DOI:
10.1039/D3LC00015J
(Paper)
Lab Chip, 2023,
23, 2029-2038
Towards an active droplet-based microfluidic platform for programmable fluid handling†
Received
5th January 2023
, Accepted 27th March 2023
First published on 27th March 2023
Abstract
Droplet-based microfluidic systems have emerged as powerful alternatives to conventional high throughput screening platforms, due to their operational flexibility, high-throughput nature and ability to efficiently process small fluid volumes. However, the challenges associated with performing bespoke operations on user-defined droplets often limit their utility in screening applications that involve complex workflows. To this end, the marriage of droplet- and valve-based microfluidic technologies offers the prospect of balancing the controllability of droplet manipulations and analytical throughput. In this spirit, we present a microfluidic platform that combines the capabilities of integrated microvalve technology with droplet-based sample compartmentalization to realize a highly adaptable programmable fluid handling functionality. The microfluidic device consists of a programmable formulator linked to an automated droplet generation device and storage array. The formulator leverages multiple inputs coupled to a mixing ring to produce combinatorial solution mixtures, with a peristaltic pump enabling titration of reagents into the ring with picoliter resolution. The platform allows for the execution of user-defined reaction protocols within an array of storage chambers by consecutively merging programmable sequences of pL-volume droplets containing specified reagents. The precision in formulating solutions with small differences in concentration is perfectly suited for the accurate estimation of kinetic parameters. The utility of our platform is showcased through the performance of enzymatic kinetic measurements of beta-galactosidase and horseradish peroxidase with fluorogenic substrates. The presented platform provides for a range of automated manipulations and paves the way for a more diverse range of droplet-based biological experiments.
Introduction
Over the last three decades, microfluidic systems have emerged as exceptional tools for the investigation of a range of chemical and biological processes. Their ability to manipulate small sample volumes (on the fL–μL scale) is accompanied by a number of additional advantages for biological experimentation including enhanced mass and heat transport, improved spatiotemporal control of reagents, small instrumental footprints and low unit costs.1 Significantly, the accessibility of soft lithographic fabrication methods has transformed the ease with which complex microfluidic systems may be fabricated, and enabled the manufacturing of a range of functional fluidic components.2 Most notably, integrated pneumatic valves may be fabricated in large numbers and used to control fluid flow in a rapid and precise manner.3 These valves can be used to control reagent flows through serially connected chambers, with a view to performing complex experimental workflows.4 Perhaps, the most compelling advantage associated with such “programmable” microfluidic platforms is the ability to control fluid flow when multiple operations are performed in a rapid or simultaneous fashion. Indeed, the development of programmable microfluidic systems that can be configured by the end-user to perform a variety of fluid-handling protocols will almost certainly enhance the pervasiveness of microfluidic systems in biological research.
Despite the many benefits of working in low Reynolds number (or laminar flow) environments, efficient mixing of fluids in continuous-flow systems can often be challenging.5 Additionally, when multiplexing functional components (to enhance analytical throughput), the footprint, system complexity and required control architecture increase significantly, with cross-contamination becoming an ever-present issue.6 In contrast, droplet-based microfluidic technologies possess a number of features that make them useful in a range of scenarios. In these systems, small amounts of reagents can be compartmentalized into pL-volume droplets within an immiscible carrier fluid, with the formed compartments acting as isolated vessels that can be further manipulated, processed and analysed. In addition, droplet-based microfluidic systems offer additional attractive features, such as the ability to minimise Taylor dispersion and prevent microchannel fouling. To date, the vast majority of droplet-based systems reported in the literature have focused on the formation of water-in-oil droplets, where water forms the discrete phase and an immiscible oil acts as the continuous phase, enveloping the discrete phase and wetting the microchannel surface. The ability to generate monodisperse droplets at kHz frequencies allows enormous numbers of experiments to be performed per unit time and significantly aids in the performance of kinetic analyses7,8 and screening experiments.9–12 After their generation, droplets can be manipulated in a number of ways, with operations such as droplet splitting,13 fusion,14,15 sorting,16 and trapping17,18 being simple to implement within chip-based formats. In addition, more exotic approaches can be used to manipulate droplets. For example, different sized droplets can be either confined in asymmetric traps that can induce merging of pairs of droplets having different contents19 or used as logic circuits.20 The almost unlimited number of droplets that can be generated from a single device and the high speed at which droplet operations can be executed means that droplet-based microfluidic systems operate at exceptional throughput. This feature has been exploited to perform a range of challenging experiments, including digital PCR,21,22 single cell sequencing,23,24 directed evolution25 and high-throughput single-cell analysis.26,27
Despite their utility in biological experimentation, multi-step manipulations in droplet-based microfluidic devices remain non-trivial since droplets are sensitive to pressure drop changes throughout the fluidic system.28 In addition, droplet-based systems are less well suited to multi-parameter screening applications, due to the limited number of inputs that can be integrated within a single platform.29 To address these issues, microvalves can be used to both isolate functional components and control droplet manipulations in a user-defined manner. The marriage of droplet-based microfluidic systems with valve-based platforms, offers the possibility of balancing controllability and analytical throughput, where specific flow paths can be controlled and programmed via the strategic placement of valves within a microfluidic circuit. Importantly, this ensures that the operation of an individual functional unit will not significantly influence other components, since fluidic paths are physically isolated. It should be noted that sample compartmentalization has previously been exploited in valve-based devices to achieve high levels of functionality. For example, this idea has been applied to a protein crystallization screen, where programmed mixtures of buffers and protein can be formulated in droplets and transported into large-cross section channels for incubation.30 Here, the combination of programmable mixing and droplet-based flows allowed for the flexible and precise formulation of sub-nL volumes of fluid and their subsequent transport to defined locations without cross-contamination. Although useful, it should be noted that once a solution was encapsulated within a droplet, it was no longer possible to add additional reagents to a droplet, making multistep reactions impossible.
The performance of multiple, connected operations within droplet-based microfluidic platforms often requires the integration of an addressable unit for storing droplets.31–33 Storage arrays are critical for long-term experimentation and also enable the observation and monitoring of droplet populations over extended periods of time. For example, Lee et al. developed a static droplet array platform, in which integrated valves can be used to control the generation and immobilization of droplets.34–36 More recently, the same group proposed a highly addressable static droplet array, comprising a “fluidic” layer, “control” layer and “block layer” to allow manipulation of individual droplets,37,38 with the “block” layer acting as a pressure resistor by closing the path of the control channels. Whilst useful, fabrication of such a three-layer chip is complex and requires relatively high pressures to close blocking valves. Accordingly, there is a need for simple and programmable droplet-based microfluidic systems able to perform complex biochemical workflows.
Enzymes catalyse biochemical reactions and modulate the physiological processes required for life. As such, the study and understanding of enzymatic processes and their associated kinetics is of broad interest. It is well known that reaction rates can be highly sensitive to small changes in the substrate concentration.39 Accordingly, proper assessment of enzyme kinetics is often determined by the ability to vary substrate concentrations in a precise manner. Manual variation of reaction parameters during screens is both complex and time-intensive, and rarely effective in generating information rich datasets. Conversely, programmable droplet-based microfluidic devices are ideally suited to performing large numbers of experiments in a robust, rapid and quantitative manner, whilst consuming only minimal amounts of sample. To ensure utility in biocatalysis, they must be able to formulate complex, user-defined reagent volumes, mix various reagents in a rapid manner, transport processed volumes to defined locations and quantify the products of any reaction. To this end, we present the development of a droplet-based microfluidic platform capable of executing user-defined reactions through the programmable formulation and combination of reagents in an array of pL-volume droplets. The platform consists of two main parts: a programmable formulator integrated with a T-junction geometry (to generate a series of droplets with precisely controlled payloads) and a droplet pairing/merging module (to initiate enzymatic reactions). We show that when using less than 1 μL of sample, the platform can formulate up to 100 droplets in a rapid and precise fashion, with dead volumes being significantly smaller (tens of nL) than those associated with syringe pump-driven methods. The utility of the platform is showcased by investigating the kinetics of two different enzymatic systems over timescales of several seconds. These experiments demonstrate the ease with which kinetic parameters can be extracted from miniscule volumes of sample and suggest a general utility of the platform in a range of biological studies.40
Materials and methods
Materials
Aqueous solutions were prepared in pH 7 phosphate buffered saline (Life Technologies, Zug, Switzerland). Horseradish peroxidase and beta-galactosidase were purchased as lyophilised powders (Merck, Wehrtenstein, Switzerland). Fluorescein di-beta-D-galactopyranoside (FDG) (Sigma-Aldrich, Buchs, Switzerland), resorufin beta-D-galactopyranoside (RBG) (Sigma-Aldrich, Buchs, Switzerland) and Amplex Red (AR) (Adipogen AG, Liestal, Switzerland) were used as fluorogenic substrates in kinetic experiments.
Microfluidic device fabrication
Since the microfluidic device used in the current work integrates features of varying height, master moulds were fabricated using multi-step resist coating and development techniques. The complete workflow is provided in Fig. S1.† In brief, microfluidic channels having semi-circular cross sections are generated by the melting and reflowing of a positive AZ 4620 photoresist (MicroChem GmbH, Ulm, Germany), whilst microfluidic channels having rectangular cross sections were fabricated using SU-8 (MicroChem, Ulm, Germany). The fluidic layer comprises 20 μm high microchannels (with either a rectangular or semi-circular cross section), a droplet storage array chamber (with a height of 80 μm) and 40 μm high channel salt electrodes. The fluidic layer mold was created by spin-coating AZ 4620 onto a silicon wafer at a speed of 3750 rpm for 40 seconds. The coated wafer was soft-baked at 126 °C for 8 minutes and then cooled to ambient temperature over a period of 10 minutes. Photolithographic patterning of the photoresist was performed using a UV-KUB 3 mask aligner (KLOE, St Mathieu de Tréviers, France), with the appropriate exposure time being calculated for an incident intensity of 400 mJ cm−2. The exposed wafer was then baked again at 105 °C. Next, the wafer was developed in an AZ300 MIF developer (MicroChem, Ulm, Germany), until the desired microstructures were visible to the naked eye. The wafer was then washed in deionized water and dried under a stream of nitrogen. Semi-circular cross section channel features were generated by melting and re-flowing the AZ 4620 photoresist.41 Here, the temperature was increased from ambient to 65 °C, heated up to 150 °C at a rate of 10 °C per hour, and then cooled to ambient temperature. The master mold for the control channel layer was fabricated in SU-8 on a standard silicon wafer and following standard lithographic procedures described elsewhere.42
A high-precision, two-photon polymerization 3D printer (Photonic Professional GT2, Nanoscribe, Eggenstein-Leopoldshafen, Germany) equipped with a 25×, NA 0.8 plan apochromat air objective lens (Zeiss, Oberkochen, Germany), was used to print the storage chamber array and electrode channels on the fluidic wafer. After printing, the wafer was developed in SU-8 developer (MicroChem, Ulm, Germany). for 12 minutes, washed in isopropanol for 5 minutes, and baked in an oven at 200 °C for 2 hours.
Silicon wafers were treated with chlorotrimethylsilane (Sigma Aldrich, Buchs, Switzerland) in a vacuum chamber for 2 hours, to inhibit the adhesion of PDMS to the silicon wafer surface. Microfluidic devices were made from polydimethylsiloxane (PDMS) (Sylgard 184, Dow Corning, Dayton, USA). The fluidic layer was made using a 5
:
1 ratio of base to crosslinker, while the control layer was made with 20
:
1 base to crosslinker. In both cases, base and crosslinker were mixed together thoroughly and then de-gassed in a vacuum desiccator for 30 minutes. The 20
:
1 mixture was spin-coated at 2600 rpm on the control wafer for a period of 40 seconds and allowed to rest at room temperature for 2 hours. The 5
:
1 mixture was poured onto the fluidic wafer and degassed. The mixture was then cured for 15 minutes at 70 °C. After curing, the fluidic channel layer was peeled off the support wafer. Individual devices were diced using a scalpel. Structured devices were carefully aligned with the control layer under a stereoscope, with trapped air bubbles being removed by the application of pressure. Once aligned, the wafer was cured again for between 4 and 5 hours at 70 °C. This ensures a strong bond between the two layers due to the diffusion of the curing reagent from the control layer into the fluidic layer. The complete device was then removed and holes punched to form outlets and inlets. The final PDMS device was plasma bonded to a glass slide after treating both surfaces in an air plasma (EMITECH K1000X, Quorum Technologies, East Sussex, United Kingdom) and kept in a 70 °C oven for between 4 and 5 hours.43
Fluidic mixing and droplet generation
All pressure control channels were prefilled with water and connected to solenoid valves (MH1, Festo Incorporated, Germany), providing a pressure of 2 bar. A pressure pump (Elveflow, Paris, France) was used to control fluid flow in the fluidic channel layer. Electrode channels were filled with a 4 M NaCl solution by application of a constant pressure of 0.5 bar.44 Copper wire (Distrelec, Nänikon, Switzerland) was soldered onto steel tubing (Distrelec, Nänikon, Switzerland) and inserted into the electrode channel ports and used to connect the electrode channels with a TREK 220 high voltage amplifier (Acal BFi, Gröbenzell, Germany).
Optical detection system
The optical detection system comprised a Nikon Ti-E inverted microscope (Nikon, Zurich, Switzerland) equipped with an x–y motorized translation stage (Mad City Labs, Madison, USA), a SpectraX LED source (Lumencor, OR, USA) and a set of excitation and emission filters (AHF, Tübingen, Germany). Fluorescence images were acquired using a ORCA-Flash 4.0 CMOS camera (Hamamatsu, Solothurn, Switzerland) and processed using MicroManager control software (University of California, USA).45
Enzymatic assays
Horseradish peroxidase (HRP) assay.
The enzymatic reaction was performed in 50 mM, pH 7.5 phosphate buffer at 22 °C. The final concentrations of HRP and H2O2 were fixed at 180 nM and 200 μM, respectively. Upon excitation at 542 nm, the reaction course was monitored using a 620/52 nm emission filter.
Beta-galactosidase (β-gal) assay.
The enzymatic reaction was performed in 100 mM, pH 8.0 phosphate buffer at 22 °C. The final concentration of β-gal was 900 nM and 200 nM when the reaction was performed using FDG and RBG as substrates respectively. The reaction was performed using either FDG or RBG as substrates. The reaction course was monitored using one of the following optical filter combinations: RBG: 542/27 nm excitation and 590/50 nm emission; FDG: 475/35 nm excitation and 530/43 nm emission.
Data processing and analysis
Data were processed using OriginPro 9.5 (OriginPro, MA, USA), with the Michaelis–Menten model being used to extract steady-state kinetic data. To analyse inhibition kinetics, we used competitive, uncompetitive and non-competitive inhibition models and global data analysis (eqn (S1)–(S3) in the ESI†). Inhibition models were compared using the Akaike Information Criterion (AIC) and the Bayesian Information Criteria (BIC) test.
Results and discussion
The formulator was designed to provide for active chemical control of an enzymatic reaction by precisely and quickly adjusting reagent concentrations in situ. The opening and closing of all fluidic channel paths on the chip were managed by 24 valves pneumatic valves. The valve-based microfluidic circuit enables the creation of droplets with user-defined payloads (Fig. 1). Since all tubing parts connected to the microfluidic inlets were prefilled, the loading volume in the current system is determined by the dimensions of the inlet channels and is on the order of a few nLs. The formulator comprises five independently addressable input channels (4 sample inputs and 1 buffer input) that are connected to a mixing ring to allow the production of combinatorial mixtures of reagents. To ensure efficient operation, mixing performance must be optimized. Valves positioned in the sample input channels can be actuated to choose the specific reagent that will be delivered into the mixing ring (Fig. 1A). The mixing ring comprises a circular channel with one inlet and one outlet (to either waste or sample). Three valves, termed “pumping valves”, are positioned in sequence and used to create a peristaltic pump that controls the amount of each reagent pumped into the mixing ring. Valves located in the mixing ring can then be used to circulate and mix the introduced fluid (Fig. S2 and S3†). Actuation of these “mixing valves” enhances mixing through rapid alternation of the flow direction. After mixing is complete, the “formulated” solution is driven towards the section where droplet generation takes place (Fig. 1A: top). Between each formulation step, the ring is washed repeatedly with buffer to remove residual fluid and potential contaminants (Movie S1†). Valve actuation allows precise metering of user-defined volumes of reagents into droplets (Fig. S3†).
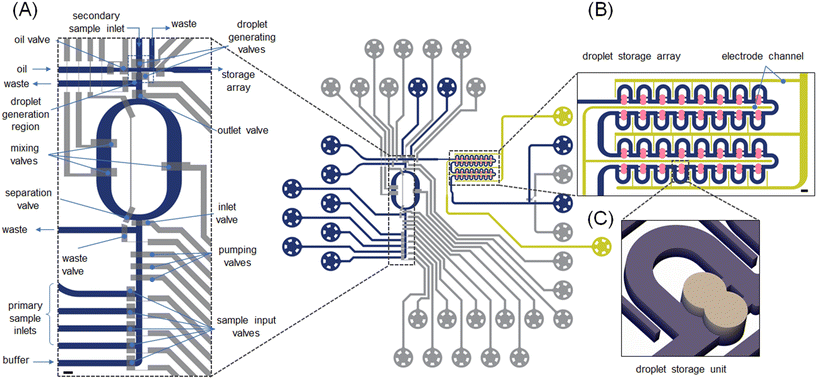 |
| Fig. 1 Microfluidic platform for programmable fluid handling. Control lines (15 μm deep, top layer) are shown in grey, fluidic lines (20 μm deep, bottom layer) are shown in blue, salt electrodes are shown in yellow and the droplet storage array is shown in ochre. (A) Enlarged view of the microfluidic formulator and droplet generation regions. Input channels for sample injection, the mixing ring and the droplet generating unit are emphasized. The fluidic circuit upstream of the mixing ring comprises one buffer and four sample inputs, which deliver bespoke fluid volumes into the mixing ring. The mixing ring integrates an inlet valve, two separation valves and one outlet valve. These allow for the introduction of fluid into the ring, rapid mixing of the contents and delivery of the formulated solution to the droplet generator. The droplet generation region comprises waste outlets, an oil inlet and two droplet generation valves and one droplet outlet channel leading to the storage array. Scale bar: 250 μm. (B) Enlarged view of the storage array consisting of fluidic lines (40 μm deep, blue), liquid salt electrodes (50 μm deep, yellow) and trapping chambers (80 μm deep, ochre) where droplet merging occurs. The droplet positioning is driven by pressure differences. Each droplet trap can accommodate two droplets, with their merging being triggered by application of an AC electric field to the salt-water electrodes. Scale bar: 100 μm. | |
The design of the droplet generator (Fig. S4†) allows the generation of two droplets from different inputs. One droplet is formed from the formulated fluid, with a second droplet being formed from the “secondary sample inlet” (Fig. 1A). Here, the secondary sample inlet is used to introduce additional reagents, such as an enzyme substrate, into the system. The droplet pairs are then transported to the droplet storage array (Fig. 1B and C) where merging can occur. These droplets can be delivered to any chamber within the storage array (4 rows consisting of 8 merging chambers each). This means that up to 32 completely different conditions can be probed during each experiment. The microfluidic channels in the storage array have a height of 30 μm and thus contained droplets adopt an allantoid shape. Conversely, the chambers in the storage array are 80 μm high and thus droplets adopt a spherical shape, which promotes trapping. Each chamber is sequentially populated by a droplet pair until all 32 chambers are fully occupied (Fig. S5†). Merging of the contained droplet pairs, and thus reaction initiation, is affected by the application of an electric field (as discussed later) across the droplet interface.
As noted, to pump reagents into the mixing ring, sample input valves, the ring inlet valve and the outlet valve are opened, whilst the pumping valves cycle through a user defined number of periods. To evaluate the accuracy and precision of fluid delivery into the mixing ring, we first determined the exact volume of a single injection (performed by a pumping valve sequence). A single injection sequence controls the minimum volume that can be pumped into the ring. Formulator performance was assessed by performing serial 200 pL additions of a FITC solution into the ring and measuring the average fluorescence intensity in the region marked by the blue rectangle in Fig. 2A after mixing (Fig. S6, Movie S1†). As can be observed, the concentration of FITC in the mixing ring increases in a quasi-linear fashion with the number of injection sequences (Fig. 2B), with no more than 70 injection sequences being used in a single experiment. The robustness of the injection sequences was further verified by preparing a dilution series of two fluorescent dye (Resorufin and FITC) solutions and forming droplets with user-defined payloads. Calibration curves reporting fluorescence from the formed droplets are shown in Fig. S7.†
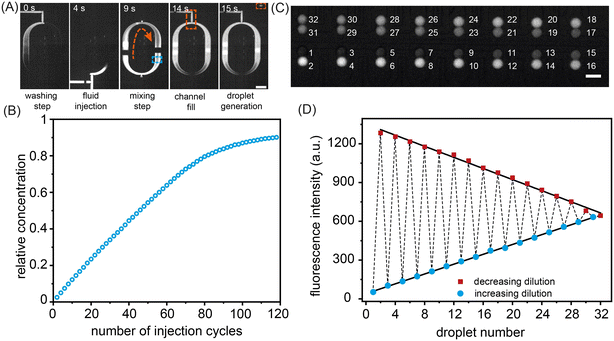 |
| Fig. 2 Device operation and injection cycle characterization. (A) Operation of the mixing ring. Prior to the injection of the target compound, the ring is first washed with buffer solution. After injection, mixing of the ring contents is achieved by periodic actuation of the mixing valves. The fully mixed fluid is then delivered to the droplet generation region, where the droplet generating valve controls droplet formation. Scale bar: 500 μm. (B) Ring injection calibration. Variation of relative concentration as a function of the number of injection cycles. Data indicate a quasi-linear increase in fluorescence intensity over the first 70 injections. The black line represents a linear fit to the data for the first 70 injection cycles. Scale bar: 100 μm. (C) Formation of a combinatorial droplet array. Fluorescence image of the storage array filled with 32 droplets containing FITC at varying concentrations. The numbers report the order in which droplets were loaded. Scale bar: 100 μm. (D) Extracted fluorescence intensities from the droplet storage array shown in (C). Droplets containing increasing and decreasing FITC concentrations are displayed as circles and rectangles, respectively. The dashed line indicates the order of droplet loading in the storage array. The linearity of both fits confirms the precision of the formulation process. | |
Fig. 2C shows a color gradient formed in the storage array through the capture of 32 sets of droplet pairs. Here, each droplet contains a specific FITC concentration formulated in the mixing ring. Each solution type is introduced and arrayed, with the contents of droplets within each storage chamber being decoded prior to merging (Fig. 2D). The linearity of the extracted fluorescence intensities from the droplet array droplets demonstrates the excellent droplet metering capabilities of the system. Next, the efficiency of droplet fusion within the storage chambers was evaluated (Fig. 3). The microwell structure of each storage chamber provides a simple route for assembling reagent combinations through droplet merging (Fig. 3A). Droplet fusion is facilitated by liquid salt electrodes (two distinct channels filled with a 4 M NaCl solution) located next to the merging chambers (Fig. 3B, Movie S2†). Careful positioning of the electrodes ensures both robust droplet fusion and rapid mixing of droplet contents after merging. As can be seen in Fig. S8,† the application of a 10 kHz, square voltage waveform (pulse duration: 10 ms), to the salt electrodes proved well-suited for droplet merging and significantly more robust than an application of a similar sinusoidal voltage waveform. Measurement of fluorescence from droplets prior to and after merging indicates rapid and efficient mixing of droplet contents within 600 ms (Fig. 3C, Movie S3†).
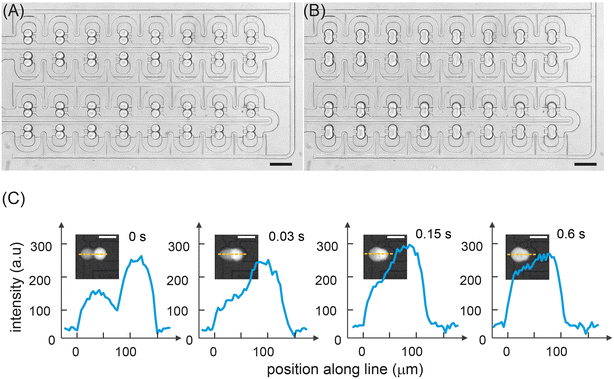 |
| Fig. 3 Droplet storage and fusion. (A) Brightfield image of the storage array filled with droplets prior to merging. (B) Brightfield image of the same array immediately after droplet merging. Scale bars: 200 μm. (C) Fluorescence images and line scans across the long axis of a droplet pair as a function of time after the application of a 1 kHz, square voltage waveform to the salt electrodes. Mixing is assessed to be completed 600 ms after droplet merging has occurred. | |
Enzyme kinetics and inhibition study
To demonstrate the suitability of our platform for the study of enzyme kinetics, we performed either 16 or 32 parallel catalysed reactions under varying substrate concentrations for two model enzymes: horseradish peroxidase (HRP) and beta-galactosidase (β-gal). HRP was chosen as a model enzyme since its structure and kinetic parameters have been thoroughly investigated,46 and it is used in a variety of biosensing47 and biotechnological applications.48 β-gal is an exoglycosidase widely used as a reporter marker in gene expression studies.49 For reactions involving HRP, Amplex Red (AR) was used as the substrate, whilst in the case of β-gal, resorufin-b-D-galactopyranoside (RBG) and fluorescein di-b-D-galactopyranoside (FDG) were chosen. In all experiments, the fluorescent intensities of substrate- and enzyme-containing droplets were measured before and after merging. Each merged droplet pair has a specific chemical composition and thus can be considered as an independent experiment. It should also be noted that the sampling rate and mixing dead-time (approximately 600 ms) did not allow the monitoring of fast pre-steady-state kinetics. We performed each measurement three times, with the extracted kinetic parameters representing the standard deviation of the mean (Fig. S9†).
First, we measured the steady-state kinetics of HRP towards AR. Here, the reaction converts the non-fluorescent AR to the highly fluorescent resorufin, with H2O2 acting as a co-substrate. Monitoring the formation rate of resorufin as a function of AR concentration provides detailed information on the catalytic behaviour of HRP.50 To extract kinetic parameters, the rates of 16 HRP-catalysed reactions were measured using various (between 5.8 μM and 155.2 μM) substrate concentrations (Fig. 4). Specifically, storage chambers were loaded sequentially and in a way that ensured the robust production of enzyme and substrate droplet pairs. As noted, in each droplet pair the droplet containing substrate is produced at a (variable) concentration dictated by the mixing ring, with the droplet containing enzyme being produced at a fixed enzyme concentration of 180 nM. Droplet pairs are then trapped and merged, and time-lapse fluorescence images of all droplets in the storage array are acquired, as shown in Fig. 4A. For each merged droplet pair, a signal trace was then generated by measuring fluorescence emission over a 25 × 25 pixel area for the desired time interval, with the increase in the fluorescence intensity being directly proportional to the amount of product formed (Fig. 4B). Next, the recorded signal traces were converted to report Resorufin concentration (Fig. S7†) and initial reaction velocities, Vapp, extracted. As expected, an increase in AR concentration leads to a larger initial reaction velocity (Fig. 4C). Fitting initial reaction velocity data to the Michaelis–Menten model, yields a Km of 20 ± 1.8 μM and a kcat of 280 ± 1.6 s−1. These values are in broad agreement with previously determined kinetic parameters obtained from bulk measurements, i.e. Km = 80 μM and kcat = 240 s−1.51
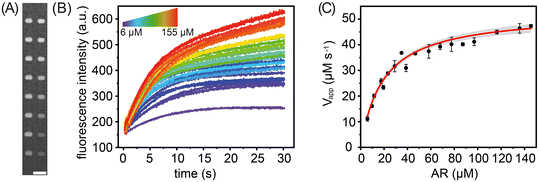 |
| Fig. 4 Horseradish peroxidase steady-state kinetics towards Amplex Red. (A) Fluorescence image of 16 merged (droplet) reaction pairs held within the storage array. Differences in the fluorescence intensity report variations in substrate concentration. Scale bar: 200 μm. (B) Extracted kinetic traces for substrate concentration ranging from 6 to 155 μM. Each kinetic trace is fitted with an exponential curve to extract the reaction velocities. (C) Michaelis–Menten plot for horseradish peroxidase towards AR. The solid line represents a hyperbolic fit to the data, with the grey shading reporting a 95% confidence interval. | |
We next investigated the steady-state kinetics of beta-galactosidase using the non-fluorescent substrate FDG (Fig. 5A and B). FDG comprises a fluorophore with two covalently attached D-galactose molecules. Enzymatic cleavage of the glycosidic bond results in a fluorescence signal increase that reports reaction progress (Fig. 5B).52 FDG transforms through a non-fluorescent intermediate where one D-galactose molecule is removed. It has been demonstrated that intermediate formation leads to an initial short lag phase in the signal formation. In the current study, substrate concentrations were varied between 4 μM and 70 μM and the enzyme concentration fixed at 900 nM. The obtained kinetic parameters, Km = 19.8 ± 1.4 μM and kcat = 20.1 ± 0.7 s−1, are again in good agreement with the literature, i.e. Km = 18 μM and kcat = 17.1 s−1.52 Additionally, we measured the steady-state kinetics of beta-galactosidase towards RBG (Fig. S10†), obtaining a Km of 408 ± 140.5 μM and a kcat value of 61 ± 3.0 s−1. Both are in excellent agreement with literature values of Km = 333 ± 130 μM and kcat = 64 ± 8 s−1.53
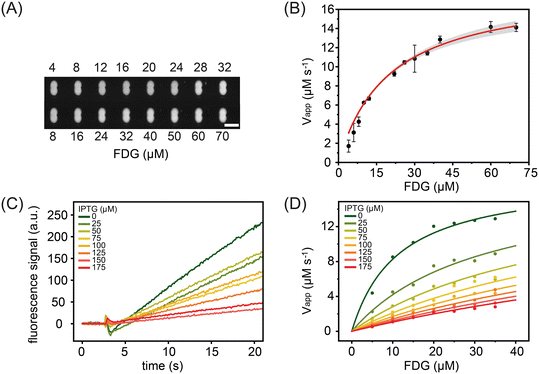 |
| Fig. 5 Beta-galactosidase kinetics and inhibition study for droplets containing the FDG as substrate and IPTG as inhibitor at varying concentrations. (A) Fluorescence image of the storage array after droplet merging indicates the progress of the enzymatic reaction. Scale bar: 100 μm. (B) Michaelis–Menten plot for beta-galactosidase towards FDG. The solid line represents a hyperbolic fit to the data, with the grey shading reporting a 95% confidence interval. (C) Kinetic traces for beta-galactosidase towards (50 μM) FDG at different IPTG inhibitor concentrations (0 μM to 175 μM). The raw traces highlight a 2 second pre-merging signal followed by a mixing and subsequent reaction. (D) A global fit to the inhibition kinetic data for inhibitor concentrations between 25 and 175.0 μM. The obtained reaction velocities for each of the seven inhibitor concentrations were used to extract the enzyme kinetics constants and the enzyme inhibitor dissociation constants. | |
In addition to measuring the steady-state parameters, Km and kcat, we also studied the behaviour of the enzymatic reaction in the presence of inhibitors. Common inhibition screening experiments aim to determine only the IC50 value (the inhibitor concentration that reduces the reaction rate by 50%). Such an approach will yield IC50 values that depend on the concentrations of the enzyme, substrate and the chemical nature of the substrate itself. Since the inhibition constant, Ki, is not affected by these parameters,54 we used our platform to determine Ki values for isopropyl β-D-1-thiogalactopyranoside (IPTG); a substrate analogue that binds to the active site of beta-galactosidase and results in competitive inhibition of enzymatic activity.47 The results of such an analysis are shown in Fig. 5C, with data being generated by recording 25 seconds of droplet fluorescence at IPTG inhibitor concentrations between 0 μM and 175 μM. Data sets were also acquired for droplets containing the enzyme solution at a fixed concentration and then paired with droplets containing FDG concentrations between 5 and 35 μM and IPTG concentrations between 0 μM and 175 μM. As shown in Fig. 5D, initial rates decrease with inhibitor concentration. A global analysis of the reaction velocities extracted at seven inhibitor concentrations was performed using competitive, non-competitive and uncompetitive inhibition models. As expected, the lowest residual values were determined when using a competitive inhibition model (Table S1†), with analysis yielding an inhibition constant, Ki, of 36 ± 7 μM, a value consistent with previous measurements that report the inhibition constant over the range of 62–80 μM.55
Conclusions
We have described the development of a new type of programmable microfluidic platforms. The system is capable of measuring enzyme kinetic parameters in an entirely automated fashion, by allowing the execution of bespoke (multistep) protocols in an array of individually addressable droplets. This is engendered through the integration of both valve-based and droplet-based microfluidic fluid handling concepts. Such an approach enables the characterization of enzymatic reaction kinetics using only a few nanoliters of reagents. Central to the programmable nature of the described workflow is the ability to generate, immobilize and merge a user defined number of droplets. This capability means that the formulation of reagent mixtures becomes independent of the volume of the droplet container. When combined with valve-based fluid handling, the droplet merging method enables the precise, user-defined, formulation of solutions containing multiple reagents in an array of pL-volume droplets. This functionality forms the basis of a programmable microfluidic processor, allowing a single device to perform numerous experiment types. We initially used the microfluidic platform to generate a sequence of droplets that form a concentration gradient within a droplet storage array to extract kinetic information. We demonstrated the efficacy of our system by extracting the Michaelis constants of horseradish peroxidase and beta-galactosidase against AR and FDG, respectively. In addition, the application of the platform to the study of enzyme inhibition confirms its suitability for the rapid and precise measurement of inhibitor action in an automated manner. Indeed, in its current form, the platform could be used in a variety of pharmacokinetic applications, such as monitoring the interaction between synergists and antagonists and their concentration dependency.56 On a more general level, the platform could be applied in other applications that more fully exploit the ability to vary the contents of each storage chamber. Whilst the current hybrid platform does not have the throughput of traditional droplet-based microfluidic experiments, it offers exceptional precision in controlling the payload of pL-volume droplets. Furthermore, it would be quite straightforward to scale-out the platform to integrate several hundred addressable chambers, without requiring extensive modifications to the control architecture.57
Author contributions
X. C., T. B., S. S. and A. dM. conceived the project and devised the research plan. X. C. and T. B. conducted the experimental work and analysed the data. T. Y., J. W., A. S. and P. F. contributed resources. SS and A. dM. wrote the manuscript.
Conflicts of interest
The authors declare no conflict of interest.
Acknowledgements
The authors acknowledge ETH Zörich for a partial financial support. The authors would like to acknowledge support from the Swiss National Science Foundation (grant number: 205321/176011/1) and S&T Program of Guangzhou Laboratory (grant number: SRPG22-020).
References
- D. T. Chiu, A. J. deMello, D. Di Carlo, P. S. Doyle, C. Hansen, R. M. Maceiczyk and R. C. R. Wootton, Chem, 2017, 2, 201–223 CAS.
- M. A. Unger, H.-P. Chou, T. Thorsen, A. Scherer and S. R. Quake, Science, 2000, 288, 113–116 CrossRef CAS PubMed.
- T. Thorsen, S. J. Maerkl and S. R. Quake, Science, 2002, 298, 580–584 CrossRef CAS PubMed.
- S. Kim, A. M. Streets, R. R. Lin, S. R. Quake, S. Weiss and D. S. Majumdar, Nat. Methods, 2011, 8, 242–245 CrossRef CAS PubMed.
- K. Ward and Z. H. Fan, J. Micromech. Microeng., 2015, 25, 094001 CrossRef PubMed.
- L. Li, J. Q. Boedicker and R. F. Ismagilov, Anal. Chem., 2007, 79, 2756–2761 CrossRef CAS PubMed.
- D. Hess, V. Dockalova, P. Kokkonen, D. Bednar, J. Damborsky, A. DeMello, Z. Prokop and S. Stavrakis, Chem, 2021, 7, 1066–1079 CAS.
- M. Vasina, P. Vanacek, J. Hon, D. Kovar, H. Faldynova, A. Kunka, T. Buryska, C. P. S. Badenhorst, S. Mazurenko, D. Bednar, S. Stavrakis, U. T. Bornscheuer, A. deMello, J. Damborsky and Z. Prokop, Chem Catal., 2022, 2, 2704–2725 CrossRef CAS.
- E. Brouzes, M. Medkova, N. Savenelli, D. Marran, M. Twardowski, J. B. Hutchison, J. M. Rothberg, D. R. Link, N. Perrimon and M. L. Samuels, Proc. Natl. Acad. Sci. U. S. A., 2009, 106, 14195–14200 CrossRef CAS PubMed.
- B. El Debs, R. Utharala, I. V. Balyasnikova, A. D. Griffiths and C. A. Merten, Proc. Natl. Acad. Sci. U. S. A., 2012, 109, 11570–11575 CrossRef CAS PubMed.
- X. Cao, Y. Du, A. Küffner, J. Van Wyk, P. Arosio, J. Wang, P. Fischer, S. Stavrakis and A. deMello, Small, 2020, 16, 1907534 CrossRef CAS PubMed.
- J. Rutkauskaite, S. Berger, S. Stavrakis, O. Dressler, J. Heyman, X. C. i Solvas, A. deMello and L. Mazutis, iScience, 2022, 25, 104515 CrossRef CAS PubMed.
- A. R. Abate and D. A. Weitz, Lab Chip, 2011, 11, 1911–1915 RSC.
- M. Zagnoni and J. M. Cooper, Lab Chip, 2009, 9, 2652–2658 RSC.
- X. Niu, S. Gulati, J. B. Edel and A. J. deMello, Lab Chip, 2008, 8, 1837–1841 RSC.
- J.-C. Baret, O. J. Miller, V. Taly, M. Ryckelynck, A. El-Harrak, L. Frenz, C. Rick, M. L. Samuels, J. B. Hutchison and J. J. Agresti, Lab Chip, 2009, 9, 1850–1858 RSC.
- A. Huebner, D. Bratton, G. Whyte, M. Yang, A. J. Demello, C. Abell and F. Hollfelder, Lab Chip, 2009, 9, 692–698 RSC.
- W. Wang, C. Yang and C. M. Li, Lab Chip, 2009, 9, 1504–1506 RSC.
- R. F. Tomasi, S. Sart, T. Champetier and C. N. Baroud, Cell Rep., 2020, 31, 107670 CrossRef CAS PubMed.
- D. Zaremba, S. Błoński and P. M. Korczyk, Lab Chip, 2021, 21, 1771–1778 RSC.
- A. S. Whale, J. F. Huggett, S. Cowen, V. Speirs, J. Shaw, S. Ellison, C. A. Foy and D. J. Scott, Nucleic Acids Res., 2012, 40, e82 CrossRef CAS PubMed.
- Y. Schaerli, R. C. Wootton, T. Robinson, V. Stein, C. Dunsby, M. A. Neil, P. M. French, A. J. DeMello, C. Abell and F. Hollfelder, Anal. Chem., 2009, 81, 302–306 CrossRef CAS PubMed.
- A. M. Klein, L. Mazutis, I. Akartuna, N. Tallapragada, A. Veres, V. Li, L. Peshkin, D. A. Weitz and M. W. Kirschner, Cell, 2015, 161, 1187–1201 CrossRef CAS PubMed.
- F. Lan, B. Demaree, N. Ahmed and A. R. Abate, Nat. Biotechnol., 2017, 35, 640–646 CrossRef CAS PubMed.
- Y. Liu, L. Sun, H. Zhang, L. Shang and Y. Zhao, Chem. Rev., 2021, 121, 7468–7529 CrossRef CAS PubMed.
- L. Mazutis, J. Gilbert, W. L. Ung, D. A. Weitz, A. D. Griffiths and J. A. Heyman, Nat. Protoc., 2013, 8, 870–891 CrossRef CAS PubMed.
- E. M. Payne, D. A. Holland-Moritz, S. Sun and R. T. Kennedy, Lab Chip, 2020, 20, 2247–2262 RSC.
- L. Mazutis, J.-C. Baret, P. Treacy, Y. Skhiri, A. F. Araghi, M. Ryckelynck, V. Taly and A. D. Griffiths, Lab Chip, 2009, 9, 2902–2908 RSC.
- J.-C. Chang, Z. Swank, O. Keiser, S. J. Maerkl and E. Amstad, Sci. Rep., 2018, 8, 1–9 Search PubMed.
- B. T. Lau, C. A. Baitz, X. P. Dong and C. L. Hansen, J. Am. Chem. Soc., 2007, 129, 454–455 CrossRef CAS PubMed.
- M. Sun, S. S. Bithi and S. A. Vanapalli, Lab Chip, 2011, 11, 3949–3952 RSC.
- W. Shi, J. Qin, N. Ye and B. Lin, Lab Chip, 2008, 8, 1432–1435 RSC.
- M. Duchamp, M. Arnaud, S. Bobisse, G. Coukos, A. Harari and P. Renaud, Micromachines, 2021, 12, 1076 CrossRef PubMed.
- H.-H. Jeong, S. H. Jin, B. J. Lee, T. Kim and C.-S. Lee, Lab Chip, 2015, 15, 889–899 RSC.
- B. Lee, S. H. Jin, Y.-M. Noh, S.-G. Jeong, H.-H. Jeong and C.-S. Lee, Sens. Actuators, B, 2018, 273, 1572–1578 CrossRef CAS.
- S. H. Jin, S. S. Lee, B. Lee, S.-G. Jeong, M. Peter and C.-S. Lee, Anal. Chem., 2017, 89, 9722–9729 CrossRef CAS PubMed.
- S. Jang, B. Lee, H.-H. Jeong, S. H. Jin, S. Jang, S. G. Kim, G. Y. Jung and C.-S. Lee, Lab Chip, 2016, 16, 1909–1916 RSC.
- H.-H. Jeong, B. Lee, S. H. Jin, S.-G. Jeong and C.-S. Lee, Lab Chip, 2016, 16, 1698–1707 RSC.
- A. G. McDonald and K. F. Tipton, Molecules, 2022, 27, 263 CrossRef CAS PubMed.
- O. Kirk, T. V. Borchert and C. C. Fuglsang, Curr. Opin. Biotechnol., 2002, 13, 345–351 CrossRef CAS PubMed.
- F. Volpetti, J. Garcia-Cordero and S. J. Maerkl, PLoS One, 2015, 10, e0117744 CrossRef PubMed.
- J. Probst, C. N. Borca, M. A. Newton, J. van Bokhoven, T. Huthwelker, S. Stavrakis and A. deMello, ACS Meas. Sci. Au, 2021, 1, 27–34 CrossRef CAS PubMed.
- D. Qin, Y. Xia and G. M. Whitesides, Nat. Protoc., 2010, 5, 491 CrossRef CAS PubMed.
- A. Sciambi and A. R. Abate, Lab Chip, 2014, 14, 2605–2609 RSC.
- A. Edelstein, N. Amodaj, K. Hoover, R. Vale and N. Stuurman, Curr. Protoc. Mol. Biol., 2010, 92, 14.20.11–14.20.17 Search PubMed.
- P. Baumann, M. Spulber, O. Fischer, A. Car and W. Meier, Small, 2017, 13, 1603943 CrossRef PubMed.
- A. C. Patel, S. Li, J.-M. Yuan and Y. Wei, Nano Lett., 2006, 6, 1042–1046 CrossRef CAS PubMed.
- A. M. Azevedo, V. C. Martins, D. M. Prazeres, V. Vojinovic, J. M. Cabral and L. P. Fonseca, Biotechnol. Annu. Rev., 2003, 9, 1387–2656 Search PubMed.
- D. Anson and M. Limberis, J. Biotechnol., 2004, 108, 17–30 CrossRef CAS PubMed.
- G. H. Seong, J. Heo and R. M. Crooks, Anal. Chem., 2003, 75, 3161–3167 CrossRef CAS PubMed.
- M. Glettenberg and C. M. Niemeyer, Bioconjugate Chem., 2009, 20, 969–975 CrossRef CAS PubMed.
- Z. Huang, Biochemistry, 1991, 30, 8535–8540 CrossRef CAS PubMed.
- Y. Xie, D. Ahmed, M. I. Lapsley, S.-C. S. Lin, A. A. Nawaz, L. Wang and T. J. Huang, Anal. Chem., 2012, 84, 7495–7501 CrossRef CAS PubMed.
- C. Yung-Chi and W. H. Prusoff, Biochem. Pharmacol., 1973, 22, 3099–3108 CrossRef PubMed.
- D. H. Juers, B. Rob, M. L. Dugdale, N. Rahimzadeh, C. Giang, M. Lee, B. W. Matthews and R. E. Huber, Protein Sci., 2009, 18, 1281–1292 CrossRef CAS PubMed.
- M. B. Colovic, D. Z. Krstic, T. D. Lazarevic-Pasti, A. M. Bondzic and V. M. Vasic, Curr. Neuropharmacol., 2013, 11, 315–335 CrossRef CAS PubMed.
- C. L. Hansen, E. Skordalakes, J. M. Berger and S. R. Quake, Proc. Natl. Acad. Sci. U. S. A., 2002, 99, 16531–16536 CrossRef CAS PubMed.
|
This journal is © The Royal Society of Chemistry 2023 |
Click here to see how this site uses Cookies. View our privacy policy here.