Similarity of multicomponent nanomaterials in a safer-by-design context: the case of core–shell quantum dots†
Received
27th May 2023
, Accepted 11th December 2023
First published on 15th January 2024
Abstract
Concepts of similarity, such as grouping, categorization, and read-across, enable a fast comparative screening of hazard, reducing animal testing. These concepts are established primarily for molecular substances. We demonstrate the development of multi-dimensional similarity assessment methods that can be applied to multicomponent nanomaterials (MCNMs) for the case of core–shell quantum dots (QDs). The term ‘multicomponent’ refers to their structural composition, which consists of up to four different heavy metals (cadmium, zinc, copper, indium) in different mass percentages, with different morphologies and surface chemistries. The development of concepts of similarity is also motivated by the increased need for comparison of innovative against conventional materials in the safe and sustainable by design (SSbD) context. This case study thus considers the industrial need for an informed balance of functionality and safety: we propose two different approaches to compare and rank the case study materials amongst themselves and against well-known benchmark materials, here ZnO NM110, BaSO4 NM220, TiO2 NM105, and CuO. Relative differences in the sample set are calibrated against the biologically relevant range. The choice of properties that are subjected to similarity assessment is guided by the integrated approaches to testing and assessment (IATA) for the inhalation hazard of simple nanomaterials, which recommends characterizing QDs by (i) dynamic dissolution in lung simulant fluids and (ii) the surface reactivity in the abiotic ferric reducing ability of serum (FRAS) assay. In addition, the similarity of fluorescence spectra was assessed as a measure of the QD performance for the intended functionality as a color converter. We applied two approaches to evaluate the data matrix: in the first approach, specific descriptors for each assay (i.e., leachable mass (%) and mass based biological oxidative damage (mBOD)) were selected based on expert knowledge and used as input data for generation of similarity matrices. The second approach introduces the possibility of evaluating multidimensional raw data by a meaningful similarity analysis, without the need for predefined descriptors. We discuss the strengths and weaknesses of each of the two approaches. We anticipate that the similarity assessment approach is transferable to the assessment of further advanced materials (AdMa) that are composed of multiple components.
Environmental significance
Due to their exceptional optical properties, quantum dots (QDs) are increasingly used as color converters in light-emitting diodes and displays. The lowest energy losses during their application are obtained by QDs that are cadmium-based. Cadmium, due to its toxicity, is a regulated substance under the Restriction of Hazardous Substances (RoHS) Directive, limiting its large-scale use in commercial products. Cd leaching from products containing QDs can be of high concern for both human and environmental health, and the reactivity of the QD particles requires assessment. Due to this significant trade-off between hazard and performance, one must evaluate the design options for safer, yet functional QDs, finding a balance between all safe and sustainable by design (SSbD) dimensions.
|
1. Introduction
Current advances in technology are leading to increasingly complex materials, such as multicomponent nanomaterials (MCNMs). Since many such materials are already on the market, safety information is required. Case-by-case consideration of exposure, hazard, and therefore risk is slow – traditionally using large numbers of animals – and requires a high number of resources. Methods such as grouping of similar substances allow the read-across of data between group members,1 and thereby reduce the burden of animal testing. To justify such an approach in a regulatory context, significant evidence is required to demonstrate similarity between materials; this can be difficult unless a specific strategy is adopted. The concluded EU funded Horizon 2020 project, GRACIOUS, developed a framework and guidance for grouping similar nanomaterials. In contrast to conventional chemicals, nanomaterials cannot be grouped based on structural chemical similarity alone.2 The GRACIOUS framework starts by guiding the development of an appropriate grouping hypothesis.3 The hypothesis proposes similarities in terms of (i) physicochemical characteristics (what they are), (ii) fate or toxicokinetics (where they go) and (iii) hazards (what they do) for each group member. Testing of the hypothesis is achieved via tailored integrated approaches to testing and assessment (IATA), which guide the user to collect existing information, or to generate key relevant information to fill data gaps. The IATA, therefore, allow the collation of the most appropriate information needed to test the grouping hypothesis4–6 which can then be used to support a similarity assessment. The GRACIOUS project generated several quantitative methods to assess the similarity of nanomaterials, both ionizable and non-ionizable like e.g., organic pigments,7 by using intrinsic and/or extrinsic properties deemed important by the IATA nodes, thereby reducing the reliance on expert judgment.8 Yet, grouping and similarity assessment of MCNMs require further elaboration due to their greater complexity in composition.
Among these methods, similarity matrices have often been the preferred approach as they are an easy tool, recommended by ECHA.9 Such a matrix graphically displays the calculated pairwise distance or correlation between grouped materials. It has been recently proved effective in several studies where different mathematical methods were tested.8,10,11 Similarity matrices enable immediate visualization of differences and similarities in a group, for a specific property or a combination of properties. They can reflect the similarity between materials for a bioassay, making it accessible and easy to understand for a wider public; furthermore, they help to find relationships with intrinsic physicochemical parameters that, if tailored during a material's synthesis, can be linked to its safety. Identification of design principles is key12 and aligns both with the best practice in industry13 and with the targets of the recently published safe and sustainable by design (SSbD) framework of the European Commission.14 The choice of the preferred technological alternative is based on the trade-offs between performance, safety and sustainability, which have been addressed in only a few MCNM case studies.15–17 Our work addresses the safety of quantum dots (QDs) and their functionality by screening methods, and thus enables a low-TRL approach to social and economic sustainability, as suggested by the Joint Research Centre (JRC) of the European Commission.14
When applying the existent grouping approaches to QDs, because of their multicomponent nature, more than one component should be addressed at a time, for a certain physicochemical property and/or hazard endpoint. In addition, considerations of hazard and grouping will need to include the potential for different components to interact and generate mixture effects (e.g., synergism through concentration addition or independent action). Due to the potential release of heavy metal ions and complex biological interactions, QDs challenge the existing risk assessment and materials management paradigm.
The present case of core–shell QDs consisted of up to four different heavy metals (e.g., cadmium, zinc, copper, indium), which are present in different mass percentages.18 These multicomponent nanomaterials are developed for their optical properties which allow them to be used in light-emitting diodes,19,20 photovoltaics,21 medical imaging and biosensors.22,23 The market of these multicomponent nanomaterials is anticipated to grow with a forecasted revenue of $16 billion by 2027.24 A number of commercially available QDs are cadmium-based, which could lead to human health issues and environmental damage, related to their production, use, and end-of-life. In fact, because of its toxicity, cadmium is a regulated substance under the Restriction of Hazardous Substances (RoHS) Directive,25 and is banned in the EU in jewellery, brazing sticks and all plastics.26 As a result, semiconductor QDs with low cadmium content and cadmium-free alternatives have been developed. These QDs are highly interesting due to their unique composition-tunable fluorescence across the visible and near infrared region, high photostability, and long excited-state lifetimes.27
In the case of other nanomaterials (NMs),28 inhalation is likely to be one of the most important routes of exposure for the human body. Upon deposition in the respiratory tract, NMs first come into contact with the lung lining fluid (pH 7.4). After cellular uptake, they may also be exposed to the acidic phagolysosomal fluid (pH 4.5). The possibility of NM contact with either fluid leads to a diversity of environments that could promote material dissolution. The physicochemical properties of the specific material will drive whether they dissolve in either the lung lining fluid or the phagolysosomal fluid, or whether they persist within the body for an extended period of time. Either the released ions, the reactive NMs themselves, or a combination thereof can drive adverse outcomes such as cell death, inflammation29,30 and/or genotoxicity.
Here, we demonstrate how elements of the grouping IATA from the GRACIOUS framework, combined with new methods for assessing similarity, can be used to provide SSbD guidance for QDs. We selected established test guideline protocols and standard operation procedures31 for data generation: the QD dissolution behaviour in extracellular lung simulant fluid (LSF, pH 7.4) and phagolysosomal simulant fluid (PSF, pH 4.5),32 as well as the abiotic FRAS assay, to determine the overall surface reactivity of QDs33 when exposed to human blood serum. These two assays have been previously included in the inhalation IATA.6
In this study, we aim to present a way to inform decision-making for the SSbD development of MCNMs, by management practices for components of known toxicity issues. To do so, we use concepts of pairwise similarity and ranking on QDs, both with regard to properties related to hazard and with regard to their functionality, as needed in the SSbD context.
A full SSbD assessment, including the entire product lifecycle, remains beyond the scope and will require further assessment steps.14 We demonstrate how analysing the same data source via multiple approaches can give rise to different outcomes, when addressing the challenging task of MCNM similarity assessment.
2. Materials and methods
2.1. Materials
The tested materials were obtained from PlasmaChem. The specific QDs were synthesized in organic solvent and are hydrophobic. This is by intention for their incorporation in a solid matrix, serving as light converters, e.g., in TV screens. The organic surface treatment reduces the hydrophobicity. For benchmarking purposes, we selected well-characterized mono-constituent NMs obtained from the JRC (ZnO NM110, TiO2 NM105, BaSO4 NM220) and PlasmaChem (CuO). Table 1 provides information on the size and the elemental composition for each QD and reference material. A complete overview of the physicochemical properties, i.e., particle shape (TEM) and surface chemistry (XPS), can be found in the ESI† (Table S1). Human blood serum (HBS) was purchased from Sigma Aldrich (P2918-100ml).
Table 1 Minimum external dimension and elemental composition for five QDs and four reference materials. More information on the characterization can be found in Table S1.† The colour of each QD is related to the colour code used in the graphics in the following sections
Material name |
Minimum external dimension (nm) |
Elemental composition (% wt) |
|
3.3 |
Zn: 19.2 |
Cu: 0.31 |
In: 9.03 |
|
3.4 |
Zn: 1.46 |
Cu: 0.02 |
In: 0.34 |
|
4.6 |
Zn: 1.58 |
Cu: 0.21 |
In: 0.46 |
|
5.7 |
Zn: 33.87 |
Cd: 10.58 |
Se: 5.69 |
|
5.2 |
Zn: 32.73 |
Cd: 20.47 |
Se: 7.09 |
ZnO-NM110 |
70 |
Zn: 80 |
TiO2-NM105 |
21 |
Ti: 60 |
CuO |
6 |
Cu: 80 |
BaSO4-NM220 |
19 |
Ba: 59 |
2.2. Continuous flow system (CFS) dissolution testing
We employed the dissolution setup previously described by Koltermann-Jülly et al. and Keller et al.34,35 The setup is in agreement with ISO19057:2017.31 It involves three main components: (i) the flow-through cell, (ii) the lung simulant medium, and (iii) the quantification technique for the dissolved-ion fraction (see Fig. S1†). Briefly, ∼1 mg of sample was weighted onto a 5 kDa cellulose triacetate membrane (Sartorius Stedim Biotech GmbH, Göttingen, Germany) adding 1–2 drops of ethanol (to ensure complete wetting); the membrane was positioned in the flow-through cell, locked with a 0.45 μm filter. The cell was filled with phagolysosomal simulant fluid (PSF, pH 4.5) or lung simulant fluid (LSF, pH 7.4) (full composition described in Table S2†). The temperature of the flow-through cells was kept constant at 37 ± 0.5 °C. The flow rate was adjusted to 2 mL h−1. Fourteen 10 mL eluate samples were collected over a period of 7 days. After this period, the eluate samples were analyzed through inductively coupled plasma mass spectrometry (ICP-MS) (Nexion 2000b, Perkin Elmer Inc., Waltham, USA) to determine the concentration of ions. Further details on sample preparation and ICP-MS operation can be found in ref. 36.
All dissolution experiments were done in duplicate with an average standard deviation at each sampling point of 1–3%.
2.3. Ferric reduction ability of serum (FRAS)
The FRAS assay is a screening tool to quantify the degree of oxidative damage induced by nanomaterials on human blood serum (HBS) by the observation of its ferric reducing capacity. The depletion of the total antioxidants in HBS due to interaction with ROS produced by the investigated material is quantified using the Fe–2,4,6-tripyridyl-s-triazine (TPTZ) complex. For our reactivity testing measurements, we adopted the FRAS assay multidose protocol published by Gandon et al.33 The protocol involves three fundamental steps: (i) the preincubation of the QDs with HBS for 3 h at 37 °C, (ii) the separation of the QDs from HBS via ultracentrifugation (AUC-Beckman XL centrifuge (Brea, CA, USA) at 11
900 rpm for 150 min) and (iii) the transfer of 100 μL of QD-free centrifuged HBS supernatant to 2 mL of the FRAS reagent solution that contains the Fe(III)–TPTZ complex. Afterwards, the UV-vis spectrum of the iron complex solution is recorded to determine the total antioxidant depletion as a measure of the oxidative potential of materials. A Trolox calibration curve is used as a reference antioxidant to interpret the material oxidative damage results. FRAS assay dose–response curves are built based on triplicate measurements, and we report in Fig. 3 the average standard deviation at each dose level. Additionally, we employ the method developed by Peijnenburg et al.37 to assess the relevance of nanomaterial dissolution during reactivity testing. For this step, we prepare QD samples to evaluate the ion contribution. After an ultracentrifugation step, the ion concentration in QD-free HBS supernatants was determined by ICP-MS (Perkin Elmer, Nexion 2000b, Waltham, MA, USA). Using hydrophilic metal salts (ZnCl2, CuCl2, InCl3, and CdCl2), we prepared ion solutions with equivalent concentrations to those found by the ICP-MS step, and the associated oxidative damage in HBS was measured by the FRAS method. For each QD and ion dose, triplicate measurements were performed. A reactivity test with potential ion interference (DCFH2, based on fluorescence) was excluded.
2.4. Similarity assessment and ranking methods
2.4.1. Data matrix.
The sample set, or ‘data matrix’ in the terminology of the Read-Across Assessment Framework (RAAF), shall not only contain the different SSbD versions of a material (here the five QDs), but shall also contain the reference compounds of a structurally related material with higher-tier data (ideally assessed by CLP categories). Such reference materials also serve as positive and negative controls, and this is often assay-specific. In this manner, relative differences between the material’s versions can be calibrated against the biologically relevant range.8 In the present case, ZnO NM110, BaSO4 NM220, TiO2 NM105, and CuO serve as well-known reference materials. Additionally, and to reduce complexity, for the FRAS assay, CuO and BaSO4 NM220 serves respectively as the positive and negative control. On the other hand, for the dynamic dissolution, ZnONM110 and TiO2 NM105 are the respective positive and negative control. Furthermore, truncation of the data matrix to the biologically relevant range helps to focus the assessment on relevant differences between SSbD versions (e.g., if different SSbD versions are all very slowly soluble, the differences will not be visible in the similarity assessment).
2.4.2. Euclidean distance metrics.
The standard and widely used Euclidean distance algorithm has been selected as one of the methods for pairwise comparison.8 In two dimensions, the Euclidean distance is the length of the line segment between two points on the plane and is calculated by applying the conventional Pythagorean theorem. The generic formula is the square root of the sum of squares of the differences between coordinates of points in a multidimensional space. Threshold values are set by including in the analysis well-characterized mono-constituent NMs for benchmarking purposes. This method has been successfully applied by Jeliazkova et al. for grouping organic pigments.7 There are several reasons behind this choice: first, it is a widely known distance metric, usually selected as the first choice of metric distances, second, it works well with any numerical descriptor value, and third, it is supported by a user-friendly website (eNanoMapper) that also generates the graphical output, becoming accessible to a wide public.38 The user-friendly feature will be crucial for our discussion: indeed, it allows experts in the materials chemistry field, who know the data input very well, but have limited computational knowledge, to create descriptors which are representative of the data set under investigation. The eNanoMapper similarity web tool creates a bridge between different fields of knowledge, allowing a more in-depth evaluation of the case study, which is not limited to the model but includes scientific expertise.38
In addition, to simultaneously identify the associations between intrinsic physicochemical properties and assay generated descriptors, a heatmap of the similarity was generated. The heatmap enables pattern recognition based on the degree of similarity between the QDs and reference materials in the intentionally selected descriptor space. This algorithm uses distance measures to cluster similar data points, here again the Euclidean distance.
2.4.3. Bayesian based metrics.
The Bayesian based metric is a model-based approach using probability distributions to characterize dose–response curves for each MCNM and compare them probabilistically by comparing the likelihood functions for every pair of MCNMs.10 For comparison, the Bayes factor (BF) statistic has the advantage of comparing distributions of properties rather than distances between raw data points. The method has been successfully applied by Tsiliki et al. for grouping NMs under different scenarios10 and different grouping hypotheses.7,39,40 The main difference between the BF approach and the previously presented approach is that based on the given data (in this case a dose–response data matrix for a pair of MCNMs), it estimates the function that best describes them across a range of both observed and unobserved data points, and at the same time can incorporate the assumption of similarity. In fact, the BF compares the model of similarity against the model of dissimilarity; if the resulting BF is a high value, then the two MCNMs are considered to be similar, otherwise dissimilar. In order to set threshold values and distinguish MCNMs into high and low similarity groups, we include in the analysis well-characterized mono-constituent NMs for benchmarking purposes. The BF can be affected up to a degree by this original partition, as any other stochastic algorithm is affected by its initial state. For the purposes of this analysis, the BF method is extended to incorporate the different data sets and produce one integrated value for all data sets. The integration was applied twice here, to integrate dissolution data for the different ions and to calculate one BF value across the different data sets. More details on equations and parameters can be found in Tsiliki et al. 2022.10 For a complete overview of the method and its applications, please refer to ref. 41 in this same issue.
2.4.4. Weighted ordered weighted average (WOWA) similarity.
The proposed method is a metric distance-based similarity assessment where the resulting similarities are not affected by the assessed dataset (i.e., adding or removing candidates doesn’t change previously calculated similarities). It makes use of the arsinh function (i.e., inverse hyperbolic sine) to transform data prior to scaling and evaluates similarity by integrating distances among all the rescaled parameters through the weighted ordered weighted average (WOWA)42 aggregation function. More details with respect to equations and parameters can be found in Zabeo et al. 2021.11 The methodology evaluates distances among toxicological endpoints by evaluating the whole dose–response curve as opposed to the usual distance based on specific descriptors (e.g., NOEC, LC50, etc.). Such a distance is assessed by evaluating the Jensen–Shannon distance among dose–response curves fitted through the application of the SimpleBMD tool43 from the same authors. The Jensen–Shannon distance is the square root of the Jensen–Shannon divergence,44 it respects identity, symmetry and triangular inequality being therefore an appropriate metric. Threshold values are set by including in the analysis well-characterized mono-constituent NMs for benchmarking purposes. A detailed explanation of the methodology is presented in ref. 45 in this same issue.
3. Results and discussion
3.1. Abiotic dissolution under lysosomal conditions
The deposition of MCNMs in the upper and lower lungs involves their interaction with mucus and the lung lining fluid, respectively, as the lungs’ first lines of defense. The solubility of QDs is dependent on the pH environment; hence, the physicochemical properties of the QDs are generally expected to play a key role in their dissolution process in either mucus or the lung lining fluid (pH 7.4), or after cellular uptake and localization into the acidic phagolysosomal fluid (PSF, pH 4.5). Furthermore, QDs may persist within the extracellular regions or the lymph nodes of the lungs for an extended period. We tested the dissolution in two different pH regimes (Fig. 1 and S2†). The studied series of QDs showed almost no dissolution of their main components when exposed to the neutral-pH extracellular lung simulant fluid (see Fig. S2†): zinc was leached up to 2–6%, on average across all QDs in the lung lining fluid after 168 h (one week). Only the minor component (0.5% mass content) copper leached more than 50% of its initial content after one week in the LSF.
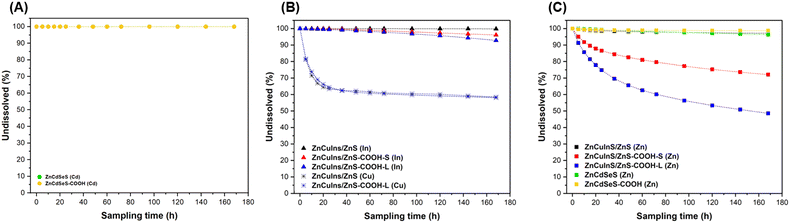 |
| Fig. 1 Time-dependent dissolution kinetics of quantum dots (QDs) in PSF. (A) Cd-based comparison, (B) In- and Cu-based comparison and (C) Zn-based comparison. The color codes indicate the QD identity whereas different symbols are used to indicate the different ion dissolution kinetics: ● = cadmium, * = copper, ▲ = indium and ■ = zinc. All dissolution experiments were done in duplicate with an average standard deviation at each sampling point of 1–3%. | |
On the other hand, we observed different dissolution scenarios in the phagolysosomal simulant fluid that varied between QDs. Fig. 1 plots the time course of ‘undissolved%’ for the main components (Cu, Zn, In and Cd).
In the two ZnCdSeS QD cases, incongruent dissolution occurred, and no Cd (see Fig. 1A), but only Zn leached between 1 and 4% of its initial mass percentage, as observed in Fig. 1C. Moreover, the surface chemistry, as observed from the XPS results from Table 1, seems to impact the zinc leaching behavior. The organic coating performs as a protective layer that hinders the leaching. These results are expected to impact the QD reactivity and toxicity. The selenium concentration was too close to the limit of detection (LOD), making it challenging to deliver an accurate analysis of its leaching behaviour; hence, this element is not included in the figures.
In the three ZnCuInS QD cases, Zn ion release up to 50% of their initial Zn content was observed (Fig. 1C), in contrast to indium (∼5%) (Fig. 1B). The relative differences in leaching are most likely linked to the Zn presence both in the shell and the core of the QDs, whereas In is only present in the core. The hydrophilic-surface modified ZnCuInS/ZnS QDs (ZnCuInS/ZnS-COOH-S and ZnCuInS/ZnS-COOH-L), shown in red and blue, have a faster kinetics of Zn release than their hydrophobic counterparts (ZnCuInS/ZnS). For ZnCuInS/ZnS-COOH-S, copper dissolution is not displayed, as its initial % wt was only 0.02%; therefore the released amount was too close to the LOD of the instrument to be considered significant. It is important to note that the mass of particles which remain undissolved cannot be neglected in the hazard assessment, as they will have some surface area-dependent toxicity, but the release of a high amount of toxic metal ions directly in the cell is toxicologically more potent. For this reason, we include in the discussion a new physicochemical descriptor that accounts for the total (intracellular) leachable particle mass (in PSF) and multicomponent character of the QDs, named ‘leachable mass %’ which is defined as the percentage of the initial loaded mass that turns into ions of a specific element. This descriptor can be used to explore how dissolution may be related to an ion-induced hazard endpoint.46 For single-component metal oxides, the dissolution rate and half times have been employed as descriptors for dissolution.47 However, for multicomponent nanomaterials, the dissolution rate and half times are less representative and can lead to erroneous conclusions. Moreover, high PSF leaching is typically hazardous, since cellular particle uptake followed by PSF leaching leads to a highly localized bioavailable ion dose inside the cell, which can be highly toxic. On the other hand, high leaching under neutral conditions (LSF) is typically considered of low hazard since ions are released outside the cell where they are typically quickly diluted avoiding local accumulation of these ions, and cellular ion uptake is controlled very tightly – while particle uptake is often not.
Fig. S4A† reports the values for dissolved ions % and leachable mass % for each QD in PSF. Fig. S4B† explains the difference between the two descriptors, taking as an example two QDs: ZnCuInS/ZnS-COOH-S and ZnCdSeS. On the left side, absolute ion concentration in the liquid containing the leached ions, calculated from ICPMS, is shown. For ZnCuInS/ZnS-COOH-L, we observe 51% and 42% for Zn and Cu, respectively. However, given the low mass percentage of both the elements in the QDs compared to organic polymer and sulfur, the corresponding leachable metal mass % accounts only for 0.8% and 0.1% for Zn and Cu, respectively. For ZnCdSeS instead, despite the lower relative dissolved fraction of the Zn ions (4% as compared to 51%), we find a higher release in Zn mass (1.3% as compared to 0.8%) due to the higher wt% of Zn in the ZnCdSeS QD structure (34% wt). Thus, the sum of leachable metal mass % over all leaching metals allows for direct calculation of the leached ion mass from the total QD mass, which is typically recorded for dosimetry purposes. As an outlook for methodology transfer, we highlight that the CFS with ICPMS analytics is only applicable to inorganic, ionizing nanomaterials, but the CFS also was adapted by using different detection techniques such as UV-vis or LC-MS to target organic materials (i.e., organic pigments).7 The same approach could then also address the multicomponent leaching of additives from micro- and nanoplastics as another MCNM case.
3.2. Fluorescence spectroscopy and transformation
In the SSbD context of product development, the different designed versions of a MCNM (here QDs) must also be ranked according to their intended functionality, to balance the four different dimensions of SSbD. As defined by the JRC, structural modifications at the design phase can impact the service of a product and the extent of its function, together with its quality and duration. Specifically for QDs acting as colour converters, the sustainability benefit by energy savings during the use phase are enabled by the high fluorescence yield and narrow emission spectra.48–51 Some modifications may result in low functionality, and/or may have unacceptable hazard profiles; in industry, either of the criteria would lead to a stop being applied to the further development of this newly designed version. Fig. 2A showcases the relative quantum yield (%) of Cd-free and Cd-containing QDs, highlighting how the Cd-containing versions (here ZnCdSeS and ZnCdSeS-COOH) are considerably more performant (40–90%) than the Cd-free QDs (20 to 60%). Values were taken from the producer website (http://www.plasmachem.com).
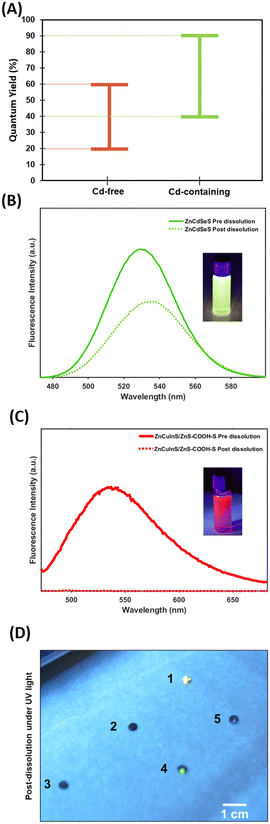 |
| Fig. 2 Quantum yield and fluorescence spectra of the tested QDs before and after PSF exposure. (A) Quantum yield of Cd-free and Cd-containing QDs from Plasmachem (http://www.plasmachem.com). (B and C) Fluorescence emission spectra of pre-dissolution and post dissolution in PSF of ZnCdSeS and ZnCuInS/ZnS-COOH-S QDs, respectively. Inset photos represent the pre-dissolution QDs under UV light showcasing the material's photophysical properties. (D) Post-dissolution centrifuge recovered QDs deposited on TEM grids exposed to UV light. Code: 1. ZnCuInS/ZnS, 2. ZnCuInS/ZnS-COOH-S, 3. ZnCuInS/ZnS-COOH-L, 4. ZnCdSeS, 5. ZnCdSeS-COOH. 1, 4, and 5 QDs show retained photophysical properties after the dissolution process. | |
The fluorescence of QDs is related closely to their functionality and, as an effect of quantum confinement, the electron cloud in the particle, the fluorescence is very sensitive to the shape, size, and composition of the semiconductor particles; therefore, any change in the fluorescence spectrum after abiotic testing is a hallmark sign of transformation of the QD materials. Generally, the emission wavelength is monotonously dependent on the size of the quantum dot. If the QD size is reduced, a blue shift is expected. Consequently, fluorescence can be substantially impacted by leaching due to either change in size, shape, or elemental composition. Here, we observed that the QDs, particularly those with highly hydrophobic surfaces (i.e., ZnCdSeS), retain their optical properties with minimal shifts in their emission spectra (Fig. 2B). For more soluble QDs, like the ones with a hydrophilic surface coating, EDXS-TEM reveals the breakdown of their crystalline nanostructure after incubation in PSF, with no trace of their optical emission spectra and no substantial QD structural details (see Fig. S3 and S6†). The evidence on structural transformation from fluorescence properties is thus in agreement with and complementary to the detection of ion leaching. For completeness, Fig. S3† showcases the fluorescence emission spectra of the other three QDs, where ZnCuInS/ZnS retains its optical properties, ZnCuInS/ZnS-COOH-L shows no substantial trace of fluorescence, and ZnCdSeS-COOH, because of low recovered amounts, presents a discernable low signal-to-noise ratio emission spectrum. We further investigated the transformation of structural details after abiotic testing in PSF with electron microscopy methods (Fig. S6†). In the representative TEM micrographs, we found traces of QDs only in the ZnCuInS/ZnS and ZnCdSeS cases. The other samples contained mostly Zn and S as trace elements. All of the TEM grids with post-dissolution hydrophilic QDs contained other elemental traces (Na, Ca, K, Mg, and Cl) from the PSF medium.
3.3. FRAS assay
At the cellular level, nanomaterial exposure can induce oxidative stress by the production of reactive oxygen species (ROS). Here we evaluated dose dependent changes in cellular antioxidant depletion (FRAS assay) in response to QD exposure (Fig. 3A–C). Due to the semiconducting properties of this set of materials, QDs can directly impact the formation of ROS from electron or hole donation. The descriptor selected to compare QDs with each other is the mass based biological oxidative damage (see Table 2). A mass-based metric was preferred to align with mass-based assessment in regulatory testing. However, the ranking may change when potency is compared in other dose metrics52 considering the difference in the surface area of the five QDs. Fig. 3D reports the reactivity results in surface-based metrics. We followed the method described by Peijnenburg et al. to measure the released ion contribution to reactivity, expressed as % of total MCNM reactivity (Table 2).53 Briefly, the dissolved ion mass in serum was quantified by ICPMS for each QD; then, reconstituted samples at that ion concentration (recreated by using common salts) were measured for FRAS reactivity, expressed as % of total MCNM reactivity (Table 2). As for the Cd-free QD series, ZnCuInS/ZnS-COOH-S presented significant reactivity with a relatively steep dose–response curve with an exponential trend. The collective ion contributions (Cu2+, Zn2+ and In3+) at a dose of 7.33 g L−1 were tested to have a negligible impact on the ferric reduction ability of HBS. Hence, the reactivity of this QD is mostly attributed to the particles. The ZnCuInS/ZnS-COOH-L dose–response curve displayed a more linear trend. The ions (Cu2+, Zn2+ and In3+) show a 51% contribution to reactivity, at a concentration of 7.2 g L−1; hence, both ions and particles contribute substantially to the overall material reactivity. On the other hand, the hydrophobic ZnCuInS/ZnS QDs presented lower reactivity with a dose–response curve around 10
000 nmol TEU L−1 oxidative damage across most data points. In this case, the ion mixture reactivity is higher than that of the same data point tested for the relevant QDs; because this material is not surface functionalized and the ion percentage content is more significant than for the other two cases, its reactivity, specifically from copper and zinc ions, based on our dissolution experimental data, is hypothesized to substantially contribute to the QD overall reactivity as shown in Fig. 1A.
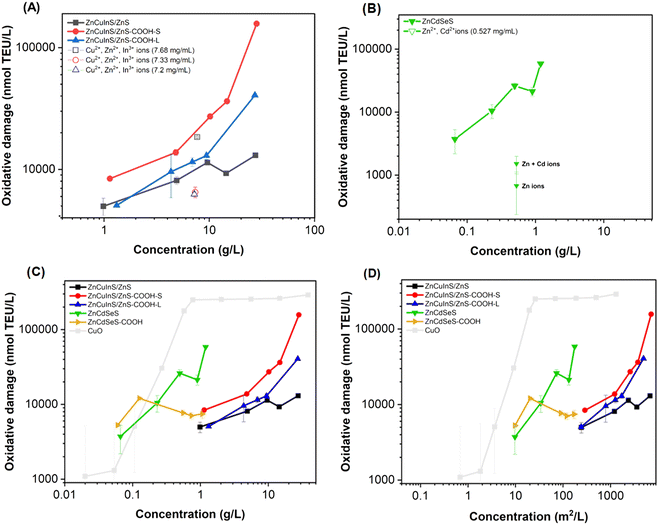 |
| Fig. 3 Dose–response curves from the FRAS assay for the series of pristine ZnCuInS/ZnS and ZnCdSeS and surface-modified ZnCuInS/ZnS and ZnCdSeS QDs. (A and B) Dose–response data of QDs (lines and symbols) and their released ion (color-coded open symbols) concentrations. TEU, Trolox equivalent units. (C and D) Compiled dose–response curves contrasted against the CuO oxidative damage in terms of mass and surface area dose metrics, respectively. For each QD and ion dose, triplicate measurements were performed (standard deviation is reported in the figures). | |
Table 2 Attribution of parts of the MCNM reactivity to ion-induced oxidative damage. For each QD and ion dose, triplicate measurements were performed (standard deviation is reported in Fig. 3)
QD |
Selected MCNM concentration to check ion-vs.-particle contribution to reactivitya (g L−1) |
Total MCNM reactivity by FRAS (nmol TEU L−1) |
Measured and reconstituted concentration of ions (mg L−1) |
Ion release (% of MCNM metal content) |
Ion-induced FRAS reactivity (% of MCNM reactivity) |
Concentration selected according to Peijnenburg et al.53
|
ZnCuInS/ZnS |
7.8 |
18 412 |
Zn: 71.3 |
Zn: 0.17835 |
100% |
Cu: 9.5 |
Cu: 0.0004 |
In: 5.0 |
In: 0.0059 |
ZnCuInS/ZnS-COOH-S |
7.33 |
6495 |
Zn: 0.34 |
Zn: negligible |
37% |
Cu: 9.86 |
Cu: negligible |
In: 4.27 |
In: 0.0002 |
ZnCuInS/ZnS-COOH-L |
7.20 |
6244 |
Zn: 105.7 |
Zn: 0.023 |
51% |
Cu: 10.8 |
Cu: 0.0003 |
In: 1.8 |
In: 0.0001 |
ZnCdSeS |
0.53 |
1516 |
Zn: 0.95 |
Zn: 0.18 |
6% |
Cd: 0.011 |
Cd: 0.0021 |
ZnCdSeS and its surface-functionalized derivative QDs (ZnCdSeS-COOH) present higher reactivity at lower concentrations when compared against the Cd-free QDs (Fig. 3C). The hydrophobic version of the material does not behave as ZnCuInS/ZnS at high concentration. Indeed, ZnCuInS/ZnS reaches supersaturation at levels where the oxidative damage is lower than 10
000 nmol TEU L−1. Instead, ZnCdSeS presents a linear trend with a steep slope that, after extrapolation, can lead to high levels of oxidative damage (∼100
000 nmol TEU L−1) with doses around 10 g L−1. This QD material is found to be highly reactive, comparable to the reactivity observed for CuO at lower doses. The observed reactivity may be – at least partially – induced by leached ions rather than particles. The ion contribution to reactivity (Zn2+ and Cd2+) was tested at a dose of 0.53 g L−1 (see Fig. 3B). The contribution of Zn2+ ions was considered first and then it was combined with the contribution of Cd2+ ions.
The results show a slightly higher oxidative damage when Cd2+ ions are included, meaning that despite the low amount of cadmium dissolved in the serum at the tested concentration, it cannot be neglected. Overall, the contribution to reactivity coming from the ions is attributed to only 6% of the oxidative damage, meaning that the reactivity is mainly attributed to the particles. For ZnCdSeS-COOH instead, no quantifiable ion contribution to reactivity was found, and not shown in Table 2.
Despite the hydrophobic-surface coating on the ZnCdSeS QDs, their reactivity was found to induce the highest HBS antioxidant depletion, regardless of their similar specific surface areas. When the mass dose was expressed as surface area, the dose–response curves showed two distinctive correlation regimes (Fig. 3D): the first one at lower doses, where the oxidative damage by the Cd-containing QDs is higher than that of the Cd-free QDs also in surface metric, and the second one, where the dose–response curves of the three Cd-free QDs are more similar to each other in surface metric. The Zn content at the surface, independent of the size of the material, is hypothesized to govern the oxidative damage. The difference in Zn content between ZnCdSeS and ZnCdSeS–COOH (33.9% and 32.7%, respectively) is almost insignificant. However, as per XPS surface analysis (Table S1†), consistently more zinc surface-based sites were readily available on the surface of ZnCdSeS. This may be one of the reasons why the hydrophobic material is more reactive than its hydrophilic version.
Our results indicate that organic modification or surface treatment, which is intended to increase the compatibility of the material with the intended matrix (often polymer, rarely water), can play a major role in its reactivity. The organic surface treatment can shield the reactive surface sites by steric hindrance – as probably occurred for the Cd-containing QDs – and was presumably not compensated by the increased dispersed surface area. On the other hand, the increase of dispersed surface seems to have increased the reactivity of the Cd-free quantum dots with the organic surface treatment. Nevertheless, in both cases, even a release of less than 1% of the contained metals as ions can contribute substantially to the total observed reactivity (Table 2), and in one case could entirely explain the MCNM reactivity.
4. Similarity assessment
4.1. Important considerations for similarity analysis and grouping of MCNMs
Answering the first of the key questions required for grouping as set out in the GRACIOUS framework (what they are, where they go and what they do?), Table 3 shows the pre-screening results of the chemical and physical characteristics of the QDs. A description of their main chemical constituents, structure, size, mass specific surface area, and surface functionalization is provided for each QD. Once a description of ‘what they are?’ is given, the questions ‘where they go’ and ‘what they do’ need to be addressed, identifying what may be of concern for human hazard.2 The core–shell structure of each QD and the surface functionalization of the shell play an important role in the particle fate upon inhalation, and they have a strong influence on the dissolution rate of single element components. Indeed, metals located in the outer shell of the QDs might dissolve more easily and quickly under physiological conditions, as compared to the metals located in the core. Also, the non-functionalized QDs contain a relatively high mass fraction of heavy metals, which are more easily accessible compared to the functionalized ones. However, reporting the relative contribution to dissolution for each metal element do not provide absolute information on the dose of metal released. It is more relevant, from a risk assessment point of view, to report the amount of leachable metal mass per mg of material, in a specific physiological medium. A similar mass-based descriptor was used by Karlsson et al.46 to describe dissolution of multicomponent alloys.
Table 3 Pre-screening results of the chemical and physical characteristics of the QDs, answering the question of ‘what they are?’. More information on the characterization can be found in Table S1†
What they are? |
ZnCdSeS-COOH |
ZnCuInS/ZnS-COOH-S |
ZnCuInS/ZnS-COOH-L |
ZnCdSeS |
ZnCdSeS-COOH |
CLP = classification labelling and packaging of substances and mixtures.
|
Structure |
ZnCuInS core/ZnS shell |
ZnCuInS core/ZnS shell |
ZnCuInS core/ZnS shell |
No internal structure |
No internal structure |
Surface functionalization |
None hydrophobic |
–COOH hydrophilic |
–COOH hydrophilic |
None hydrophobic |
–COOH hydrophilic |
Specific surface area (m2 g−1) |
255 |
247 |
183 |
147 |
162 |
Size (nm) |
3.3 |
3.4 |
4.6 |
5.7 |
5.2 |
Zn |
Very toxic to aquatic life, with long lasting effects (CLPa) |
Cu |
Very toxic to aquatic life, with long lasting effects; toxic if inhaled; harmful if swallowed and causes serious eye irritation (CLPa) |
In |
Causes damage to organs through prolonged or repeated exposure (CLPa) |
Cd |
Fatal if inhaled; very toxic to aquatic life, with long lasting effects; may cause cancer or damage to organs through prolonged or repeated exposure; suspected of causing genetic defects, damaging fertility or the unborn child (CLPa) |
Se |
May cause cancer, may damage fertility or the unborn child; harmful to aquatic life with long lasting effects, may cause harm to breast-fed children, and may cause an allergic skin reaction (CLPa) |
For this reason, we propose that the leachability of each heavy metal contained in the QDs should be assessed. Given that QDs were seen to be more affected by acidic rather than neutral pH, the phagolysosomal simulant fluid (PSF, pH 4.5) was selected for the assessment of leachability. In addition, QD and/or ion reactivity is to be included in the evaluation. If partial metal leaching is observed, intact or transformed particles together with metal ions are assumed to be bioavailable, and if bioactive, can ultimately lead to toxic effects (i.e., antioxidant damage, ROS generation, inflammation, etc.). Based on the finding that a leachate consisting of <1% of the contained metals induces oxidative damage comparable to that of the remaining particles (Fig. 3 and S4†), the simplest grouping would only consider the QD core/shell composition. This may constitute a “tier 0” assessment and provides a “design principle” in the JRC SSbD terminology, whereas our experimental testing constitutes already a higher-tier approach.
The leachability of the material will have a direct influence on the mode of action of a material's bioactivity. If no metal leaching is observed, the hazard may be driven by multicomponent particles (QDs) themselves, which has been shown to depend on various factors including the type of surface material54 (functionalization), crystallinity55 and shape of the particles.56 Even when particles show no specific toxicity and do not dissolve (poorly soluble low toxicity class (PSLT class)),57 there is a certain threshold dose for toxic effects due to long-term particle persistent and excessive build-up of dose, whereas other particles (such as QDs) induce specific toxicity (e.g. due to surface functionalization). In this last case, the surface chemistry of the particles and their bioavailability needs to be further assessed. In contrast, if complete metal leaching is observed (i.e., just the polymer matrix is left undissolved), only the potential ion induced toxicity needs to be considered.53 Finally, in the most complex case, both ions and multicomponent particles are potentially present in the lungs because of partial leaching of the metals in analysis. Here, both particle and ion induced toxicity might be expected.
For complex materials, a further important question about their fate concerns the formation of transformation products. If transformation occurs, mixture effects caused by the multiple transformed components, generated from the primary QD structure, can lead to different interactions with biological sites and need to be additionally considered. After the main concerns connected to their structures are identified, suitable methods to assess their potential hazard need to be selected.
The FRAS assay has been previously identified as a suitable in chemico method supporting decision making in grouping of NFs.39,47 Grouping approaches are explicitly considered by the REACH legal text58 and has been put into practice by ECHA for hazardous chemicals.59 For this study, we take the so-called ‘analogue approach’, as described in the RAAF document,1 given that read-across is employed between a small number of structurally similar substances with no trend or regular pattern on the properties.
Developing grouping approaches for MCNMs like QDs can benefit both the scientific community and regulatory authorities. The novelty of the present study does not lie in developing new experimental methods to assess MCNMs, but in comparing different approaches to similarity assessment, aiming to support the grouping, and ranking of MCNMs. Often ranking is done to identify the most toxic member of the group and to do apical testing on this form so that the precautionary principle can be applied. However, for SSbD purposes, the ranking provides a perspective in terms of trade-off decisions between safety and functionality.
In the first approach, the descriptors presented in this section will be the input data, and in the second approach, multidimensional raw data will feed the similarity analysis. We will show that different approaches can lead to different similarity results, even within the same initial experimental data sets.
4.2. Similarity assessment with respect to hazard
4.2.1. Similarity assessment by using selected descriptors as input data.
In the first approach to similarity assessment, two descriptors have been used as input data to build similarity matrices based on pairwise similarity of QDs in terms of the Euclidean distance. Different assays and descriptors for surface reactivity have been recommended.6,39 Here, we demonstrated the assessment on the mass based biological oxidative damage (mBOD) at 1 g L−1 (an intermediate concentration level suitable for discriminating the FRAS dose–response curves for all of the particles investigated here) and, for bio-dissolution, the leachable mass % (all element components added) during 7 days of exposure to PSF (pH 4.5) in a continuous flow system (see Table 4). The combination of the results obtained for each descriptor through pairwise similarity analysis is proposed here for material grouping and ranking with respect to benchmark materials. Four conventional single component metal oxide particles have been included as reference materials: CuO and ZnO-NM110 as positive controls, and BaSO4-NM220 and TiO2-NM105 as negative controls, respectively, for surface reactivity and dissolution. It is important to rank the QDs based on their physicochemical data and to compare the range of their values to the responses of well-known benchmark materials. Fig. 4 shows the results obtained within the Euclidean distance approach for the QD series. Regarding the leachable mass %, a descriptor of dissolution at acidic pH, all five QDs appear quite similar to each other (indicated by greenish colours in Fig. 4A). The similarity to the benchmark materials is particularly interesting. ZnCdSeS shows the shortest distance to CuO and ZnO, commonly known as “quickly dissolving” materials, even if it's only 2% of the positive control.6,35,47 This suggests that a possible mode of action for this QD material is via release of ions. On the other hand, the three ZnCuInS/ZnS variants are very similar to TiO2 (very slow dissolution) and present larger distances to both CuO and ZnO (Fig. 4A). Although the chosen descriptor is novel, it results in plausible results also for the well-characterized mono-constituent NMs: the dark red colour highlights the very low similarity between CuO and TiO2, and the yellow colour marks the intermediate distance between either and BaSO4, fully in line with earlier similarity analyses of the overall dissolution rate or overall halftime.8
Table 4 Leachable mass % and mBOD descriptors for the selected benchmarks and the 5 QDs
|
Leachable mass % (during 7 d in PSF, all components added) |
mBOD (nmol TEU mg−1) from FRAS at 1 g L−1 dose |
ZnO-NM110 |
45 |
25.1 |
TiO2-NM105 |
0.31 |
5.0 |
CuO |
77 |
310 |
BaSO4-NM220 |
22 |
0.3 |
ZnCuInS/ZnS |
0.59 |
5.1 |
ZnCuInS/ZnS-COOH-S |
0.42 |
12 |
ZnCuInS/ZnS-COOH-L |
0.88 |
3.8 |
ZnCdSeS |
1.30 |
49.2 |
ZnCdSeS-COOH |
0.42 |
7.0 |
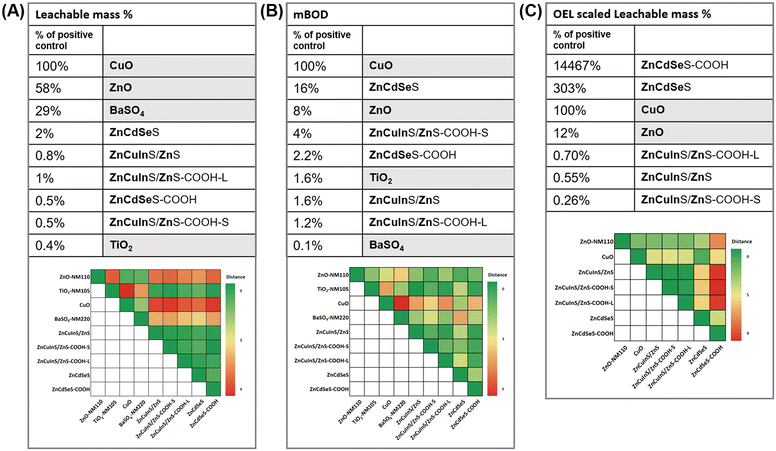 |
| Fig. 4 Ranking and similarity analysis on three selected descriptors. All similarity plots show Euclidean distance. A) Leachable mass % after one week dissolution in PSF, all components added; B) reactivity by the FRAS assay as mass-based oxidative damage at 1 g L−1; C) leachable mass of each element scaled by its respective OEL. In all cases, CuO and ZnO serve as positive controls, whereas BaSO4 and TiO2 as negative controls. | |
Regarding the mBOD, a dark red square (indicating very low similarity) marks the comparison between CuO and BaSO4, which are well-known to be highly reactive and non-reactive, respectively.39 Comparing the opposite mono-constituent NMs for benchmarking is important to confirm that the similarity assessment algorithm is working well with the selected descriptor.8 In the QD case, ZnCdSeS is the closest in the distance to CuO and seems to differ from the other 4 QDs in the series. The results adequately reflect the dose response curves in Fig. 3C.
In addition to the similarity matrix, Fig. 4 shows the rankings based on each single descriptor as a table. According to the safe and sustainable by design concept from the JRC of the European Commission, a relative ranking of materials is also a valuable tool for risk assessment purposes.14 Here, we opted to give for each test material a relative potency as a percentage compared to the materials used for benchmarking. The proposed approach provides relevant information to assess if the QD material ranked highest in performance (e.g., from fluorescence) is still similar to the alternatives in reactivity and metal leaching, and how the entire QD family compares with respect to materials that share similar concerns (i.e., benchmarks). A heat map of the similarity analysis which further considers the size and specific surface area of the QDs and the benchmark materials (Fig. 5) confirm once more that benchmark materials are well recognized as such by the chosen descriptors.
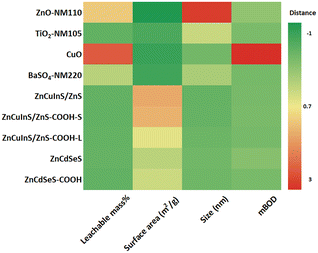 |
| Fig. 5 Heatmap showing similarities based on assay descriptors (leachable mass % and mBOD) and intrinsic physicochemical properties (size and surface area). The legend color bar represents the association between the descriptors and the AdMa. Here red indicates an increase in assay descriptors and intrinsic physicochemical property values compared to the mean for QDs and reference materials, and green indicates a decrease in these values compared to the mean. | |
However, the ranking of QDs based on the discussed descriptors is not yet directly linked to a hazard endpoint, even though the proposed descriptors can guide grouping decisions. In order to account for the different hazardous metals present in the QDs, we suggest scaling the leachable mass% of each component by the value of the occupational exposure limit (OEL) of that element (see Table S3†). OELs may be a useful tool to create a bridge between physicochemical data and hazard prediction. If the descriptors are scaled for the potential induced hazard, they are better positioned for ranking. Fig. 4B highlights that the big difference between the Cd-containing and Cd-free QDs results from the scaling of each leaching element by its Maximale Arbeitsplatz-Konzentration (MAK-values), which are commonly accepted OELs for the inhalation exposure.14 This newly constructed descriptor differentiates more between CuO and ZnO and positions the Cd-free QDs more similar to ZnO, but ranks them lower than ZnO, even when all leaching metals are considered. Considering that also the reactivity of the Cd-free QDs was on the same order, but ranked lower than ZnO, the safety measures for ZnO, for which nanoforms are registered in the REACH dossier, could serve as guidance for safety measures applicable to the Cd-free QDs. In contrast, the Cd-containing QDs rank higher than ZnO, demonstrating the need to limit their use or find alternatives. In future, ranking can be validated by empirical hazard data from further in vitro and in vivo studies if required.
4.2.2. Similarity assessment by using multidimensional raw data as input data.
In this section, multidimensional raw data were used directly to build data matrices of pairwise similarity values between QDs using two different computational approaches, the Bayesian based and the WOWA based similarity approaches. To describe abiotic dissolution, the concentration of ions vs. time was selected as representative input data, whereas for surface reactivity, the dose–response curves obtained by the FRAS assay (i.e., oxidative damage vs. concentration) were used as designated raw data. In order to integrate the dissolution of all different ions, % wt for each element has been used for both approaches. Specifically, a single dissolution kinetics was generated by applying a weighted average at each time point using the QD composition percentages as weights. This approach, if proved to be efficient, can save time and effort, as no descriptor is needed nor previous knowledge of the investigated data set. Data can be translated directly from the raw data to the similarity matrix display. (Fig. 6).
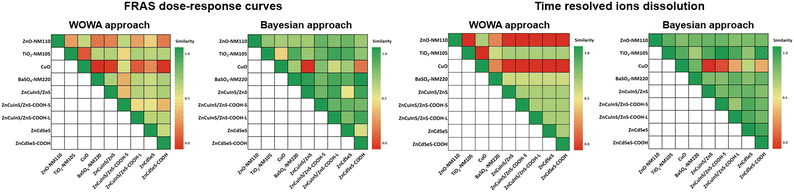 |
| Fig. 6 Similarity assessment on multidimensional raw data (FRAS dose response and time resolved ion dissolution) by two alternative algorithms, the WOWA and Bayesian approaches. | |
For surface reactivity, the WOWA approach identifies the low similarity (dark red) of CuO vs. BaSO4, quite consistent with the descriptor-based approach (Fig. 4B), and finds similarity within the QD family except for ZnCuInS/ZnS-COOH-S, which is more reactive in the raw data (Fig. 3C). The similarity plots obtained using the Bayesian approach do not identify the same benchmarks as most similar and least similar but do identify similar patterns for the QD family, with a difference in magnitude (Fig. 6). We attribute the different results to the very different approaches to multidimensional data, e.g., the slope of dose–response curves may be similar, and yet the effect threshold may be very different. Both contribute to the Bayesian comparison of the multidimensional raw data but are not equally relevant for toxicity rankings. As another complication, the material with the lowest reactivity, BaSO4, is below the limit of quantifiable reactivity for low doses, resulting in a narrow range of data. In consequence, the Bayesian approach to the dose–response curve has a hard time assigning the expected low similarity (dark red) to the pair CuO vs. BaSO4, and instead finds a higher similarity of ZnO vs. BaSO4 (Fig. 6), and within the QD family. Nevertheless, the two approaches seem to agree with each other on the pairs of similar QDs identified (Fig. 6), although the WOWA approach suggests lower similarity values overall.
For time resolved dissolution, the WOWA approach is again in good agreement with what is expected for the well-characterized NMs used for benchmarking. Indeed, ZnO is found to be very different to TiO2 and very similar to CuO, matching the visual impression of the kinetics.35,36 However, it does not discriminate between the dissolution behaviour of the family of Cd-free and Cd-containing QDs. The Bayesian approach considers essentially all materials as quite similar to each other, except for CuO, which is found to be different from all other materials. This is surprising and – at least for the mono-constituent NMs – does not match the assessment by more conventional descriptors such as the halftime or dissolution rate, which was successfully assessed by the same algorithm before.8
4.2.3. Combination of similarity output from different physicochemical properties for the purpose of grouping.
As stated above, similarity assessment on single descriptors is not directly linked to a hazard endpoint but can help to guide grouping decisions. As an additional tool to support SSbD decisions, one can assess all properties – excluding the functionality – in one similarity value. Combination of properties, in the form of multidimensional data matrices of each one descriptor per property, analyzed by a multidimensional distance to represent the pairwise similarity between two materials, has been previously demonstrated.8 Here instead, we try to combine multidimensional raw data (vectors instead of scalars) in one pairwise similarity between two materials, by using the WOWA and the Bayesian approaches. The ‘WOWA distance’ and Bayesian aggregation similarity metrics presented in Fig. 7 were obtained by integrating intrinsic physicochemical properties, kinetics, and surface reactivity. More precisely, single parameter similarities are evaluated separately for surface area, mean diameter, time resolved ion dissolution, and surface reactivity (the last two were presented in section 4.2.2. above). The integration of values is performed by giving reactivity a slightly higher importance relative to other parameters. Interestingly, the WOWA approach finds a distance of 0.76 between CuO and BaSO4, this seems to reflect well the properties of the materials, very different in terms of surface reactivity, but not as different in terms of abiotic dissolution, mass specific surface area, and size. Again, a higher similarity is observed between TiO2 and BaSO4. They both show similar surface reactivity behaviour, together with a similar size and mass specific surface area, differing mainly in the dissolution behaviour as TiO2 is well-known to be extremely low dissolving, here indeed chosen as a negative control for surface reactivity. As for the QDs, a higher similarity is observed between the ones similar in composition i.e., ZnCdSeS with ZnCdSeS-COOH, and ZnCuInS/ZnS with ZnCuInS/ZnS-COOH-L and ZnCuInS/ZnS-COOH-S. The aggregated Bayesian factor distance is less differentiated and assigns overall high similarities. The Bayesian aggregated approach finds a distance of only 0.37 between CuO and BaSO4, the respective positive and negative controls for the surface reactivity assay. Instead, coherently to previous studies, a higher similarity between TiO2 and BaSO4 is observed. The QDs form a block of relatively high similarity values, particularly between ZnCuInS/ZnS and ZnCuInS/ZnS-COOH-L, and also ZnCdSeS-COOH.
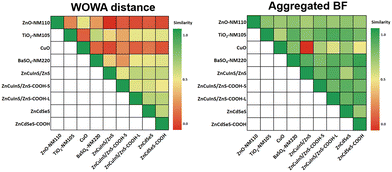 |
| Fig. 7 Combination of both intrinsic and extrinsic physicochemical properties relevant to the safety assessment – excluding functionality – in a unique weighted matrix showing overall similarity and dissimilarities of materials. On the left, WOWA distances; on the right, aggregated Bayesian factors. | |
4.3. Similarity assessment with respect to functionality
To represent a typical industrially relevant SSbD decision need, similarity-based hazard assessment of QDs can be combined with similarities on a functional parameter (here: fluorescence intensity per initial mass of QDs). We reiterate that the QDs' purpose is to act as colour converters in TV screens, where they increase the energy efficiency due to lower non-radiative losses than the conventional pigment-based colour filters.48,49,51,60 In both the Bayesian and the WOWA generated matrices shown in Fig. 8, ZnCdSeS appears to be different from its hydrophilic analogue and from ZnCuInS/ZnS-COOH-S. This trend is also supported by the lack of stability, where both ZnCdSeS-COOH and ZnCuInS/ZnS-COOH-S significantly lose their fluorescence properties after abiotic dissolution in PSF, even if this is only an indicative measurement since PSF is irrelevant in the intended application. When instead comparing ZnCdSeS with ZnCuInS/ZnS and ZnCuInS/ZnS-COOH-L, different outcomes are observed by using the two methods. The WOWA approach finds high similarity between ZnCdSeS and ZnCuInS/ZnS. Interestingly, the Bayesian approach proposes a reversed outcome, well predicting the dissimilarity between ZnCdSeS and ZnCuInS/ZnS-COOH-L, but not the similarity between ZnCdSeS and ZnCuInS/ZnS. As before with dissolution kinetics, the shape of two curves (here: fluorescence spectra) can be compared in many different ways, and industrial standards of a specified colour gamut and quantum efficiency would add dimensions to a more realistic industrial SSbD decision-making. One notes that the similarity matrix of functionality is not the same as the hazard similarity matrix, and that the ranking of functionality is not the inverse of the ranking of hazard. There is hence no 1
:
1 trade-off between hazard and functionality, which also implies that an SSbD optimization is possible.
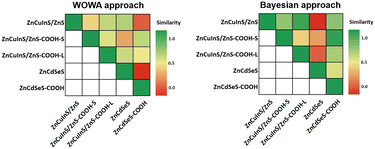 |
| Fig. 8 Similarity of functionality, represented by fluorescence spectra. | |
5. Summary and conclusions
In summary of the case of QDs, our work shows how similarity analysis and ranking can provide a perspective in terms of trade-off decisions between SSbD dimensions, exemplifying how ranking with respect to safety and functionality aspects can lead to different outcomes. On the one hand, Cd-containing QDs rank high with respect to performance (here reflected by their quantum yield). On the other hand, such Cd-containing materials, especially the one without hydrophilic polymer functionalization, show higher surface reactivity compared to the Cd-free versions. Additionally, low dissolution in lung simulant fluids suggests that these Cd-containing QDs are likely to persist in the particle form potentially bioaccumulating in the lung compartment. Both the QDs' intended functionality for use as color converters in displays and the IATA-derived screening of reactivity and leaching were assessed against each other by similarity and ranking approaches. The hazard screening included appropriate well-known reference materials (ZnO-NM110, TiO2-NM105, CuO, BaSO4 NM220) where higher tier data are available, enabling read across. As an example, the present study provides a first indication that safety measures for Cd-free QDs, which are Zn-based (ZnCuInS/ZnS, ZnCuInS/ZnS-COOH-S and ZnCuInS/ZnS-COOH-L), may be derived from adequate risk management of ZnO, for which a REACH dossier can serve as a source. Interestingly, such an assessment simply based on the cumulative OELs of the main components Cd or Zn would have suggested exactly this result. Among the different Zn-based (and thus Cd-free) QDs, there was no 1
:
1 trade-off between hazard and benefit (e.g., sustainability, functionality), which implies that an SSbD optimization is possible in this case.
In conclusion of the safe-and-sustainable-by-design perspective, the trade-offs between hazard and benefit may not always be so suggestive of the best compromise, but we maintain that the tools for similarity and ranking of both hazard-related descriptors and benefit-related descriptors are transferable to many other cases with some general caveats:
• The assessment of an MCNM by an assessment of the individual components can serve as a first guess even before synthesis of the MCNM.
• Descriptors can be adapted to multicomponent materials including MCNMs, e.g., by evaluation of the fraction of leachable mass for specific components, or by cumulative OELs scaled by the component specific leachable mass.
• The data matrix must include reference materials for the specific concern. These should be in the applicability domain of the assay and should have higher tier (in vivo) data and/or known classification with H-phrases. We anticipate that challenges may arise when transformation generates new species which then also contribute to an observed effect. Additional reference materials might have to be selected during the assessment phase by the IATA to enable comprehensive similarity analysis and ranking.
• Data matrices should be truncated to the biologically relevant range defined by controls or reference materials, such that the SSbD recommendations are not derived from irrelevant differences between the different materials.
The comparative testing by novel approach methodologies (NAMs), guided by the IATA, can then inform decision making in a SSbD context, increasing the screening speed within a set of materials and avoiding unnecessary animal testing. If approaching market launch, regulatory dossiers will require NAM-animal translation, the quality of which still needs to be improved. During early product development stages (i.e., ideation to lab phase), the materials to screen are many and the budget is often low: grouping, pairwise similarity and ranking are valuable tools for innovators, which help to discriminate the option with the best balance of functionality, safety, and sustainability. We anticipate that the present concepts are also transferable to the early screening for lifecycle-induced releases, provided that the relevant stress can be simulated and provided that suitable reference materials exist. For example, for advanced plastic additives, existing regulation suggests this approach by specifying reference materials (leachable tin-organic additives) in the Medical Device Directive. Materials that may undergo other ways of degradation, e.g., enzymatic, UV, will require other approaches as relevant for the assessment.
However, different similarity approaches may lead to different similarity outcomes, as they can be based on different algorithms and the data input can be driven by expert knowledge. The best approach will have to be carefully selected based on the available data set. Based on the collected experience, both WOWA and Bayesian computational tools will be made available in the SUNSHINE e-Infrastructure so as to allow for user-friendly similarity assessment.
Concepts identified in this work will be transferred to other case studies within the NMBP-16 projects (HARMLESS, SUNSHINE and DIAGONAL) for further testing and validation. Within HARMLESS specifically, we translate these concepts to perovskites for automotive catalysis, which are also inherently multicomponent but are characterized by different structural features and larger sizes. Within SUNSHINE, multicomponent advanced nanomaterials for paints will be assessed. Screening methods may vary from case study to case study, because of different concerns, but they share the same challenge of evaluating multicomponent advanced materials.
Author contributions
Veronica Di Battista: conceptualization; formal analysis; investigation; methodology; visualization; writing—original draft. Karla R. Sanchez-Lievanos: investigation; methodology; visualization; writing—original draft. Nina Jeliazkova: methodology; writing—review and editing. Fiona Murphy: writing—review and editing. Georgia Tsiliki: data curation; formal analysis; methodology; writing—review and editing. Alex Zabeo: data curation; formal analysis; methodology; writing—review and editing. Agnieszka Gajewicz-Skretna: data curation; formal analysis; methodology; writing—review and editing. Alicja Mikołajczyk: writing—review and editing. Danail Hristozov: writing—review and editing. Vicki Stone: writing—review and editing. Otmar Schmid: writing—review and editing. Neil Hunt: writing—review and editing. Agnes G. Oomen: writing—review and editing. Wendel Wohlleben: conceptualization; resources; supervision; writing—review and editing.
Conflicts of interest
There are no conflicts to declare.
Acknowledgements
The research for this work is a joint collaboration of the three NMBP-16 projects HARMLESS (GA no. 953183, http://www.harmless-project.eu), SUNSHINE (GA no. 952924, http://www.h2020sunshine.eu) and DIAGONAL (GA no. 953152, http://www.diagonalproject.eu). They have received funding from the European Union's Horizon 2020 research and innovation programme in the call ‘Safe by design, from science to regulation: multi-component nanomaterials’ and they address the same challenge of assessing the risk of complex multicomponent nanomaterials in different environments. We also thank Camilla Del Pivo (LEITAT), Richard Cross (UKCEH) and Keld Jensen (NRCWE) for earlier characterization of QDs in the GRACIOUS project.
Notes and references
-
ECHA, Read-Across Assessment Framework (RAAF), it can be found under https://echa.europa.eu/documents/10162/13628/introduction_to_raaf_en.pdf/00afcd9b-bd8e-4d71-9a7d-4e7b41355e01#:~:text=The%20RAAF%20increases%20transparency%20on%20how%20ECHA%20assesses,be%20met%20in%20conducting%20an%20acceptable%20read-across%20under, 2017 Search PubMed.
- V. Stone, S. Gottardo, E. A. J. Bleeker, H. Braakhuis, S. Dekkers, T. Fernandes, A. Haase, N. Hunt, D. Hristozov, P. Jantunen, N. Jeliazkova, H. Johnston, L. Lamon, F. Murphy, K. Rasmussen, H. Rauscher, A. S. Jiménez, C. Svendsen, D. Spurgeon, S. Vázquez-Campos, W. Wohlleben and A. G. Oomen, A framework for grouping and read-across of nanomaterials- supporting innovation and risk assessment, Nano Today, 2020, 35, 100941 CrossRef
.
- F. A. Murphy, H. J. Johnston, S. Dekkers, E. A. Bleeker, A. G. Oomen, T. F. Fernandes, K. Rasmussen, P. Jantunen, H. Rauscher and N. Hunt, How to formulate hypotheses and IATAs to support grouping and read-across of nanoforms, ALTEX, 2022, 125–140 Search PubMed
.
- L. Di Cristo, A. G. Oomen, S. Dekkers, C. Moore, W. Rocchia, F. Murphy, H. J. Johnston, G. Janer, A. Haase, V. Stone and S. Sabella, Grouping Hypotheses and an Integrated Approach to Testing and Assessment of Nanomaterials Following Oral Ingestion, Nanomaterials, 2021, 11, 2623 CrossRef CAS PubMed
.
- F. Murphy, S. Dekkers, H. Braakhuis, L. Ma-Hock, H. Johnston, G. Janer, L. di Cristo, S. Sabella, N. R. Jacobsen and A. G. Oomen, An integrated approach to testing and assessment of high aspect ratio nanomaterials and its application for grouping based on a common mesothelioma hazard, NanoImpact, 2021, 22, 100314 CrossRef CAS
.
- H. M. Braakhuis, F. Murphy, L. Ma-Hock, S. Dekkers, J. Keller, A. G. Oomen and V. Stone, An Integrated Approach to Testing and Assessment to Support Grouping and Read-Across of Nanomaterials After Inhalation Exposure, Appl. In Vitro Toxicol., 2021, 7, 112–128 CrossRef CAS
.
- N. Jeliazkova, L. Ma-Hock, G. Janer, H. Stratmann and W. Wohlleben, Possibilities to group nanomaterials across different substances – A case study on organic pigments, NanoImpact, 2022, 26, 100391 CrossRef CAS PubMed
.
- N. Jeliazkova, E. Bleeker, R. Cross, A. Haase, G. Janer, W. Peijnenburg, M. Pink, H. Rauscher, C. Svendsen, G. Tsiliki, A. Zabeo, D. Hristozov, V. Stone and W. Wohlleben, How can we justify grouping of nanoforms for hazard assessment? Concepts and tools to quantify similarity, NanoImpact, 2022, 25, 100366 CrossRef CAS
.
-
ECHA, Appendix R.6-1 for nanoforms applicable to the Guidance on QSARs and Grouping of Chemicals, 2019 Search PubMed.
- G. Tsiliki, D. Ag Seleci, A. Zabeo, G. Basei, D. Hristozov, N. Jeliazkova, M. Boyles, F. Murphy, W. Peijnenburg, W. Wohlleben and V. Stone, Bayesian based similarity assessment of nanomaterials to inform grouping, NanoImpact, 2022, 25, 100389 CrossRef CAS PubMed
.
- A. Zabeo, G. Basei, G. Tsiliki, W. Peijnenburg and D. Hristozov, Ordered Weighted Average Based grouping of nanomaterials with Arsinh and Dose Response similarity models, NanoImpact, 2021, 100370 Search PubMed
.
- L. M. Gilbertson, L. Pourzahedi, S. Laughton, X. Gao, J. B. Zimmerman, T. L. Theis, P. Westerhoff and G. V. Lowry, Guiding the design space for nanotechnology to advance sustainable crop production, Nat. Nanotechnol., 2020, 15, 801–810 CrossRef CAS
.
-
WBCSD, Chemical Industry Methodology for Portfolio Sustainability Assessments (PSA), it can be found at https://www.wbcsd.org/Programs/Circular-Economy/Resources/Chemical-Industry-Methodology-for-Portfolio-Sustainability-Assessments, 2018 Search PubMed.
-
EC, Safe and Sustainable by Design chemicals and materials, can be found under https://op.europa.eu/en/publication-detail/-/publication/eb0a62f3-031b-11ed-acce-01aa75ed71a1/language-en.Journal, 2022 Search PubMed.
- M. M. Falinski, D. L. Plata, S. S. Chopra, T. L. Theis, L. M. Gilbertson and J. B. Zimmerman, A framework for sustainable nanomaterial selection and design based on performance, hazard, and economic considerations, Nat. Nanotechnol., 2018, 13, 708–714 CrossRef CAS PubMed
.
- L. Rubio, G. Pyrgiotakis, J. Beltran-Huarac, Y. Zhang, J. Gaurav, G. Deloid, A. Spyrogianni, K. A. Sarosiek, D. Bello and P. Demokritou, Safer-by-design flame-sprayed silicon dioxide nanoparticles: the role of silanol content on ROS generation, surface activity and cytotoxicity, Part. Fibre Toxicol., 2019, 16, 1–15 CrossRef CAS
.
- J. K. Hedlund Orbeck and R. J. Hamers, Surface properties and interactions of transition metal oxide nanoparticles: A perspective on sustainability, J. Vac. Sci. Technol., A, 2020, 38, 031001 CrossRef CAS
.
- S. Karcher, E. L. Willighagen, J. Rumble, F. Ehrhart, C. T. Evelo, M. Fritts, S. Gaheen, S. L. Harper, M. D. Hoover and N. Jeliazkova, Integration among databases and data sets to support productive nanotechnology: Challenges and recommendations, NanoImpact, 2018, 9, 85–101 CrossRef PubMed
.
- Y. H. Ko, P. Prabhakaran, S. Choi, G. J. Kim, C. Lee and K. S. Lee, Environmentally friendly quantum-dot color filters for ultra-high-definition liquid crystal displays, Sci. Rep., 2020, 10, 15817 CrossRef CAS PubMed
.
- F. Q. Zhao, J. H. Hao and K. Wang, Cadmium-free and lead-free environment-friendly blue quantum dots and light-emitting diodes, Yejing Yu Xianshi, 2021, 36, 203–215 CAS
.
- J. C. Amaral-Júnior, A. A. P. Mansur, I. C. Carvalho and H. S. Mansur, Optically photoactive Cu–In–S@ZnS core-shell quantum dots/biopolymer sensitized TiO2 nanostructures for sunlight energy harvesting, Opt. Mater., 2021, 121, 111557 CrossRef
.
-
B. Zhang, Y. Wang, R. Hu, I. Roy and K.-T. Yong, in Handbook of Photonics for Biomedical Engineering, ed. A. H.-P. Ho, D. Kim and M. G. Somekh, Springer Netherlands, Dordrecht, 2017, pp. 841–870, DOI:10.1007/978-94-007-5052-4_7
.
-
D. A. Granada-Ramírez, J. S. Arias-Cerón, P. Rodriguez-Fragoso, F. Vázquez-Hernández, J. P. Luna-Arias, J. L. Herrera-Perez and J. G. Mendoza-Álvarez, in Nanobiomaterials, ed. R. Narayan, Woodhead Publishing, 2018, pp. 411–436, DOI:10.1016/B978-0-08-100716-7.00016-7
.
-
ER, Quantum Dots Market By Material (Cadmium-Based, Cadmium-Free), By Product (Displays, Lasers, Solar Cells, Medical Devices, Photodetectors, Others), By End User (Consumer, Commercial, Healthcare, Defense, Telecommunications) and By Regions Forecasts to 2027, it can be found under https://www.emergenresearch.com/industry-report/quantum-dots-market.Journal, 2020.
-
CDD (EU), Restricted Substances Referred to in Article 4(1) and Maximum Concentration Values Tolerated by Weight in Homogeneous Materials, Official Journal of the European Union, 2015, Annex II Search PubMed.
-
ECHA, ANNEX XVII TO REACH – Conditions of restriction, Entry 23: Cadmium, it can be found under https://echa.europa.eu/documents/10162/3bfef8a3-8c97-4d85-ae0b-ac6827de49a9, 2011.
-
A. Mnoyan, Y. Lee, H. Jung, S. Kim and D. Y. Jeon, in Phosphors, Up Conversion Nano Particles, Quantum Dots and Their Applications, ed. R.-S. Liu, Springer Singapore, Singapore, 2016, vol. 2, pp. 437–471, DOI:10.1007/978-981-10-1590-8_15
.
- D. B. Warheit and E. M. Donner, Risk assessment strategies for nanoscale and fine-sized titanium dioxide particles: Recognizing hazard and exposure issues, Food Chem. Toxicol., 2015, 85, 138–147 CrossRef CAS
.
- W. Yang, J. I. Peters and R. O. Williams, Inhaled nanoparticles—A current review, Int. J. Pharm., 2008, 356, 239–247 CrossRef CAS PubMed
.
- G. Oberdörster, J. N. Finkelstein, C. Johnston, R. Gelein, C. Cox, R. Baggs and A. C. Elder, Acute pulmonary effects of ultrafine particles in rats and mice, Res. Rep. - Health Eff. Inst., 2000, 5–74 Search PubMed
, disc. 75–86.
-
OECD, PD ISO/TR 19057:2017 - Nanotechnologies. Use and application of acellular in vitro tests and methodologies to assess nanomaterial biodurability, it can be found under http://shop.bsigroup.com/en/ProductDetail/?pid=000000000030348648, 2017.
-
OECD, GD318: Guidance Document for the testing of dissolution and dispersion stability and the use of the data for further environmental testing and assessing strategies, it can be found under https://one.oecd.org/document/ENV/JM/MONO(2020)9/en/pdf.Journal, 2020.
-
A. Gandon, K. Werle, N. Neubauer and W. Wohlleben, Surface reactivity measurements as required for grouping and read-across: An advanced FRAS protocol, IOP Publishing, 2017 Search PubMed
.
- J. G. Keller, U. M. Graham, J. Koltermann-Jülly, R. Gelein, L. Ma-Hock, R. Landsiedel, M. Wiemann, G. Oberdörster, A. Elder and W. Wohlleben, Predicting dissolution and transformation of inhaled nanoparticles in the lung using abiotic flow cells: The case of barium sulfate, Sci. Rep., 2020, 10, 458 CrossRef CAS
.
- J. Koltermann-Jülly, J. G. Keller, A. Vennemann, K. Werle, P. Müller, L. Ma-Hock, R. Landsiedel, M. Wiemann and W. Wohlleben, Abiotic dissolution rates of 24 (nano)forms of 6 substances compared to macrophage-assisted dissolution and in vivo pulmonary clearance: Grouping by biodissolution and transformation, NanoImpact, 2018, 12, 29–41 CrossRef
.
- J. G. Keller, W. Peijnenburg, K. Werle, R. Landsiedel and W. Wohlleben, Understanding Dissolution Rates via Continuous Flow Systems with Physiologically Relevant Metal Ion Saturation in Lysosome, Nanomaterials, 2020, 10, 311 CrossRef CAS
.
- W. J. G. M. Peijnenburg, E. Ruggiero, M. Boyles, F. Murphy, V. Stone, D. A. Elam, K. Werle and W. Wohlleben, A Method to Assess the Relevance of Nanomaterial Dissolution during Reactivity Testing, Materials, 2020, 13, 2235 CrossRef CAS PubMed
.
-
N. Jeliazkova, Browser-based similarity analysis by Euclidean and x-fold algorithms, https://enanomapper.adma.ai/projects/gracious/similarity, 2023 Search PubMed
.
- D. Ag Seleci, G. Tsiliki, K. Werle, D. A. Elam, O. Okpowe, K. Seidel, X. Bi, P. Westerhoff, E. Innes, M. Boyles, M. Miller, A. Giusti, F. Murphy, A. Haase, V. Stone and W. Wohlleben, Determining nanoform similarity via assessment of surface reactivity by abiotic and in vitro assays, NanoImpact, 2022, 26, 100390 CrossRef CAS PubMed
.
- L. Di Cristo, V. C. Ude, G. Tsiliki, G. Tatulli, A. Romaldini, F. Murphy, W. Wohlleben, A. G. Oomen, P. P. Pompa and J. Arts, Grouping of orally ingested silica nanomaterials via use of an integrated approach to testing and assessment to streamline risk assessment, Part. Fibre Toxicol., 2022, 19, 1–26 CrossRef
.
- A. Z. Georgia Tsiliki, V. Stone and D. Hristozov, A similarity assessment method for multicomponent nanomaterials, Environ. Sci.: Nano, 2023 Search PubMed
, under submission.
- V. Torra, The weighted OWA operator, Int. J. Intell. Syst., 1997, 12, 153–166 CrossRef
.
-
G. Basei, A. Zabeo and D. Hristozov, A tool for the Dose-Response and Benchmark Dose analysis of (eco)toxicological data: Simple BMD, 2023, Under submission Search PubMed.
- J. Lin, Divergence measures based on the Shannon entropy, IEEE Trans. Inf. Theory, 1991, 37, 145–151 CrossRef
.
- G. T. Alex Zabeo, V. Stone, W. Wohlleben and D. Hristozov, WOWA Based similarity of Multi-component nanomaterials, Environ. Sci.: Nano, 2023 Search PubMed
, under submission.
- H. L. Karlsson, N. S. Vallabani, X. Wang, M. Assenhöj, S. Ljunggren, H. Karlsson and I. Odnevall, Health hazards of particles in additive manufacturing: a cross-disciplinary study on reactivity, toxicity and occupational exposure to two nickel-based alloys, Sci. Rep., 2023, 13, 20846 CrossRef CAS PubMed
.
- J. Keller, M. Persson, P. Müller, L. Ma-Hock, K. Werle, J. Arts, R. Landsiedel and W. Wohlleben, Variation in dissolution behavior among different nanoforms and its implication for grouping approaches in inhalation toxicity, NanoImpact, 2021, 100341 CrossRef CAS
.
-
W. Wohlleben, C. Punckt, J. Aghassi-Hagmann, F. Siebers, F. Menzel, D. Esken, C.-P. Drexel, H. Zoz, H. U. Benz, A. Weier, M. Hitzler, A. I. Schäfer, L. D. Cola and E. A. Prasetyanto, in Metrology and Standardization for Nanotechnology: Protocols and Industrial Innovations, ed. E. Mansfield, D. L. Kaiser, D. Fujita and M. Van de Voorde, John Wiley & Sons, 2017, pp. 411–464 Search PubMed
.
- J. S. Steckel, J. Ho, C. Hamilton, J. Xi, C. Breen, W. Liu, P. Allen and S. Coe-Sullivan, Quantum dots: The ultimate down-conversion material for LCD displays, J. Soc. Inf. Disp., 2015, 23, 294–305 CrossRef CAS
.
- J. Liu, J. Katahara, G. Li, S. Coe-Sullivan and R. H. Hurt, End-of-life environmental impact of consumer nanocomposites - a case study on quantum dot lighting, Environ. Sci. Technol., 2012, 46, 3220–3227 CrossRef CAS
.
- S. Coe-Sullivan, Optoelectronics: Quantum dot developments, Nat. Photonics, 2009, 3, 315–316 CrossRef CAS
.
- L. M. Stabryla, P. J. Moncure, J. E. Millstone and L. M. Gilbertson, Particle-Driven Effects at the Bacteria Interface: A Nanosilver Investigation of Particle Shape and Dose Metric, ACS Appl. Mater. Interfaces, 2023, 15, 39027–39038 CrossRef CAS
.
- W. J. Peijnenburg, E. Ruggiero, M. Boyles, F. Murphy, V. Stone, D. A. Elam, K. Werle and W. Wohlleben, A Method to Assess the Relevance of Nanomaterial Dissolution during Reactivity Testing, Materials, 2020, 13, 2235 CrossRef CAS PubMed
.
- O. Schmid and T. Stoeger, Surface area is the biologically most effective dose metric for acute nanoparticle toxicity in the lung, J. Aerosol Sci., 2016, 99, 133–143 CrossRef CAS
.
- P. H. Danielsen, K. B. Knudsen, J. Štrancar, P. Umek, T. Koklič, M. Garvas, E. Vanhala, S. Savukoski, Y. Ding and A. M. Madsen, Effects of physicochemical properties of TiO2 nanomaterials for pulmonary inflammation, acute phase response and alveolar proteinosis in intratracheally exposed mice, Toxicol. Appl. Pharmacol., 2020, 386, 114830 CrossRef
.
- F. Cosnier, C. Seidel, S. Valentino, O. Schmid, S. Bau, U. Vogel, J. Devoy and L. Gaté, Retained particle surface area dose drives inflammation in rat lungs following acute, subacute, and subchronic inhalation of nanomaterials, Part. Fibre Toxicol., 2021, 18, 1–21 CrossRef
.
- K. E. Driscoll and P. J. Borm, Expert workshop on the hazards and risks of poorly soluble low toxicity particles, Inhalation Toxicol., 2020, 32, 53–62 CrossRef CAS
.
-
EU, Methodology for derivation of occupational exposure limits of chemical agents : the General Decision-Making Framework of the Scientific Committee on Occupational Exposure Limits (SCOEL) 2017, 2018.
-
ECHA, Assessing chemicals in groups: faster action on harmful chemicals, can be found under https://open.spotify.com/episode/5XCT4CdDaKpvp9BgVTfnfk?si=_BJ806V4Ryy0O19BGt7eyw&nd=1, 2021.
- W. Stark, P. Stoessel, W. Wohlleben and A. Hafner, Industrial applications of nanoparticles, Chem. Soc. Rev., 2015, 44, 5793–5805 RSC
.
|
This journal is © The Royal Society of Chemistry 2024 |
Click here to see how this site uses Cookies. View our privacy policy here.