DOI:
10.1039/C8LC00039E
(Perspective)
Lab Chip, 2018,
18, 1831-1837
Maximizing the impact of microphysiological systems with in vitro–in vivo translation
Received
14th January 2018
, Accepted 12th May 2018
First published on 4th June 2018
Abstract
Microphysiological systems (MPS) hold promise for improving therapeutic drug approval rates by providing more physiological, human-based, in vitro assays for preclinical drug development activities compared to traditional in vitro and animal models. Here, we first summarize why MPSs are needed in pharmaceutical development, and examine how MPS technologies can be utilized to improve preclinical efforts. We then provide the perspective that the full impact of MPS technologies will be realized only when robust approaches for in vitro–in vivo (MPS-to-human) translation are developed and utilized, and explain how the burgeoning field of quantitative systems pharmacology (QSP) can fill that need.
1. Why MPSs are needed in pharmaceutical development
The field of Microphysiological Systems (MPS)—also called tissue chips or physiome-, organ-, or human-on-a-chip—is developing quickly, fueled by strong support from federal sources, the pharmaceutical industry's continuing need for more predictive preclinical models, and promising early results from academic laboratories. Functional MPSs representing tissues from numerous organs including liver, lung, gut, skin, muscle, bone, brain, and more have been reported in the last decade.1–3 These engineered, biomimetic constructs are typically micro- or meso-fluidic scale devices that are designed to better emulate in vitro the biology of human tissues and organs by utilizing human cells, especially primary or induced pluripotent stem cell (iPSC)-derived cells; three-dimensional micro-architectures and biologically based substrates and compatible materials; multiple cell co-culture; long-term (weeks+) tissue functionality; and more physiological mechanical and chemical cues. In a sign the field is maturing, transferability to and reproducibility by second parties are being systematically tested, e.g., via the Tissue Chip Testing Centers in the US,4,5 and offerings of commercial MPS products and services have begun.1
While basic research on biological mechanisms is already benefiting, a major motivation for the field is the continuing challenge of translating preclinical knowledge to human clinical outcomes in the pharmaceutical industry. That approximately 9 of 10 drug candidates entering clinical trials fail to gain approval is well-documented.6,7 Clearly, new approaches to improve these outcomes are sorely needed.
Understanding why drug candidates fail and how other developments have impacted success rates can be instructive for maximizing the impact of new technologies such as MPSs. An analysis of the BioMedTracker database from 2003–2011 for 835 companies and 4451 drugs found that the root causes for suspended programs at phase 3 were 54% efficacy, 9% safety, 18% commercial and 19% unknown, and at NDA/BLA (new drug application/biologics license application) were 58% efficacy, 31% safety, 8% commercial and 13% unknown.7 In a separate analysis for 2011–2012 of data from Thomson Reuters, Drugs of Today, Arrowsmith found that failures from phase II through submission were attributed 56% to efficacy, 28% safety, 7% strategic, 5% commercial and 5% operations.6 More granularly, phase II failures were attributed to 59% efficacy, 22% safety, 16% strategic, and 3% other, and phase III and submission failures were attributed 52% to efficacy, 35% safety and 13% other. With failure rates in phase I of ∼35% (ref. 7) being presumably mainly from safety, cumulatively from phase I to submission about 50% of all failures are from safety. A majority of the most expensive failures (in phases II and III), however, are associated with efficacy. Nonetheless, it should be recognized that toxicity in early phases often limits dosing levels in later phases, with subsequent failures then attributed to lack of efficacy rather than toxicity. Regardless, a significant need for improved prediction clearly remains on both fronts. Interestingly, a substantial portion of failures in phases II and III are attributed to strategy, financial, and commercial reasons, so obtaining better information to rationalize decisions at the earliest stages could also reduce late, expensive program cancellations.
Despite the overall statistics, improvements in certain areas have been made. The attrition from phase 1 entrance to regulatory submission due to pharmacokinetics (PK) and bioavailability was reduced from about 40% in 1991 to less than 10% in 2000, and below 1% in phases II and III by 2007–2011.6,8–10 Clearly, the concerted efforts in the 1990’s to assess ADME/DMPK (absorption, distribution, metabolism, excretion/drug metabolism and pharmacokinetics) much earlier as part of the lead qualification process, coupled with new technologies making that possible (e.g., combinatorial chemistry and high throughput screening), markedly reduced PK/bioavailability as a significant cause of clinical trial failure.11 More recently, the use of biomarkers for clinical trial patient selection has demonstrably improved success rates: drug development programs that used such biomarkers had 25.9% likelihood of approval from phase I to approval while those that didn't had only 8.4% approval rates,12 with benefits found at every phase transition. As patient selection biomarkers are typically related to drug mechanism-of-action, these data suggest that improving understanding of mechanisms can be highly beneficial to clinical success, if utilized in trial decision making.
These reasons of pharmaceutical success and failure provide guidance to where MPS technology might best be applied to impact translation of preclinical results to clinical success. Given the current state of MPS technology—showing promise but not fully demonstrated—our aims in this Perspective are to examine how MPS technologies could improve preclinical-to-clinical translation, and to show how utilizing a quantitative systems pharmacology (QSP) framework would be highly beneficial in those efforts.
2. How MPS technologies may improve preclinical drug discovery efforts
MPS technologies provide new in vitro approaches, early in the drug discovery and development process, to understand disease mechanisms and progression and assess drug–biology interactions in a more physiologically-relevant manner. The advantages compared to traditional cell cultures and animal testing are numerous. The increased tissue complexity in disease MPSs provides a more realistic assessment platform than immortalized cell lines and two-dimensional monocultures common to in vitro assays, and may suffice as alternatives for animal models in some cases. The use of human cell sources, including primary cells and iPSCs, also eliminates the issue of cross-species specificity that often confounds results from animal models. MPSs or multi-MPS platforms provide new testbeds in early development for evaluating potential drug–drug interactions as well as dosing and dose–response relationships of drug combinations for both efficacy and toxicity purposes. Assessment of responses may be in the form of measuring chemical entities via sampling of media or tissue or in some MPSs via rapid, noninvasive measurements such as electrical and mechanical outputs (e.g., neural action potentials and muscular contractile activity13). MPS models of disease have potential for discovery of response biomarkers. Further, tissue or cell sourcing from multiple donors could provide insights into patient variability and enable patient stratification regarding who will benefit most from a therapy. Sourcing from human iPSCs provides additional advantages for disease modeling, such as monogenetic disorders for studies of disease mechanism and drug responses, as well as generating the equivalent of a patient population harboring the same mutation. Examples of MPSs focused on disease biology include efforts to represent cancer metastasis in liver,14,15 thrombosis in lung,16 chronic obstructive pulmonary disease in lung,17 Barth syndrome in heart,18 diabetes in an islet-liver pair,19 non-alcoholic fatty liver disease (NAFLD)20 and systemic inflammation in interconnected gut and liver MPSs.21 Complex diseases that involve multiple organs, such as diabetes and metabolic syndrome, can now be modeled by platforms comprising multiple interconnected, interacting MPSs. Within an MPS or multi-MPS platforms, sourcing all cells from a single patient or differentiated from a single iPSC source is desirable for precision medicine applications. Although some therapeutic areas have substantially better drug approval rates than others, all have great room for improvement and so none are particularly more or less valuable targets for MPS development.6,8–10,12
The characteristics of MPSs that hold promise for efficacy studies may also improve early in vitro assessment of adverse effects. For example, immune-competent MPSs would enable studies of the effect of immune cells on drug–biology interactions. Inflammation is hypothesized to be a major cause of idiosyncratic drug toxicity in humans22,23 and appropriately designed immune-competent MPSs could enable much earlier assessment than presently available. Additionally, numerous MPSs are being designed to operate for weeks or months, which could improve the in vitro assessment of chronic toxicities. Evaluation of toxic response markers over time could provide guidance for clinical patient monitoring. This promise notwithstanding, MPSs tend to be more operationally complex and resource intensive to use than typical in vitro toxicity screens, so the most realistic applications may be in assessment of toxicities related to multi-cell or -organ interactions that are difficult to detect with simpler but higher throughput assays.
While failures due directly to PK and bioavailability have been greatly reduced, drug safety and efficacy are directly related to dose, exposure, biotransformation to active metabolites, and uptake at sites of action, so improved early evaluation of PK and drug disposition can still impact preclinical-to-clinical translation. For example, for drug metabolism modes that utilize ubiquitously expressed enzymes instead of CYP or UGT (which are adequately modeled in liver-derived cultures),24,25 a grouping of MPSs representing liver, kidney, gut, lung, heart, fat, etc., could be used to assess drug disappearance in many tissues.26 Further, MPSs utilizing primary human cell sources may enable more relevant assessment than cell lines, for example, BBB or kidney MPSs27,28 utilizing primary or iPSC-derived cells compared to existing in vitro models using MDCK-MDR1 or HK-2 cell lines, respectively.
An essential requirement for pharmacologic applications of MPSs is the quantitative evaluation of drug behavior and tissue responses with markers and endpoints as relevant to the clinic as possible, and subsequent in vitro–in vivo translation of these findings to human. The utility of computational models to design MPS technologies and analyze MPS data is well-demonstrated,26,30–34 however, there is still a scientific gap on how to correlate, extrapolate or otherwise translate quantitative MPS data to human clinical outcomes. In the following sections, we describe how quantitative systems pharmacology (QSP) could and should be utilized to fill that gap.
3. Quantitative systems pharmacology (QSP) is coming of age
Despite MPSs better representing human physiology, some level of post-experimental analysis is needed because MPSs are still in vitro systems that lack true systemic blood flow, waste removal, hormonal and immune systems, a microbiome, and many other defining in vivo contextual systems and cues. While some measures from MPSs may scale easily from in vitro to in vivo, for example based on relative cell numbers, many have no direct analog in vivo and will require more sophisticated analysis to determine meaning for the in vivo context. We believe QSP is a powerful, integrative framework for such interpretation and translation efforts (Fig. 1).
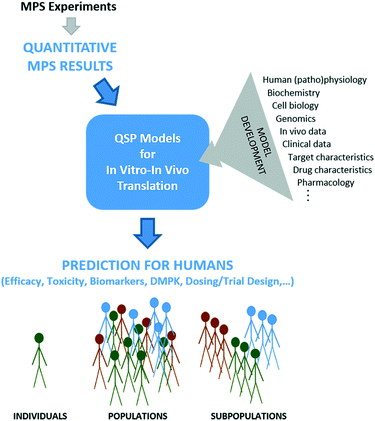 |
| Fig. 1 Quantitative systems pharmacology models of the biology and pharmacology for specific applications can be used to translate in vitro results from MPSs into the in vivo human context. QSP models are initially developed based on an array of biological and (patho)physiological data as well as information about target and drug characteristics (upper right). Then, quantitative information derived from MPS experimentation provides values or ranges of specific parameters to the models (top). MPS results might relate specifically to drug activity or might provide biology-specific parameters with which to define virtual patient biology for simulations of drug activity derived from other sources. Simulation and analysis using MPS inputs are then possible to predict aspects of drug efficacy, toxicity, DMPK, etc., for virtual patients (individuals, populations, subpopulations) accounting for knowledge gained from MPSs (bottom). | |
QSP can be defined as the “quantitative analysis of the dynamic interactions between drug(s) and a biological system that aims to understand the behavior of the system as a whole, as opposed to the behavior of its individual constituents”.35 As such, QSP thinking goes beyond traditional pharmacokinetic/pharmacodynamics (PKPD) modeling to integrate more mechanistic information from a broader range of sources, such as mechanistic in vitro data (e.g., from MPSs), target characteristics, drug properties, in vivo data, human physiology, genetic information, human pathology and prior clinical data.36
The transition from standard PK models to physiologically-based PK (PBPK) models is a major example of moving towards “systems thinking,” by placing preclinical data into a more complete human context. Use of PBPK models in pharmaceutical development is increasing rapidly.37,38 Integrating PBPK modeling with mechanistic models of relevant biology and PD will enable the interrogation of mechanisms of action and response to drugs for diverse dosing regimens, while accounting for interspecies, population, and disease state differences.
Several examples demonstrate how QSP modeling can incorporate numerous data sources into a quantitative mechanistic framework to test hypotheses, explore the relative importance of uncertainties, and extend understanding to multiple patient populations. While these examples do not utilize data from MPSs, the possibility to do so is clear.
In one of the most far reaching, integrative QSP efforts within the pharmaceutical industry to date, Kirouac et al. developed a mechanism-based model of the MAPK signaling network in a BRAFV600-mutant colorectal cancer to investigate the potential benefit of combining three oncology drugs with targets on the same biochemical pathway.39 The QSP model integrates information from multiple sources on multiple scales (cellular biochemistry, genetics, in vitro cell growth, in vivo tumor kinetics in patients and xenograft models, and clinical tumor responses). With it, they identified mechanisms of differential activity of MEK and ERK inhibitors as well as differential effects of supra-physiological MEK activity on resistance to MEK, ERK, and RAF inhibitors. They simulated combination dose–response matrices for the three drugs to inform combination studies for multiple cancer indications, and identified alternate dosing regimens that might have better tolerability. They report they are using the results to strategize indications and drug combination regimens for clinical trials.
An example of the success of a QSP framework for toxicity applications is DILIsym®, a mathematical model for predicting drug induced liver injury (DILI) from mechanistic in vitro toxicity assays such as those for bile acid transporter inhibition, mitochondrial oxygen consumption rate and oxidative stress.40 DILIsym® comprises multiple interacting sub-models (e.g., reactive metabolite generation, immune responses, bile acid homeostasis) to simulate the effect of a drug on the liver as a whole across multiple mechanisms of toxicity. Important insights into risks and mechanisms of DILI have been gained through its use regarding the toxicity and relative DILI risk of multiple drugs, as well as insights into species differences.41–43 DILIsym® is a particularly good example of integrating experimental and modeling paradigms, in that the toxicity assays provide quantitative metrics and the model utilizes these as inputs to its mechanistic algorithm to translate in vitro measurements into risk of hepatotoxicity in vivo.
Another example is a study used to extend the intravenous application of Tamiflu to infants as young as 2 weeks, a population at increased risk of complications and death from influenza.44,45 Complementing the existing data on oral treatment in children under 2 years and toxicology data in newborn animals, an age-dependent PBPK model of the prodrug and its metabolite in both monkey and human was utilized to demonstrate that intravenous infusions of Tamiflu would produce plasma profiles similar to oral doses and thereby not have higher safety risks. This new analysis provided the basis for the US Food and Drug Administration to forego requiring an additional toxicological study and to approve Tamiflu treatment of influenza in infants as young as 2 weeks.44
As these examples illustrate, QSP models that account for human biology and (patho)physiology as well as drug characteristics can provide actionable insights at multiple stages of drug development. Other reviews and publications provide additional examples.35,40,46–50
4. Maximizing MPS value through QSP for in vitro–in vivo translation
While integration of QSP models with MPS-based research is still nascent,51 early applications for MPS design and data analysis have already demonstrated its value. The seminal work on model-guided MPS design and data analysis was carried out by Shuler et al.,30–32,52–54 who utilized PBPK models to design multi-MPS platforms based on scaling blood residence times in organs, and to analyze mechanistic data on drug toxicity studies with multi-MPS platforms. More recently, mechanistic models of drug kinetics in single liver and liver-gut MPS platforms have been shown to be more useful than traditional PK models for estimating intrinsic ADME parameters, probe mechanisms of LPS-induced inflammation, and evaluate inter-MPS communication on PK processes.26,33 Multiple studies have demonstrated the critical nature of quantitative modeling for pharmacological applications of multi-MPS platforms, which are even more complex than single MPSs.29,54 To address the difficult question of appropriate scaling in MPS design51,55–57 and move scaling from allometry to function-based, Maass et al.34 devised a new, functional approach. Their mechanistic model-based, multi-functional scaling method identifies physical design and operational parameters for single and multi-MPS platforms based on initial specification of required biological function. A proof-of-concept study focused on drug exposure demonstrated significant differences in MPS design based on this functional scaling approach compared to allometric scaling approaches.
With the benefits of model-based MPS design and data analysis demonstrated, it is time to extend MPS-QSP integration to the translation of MPS-derived data (Fig. 1). QSP models are helpful whenever multiple functions operate simultaneously and/or are nonlinearly related, which is the case in most of human (patho)physiology and is necessarily repeated in MPSs that strive to recapitulate that. The examples in section 3 illustrate the power of integrative, mechanistic models to translate in vitro experimental data to humans, in the context of known human physiology, drug and target properties, and other available knowledge. A seminal application of QSP for in vitro–in vivo translation of MPS data was the study of Tsamandouras et al.25 assessing population variability in hepatic drug metabolism using the liver MPS. In this, a population PBPK model was developed for lidocaine to extrapolate in vitro MPS data to the observed PK variability in vivo.
To understand how QSP models could be used to interpret MPS-generated data, a few thought experiments are useful. In the DILI study in section 3, for example, MPSs that embody biological functions or context important for evaluation of specific toxicity mechanisms, and better than existing in vitro assays, could provide inputs to DILIsym® or similar QSP models for hepatotoxicity predictions. In the study by Kirouac of the MAPK pathway for cancer therapy,39 a possible use would be MPSs representing tumor-organ systems to provide alternate (human) data to xenograft models on individual drug dose–responses as inputs for the model investigations of drug combinations. Conversely, such MPSs could provide human-based in vitro testbeds for validating the dose–response matrices of drug combinations that were predicted prior to or instead of further animal studies.
This points out that a key area of MPS-QSP benefit is in the evaluation and understanding of multi-drug situations, related to either efficacy39,46 or the complications of drug–drug interactions. An example of the latter is Long et al., in which the effect of tocilizumab, an IL-6 receptor antagonist, on simvastatin PK in rheumatoid arthritis patients58 could be recapitulated over two weeks in an immune-competent liver MPS under inflammatory conditions.59 Further experimentation to investigate stimulus–response relationships for both drugs and inflammatory levels would benefit from a QSP model encompassing mechanisms of inflammatory modulation of drug metabolism as well as tocilizumab and simvastatin PK to extrapolate the in vitro findings to the in vivo patient setting.
Numerous other applications in which QSP translation will be needed to maximally utilize MPS-based data can be envisioned. A promising use of MPSs is to understand tissue site of action PD and toxicodynamics as well as evaluate potential efficacy and toxicity biomarkers. Tissue accumulation and therapeutic effects could be quantitatively evaluated in MPSs, and QSP models could extrapolate to in vivo organ accumulation and predict PK/PD and toxicokinetic/toxicodynamic relationships at sites of action. Given that patient selection biomarkers result in a more than three-fold increase in likelihood of drug approval,12 disease MPSs could provide a major new tool for discovery and/or evaluation of disease biomarkers. In concert, QSP models could put such information in human context to understand relationships to disease mechanism and drug mechanism-of-action, possibly helping speed new biomarkers into the clinic. Further, MPS-QSP studies can be used to evaluate the kinetics of biomarkers, which could then inform the timing of biomarker sampling in clinical trials.
MPS technologies have the potential to provide an experimental framework to accurately assess drug responses for patient sub-populations and even individuals, and thus, integrative MPS-QSP approaches should better enable precision and personalized medicine applications to find proper treatment options for individuals. For example, adverse drug effects for populations with renal or hepatic impairment can be experimentally evaluated in appropriate MPSs and then, dose adjustments for these patients can be calculated with QSP models. Moreover, therapeutic windows of combination treatments could be assessed using MPS data-driven QSP models. MPSs from various organ systems can provide testbeds for preclinical evaluation of therapeutic and adverse effects of combination therapies, while QSP models adjust the dosing regimen for the clinic.
A final hurdle for realizing the potential of MPS technologies, QSP models, and their integration for drug development is the acceptance of information derived from them by regulatory bodies.60 It is promising that results from computational models, including mechanistic QSP models, are increasingly part of successful regulatory filings.46,47,61,62 Modeling contributions in recent years in INDs, NDAs and BLAs include estimating first in human dose,63 predicting drug–drug interactions,64,65 extensions of drug PK/PD to special patient populations (e.g., hepatic and renal impairment, and pediatrics38,63), and understanding disease progression as well as dose–exposure–response relationships,46,66 among other things. Favorably, a major goal of the US Food and Drug Administration in 2018–2022 is to update its regulatory science and review expertise to facilitate the development and application of “model-informed drug development”.67 While use of MPS technologies to directly influence regulatory decisions is not yet demonstrated, it is our expectation that mechanistic systems methods, involving both MPSs and QSP models, will continue to gain acceptance for regulatory decisions.
5. Conclusion
In conclusion, the perspective that MPS technologies hold great promise for impacting preclinical-to-clinical translation in the pharmaceutical arena is supported from multiple directions: an understanding of the unaddressed needs in the drug R&D pipeline; the demonstration that MPSs better recapitulate human physiology than more traditional in vitro models; recognition of the value of mechanistic QSP models within biomedical and pharmaceutical R&D for integrating and interpreting data from MPSs alongside other diverse sources; and the support from regulatory agencies to include and accept computational modeling in support of regulatory applications. The MPS field will arguably have its greatest impact going forward by utilizing quantitative model-based approaches, i.e., quantitative systems pharmacology, for MPS design, data analysis and in vitro–in vivo translation.
Author contributions
CLS and MC conceptualized the research and wrote and edited the manuscript.
Conflicts of interest
None to declare.
Acknowledgements
Financial support for this project was provided by the NIH Tissue Chip Testing Center Program Microphysiological Systems Program (NIH-U24TR001951).
References
- S. Ahadian, R. Civitarese, D. Bannerman, M. H. Mohammadi, R. Lu, E. Wang, L. Davenport-Huyer, B. Lai, B. Zhang, Y. Zhao, S. Mandla, A. Korolj and M. Radisic, Adv. Healthcare Mater., 2018, 7(2) DOI:10.1002/adhm.201700506
.
- E. W. Esch, A. Bahinski and D. Huh, Nat. Rev. Drug Discovery, 2015, 14, 248–260 CrossRef PubMed
.
- U. Marx, T. B. Andersson, A. Bahinski, M. Beilmann, S. Beken, F. R. Cassee, M. Cirit, M. Daneshian, S. Fitzpatrick, O. Frey, C. Gaertner, C. Giese, L. Griffith, T. Hartung, M. B. Heringa, J. Hoeng, W. H. de Jong, H. Kojima, J. Kuehnl, M. Leist, A. Luch, I. Maschmeyer, D. Sakharov, A. J. Sips, T. Steger-Hartmann, D. A. Tagle, A. Tonevitsky, T. Tralau, S. Tsyb, A. van de Stolpe, R. Vandebriel, P. Vulto, J. Wang, J. Wiest, M. Rodenburg and A. Roth, ALTEX, 2016, 33, 272–321 Search PubMed
.
- L. A. Low and D. A. Tagle, Clin. Transl. Sci., 2017, 10, 237–239 CrossRef PubMed
.
- L. A. Low and D. A. Tagle, Lab Chip, 2017, 17, 3026–3036 RSC
.
- J. Arrowsmith and P. Miller, Nat. Rev. Drug Discovery, 2013, 12, 569 CrossRef PubMed
.
- M. Hay, D. W. Thomas, J. L. Craighead, C. Economides and J. Rosenthal, Nat. Biotechnol., 2014, 32, 40–51 CrossRef PubMed
.
- I. Kola and J. Landis, Nat. Rev. Drug Discovery, 2004, 3, 711–715 CrossRef PubMed
.
- J. Arrowsmith, Nat. Rev. Drug Discovery, 2011, 10, 87 CrossRef PubMed
.
- J. Arrowsmith, Nat. Rev. Drug Discovery, 2011, 10, 328–329 CrossRef PubMed
.
- D. B. Kassel, Curr. Opin. Chem. Biol., 2004, 8, 339–345 CrossRef PubMed
.
- Clinical Development Success Rates 2006–2015, https://www.bio.org/sites/default/files/Clinical%20Development%20Success%20Rates%202006-2015%20-%20BIO,%20Biomedtracker,%20Amplion%202016.pdf, (accessed January 6, 2018).
- C. Oleaga, C. Bernabini, A. S. Smith, B. Srinivasan, M. Jackson, W. McLamb, V. Platt, R. Bridges, Y. Cai, N. Santhanam, B. Berry, S. Najjar, N. Akanda, X. Guo, C. Martin, G. Ekman, M. B. Esch, J. Langer, G. Ouedraogo, J. Cotovio, L. Breton, M. L. Shuler and J. J. Hickman, Sci. Rep., 2016, 6, 20030 CrossRef PubMed
.
- A. M. Clark, S. E. Wheeler, C. L. Young, L. Stockdale, J. Shepard Neiman, W. Zhao, D. B. Stolz, R. Venkataramanan, D. Lauffenburger, L. Griffith and A. Wells, Lab Chip, 2016, 17, 156–168 RSC
.
- S. E. Wheeler, A. M. Clark, D. P. Taylor, C. L. Young, V. C. Pillai, D. B. Stolz, R. Venkataramanan, D. Lauffenburger, L. Griffith and A. Wells, Br. J. Cancer, 2014, 111, 2342–2350 CrossRef PubMed
.
- A. Jain, R. Barrile, A. D. van der Meer, A. Mammoto, T. Mammoto, K. De Ceunynck, O. Aisiku, M. A. Otieno, C. S. Louden, G. A. Hamilton, R. Flaumenhaft and D. E. Ingber, Clin. Pharmacol. Ther., 2018, 103(2), 332–340 CrossRef PubMed
.
- K. H. Benam, R. Novak, J. Nawroth, M. Hirano-Kobayashi, T. C. Ferrante, Y. Choe, R. Prantil-Baun, J. C. Weaver, A. Bahinski, K. K. Parker and D. E. Ingber, Cell Syst., 2016, 3, 456–466 CrossRef PubMed
e454.
- G. Wang, M. L. McCain, L. Yang, A. He, F. S. Pasqualini, A. Agarwal, H. Yuan, D. Jiang, D. Zhang, L. Zangi, J. Geva, A. E. Roberts, Q. Ma, J. Ding, J. Chen, D. Z. Wang, K. Li, J. Wang, R. J. Wanders, W. Kulik, F. M. Vaz, M. A. Laflamme, C. E. Murry, K. R. Chien, R. I. Kelley, G. M. Church, K. K. Parker and W. T. Pu, Nat. Med., 2014, 20, 616–623 CrossRef PubMed
.
- S. Bauer, C. Wennberg Huldt, K. P. Kanebratt, I. Durieux, D. Gunne, S. Andersson, L. Ewart, W. G. Haynes, I. Maschmeyer, A. Winter, C. Ammala, U. Marx and T. B. Andersson, Sci. Rep., 2017, 7, 14620 CrossRef PubMed
.
- T. Kostrzewski, T. Cornforth, S. A. Snow, L. Ouro-Gnao, C. Rowe, E. M. Large and D. J. Hughes, World J. Gastroenterol., 2017, 23, 204–215 CrossRef PubMed
.
- W. L. K. Chen, C. Edington, E. Suter, J. Yu, J. J. Velazquez, J. G. Velazquez, M. Shockley, E. M. Large, R. Venkataramanan, D. J. Hughes, C. L. Stokes, D. L. Trumper, R. L. Carrier, M. Cirit, L. G. Griffith and D. A. Lauffenburger, Biotechnol. Bioeng., 2017, 114, 2648–2659 CrossRef PubMed
.
- P. J. Shaw, P. E. Ganey and R. A. Roth, Toxicol. Sci., 2010, 118, 7–18 CrossRef PubMed
.
- R. A. Roth, A. R. Maiuri and P. E. Ganey, J. Pharmacol. Exp. Ther., 2017, 360, 461–470 CrossRef PubMed
.
- U. Sarkar, K. C. Ravindra, E. Large, C. L. Young, D. Rivera-Burgos, J. Yu, M. Cirit, D. J. Hughes, J. S. Wishnok, D. A. Lauffenburger, L. G. Griffith and S. R. Tannenbaum, Drug Metab. Dispos., 2017, 45, 855–866 CrossRef PubMed
.
- N. Tsamandouras, T. Kostrzewski, C. L. Stokes, L. G. Griffith, D. J. Hughes and M. Cirit, J. Pharmacol. Exp. Ther., 2017, 360, 95–105 CrossRef PubMed
.
- N. Tsamandouras, W. L. K. Chen, C. D. Edington, C. L. Stokes, L. G. Griffith and M. Cirit, AAPS J., 2017, 19, 1499–1512 CrossRef PubMed
.
- T. Qian, S. E. Maguire, S. G. Canfield, X. Bao, W. R. Olson, E. V. Shusta and S. P. Palecek, Sci. Adv., 2017, 3, e1701679 CrossRef PubMed
.
- E. J. Weber, A. Chapron, B. D. Chapron, J. L. Voellinger, K. A. Lidberg, C. K. Yeung, Z. Wang, Y. Yamaura, D. W. Hailey, T. Neumann, D. D. Shen, K. E. Thummel, K. A. Muczynski, J. Himmelfarb and E. J. Kelly, Kidney Int., 2016, 90, 627–637 CrossRef PubMed
.
- C. D. Edington, W. L. K. Chen, E. Geishecker, T. Kassis, L. R. Soenksen, B. M. Bhushan, D. Freake, J. Kirschner, C. Maass, N. Tsamandouras, J. Valdez, C. D. Cook, T. Parent, S. Snyder, J. Yu, E. Suter, M. Shockley, J. Velazquez, J. J. Velazquez, L. Stockdale, J. P. Papps, I. Lee, N. Vann, M. Gamboa, M. E. LaBarge, Z. Zhong, X. Wang, L. A. Boyer, D. A. Lauffenburger, R. L. Carrier, C. Communal, S. R. Tannenbaum, C. L. Stokes, D. J. Hughes, G. Rohatgi, D. L. Trumper, M. Cirit and L. G. Griffith, Sci. Rep., 2018, 8, 4530 CrossRef PubMed
.
- J. H. Sung, C. Kam and M. L. Shuler, Lab Chip, 2010, 10, 446–455 RSC
.
- A. Ghanem and M. L. Shuler, Biotechnol. Prog., 2000, 16, 334–345 CrossRef PubMed
.
- H. E. Abaci and M. L. Shuler, Integr. Biol., 2015, 7, 383–391 RSC
.
- J. Yu, N. A. Cilfone, E. M. Large, U. Sarkar, J. S. Wishnok, S. R. Tannenbaum, D. J. Hughes, D. A. Lauffenburger, L. G. Griffith, C. L. Stokes and M. Cirit, CPT: Pharmacometrics Syst. Pharmacol., 2015, 4, 585–594 CrossRef PubMed
.
- C. Maass, C. L. Stokes, L. G. Griffith and M. Cirit, Integr. Biol., 2017, 9, 290–302 RSC
.
- P. H. van der Graaf and N. Benson, Pharm. Res., 2011, 28, 1460–1464 CrossRef PubMed
.
- K. Gadkar, D. C. Kirouac, D. E. Mager, P. H. van der Graaf and S. Ramanujan, CPT: Pharmacometrics Syst. Pharmacol., 2016, 5, 235–249 CrossRef PubMed
.
- H. M. Jones, Y. Chen, C. Gibson, T. Heimbach, N. Parrott, S. A. Peters, J. Snoeys, V. V. Upreti, M. Zheng and S. D. Hall, Clin. Pharmacol. Ther., 2015, 97, 247–262 CrossRef PubMed
.
- E. Luzon, K. Blake, S. Cole, A. Nordmark, C. Versantvoort and E. G. Berglund, Clin. Pharmacol. Ther., 2017, 102(1) DOI:10.1002/cpt.539
.
- D. C. Kirouac, G. Schaefer, J. Chan, M. Merchant, C. Orr, S. A. Huang, J. Moffat, L. Liu, K. Gadkar and S. Ramanujan, NPJ Syst. Biol. Appl., 2017, 3, 14 CrossRef PubMed
.
- J. L. Woodhead, P. B. Watkins, B. A. Howell, S. Q. Siler and L. K. M. Shoda, Drug Metab. Pharmacokinet., 2017, 32, 40–45 CrossRef PubMed
.
- B. A. Howell, Y. Yang, R. Kumar, J. L. Woodhead, A. H. Harrill, H. J. Clewell, 3rd, M. E. Andersen, S. Q. Siler and P.
B. Watkins, J. Pharmacokinet. Pharmacodyn., 2012, 39, 527–541 CrossRef PubMed
.
- J. L. Woodhead, K. Yang, S. Q. Siler, P. B. Watkins, K. L. Brouwer, H. A. Barton and B. A. Howell, Front. Pharmacol., 2014, 5, 240 Search PubMed
.
- D. M. Longo, Y. Yang, P. B. Watkins, B. A. Howell and S. Q. Siler, CPT: Pharmacometrics Syst. Pharmacol., 2016, 5, 31–39 CrossRef PubMed
.
- M. A. Kamal, E. P. Acosta, D. W. Kimberlin, L. Gibiansky, P. Jester, V. Niranjan, B. Rath, B. Clinch, P. J. Sanchez, K. Ampofo, R. Whitley and C. R. Rayner, Clin. Pharmacol. Ther., 2014, 96, 380–389 CrossRef PubMed
.
- N. Parrott, B. Davies, G. Hoffmann, A. Koerner, T. Lave, E. Prinssen, E. Theogaraj and T. Singer, Clin. Pharmacokinet., 2011, 50, 613–623 CrossRef PubMed
.
- M. C. Peterson and M. M. Riggs, CPT: Pharmacometrics Syst. Pharmacol., 2015, 4, e00020 Search PubMed
.
- S. M. Huang, D. R. Abernethy, Y. Wang, P. Zhao and I. Zineh, J. Pharm. Sci., 2013, 102, 2912–2923 CrossRef PubMed
.
- V. R. Knight-Schrijver, V. Chelliah, L. Cucurull-Sanchez and N. Le Novere, Comput. Struct. Biotechnol. J., 2016, 14, 363–370 CrossRef PubMed
.
- K. Gadkar, D. Kirouac, N. Parrott and S. Ramanujan, Drug Discovery Today: Technol., 2016, 21-22, 57–65 CrossRef PubMed
.
- S. A. Visser, D. P. de Alwis, T. Kerbusch, J. A. Stone and S. R. Allerheiligen, CPT: Pharmacometrics Syst. Pharmacol., 2014, 3, e142 CrossRef PubMed
.
- C. L. Stokes, M. Cirit and D. A. Lauffenburger, CPT: Pharmacometrics Syst. Pharmacol., 2015, 4, 559–562 CrossRef PubMed
.
- K. Viravaidya, A. Sin and M. L. Shuler, Biotechnol. Prog., 2004, 20, 316–323 CrossRef PubMed
.
- J. H. Sung, B. Srinivasan, M. B. Esch, W. T. McLamb, C. Bernabini, M. L. Shuler and J. J. Hickman, Exp. Biol. Med., 2014, 239, 1225–1239 CrossRef PubMed
.
- D. A. Tatosian and M. L. Shuler, Biotechnol. Bioeng., 2009, 103, 187–198 CrossRef PubMed
.
- J. H. Sung, M. B. Esch, J. M. Prot, C. J. Long, A. Smith, J. J. Hickman and M. L. Shuler, Lab Chip, 2013, 13, 1201–1212 RSC
.
- J. P. Wikswo, E. L. Curtis, Z. E. Eagleton, B. C. Evans, A. Kole, L. H. Hofmeister and W. J. Matloff, Lab Chip, 2013, 13, 3496–3511 RSC
.
- N. Ucciferri, T. Sbrana and A. Ahluwalia, Front. Bioeng. Biotechnol., 2014, 2, 74 Search PubMed
.
- T. J. Long, P. A. Cosgrove, R. T. Dunn, 2nd, D. B. Stolz, H. Hamadeh, C. Afshari, H. McBride and L. G. Griffith, Drug Metab. Dispos., 2016, 44, 1940–1948 CrossRef PubMed
.
- C. Schmitt, B. Kuhn, X. Zhang, A. J. Kivitz and S. Grange, Clin. Pharmacol. Ther., 2011, 89, 735–740 CrossRef PubMed
.
- C. A. Livingston, K. M. Fabre and D. A. Tagle, Comput. Struct. Biotechnol. J., 2016, 14, 207–210 CrossRef PubMed
.
- C. Wagner, Y. Pan, V. Hsu, V. Sinha and P. Zhao, Clin. Pharmacokinet., 2016, 55, 475–483 CrossRef PubMed
.
- Guidance for Industry: Population Pharmacokinetics, U.S. Department of Health and Human Services, Food and Drug Administration, Washington, DC. (1999). https://www.fda.gov/downloads/drugs/guidances/UCM072137.pdf, (accessed January 6, 2018).
- C. Wagner, P. Zhao, Y. Pan, V. Hsu, J. Grillo, S. M. Huang and V. Sinha, CPT: Pharmacometrics Syst. Pharmacol., 2015, 4, 226–230 CrossRef PubMed
.
- C. Wagner, Y. Pan, V. Hsu, J. A. Grillo, L. Zhang, K. S. Reynolds, V. Sinha and P. Zhao, Clin. Pharmacokinet., 2015, 54, 117–127 CrossRef PubMed
.
- Guidance for Industry: In Vitro Metabolism and Transporter Mediated Drug-Drug Interaction Studies. U.S. Department of Health and Human Services. https://www.fda.gov/downloads/Drugs/GuidanceComplianceRegulatoryInformation/Guidances/UCM581965.pdf, (accessed January 6, 2018).
- T. A. Leil and R. Bertz, Front. Pharmacol., 2014, 5, 247 Search PubMed
.
- PDUFA Reauthorization performance goals and procedures fiscal years 2018 through 2022. https://www.fda.gov/downloads/forindustry/userfees/prescriptiondruguserfee/ucm511438.pdf, (accessed January 6, 2018).
|
This journal is © The Royal Society of Chemistry 2018 |
Click here to see how this site uses Cookies. View our privacy policy here.