DOI:
10.1039/C8LC00547H
(Paper)
Lab Chip, 2018,
18, 3196-3206
An automated in vitro motility assay for high-throughput studies of molecular motors
Received
29th May 2018
, Accepted 31st July 2018
First published on 11th September 2018
Abstract
Molecular motors, essential to force-generation and cargo transport within cells, are invaluable tools for powering nanobiotechnological lab-on-a-chip devices. These devices are based on in vitro motility assays that reconstitute molecular transport with purified motor proteins, requiring a deep understanding of the biophysical properties of motor proteins and thorough optimization to enable motility under varying environmental conditions. Until now, these assays have been prepared manually, severely limiting throughput. To overcome this limitation, we developed an in vitro motility assay where sample preparation, imaging and data evaluation are fully automated, enabling the processing of a 384-well plate within less than three hours. We demonstrate the automated assay for the analysis of peptide inhibitors for kinesin-1 at a wide range of concentrations, revealing that the IAK domain responsible for kinesin-1 auto-inhibition is both necessary and sufficient to decrease the affinity of the motor protein for microtubules, an aspect that was hidden in previous experiments due to scarcity of data.
Introduction
Optimized by a billion years of evolution to work on a nanometer scale, motor proteins have been shown to be ideal tools for biomolecular motor powered diagnostic devices.1–4 Reliable function of such devices requires thorough optimization of sample solutions5 and surface chemistries.6,7 Moreover, molecular motors are important for many vital cellular functions such as axon guidance during neural development and regeneration,8 the resupply of the synapse by axonal transport,9 cell division,10 cellular motility,11 and muscle contraction.12 Consequently, motor proteins are involved in a multitude of diseases: (i) many neurodegenerative diseases are linked to a disruption of axonal transport13 (e.g. Alzheimer's, Amyotrophic Lateral Sclerosis, Huntington and others). (ii) The regeneration of injured nerve fibers requires working axon guidance.14 (iii) Cancer cells depend on a working cytoskeletal machinery for cell division and inhibiting cell motility can decrease cancer invasiveness and formation of metastases.15 Therefore, motor proteins are interesting targets for bio-nanotechnology, basic biological and biophysical research as well as for pharmaceutical studies.
In bio-nanotechnology as well as basic research, in vitro motility assays have been instrumental to studying the biophysical properties of molecular motors.16–26 Most prominently, in so called “gliding motility assays”, cytoskeletal filaments are propelled along a surface by adsorbed motor proteins (see Fig. 1 center). Commonly, such assays are performed in the laboratory by using simple flow-cells where channels are formed by strips of double-sided sticky tape or parafilm sandwiched between two coverslips.27,28 While such assays are well suited for small-scale studies,29,30 their throughput is limited by the number of channels per coverslip (usually a maximum of four) and the necessity to manually exchange solutions in the channels. In pharmaceutical research, high-throughput assays analyzing motor proteins have therefore either relied on measuring ATPase activity31–34 or on observing changes in cell morphology.35 While a number of small-molecule inhibitors for motor proteins were successfully identified, clinical data for these compounds have largely been disappointing, mainly due to incomplete inhibition or rapid formation of resistance.36 To overcome these limitations, detailed studies of the inhibition mechanism are indispensable36 and expensive failures in clinical trials will be avoidable, if the inhibition mechanism is known early during drug development. However, the frequently used ATPase activity only indirectly reflects molecular transport, which is the biologically relevant activity of a motor protein. For example, a reduction of ATPase activity can be caused by i) inhibition of the motor in rigor state (attached to the microtubule), ii) inhibition of the motor in ADP state (detached from the microtubule), or iii) depolymerization of microtubules. An ATPase assay is unable to distinguish between these possibilities whereas an in vitro motility assay will make the difference obvious because the microtubules will i) be stopped but remain attached to the surface, ii) detach from the surface but remain in solution or iii) be completely gone. Consequently, ATPase assays can always only be the starting point for a more thorough analysis requiring additional experiments. They contain little information about the mechanism of inhibition and give no indication of potential off-target effects. Cell morphology screens, on the other hand, are experimentally complex, often hard to interpret and difficult to evaluate automatically. Here, we present a novel assay that greatly improves the throughput of in vitro motility assays, allowing the direct observation of molecular transport by molecular motor proteins. Thus, in addition to providing a powerful tool for advanced biophysical studies, our assay is expected to enable the study of inhibition mechanisms early in the process of screening for drugs targeting molecular motors.
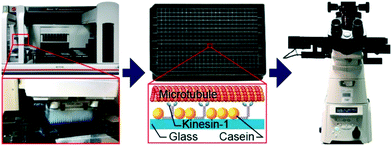 |
| Fig. 1 Automated assay preparation and imaging. The assay is prepared by a pipetting robot (left) in a 384-well plate (center top). Each well contains a microtubule gliding assay where surface-adsorbed kinesin-1 motors propel microtubules along the surface (center bottom). Movement of the fluorescently-labeled microtubules is recorded by a motorized inverted microscope (right). | |
The throughput of our assay allowed us to analyze a wide range of concentrations of peptide inhibitors for kinesin-1, revealing a novel aspect of kinesin-1 autoinhibition.
Results
The assay
Using a pipetting robot (Fig. 1 left), we automated the sample preparation in glass-bottom 384-well plates (Fig. 1 center). Briefly, the pipetting program consisted of four steps (see Methods for more details): (i) surface blocking with casein. (ii) Adhesion of kinesin-1 motor proteins. (iii) Addition of fluorescently labeled microtubules. (iv) Addition of compound solution (without removal of the microtubule solution). In order to improve long-term stability of the assay, we used long-lifetime kinesin-1 expressed in insect cells37 and an oxygen scavenger system that does not affect the pH of the buffer.38 For automated image acquisition, the 384-well plate was transferred to a motorized fluorescence microscope equipped with an autofocus system (Fig. 1 right). The underside of the 384-well plate was silanized with perfluorodecyltrichlorosilane (FDTS), making it highly hydrophobic. Silanization allowed us to use a water-immersion objective because the immersion medium remained attached to the objective and did not spread over the glass bottom of the 384-well plate. Each well was imaged for 11 s at a frame-rate of 1/s. With this setup, it was possible to prepare and image 384 assays in less than two hours. From personal experience, we estimate that in the same time, a well-trained researcher commonly prepares and images roughly 8 assays in conventional flow-cells. In order to cope with the 48-fold increase in data volume, we automated the data analysis. We adapted a MATLAB algorithm39 to run fully automated on a high-performance computing cluster. The applied algorithm identifies microtubule ends in each frame and localizes their positions with sub-pixel resolution (Fig. 2a). This data forms the basis for the analysis of (i) microtubule velocity, (ii) microtubule number (iii) microtubule length, and (iv) microtubule bundling (Fig. 2b–d; see Methods for more details). Data analysis for a 384-well plate was performed in under an hour, depending on available computing power.
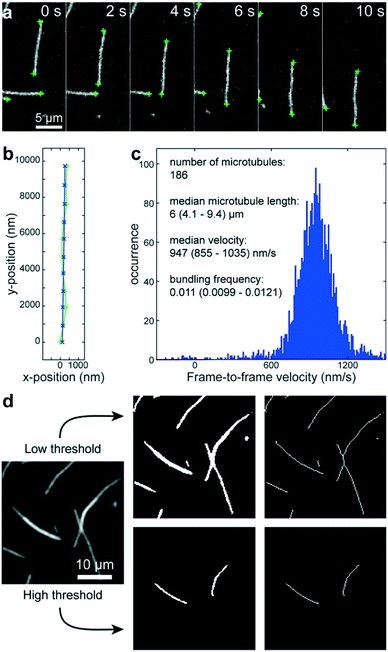 |
| Fig. 2 Automated data analysis. a) Fluorescence micrographs of microtubules moving in an in vitro gliding motility assay. Microtubule ends (green crosses) are identified and localized with sub-pixel resolution by a software algorithm.39 b) The algorithm connects the end positions in consecutive frames into tracks (light green line and + markers) and fits a polynomial path (blue line and x markers) to each track. c) The frame-to-frame distances along the path are used to calculate frame-to-frame velocities for all microtubule ends (blue histogram). The number of microtubules is estimated to be half the visible microtubule ends. The microtubule length is estimated from the distances between the two ends of individual microtubules (assuming that microtubules are mostly straight). d) Workflow of microtubule bundling evaluation (see Methods for details). | |
Validation
To benchmark the performance of our automated assay, we compared results obtained in conventional flow-cells, with results from a 384-well plate (Fig. 3). In the presence of 1 mM ATP, microtubules were moving smoothly at median (and interquartile range) velocities of 830 (774–867) nm s−1 and 947 (855–1035) nm s−1 in the flow-cell and the 384-well plate, respectively (Fig. 3b). The difference in velocity (p < 2 × 10−308) is attributed to a difference in the microscope temperature during imaging40 (25 °C and 27 °C for the flow-cell and the 384-well plate, respectively). Microtubule velocity (Fig. 3c), number (Fig. 3d), length (Fig. 3e) stayed almost constant over the course of four hours in both assays (absolute linear regression slope <0.003 nm s−2, <0.003 1/s, and <0.1 nm s−1 for microtubule velocity, number and length, respectively). Therefore, we can safely assume that results obtained within this time-frame are comparable. Notably, the microtubule density was higher on the 384-well plate, likely because the wells have a higher volume-to-surface ratio than flow-cells. Also, the microtubule length was slightly lower on the 384-well plate; likely because microtubules were sheared during pipetting by the robot. Bundling was negligible in both cases (bundling ratio of 0.018 (0.0137–0.0242) for the 384-well plate and 0 (0–0.0004) for the flow-cell). Microtubule velocity, varied very little between different wells within the 384-well plate as shown for columns 11 and 12 out of 24 for two 384-well plates imaged on two different days (Fig. 3f). We always used two columns of the 384-well plate as internal controls, because our assay is very sensitive to the ambient temperature: using MATLAB's bootci function, we estimated the median (and 95% confidence interval) of an example control well to be 822 (817–830). At 25 °C, a velocity change of 7 nm s−1 corresponds approximately to a temperature difference of 0.05 °C.40
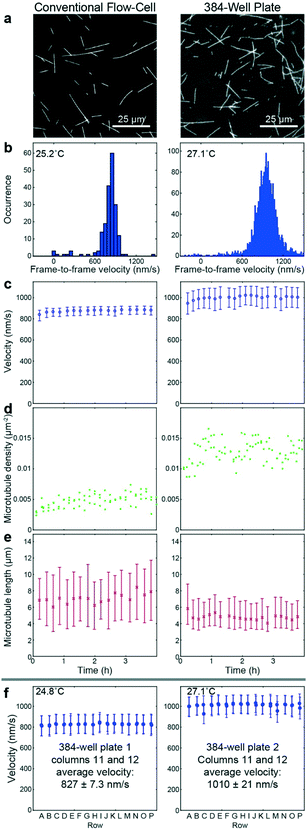 |
| Fig. 3 Assay validation. Microtubule gliding assays prepared manually in a conventional flow-cell (left) and automated in a 384-well plate (right). (a) Fluorescence micrographs. The right image (imaged using a 60× objective) is cropped to match the magnification of the left image (imaged using a 100× objective). (b) Histograms of frame-to-frame velocities of microtubules in the flow-cell (left) and the 384-well plate (right). (c–e) Time dependency of velocity (c), microtubule number (d), microtubule length (e). (f) Example data from individual control wells of columns 11 and 12 of two 384-well plates that were imaged on different days. The given average velocity is the mean and standard deviation of the median velocities of the individual wells. Micrographs were cropped in order to fit multiple micrographs into one panel. (c, e and f) Markers and error bars represent median values and interquartile ranges, respectively. Each data point corresponds to typically 1300 to 2000 (c left), 9000 to 15 000 (c right) or 300–500 (f) frame-to-frame velocities, and 20 to 40 (e left) or 200 to 300 (e right) microtubule length measurements. | |
As positive control for the detection of kinesin-1 inhibition we chose adenylyl-imidodiphosphate (AMPPNP) (Fig. 4), a slowly-hydrolysable ATP analogue. AMPPNP is known to be a competitive inhibitor of kinesin-1 that inhibits the motor in a state where it is strongly bound to the microtubule.41–43 Consequently, AMPPNP significantly decreased the kinesin-1 gliding velocity (linear regression over the first 5 AMPPNP concentrations (±95% confidence bounds): −0.861 ± 0.0057 nm s−1 μM−1) with half-maximal velocity at a concentration of 400 μM (Fig. 4a).
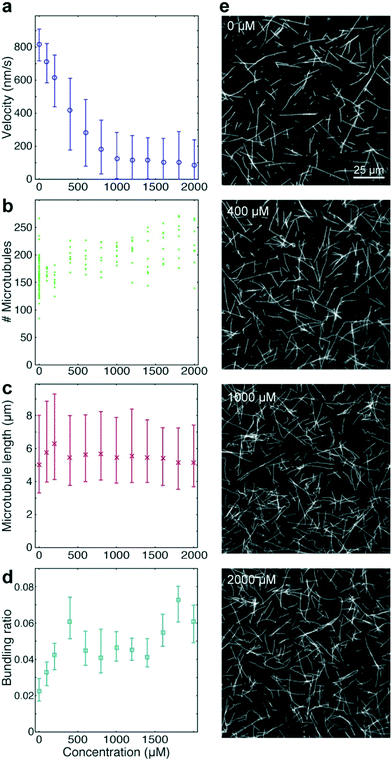 |
| Fig. 4 High-throughput analysis of kinesin-1 inhibition by AMPPNP. Quantified effects of AMPPNP at given concentrations on microtubule velocities (a), microtubule number (b), microtubule length (c), and bundling ratio (d). (f) Fluorescence micrographs show example frames of microtubule gliding assays in the presence of AMPPNP at the given concentrations. (a, c and d) Markers and error bars represent median values and interquartile ranges, respectively. Each data point corresponds to typically 17 000 to 26 000 frame-to-frame velocities (a), 2500 to 3700 microtubule length measurements (c), or 88 images analyzed for bundling (d). | |
At the same time, we observed an increase in microtubule number (Fig. 4b; linear regression: 31 ± 5.5 microtubules per mM AMPPNP) without significant changes in microtubule length (linear regression: −0.2 ± 0.25 μm mM−1 AMPPNP; Fig. 4c), indicating that the increase in microtubule number was caused by an increase in the average affinity between microtubules and kinesin-1 motors. Microtubule bundling was low (Fig. 4d; median bundling ratio 0.05 (0.042–0.065) for constant velocities at AMPPNP concentrations ≥1 mM) and was attributed to random encounters of microtubules with each other due to increased microtubule density.
High-throughput analysis of kinesin-1 inhibition by peptides
Because of the increased throughput of our assay, we were able to measure and analyze the effect of four synthetic peptides (Table 1) at a variety of fine-grained concentration steps in less than three hours. The utilized peptides have not been previously tested for their effect on kinesin-1, but some of them were similar to known kinesin-1 inhibiting peptides. Peptide P1 was the Drosophila melanogaster homolog of the IAK-domain from the tail region of Homo sapiens kinesin-1, known to be responsible for the auto-inhibition of kinesin-1.44–48 Thus P1 was expected to inhibit the motor in a conformation that has low microtubule affinity.44,46 Peptide P2 contained the inhibitory IAK domain from P1 and an adjacent microtubule-binding domain.49
Table 1 Overview of the compounds used in the screening
Name |
Sequence |
Comment |
P1 |
H2N-RGPQAQIAKPIRSGQGAI-CONH2 |
Predicted IAK auto-inhibition domain from drosophila kinesin-1 |
P2 |
H2N-EAVRQKHLGRRGPQAQIAKPIRSGQGAI-CONH2 |
IAK domain (P1) with additional microtubule binding motif |
P3 |
H2N-EAVRQKHLGRRGPQA-CONH2 |
Only additional microtubule binding motif from P2, no IAK domain |
P4 |
H2N-RGKHAGAISQRQPKAIPQEGGVIRAQLR-CONH2 |
Same amino-acid content as P2 but random order |
Peptide P3 contained the same microtubule-binding domain as P2 but no IAK domain. Finally, P4 had the same amino acid content as P2 but the sequence was randomized. 12–36 different concentrations of each peptide were tested in quadruples, so that (together with the AMPPNP controls described above) a total of 384-wells was processed and analyzed (Fig. 5). All peptides were added to gliding assays in the presence of 1 mM ATP.
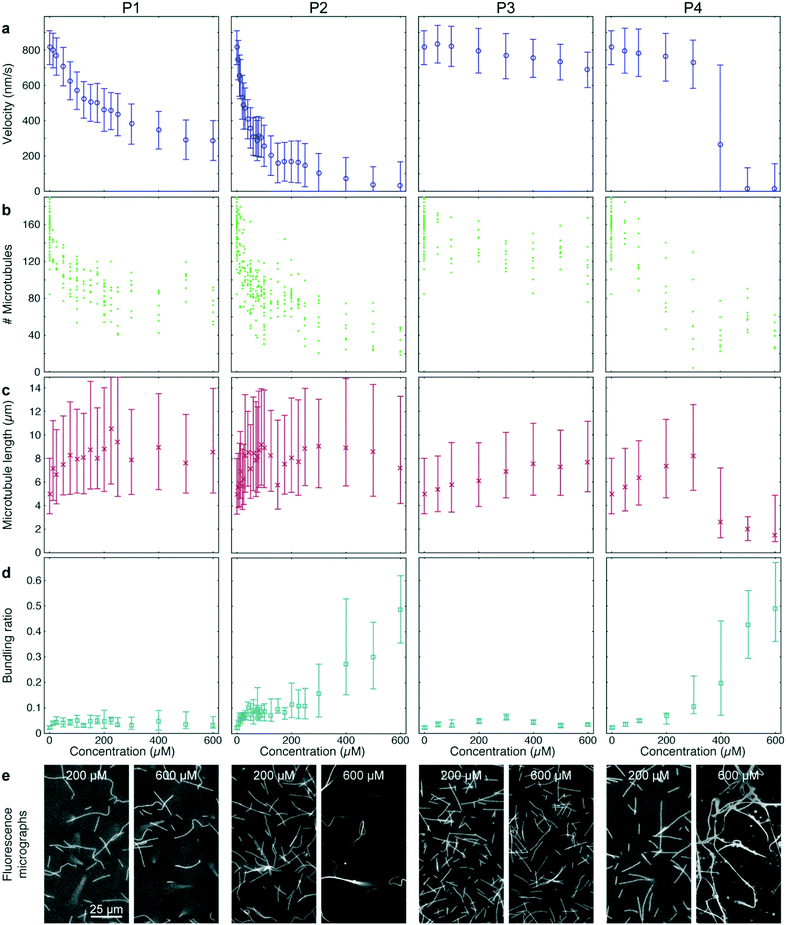 |
| Fig. 5 High-throughput analysis of kinesin-1 inhibition by synthetic peptides. Quantified effects of four peptides (P1, P2, P3, P4) at given concentrations on microtubule velocities (a), microtubule number (b), microtubule length (c) and microtubule bundling (d). (e) Fluorescence micrographs of microtubule gliding assays in the presence of the four peptides at the given concentrations. (a, c and d) Markers and error bars represent median values and interquartile ranges, respectively. Each data point corresponds to typically 4000 to 18 000 frame-to-frame velocities (a), 1500 to 4400 microtubule length measurements (c), or 88 images analyzed for bundling (d). | |
P1 and P2 strongly reduced the microtubule gliding velocity (linear regression over the first 5 peptide concentrations: −1.99 ± 0.049 nm s−1 μM−1 and −13.3 ± 0.19 nm s−1 μM−1 for P1 and P2, respectively), reaching half-maximal velocity at inhibitor concentrations of 250 μM and 40 μM, respectively (Fig. 5a, 1st and 2nd column). In contrast, P3, which was identical to P2 except that it is missing the IAK motif, has only a minimal effect on the microtubule gliding velocity (Fig. 5a, 3rd column; linear regression over the first 5 peptide concentrations: −0.13 ± 0.013 nm s−1 μM−1). For the randomized peptide P4, only a small effect on the gliding velocity was observed for up to 300 μM (linear regression: −0.25 ± 0.017 nm s−1 μM−1). Surprisingly, at concentrations >300 μM, P4 caused a sharp transition to strong velocity inhibition (linear regression 300 μM to 500 μM: −0.887 ± 0.043 nm s−1 μM−1). Examining individual microtubules and bundles at a concentration of 400 μM P4 showed that individual microtubules had an average velocity of 769 (680–861) nm s−1, while bundles had a velocity of 84 (60–132) nm s−1, explaining the large interquartile range of that data point (Fig. 5a 4th column).
Further analysis of the data showed that all peptides caused a decrease in microtubule number (Fig. 5b). The decrease in microtubule number was particularly pronounced for P1 and P2 (linear regression over the first 5 peptide concentrations: −1.0 ± 0.17 microtubules per μM1 and −2.8 ± 0.78 microtubules per μM for P1 and P2, respectively). In contrast, P3 had only a weak effect (linear regression over the first 5 peptide concentrations: −0.14 ± 0.047 microtubules per μM). Notably, P4 caused a sigmoidal decrease of microtubule number: it had no significant effect up to 50 μM (p = 0.597) but sharply reduced the microtubule number at concentrations greater than 50 μM (linear regression 50 μM to 300 μM: −0.40 ± 0.098 microtubules per μM). Conversely, we observed an increase in median microtubule length with increasing peptide concentration (Fig. 5c; linear regression over the first 5 peptide concentrations: 54 ± 1.3 nm μM−1, 70 ± 4.0 nm μM−1, 6.3 ± 0.31 nm μM−1 and 12.9 ± 0.37 nm μM−1 for P1, P2, P3 and P4, respectively).
A closer look at the fluorescence micrographs (Fig. 5e) revealed that P2 and P4 caused strong microtubule bundling, particularly at concentrations of 300 μM and above, we measured a significant increase in microtubule bundling ratio (Fig. 5d; p = 3 × 10−49). At low concentrations only P2 showed a strong increase in microtubule bundling (linear regression over the first 5 peptide concentrations: 0.0025 ± 0.00011 1/μM). P1, P3 and P4 caused only minimal bundling (linear regression over the first 5 peptide concentrations: 3.3 × 10−4 ± 2.9 × 10−5 1/μM, 3.8 × 10−4 ± 2.2 × 10−5 1/μM and 1.31 × 10−4 ± 6.1 × 10−6 1/μM, for P1, P3 and P4, respectively).
Methods
Unless mentioned otherwise, all chemicals were obtained from Sigma-Aldrich.
Expression and purification of tubulin and kinesin-1
Porcine tubulin was purified from porcine brain (Vorwerk Podemus, Dresden, Germany) using established protocols as described previously.50 Kinesin-1 from Drosophila melanogaster was expressed in insect cells and purified as described previously.37
Flow-cell assay preparation
Experiments in flow-cells were performed as previously described.37
384-Well plate preparation
175 μm glass-bottom 384-well plates (Greiner Bio-One) were cleaned by sonication with detergent, ethanol and water as previously described.27 Subsequently, the wells were sealed using adhesive sealing sheets (Thermo Scientific) and the underside of the glass was silanized with FDTS by placing the plate next to a 50 μl drop of FDTS in a desiccator under vacuum for 3 h.
Automated sample preparation
Using glass-bottom 384-well plates, allowed us to automate sample preparation using a pipetting robot (Biomek FXp; Beckman Coulter). The pipetting program consisted of four steps (performed simultaneously for each well using the 384-channel pipetting head of the robot): first the surface of the well was blocked by adding 15 μl of a casein solution (BRB80 (80 mM piperazine-N,N′-bis(2-ethanesulfonic acid) (PIPES)/KOH, pH 6.8, 1 mM ethylene glycol tetraacetic acid (EGTA), 1 mM MgCl2) supplemented with 0.5 mg ml−1 casein). After 3 min incubation, the casein solution was removed and replaced with 15 μl kinesin-1 solution (BRB80 supplemented with 0.2 mg ml−1 casein, 0.004 mg ml−1 kinesin-1, 20 mM dithiothreitol (DTT) and 0.01 mM ATP). After 3 min, the kinesin-1 solution was removed and the well was washed twice with 45 μl casein solution. After removal of the last wash solution, 5 μl of microtubule solution (102 nM polymerized tubulin, 240 mM D-glucose, 0.03 mg ml−1 catalase, 120 mM DTT, 30 μM taxol in BRB80) was added. Finally, 10 μl of inhibitor solution (1.5 mM ATP, 1 mg ml−1 pyranose oxidase and the given concentrations of inhibitor in BRB80) were added without removal of the microtubule solution. Thus, each well contained 15 μl of test solution (34 nM polymerized tubulin, 80 mM D-glucose, 0.01 mg ml−1 catalase, 40 mM DTT, 10 μM taxol, 1 mM ATP, 0.67 mg ml−1 pyranose oxidase and the given concentrations of inhibitor in BRB80). Exchanging solutions took less than 10 s. Also, 5–7 μl of solution remained in each well, ensuring that the well bottoms never dried out during sample preparation. To prevent evaporation after sample preparation and to keep the thickness of the aqueous phase above the glass bottom relatively constant, 15 μl of mineral oil (light oil (neat), BioXtra, suitable for mouse embryo cell culture) were layered on top of the inhibitor solution by the robot.
Automated imaging
Automated imaging was performed on a Nikon Eclipse Ti microscope (Nikon) equipped with a Perfect Focus System (PFS) using a 1.3 PlanApo 60× water immersion objective (Nikon). We used a water-immersion objective in combination with a 384-well plate that had a bottom glass surface silanized with FDTS. That way, the plate bottom was hydrophobic while the objective was hydrophilic, causing the water immersion medium to stick to the objective and not the plate surface, allowing us to image the whole plate with a single drop of deionized water as immersion medium. Each well was imaged for 11 s at a frame rate of 1/s (a total of 12 frames, matching the number of cores in a node of the high-performance computing cluster for optimal parallel analysis). The optimal imaging duration depends on several factors: (i) optical resolution, (ii) signal-to-noise ratio, and (iii) velocity of the microtubules. The first two parameters determine the tracking accuracy (positional error) with which we can measure the position of the microtubule tips. In our current setup, this error is approximately 100 nm. The third parameter determines how far the microtubules move between two frames. The faster the microtubules the lower the relative error in measuring the microtubule velocity. We chose the frame rate and number of frames such that we have a good compromise between being able to detect slow movement of microtubules and total imaging time. If the accuracy of measuring slow movement is not critical, imaging times could be reduced down to 1 s or less. Also, by increasing the labeling and/or the light intensity and by switching to an objective with higher numerical aperture, the tracking accuracy could be improved (and consequently the imaging duration could be reduced).
Each well was imaged close to the center. The oil helped to keep the thickness of the fluid relatively constant over the entire well. Each well contained 15 μl. The area of the well is 20 mm2, resulting in an average fluid thickness of 740 μm. Over the entire area of the well, the fluid was thicker than the working distance of our objective (250 μm), ensuring that the gliding assay at the bottom of the well was not affected by the oil–water interface.
Automated data analysis
We adapted a MATLAB algorithm39 to run fully automated on a high-performance computing cluster (210 Intel Xeon E5-2670v3 12-core processors; maintained by the Scientific Computing Facility at the Max-Planck Institute of Molecular Cell Biology and Genetics). This algorithm identifies microtubule ends in each frame and localizes their positions with sub-pixel resolution (Fig. 2a). This data then forms the basis for three types of information: (i) the microtubule number was estimated as half the number of visible ends. (ii) The microtubule length was estimated for objects that have only two ends (individual microtubules that are not crossing other microtubules) as the distance between the ends. (iii) The microtubule velocity was calculated by connecting individual end positions into tracks (green trace in Fig. 2b) according to a previously published algorithm.39 Subsequently, second-order polynomial paths (blue trace in Fig. 2b) were fitted to these tracks. The distances along the respective paths divided by the time between frames gave the frame-to-frame velocities for each microtubule end (Fig. 2c). By applying two different thresholds, we were able to automatically measure the microtubule bundling ratio in two steps (Fig. 2d): (i) the fluorescence image (Fig. 2d left) was converted to black and white twice: first with a low threshold (1.6 times the standard deviation of the background noise of the images) that captures all microtubules (Fig. 2d center-top) and then with a high threshold that captures only parts of the image that are brighter than a single microtubule (Fig. 2d center-bottom). The high threshold was 1.6 times the median intensity of all microtubule ends in the image. The intensity of the microtubule ends was obtained from the height-parameter of the fit used to determine the position of the microtubule end. (ii) Both black-and-white images were thinned to single-pixel lines using the ‘bwmorph’ MATLAB function51 and single unconnected pixels were deleted (Fig. 2d right). The bundling ratio was then defined as the ratio of the number of white pixels in the respective thinned images, corresponding to the ratio of microtubule bundle length to total microtubule length in the image. It has to be noted, that this algorithm does not distinguish between bundles of two microtubules and bundles of more than two microtubules. Thus, microtubule bundling is underestimated when large bundles are present.
Statistics
All experiments were performed at least twice. For the flow cell experiment (Fig. 3 left), a single flow-cell was imaged at three different positions in 10 min intervals. Within that time, the microtubules travel approximately 5 fields of view. Thus, at each time interval, different microtubules were imaged. For all 384-well plate experiments, each condition was measured in four independent wells (with 88 different conditions plus 32 control wells, each experiment consisted of 384 wells), once 20 min after plate preparation and again 110 min after plate preparation. For the long-term 384-well plate experiment (Fig. 3 right) the plate was measured one additional time after 200 min. Linear regression was performed with the MATLAB ‘polyfit’ functions and 95% confidence intervals were calculated with the polyparci function.52 Statistical significance was determined with the Wilcoxon ranksum test53 using the MATLAB ‘ranksum’ function. Note that, due to double-precision floating point rounding, ‘ranksum’ returns 0 for extremely small values (p < 2 × 10−308). Thus, in these cases it was not possible to give exact p values.
Code availability
The source code used for data evaluation is available on Github: https://github.com/thawn/AutoTipTrack
Data availability
The data that support the findings of this study are available from the corresponding author upon reasonable request.
Discussion
Assay performance
Improved experimental throughput and advanced automated data evaluation algorithms enabled us to obtain and analyze data from hundreds of in vitro microtubule gliding motility assays. An experiment with a full 384-well plate took less than two hours of assay preparation and data acquisition and less than one hour of data analysis. Acquiring and analyzing the same amount of data using a conventional, flow-cell based assay would have required months of work. With the improved oxygen scavenger system using pyranose oxidase instead of glucose oxidase,38 the assay had the same long-term stability and number of stuck microtubules as a highly optimized, well-established gliding motility assay performed in a flow-cell.37 Apart from increased throughput, the assay also significantly improves comparability between results because hundreds of assays can be performed under identical experimental conditions. Automated data evaluation makes full use of the wide spectrum of information that an in vitro motility assay provides. Towards this end, our algorithm is able to automatically evaluate transport velocity, microtubule number, microtubule length and microtubule bundling. This wealth of information enabled a detailed study of the concentration-dependent effect of five different compounds on kinesin-1-driven microtubule motility. A note of caution: because of our experimental setup where inhibitors are added simultaneously to all wells by the robot, but imaging is performed sequentially one well after another over the course of 90 min, the assay in its current form is only suitable for steady-state measurements. Time critical kinetic measurements would require switching to a microscope with integrated pipetting robot which would add the inhibitor solution directly before the start of imaging to each well. In that setup, complex kinetic studies could be performed largely automated.
The validity of our high-throughput approach was confirmed by the results on AMPPNP which agree well with previous results from the literature.41–43 As previously described,42 in contrast to bulk ATPase activity, microtubule gliding velocity in the presence of AMPPNP does not follow a competitive inhibition curve. This is likely because of cooperative effects between the many motors driving a single microtubule.54 The same is true for the observed effects of P1 through P3 on microtubule velocity, which also do not follow standard inhibition kinetics but agree well with previous studies using similar peptides.49,55,56
Kinesin-1 inhibition by peptides from the tail domain
Kinesin-1's tail domain is known to be responsible for reducing the ATPase activity of the motor protein.44 This effect was later pinpointed to the IAK domain, which has been shown to be necessary46 and sufficient49 for reducing kinesin-1 ATPase activity. It has also been shown, that the IAK domain is necessary to reduce, the microtubule-binding affinity of kinesin-1,46 which is believed to be important for reducing needless ATP consumption and preventing impaired transport because of overcrowded microtubules in vivo. However, in vitro motility experiments with kinesin tail-peptides have shown that the IAK domain slows down microtubule gliding,49 which would indicate that the motors stop while they are still attached to the microtubule, hindering transport. To resolve this conundrum, we carefully re-examined the effect of kinesin-1 tail peptides on in vitro motility assays. Because we assessed more information than just the ATPase activity of the motor protein and the velocity of gliding microtubules, we were able to obtain additional information about the mechanism of inhibition. The combination of decrease in microtubule number and increase in microtubule length indicates that the peptides caused a decrease in motor-microtubule affinity: a decreased motor-microtubule affinity acted like a decreased motor density, causing microtubules to detach from the surface. Because short microtubules were held to the surface by fewer motor proteins than long microtubules, short microtubules were the first to detach. This explains the observed decrease in microtubules in conjunction with an increase in the median length of the remaining microtubules. Thus, our results on microtubule number and length demonstrate that P1 – consisting only of the IAK domain – as well as P2 – containing the IAK domain and an additional microtubule binding motif – significantly reduced the motor-microtubule affinity. In contrast, P3 – the microtubule binding motif alone – had only a minimal effect on the affinity. Together, these results show that the IAK domain is not only necessary but also sufficient to reduce the motor-microtubule affinity. While it has been previously demonstrated that the IAK domain is necessary to reduce motor-microtubule affinity,46 to our knowledge, the data presented here is the first direct evidence that the IAK domain is also sufficient to do so. With this finding, we confirmed that the main mechanism of kinesin-1 autoinhibition by the IAK domain was in fact a reduction in motor-microtubule affinity and that the reduction in gliding velocity was merely a minor side-effect of the inhibition that should not have a major impact on transport in vivo.
The reason why previous studies involving in vitro gliding motility assays with similar peptides49,55,56 did not report a decrease in the motor-microtubule affinity is likely because this effect is less obvious than the reduction in the gliding velocity: deducing changes of motor-microtubule affinity requires the simultaneous measurement of microtubule number and length. Moreover, detecting a statistically significant change in these values requires data from several fields of view because one field of view contains only a limited number of microtubules. In contrast, evaluation of the microtubule gliding velocity can be performed very accurately simply by observing a single field of view over a longer time. Thus, the increased throughput and improved data analysis of our assay allowed us to detect an effect that was overlooked in previous small-scale studies.
Microtubule-binding activity of peptides
Quantifying microtubule bundling gave us valuable additional information about the inhibition mechanisms of the peptides: it is known that microtubule bundling can be caused rather non-specifically by electrostatic interaction of a positively charged molecule with the negatively charged microtubule lattice57 or by sequence-specific interaction.58 At concentrations below 300 μM, P2 caused significantly more microtubule bundling than its randomized-sequence counterpart P4. Both peptides have the same net charge (predicted to be +6 at neutral pH). Therefore, the difference in bundling must be caused by sequence-specific binding of P2 to microtubules. In contrast, at higher concentrations, both peptides caused similar bundling. At these concentrations, it is likely that the electrostatic interactions of the positively charged peptides prevailed over any sequence-specific interactions. The strong bundling caused by high concentrations of P4 coincided with a sudden decrease in microtubule velocity, indicating that the interaction of P4 with the microtubule rather than with the motor protein was the cause for the observed slow-down. This conclusion is also supported by the observation that at 400 μM P4, individual microtubules were much faster than bundles. The observations made for P4 are a good example, where our assay provided additional information that helped prevent the misinterpretation that a reduced motor activity was caused by the interaction of the peptide with the motor-protein, which would have been impossible with an ATPase assay.
Quantification of microtubule bundling was important for proper interpretation of the microtubule number and the microtubule length because it is impossible to precisely measure these values for individual microtubules in a bundle. We found that at bundling ratios greater than 0.2, values for microtubule length and microtubule number became unreliable as illustrated by the sharp decline in the measured microtubule length for concentrations of 400 μM P4 and above (Fig. 5c, 4th column).
Notably, in a previous study, a 64 amino-acid-long tail peptide of kinesin-1 did not decrease but increase the motor-microtubule affinity.44 However, the peptide used in that study is much longer and has seven additional positively charged residues, which likely further strengthened the electrostatic interaction between the peptide and the negatively charged microtubule lattice,59 overcoming the effect of the IAK domain.
Conclusions
In summary, our high-throughput automated microtubule gliding assay was successfully validated by reproducing known effects of AMPPNP and other peptide inhibitors on the kinesin-1 motor domain. The increased throughput of our assay will enable the rapid optimization of biomolecular motor-powered lab-on-a-chip devices. For example, our assay makes it easy to optimize sample solutions to find optimal conditions for the used motor protein. Moreover, it should be possible to adapt the assay to other kinesin-family motors, and even myosin60 (in conjunction with gliding actin filaments) to find the motor protein that is optimal for a given lab-on-a-chip device.
Because of the increased amount of data and the very rigorous automated data evaluation algorithm, we were able to obtain additional new information about the interaction between motor protein and microtubule as well as about the interaction between the studied peptides and microtubules. This allowed us to further elucidate the biophysical mechanism of kinesin-1 auto-inhibition. That way, we demonstrated that our assay is uniquely suited for biophysical studies of motor proteins.
Because our assay works with an immersion objective, it can be combined with TIRF microscopy, making it sensitive enough to detect single molecules. Therefore it should be possible to adapt the assay to a stepping geometry where the microtubules are immobilized on the surface and the movement of individual motor proteins along the microtubules is analyzed.28 Thus, our assay has the potential to provide a paradigm shift in how biophysical studies are performed: from highly customized, specialized assays to a more standardized assay that can quickly provide and analyze massive amounts of data, making it easier to test many different conditions. The increased throughput will facilitate faster testing of hypotheses and development of theoretical models in biophysics.
In addition, the increased throughput, for the first time, enables the use of in vitro motility assays for pharmaceutical studies. Because our assay allows a multidimensional analysis of the physiological function of motor proteins, it will help to get a comprehensive picture of the pharmacodynamics of drug candidates targeting such proteins. This will reduce the probability of false-positive and false-negative results as well as give good indications of off-target effects. Because of its comprehensiveness and considering its throughput, we believe that our assay is well suited for hit validation resulting from fast and extremely high-throughput methods such as thermophoresis,61 DNA encoded combinatorial libraries62 or in silico modeling.63
Conflicts of interest
There are no conflicts to declare.
Acknowledgements
The authors would like to thank the following services and facilities: Technology Development Studio (TDS), Protein Expression Facility and Scientific Computing Facility of the Max Planck Institute of Molecular Cell Biology and Genetics; Biomolecular Synthesis Facility of B CUBE — Center for Molecular Bioengineering, Technische Universität Dresden. Furthermore, financial support from the European Union's Seventh Framework programme under grant agreement number 613044 (ABACUS) and Horizon 2020 research and innovation programme under grant agreement number 732482 (Bio4Comp), the European Research Council (Starting Grant No. 242933, NanoTrans), the European Social Funds (Grant No. 100111059, MindNano and Grant No. 100107464, ChemIT), and the German Research Foundation (Cluster of Excellence Center for Advancing Electronics Dresden and the Heisenberg Program) is acknowledged.
References
- T. Fischer, A. Agarwal and H. Hess, Nat. Nanotechnol., 2009, 4, 162–166 CrossRef PubMed.
- P. Katira and H. Hess, Nano Lett., 2010, 10, 567–572 CrossRef PubMed.
- T. Korten and S. Diez, Lab Chip, 2008, 8, 1441–1447 RSC.
- T. Korten, A. Månsson and S. Diez, Curr. Opin. Biotechnol., 2010, 21, 477–488 CrossRef PubMed.
- S. Korten, N. Albet-Torres, F. Paderi, L. ten Siethoff, S. Diez, T. Korten, G. te Kronnie and A. Månsson, Lab Chip, 2013, 13, 866–876 RSC.
- J. Clemmens, H. Hess, R. Lipscomb, Y. Hanein, K. F. Bohringer, C. M. Matzke, G. D. Bachand, B. C. Bunker and V. Vogel, Langmuir, 2003, 19, 10967–10974 CrossRef.
- M. G. L. van den Heuvel, C. T. Butcher, R. M. M. Smeets, S. Diez and C. Dekker, Nano Lett., 2005, 5, 1117–1122 CrossRef PubMed.
- L. A. Lowery and D. V. Vactor, Nat. Rev. Mol. Cell Biol., 2009, 10, 332–343 CrossRef PubMed.
-
M. M. Black, in Methods in Cell Biology, ed. K. K. Pfister, Academic Press, 2016, vol. 131, pp. 1–19 Search PubMed.
- J. M. Scholey, I. Brust-Mascher and A. Mogilner, Nature, 2003, 422, 746–752 CrossRef PubMed.
- T. D. Pollard, Nature, 2003, 422, 741–745 CrossRef PubMed.
- I. Morano, J. Mol. Med., 2003, 81, 481–487 CrossRef PubMed.
- E. Chevalier-Larsen and E. L. F. Holzbaur, Biochim. Biophys. Acta, Mol. Basis Dis., 2006, 1762, 1094–1108 CrossRef PubMed.
- J. W. Faweett and A. R. J. Keynes, Annu. Rev. Neurosci., 1990, 13, 43–60 CrossRef PubMed.
- E. Sahai, Curr. Opin. Genet. Dev., 2005, 15, 87–96 CrossRef PubMed.
- C. L. Asbury, A. N. Fehr and S. M. Block, Science, 2003, 302, 2130–2134 CrossRef PubMed.
- V. Bormuth, V. Varga, J. Howard and E. Schäffer, Science, 2009, 325, 870–873 CrossRef PubMed.
- V. Bormuth, B. Nitzsche, F. Ruhnow, A. Mitra, M. Storch, B. Rammner, J. Howard and S. Diez, Biophys. J., 2012, 103, L4–L6 CrossRef PubMed.
- M. Braun, Z. Lansky, S. Bajer, G. Fink, A. A. Kasprzak and S. Diez, Cytoskeleton, 2013, 70, 515–521 CrossRef PubMed.
- D. L. Coy, M. Wagenbach and J. Howard, J. Biol. Chem., 1999, 274, 3667–3671 CrossRef PubMed.
- G. Fink, L. Hajdo, K. J. Skowronek, C. Reuther, A. A. Kasprzak and S. Diez, Nat. Cell Biol., 2009, 11, 717–723 CrossRef PubMed.
- J. Howard, A. J. Hudspeth and R. D. Vale, Nature, 1989, 342, 154–158 CrossRef PubMed.
- B. Nitzsche, E. Dudek, L. Hajdo, A. A. Kasprzak, A. Vilfan and S. Diez, Proc. Natl. Acad. Sci. U. S. A., 2016, 113, E6582–E6589 CrossRef PubMed.
- L. Scharrel, R. Ma, R. Schneider, F. Jülicher and S. Diez, Biophys. J., 2014, 107, 365–372 CrossRef PubMed.
- R. Schneider, T. Korten, W. J. Walter and S. Diez, Biophys. J., 2015, 108, 2249–2257 CrossRef PubMed.
- V. Varga, C. Leduc, V. Bormuth, S. Diez and J. Howard, Cell, 2009, 138, 1174–1183 CrossRef PubMed.
- B. Nitzsche, V. Bormuth, C. Bräuer, J. Howard, L. Ionov, J. Kerssemakers, T. Korten, C. Leduc, F. Ruhnow and S. Diez, Methods Cell Biol., 2010, 95, 247–271 Search PubMed.
- T. Korten, B. Nitzsche, C. Gell, F. Ruhnow, C. Leduc and S. Diez, Methods Mol. Biol., 2011, 783, 121–137 CrossRef PubMed.
- K. J. Böhm, P. Steinmetzer, A. Daniel, M. Baum, W. Vater and E. Unger, Cell Motil. Cytoskeleton, 1997, 37, 226–231 CrossRef.
- R. Sakowicz, M. S. Berdelis, K. Ray, C. L. Blackburn, C. Hopmann, D. J. Faulkner and L. S. Goldstein, Science, 1998, 280, 292–295 CrossRef PubMed.
- S. DeBonis, D. A. Skoufias, L. Lebeau, R. Lopez, G. Robin, R. L. Margolis, R. H. Wade and F. Kozielski, Mol. Cancer Ther., 2004, 3, 1079–1090 Search PubMed.
- J. C. Funk, A. S. Davis, J. A. Hopkins and K. M. Middleton, Anal. Biochem., 2004, 329, 68–76 CrossRef PubMed.
- C. D. Cox, M. J. Breslin, B. J. Mariano, P. J. Coleman, C. A. Buser, E. S. Walsh, K. Hamilton, H. E. Huber, N. E. Kohl, M. Torrent, Y. Yan, L. C. Kuo and G. D. Hartman, Bioorg. Med. Chem. Lett., 2005, 15, 2041–2045 CrossRef PubMed.
- C. A. Parrish, N. D. Adams, K. R. Auger, J. L. Burgess, J. D. Carson, A. M. Chaudhari, R. A. Copeland, M. A. Diamond, C. A. Donatelli, K. J. Duffy, L. F. Faucette, J. T. Finer, W. F. Huffman, E. D. Hugger, J. R. Jackson, S. D. Knight, L. Luo, M. L. Moore, K. A. Newlander, L. H. Ridgers, R. Sakowicz, A. N. Shaw, C.-M. M. Sung, D. Sutton, K. W. Wood, S.-Y. Zhang, M. N. Zimmerman and D. Dhanak, J. Med. Chem., 2007, 50, 4939–4952 CrossRef PubMed.
- T. U. Mayer, T. M. Kapoor, S. J. Haggarty, R. W. King, S. L. Schreiber and T. J. Mitchison, Science, 1999, 286, 971–974 CrossRef PubMed.
- S. M. Myers and I. Collins, Future Med. Chem., 2016, 8, 463–489 CrossRef PubMed.
- T. Korten, S. Chaudhuri, E. Tavkin, M. Braun and S. Diez, IEEE Trans. NanoBiosci., 2016, 15, 62–69 Search PubMed.
- M. Swoboda, J. Henig, H. M. Cheng, D. Brugger, D. Haltrich, N. Plumere and M. Schlierf, ACS Nano, 2012, 6, 6364–6369 CrossRef PubMed.
- F. Ruhnow, D. Zwicker and S. Diez, Biophys. J., 2011, 100, 2820–2828 CrossRef PubMed.
- V. Schroeder, T. Korten, H. Linke, S. Diez and I. Maximov, Nano Lett., 2013, 13, 3434–3438 CrossRef PubMed.
- S. A. Kuznetsov and V. I. Gelfand, Proc. Natl. Acad. Sci. U. S. A., 1986, 83, 8530–8534 CrossRef.
- S. A. Cohn, A. L. Ingold and J. M. Scholey, J. Biol. Chem., 1989, 264, 4290–4297 Search PubMed.
- K. Kawaguchi and S. I. Ishiwata, Science, 2001, 291, 667–669 CrossRef PubMed.
- D. L. Coy, W. O. Hancock, M. Wagenbach and J. Howard, Nat. Cell Biol., 1999, 1, 288–292 CrossRef PubMed.
- M. F. Stock, J. Guerrero, B. Cobb, C. T. Eggers, T.-G. Huang, X. Li and D. D. Hackney, J. Biol. Chem., 1999, 274, 14617–14623 CrossRef PubMed.
- D. D. Hackney and M. F. Stock, Nat. Cell Biol., 2000, 2, 257–260 CrossRef PubMed.
- D. D. Hackney, N. Baek and A. C. Snyder, Biochemistry, 2009, 48, 3448–3456 CrossRef PubMed.
- H. Y. K. Kaan, D. D. Hackney and F. Kozielski, Science, 2011, 333, 883–885 CrossRef PubMed.
- H. Yonekura, A. Nomura, H. Ozawa, Y. Tatsu, N. Yumoto and T. Q. P. Uyeda, Biochem. Biophys. Res. Commun., 2006, 343, 420–427 CrossRef PubMed.
- M. Castoldi and A. V. Popov, Protein Expression Purif., 2003, 32, 83–88 CrossRef PubMed.
- L. Lam, S.-W. Lee and C. Y. Suen, IEEE Trans. Pattern Anal. Mach. Intell., 1992, 14, 869–885 CrossRef.
-
S. Strider, polyparci - File Exchange - MATLAB Central, http://de.mathworks.com/matlabcentral/fileexchange/39126-polyparci.
-
J. D. Gibbons and S. Chakraborti, in International Encyclopedia of Statistical Science, ed. M. Lovric, Springer, Berlin Heidelberg, 2011, pp. 977–979 Search PubMed.
- C. Leduc, N. Pavin, F. Jülicher and S. Diez, Phys. Rev. Lett., 2010, 105, 128103 CrossRef PubMed.
- A. Nomura, T. Q. P. Uyeda, N. Yumoto and Y. Tatsu, Chem. Commun., 2006, 3588–3590 RSC.
- K. R. S. Kumar, T. Kamei, T. Fukaminato and N. Tamaoki, ACS Nano, 2014, 8, 4157–4165 CrossRef PubMed.
- D. J. Needleman, M. A. Ojeda-Lopez, U. Raviv, H. P. Miller, L. Wilson and C. R. Safinya, Proc. Natl. Acad. Sci. U. S. A., 2004, 101, 16099–16103 CrossRef PubMed.
- C. W. Scott, A. B. Klika, M. M. S. Lo, T. E. Norris and C. B. Caputo, J. Neurosci. Res., 1992, 33, 19–29 CrossRef PubMed.
- M. A. Seeger and S. E. Rice, J. Biol. Chem., 2010, 285, 8155–8162 CrossRef PubMed.
- N. Albet-Torres and A. Månsson, Langmuir, 2011, 27, 7108–7112 CrossRef PubMed.
- P. Striebeck and D. J. Manstein, FASEB J., 2012, 26, 964.966 Search PubMed.
- F. V. Reddavide, W. Lin, S. Lehnert and Y. Zhang, Angew. Chem., Int. Ed., 2015, 54, 7924–7928 CrossRef PubMed.
- S. C. Hopkins, R. D. Vale and I. D. Kuntz, Biochemistry, 2000, 39, 2805–2814 CrossRef PubMed.
|
This journal is © The Royal Society of Chemistry 2018 |
Click here to see how this site uses Cookies. View our privacy policy here.