRs has enabled a variety of applications in refractive-index-sensing which can be leveraged in biomedical applications.166 With improving nanofabrication processes, enhancement in color saturation capabilities, and high customization, plasmonic nanostructures have a wide scope of applications which utilize structural color.148
The SPR effect renders plasmonic nanostructures particularly suitable for high resolution color generation at the optical diffraction limit.148 Kumar et al. achieved bright-field color prints with diffraction-limited resolution by raising silver (Ag) or gold (Au) nanodisks above a plasmonic backreflector film via nanopillars made of hydrogen silsesquioxane (HSQ). The resulting pixels of 250 nm pitch were able to generate individual colors.167 Subsequent work by Tan et al. expanded on this system and demonstrated photorealistic color printing by varying nanodisk size, spacing and dual-size variations within a single pixel of 800 × 800 nm.168 By utilizing aluminum (Al) as the plasmonic material, a low-cost, environmentally friendly, and minimally lossy system was achieved in comparison to its Ag and Au counterparts.
Exploring various geometries of plasmonic structures has expanded possibilities for customization. For instance, configurations of nanohole arrays, where extraordinary optical transmission can hinder loss,169 have been designed to control and enhance structural color output (Fig. 3(a)).160,170,171 Additionally, nanoclusters can be leveraged to achieve an engineered color response. To this end, King et al. fabricated ultraviolet and visible Fano resonant aluminum nanoclusters to colorimetrically sense changes in refractive index in the particle surroundings (Fig. 3(b)).172 Though most nanohole color filters are polarization insensitive due to the symmetric nature of the nanohole lattice,161,173 the work of Dai et al. showed an added benefit of utilizing a triangular lattice array, which enabled improved color purity and a resolution of 12
700 dpi.161
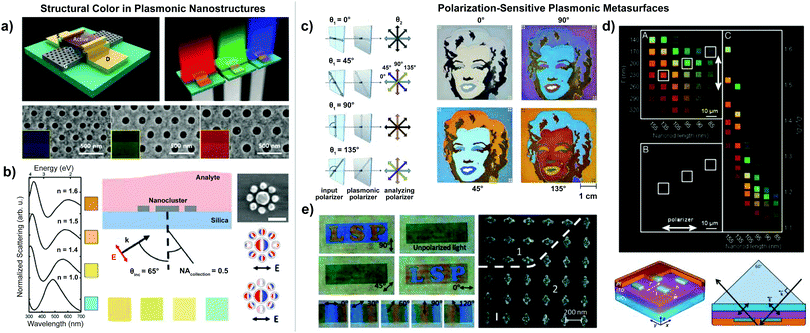 |
| Fig. 3 (a) Top-to-bottom: Schematic of color thin-film transistor by Heo et al. with color response by transmitted incident light shown to the left. SEM images of nanohole structure with scale bar 500 nm.170 (b) Left-to-right: Theoretical spectra by King et al. of a nanocluster in air (n = 1.0), PDMS (n = 1.4), PMMA (n = 1.5), and diphenyl ether (n = 1.6). Schematic of refractive index sensing setup, with labeled angle of incidence and polarization of excitation light. Aluminum nanocluster with charge plot (negative, red; positive, blue) at the subradiant mode of the Fano resonance.172 (c) Left-to-right: Schematic of the angle variation of the incident polarizer (θ1) and the analyzing polarizer (θ2) in the experimental setup by Duempelmann et al. Color response for varying θ2 angles, resulting in four different images of Marilyn Monroe.175 (d) Top-to-bottom: Image of Al pixels with polarized image of pixel array (part A), image of the array with polarizer parallel to x-axis (part B), unpolarized image of pixels sorted according to Dy/Dx ratio and length (l) taken with scattering DSLR camera (part C). Bottom: Schematic showing the Al pixel unit cell by Olson et al. with labeled physical parameters (length l, width w, height h, and edge-to-edge spacing Γ). Diagram of excitation geometry for the pixel, where p-polarized white light propagates across the yz plane.68 (e) Left-to-right: Transmission images of localized surface plasmon (LSP) pattern sample (above) and twisted chromatic plasmonic polarizers sample (below) by Ellenbogen et al. for varying angles of polarized light. SEM image of LSP sample showing that the background nanoantennas (region “2”) are perpendicular to the letter (“LSP”) nanoantennas (region “1”).152 (a–c and e) Reprinted (adapted) with permission from ref. 152, 170, 172 and 175. Copyright 2020, 2015, 2016, 2012 American Chemical Society. (d) Copyright 2014 National Academy of Sciences ref. 68. | |
Polarization-sensitive plasmonic metasurfaces
Many metasurfaces are composed of symmetric nanoresonator unit-cell elements arranged in regular, periodic patterns to achieve vibrant color output. However, breaking symmetry in metasurface lattice or nanoresonator geometry can enable a polarization-sensitive color response.159 In plasmonic nanostructures, polarization of the incident light directly impacts the excitation of localized surface plasmon resonances (LSPRs).174 Several researchers have leveraged polarization-dependent plasmonic nanostructures for colorimetric applications. For example, Goh et al. fabricated tunable color pixels with linear polarization dependence using nanoscale ellipses and squares. Different polarization directions revealed two sets of color patterns in the same nanostructure, one square-shaped and the other star-shaped.71 Similarly, Duempelmann et al. achieved directional structural colors with tunable, symmetry-breaking aluminum plasmonic nanowires. Due to the asymmetry, plasmon resonances were only excited by a certain direction of incident light. The structure appeared colorful in one angle of observation, but colorless in the opposite direction.174
High aspect ratio geometries have also been implemented to achieve polarization-dependent color response. Duempelmann et al. developed a plasmonic phase retarder using silver nanowires. The nanowire geometry was optimized to decrease angle and orientation dependence and tune the LSPR effect into the visible spectrum. Rotating a polarizer displayed four distinct colors (Fig. 3(c)).175 Olson et al. constructed vibrant plasmonic color pixels from Al nanorod arrays, which produced a spectrally narrow, polarization-tunable optical response (Fig. 3(d)).68
Cross-shaped geometries have activated polarization-based phenomena in plasmonic metasurfaces. The work of Li et al. presented periodically arranged cross-shaped plasmonic nanoapertures fabricated in an aluminum film, which exhibited a polarization-switchable color response in transmission.176 Ellenbogen et al. developed plasmonic optical nanoantennas with high sensitivity to polarized light. This system, termed chromatic plasmonic polarizers (CPP), exhibited a visible color response to birefringent chicken breast tissue and plastic foil due to their cross-shaped structure (Fig. 3(e)). Based on selective excitation of either the vertical or horizontal LSPR, incident light polarized along the short cross axis displayed a blue color, while light polarized along the long axis showed yellow.152 This finding offers key insights into biomedical applications, where nanophotonics solutions can contribute to tissue imaging without invasive staining procedures.
Material challenges in plasmonic metasurfaces
Plasmonic metasurfaces effectively produce structural colors. However, the resonance mechanism of each unit-cell nanostructure, material limitations, and production methods pose notable challenges. For instance, refractive-index-based LSPR sensing relies on specific environmental conditions and requires surface contact, which is not always possible in clinical settings.172 Plasmonic diffraction gratings produce vivid colors, yet they provide limited spatial resolution.153 However, certain variations in the nanostructures have improved plasmonic resolution. For example, disk-hole coupling has achieved ∼100
000 dpi.148 Nonetheless, fabrication of such nanostructures is challenging to replicate for large scale manufacturing.
Moreover, intrinsic material loss in plasmonic metasurfaces can significantly limit color saturation.177–179 The cost of fabrication and environmental degradation present further hurdles to high-throughput, durable applications. Typical plasmonic metals include gold, copper, silver, and aluminum. Gold and silver are too costly to be considered practical, while copper, silver, and aluminum exhibit rapid oxidation that renders them non-enduring candidates.180–183 Gold is more robust, yet does not produce blue light due to its strong transition band. Aluminum, which forms a thin, finite oxide layer upon environmental contact, is a low-cost, relatively stable plasmonic material. However, it exhibits notable loss due to its interband transition.184 Though imperfect, aluminum has been frequently utilized by researchers as a lightweight material with favorable optical properties and could be an option for wide scale production in the future.148,158
Structural color in dielectric nanostructures
All-dielectric nanostructures have been demonstrated as efficient generators of saturated structural color in comparison to their plasmonic counterparts. This is attributed to their low dissipative losses and high refractive index contrast with respect to the surrounding environment.41,185,186 When spatially confined in three dimensions to form subwavelength nanoparticles, these all-dielectric systems exhibit spectrally distinct electric and magnetic Mie resonances that are tuned by altering resonator geometry and material crystallinity.187,188
Examples of high-refractive-index dielectric materials exhibiting strong scattering behavior include silicon (Si), gallium arsenide (GaAs), and gallium phosphide (GaP).189 Further systems which exhibit low losses at visible frequencies encompass silicon nitride (Si3N4) or titanium dioxide (TiO2). A multitude of periodic arrangements can be leveraged to construct all-dielectric metasurfaces. The configuration of Mie-resonant dielectric nanoparticles in two-dimensional lattices of different geometries can alter the metasurface optical response.35 Therein, for example, changes in periodic nanostructure spacings can alter color response due to partial reflection of the incident light and changes in the index of refraction. Further, two-dimensionally-confined dielectric nanostructures that form sub-wavelength gratings can be patterned periodically to generate high-quality-factor resonances190–192 with potential to generate high-purity structural color.193–195
A variety of silicon metasurfaces have generated vibrant colors over a range of frequencies, for which we highlight examples here. To leverage the potential of low-order electric and magnetic Mie resonances, Proust et al. developed an all-dielectric silicon nanopillar array with variation in the nanostructure diameter and aspect ratio to achieve a colorimetric optical response by reproducing a Mondrian painting on the micrometer scale (Fig. 4(a)).187 Højlund-Nielsen et al. tested the color output of square silicon nanostructures in a square lattice with varying periodicity. The resulting silicon diffraction gratings produced strong, uniform structural colors over a large angular interval196 Cross-shaped patterns also elicit compelling color effects from all-dielectric metasurfaces. Vashistha et al. utilized arrays of equilateral cross-shaped Si nanoresonators to obtain narrow resonances, high hue, and high saturation in the visible spectrum. The nanoresonators were polarization-insensitive, yet allowed ample color control with the dimensional parameters in the cross structure. A range of colors was produced by adjusting the length, width, and periodicity of the crosses.188
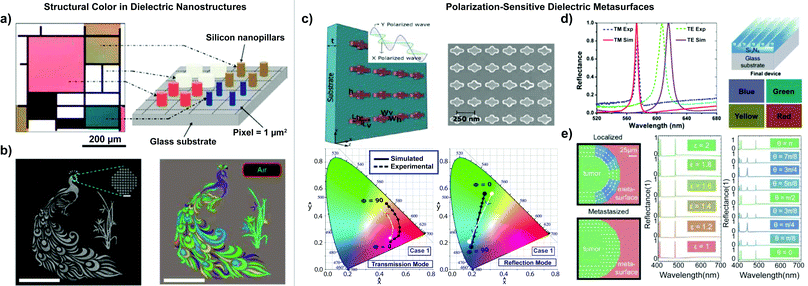 |
| Fig. 4 (a) Mondrian micropainting by Proust et al. made with silicon nanoparticles on glass and labeled schematic of all-dielectric metasurface structure.187 (b) Left-to-right: Top-view SEM image by Yang et al. of a Si metasurface patterned to show a peacock with an orchid (scale bar of 100 μm). Bright-field microscope image of the peacock with orchid in air.72 (c) Top-to-bottom: Dielectric metasurface by Vashistha et al. containing nonsymmetric cross-shaped Si nanoantennas with inset schematic of wave propagation for x-polarized (Φ = 0°) and y-polarized (Φ = 90°) normally incident light. To the right, an SEM image showing the top view of the dielectric metasurface with scale bar 250 nm. Below, a graph of polarization-based color changes from magenta to yellow for transmission mode and from green to blue for reflection mode (simulation results for polarization angle of incident white light (Φ) from 0° to 90° with ΔΦ = 10° are shown, and blue numbers correspond to ideal color wavelengths).184 (d) Left-to-right: Spectral response of the red-yellow polarization-controlled tunable color filters by Uddin et al., with a yellow filter for TM polarization and green filter for TE polarization. Schematic of final GMR color filter structure, with perceived colors calculated using the experimental reflectance values of the pixels.194 (e) Left-to-right: Schematic illustration by Poulikakos et al. of the metasurface colorimetric response for a localized tumor and a metastasized tumor. Reflectance spectra showing colorimetric sensing of the metasurface for isotropic media with varying relative permittivity ε = 1 : 0.2 : 2, with insets and line colors corresponding to reflected structural color. Colorimetric sensing capabilities for an anisotropic sample matching linearly birefringent properties of fibrous biological tissue for ε = 2 and varying orientation angle θ of the tissue optical axis.195 (a) Reprinted (adapted) with permission from ref. 187. Copyright 2016 American Chemical Society. (b and c) Creative Commons Attribution 4.0 International License. (d) [Reprinted/adapted] with permission from ref. 194. Copyright The Optical Society. (e) Reprinted (adapted) with permission from ref. 195. Copyright 2016 American Chemical Society. | |
In addition to geometry, the surrounding environment of the metasurface can play a crucial role in the customization of the color response. Yang et al. fabricated silicon nanostructures and showed that increasing the refractive index of the surrounding medium will improve vibrancy of the metasurface structural color response (Fig. 4(b)).72 The increase in the refractive index causes the electric dipole resonance to undergo a redshift, whereas the magnetic dipole resonance is insensitive to environmental changes. The narrower-bandwidth spectral response in transmission and reflection, resulting from subsequent destructive and constructive interference of the electric and magnetic resonances, generated structural color of improved brightness and purity.72,197
Polarization-sensitive dielectric metasurfaces
The optical properties of all-dielectric metasurfaces provide a stable platform for polarization-sensitive nanophotonics. Researchers have leveraged symmetry breaking to achieve polarization-based color response. For example, Vashistha et al. developed an all-dielectric color filter using non-equilateral cross-shaped Si nanoantennas. The color filter was sensitive to polarization angle changes in transmission and reflection modes due to the asymmetric design (Fig. 4(c)). Furthermore, the team achieved an array of high-quality colors by varying the size and aspect ratio of the crosses.184 Instead of cross shapes, Wang et al. tuned the dimensions of rectangular nanoblocks to produce vibrant, polarization-sensitive colors in transmission. His team fabricated asymmetric monocrystalline silicon nanoblocks that were arranged in arrays on a flexible substrate. Different polarization angles shifted the transmission wavelength, giving access to a wide range of colors. The color filter demonstrated subwavelength resolution in the visible wavelength region.198 In another study, Shibanuma et al. developed dielectric metasurfaces that shifted optical response from high transmission to high reflection due to changes in incident light polarization. The metasurfaces contained arrays of high-refractive-index dielectric dimers. The excited electric and magnetic dipole modes of the dimers were coupled to form hybridized modes. Incident polarization manipulated the hybridized modes, thus varying the optical response.189 Asymmetric, elliptical nanopillars fabricated from TiO2 were developed by Yang et al. to circumvent Ohmic loss in all-dielectric colorimetric metasurfaces.199 The observed narrow-bandwidth reflectance spectra resulted in polarization-sensitive structural colors of high saturation, efficiency (>90%) and resolution.
Guided-mode-resonant dielectric metasurfaces provide an additional versatile avenue to generate polarization-sensitive structural color. The Guided Mode Resonance (GMR) effect occurs in thin-film structures with diffractive elements and an optical waveguide layer. Therein, leaky modes, arising from periodic modulation in the waveguide, can generate a narrow-bandwidth resonant response.156,157 In GMR metasurfaces, the resonance wavelength can be tuned using refractive index, pattern periodicity, waveguide thickness, and incident angle. Notably, dielectric GMR reflective filters have achieved structural colors with high efficiency, purity, and a narrow bandwidth. For example, Uddin et al. designed a GMR-based color filter array using subwavelength silicon nitride gratings on a glass substrate with an efficiency of ∼95% and a narrow bandwidth of ∼12 nm. The filter separated the incident transverse electric (TE)-polarized white light into its three primary colors: red, green, and blue.193
Manipulating metasurfaces to create an anisotropic geometry allows for selective response to polarized light. Uddin et al. fabricated polarization-controlled tunable color filters using subwavelength gratings etched into a thin Si3N4 film on a glass substrate. Depending on grating dimensions, the authors demonstrated two color filters which exhibited red-yellow and green-blue reflected structural color upon transverse electric (TE) or transverse magnetic (TM) polarized light excitation, respectively (Fig. 4(d)).194 In a further study which explored the scope of potential applications, Magnusson et al. utilized similar guided-mode-resonant grating structures for refractive-index-based biochemical sensing with high sensitivity and selectivity.200 Guided-mode-resonant dielectric metasurfaces have also been leveraged to quantitatively map the presence and orientation of birefringent media onto structural color. Poulikakos et al. developed a guided-mode-resonant dielectric metasurface from dispersion-engineered Si3N4 and SiO2 layers on a SiO2 substrate. The reflective and rotational symmetry breaking achieved by the rhombohedral metasurface unit-cell-element enabled each incident polarization state to be attributed to a distinct colorimetric response (Fig. 4(e)).195 The results of this numerical study hold potential for future applications in polarization-sensitive biomedical applications.
Material challenges in dielectric metasurfaces
High-refractive-index dielectric materials have enabled a new class of metasurfaces with versatile advantages. However, common material platforms such as silicon (Si), silicon nitride (Si3N4) or titanium dioxide (TiO2) also present drawbacks that should be considered for nanophotonics applications. For example, silicon exhibits intrinsic, non-negligible material loss at visible frequencies, which can limit color saturation and range.201,202 Several engineering methods have been put forward to address this challenge, such as thermal annealing, geometric tuning, or addition of an antireflective layer.203–205 Si3N4 and TiO2 have been explored as alternatives with low material loss in the visible spectrum. Contrary to Si3N4, both TiO2 and monocrystalline silicon (c-Si), are not readily compatible with Complementary Metal Oxide Semiconductor (CMOS) fabrication and would therefore face challenges in mass production.148,202,206–209
In general, high refractive index dielectric nanostructures generate Mie resonances with high-quality reflection spectra, leading to vibrant colors.154,210 However, current research demonstrates that producing additive colors from dielectric metasurfaces in transmission mode remains challenging. As a potential CMOS-compatible alternative, amorphous silicon (a-Si) can be used, yet high material loss would hinder color saturation.206 In the future, hybrid platforms that integrate plasmonic and dielectric nanostructures could provide another solution for additive color generation.148
Polarization-tunable structural color in hybrid and dynamic metasurfaces
In addition to metasurfaces composed purely of metallic or dielectric nanostructures, hybrid metasurfaces, which combine the strengths of various material platforms, have been demonstrated as effective generators of structural color, offering new avenues toward dynamic color tunability.69,162,211–215 We now focus on examples of hybrid metasurfaces that have produced polarization-tunable structural color.
Metasurface gratings, an inherently polarization-tunable optical system, are a versatile platform to demonstrate the combined advantages of metallic and dielectric metasurfaces. Kim et al. developed one-dimensional poly(methyl methacrylate) (PMMA) gratings on a lossy metallic substrate with the ability to generate structural color of high vibrancy and contrast.211 Their hybrid nanograting leveraged phase shifts between spectrally separate resonances arising from orthogonal TE and TM grating modes to achieve a sharp spectral response when observed between crossed polarizers. For silver (Ag), tungsten (W) and platinum (Pt) substrates, this study was able to achieve coverage of the sRGB color gamut at 55%, 69% and 90%, respectively. Metallic nanogratings on dielectric films have been presented by Koirala et al., where an ultrathin aluminum (Al) grating was fabricated on a subwavelength silicon nitride waveguide. The resulting broad color palette, observed in transmission mode upon tuning incident light polarization and grating geometry, was attributed to reinforcement of electric and magnetic field profiles in the silicon nitride waveguide layer.212
Metal–insulator–metal grating metasurfaces, which act as plasmonic nanoresonators, were demonstrated by Xu et al. as highly efficient, high-resolution color filters across the visible spectrum.213 In the work by Jung et al. an etalon structure composed of a subwavelength nanoscale silicon dioxide (SiO2) and Ag layers was fabricated, where the upper Ag layer was structured as a nanograting to enable a polarization-tunable color filter.162 Therein, changing the refractive index of the dielectric environment and the thickness of the SiO2 spacer layer varied the generated color. As an additional benefit, an increased transmission and higher resolution color images were observed with this system. Further studies163,164 showed numerically that stacked multilayered thin films of metal and dielectric grating structures were able to create an optical coupling of the resonance fields at the metal–dielectric interface. This effect suppresses the noise caused by higher order diffraction, which would then narrow the Full Width at Half Maximum (FWHM), leading to an improved color purity and transmission. Additional noteworthy material systems include chalcogenides, such as germanium antimony telluride (GST), where transitions between amorphous and crystalline phases yield dielectric to plasmonic material properties, respectively. To this end, Gholipour et al. put forward a GST nanograting where additional tailoring of structural color was achieved by phase change.216
Liquid crystals exhibit optical properties which are tunable through variations in electric and magnetic fields, chemical composition, or temperature. These parameters offer an additional versatile platform to enhance the polarization tunability of metasurfaces. Olson et al. utilized periodic arrays of Al nanostructures as switchable pixels in liquid crystal displays with high chromaticity.69 Plasmonic nanohole arrays, assembled in a rectangular lattice, were interfaced with a twisted nematic liquid crystal by Lee et al. to obtain an electrically- and polarization-tunable metasurface color filter.214 Sharma et al. included a liquid crystal layer on top of an Al metasurface between two indium tin oxide (ITO) coated glass substrates which behaved as an electrode in their device.215 By changing the voltage that is applied across the electrode, the device was able to both gradually and abruptly change its color. The observed color-tunability occurred as the liquid crystal varied its orientation along the electric field, thus altering the incident light polarization.
Colorimetric metasurfaces in tissue imaging: challenges and opportunities
The ability of polarization-sensitive, colorimetric metasurfaces to quantitatively map the polarization state of light onto structural color holds great promise for biomedical imaging and disease visualization, as shown schematically in Fig. 5. As was highlighted for specific examples in this work (Fig. 1), the presence and orientation of fibrous tissue is an indicator of a range of serious diseases affecting major organs of the human body (Fig. 5(a)). In contrast to biochemical markers, which can provide disease-specific information at the cellular, molecular or genetic level,12 the ability of polarized light to probe tissue microstructure is translatable across diseases and organs, thus rendering a powerful versatility to all-optical analysis. Moreover, recent developments in biocompatible and biodegradable inorganic nanostructures for biomedical applications, such as black phosphorous nanosheets, present potential prospects for the manipulation of tissues in diagnostic and theranostic applications.217–220 The anisotropic nature of these material platforms offers opportunities for analysis via polarimetry and may provide a direct impact in the design and development of nano-optical metasurfaces in the future. Additionally, metasurfaces enable label-free visualization, thus circumventing several drawbacks encountered with fluorescent probes such as photoblinking, photobleaching and result convolution.221
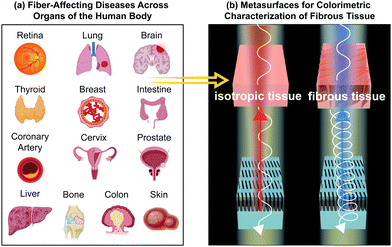 |
| Fig. 5 Schematic illustration of the opportunities for colorimetric metasurfaces to visualize the origin and progression of disease. Fibrous tissue from a range of fiber-affecting diseases exhibits a selective interaction with polarized light (part a). Colorimetric metasurfaces can be designed to quantitatively map the polarizing properties of fibrous biological tissue onto structural color (part b). Part a created with BioRender.com. | |
Leveraging colorimetric metasurfaces to visualize tissue microstructure (Fig. 5(b)) presents several advantages over existing high-sensitivity optical techniques, where this work focused on Mueller Matrix polarimetry. The ability of nano-optical structures to enhance the intensity and selectivity of light–matter interactions222,223 holds potential to overcome detection challenges arising from the naturally weak optical anisotropy of biological tissue. Encoding the polarization state of light onto the structural color response of a metasurface allows for a user-friendly readout of the polarizing properties of a medium of interest, thus circumventing extensive data post-processing required in Mueller Matrix polarimetry and alternative methodologies.224 The facile tunability of metasurface optical properties, based on the material, geometry and arrangement of their nanoresonator unit-cell elements, allows for the design of customizable metasurfaces which can be adapted for various applications. Additionally, when metasurfaces are leveraged to probe the polarization state of light, direct contact between the metasurface and the material of interest is not required, as shown schematically in Fig. 5(b). Importantly, this circumvents artefacts arising from tissue roughness, which can realistically range around 1μm for sectioned tissue samples.225 The opportunities of contact-free nanophotonic detection lie in contrast to alternative metasurface sensing techniques. These methods frequently rely on sensitivity to the refractive-index environment, resulting from concentrated electromagnetic near fields, which decay exponentially from the sensing interface. Finally, perhaps the most compelling property of metasurfaces lies in their ability to scale down the complex manipulation of light to a single optical surface. This enables a host of affordable, mobile and readily translatable applications in the clinic and biomedical industry including ex vivo platforms in pathology and bioimaging or in vivo devices which could be utilized intra-operatively. For example, metasurfaces could be integrated into in vivo imaging systems as metalenses on optical fibers.226–229 Pahlevaninezhad et al. attached a metalens to an optical coherence tomography catheter to perform endoscopic imaging in vivo. Utilizing the high resolution of the metalens, the smallest FWHMs of the nano-optic endoscope were 6.37 μm (tangential) and 6.53 μm (saggital).230 Metasurface-based devices can achieve subwavelength resolution at submillimeter thickness. Thus, metasurfaces provide a uniquely precise, portable bioimaging solution compared to traditional spectrometers.228,229,231
Along the path to clinically- and industrially-translatable colorimetric metasurfaces, challenges must be overcome. The complex optical properties of fibrous biological tissue play a central role when leveraging metasurfaces to visualize tissue microstructure. The superposition of depolarization, diattenuation and retardance (eqn (4)) which constitute the polarizing properties of a tissue of interest, must be accounted for when designing a metasurface probe or assessing its optical response. For example, depolarization, which varies based on the refractive index and size of scatterers present in a medium,79 significantly increases at tissue thicknesses greater than 0.5 mm.232 While tissue biopsies are typically below thicknesses where depolarization plays a significant role,12 these effects may become more significant in backscattering applications on bulk tissue.28 Fortunately, the tunability of metasurface design presents opportunities to optically separate competing physical effects in a sensing or imaging application.195
Nanofabrication methodologies which are cost-effective at an industrial scale, preferably compatible with high throughput roll-to-roll processes,233 are required to fabricate translatable metasurface devices. While electron-beam lithography has proven to be an accurate and reliable technique to demonstrate proof-of-concept metasurfaces at the research scale (ca. 100 × 100 μm2), metasurfaces at a larger scale, e.g. expanding over the surface of a 1 × 3 inch microscope coverslip, would require long electron-beam exposure times which are not economically viable or serial stitching processes.174 Alternative, scalable technologies include nanoimprint lithography,234 where high-throughput and low-cost pattern transfer of high-resolution nanostructures is achieved by compression molding from a reusable template. Laser interference lithography (IL)235 presents a further opportunity for large-scale patterning with laser sources, where nanoscale features can be achieved by illumination at ultraviolet wavelengths with extreme ultraviolet interference lithography (EUV-IL),236 or immersion interference lithography.235,237 These cost-effective, scalable fabrication techniques could increase the accessibility of metasurface-based technology, enable widespread implementation of metasurfaces into bioimaging devices, and reduce the need for expensive optical equipment in biomedical and clinical environments. The optical complexity of biological tissue and challenges of mass production present obstacles to immediate clinical use. However, with demonstrated tunability and emerging scalable processes, metasurfaces have the potential to overcome existing limitations and transform the future of medical diagnostics.
Outlook
Precisely and rationally designed material anisotropy and colorimetry in nano-optical metasurfaces present exciting opportunities for quantitative imaging of biological tissue. While the enhanced electromagnetic near fields in metasurfaces have enabled a host of successful refractive-index-based or surface-enhanced sensing applications, the avenue of enhanced detection of material anisotropy with metasurfaces remains largely unexplored. In combination with their ability to generate polarization-sensitive structural color, such metasurfaces hold promise to enable a new class of quantitative, miniaturized, cost-effective and user-friendly bioimaging platforms with facile implementation in the clinic and biomedical industry.
Author contributions
Following CRediT definitions: conceptualization: Z. H., T. P., J. J. M., L. V. P.; funding acquisition: L. V. P.; investigation: Z. H., T. P., J. J. M., L. V. P.; project administration: L. V. P.; supervision: L. V. P.; validation: Z. H., T. P., J. J. M., L. V. P.; visualization: Z. H., T. P., L. V. P.; writing – original draft: Z. H., T. P., J. J. M., L. V. P.; writing – review & editing: Z. H., T. P., J. J. M., L. V. P.
Conflicts of interest
There are no conflicts of interest to declare.
Acknowledgements
The authors thank Aniket S. Puri for insightful discussions, as well as Elissa A. Klopfer and I-Hsun Wu for artistic rendering. Lisa V. Poulikakos gratefully acknowledges funding from the UC San Diego Jacobs School of Engineering, 13991-1023256.
References
- S. L. Friedman, Gastroenterology, 2008, 134, 1655–1669 CrossRef CAS PubMed.
- D. S. James, A. N. Jambor, H.-Y. Chang, Z. Alden, K. B. Tilbury, N. K. Sandbo and P. J. Campagnola, J. Biomed. Opt., 2019, 25, 1 Search PubMed.
- P. Whittaker, R. A. Kloner, D. R. Boughner and J. G. Pickering, Basic Res. Cardiol., 1994, 89, 397–410 CrossRef CAS PubMed.
- N. Ghosh, N. Ghosh, M. A. Wallenburg, S.-H. Li, R. D. Weisel, B. C. Wilson, R.-K. Li and I. A. Vitkin, J. Biomed. Opt., 2010, 15, 047009 CrossRef PubMed.
- K. Hsiao, P. Chapman, S. Nilsen, C. Eckman, Y. Harigaya, S. Younkin, F. Yang and G. Cole, Science, 1996, 274, 99–102 CrossRef CAS PubMed.
- L. W. Jin, K. A. Claborn, M. Kurimoto, M. A. Geday, I. Maezawa, F. Sohraby, M. Estrada, W. Kaminksy and B. Kahr, Proc. Natl. Acad. Sci. U. S. A., 2003, 100, 15294–15298 CrossRef CAS PubMed.
- S. L. Jacques, J. C. Ramella-Roman and K. Lee, J. Biomed. Opt., 2002, 7, 329–340 CrossRef PubMed.
- P. P. Provenzano, K. W. Eliceiri, J. M. Campbell, D. R. Inman, J. G. White and P. J. Keely, BMC Med., 2006, 4, 38 CrossRef PubMed.
- M. W. Conklin, J. C. Eickhoff, K. M. Riching, C. A. Pehlke, K. W. Eliceiri, P. P. Provenzano, A. Friedl and P. J. Keely, Am. J. Pathol., 2011, 178, 1221–1232 CrossRef PubMed.
- Y. K. Tao, D. Shen, Y. Sheikine, O. O. Ahsen, H. H. Wang, D. B. Schmolze, N. B. Johnson, J. S. Brooker, A. E. Cable, J. L. Connolly and J. G. Fujimoto, Proc. Natl. Acad. Sci., 2014, 111, 15304–15309 CrossRef CAS PubMed.
- O. Nadiarnykh, R. B. LaComb, M. A. Brewer and P. J. Campagnola, BMC Cancer, 2010, 10, 94 CrossRef PubMed.
-
H. Mohan, Textbook of Pathology, Jaypee Brothers Medical Publishers, New Dehli, NCT Dehli, 6th edn, 2010 Search PubMed.
- M. W. Pickup, J. K. Mouw and V. M. Weaver, EMBO Rep., 2014, 15, 1243–1253 CrossRef CAS PubMed.
- P. Sivakumar, C. Kitson and G. Jarai, Connect. Tissue Res., 2019, 60, 62–70 CrossRef CAS PubMed.
- S.-Y. Lu and R. A. Chipman, J. Opt. Soc. Am. A, 1996, 13, 1106 CrossRef.
- N. Ghosh, M. F. G. Wood, S. Li, R. D. Weisel, B. C. Wilson, R.-K. Li and I. A. Vitkin, J. Biophotonics, 2009, 2, 145–156 CrossRef CAS PubMed.
- R. Oldenbourg and G. Mei, J. Microsc., 1995, 180, 140–147 CrossRef CAS PubMed.
- K. R. Levental, H. Yu, L. Kass, J. N. Lakins, M. Egeblad, J. T. Erler, S. F. T. Fong, K. Csiszar, A. Giaccia, W. Weninger, M. Yamauchi, D. L. Gasser and V. M. Weaver, Cell, 2009, 139, 891–906 CrossRef CAS PubMed.
- J. Kim, J. Feng, C. A. R. Jones, X. Mao, L. M. Sander, H. Levine and B. Sun, Nat. Commun., 2017, 8, 842 CrossRef PubMed.
- P. V. Taufalele, J. A. VanderBurgh, A. Muñoz, M. R. Zanotelli and C. A. Reinhart-King, PLoS One, 2019, 14, e0216537 CrossRef CAS PubMed.
- P. J. Campagnola and C.-Y. Dong, Laser Photonics Rev., 2011, 5, 13–26 CrossRef CAS.
- X. Chen, O. Nadiarynkh, S. Plotnikov and P. J. Campagnola, Nat. Protoc., 2012, 7, 654–669 CrossRef CAS PubMed.
- M. R. Hee, E. A. Swanson, J. G. Fujimoto and D. Huang, J. Opt. Soc. Am. B, 1992, 9, 903–908 CrossRef.
- S. D. Giattina, B. K. Courtney, P. R. Herz, M. Harman, S. Shortkroff, D. L. Stamper, B. Liu, J. G. Fujimoto and M. E. Brezinski, Int. J. Cardiol., 2006, 107, 400–409 CrossRef PubMed.
- F. A. South, E. J. Chaney, M. Marjanovic, S. G. Adie and S. A. Boppart, Biomed. Opt. Express, 2014, 5, 3417 CrossRef PubMed.
- J. Schellman and H. P. Jensen, Chem. Rev., 1987, 87, 1359–1399 CrossRef CAS.
-
N. Ghosh, M. Wood and A. Vitkin, Handbook of Photonics for Biomedical Science, 2010, pp. 253–282 Search PubMed.
- J. Qi and D. S. Elson, J. Biophotonics, 2017, 10, 950–982 CrossRef PubMed.
- M. Villiger, D. Lorenser, R. A. McLaughlin, B. C. Quirk, R. W. Kirk, B. E. Bouma and D. D. Sampson, Sci. Rep., 2016, 6, 28771 CrossRef CAS PubMed.
- J. Qi, M. Ye, M. Singh, N. T. Clancy and D. S. Elson, Biomed. Opt. Express, 2013, 4, 2433 CrossRef PubMed.
- N. T. Clancy, S. Arya, J. Qi, D. Stoyanov, G. B. Hanna and D. S. Elson, Biomed. Opt. Express, 2014, 5, 4108 CrossRef PubMed.
- J. Qi and D. S. Elson, Sci. Rep., 2016, 6, 25953 CrossRef CAS PubMed.
- S. Harris, Nat. Photonics, 2010, 4, 748–749 CrossRef CAS.
- S. Jahani and Z. Jacob, Nat. Nanotechnol., 2016, 11, 23–36 CrossRef CAS PubMed.
- P. Genevet, F. Capasso, F. Aieta, M. Khorasaninejad and R. Devlin, Optica, 2017, 4, 139–152 CrossRef CAS.
- B. Liedberg, C. Nylander and I. Lunström, Sens. Actuators, 1983, 4, 299–304 CrossRef CAS.
- J. N. Anker, W. P. Hall, O. Lyandres, N. C. Shah, J. Zhao and R. P. Van Duyne, Nat. Mater., 2008, 7, 442–453 CrossRef CAS PubMed.
- F. Yesilkoy, E. R. Arvelo, Y. Jahani, M. Liu, A. Tittl, V. Cevher, Y. Kivshar and H. Altug, Nat. Photonics, 2019, 13, 390–396 CrossRef CAS.
- F. Neubrech, C. Huck, K. Weber, A. Pucci and H. Giessen, Chem. Rev., 2017, 117, 5110–5145 CrossRef CAS PubMed.
- A. Tittl, A. Leitis, M. Liu, F. Yesilkoy, D. Y. Choi, D. N. Neshev, Y. S. Kivshar and H. Altug, Science, 2018, 360, 1105–1109 CrossRef CAS PubMed.
- M. L. Tseng, Y. Jahani, A. Leitis and H. Altug, ACS Photonics, 2021, 8, 47–60 CrossRef CAS.
- J. K. Gansel, M. Thiel, M. S. Rill, M. Decker, K. Bade, V. Saile, G. von Freymann, S. Linden and M. Wegener, Science, 2009, 325, 1513–1515 CrossRef CAS PubMed.
- M. Hentschel, M. Schäferling, T. Weiss, N. Liu and H. Giessen, Nano Lett., 2012, 12, 2542–2547 CrossRef CAS PubMed.
- E. S. A. Goerlitzer, R. Mohammadi, S. Nechayev, K. Volk, M. Rey, P. Banzer, M. Karg and N. Vogel, Adv. Mater., 2020, 32, 2001330 CrossRef CAS PubMed.
- M. Hentschel, M. Schäferling, X. Duan, H. Giessen and N. Liu, Sci. Adv., 2017, 3, e1602735 CrossRef PubMed.
- B. Frank, X. Yin, M. Schäferling, J. Zhao, S. M. Hein, P. V. Braun and H. Giessen, ACS Nano, 2013, 7, 6321–6329 CrossRef CAS PubMed.
- A. G. Mark, J. G. Gibbs, T. C. Lee and P. Fischer, Nat. Mater., 2013, 12, 802–807 CrossRef CAS PubMed.
- K. M. McPeak, C. D. van Engers, S. Bianchi, A. Rossinelli, L. V. Poulikakos, L. Bernard, S. Herrmann, D. K. Kim, S. Burger, M. Blome, S. V. Jayanti and D. J. Norris, Adv. Mater., 2015, 27, 6244–6250 CrossRef CAS PubMed.
- C. Zhou, X. Duan and N. Liu, Acc. Chem. Res., 2017, 50, 2906–2914 CrossRef CAS PubMed.
- L. V. Poulikakos, P. Thureja, A. Stollmann, E. De Leo and D. J. Norris, Nano Lett., 2018, 18, 4633–4640 CrossRef CAS PubMed.
- H.-E. Lee, H.-Y. Ahn, J. Mun, Y. Y. Lee, M. Kim, N. H. Cho, K. Chang, W. S. Kim, J. Rho and K. T. Nam, Nature, 2018, 556, 360–365 CrossRef CAS PubMed.
- J. Karst, N. H. Cho, H. Kim, H. E. Lee, K. T. Nam, H. Giessen and M. Hentschel, ACS Nano, 2019, 13, 8659–8668 CrossRef CAS PubMed.
- Q. Zhang, T. Hernandez, K. W. Smith, S. A. H. Jebeli, A. X. Dai, L. Warning, R. Baiyasi, L. A. McCarthy, H. Guo, D.-H. Chen, J. A. Dionne, C. F. Landes and S. Link, Science, 2019, 365, 1475–1478 CrossRef CAS PubMed.
- A. García-Etxarri and J. A. Dionne, Phys. Rev. B: Condens. Matter Mater. Phys., 2013, 87, 235409 CrossRef.
- C. S. Ho, A. Garcia-Etxarri, Y. Zhao and J. Dionne, ACS Photonics, 2017, 4, 197–203 CrossRef CAS.
- M. L. Solomon, J. A. Dionne, J. M. Abendroth, L. V. Poulikakos and J. Hu, J. Am. Chem. Soc., 2020, 142, 18304–18309 CrossRef CAS PubMed.
- E. Mohammadi, K. L. Tsakmakidis, A. N. Askarpour, P. Dehkhoda, A. Tavakoli and H. Altug, ACS Photonics, 2018, 5, 2669–2675 CrossRef CAS.
- M. L. Solomon, A. A. E. Saleh, L. V. Poulikakos, J. M. Abendroth, L. F. Tadesse and J. A. Dionne, Acc. Chem. Res., 2020, 53, 588–598 CrossRef CAS PubMed.
- A. Arbabi, Y. Horie, M. Bagheri and A. Faraon, Nat. Nanotechnol., 2015, 10, 937–943 CrossRef CAS PubMed.
- J. Zhou, H. Qian, C.-F. Chen, J. Zhao, G. Li, Q. Wu, H. Luo, S. Wen and Z. Liu, Proc. Natl. Acad. Sci. U. S. A., 2019, 116, 11137–11140 CrossRef CAS PubMed.
- N. A. Rubin, A. Zaidi, M. Juhl, R. P. Li, J. P. B. Mueller, R. C. Devlin, K. Leósson and F. Capasso, Opt. Express, 2018, 26, 21455 CrossRef CAS PubMed.
- N. A. Rubin, G. D’Aversa, P. Chevalier, Z. Shi, W. T. Chen and F. Capasso, Science, 2019, 364, eaax1839 CrossRef PubMed.
- Y. Hu, X. Wang, X. Luo, X. Ou, L. Li, Y. Chen, P. Yang, S. Wang and H. Duan, Nanophotonics, 2020, 9, 3755–3780 CrossRef.
- S. M. Kamali, E. Arbabi, A. Arbabi and A. Faraon, Nanophotonics, 2018, 7, 1041–1068 CAS.
- F. Yesilkoy, R. A. Terborg, J. Pello, A. A. Belushkin, Y. Jahani, V. Pruneri and H. Altug, Light: Sci. Appl., 2018, 7, 17152 CrossRef PubMed.
- H. Kwon, E. Arbabi, S. M. Kamali, M. Faraji-Dana and A. Faraon, Nat. Photonics, 2020, 14, 109–114 CrossRef CAS.
- J. Zhou, H. Qian, J. Zhao, M. Tang, Q. Wu, M. Lei, H. Luo, S. Wen, S. Chen and Z. Liu, Natl. Sci. Rev., 2020, nwaa176 Search PubMed.
- J. Olson, A. Manjavacas, L. Liu, W. S. Chang, B. Foerster, N. S. King, M. W. Knight, P. Nordlander, N. J. Halas and S. Link, Proc. Natl. Acad. Sci. U. S. A., 2014, 111, 14348–14353 CrossRef CAS PubMed.
- J. Olson, A. Manjavacas, T. Basu, D. Huang, A. E. Schlather, B. Zheng, N. J. Halas, P. Nordlander and S. Link, ACS Nano, 2016, 10, 1108–1117 CrossRef CAS PubMed.
- K. Kumar, H. Duan, R. S. Hegde, S. C. W. Koh, J. N. Wei and J. K. W. Yang, Nat. Nanotechnol., 2012, 7, 557–561 CrossRef CAS PubMed.
- X. M. Goh, Y. Zheng, S. J. Tan, L. Zhang, K. Kumar, C.-W. Qiu and J. K. W. Yang, Nat. Commun., 2014, 5, 5361 CrossRef CAS PubMed.
- W. Yang, S. Xiao, Q. Song, Y. Liu, Y. Wu, S. Wang, J. Yu, J. Han and D.-P. Tsai, Nat. Commun., 2020, 11, 1864 CrossRef CAS PubMed.
-
E. Collett, Field Guide to Polarization, SPIE, 2005 Search PubMed.
-
C. F. Bohren and D. R. Huffman, Absorption and scattering of light by small particles, 1983, vol. 35 Search PubMed.
- E. Du, H. He, N. Zeng, M. Sun, Y. Guo, J. Wu, S. Liu and H. Ma, J. Biomed. Opt., 2014, 19, 076013 CrossRef CAS PubMed.
- N. Ghosh, M. F. G. Wood and I. A. Vitkin, J. Biomed. Opt., 2008, 13, 044036 CrossRef PubMed.
- S. Shrestha, A. Deshpande, T. Farrahi, T. Cambria, T. Quang, J. Majeski, Y. Na, M. Zervakis, G. Livanos and G. C. Giakos, Biomed. Signal Process. Control, 2018, 40, 505–518 CrossRef.
- N. Ghosh and A. I. Vitkin, J. Biomed. Opt., 2011, 16, 110801 CrossRef PubMed.
- N. Ghosh, P. K. Gupta, A. Pradhan and S. K. Majumder, Phys. Lett. A, 2006, 354, 236–242 CrossRef CAS.
- V. Sankaran and J. T. Walsh, Photochem. Photobiol., 1998, 68, 846–851 CAS.
- D. H. Goldstein, Appl. Opt., 1992, 31, 6676 CrossRef CAS PubMed.
- H. He, N. Zeng, E. Du, Y. Guo, D. Li, R. Liao and H. Ma, Photonics Lasers Med., 2013, 2, 129–137 Search PubMed.
- S. Badieyan, A. Ameri, M. R. Razzaghi, H. Rafii-Tabar and P. Sasanpour, Photodiagn. Photodyn. Ther., 2019, 26, 90–96 CrossRef PubMed.
- M. Sun, H. He, N. Zeng, E. Du, Y. Guo, S. Liu, J. Wu, Y. He and H. Ma, Biomed. Opt. Express, 2014, 5, 4223 CrossRef PubMed.
- S. Badieyan, A. Ameri, M. R. Razzaghi, H. Rafii-Tabar and P. Sasanpour, Photodiagn. Photodyn. Ther., 2019, 26, 90–96 CrossRef PubMed.
- Y. Wang, H. He, J. Chang, C. He, S. Liu, M. Li, N. Zeng, J. Wu and H. Ma, J. Biomed. Opt., 2016, 21, 071112 CrossRef PubMed.
- T. Liu, M. Lu, B. Chen, Q. Zhong, J. Li, H. He, H. Mao and H. Ma, J. Biophotonics, 2019, 12, e201900151 Search PubMed.
- Y. Wang, H. He, J. Chang, N. Zeng, S. Liu, M. Li and H. Ma, Micron, 2015, 79, 8–15 CrossRef PubMed.
- R. Anderson, Appl. Opt., 1992, 31, 11 CrossRef CAS PubMed.
- G. E. Jellison and F. A. Modine, Appl. Opt., 1997, 36, 8190 CrossRef CAS PubMed.
-
N. Ghosh, M. Wood and A. Vitkin, in Handbook of Photonics for Biomedical Science, ed. V. V. Tuchin, CRC Press/Taylor & Francis, Boca Raton, 2010, pp. 253–282 Search PubMed.
- Y. Guo, N. Zeng, H. He, T. Yun, E. Du, R. Liao, Y. He and H. Ma, Opt. Express, 2013, 21, 18361 CrossRef PubMed.
- H. He, N. Zeng, E. Du, Y. Guo, D. Li, R. Liao and H. Ma, Photonics Lasers Med., 2013, 2, 129–137 Search PubMed.
- Y. Qiu, T. Jin, E. Mason and M. C. W. Campbell, Transl. Vis. Sci. Technol., 2020, 9, 1–15 CAS.
- Y. Zhao, M. Reda, K. Feng, P. Zhang, G. Cheng, Z. Ren, S. G. Kong, S. Su, H. Huang and J. Zang, IEEE Sens. J., 2020, 20, 7208–7215 Search PubMed.
- P. Schucht, H. R. Lee, H. M. Mezouar, E. Hewer, A. Raabe, M. Murek, I. Zubak, J. Goldberg, E. Kövari, A. Pierangelo and T. Novikova, IEEE Trans. Med. Imaging, 2020, 39, 4376–4382 Search PubMed.
- Y. Dong, J. Qi, H. He, C. He, S. Liu, J. Wu, D. S. Elson and H. Ma, Biomed. Opt. Express, 2017, 8, 3643 CrossRef CAS PubMed.
- I. Ahmad, M. Ahmad, K. Khan, S. Ashraf, S. Ahmad and M. Ikram, J. Biomed. Opt., 2015, 20, 056012 CrossRef PubMed.
-
N. Ghosh, M. Wood and A. Vitkin, Handbook of Photonics for Biomedical Science, 2010, pp. 253–282 Search PubMed.
- A. Gillessen, B. Voß, J. Rauterberg and W. Domschke, Scand. J. Gastroenterol., 1993, 28, 688–689 CrossRef CAS PubMed.
- B. Rosner, R. J. Glynn and M. Ting, Lee, Biometrics, 2003, 59, 1089–1098 CrossRef PubMed.
- W. S. Noble, Nat. Biotechnol., 2006, 24, 1565–1567 CrossRef CAS PubMed.
-
B. Zhou, J. Xuan, H. Zhao, G. J. Chepko, M. T. Freedman and K. Y. Zou, 2007 IEEE/NIH Life Science Systems and Applications Workshop, LISA, IEEE Computer Society, 2007, pp. 217–220.
- M. Kotru and N. Singh, Indian J. Orthop., 2007, 41, 97–100 Search PubMed.
- B. Saikia, K. Gupta and U. N. Saikia, Diagn. Pathol., 2008, 3, 25 CrossRef PubMed.
- Y. Lecun, Y. Bengio and G. Hinton, Nature, 2015, 521, 436–444 CrossRef CAS PubMed.
-
F. Limaiem, A. Rehman and T. Mazzoni, Cancer, Papillary Thyroid Carcinoma (PTC), StatPearls Publishing, 2020 Search PubMed.
- H. He, R. Liao, N. Zeng, P. Li, Z. Chen, X. Liu and H. Ma, J. Light Technol., 2019, 37, 2534–2548 CAS.
- D. B. Strader, T. Wright, D. L. Thomas and L. B. Seeff, Hepatology, 2004, 39, 1147–1171 CrossRef PubMed.
- R. M. Haralick, I. Dinstein and K. Shanmugam, IEEE Trans. Syst. Man Cybern., 1973, SMC-3, 610–621 Search PubMed.
- J. M. O’Rourke, V. M. Sagar, T. Shah and S. Shetty, World J. Gastroenterol., 2018, 24, 4436–4447 CrossRef PubMed.
- A. Sood, V. Midha and A. Singh, Curr. Gastroenterol. Rep., 2014, 16, 418 CrossRef PubMed.
- W. Sheng, W. Li, J. Qi, T. Liu, H. He, Y. Dong, S. Liu, J. Wu, D. Elson and H. Ma, Photonics, 2019, 6, 34 CrossRef CAS.
- H. Tamura, S. Mori and T. Yamawaki, IEEE Trans. Syst. Man Cybern., 1978, 8, 460–473 Search PubMed.
- M. C. Montalto, J. Pathol. Inform., 2016, 7, 18 CrossRef PubMed.
- G. C. Giakos, Int. J. Signal Imaging Syst. Eng., 2010, 3, 3–12 CrossRef.
-
J. W. Turkey, Exploratory data analysis, Pearson, 1977 Search PubMed.
-
G. C. Giakos, S. Marotta, S. Shrestha, A. Deshpande, T. Farrahi, L. Zhang, T. Cambria, A. Blinzler, T. Quang, Y. Na, G. Livanos, M. Zervakis and S. Musa, IST 2015 - 2015 IEEE International Conference on Imaging Systems and Techniques, Proceedings, Institute of Electrical and Electronics Engineers Inc., 2015.
- K. Kadota, K. Suzuki, S. S. Kachala, E. C. Zabor, C. S. Sima, A. L. Moreira, A. Yoshizawa, G. J. Riely, V. W. Rusch, P. S. Adusumilli and W. D. Travis, Mod. Pathol., 2012, 25, 1117–1127 CrossRef PubMed.
- H. A. Carvalho, P. H. N. Saldiva, T. Y. Takagaki and V. L. Capelozzi, Histopathology, 1997, 31, 420–429 CrossRef CAS PubMed.
- B. T. Hyman, C. H. Phelps, T. G. Beach, E. H. Bigio, N. J. Cairns, M. C. Carrillo, D. W. Dickson, C. Duyckaerts, M. P. Frosch, E. Masliah, S. S. Mirra, P. T. Nelson, J. A. Schneider, D. R. Thal, B. Thies, J. Q. Trojanowski, H. V. Vinters and T. J. Montine, Alzheimer's Dementia, 2012, 8, 1–13 CrossRef PubMed.
- C. R. Jack, D. A. Bennett, K. Blennow, M. C. Carrillo, B. Dunn, S. B. Haeberlein, D. M. Holtzman, W. Jagust, F. Jessen, J. Karlawish, E. Liu, J. L. Molinuevo, T. Montine, C. Phelps, K. P. Rankin, C. C. Rowe, P. Scheltens, E. Siemers, H. M. Snyder, R. Sperling, C. Elliott, E. Masliah, L. Ryan and N. Silverberg, Alzheimer's Dementia, 2018, 14, 535–562 CrossRef PubMed.
- C. Ising, M. Stanley and D. Holtzman, Clin. Pharmacol. Ther., 2015, 98, 469–471 CrossRef CAS PubMed.
- M. N. Sabbagh and K. Blennow, Neurol. Ther., 2019, 8, 33–36 CrossRef PubMed.
-
A. J. Izenman, Modern multivariate statistical tehniques. Regression, classification, and Manifold learning (Springer texts in statistics), Springer, New York, NY, 2013 Search PubMed.
- G. Biau and E. Scornet, Test, 2016, 25, 197–227 CrossRef.
-
W. Iba and P. Langley, Machine Learning Proceedings 1992, Elsevier, 1992, pp. 233–240 Search PubMed.
- Y. Koronyo, D. Biggs, E. Barron, D. S. Boyer, J. A. Pearlman, W. J. Au, S. J. Kile, A. Blanco, D.-T. Fuchs, A. Ashfaq, S. Frautschy, G. M. Cole, C. A. Miller, D. R. Hinton, S. R. Verdooner, K. L. Black and M. Koronyo-Hamaoui, JCI Insight, 2017, 2, e93621 CrossRef PubMed.
- M. R. Hee, C. A. Puliafito, C. Wong, E. Reichel, J. S. Duker, J. S. Schuman, E. A. Swanson and J. G. Fujimoto, Am. J. Ophthalmol., 1995, 120, 65–74 CrossRef CAS PubMed.
- M. J. Davies and A. C. Thomas, Br. Heart J., 1985, 53, 363–373 CrossRef CAS PubMed.
- L. C. U. Junqueira, G. Bignolas and R. R. Brentani, Histochem. J., 1979, 11, 447–455 CrossRef CAS PubMed.
- D. Chapman, K. T. Weber and M. Eghbali, Circ. Res., 1990, 67, 787–794 CrossRef CAS PubMed.
- M. Salvati, A. Pichierri, M. Piccirilli, G. M. F. Brunetto, A. D’Elia, S. Artizzu, F. Santoro, A. Arcella, F. Giangaspero, A. Frati, L. Simione and A. Santoro, J. Neurosurg., 2012, 117, 204–211 CAS.
- R. Soffietti, B. G. Baumert, L. Bello, A. Von Deimling, H. Duffau, M. Frénay, W. Grisold, R. Grant, F. Graus, K. Hoang-Xuan, M. Klein, B. Melin, J. Rees, T. Siegal, A. Smits, R. Stupp and W. Wick, Eur. J. Neurol., 2010, 17, 1124–1133 CrossRef CAS PubMed.
- P. G. Gritsenko, O. Ilina and P. Friedl, J. Pathol., 2012, 226, 185–199 CrossRef CAS PubMed.
- T. F. Anderson and A. G. Richards, J. Appl. Phys., 1942, 13, 748–758 CrossRef CAS.
- H. Ghiradella, Appl. Opt., 1991, 30, 3492 CrossRef CAS PubMed.
- Y. Fu, C. A. Tippets, E. U. Donev and R. Lopez, Wiley Interdiscip. Rev.: Nanomed. Nanobiotechnol., 2016, 8, 758–775 Search PubMed.
- D. G. Stavenga, H. L. Leertouwer, N. J. Marshall and D. Osorio, Proc. R. Soc. B, 2011, 278, 2098–2104 CrossRef PubMed.
- J. Lee, T. Terlier, Y. J. Jang, K. Lee and Y. Lee, Surf. Interface Anal., 2020, 52, 656–664 CrossRef CAS.
- B.-K. Hsiung, R. H. Siddique, D. G. Stavenga, J. C. Otto, M. C. Allen, Y. Liu, Y.-F. Lu, D. D. Deheyn, M. D. Shawkey and T. A. Blackledge, Nat. Commun., 2017, 8, 2278 CrossRef PubMed.
- J. D. Forster, H. Noh, S. F. Liew, V. Saranathan, C. F. Schreck, L. Yang, J.-G. Park, R. O. Prum, S. G. J. Mochrie, C. S. O’Hern, H. Cao and E. R. Dufresne, Adv. Mater., 2010, 22, 2939–2944 CrossRef CAS PubMed.
- S. Magkiriadou, J.-G. Park, Y.-S. Kim and V. N. Manoharan, Opt. Mater. Express, 2012, 2, 1343 CrossRef CAS.
- S. Magkiriadou, J.-G. Park, Y.-S. Kim and V. N. Manoharan, Phys. Rev. E: Stat., Nonlinear, Soft Matter Phys., 2014, 90, 062302 CrossRef CAS.
- J.-G. Park, S.-H. Kim, S. Magkiriadou, T. M. Choi, Y.-S. Kim and V. N. Manoharan, Angew. Chem., Int. Ed., 2014, 53, 2899–2903 CrossRef CAS PubMed.
- Y. Shen, V. Rinnerbauer, I. Wang, V. Stelmakh, J. D. Joannopoulos and M. Soljač, ACS Photonics, 2015, 2, 23 CrossRef.
- Y. Liu, H. Wang, J. Ho, R. C. Ng, R. J. H. Ng, V. H. Hall-Chen, E. H. H. Koay, Z. Dong, H. Liu, C.-W. Qiu, J. R. Greer and J. K. W. Yang, Nat. Commun., 2019, 10, 4340 CrossRef PubMed.
- B. Yang, H. Cheng, S. Chen and J. Tian, Mater. Chem. Front., 2019, 3, 750–761 RSC.
- S. H. Ko, Nature, 2019, 570, 312–313 CrossRef CAS PubMed.
- M. M. Ito, A. H. Gibbons, D. Qin, D. Yamamoto, H. Jiang, D. Yamaguchi, K. Tanaka and E. Sivaniah, Nature, 2019, 570, 363–367 CrossRef CAS.
- Y. Wang, Q. Zhao and X. Du, Mater. Horiz., 2020, 7, 1341–1347 RSC.
- T. Ellenbogen, K. Seo and K. B. Crozier, Nano Lett., 2012, 12, 1026–1031 CrossRef CAS PubMed.
- A. Kristensen, J. K. W. Yang, S. I. Bozhevolnyi, S. Link, P. Nordlander, N. J. Halas and N. A. Mortensen, Nat. Rev. Mater., 2016, 2, 16088 CrossRef.
- A. B. Evlyukhin, S. M. Novikov, U. Zywietz, R. L. Eriksen, C. Reinhardt, S. I. Bozhevolnyi and B. N. Chichkov, Nano Lett., 2012, 12, 3749–3755 CrossRef CAS.
- P. D. Terekhov, K. V. Baryshnikova, Y. Greenberg, Y. H. Fu, A. B. Evlyukhin, A. S. Shalin and A. Karabchevsky, Sci. Rep., 2019, 9, 3438 CrossRef PubMed.
- G. Quaranta, G. Basset, O. J. F. Martin and B. Gallinet, Laser Photonics Rev., 2018, 12, 1800017 CrossRef.
- Y. Zhou, B. Wang, Z. Guo and X. Wu, Nanomaterials, 2019, 9, 837 CrossRef CAS PubMed.
- M. Keshavarz Hedayati and M. Elbahri, Plasmonics, 2017, 12, 1463–1479 CrossRef.
- Y. Gu, L. Zhang, J. K. W. Yang, S. P. Yeo and C.-W. Qiu, Nanoscale, 2015, 7, 6409–6419 RSC.
-
S. A. Maier, Plasmonics: Fundamentals and Applications, Springer Science + Business Media LLC, New York, NY, 2007 Search PubMed.
- P. Dai, Y. Wang, X. Zhu, H. Shi, Y. Chen, S. Zhang, W. Yang, Z. Chen, S. Xiao and H. Duan, Nanotechnology, 2018, 29, 395202 CrossRef PubMed.
- Y. Jung, H. Jung, H. Choi and H. Lee, Nano Lett., 2020, 20, 6344–6350 CrossRef CAS PubMed.
- S. Ren, L. Zhou, J. Yang and Y. Zhou, Plasmonics, 2021, 16, 167–173 CrossRef CAS.
- C. Jiang, S. Liang, L. Wan, K. Du, W. Zhang, Z. Li, S. Jin Chua and T. Mei, Appl. Opt., 2020, 59, 4432 CrossRef CAS PubMed.
- P. K. Jain, X. Huang, I. H. El-Sayed and M. A. El-Sayed, Acc. Chem. Res., 2008, 41, 1578–1586 CrossRef CAS PubMed.
- J. N. Anker, W. P. Hall, O. Lyandres, N. C. Shah, J. Zhao and R. P. Van Duyne, Nat. Mater., 2008, 7, 442–453 CrossRef CAS PubMed.
- K. Kumar, H. Duan, R. S. Hegde, S. C. W. Koh, J. N. Wei and J. K. W. Yang, Nat. Nanotechnol., 2012, 7, 557–561 CrossRef CAS PubMed.
- S. J. Tan, L. Zhang, D. Zhu, X. M. Goh, Y. M. Wang, K. Kumar, C.-W. Qiu and J. K. W. Yang, Nano Lett., 2014, 14, 4023–4029 CrossRef CAS PubMed.
- T. W. Ebbesen, H. J. Lezec, H. F. Ghaemi, T. Thio and P. A. Wolff, Nature, 1998, 391, 667–669 CrossRef CAS.
- S. J. Heo, S. Kim, G. Pyo, J. H. Yang, J. H. Shin, H.-J. Kwon, H. Kang, G. H. Lee and J. E. Jang, ACS Appl. Electron. Mater., 2020, 2, 2489–2497 CrossRef CAS.
- H. Yun, S.-Y. Lee, K. Hong, J. Yeom and B. Lee, Nat. Commun., 2015, 6, 7133 CrossRef PubMed.
- N. S. King, L. Liu, X. Yang, B. Cerjan, H. O. Everitt, P. Nordlander and N. J. Halas, ACS Nano, 2015, 9, 10628–10636 CrossRef CAS PubMed.
- F. F. Mahani, A. Mokhtari and M. Mehran, Nanotechnology, 2017, 28, 385203 CrossRef PubMed.
- L. Duempelmann, D. Casari, A. Luu-Dinh, B. Gallinet and L. Novotny, ACS Nano, 2015, 9, 12383–12391 CrossRef CAS PubMed.
- L. Duempelmann, A. Luu-Dinh, B. Gallinet and L. Novotny, ACS Photonics, 2016, 3, 190–196 CrossRef CAS.
- Z. Li, A. W. Clark and J. M. Cooper, ACS Nano, 2016, 10, 492–498 CrossRef CAS PubMed.
- J. B. Khurgin and G. Sun, Appl. Phys. Lett., 2010, 96, 181102 CrossRef.
- A. Raman, W. Shin and S. Fan, Phys. Rev. Lett., 2013, 110, 183901 CrossRef PubMed.
- O. D. Miller, A. G. Polimeridis, M. T. H. Reid, C. W. Hsu, B. G. DeLacy, J. D. Joannopoulos, M. Soljačić and S. G. Johnson, Opt. Express, 2015, 24, 3329–3364 CrossRef PubMed.
- D. Inoue, A. Miura, T. Nomura, H. Fujikawa, K. Sato, N. Ikeda, D. Tsuya, Y. Sugimoto and Y. Koide, Appl. Phys. Lett., 2011, 98, 093113 CrossRef.
- A. Henglein, Chem. Mater., 1998, 10, 444–450 CrossRef CAS.
- P. M. Schwab, C. Moosmann, K. Dopf and H.-J. Eisler, Opt. Express, 2015, 23, 26533 CrossRef CAS PubMed.
- H. Wang, F. Tam, N. K. Grady and N. J. Halas, J. Phys. Chem. B, 2005, 109, 18218–18222 CrossRef CAS PubMed.
- V. Vashistha, G. Vaidya, P. Gruszecki, A. E. Serebryannikov and M. Krawczyk, Sci. Rep., 2017, 7, 8092 CrossRef PubMed.
- L. Cao, P. Fan, E. S. Barnard, A. M. Brown and M. L. Brongersma, Nano Lett., 2010, 10, 2649–2654 CrossRef CAS PubMed.
- S. R. Amanaganti, M. Ravnik and J. Dontabhaktuni, Sci. Rep., 2020, 10, 15599 CrossRef CAS PubMed.
- J. Proust, F. Bedu, B. Gallas, I. Ozerov and N. Bonod, ACS Nano, 2016, 10, 7761–7767 CrossRef CAS PubMed.
- V. Vashistha, G. Vaidya, R. S. Hegde, A. E. Serebryannikov, N. Bonod and M. Krawczyk, ACS Photonics, 2017, 4, 1076–1082 CrossRef CAS.
- T. Shibanuma, S. A. Maier and P. Albella, Appl. Phys. Lett., 2018, 112, 063103 CrossRef.
- M. Lawrence, D. R. Barton and J. A. Dionne, Nano Lett., 2018, 18, 1104–1109 CrossRef CAS PubMed.
- E. Klopfer, M. Lawrence, D. R. Barton, J. Dixon and J. A. Dionne, Nano Lett., 2020, 20, 5127–5132 CrossRef CAS PubMed.
- M. Lawrence, D. R. Barton, J. Dixon, J.-H. Song, J. van de Groep, M. L. Brongersma and J. A. Dionne, Nat. Nanotechnol., 2020, 15, 956–961 CrossRef CAS PubMed.
- M. J. Uddin and R. Magnusson, Opt. Express, 2013, 21, 12495 CrossRef PubMed.
- M. J. Uddin, T. Khaleque and R. Magnusson, Opt. Express, 2014, 22, 12307 CrossRef CAS PubMed.
- L. V. Poulikakos, M. Lawrence, D. R. Barton, S. S. Jeffrey and J. A. Dionne, ACS Photonics, 2020, 7, 3216–3227 CrossRef CAS.
- E. Højlund-Nielsen, J. Weirich, J. Nørregaard, J. Garnaes, N. Asger Mortensen and A. Kristensen, J. Nanophotonics, 2014, 8, 083988 CrossRef.
- C.-S. Park, I. Koirala, S. Gao, V. R. Shrestha, S.-S. Lee and D.-Y. Choi, Opt. Express, 2019, 27, 667 CrossRef CAS PubMed.
- X. Wang, J. Chen, T. Guo and Y. Shi, Opt. Express, 2020, 28, 21704 CrossRef CAS PubMed.
- B. Yang, W. Liu, Z. Li, H. Cheng, S. Chen and J. Tian, Adv. Opt. Mater., 2018, 6, 1701009 CrossRef.
- R. Magnusson, D. Wawro, S. Zimmerman and Y. Ding, Sensors, 2011, 11, 1476–1488 CrossRef CAS PubMed.
- C. Wu, N. Arju, G. Kelp, J. A. Fan, J. Dominguez, E. Gonzales, E. Tutuc, I. Brener and G. Shvets, Nat. Commun., 2014, 5, 3892 CrossRef CAS PubMed.
- D. Sell, J. Yang, S. Doshay and J. A. Fan, Adv. Opt. Mater., 2017, 5, 1700645 CrossRef.
- Z. Wang, X. Xu, D. Fan, Y. Wang, H. Subbaraman and R. T. Chen, Sci. Rep., 2016, 6, 24106 CrossRef CAS PubMed.
- S. V. Makarov, A. N. Tsypkin, T. A. Voytova, V. A. Milichko, I. S. Mukhin, A. V. Yulin, S. E. Putilin, M. A. Baranov, A. E. Krasnok, I. A. Morozov and P. A. Belov, Nanoscale, 2016, 8, 17809–17814 RSC.
- Z. Dong, J. Ho, Y. F. Yu, Y. H. Fu, R. Paniagua-Dominguez, S. Wang, A. I. Kuznetsov and J. K. W. Yang, Nano Lett., 2017, 17, 7620–7628 CrossRef CAS PubMed.
- C.-S. Park, V. R. Shrestha, W. Yue, S. Gao, S.-S. Lee, E.-S. Kim and D.-Y. Choi, Sci. Rep., 2017, 7, 2556 CrossRef PubMed.
- D. J. Moss, R. Morandotti, A. L. Gaeta and M. Lipson, Nat. Photonics, 2013, 7, 597–607 CrossRef CAS.
- P. Muellner, E. Melnik, G. Koppitsch, J. Kraft, F. Schrank and R. Hainberger, Procedia Eng., 2015, 120, 578–581 CrossRef CAS.
- Z. Zhou, J. Li, R. Su, B. Yao, H. Fang, K. Li, L. Zhou, J. Liu, D. Stellinga, C. P. Reardon, T. F. Krauss and X. Wang, ACS Photonics, 2017, 4, 544–551 CrossRef CAS.
- Y. Kivshar and A. Miroshnichenko, Opt. Photonics News, 2017, 28, 24–31 CrossRef.
- Y. Kim, K. Jung, J. Cho and J. K. Hyun, ACS Nano, 2019, 13, 10717–10726 CrossRef CAS PubMed.
- I. Koirala, V. R. Shrestha, C.-S. Park, S. Gao, S.-S. Lee and D.-Y. Choi, Sci. Rep., 2017, 7, 13574 CrossRef PubMed.
- T. Xu, Y.-K. Wu, X. Luo and L. J. Guo, Nat. Commun., 2010, 1, 59 CrossRef PubMed.
- Y. Lee, M.-K. Park, S. Kim, J. H. Shin, C. Moon, J. Y. Hwang, J.-C. Choi, H. Park, H.-R. Kim and J. E. Jang, ACS Photonics, 2017, 4, 1954–1966 CrossRef CAS.
- M. Sharma, N. Hendler and T. Ellenbogen, Adv. Opt. Mater., 2020, 8, 1901182 CrossRef CAS.
- B. Gholipour, A. Karvounis, J. Yin, C. Soci, K. F. MacDonald and N. I. Zheludev, NPG Asia Mater., 2018, 10, 533–539 CrossRef CAS.
- C. Xing, S. Chen, M. Qiu, X. Liang, Q. Liu, Q. Zou, Z. Li, Z. Xie, D. Wang, B. Dong, L. Liu, D. Fan and H. Zhang, Adv. Healthcare Mater., 2018, 7, 1701510 CrossRef PubMed.
- M. Qiu, A. Singh, D. Wang, J. Qu, M. Swihart, H. Zhang and P. N. Prasad, Nano Today, 2019, 25, 135–155 CrossRef CAS.
- M. Luo, T. Fan, Y. Zhou, H. Zhang and L. Mei, Adv. Funct. Mater., 2019, 29, 1808306 CrossRef.
- Z. Tang, N. Kong, J. Ouyang, C. Feng, N. Y. Kim, X. Ji, C. Wang, O. C. Farokhzad, H. Zhang and W. Tao, Matter, 2020, 2, 297–322 CrossRef.
- T. Y. Chang, M. Huang, A. A. Yanik, H. Y. Tsai, P. Shi, S. Aksu, M. F. Yanik and H. Altug, Lab Chip, 2011, 11, 3596–3602 RSC.
- Y. Lee, S. J. Kim, H. Park and B. Lee, Sensors, 2017, 17, 1726 CrossRef PubMed.
- M. L. Tseng, Y. Jahani, A. Leitis and H. Altug, ACS Photonics, 2021, 3, 21 Search PubMed.
- N. Ghosh, M. F. G. Wood and I. A. Vitkin, J. Biomed. Opt., 2008, 13, 044036 CrossRef PubMed.
- H. K. Graham, N. W. Hodson, J. A. Hoyland, S. J. Millward-Sadler, D. Garrod, A. Scothern, C. E. M. Griffiths, R. E. B. Watson, T. R. Cox, J. T. Erler, A. W. Trafford and M. J. Sherratt, Matrix Biol., 2010, 29, 254–260 CrossRef CAS PubMed.
- X. Yu, S. Zhang, M. Olivo and N. Li, Photonics Res., 2020, 8, 1703 CrossRef CAS.
- P. Vaiano, B. Carotenuto, M. Pisco, A. Ricciardi, G. Quero, M. Consales, A. Crescitelli, E. Esposito and A. Cusano, Laser Photonics Rev., 2016, 10, 922–961 CrossRef CAS.
- S. Zhang, C. L. Wong, S. Zeng, R. Bi, K. Tai, K. Dholakia and M. Olivo, Nanophotonics, 2021, 10, 259–293 CAS.
- D. Lee, J. Gwak, T. Badloe, S. Palomba and J. Rho, Nanoscale Adv., 2020, 2, 605–625 RSC.
- H. Pahlevaninezhad, M. Khorasaninejad, Y.-W. Huang, Z. Shi, L. P. Hariri, D. C. Adams, V. Ding, A. Zhu, C.-W. Qiu, F. Capasso and M. J. Suter, Nat. Photonics, 2018, 12, 540–547 CrossRef CAS PubMed.
- B. Li, W. Piyawattanametha and Z. Qiu, Micromachines, 2019, 10, 310 CrossRef PubMed.
- V. Sankaran, J. T. Walsh and D. J. Maitland, J. Biomed. Opt., 2002, 7, 300 CrossRef PubMed.
- S. H. Ahn and L. J. Guo, ACS Nano, 2009, 3, 2304–2310 CrossRef CAS PubMed.
- S. Y. Chou, P. R. Krauss and P. J. Renstrom, Science, 1996, 272, 85–87 CrossRef CAS.
- D. Xia, Z. Ku, S. C. Lee and S. R. J. Brueck, Adv. Mater., 2011, 23, 147–179 CrossRef CAS PubMed.
- F. Juillerat, H. H. Solak, P. Bowen and H. Hofmann, Nanotechnology, 2005, 16, 1311–1316 CrossRef CAS.
- R. Sidharthan, V. M. Murukeshan and K. Sathiyamoorthy, J. Nanosci. Nanotechnol., 2012, 12, 6428–6431 CrossRef CAS.
Footnote |
† Authors contributed equally. |
|
This journal is © The Royal Society of Chemistry 2021 |