DOI:
10.1039/D0BM01153C
(Paper)
Biomater. Sci., 2021,
9, 1272-1290
Development of a stacked, porous silk scaffold neuroblastoma model for investigating spatial differences in cell and drug responsiveness†
Received
12th July 2020
, Accepted 19th November 2020
First published on 24th November 2020
Abstract
Development of in vitro, preclinical cancer models that contain cell-driven microenvironments remains a challenge. Engineering of millimeter-scale, in vitro tumor models with spatially distinct regions that can be independently assessed to study tumor microenvironments has been limited. Here, we report the use of porous silk scaffolds to generate a high cell density neuroblastoma (NB) model that can spatially recapitulate changes resulting from cell and diffusion driven changes. Using COMSOL modeling, a scaffold holder design that facilitates stacking of thin, 200 μm silk scaffolds into a thick, bulk millimeter-scale tumor model (2, 4, 6, and 8 stacked scaffolds) and supports cell-driven oxygen gradients was developed. Cell-driven oxygen gradients were confirmed through pimonidazole staining. Post-culture, the stacked scaffolds were separated for analysis on a layer-by-layer basis. The analysis of each scaffold layer demonstrated decreasing DNA and increasing expression of hypoxia related genes (VEGF, CAIX, and GLUT1) from the exterior scaffolds to the interior scaffolds. Furthermore, the expression of hypoxia related genes at the interior of the stacks was comparable to that of a single scaffold cultured under 1% O2 and at the exterior of the stacks was comparable to that of a single scaffold cultured under 21% O2. The four-stack scaffold model underwent further evaluation to determine if a hypoxia activated drug, tirapazamine, induced reduced cell viability within the internal stacks (region of reduced oxygen) as compared with the external stacks. Decreased DNA content was observed in the internal stacks as compared to the external stacks when treated with tirapazamine, which suggests the internal scaffold stacks had higher levels of hypoxia than the external scaffolds. This stacked silk scaffold system presents a method for creating a single culture model capable of generating controllable cell-driven microenvironments through different stacks that can be individually assessed and used for drug screening.
Introduction
Neuroblastoma (NB) is an orphan disease; therefore there are few preclinical in vitro models available for disease modeling. Its prevalence is 1 in 7000 live births and accounts for 15% of childhood cancer related deaths.1 Treatment for NB includes surgery, chemotherapy, radiation, and immunotherapy. However, for high-risk NB patients, the prognosis is dismal with a less than 50% long-term survival rate.2,3 Developing new treatment options for recurrent or relapsed NB may increase the survival rates for these patients. In vitro models are used to develop and identify new treatment strategies; however, these models often lack features of the tumor microenvironment, such as a three-dimensional structure which results in regional differences in the cell phenotype, pH, oxygen tension, and nutrient access. The existing models used for initial drug screening provide many benefits such as rapid, high-throughput testing, but often result in identifying drug candidates that fail to translate to clinical treatment strategies.
Many solid tumors demonstrate regions of reduced oxygen tension, otherwise known as hypoxia, ranging from 0.3% to 4.2% O2.4–9 In tumors, rapid proliferation and high levels of oxygen consumption lead to depleted oxygen levels, particularly in cells far from the vasculature.10 Rapid growth in tumors without adequate vasculature leads to gradients of oxygen, nutrients, and pH.11 Cancer cells that are under hypoxic stress have an altered metabolic pathway, relying on glycolysis instead of mitochondrial respiration (Warburg effect).4,5,12 Hypoxic stress has also been shown to cause adaptations in tumor cells that promote a more aggressive tumor phenotype.13,14 These changes include the promotion of migration, invasion, resistance to apoptosis, and increased angiogenesis.6,7,15–17 These changes are driven, in part, by hypoxia-inducible factor-1 (HIF-1), a transcription factor that responds to low oxygen conditions. Additionally, hypoxia induces resistance to many anti-cancer drugs through HIF-1-dependent and independent mechanisms.18
Current NB hypoxia models rely on chemical induction of hypoxia using dimethyloxallyl glycine (DMOG: a hypoxia inducible factor-1, or HIF-1, activator), CoCl2 (mimics HIF-1 activation), or environmentally controlled incubators.19 In NB cell lines, the use of low oxygen incubators and DMOG has been shown to confer important pathway changes, which persisted when cells were implanted in vivo.20 However, low oxygen incubators and DMOG are primarily useful in identifying cellular changes at the molecular level. In tumors, hypoxia is cell-driven, existing in a gradient with the highest oxygen tension close to the vasculature and lowest oxygen tension distant from the vasculature. Furthermore, cells under hypoxia can impact the surrounding tumor and stromal cells through altered paracrine signaling, extracellular matrix (ECM) deposition, and increased inflammation.21 This can have an impact on the tumor's responsiveness to therapeutics and metastatic potential. Additionally, in the tumor microenvironment hypoxia is not the only environmentally altered feature as reduced pH and access to nutrients occur often concurrently with the reduction in oxygen concentrations. Therefore, though highly controlled systems that probe the effect of hypoxia on cells are advantageous, these systems ignore nutrient deprivation and acid accumulation in comparison with a cell-driven approach to low oxygen.
Three-dimensional culture models and rodent models that utilized human cell lines or human primary tumor specimens support the combination of cell driven oxygen, nutrient, and pH changes. Many current 3D in vitro models are capable of generating hypoxic regions. The commonly used spheroid models (cell aggregates) demonstrate a hypoxic core once the tumor reaches a size greater than 200 μm.22–24 Spheroids have been used to study the therapeutic response to hypoxia as well as alterations in pathway expression.23,25 However, it is difficult to spatially control spheroid growth, and analysis of different regions of the spheroid is challenging. Hydrogel systems have also been used to model hypoxia. For example, cells can be grown within hydrogels composed of collagen, agarose, and Matrigel.26–28 These natural hydrogels have high batch–batch variability and weak mechanical properties.29 Porous scaffolds fabricated via salt-leaching, gas foaming, or lyophilization, derived from natural and synthetic polymers, have begun to address the concerns of weak mechanical properties of hydrogel-based approaches. Synthetic polymers and natural polymers with limited ability to be degraded by endogenous proteases, such as poly(ε-caprolactone), cellulose, and silk fibroin,30–33 further provide structural and mechanical integrity for in vitro cancer models. Xenograft NB models that utilize human cell lines or patient-derived specimens are advantageous for studying tumor engraftment and progression.34,35 However, rodent models are costly and working with patient-derived xenograft models requires significant infrastructure limiting their utility for evaluating many different drugs.36 Three-dimensional, in vitro NB models, generalizable to other solid tumor models as well, may be a bridge between traditional, monolayer drug screening assays that can test hundreds if not thousands of compounds in a short period of time and rodent models.
Previous in vitro systems have utilized stacking or layering for fabrication of multi-layer tumor models. Of these, the most commonly used is a paper-based method, which utilizes a combination of thin (<200 μm) filter paper embedded with cells in an ECM hydrogel.37–42 An alternative approach utilizes a thin cellulose scaffold embedded with cells in an ECM hydrogel that is subsequently rolled around a mandrel resulting in circumferential layering.43–46 These two layering approaches demonstrated controllable oxygen gradients, decreased proliferation in hypoxic regions, and have been used to spatially examine chemotherapeutic efficacy.32,37,38,42–44
Porous silk scaffolds can be easily fabricated from aqueous silk solutions via lyophilization, a simple and rapid fabrication strategy. Silk scaffolds possess the combined beneficial features of synthetic and natural materials including simple, mechanical robustness to support cell culturing, and the ability to be formulated with a spatially defined architecture.47–52 Furthermore, silk scaffolds, on their own, do not cause cell-specific signaling, but are readily modified to mimic the native biophysical and biochemical environments.53–55 In previous work, we demonstrated the use of three-dimensional silk scaffolds and low oxygen incubators to mimic different levels of oxygen tension observed within the tumor microenvironment using NB cell lines. We demonstrated changes in gene expression and cytokine secretion and elucidated whether the effects were due to growth of cells under low oxygen concentration, in 3D culture, or a combination of both conditions. This prior work demonstrated that 3D scaffolded NB cells cultured under ambient oxygen conditions (21% O2) have increased expression of markers relevant to hypoxic conditions (vascular endothelial growth factor: VEGF; carbonic anhydrase IX: CAIX; glucose transporter 1: GLUT1; and matrix metalloprotease: MMP9) as compared to monolayer cultured cells under ambient oxygen conditions, these increases were comparable to or greater than those of monolayer cultured cells under low oxygen conditions (1% O2).
In the present study, we generated 3D models using stacked silk fibroin scaffolds to create a cell driven hypoxia model. Silk fibroin has been demonstrated to be an effective material for tissue engineering due to its stability, biocompatibility, and lack of immunogenic response.56–59 While many studies have been performed to engineer 3D tumor models (both using scaffolds and in spheroid form), few have developed systems with controlled oxygen gradients. To achieve this, we utilized a stacking method, which has been previously employed to evaluate cell proliferation, invasion, and oxygen tension.32,37,38 Mathematical modeling was used to determine the impact of the scaffold holder geometry on oxygen diffusion, and the number of scaffolds on oxygen gradients with the scaffolds. Stacks of different thicknesses were evaluated for cellularity through double stranded DNA (dsDNA) quantification and histology. Hypoxic gradients were characterized through gene expression and pimonidazole staining and compared to cell-laden single scaffolds cultured under ambient and low oxygen conditions. This system has the potential to be applied to many different cancer types and allows an improved understanding of tumor pathways, while also presenting a novel therapeutic testing platform.
Materials and methods
Cell culturing
KELLY NB cells (Millipore Sigma, St Louis, MO) were maintained in Roswell Park Memorial Institute 1640 (RPMI) medium supplemented with 10% v/v fetal bovine serum, 100 U mL−1 penicillin, 100 μg mL−1 streptomycin, and 2 mM L-glutamine (Fisher Scientific, Hampton, NH) at 37 °C under 5% CO2 and 21% O2 in a humidified environment. SK-N-AS NB cells (ATCC, Manassas, VA) were maintained in Dulbecco's Modified Eagle's Medium (DMEM) supplemented with 10% v/v fetal bovine serum, 100 U mL−1 penicillin, 100 μg mL−1 streptomycin, 2 mM L-glutamine, and 0.1 mM NEAA (Fisher Scientific, Hampton, NH) at 37 °C under 5% CO2 and 21% O2 in a humidified environment. All cells were passaged using 0.25% trypsin-EDTA at 70–80% confluence. For low oxygen culture controls, a 37 °C, 5% CO2, and 1% O2 incubator was used.
Silk fibroin extraction
Silk fibroin from Bombyx mori silkworm cocoons (Tajima Shoji Co Yokohama, Japan), kindly provided by Dr David L. Kaplan at Tufts University, was extracted as previously described.58 Briefly, 5 g cocoons were cut into approximately 1 cm × 1 cm pieces and boiled for 30 min in 0.02 M Na2CO3 to extract the sericin. The silk fibers were then dried overnight. The dried silk fibroin fibers were dissolved in 9.3 M LiBr at 60 °C for 3 h. The dissolved silk fibroin was dialyzed in 3500 Da molecular weight cut-off dialysis tubing (Fisher Scientific, Hampton, NH) against ultrapure water for 2 days with a minimum of 6 water changes. The aqueous silk fibroin (referred to as silk from here on) solution was stored at 4 °C until further processing.
Silk scaffold fabrication
Silk scaffolds were fabricated using 2 mL of 5% silk solution in 15.6 mm cylindrical molds. Lyophilization was performed using rate-control freezing with a 2 h hold at −45 °C followed by initiating the vacuum at 280 mTorr and lyophilizing for 30 h at −25 °C. Secondary drying was performed with a 2 h hold at −4 °C, followed by ramping up to 20 °C. All steps before lyophilization were performed at a rate of 0.5 °C min−1, and all steps following engagement of the vacuum were performed at 1 °C min−1. To render the materials insoluble, the scaffolds were autoclaved following removal from the lyophilizer. The scaffolds were then vibratome sectioned to a thickness of 200 μm or 600 μm and biopsy punched to a diameter of 6 mm. They were sterilized via autoclaving in ultrapure water. Silk scaffolds fabricated using similar lyophilization procedures have been reported to contain interconnected porous structures that would allow gas exchange, nutrient transport and exchange of gases and nutrients and infiltration of cells.60,61 Additionally, the pore size has been reported to be dependent on silk concentration, ranging from approximately 20 μm to 190 μm.53,62,63
Seeding of cells on scaffolds
Cells cultured as described above were dissociated into a single cell suspension using 0.25% trypsin-EDTA. The cells were resuspended in media at a concentration of 100
000 cells per μL. For 200 μm thick silk scaffolds, seeding was performed by applying a volume of 5 μL of the cell suspension to a scaffold, waiting for 10 minutes, vertically inverting the scaffold and applying an additional 5 μL of the cell suspension. For 600 μm thick silk scaffolds, cell seeding was performed by applying a volume of 15 μL of the cell suspension to a scaffold, waiting for 10 minutes, vertically inverting the scaffold and applying an additional 15 μL of the cell suspension. The cell-seeded scaffolds were allowed to incubate at 37 °C, with ambient oxygen and 5% CO2 for 4 h to allow for initial cell seeding. For the experiments comparing 200 μm versus 600 μm silk scaffolds, SK-N-AS NB cells and KELLY NB cells were evaluated. One experiment was performed with enhanced green fluorescent protein (EGFP) expressing KELLY NB cells, kindly provided by Dr Sakthikumar Ambady, as a visual aid to evaluate cell distribution. The cell-seeded scaffolds were cultured in 48-well or 24-well plates, respectively, coated with agarose to minimize cell migration out of the scaffolds and attachment to the well plates. The cultures were ended after 1, 3, 7, 10, 14, 21, and 28 days and evaluated based on the DNA content and cell distribution via histology. For 200 μm thick scaffolds intended for stacked scaffold experiments, SK-N-AS NB cells were evaluated. The cell-seeded scaffolds were transferred to 6-well plates containing 3 mL of media and cultured for 3 days prior to stacking.
Stacking of SK-N-AS NB cell seeded scaffolds
After 3 days of culture in 200 μm thick single scaffolds, the scaffolds were stacked using a polytetrafluoroethylene (PTFE) scaffold holder composed of stacks of 2, 4, 6, or 8 scaffolds in 8 mL of medium. The number of stacked scaffolds was chosen based on COMSOL modeling that identified distinct oxygen concentration gradients throughout the stacked model. Though the 6 and 8 stacked scaffolds were predicted to exhibit large regions of hypoxia within the middle of the stacked scaffolds, 8 stacks were examined to test the limitations of the holder design. Utilizing more than 8 scaffolds within the designed scaffold holder was not feasible as the holder did not adequately hold the scaffolds in place based on visual observations (data not shown). As a control, single scaffolds were maintained in 6-well plates containing 3 mL of medium under both 21% O2 and 1% O2 (0.21 mol m−3 O2 and 0.01 mol m−3 O2, respectively). Stacked scaffolds were cultured on an orbital shaker at 75 rpm within 6-well plates at 37 °C under 21% O2. Prior research with an in vitro rolled layered, three-dimensional human ovarian adenocarcinoma model reported changes in solute concentration and gene expression throughout the layers as early as 6 h and 24 h post-model development as well as establishment of oxygen gradients within the model.43,44 In another stacked, paper-based tumor model, hypoxia-related changes of a breast cancer cell line were observed after 9 days of culture.37 A study comparing 2D versus 3D cultures of human colorectal carcinoma cell lines reported increased expression of hypoxia-related markers after 2 days and 6 days of culture.64 Given the range of time points previously evaluated for hypoxia related changes in 3D models, the genotype and phenotype outcomes reported here are from 3 days post-stacking experiments (a total of 6 days in scaffold culture or monolayer) with one longer-term experiment performed through 14 days to evaluate cell viability in the 4-stack model.
Cell response to a hypoxia-activated drug
Scaffolds seeded and developed into the 4-stack scaffold system, as described above, were treated with 20 μg mL−1 tirapazamine (Millipore Sigma, St Louis, MO). After the 3 days of treatment, cell viability was determined based on the DNA content. The 3 day time point was evaluated as this time point has been shown to be an effective time point for evaluating anticancer compounds using 3D NB spheroids.65
DNA content analysis
Scaffolds were disassembled (see Videos S1 and S2† for visualization of the disassembly process) and lysed with 0.5 mL of 0.5% Triton X-100 in Tris-EDTA (TE) buffer for 20 min at room temperature. All samples were passed through a freeze–thaw cycle (−20 °C) prior to analysis. The DNA content was analyzed using a Quant-iT™ PicoGreen™ dsDNA Assay Kit (Fisher Scientific, Hampton, NH) according to the manufacturer's protocol. After thawing, the samples were centrifuged and diluted 1
:
5 with TE buffer. The samples were incubated at room temperature for 5 min in the working PicoGreen™ solution. The DNA concentration was determined by measuring the fluorescence at an excitation wavelength of 480 nm and an emission wavelength of 520 nm (Victor Multilabel Plate Reader, PerkinElmer, Waltham, MA) and comparing to a standard curve of lambda DNA (0 μg mL−1 to 2 μg mL−1).
Histology
Cell seeded scaffolds or stacks of scaffolds were frozen in an optimal cutting temperature (OCT) compound and cryosectioned to a thickness of 20 μm. The sections were fixed in 100% methanol at 4 °C for 5 minutes, followed by 25% methanol for 5 minutes. They were then hydrated in phosphate buffered saline (PBS) for 10 minutes. Following hydration, the sections were stained with Harris hematoxylin and eosin (H&E) following standard procedures.
Pimonidazole staining
Prior to the termination of culture, the cells within the scaffolds were treated with 300 μM pimonidazole (Hypoxyprobe, Burlington, MA) for 24 h, as previously described.32 Pimonidazole is an indirect method to visualize hypoxic cells; it is reductively activated in hypoxic cells forming adducts with thiol containing proteins at pO2 < 10 mmHg. The scaffolded cells were fixed in 10% formalin and frozen in the OCT compound, and the scaffolds were cryosectioned to a thickness of 20 μm. The sections were hydrated in PBS for 10 minutes, then blocked and permeabilized in 5% NGS with 0.1% Triton X-100 for 1 h. The sections were then incubated with a redAPC conjugated secondary antibody at a dilution of 1
:
50 in PBS overnight at 4 °C. They were counter stained with Hoechst 33342 (1
:
5000) to visualize cell nuclei. Scaffolded cells not treated with pimonidazole were used as negative controls.
Brightfield imaging
Brightfield imaging of histology was performed in bright field using an upright microscope (Nikon Eclipse E600, Tokyo, Japan) with a digital camera (Spot Insight CMOS 5.1, Sterling Heights, MI).
Fluorescence imaging
Fluorescence imaging was performed using an Axiovert 200 M Zeiss inverted epi-fluor microscope (Oberkochen, Germany) using an FITC, Texas Red, or DAPI filter set. For imaging of the pimonidazole-labeled cells, the exposure time was determined based on negative controls, where the highest exposure without a positive signal was determined.
Gene expression
Stacks were separated based on the distance from the medium source with the scaffolds on the exterior being combined, and then the next layer being combined, until the two center-most scaffolds were combined. RNA was isolated from scaffolds or stacks after 3 days of culture using a TRIzol reagent according to the manufacturer's instructions. RNA was dissolved in ultrapure molecular grade water and the concentration was measured at an absorbance of 260 nm using a spectrophotometer (Nanodrop 2000, Thermo Scientific). RNA (1 μg) was converted to cDNA using a High-Capacity cDNA Reverse Transcription Kit (Applied Biosystems, Foster City, CA). qRT-PCR was performed on a 7500 Real Time PCR System (Applied Biosystems, Foster City, CA) using SYBR Green qPCR master mix (Applied Biosystems, Foster City, CA). SDHA was used as an internal control as has been previously demonstrated in NB.14,66–68 The relative expression was quantified using the ΔΔ cycle threshold method. Data were normalized to monolayer, 21% O2 cultured cells. Table 1 lists the primer sequences used in this study.
Table 1 List of primers used for qRT-PCR
Gene |
Sequence |
CAIX: carbonic anhydrase XI; GLUT1: glucose transporter 1; IGFBP3: insulin-like growth factor-binding protein 3; VEGF: vascular endothelial growth factor; MMP9: matrix metalloprotease 9; and SDHA: succinate dehydrogenase complex flavoprotein subunit A. |
CAIX
|
F – GGGTGTCATCTGGACTGTGTT |
R – CTTCTGTGCTGCCTTCTCATC |
GLUT1
|
F – CCTGCAGTTTGGCTACAACA |
R – GTGGACCCATGTCTGGTTG |
IGFBP3
|
F – TCTGCGTCAACGCTAGTGC |
R – GCTCTGAGACTCGTAGTCAACT |
VEGF
|
F – AGGAGGAGGGCAGAATCATCA |
R – CTCGATTGGATGGCAGTAGCT |
MMP9
|
F – TTCTGCCCGGACCAAGGATA |
R – ATGCCATTCACGTCGTCCTT |
SDHA (housekeeper) |
F – TGGGAACAAGAGGGCATCTG |
R – CCACCACTGCATCAAATTCATG |
Mathematical modeling of oxygen concentrations
Mathematical modeling was utilized to determine oxygen gradients within the scaffolds. Simulated oxygen diffusion and consumption were performed on a commercially available finite element platform (COMSOL, Burlington, MA). 3D axisymmetric modeling was utilized based on the conditions of cell laden scaffolds cultured in the holder. For preliminary modeling (original scaffold holder), an assumed oxygen concentration of 21% O2 at the interface of the holder well with the medium reservoir was used. For the design of the final scaffold holder, an oxygen concentration of 21% O2 at the interface of the PTFE piece touching the scaffold and medium reservoir was assumed (based on mixing). These models were run through a “transport of diluted species” module incorporating both diffusion and consumption rates (modeled using Michaelis Menten kinetics) available from the literature.69–73
The model utilized the following equations:
Rate of oxygen consumption,
|  | (1) |
Transport of diluted species,
| 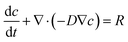 | (2) |
where
c is the concentration of oxygen and the following values are used:
D is the oxygen diffusion coefficient: 2.6 × 10
−9 m
2 s
−1 (assuming culture medium is water), OCR is the oxygen consumption rate: −3.09 × 10
−4 mol m
−3 s
−1, and MM is the Michaelis–Menten coefficient: 0.0046 mol m
−3. The initial conditions and the boundary conditions at the surface were set at an oxygen concentration of 0.21 mol m
−3. The model assumes uniform oxygen consumption within the scaffold and that the scaffolds do not limit oxygen diffusion.
Statistical analysis
Data are presented as mean ± SEM for all gene expression data. Data are presented as mean ± SD for all other data. All data are presented as the mean of three biological replicates, except for the 200 μm vs. 600 μm study which is presented as the mean of three experimental replicates. Statistical significance was determined by one-way ANOVA followed by Tukey's honestly significant difference test in GraphPad Prism (version 5.0; San Diego, CA). For the comparison of only two groups, statistics were determined using an unpaired or paired t-test. Statistics for qRT-PCR were obtained using the delta Ct (gene of interest–housekeeping gene) for each condition. Significance was determined at p < 0.05. The specific statistical test applied to each data set are noted within the figure legends.
Results
Comparison of cell proliferation and cellular distribution throughout 200 μm and 600 μm thick silk scaffolds
For this work, we wanted to develop a scaffolded NB model with thicknesses that could help overcome diffusion and cell-driven transport limitations for oxygen and nutrients in order to develop an in vitro tumor model that mimicked the aspects of the in vivo microenvironment. In the first experiments, we sought to understand the impact of scaffold thickness on cell growth and infiltration. Scaffolds, 200 μm and 600 μm in thickness, were seeded with SK-N-AS and KELLY NB cells and were evaluated for cell proliferation based on the DNA content and metabolic activity (Fig. 1 and Fig. S1†). Scaffolds of 200 μm thickness were evaluated based on known maximum physiological distances between capillaries that support adequate nutrient and oxygen transport for cell viability. Six-hundred micrometer scaffolds were evaluated as this thickness, with a comparable cell density to the 200 μm thick scaffolds, was predicted to have limited nutrient and oxygen transport throughout the bulk of the scaffold. Both 200 μm and 600 μm scaffolds supported cell proliferation as indicated by the increase in the DNA content and metabolic activity. Specifically, both exhibited an initial drop in DNA from day 1 to day 3 (Fig. 1A). Following this, DNA increased from 1.71 μg per scaffold (day 1) to a maximum DNA content of 4.08 μg per scaffold (day 10) for 200 μm and from 2.67 μg per scaffold (day 1) to a maximum DNA content of 3.04 μg per scaffold (day 14) for 600 μm. Similar results were observed with the metabolic activity of KELLY NB cells and SK-N-AS NB cells with maximum increases of 1.69 fold (SK-N-AS, 200 μm, from day 1 to day 14), 1.4 fold (SK-N-AS, 600 μm, from day 1 to day 14), 2.4 fold (KELLY, 200 μm, from day 1 to day 28), and 1.9 fold (KELLY, 600 μm, from day 1 to day 28) (Fig. 1B and Fig. S1A†). No differences in viability and DNA content were seen between 200 μm and 600 μm scaffolds after the initial 3 days of growth. As the cells were seeded proportionally (1 million cells on 200 μm and 3 million cells on 600 μm scaffolds), this suggests that the cells had a reduced ability to grow in the 600 μm scaffolds. Examination of cell infiltration through H&E staining demonstrated the presence of cells in the periphery of both the 200 μm and 600 μm scaffolds (Fig. 1C and D). Over time, the cells fully infiltrated into the 200 μm scaffolds, creating visually evenly distributed cells throughout the scaffold. In comparison, the 600 μm scaffolds demonstrated limited cell distribution throughout the scaffold, even over time, with a dense layer of cells on the outer surface, but limited cells in the center of the scaffold. The same response was observed with KELLY NB cells (Fig. S1B and C†). This suggests that the interior of the scaffold was not conducive to cell growth, likely due to the transport limitations of gases, nutrients and metabolites. Based on these observations, subsequent experiments were performed with 200 μm scaffolds.
 |
| Fig. 1 Impact of scaffold thickness on cell growth and distribution. (A) Impact of scaffold thickness on SK-N-AS growth over time as determined from the DNA content and (B) metabolic activity via resazurin. Data are presented as mean ± SD of three individual samples. Hematoxylin and eosin staining shows SK-N-AS NB cell distribution throughout scaffolds over time in (C) 200 μm scaffolds and (D) 600 μm scaffolds. Black arrows indicate areas of high cell density, while red arrows indicate areas of low cell density. | |
Development of the stacked culture system
Since growing NB cells for an extended period of time within the 200 μm thick scaffolds supports cell viability and cellular distribution through the full thickness, we sought to develop a method to incorporate the 200 μm thick scaffolds into a stacked scaffold model on the millimeter scale. To facilitate the stacking of 200 μm scaffolds, a holder was designed and fabricated using PTFE. The first iteration of scaffold holders was designed to hold stacks of scaffolds between a solid piece of PTFE and a piece of PTFE with a hole for a medium reservoir much like a well plate (Fig. S2A†). This design would create a hypoxic gradient from the interface of the media to the solid PTFE bottom. However, analysis of cell-laden scaffold layers cultured in this scaffold holder (gene expression and COMSOL modeling) demonstrated that the deep and narrow reservoir between the scaffold and the main medium reservoir causes the cells within the scaffolds to be in a hypoxic state (Fig. S2B†). Analysis of gene expression of the scaffolded NB cells cultured as a 3-stack model demonstrated no differences in VEGF, CAIX, or GLUT1 between layers of the stack (Table S1†). In addition, compared to single scaffolds in 21% O2 and 1% O2, the delta Ct of the stacked scaffolds were more similar to cell-laden scaffolds cultured in 1% O2. This is due to the metabolic nature of NB cells outcompeting the oxygen diffusion rate. Based on these preliminary results, we identified a key parameter of the scaffold holder, the depth of the channel between the medium reservoir and the surface of the scaffolds, to evaluate further for optimizing oxygen tension at the scaffold surface.
Subsequently, COMSOL modeling was performed to identify the channel depth that supported oxygen diffusion to the scaffold surface (Fig. S2C–F†). The channel is defined as the region where the reservoir of the medium would sit and come in contact with the scaffold, an essential feature as a result of needing to hold the scaffolds in place. Because of this feature, diffusion limitations may occur if its depth is too large, as evidenced from experiments and computation with the initial scaffold holder design. Reservoir depths of 5 mm, 2.5 mm, 1 mm, and 0.5 mm were modeled. Scaffold holders of 5 mm and 2.5 mm demonstrated hypoxic conditions within a single scaffold, suggesting that oxygen diffusion was too slow to the scaffold. Modeling depths of 1 mm and 0.5 mm suggested that the scaffolds remain within the normal oxygen range for tissue, indicating that oxygen diffusion was able to overcome the high metabolic rate of the cells. This was further confirmed by calculating oxygen diffusion times using the approximation from a 1D random walk:
, with D = 2.6 × 10−9 m2 s−1. The oxygen diffusion time through the modeled depths were 80 min, 20 min, 3 min and 1 min for 5 mm, 2.5 mm, 1 mm, and 0.5 mm, respectively. For subsequent work, 0.5 mm was selected for the reservoir depth as it allowed for the most oxygen to reach the scaffold (based on the diffusion rate) and was on the lower end of the range that the PTFE could be machined.
Scaffold holders were designed to be expandable (for stacks of different thicknesses) and fit within a standard 6-well plate (Fig. 2A–D). Scaffolds could be pre-stacked within the holder (Fig. S3A†) and cultured for a set time point (Fig. 2D), the scaffold holder is opened to access the scaffolds (Fig. S3B†), and the cell-laden scaffolds are separated to individually assess each layer (Fig. S3C and S3D†). COMSOL modeling of scaffold holders demonstrated the potential for a range of oxygen gradients through a cell-laden scaffold (Fig. 3). This modeling assumed an oxygen concentration of 0.21 mol m−3 at the surface of the medium level with the PTFE holder due to the use of a shaker to enhance oxygen diffusion within the bulk medium. COMSOL modeling of two stacked scaffolds demonstrated an oxygen concentration of approximately 0.06 mol m−3 to 0.08 mol m−3 within the scaffolds (Fig. 3A). Increased scaffold number in the stacks demonstrated lower concentrations of oxygen within the center of the stack. Modeling of four, six and eight scaffolds demonstrated a range of oxygen concentrations from 0.04 mol m−3 to 0.06 mol m−3 (exterior) to <0.02 mol m−3 (interior) (Fig. 3B–D). These oxygen levels are similar to those of physiological tumors, which have been shown to range from <0.07 mol m−3 to 0.04 mol m−3 to 0.05 mol m−3.4,74,75
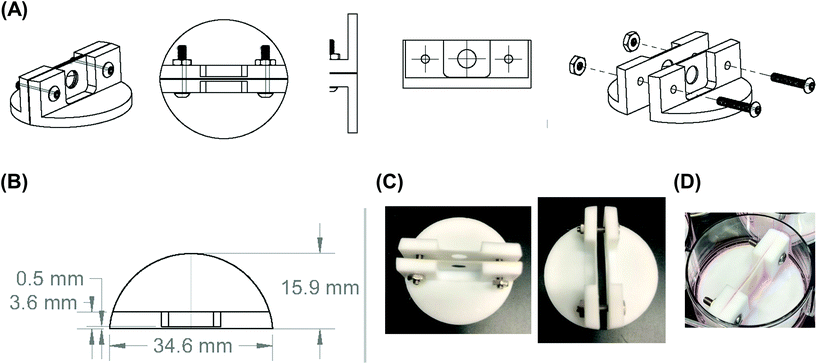 |
| Fig. 2 Final design of the scaffold holder. (A) Computer-aided design drawing of the final scaffold holder. (B) Computer-aided design drawing with dimensions of the final scaffold holder design. (C) Images of the fabricated PTFE scaffold holder. (D) Image of the scaffold holder containing a stacked, four silk scaffold containing NB cells at the end of an experiment. | |
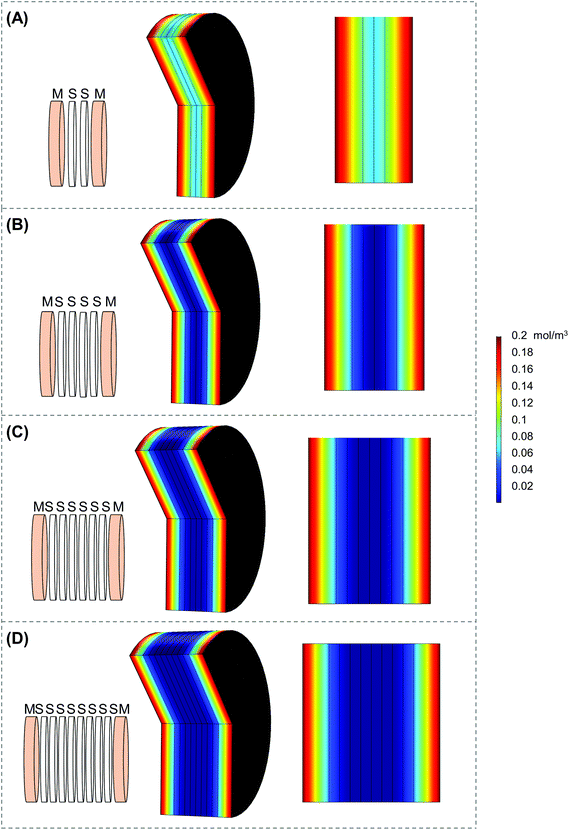 |
| Fig. 3 COMSOL modeling of the stack scaffolds. COMSOL modeling of stacks of (A) two scaffolds, (B) four scaffolds, (C) six scaffolds, and (D) eight scaffolds utilizing parameters from the final scaffold holder design. “M” denotes medium and “S” denotes silk scaffold. | |
Histological evaluation of the stacked scaffold model
Utilizing the stacking system, we first sought to observe the impact of stacking scaffolds on cell distribution throughout the different layers. SK-N-AS NB cells were grown within the stack scaffold models composed of 2, 4, 6, and 8 scaffolds for 3 days on a shaker under ambient oxygen conditions and compared to non-stacked scaffolds under ambient and low oxygen conditions. Hematoxylin and eosin staining of stacks demonstrated that individual scaffolds and stacks of two scaffolds had cells present throughout the scaffold (Fig. 4A–C). In stacks of four scaffolds, cells were present throughout, although more cells were observed on the exterior as compared to the interior (Fig. 4D). Similar results were observed in the stacks of six and eight scaffolds where cells were distributed throughout with a higher concentration on the exterior (Fig. 4E and S3B†). It should be noted that histological artifacts can be observed within the images including varying scaffold widths (Fig. 4DversusFig. 4C) and delamination (Fig. 4D and E). The appearance of different scaffold widths in the images is presumably due to pieces of the silk scaffolds breaking off during sectioning since maintaining the entirety of the silk scaffolds on the slides is challenging even when using charged glass slides. The delimitation of the silk scaffold layers likely occurred during the OCT embedding process when the OCT infiltrated into the bulk of the layered silk scaffolds.
 |
| Fig. 4 Visualization of cell distribution in stacked scaffolds. Hematoxylin and eosin staining shows SK-N-AS NB cell distribution throughout the stacked scaffolds 3 days post-stacking comparable to single scaffolds under (A) ambient and (B) low oxygen conditions 3 days post-stacking. Stacking of (C) two, (D) four, and (E) six scaffolds shows cells distributed throughout the entire scaffold thickness. Images with a ′ or ′′ denote magnified images of the associated low magnification image. Images in (A), (B), (C), (D), and (E) are on the same scale as the scale bar presented in (A). Images in (A′), (B′), (C′), (D′), (D′′), (E′), and (E′′) are on the same scale with the scale bar presented in (A′). | |
DNA content within each stacked scaffold layer emulates the expected impact of the microenvironment on cell growth
Next, we sought to determine the impact of stacking on DNA content at each layer (Fig. 5). The analysis of DNA content in the ambient oxygen single scaffold model as compared to that in the single scaffold model in low oxygen demonstrated a significant decrease in DNA from 3.2 μg per scaffold to 1.4 μg per scaffold (Fig. 5). The analysis of the double stack scaffolds demonstrated similar DNA content to cell-laden scaffolds cultured in ambient oxygen (3.7 μg per scaffold vs. 3.2 μg per scaffold), and a significant increase in DNA content as compared to cell-laden scaffolds cultured under low oxygen conditions (Fig. 5). In quadruple stacked scaffolds the interior of the stack resembled the single low oxygen scaffold in DNA content (1.2 μg per scaffold vs. 1.4 μg per scaffold, stacked and low oxygen, respectively), while the exterior of the stack resembled the single ambient oxygen scaffold (3.3 μg per scaffold vs. 3.2 μg per scaffold, stacked and ambient oxygen, respectively) (Fig. 5). The exterior and interior of a quadruple stack exhibited significant differences in DNA content from cell-laden scaffolds cultured under low and ambient oxygen conditions, respectively. A similar trend was observed in sextuple stacked scaffolds where the DNA content decreased from 2.8 μg per scaffold in the exterior vs. 2.2 μg per scaffold in the middle layer vs. 1.5 μg per scaffold in the interior (Fig. 5). No significant differences were observed between the single cell-laden scaffolds cultured under ambient oxygen and the exterior or middle of the sextuple stack. Similarly, no significant differences were observed between single scaffolds under low oxygen and the middle or interior of the sextuple stack. Evaluation of DNA in the octuple stacked scaffolds demonstrated a lowered change in DNA content as compared to smaller stacks. The DNA content ranged from 3.3 μg per scaffold at the exterior to 2.0 μg per scaffold at the interior (Fig. S4A†). No significant differences were present between any of the layers of the stack. This suggests that eight scaffolds may not be capable of demonstrating a gradient of the microenvironment.
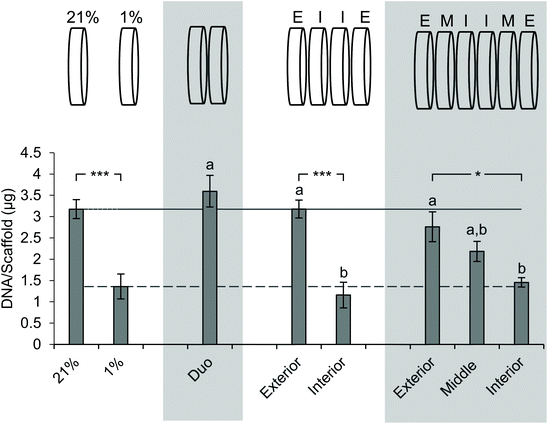 |
| Fig. 5 Impact of stacking on the DNA content of scaffold layers. Evaluation of the DNA content of SK-N-AS NB stacked scaffolds at each layer 3 days post-stacking. 21% (ambient oxygen) and 1% (low oxygen) represent single cell-laden scaffolds cultured at the defined oxygen concentration. Each stack thickness is presented in the graph above. “E” corresponds to the exterior, “I” corresponds to the interior, and “M” corresponds to the middle. Data are presented as mean ± SD of three independent experiments. ‘a’ denotes no statistical significance when compared with the DNA content of cells cultured in single scaffolds under ambient oxygen; ‘b’ denotes no statistical significance when compared with the DNA content of cells cultured in single scaffolds at low oxygen. Asterisks indicate statistical significance between the groups (***p < 0.001, **p < 0.01, *p < 0.05). | |
Staining with pimonidazole as a hypoxia marker indicates cell-driven hypoxic gradients
To visualize hypoxic gradient SK-N-AS stacks of 2, 4, 6, and 8, the scaffolds were stained for pimonidazole after 3 days of culture (Fig. 6A–D and Fig. S5A–E†). As demonstrated previously,63 single cell-laden scaffolds cultured under ambient and low oxygen conditions exhibited positive pimonidazole staining (Fig. 6A and B, Fig. S5A and B†). Double stacked scaffolds and quadruple scaffolds demonstrated a gradient of pimonidazole positive staining, with higher levels of positive staining observed in the center of the scaffolds (Fig. 6C and D, Fig. S5C and D†). Double stacked scaffolds demonstrated lower pimonidazole staining then cell-laden scaffolds cultured under ambient oxygen. This is likely because a shaker was used to improve oxygen diffusion in the stacked scaffolds. Pimonidazole staining in sextuple and octuple stacked scaffolds demonstrated less distinct gradients then double and quadruple stacked scaffolds (Fig. S5E and F†). Sextuple stacked scaffolds demonstrated reduced pimonidazole staining at the exterior of the stack as compared to the interior of the stack, further confirming the changes observed in DNA content and gene expression. Octuple stacked scaffolds demonstrated no clear differences in pimonidazole staining throughout the layers of the stacked scaffolds. This further confirmed our previous results suggesting a lack of a gradient in the octuple stacks.
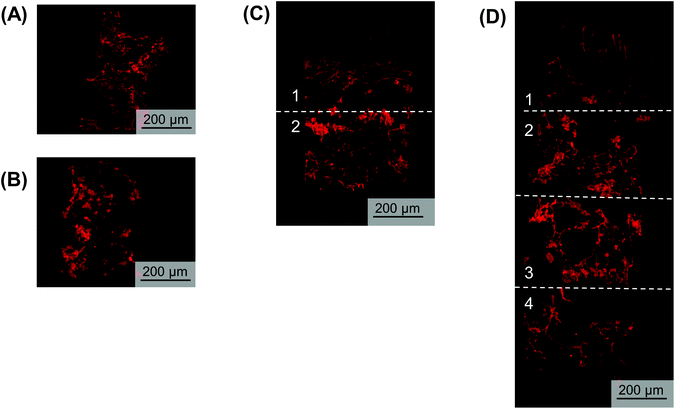 |
| Fig. 6 Pimonidazole (red) staining of NB cells within the stacked scaffolds. Pimonidazole staining of scaffolded SK-N-AS NB cells to visualize hypoxia within stacks 3 days post-stacking. (A) Single cell-laden scaffolds cultured in ambient oxygen (21% O2). (B) Single cell-laden scaffolds cultured in low oxygen (1% O2). (C) Double stacked scaffolds. (D) Quadruple stacked scaffolds. | |
Analysis of gene expression changes indicates hypoxic gradients
After determining the differences in DNA content and observing a gradient of pimonidazole staining, we sought to characterize the microenvironment of the stacks through gene expression. SK-N-AS NB cell seeded scaffolds were cultured for 3 days at ambient oxygen then moved to stacked scaffold models maintained at 21% O2 (ambient oxygen), or the single scaffolds maintained at 21% O2 or transferred to 1% O2 (low oxygen) as controls. The cell-laden stacked scaffold models or controls were cultured for an additional 3 d. The gene expression of the hypoxia-related genes VEGF, CAIX, GLUT1, and IGFBP3 was evaluated (Fig. 7). Cells cultured in the single scaffolds under ambient oxygen and low oxygen conditions demonstrated increased expression of all target genes as compared to monolayer, ambient oxygen cultures (Fig. 7). When comparing cells cultured in single scaffolds under low oxygen conditions to those under ambient oxygen conditions, the low oxygen conditions were found to induce a significant increase in the gene expression of VEGF, CAIX, and GLUT1. IGFB3 expression was not significantly different; however, a trend toward increased gene expression under low oxygen conditions was observed.
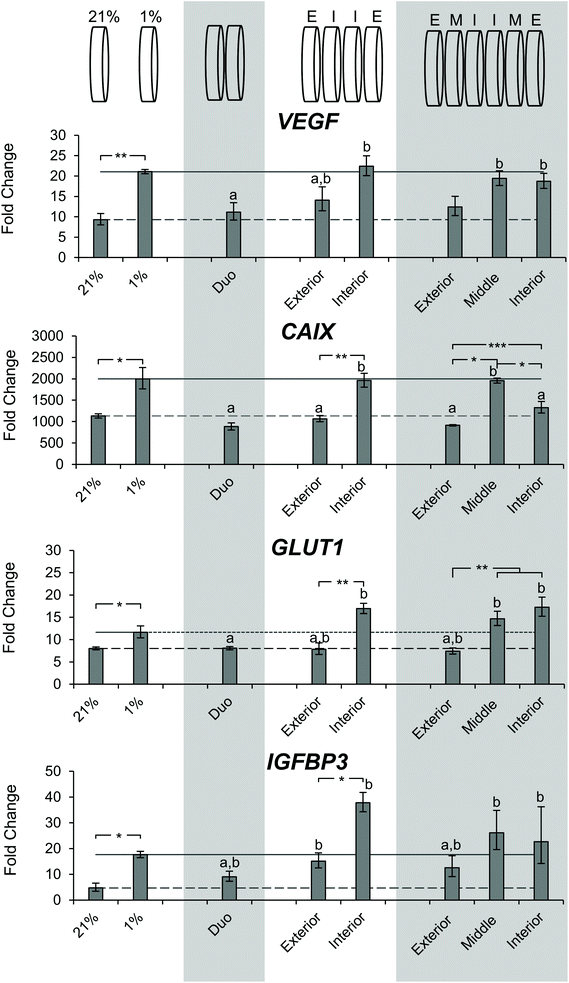 |
| Fig. 7 Evaluation of hypoxia related gene expression of SK-N-AS cells cultured in stacked scaffolds. Evaluation of hypoxia related gene expression of SK-N-AS NB cells cultured in stacked scaffolds at each individual layer as compared to 3D ambient oxygen culture 3 days post-stacking. 21% (ambient oxygen) and 1% (low oxygen) represent cell-laden, single scaffolds cultured at that oxygen concentration. Representation of each stacking thickness can be found above it in the graph. “E” corresponds to exterior, “I” corresponds to interior, and “M” corresponds to the middle. Data are presented as mean ± SD of three independent experiments. a denotes no statistical significance from cell-laden, single scaffolds cultured under ambient oxygen, ‘b’ denotes no statistical significance from cell-laden, single scaffolds cultured under low oxygen. All gene expression is normalized to a monolayer, ambient oxygen control. Asterisks indicate statistical significance between the groups (***p < 0.001, **p < 0.01, *p < 0.05). | |
The evaluation of cells from the double stacked scaffolds demonstrated similar expression levels of VEGF (11.1 vs. 9.3), CAIX (883.0 vs. 1133.2), GLUT1 (8.1 vs. 8.0), and IGFBP3 (9.1 vs. 4.7) when compared to cells from the single scaffolds cultured under ambient oxygen conditions (Fig. 7). However, when compared to cells from the single scaffolds cultured under low oxygen conditions, the cells from the double stacked scaffolds had significantly lower expression of VEGF (11.1 vs. 21.1), CAIX (883.0 vs. 1997.3) and GLUT1 (8.1 vs. 11.6) (Fig. 7).
Quadruple stacked scaffolds demonstrated stack position-dependence in hypoxia-related gene expression (Fig. 7). The cells cultured in the interior of the quadruple stacked scaffolds had increased expression of hypoxic markers as compared to the exterior with changes in VEGF (22.4 vs. 14.1 fold), CAIX (1960.4 vs. 1066.5 fold), GLUT1 (16.9 vs. 12.42 fold), and IGFBP3 (37.8 vs. 7.9 fold). The gene expression changes were significant with the exception of VEGF (Fig. 7). A comparison of the cells cultured in the interior of the quadruple stacked scaffolds with cells cultured in single scaffolds under low oxygen conditions demonstrated no significant differences in gene expression. Similarly, comparison of the cells cultured in the exterior of the quadruple stacked scaffolds with cells cultured in single scaffolds under ambient oxygen conditions showed a significant difference only in IGFBP3 expression. This suggests the presence of an oxygen gradient, where the cells cultured in the exterior scaffolds resembled scaffolded ambient oxygen culture and the cells cultured in the interior scaffolds resembled scaffolded low oxygen culture. The potential gas, nutrient, and metabolite gradients likely formed within these models are a direct result of the interplay between the path length required for molecular diffusion (the thickness of the scaffold or multi-layer scaffolds) and reactant consumption (e.g. oxygen and glucose) and metabolite production (e.g. lactic acid) due to cellular metabolism. The silk scaffold pore size and interconnected porous structure are not anticipated to greatly impact gas, nutrient, and metabolite diffusion given the micron-scale pore size compared to the angstrom-scale size of the analytes.53,62,63
The analysis of sextuple stacked scaffolds also suggested the presence of hypoxic regions in which the cells cultured within the middle and interior scaffolds demonstrated higher expression of hypoxic genes than those from the exterior scaffolds (Fig. 7). No significant differences in VEGF gene expression were observed between the cell cultures in any layer of the sextuple stacked scaffolds. However, cells cultured in the middle and interior scaffolds exhibited a significant increase in VEGF expression when compared to cells cultured in the single scaffolds under ambient oxygen conditions (19.4, 18.7, and 9.3 for middle, interior, and single scaffolds, respectively) (Fig. 7). Cells cultured in the interior and middle scaffolds exhibited a significant increase in CAIX expression when compared to cells cultured in the exterior scaffolds (1328.5, 1957.5, and 915.1 for interior, middle, and exterior, respectively) (Fig. 7). Similarly, cells cultured in the interior and middle scaffolds exhibited a significant increase in GLUT1 expression when compared to cells cultured in the exterior scaffolds (17.3, 14.7, and 7.4 interior, middle, and exterior, respectively) (Fig. 7). No changes in IGFBP3 expression were observed for cells cultured in the different layers of the sextuple stacked scaffolds (Fig. 7).
Cells cultured in the octuple stacked scaffolds demonstrated an interesting trend with an increase from the exterior scaffold to the adjacent (outer middle) scaffold, followed by a decrease in the two most interior scaffold positions (Fig. S6†). This trend was observed in VEGF, CAIX, GLUT1, and IGFBP3 expression. Cells cultured in the outer middle scaffold and interior scaffold exhibited increased VEGF expression compared to those cultured in the exterior scaffold (Fig. S6†). Cells cultured in the outer middle and inner middle scaffolds exhibited increased GLUT1 expression when compared to cells cultured in the exterior scaffold and the interior scaffold (Fig. S6†). This lack of clear trend for hypoxia response genes suggests that the octuple stacked scaffolds may be ineffective at generating hypoxic gradient due to insufficient sealing at the scaffold edges or imperfections in scaffold stacking.
MMP9 gene expression was also evaluated in the stacked scaffold models (Fig. S7†). Previous results demonstrated that changes in MMP9 expression are driven predominantly by 3D culture.63 A similar finding was observed for the cells cultured in the stacked scaffold models, where no significant difference between the different scaffold positions was observed (Fig. S7†) though a trend towards increased expression can be observed with progression towards the interior of the stacked scaffolds.
The gene expression of KELLY NB cells within the quadruple stacked scaffolds was evaluated as well (Fig. S8†). Cells grown in single scaffolds under low oxygen conditions and cells grown in the interior scaffold exhibited a significant increase in VEGF, CAIX, GLUT1 and IGFBP3 expression as compared to cells cultured in single scaffolds under ambient oxygen conditions. While no significant differences were observed between cells cultured in the interior and exterior scaffolds, there was a trend towards increased expression of VEGF, CAIX, GLUT1, and IGFBP3 from the exterior to the interior when compared to the exterior (Fig. S8†). Considering the gene expression data together for the SK-N-AS NB cells and the Kelly NB cells, the quadruple stacked scaffold model was sufficient to generate clear differences in the interior and exterior scaffolds. Therefore, the quadruple stacked scaffold model was used for further experimentation.
Time course investigation of cell proliferation within the layers of the quadruple stack model
To evaluate longer-term cell survival within the quadruple stacked scaffold model, we next sought to investigate cell viability and proliferation within the model over time. The DNA content of the interior and exterior scaffolds was evaluated after day 3, day, 7, and day 14 of culture and normalized to the exterior scaffold DNA content at day 3 (Fig. S9†). SK-N-AS NB cells from the exterior and interior stacks retained their viability over the experimental time course as indicated by the maintenance or increase in DNA content normalized to the exterior scaffold DNA content at day 3. A statistical increase in the DNA content was observed between day 3 and day 14 of the SK-N-AS NB cells cultured in the exterior scaffolds (1.0 ± 0.1 versus 2.0 ± 0.3), suggesting that cell proliferation occurred in the exterior of the scaffolds.
Cell response to a hypoxia-activated drug, tirapazamine
After determining that cells could retain their viability for an extended period of time after developing the quadruple stacked scaffold model, we sought to evaluate the response of the SK-N-AS NB cells to a hypoxia-activated drug, tirapazamine. Tirapazamine is an experimental cancer drug that induces cell death under hypoxic conditions via the formation of a toxic radical. For this work, we treated the quadruple stacked scaffold cells with 20 μg mL−1 tirapazamine, a concentration previously shown to induce cell death in a single scaffold model.63 Cells treated with tirapazamine exhibited reduced viability as compared to untreated cells when comparing the match stacked scaffold position (Fig. 8). Specifically, the cell viability of the exterior region of the tirapazamine treated group was 85% of the untreated group. The cell viability of the interior region of the tirapazamine treated group was 70% of the untreated group, where the viability normalized to the exterior of the untreated group was 82% versus 57%, respectively. These data show that the quadruple stacked scaffold model could be used to test hypoxia-activated drugs, which could be extended to testing of drugs that are active or inactive under varying microenvironment conditions within a single in vitro model system.
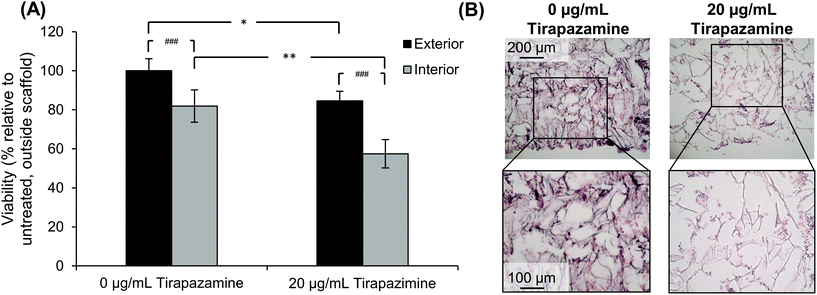 |
| Fig. 8 Response of SK-N-AS cells cultured in the quadruple stack scaffold model to the hypoxia-activated drug tirapazamine. (A) Cell viability as compared to the exterior scaffolds of the control group (0 μg mL−1 tirapazamine). Data are presented as mean ± SD for five independent samples. Asterisks indicate statistical significance between the treatment groups as determined by an unpaired T-test (*p < 0.05, **p < 0.01). Pound indicates significance between exterior and interior scaffolds as determined by a paired T-test (##p < 0.01). (B) Histological images of the quadruple stack model treated with or without 20 μg mL−1 tirapazamine. | |
Discussion
Hypoxia is a critical cell driven gradient in solid tumors that has been demonstrated to drive tumorigenesis, metastasis, and therapeutic resistance. However, few models exist that are capable of controllable oxygen gradients, specifically those on a millimeter-scale. With existing models come challenges, both in the creation of the gradients and the analysis. For example, research has demonstrated that spheroids are capable of developing oxygen gradients based on their diameter.72,76–78 However, evaluation of different regions of the spheroid is challenging, as the regions cannot be easily isolated. ECM or scaffold-based approaches have also been used; however cell infiltration and long-term spatial control continue to prove difficult.79–81 To overcome these challenges, we utilized 200 μm silk scaffolds stacked together to generate cell driven oxygen gradients that mimic those of solid tumors. These scaffolds can be easily separated allowing for layer-by-layer analysis. Silk scaffolds are mechanically strong and porous, allowing for temporal retention of scaffold dimensions and cell-driven oxygen and nutrient gradients. Furthermore, silk does not contribute biologically to the system allowing for cell-driven responses to dominate the model system, in comparison with ECM-based hydrogels (e.g. Matrigel and Cultrex) which have been demonstrated to contain bioactive proteins and peptides that directly provide signal to the cells.82
In previous work, we demonstrated that both growth in 3D silk scaffolds and oxygen concentration were able to impact key pathways in NB. Porous silk scaffolds provide a 3D structural support for cell growth while minimally impacting cell behavior directly because there are no cell-binding sites within the protein sequence. Cell attachment to silk fibroin is likely mediated through passive adsorption of proteins in the culturing environment thereby minimizing the direct impact of scaffold biochemistry on cell responses. Porous silk scaffolds can be readily fabricated through multiple strategies including lyophilization, salt-leaching (both aqueous and non-aqueous based methods), and gas-foaming. Lyophilization presents a rapid approach to fabricating porous silk scaffolds. With the use of rate controlled freezing, improved control over the porous structure can be achieved as compared to uncontrolled freezing at single set temperatures.
In the work presented here, we expanded on our previous model to create a system with differing cell-driven microenvironments that can be readily separated and evaluated on a layer-by-layer basis. Preliminary experiments were performed to evaluate the impact of silk scaffold thickness on cell growth and distribution. For this work, 600 μm thick scaffolds were chosen based on preliminary COMSOL modeling that showed a reduction of oxygen concentration throughout the scaffold thickness when the model as cell-seeded scaffolds submerged in bulk media (following our previously reported method). Furthermore, this thickness is greater than the typical distance between blood capillaries (approximately 150 μm–200 μm) but not so thick that necrosis would be an obvious outcome. Culturing the cells within the 600 μm thick silk scaffolds demonstrated a reduction in cell distribution throughout the scaffold as compared to 200 μm scaffolds over time while still having the same DNA content, whereas the 200 μm thick scaffolds exhibited cells distributed throughout the scaffold thickness through 28 days of culture. In vivo, distances of 150 μm–200 μm have been identified as the maximum diffusion distances for oxygen and nutrients due to cell-driven consumption.83,84 Scaffolds with a thickness of 200 μm achieved full cell infiltration. To create cell driven oxygen gradients, these 200 μm scaffolds can be stacked together to form multilayer stacks that mimic thicker tissue. To facilitate scaffold stacking, a scaffold holder was designed via mathematical modeling. COMSOL modeling was used to optimize the well depth of a scaffold holder to create a system capable of generating hypoxic gradients. Modeling completed on a range of scaffold numbers allowed us to predict the oxygen consumption and tension at each layer and suggested that 4 scaffolds would be necessary to generate a hypoxic gradient.
Histological evaluation of stacks ranging from 2 to 8 scaffolds demonstrated the presence of cells in each scaffold layer, regardless of stack thickness, with cell distribution similar to that of unstacked scaffolds. The use of the scaffold stacking approach allowed the creation of a tumor model on the millimeter-scale of thickness, which is more relevant to solid tumors than monolayer cultures, for both the gradients developed and as a diffusion barrier for therapeutics. Additionally, unlike spheroids or other solid models, the stacks can be separated into single scaffolds allowing the analysis of individual levels as compared to the bulk analysis typically performed on spheroid or scaffolded cultures. While other stacking based approaches exist, the most common being an ECM hydrogel infused paper-based and polymer-mesh approach, they rely on ECM hydrogels to deliver the cells on the scaffold, which can have an additional impact on cellular behavior.37–39,85
Using this in vitro system, we were able to demonstrate cell generated oxygen gradients within the tissue model. Staining of pimonidazole confirmed hypoxic gradients within the stacks. Stacks with thicknesses of two and four scaffolds demonstrated clear hypoxic gradients, with cells in the interior of the stack experiencing lower oxygen concentrations indicated by a gradient in pimonidazole staining. This is consistent with what has been observed in spheroid models as well as primary tumors.13,15,23,72 These gradients were less distinct in the stacks of six to eight scaffolds, potentially due to cell death or leaking of the scaffold holder. Furthermore, we confirmed that cells which are placed further from the source of oxygen and media showed increased gene expression of hypoxia markers VEGF, CAIX, GLUT1, and IGFBP3. This is consistent with our previous work evaluating 3D NB culture in low oxygen incubators63 as well as the work by Herrmann et al., evaluating the effect of DMOG treatment on NB cells.20 Our results also align with the work by Derda et al., where stacked layers of paper were used as a structural support for hydrogel culturing of cancer cells.37 In the layered paper approach, VEGF and IGFBP3 gene expression levels were the highest within layers farthest from the oxygen source after 9 days of culture. In a “tissue roll for analysis of cellular environment and response”, or TRACER, approach developed by Rodenhizer et al., GLUT1 gene expression of cancer cells was increased in layers farther away from the medium source after 6 h and 24 h of culture.44 Performing the gene expression and pimonidazole staining at shorter and longer time points and with a more extensive panel of gene markers, including those related to alternative metabolic pathways, stemness, and metastasis, would better define this system for modeling neuroblastoma in vitro. In addition to changes in hypoxia markers, we also observed a decrease in DNA at the interior of the stacks, indicating fewer viable cells in this region. In spheroid models, a region of necrosis at the center of the spheroid has been observed.86,87 A similar phenomenon is observed in vivo in tumors that grow rapidly and lack sufficient vascularization. This decrease in DNA could be explained by cells lacking oxygen/nutrients for proliferation or causing apoptosis. Interestingly, the octuple stacked scaffolds demonstrated a decrease in these hypoxia gene expression markers in the two interior-most layers of the stack and no changes in DNA content, which was consistent with the pimonidazole staining. This could be due to the number of scaffolds exceeding the limitations of our holder design resulting in leaking, which aligns with the gene expression results.
Though experiments directly probing metabolic changes were not performed, metabolic changes likely occur within the models developed after sustained exposure to hypoxia and glucose depletion through changes in cellular metabolism.88 Cancer cell metabolism shifts from oxidative phosphorylation, producing 30–36 ATP molecules per glucose, to aerobic glycolysis with lactate formation, producing 2 ATP molecules per glucose.89,90 Increased glutamine catabolism is also a hallmark of malignant transformation. The increased glutamine catabolism has been shown to be driven by the MYC oncogene through upregulation of glutamine transporters to fuel the TCA cycle and glutathione production under hypoxic conditions.91–96 Cancer cells also exhibit altered metabolism related to their increased dependency on glutamine through MYC oncogene upregulation of GLS. Through endocytic and cannibalistic mechanisms, cancer cells utilize alternative methods to acquire nutrients including recovery of free amino acids through lysosomal degradation of extracellular proteins via macropinocytosis,97–99 and nutrient recovery by entosis of adjacent cells.100
The metabolic changes that occur in tumor cells also impact the tumor microenvironment, primarily due to the large quantities of lactate produced through aerobic and anaerobic glycolysis. The increase in lactic acid production results in an acidification of the extracellular pH, which favors processes such as metastasis and angiogenesis. This environment is further maintained by the transmembrane protein CAIX. CAIX works to support diverse acid extruders MCT1 and MCT4, which mediate H+ efflux, thus increasing the extracellular acidification.101,102 In this acidic environment, cancer cells are more adapted to survive, thus enhancing tumor progression. Initially, acidosis triggers a stress response in cells limiting proliferation and inducing cell death. However, those cells that survive are an emergent phenotype resistant to acid-induced cell death and continue to acidify the microenvironment resulting in toxicity to other cells and survival.103 The ultimate goal of developing in vitro NB models is to develop more effective treatment strategies. Using the quadruple stacked scaffold model seeded with SK-N-AS NB cells, tirapazamine, a drug that is activated to a toxic radical under hypoxic conditions, was shown to induce reduced cell viability in the interior stacks as compared to the exterior stacks. This suggests that an in vitro NB model that allows for independent assessment of different regions may provide necessary information for drug screening. For instance, a model culturing in a low oxygen incubator setting would result in reduced cell viability when treated with hypoxia-activated drugs even though in vivo the tumor microenvironment contains regions of oxygenation that would not support drug activation.
There are some challenges and limitations of the current approach that should be addressed in future work. Further characterization of the development of the stacked scaffold models to understand the impact of culture duration on cell viability, gene expression, metabolic, and secretome changes should be performed. Additionally, a quantitative understanding of the reproducibility of generating several stacked scaffolds is necessary before being able to perform large-scale drug screening experiments. The data presented here are from multiple replicate samples and multiple independent experiments, so reproducibility of these small-scale studies is not a concern. However, for scale up and testing of multiple drugs, drug combinations, and concentrations within a single experiment, a faster approach should be developed with minimal scaffold handing, either via using large scaffolds within a single scaffold holder with independently accessible zones similar to the paper-based approach developed by Derda and colleagues37 or developing a robotic handling system for cell seeding, scaffold handling, and scaffold stacking. Additionally, the current approach is limited to a single cell type, NB cancer cells. This approach can be readily applied for other solid tumor types, including hepatocellular carcinoma and renal cell carcinoma. Developing the model to contain multiple cell types such as cancer associated fibroblasts, tumor associated macrophages, endothelial cells, and other immune cell populations, may have utility in understanding cell–cell interactions and responsiveness to other microenvironment targeting drugs. Overall, we expect this silk scaffolding approach to be broadly useful, both in understanding fundamental tumor biology and in therapeutic development, which may be adopted for many cancer types.
Conclusion
In summary, we have demonstrated a stacked porous silk scaffolding approach to modeling cell driven-oxygen gradients in tumors at the millimeter-scale. This was established through the use of COMSOL modeling to define parameters for a stacked scaffold model and scaffold holder design. The stacked scaffold model was capable of retaining cells throughout the model. Positional variation in SK-N-AS NB cell responses was demonstrated through changes in cell content (DNA), gene expression, and pimonidazole staining. The primary findings were that interior scaffolds had lower cell content as determined by DNA quantification, and the cells cultured within the interior scaffolds had increased gene expression for the hypoxia-regulated genes VEGF, IGFBP3, GLUT1, and CAIX. In addition, the gene expression changes were observed in KELLY NB cells. Using the quadruple stacked scaffold model, we confirmed cell viability through 14 days of culture and proliferation in the exterior scaffold layer. Finally, we show that a quadruple stack scaffold model can be used to evaluate the toxicity of a hypoxia-activated drug, tirapazamine, and it showed spatial differences in cytotoxicity correlating with reduced oxygen concentrations. This system represents a promising in vitro model for drug screening for NB. Future studies should develop this stacked scaffold approach further for rapid, high throughput assembly, to incorporate multiple cell types within a single model, and to extend beyond NB to other solid tumor models.
Author contributions
Conceptualization: KJO, KSM, CQR, JMC; data curation: KJO, KSM, CQR, JMC; formal analysis: KJO, KSM, JMC; funding acquisition: JMC; investigation: KJO, KSM, CQR; methodology: KJO, KSM, CQR, JMC; project administration: JMC; resources: JMC; supervision: KJO, KSM, JMC; validation: KJO, KSM; visualization: KJO, KSM, JMC; writing – original draft: KJO, KSM, JMC; writing – review & editing: KJO, KSM, CQR, JMC.
Conflicts of interest
There are no conflicts to declare.
Acknowledgements
The authors thank Elzani van Zyl (Biomedical Engineering Department at Worcester Polytechnic Institute) for her assistance with the computer-aided design drawings of the scaffold holder. The authors would like to also thank Dr Sakthikumar Ambady for providing the EGFP-transfected KELLY NB cells.
References
- W. B. London, R. P. Castleberry, K. K. Matthay, A. T. Look, R. C. Seeger, H. Shimada, P. Thorner, G. Brodeur, J. M. Maris, C. P. Reynolds and S. L. Cohn, J. Clin. Oncol., 2005, 23, 6459–6465 CrossRef CAS PubMed.
- W. B. London, R. Bagatell, B. J. Weigel, E. Fox, D. Guo, C. Van Ryn, A. Naranjo and J. R. Park, Cancer, 2017, 123, 4914–4923 CrossRef CAS PubMed.
- K. K. Matthay, J. M. Maris, G. Schleiermacher, A. Nakagawara, C. L. Mackall, L. Diller and W. A. Weiss, Nat. Rev. Dis. Primers, 2016, 2, 16078 CrossRef PubMed.
- J. A. Bertout, S. A. Patel and M. C. Simon, Nat. Rev. Cancer, 2008, 8, 967–975 CrossRef CAS PubMed.
- R. Courtnay, D. C. Ngo, N. Malik, K. Ververis, S. M. Tortorella and T. C. Karagiannis, Mol. Biol. Rep., 2015, 42, 841–851 CrossRef CAS PubMed.
- L. D’Ignazio, M. Batie and S. Rocha, Biomedicines, 2017, 5, 21 CrossRef PubMed.
- S. R. McKeown, Br. J. Radiol., 2014, 87, 20130676 CrossRef CAS PubMed.
- B. Yu, A. Shah, B. Q. Wang, N. Rajaram, Q. L. Wang, N. Ramanujam, G. M. Palmer and M. W. Dewhirst, J. Biophotonics, 2014, 7, 552–564 CrossRef CAS PubMed.
- S. R. McKeown, Br. J. Radiol., 2014, 87, 20130676 CrossRef CAS PubMed.
- S. K. Parks, Y. Cormerais and J. Pouyssegur, J. Physiol., 2017, 595, 2439–2450 CrossRef CAS.
- B. Muz, P. de la Puente, F. Azab and A. K. Azab, Hypoxia, 2015, 3, 83–92 CrossRef.
- C. R. Justus, E. J. Sanderlin and L. V. Yang, Int. J. Mol. Sci., 2015, 16, 11055–11086 CrossRef CAS PubMed.
- P. Fardin, A. Barla, S. Mosci, L. Rosasco, A. Verri, R. Versteeg, H. N. Caron, J. J. Molenaar, I. Ora, A. Eva, M. Puppo and L. Varesio, Mol. Cancer, 2010, 9, 185 CrossRef.
- L. Holmquist-Mengelbier, E. Fredlund, T. Lofstedt, R. Noguera, S. Navarro, H. Nilsson, A. Pietras, J. Vallon-Christersson, A. Borg, K. Gradin, L. Poellinger and S. Pahlman, Cancer Cell, 2006, 10, 413–423 CrossRef CAS PubMed.
- H. M. Ameis, A. Drenckhan, M. Freytag, J. R. Izbicki, C. T. Supuran, K. Reinshagen, S. Holland-Cunz and S. J. Gros, Pediatr. Surg. Int., 2016, 32, 187–192 CrossRef PubMed.
- M. R. Horsman and J. Overgaard, J. Radiat. Res., 2016, 57(Suppl 1), i90–i98 CrossRef PubMed.
- G. Jimenez-Valerio and O. Casanovas, Trends Cancer, 2017, 3, 10–18 CrossRef CAS PubMed.
- H. Doktorova, J. Hrabeta, M. A. Khalil and T. Eckschlager, Biomed. Pap., 2015, 159, 166–177 CrossRef PubMed.
- J. J. Morton, G. Bird, Y. Refaeli and A. Jimeno, Cancer Res., 2016, 76, 6153–6158 CrossRef CAS PubMed.
- A. Herrmann, M. Rice, R. Levy, B. L. Pizer, P. D. Losty, D. Moss and V. See, Oncogenesis, 2015, 4, e138 CrossRef CAS PubMed.
- V. Petrova, M. Annicchiarico-Petruzzelli, G. Melino and I. Amelio, Oncogenesis, 2018, 7, 10 CrossRef.
- J. Chiche, K. Ilc, J. Laferriere, E. Trottier, F. Dayan, N. M. Mazure, M. C. Brahimi-Horn and J. Pouyssegur, Cancer Res., 2009, 69, 358–368 CrossRef CAS PubMed.
- S. Daster, N. Amatruda, D. Calabrese, R. Ivanek, E. Turrini, R. A. Droeser, P. Zajac, C. Fimognari, G. C. Spagnoli, G. Iezzi, V. Mele and M. G. Muraro, Oncotarget, 2017, 8, 1725–1736 CrossRef PubMed.
- A. Voissiere, E. Jouberton, E. Maubert, F. Degoul, C. Peyrode, J. M. Chezal and E. Miot-Noirault, PLoS One, 2017, 12, e0181340 CrossRef PubMed.
- S. Riffle and R. S. Hegde, J. Exp. Clin. Cancer Res., 2017, 36, 102 CrossRef.
- G. Xu, F. Yin, H. Wu, X. Hu, L. Zheng and J. Zhao, J. Tissue Eng., 2014, 5, 2041731413520438 CrossRef PubMed.
- L. Chen, Z. Xiao, Y. Meng, Y. Zhao, J. Han, G. Su, B. Chen and J. Dai, Biomaterials, 2012, 33, 1437–1444 CrossRef CAS PubMed.
- T. M. Yeung, S. C. Gandhi and W. F. Bodmer, Proc. Natl. Acad. Sci. U. S. A., 2011, 108, 4382–4387 CrossRef CAS PubMed.
- M. W. Tibbitt and K. S. Anseth, Biotechnol. Bioeng., 2009, 103, 655–663 CrossRef CAS PubMed.
- E. Dondajewska, W. Juzwa, A. Mackiewicz and H. Dams-Kozlowska, Oncotarget, 2018, 9, 4935–4950 CrossRef PubMed.
- S. J. Hollister, Nat. Mater., 2005, 4, 518–524 CrossRef CAS PubMed.
- A. S. Truong and M. R. Lockett, Analyst, 2016, 141, 3874–3882 RSC.
- M. Leung, F. M. Kievit, S. J. Florczyk, O. Veiseh, J. Wu, J. O. Park and M. Zhang, Pharm. Res., 2010, 27, 1939–1948 CrossRef CAS PubMed.
- A. Kamili, A. J. Gifford, N. Li, C. Mayoh, S.-O. Chow, T. W. Failes, G. L. Eden, R. Cadiz, J. Xie, R. E. Lukeis, M. D. Norris, M. Haber, G. B. McCowage, G. M. Arndt, T. N. Trahair and J. I. Fletcher, Br. J. Cancer, 2020, 122, 680–691 CrossRef PubMed.
- T. Teitz, J. J. Stanke, S. Federico, C. L. Bradley, R. Brennan, J. Zhang, M. D. Johnson, J. Sedlacik, M. Inoue, Z. M. Zhang, S. Frase, J. E. Rehg, C. M. Hillenbrand, D. Finkelstein, C. Calabrese, M. A. Dyer and J. M. Lahti, PLoS One, 2011, 6, e19133 CrossRef CAS PubMed.
- C. Huertas-Castaño, M. A. Gómez-Muñoz, R. Pardal and F. M. Vega, Int. J. Mol. Sci., 2019, 21, 39 CrossRef PubMed.
- R. Derda, A. Laromaine, A. Mammoto, S. K. Tang, T. Mammoto, D. E. Ingber and G. M. Whitesides, Proc. Natl. Acad. Sci. U. S. A., 2009, 106, 18457–18462 CrossRef CAS PubMed.
- B. Mosadegh, M. R. Lockett, K. T. Minn, K. A. Simon, K. Gilbert, S. Hillier, D. Newsome, H. Li, A. B. Hall, D. M. Boucher, B. K. Eustace and G. M. Whitesides, Biomaterials, 2015, 52, 262–271 CrossRef CAS PubMed.
- R. Derda, S. K. Tang, A. Laromaine, B. Mosadegh, E. Hong, M. Mwangi, A. Mammoto, D. E. Ingber and G. M. Whitesides, PLoS One, 2011, 6, e18940 CrossRef CAS PubMed.
- K. A. Simon, K. M. Park, B. Mosadegh, A. B. Subramaniam, A. D. Mazzeo, P. M. Ngo and G. M. Whitesides, Biomaterials, 2014, 35, 259–268 CrossRef CAS.
- A. S. Truong and M. R. Lockett, Analyst, 2016, 141, 3874–3882 RSC.
- M. W. Boyce, G. J. LaBonia, A. B. Hummon and M. R. Lockett, Analyst, 2017, 142, 2819–2827 RSC.
- D. Rodenhizer, T. Dean, B. Xu, D. Cojocari and A. P. McGuigan, Nat. Protoc., 2018, 13, 1917–1957 CrossRef CAS.
- D. Rodenhizer, D. Cojocari, B. G. Wouters and A. P. McGuigan, Biofabrication, 2016, 8, 045008 CrossRef PubMed.
- D. Rodenhizer, E. Gaude, D. Cojocari, R. Mahadevan, C. Frezza, B. G. Wouters and A. P. McGuigan, Nat. Mater., 2016, 15, 227–234 CrossRef CAS PubMed.
- M. Young, D. Rodenhizer, T. Dean, E. D'Arcangelo, B. Xu, L. Ailles and A. P. McGuigan, Biomaterials, 2018, 164, 54–69 CrossRef CAS PubMed.
- E. Bellas, B. J. Panilaitis, D. L. Glettig, C. A. Kirker-Head, J. J. Yoo, K. G. Marra, J. P. Rubin and D. L. Kaplan, Biomaterials, 2013, 34, 2960–2968 CrossRef CAS PubMed.
- J. Brown, C. L. Lu, J. Coburn and D. L. Kaplan, Acta Biomater., 2015, 11, 212–221 CrossRef CAS PubMed.
- J. Rnjak-Kovacina, L. S. Wray, J. M. Golinski and D. L. Kaplan, Adv. Funct. Mater., 2014, 24, 2188–2196 CrossRef CAS.
- D. Franck, Y. G. Chung, J. Coburn, D. L. Kaplan, C. R. Estrada Jr. and J. R. Mauney, J. Tissue Eng., 2014, 5, 2041731414556849 Search PubMed.
- L. S. Wray, K. Tsioris, E. S. Gi, F. G. Omenetto and D. L. Kaplan, Adv. Funct. Mater., 2013, 23, 3404–3412 CrossRef CAS PubMed.
- R. D. Abbott, R. Y. Wang, M. R. Reagan, Y. Chen, F. E. Borowsky, A. Zieba, K. G. Marra, J. P. Rubin, I. M. Ghobrial and D. L. Kaplan, Adv. Healthcare Mater., 2016, 5, 1667–1677 CrossRef CAS PubMed.
- J. E. Brown, J. E. Moreau, A. M. Berman, H. J. McSherry, J. M. Coburn, D. F. Schmidt and D. L. Kaplan, Adv. Healthcare Mater., 2017, 6, 1600762 CrossRef PubMed.
- S. Yan, Q. Zhang, J. Wang, Y. Liu, S. Lu, M. Li and D. L. Kaplan, Acta Biomater., 2013, 9, 6771–6782 CrossRef CAS PubMed.
- A. K. Gupta, J. M. Coburn, J. Davis-Knowlton, E. Kimmerling, D. L. Kaplan and L. Oxburgh, J. Tissue Eng. Regener. Med., 2019, 13, 812–822 CrossRef CAS PubMed.
- C. Correia, S. Bhumiratana, L. P. Yan, A. L. Oliveira, J. M. Gimble, D. Rockwood, D. L. Kaplan, R. A. Sousa, R. L. Reis and G. Vunjak-Novakovic, Acta Biomater., 2012, 8, 2483–2492 CrossRef CAS PubMed.
- J. Rnjak-Kovacina, L. S. Wray, K. A. Burke, T. Torregrosa, J. M. Golinski, W. Huang and D. L. Kaplan, ACS Biomater. Sci. Eng., 2015, 1, 260–270 CrossRef CAS PubMed.
- D. N. Rockwood, R. C. Preda, T. Yucel, X. Wang, M. L. Lovett and D. L. Kaplan, Nat. Protoc., 2011, 6, 1612–1631 CrossRef CAS PubMed.
- L. S. Wray, J. Rnjak-Kovacina, B. B. Mandal, D. F. Schmidt, E. S. Gil and D. L. Kaplan, Biomaterials, 2012, 33, 9214–9224 CrossRef CAS PubMed.
- J. Rnjak-Kovacina, L. S. Wray, K. A. Burke, T. Torregrosa, J. M. Golinski, W. Huang and D. L. Kaplan, ACS Biomater. Sci. Eng., 2015, 1, 260–270 CrossRef CAS PubMed.
- E. Bellas, T. J. Lo, E. P. Fournier, J. E. Brown, R. D. Abbott, E. S. Gil, K. G. Marra, J. P. Rubin, G. G. Leisk and D. L. Kaplan, Adv. Healthcare Mater., 2015, 4, 452–459 CrossRef CAS PubMed.
- Q. Lv and Q. Feng, J. Mater. Sci. Mater. Med., 2006, 17, 1349–1356 CrossRef CAS PubMed.
- K. J. Ornell, K. S. Mistretta, E. Newman, C. Q. Ralston and J. M. Coburn, ACS Biomater. Sci. Eng., 2019, 5, 6742–6754 CrossRef CAS PubMed.
- V. Stankevicius, L. Kunigenas, E. Stankunas, K. Kuodyte, E. Strainiene, J. Cicenas, N. E. Samalavicius and K. Suziedelis, Biochem. Biophys. Res. Commun., 2017, 484, 726–733 CrossRef CAS PubMed.
- V. Sidarovich, M. De Mariano, S. Aveic, M. Pancher, V. Adami, P. Gatto, S. Pizzini, L. Pasini, M. Croce, F. Parodi, F. Cimmino, M. Avitabile, L. Emionite, M. Cilli, S. Ferrini, A. Pagano, M. Capasso, A. Quattrone, G. P. Tonini and L. Longo, Mol. Cancer Ther., 2018, 17, 1405–1415 CrossRef CAS PubMed.
- M. Fischer, M. Skowron and F. Berthold, J. Mol. Diagn., 2005, 7, 89–96 CrossRef CAS.
- I. Garcia, G. Mayol, J. Rios, G. Domenech, N. K. Cheung, A. Oberthuer, M. Fischer, J. M. Maris, G. M. Brodeur, B. Hero, E. Rodriguez, M. Sunol, P. Galvan, C. de Torres, J. Mora and C. Lavarino, Clin. Cancer Res., 2012, 18, 2012–2023 CrossRef CAS PubMed.
- S. A. Roth, O. H. Hald, S. Fuchs, C. Lokke, I. Mikkola, T. Flaegstad, J. Schulte and C. Einvik, Oncotarget, 2018, 9, 18160–18179 CrossRef PubMed.
- S. Raghavan, P. Mehta, E. N. Horst, M. R. Ward, K.
R. Rowley and G. Mehta, Oncotarget, 2016, 7, 16948–16961 CrossRef.
- P. Buchwald, Theor. Biol. Med. Modell., 2009, 6, 5 CrossRef PubMed.
- R. H. Swerdlow, E. Lezi, D. Aires and J. H. Lu, Biochim. Biophys. Acta, 2013, 1830, 2891–2898 CrossRef CAS PubMed.
- J. J. Casciari, S. V. Sotirchos and R. M. Sutherland, Cell Proliferation, 1992, 25, 1–22 CrossRef CAS PubMed.
- P. Han and D. M. Bartels, J. Phys. Chem., 1996, 100, 5597–5602 CrossRef CAS.
- K. L. Eales, K. E. R. Hollinshead and D. A. Tennant, Oncogenesis, 2016, 5, e190 CrossRef CAS PubMed.
- K. Groebe and P. Vaupel, Int. J. Radiat. Oncol., Biol., Phys., 1988, 15, 691–697 CrossRef CAS.
- N. Baek, O. W. Seo, M. Kim, J. Hulme and S. S. A. An, OncoTargets Ther., 2016, 9, 7207–7218 CrossRef CAS PubMed.
- A. Coulon, M. Flahaut, A. Muhlethaler-Mottet, R. Meier, J. Liberman, K. Balmas-Bourloud, K. Nardou, P. Yan, S. Tercier, J. M. Joseph, L. Sommer and N. Gross, Neoplasia, 2011, 13, 991–1004 CrossRef CAS.
- S. H. Cunningham, R. J. Mairs, T. E. Wheldon, P. C. Welsh, G. Vaidyanathan and M. R. Zalutsky, Br. J. Cancer, 1998, 77, 2061–2068 CrossRef CAS PubMed.
- K. A. Fitzgerald, J. F. Guo, E. G. Tierney, C. M. Curtin, M. Malhotra, R. Darcy, F. J. O'Brien and C. M. O'Driscoll, Biomaterials, 2015, 66, 53–66 CrossRef CAS PubMed.
- E. L. S. Fong, S. E. Lamhamedi-Cherradi, E. Burdett, V. Ramamoorthy, A. J. Lazar, F. K. Kasper, M. C. Farach-Carson, D. Vishwamitra, E. G. Demicco, B. A. Menegaz, H. M. Amin, A. G. Mikos and J. A. Ludwig, Proc. Natl. Acad. Sci. U. S. A., 2013, 110, 6500–6505 CrossRef CAS PubMed.
- Y. K. Girard, C. Wang, S. Ravi, M. C. Howell, J. Mallela, M. Alibrahim, R. Green, G. Hellermann, S. S. Mohapatra and S. Mohapatra, PLoS One, 2013, 8, e75345 CrossRef CAS PubMed.
- N. C. Talbot and T. J. Caperna, Cytotechnology, 2015, 67, 873–883 CrossRef CAS PubMed.
- P. Carmeliet and R. K. Jain, Nature, 2000, 407, 249–257 CrossRef CAS PubMed.
- M. C. Lewis, B. D. MacArthur, J. Malda, G. Pettet and C. P. Please, Biotechnol. Bioeng., 2005, 91, 607–615 CrossRef CAS PubMed.
- K. A. Simon, K. M. Park, B. Mosadegh, A. B. Subramaniam, A. D. Mazzeo, P. M. Ngo and G. M. Whitesides, Biomaterials, 2014, 35, 259–268 CrossRef CAS PubMed.
- S. Riffle, R. N. Pandey, M. Albert and R. S. Hegde, BMC Cancer, 2017, 17, 338 CrossRef PubMed.
- K. Stock, M. F. Estrada, S. Vidic, K. Gjerde, A. Rudisch, V. E. Santo, M. Barbier, S. Blom, S. C. Arundkar, I. Selvam, A. Osswald, Y. Stein, S. Gruenewald, C. Brito, W. van Weerden, V. Rotter, E. Boghaert, M. Oren, W. Sommergruber, Y. Chong, R. de Hoogt and R. Graeser, Sci. Rep., 2016, 6, 28951 CrossRef CAS PubMed.
- G. Oliynyk, M. V. Ruiz-Pérez, L. Sainero-Alcolado, J. Dzieran, H. Zirath, H. Gallart-Ayala, C. E. Wheelock, H. J. Johansson, R. Nilsson, J. Lehtiö and M. Arsenian-Henriksson, iScience, 2019, 21, 188–204 CrossRef CAS PubMed.
- O. Warburg, Science, 1956, 123, 309–314 CrossRef CAS PubMed.
- K. M. Cabanillas Stanchi, G. Bruchelt, R. Handgretinger and U. Holzer, Cancer Biol. Ther., 2015, 16, 1353–1363 CrossRef CAS PubMed.
- P. Ren, M. Yue, D. Xiao, R. Xiu, L. Gan, H. Liu and G. Qing, J. Pathol., 2015, 235, 90–100 CrossRef CAS PubMed.
- P. Gao, I. Tchernyshyov, T. C. Chang, Y. S. Lee, K. Kita, T. Ochi, K. I. Zeller, A. M. De Marzo, J. E. Van Eyk, J. T. Mendell and C. V. Dang, Nature, 2009, 458, 762–765 CrossRef CAS PubMed.
- D. R. Wise, R. J. DeBerardinis, A. Mancuso, N. Sayed, X. Y. Zhang, H. K. Pfeiffer, I. Nissim, E. Daikhin, M. Yudkoff, S. B. McMahon and C. B. Thompson, Proc. Natl. Acad. Sci. U. S. A., 2008, 105, 18782–18787 CrossRef CAS PubMed.
- A. Le, A. N. Lane, M. Hamaker, S. Bose, A. Gouw, J. Barbi, T. Tsukamoto, C. J. Rojas, B. S. Slusher, H. Zhang, L. J. Zimmerman, D. C. Liebler, R. J. Slebos, P. K. Lorkiewicz, R. M. Higashi, T. W. Fan and C. V. Dang, Cell Metab., 2012, 15, 110–121 CrossRef CAS PubMed.
- G. Qing, B. Li, A. Vu, N. Skuli, Z. E. Walton, X. Liu, P. A. Mayes, D. R. Wise, C. B. Thompson, J. M. Maris, M. D. Hogarty and M. C. Simon, Cancer Cell, 2012, 22, 631–644 CrossRef CAS PubMed.
- D. Xiao, P. Ren, H. Su, M. Yue, R. Xiu, Y. Hu, H. Liu and G. Qing, Oncotarget, 2015, 6, 40655–40666 CrossRef PubMed.
- C. Commisso, S. M. Davidson, R. G. Soydaner-Azeloglu, S. J. Parker, J. J. Kamphorst, S. Hackett, E. Grabocka, M. Nofal, J. A. Drebin, C. B. Thompson, J. D. Rabinowitz, C. M. Metallo, M. G. Vander Heiden and D. Bar-Sagi, Nature, 2013, 497, 633–637 CrossRef CAS PubMed.
- W. Palm, Y. Park, K. Wright, N. N. Pavlova, D. A. Tuveson and C. B. Thompson, Cell, 2015, 162, 259–270 CrossRef CAS PubMed.
- J. H. Overmeyer, A. M. Young, H. Bhanot and W. A. Maltese, Mol. Cancer, 2011, 10, 69 CrossRef CAS PubMed.
- J. C. Hamann, A. Surcel, R. Chen, C. Teragawa, J. G. Albeck, D. N. Robinson and M. Overholtzer, Cell Rep., 2017, 20, 201–210 CrossRef CAS PubMed.
- S. Jamali, M. Klier, S. Ames, L. F. Barros, R. McKenna, J. W. Deitmer and H. M. Becker, Sci. Rep., 2015, 5, 13605 CrossRef PubMed.
- K. G. de la Cruz-López, L. J. Castro-Muñoz, D. O. Reyes-Hernández, A. García-Carrancá and J. Manzo-Merino, Front. Oncol., 2019, 9, 1143 CrossRef PubMed.
- K. Smallbone, R. A. Gatenby, R. J. Gillies, P. K. Maini and D. J. Gavaghan, J. Theor. Biol., 2007, 244, 703–713 CrossRef CAS PubMed.
Footnote |
† Electronic supplementary information (ESI) available. See DOI: 10.1039/d0bm01153c |
|
This journal is © The Royal Society of Chemistry 2021 |
Click here to see how this site uses Cookies. View our privacy policy here.