DOI:
10.1039/C4GC01612B
(Paper)
Green Chem., 2015,
17, 1145-1158
Acid-catalyzed algal biomass pretreatment for integrated lipid and carbohydrate-based biofuels production†
Received
20th August 2014
, Accepted 12th November 2014
First published on 12th November 2014
Abstract
One of the major challenges associated with algal biofuels production in a biorefinery-type setting is improving biomass utilization in its entirety, increasing the process energetic yields and providing economically viable and scalable co-product concepts. We demonstrate the effectiveness of a novel, integrated technology based on moderate temperatures and low pH to convert the carbohydrates in wet algal biomass to soluble sugars for fermentation, while making lipids more accessible for downstream extraction and leaving a protein-enriched fraction behind. We studied the effect of harvest timing on the conversion yields, using two algal strains; Chlorella and Scenedesmus, generating biomass with distinctive compositional ratios of protein, carbohydrate, and lipids. We found that the late harvest Scenedesmus biomass had the maximum theoretical biofuel potential at 143 gasoline gallon equivalent (GGE) combined fuel yield per dry ton biomass, followed by late harvest Chlorella at 128 GGE per ton. Our experimental data show a clear difference between the two strains, as Scenedesmus was more successfully converted in this process with a demonstrated 97 GGE per ton. Our measurements indicated a release of >90% of the available glucose in the hydrolysate liquors and an extraction and recovery of up to 97% of the fatty acids from wet biomass. Techno-economic analysis for the combined product yields indicates that this process exhibits the potential to improve per-gallon fuel costs by up to 33% compared to a lipids-only process for one strain, Scenedesmus, grown to the mid-point harvest condition.
1. Introduction
Algal biofuel processes are typically focused around lipid yields where the timing of cultivation harvest can greatly affect the overall reported fuel production,1–3 and downstream processing characteristics. This is particularly true in previously published conceptual algal biofuel scenarios,3–6 where only the lipid fraction serves as a feedstock for biofuel production. In those models, the remaining biomass (made up primarily of proteins and carbohydrates) is relegated to anaerobic digestion and the resultant biogas is used to drive turbines for facility heat and power generation. Focusing on the energetic yield from algal biomass as a feedstock, through improving lipid extraction efficiency or adding pathways to additional biofuels (e.g. sugars to ethanol or other fuels) or other scalable co-products, can improve the economics and sustainability of a production process as both metrics are tied strongly to net energy yields.2,5 The challenges associated with a lipid-only approach and the potential for a selective fractionation approach to algal biofuels and bioproducts has been discussed in the context of future implementation of green engineering approaches to biofuels development.7–9 Technologies that integrate conversion of other biomass components into biofuels in an expanded biorefinery have only rarely been explored and present an opportunity to advance the field of algal biofuels processing, while reducing costs, greenhouse gas emissions and waste streams.7,10,11 Previous reports highlight advantages of algae relative to terrestrial feedstocks in terms of fuel performance and yields because of improved land use, but at the same time may create large environmental burdens depending on process details.10 Since the reports mostly deal with processes that focus on a lipid-only pathway, improvements in process energetic yields by taking advantage of additional fuel options, such as those derived from carbohydrates have the potential to significantly improve the overall algae process’ environmental footprint.10,11
Thermochemical-based routes exist for conversion of wet algal biomass, beyond strictly the lipid fraction, as is the case in the production of bio-oil (e.g. derived from hydrothermal liquefaction, HTL). However, some of the uncontrolled chemical secondary reactions of the different components (in particular the proteins) of the biomass and high heteroatom content of the oils are potential drawbacks of such technology12–14 and may translate to potentially higher costs to refine the bio-oil material into finished fuels or blendstocks. In contrast, biochemical-based conversion routes, such as the process discussed here, can more selectively convert biochemical components to specific products. By taking advantage of the recovery of both glucose and fatty acids after pretreatment of algal biomass as a form of biochemical conversion, the majority of the carbon assimilated by the algae may be used towards biofuel components.
Autotrophic algae can be rich in lipids but have the added potential to accumulate large amounts of storage and structural carbohydrates,3,15–18 though this is often treated as a disadvantage, with strain improvement schemes to direct carbon flux away from carbohydrates toward lipids.19 It is well understood that algal biomass yields and biochemical composition, in particular triacylglycerol accumulation, fatty acid composition and the relative carbohydrate and protein concentration vary, depending upon the nutrient status of the algal culture medium as well as due to other production or environmental factors.3,20–23 There is significant potential for overall cultivation productivity improvement and associated cost savings by shifting the focus of biomass production away from solely high-lipid production conditions, providing there is a downstream processing pathway that is tailored to the utilization of the entire feedstock, and thereby maximizing interconnectivity with biomass energy and materials.
Recently, the utilization of algal biomass as a feedstock for bioethanol production from the carbohydrate sources in algae has been explored; in particular for species like Chlorococcum sp. and Chlorella sp. biomass was hydrolyzed with acid to release monomeric sugars for fermentation.18,24–26 In summary, it was found that acid hydrolysis was more effective in releasing algal carbohydrates than several of the other physical treatments employed in these studies. Typically, these studies were carried out in small batches with no mixing, under conditions of 1–10% acid (w/v), temperatures of between 120–200 °C and various biomass loadings have been reported. The fate of lipid extraction in concert with sugar release using a controlled acid pretreatment reaction, integrated with fermentation of the carbohydrate fraction has not been reported in the literature, nor has the effect of different biochemical composition of the same algae strain on the effectiveness of conversion, extraction and fermentation been studied. There is a gap in the development of an integrated process and the synergistic optimization of pretreatment of algal biomass grown outdoors under production-relevant conditions to provide a range of protein, carbohydrate and lipid profiles. The objective of the work presented here was to develop a conversion process that lends itself to a scaled biofuels pathway for wet algal biomass and more specifically, integrating the downstream conversion process with a time-based cultivation and harvesting scenario, including physiological and biochemical changes as variables, for two production-relevant organisms, with a simultaneous comparative energetic yield and techno-economic cost analysis of the process relative to a previously-published baseline for a harmonized modeling assessment of a lipid (only)-extraction process.5
2. Materials and methods
2.1. Algal biomass
Biomass from two strains, Scenedesmus (LRB-AP 0401) and Chlorella (LRB-AZ 1201) was provided by Arizona State University and represents harvests taken in early-, mid-, and late-cultivation stages or high-protein (greater than 30% DW protein), high-carbohydrate (greater than 30% DW total biomass carbohydrates), and high-lipid (greater than 30% DW total lipid) content biomass, respectively. Details on the cultivation conditions used to achieve the three different biochemical compositional states are provided in ref. 23. In brief, by timing the harvest, biomass of different composition was obtained in a controlled fashion in outdoor flat panel (650 L) photobioreactors in nitrate deplete cultivation media. Cultivation time after reaching nutrient deplete conditions depended on final target biomass composition desired, which, depending on season, typically was 3 to 5 days for high carbohydrate (midpoint harvest) biomass and 6 to 9 days for high lipid (late harvest) biomass. High protein (early harvest) biomass was obtained by harvesting prior to nutrient depletion.
2.2. Biomass compositional analysis
Details of the biomass compositional measurements can be found in ref. 15 and 27–31. Protein analysis was carried out by combustion nitrogen using elemental nitrogen-to-protein conversion factors of 4.85 ± 0.12 and 4.77 ± 0.21 for Chlorella and Scenedesmus respectively, based on the measured amino acid composition for 10 and 7 representative samples from Chlorella and Scenedesmus respectively (ESI Table 1†).32 Lipid content in algal biomass was measured as total fatty acid methyl ester (FAME) content after a whole biomass in situ transesterification procedure, optimized for microalgae, and demonstrated to be agnostic for a range of different lipid types.27 In brief, lyophilized biomass was transesterified in situ with 0.3 mL of HCl–methanol (5%, v/v) for 1 h at 85 °C. FAMEs were analyzed by gas chromatography–flame ionization detection (GC-FID) on an Agilent 6890N; DB-WAX-MS column (Agilent, Santa Clara, CA) with dimensions 30 m × 0.25 mm i.d. and 0.25 μm film thickness.31 Carbohydrates in algal biomass were determined according to a reduced scale hydrolysis procedure, based on the NREL Laboratory Analytical Procedure.29 In brief, 25 ± 5 mg of lyophilized algal biomass was subjected to a two-stage sulfuric acid hydrolysis (1 h at 30 °C in 72 wt% sulfuric acid, followed by 1 h at 121 °C in 4 wt% sulfuric acid in an autoclave), after which soluble carbohydrates (glucose, xylose, galactose, arabinose, and mannose) were determined by high-performance liquid chromatography with refractive index detection (HPLC-RID).29 Starch was determined as described by Megazyme (Ireland) previously with no modifications.33
2.3. Calculation of theoretical conversion yields
Based on the biomass composition, theoretical yields were calculated assuming conversion of all fermentable sugars with a 51% theoretical ethanol fermentation yield (i.e. metabolic yield) from glucose34 and conversion of total fatty acid content of the biomass to hydrocarbon-based renewable diesel at a 78 wt% renewable diesel yield from total fatty acids (based on previously documented assumptions for lipid hydrotreating with high selectivity to diesel).5
2.4. Microscopy
A Nikon Eclipse E400 bright field microscope (New York, United States) was used to examine biomass samples before and after pretreatment under 1000× magnification with immersion oil using 4 μL of each sample.
2.5. Combined pretreatment and extraction
2.5.1. Small-scale controlled microwave pretreatment experiments.
The biomass generated was stored as a frozen paste at about 40% total solids and thawed at 4 °C until ready for pretreatment. For the microwave pretreatment experiments (4 mL total reaction volume), 2 mL of the 15% solids algal biomass slurry was pipetted into a glass microwave reaction vial along with 2 mL of the appropriate concentration of H2SO4 (see text). The reactions were carried out on a CEM Discover SP microwave (North Carolina, United States), using the following program; ramp to 145 °C with continuous stirring at resulting vapor pressure. For each biomass sample, triplicate pretreated samples were processed and immediately extracted with hexane at a 1
:
1 (v/v) ratio for 2 hours with occasional manual shaking, after which the samples were centrifuged for 10 minutes at 8437 rcf.
To study the process sequence effect, a set of triplicate samples were included where lipids were extracted prior to pretreatment, to allow for the comparison of process efficiencies. At the same scale of pretreatment as described above, lipid extraction was performed on aqueous slurry of 4 mL algae (7.5% solids w/v) at the same 1
:
1 (v/v) ratio of hexane to slurry. The hexane layer was removed and the aqueous phase was homogenized and 3.2 mL of the remaining solution was transferred to a microwave reaction vial and 0.8 mL of the dilute acid solution (10% H2SO4 w/w) was added to make a 2% (w/w) acid solution for hydrolysis. The sample was then centrifuged and the solids and liquor fractions were separated as described above.
Fermentable monosaccharides in the hydrolysate liquor were analyzed by HPLC as described above, and FAME content of the hexane-extractable lipid fraction and residual biomass were measured as described above. The recovery, extractability and yield calculations were calculated based on the baseline-measured FAME content of the starting material (also considered the FAME mass balance).
2.5.2. Intermediate-scale pretreatment experiments.
Pretreatment of biomass from both strains harvested at three different growth states was performed in a batch-type reactor, a 4 L (2 L working volume) ZipperClave reactor (Parker Autoclave Engineers, Erie, Pennsylvania, USA). The reactor system was selected to approximate reaction and reactor conditions for transition to a pilot-scale continuous reactor. While the ZipperClave reactor is not directly scalable to a commercial or pilot scale, it can provide both yield data and conversion performance using sufficient biomass to carry out fermentation of the solubilized carbohydrates. Pretreating biomass at high solids concentrations (∼25% w/w), incorporating biomass mixing, coupled with direct steam injection for rapid heating, are all important process parameters for an economical commercial reactor.35 The pretreatment conditions for the algal biomass using the ZipperClave reactor were 2% acid loading (w/w), temperature of 155 °C, reaction time of 10 minutes and solids loading of 25% (w/w).
2.5.3. Fermentation of hydrolysate liquor.
The slurry remaining after pretreatment was centrifuged at 8437 rcf for 20 min to separate the hydrolysate liquor containing the carbohydrate fraction from the pelleted lipid and protein fraction. Hydrolysates were tested for fermentability using Zymomonas mobilis and Saccharomyces cerevisiae, chosen for their well-established ability to convert glucose to ethanol.36–39 D5A (ATCC® 200062™) was chosen for Saccharomyces cerevisiae fermentations. The hydrolysate was neutralized to pH 5.2 using NaOH and filtered. The hydrolysates were fermented in 125 mL baffled shake flasks in either duplicate or triplicate, depending on the availability of hydrolysate. The seed culture was revived from cryopreservation and grown to achieve a starting OD600 of 1.5 in the fermentation. Each flask contained 125 mL of 5 g L−1 yeast extract, 10 g L−1 yeast peptone (0.5X YP media) along with the algal hydrolysate and inoculum charge. A set of control flasks with 4% glucose and YP media was also fermented along with the hydrolysates. The flasks were incubated anaerobically at 37 °C and agitated at 150 RPM. Samples were taken for carbohydrate and organic acid analysis throughout the fermentations.
The seed culture for Zymomonas mobilis 8b was revived from cryopreservation and grown to an OD600 of approximately 1.81 prior to inoculation of the flasks. Between 5 and 10 mL of the seed culture was used to inoculate the shake flasks at a 10% v/v level, resulting in an initial fermentation OD600 of 0.94. The shake flask fermentations were conducted at 33 °C with an initial pH of 5.8 in RM medium (10 g L−1 yeast extract, 2 g L−1 potassium phosphate monobasic) with 80 g L−1 glucose and 20 g L−1 xylose. The flasks were agitated at 150 RPM for a minimum of 29 hours. For these fermentation experiments, the hydrolysates were neutralized to a pH of approximately 5.8 with 28% ammonium hydroxide (NH4OH). Fermentations were performed in 125 mL shake flasks with a working volume of 50–100 mL using Zymomonas mobilis 8b.38,39 Within each shake flask, 5 g L−1 yeast extract, 1 g L−1 potassium phosphate monobasic were added to the hydrolysates resulting in a 3% dilution of the neutralized hydrolysate. Ethanol process yield calculations were based on ethanol produced relative to the initial fermentable sugars dependent on the organism used for fermentation.
2.6. Techno-economic analysis of envisioned process
The techno-economic analysis (TEA) considers the mid-harvest Scenedesmus biomass basis to quantify economic implications for this technology pathway relative to previously established TEA benchmarks,5,6 namely a recently published modeling harmonization analysis, which focused on extracting and upgrading algal lipids via a combined mechanical and solvent extraction process, while routing all remaining material to anaerobic digestion. TEA methodologies for process modeling and cash flow calculations were conducted consistently with previously published work.5,6,40 Material and energy balance outputs from rigorous Aspen Plus process simulations determined the size and number of capital equipment items. This information was used to estimate the total capital investment and facility operating expenses, which allow for running a cash flow rate of return analysis to determine the minimum fuel selling price (MFSP) at a stipulated 10% internal rate of return (IRR) as described in previous work.5,6
To avoid any artificial yield differences between the two process scenarios compared for TEA (e.g. lipid extraction alone versus the present fractionation approach) attributed to biomass composition differences, the mid-harvest Scenedesmus lipid content was slightly reduced from 26.5% measured experimentally to 25% for modeling purposes, also used as the basis in the referenced harmonization models; additionally, the present model assumes the composition data for the mid-harvest Scenedesmus biomass; 46% fermentable carbohydrates (48% total carbohydrates) and 13% protein (Table 1).
Table 1 Composition of representative biomass used for pretreatment experiments, representing three harvest times (early, mid, late) for two strains, Scenedesmus and Chlorella with biomass productivities of 0.28 and 0.23 g L−1 day−1 respectively. All data is expressed as % dry weight of representative biomass samples. ‘Other carbohydrates’ are defined as the difference between the total measured carbohydrates by HPLC and the glucose and mannose concentration, and consist of small contributions of rhamnose, xylose, arabinose, galactose, fucose, ribose,15 lipid content was measured as fatty acid methyl esters, representing the biofuel-relevant acyl-chains present in the biomass, irrespective of the molecular structure of the originating lipid
|
Scenedesmus
|
Chlorella
|
Early |
Mid |
Late |
Early |
Mid |
Late |
Ash |
6.7 |
2.3 |
2.1 |
6.1 |
3.0 |
2.8 |
Starch |
6.9 |
12.2 |
8.1 |
3.3 |
34 |
21.9 |
Non-starch glucose |
6.8 |
22.6 |
18 |
2.5 |
2.7 |
1.7 |
Mannose |
7.2 |
11.5 |
11.8 |
0 |
0 |
0 |
Other carbohydrates |
3.4 |
1.6 |
1.3 |
5.9 |
5 |
3.5 |
Protein |
34.4 |
12.8 |
8.9 |
40.8 |
13.4 |
12.9 |
Lipids (as FAME) |
6.6 |
26.5 |
40.9 |
13 |
22.1 |
40.5 |
TEA modeling for the present technology pathway follows the same process steps as the harmonization baseline process for algal cultivation and harvesting up through dewatering to 20% biomass solids, on the same order as also applied for the experimental conversion work discussed here. At this point, the process model diverges and follows the block diagram schematic presented in Fig. 4 (i.e. the focus here is to isolate and compare the conversion operations exclusively); first, the dewatered material is combined with high-pressure steam and sulfuric acid in a dilute-acid pretreatment reactor, which hydrolyzes the carbohydrates to monomeric sugars (modeled here as glucose). The pretreatment reactor design and cost details are based on a system described previously.40 The hydrolysate is flashed to approximately 18% total solids, neutralized using ammonium hydroxide, and sent to a solid-liquid separation step using centrifugation to concentrate the solids phase up to 30%. The liquid phase is cooled and sent to fermentation with a portion (10%) diverted to organism seed growth and the majority of the material (90%) fermented to ethanol in one-million gallon anaerobic reactors, following design and cost assumptions for seed train and fermentation operations documented previously.40 The ethanol product is purified from a starting titer of 2–4 wt% (based on feed sugar concentration and subsequent conversion yields) using distillation and molecular sieve dehydration, and the distillation stillage, containing yeast biomass, residual sugars and other water soluble components, is routed to anaerobic digestion (AD).
The solids phase from centrifugation is sent to lipid extraction, which uses hexane at a solvent-to-dry biomass feed ratio of 5
:
1, consistent with bench-scale experimental methods using one single-stage extraction, extrapolated out to a commercial counter-current solvent extraction column with 6 stages. The extraction does not utilize further dewatering or evaporation, but is on a wet solids basis; and is thus largely consistent with the harmonization baseline assumptions, but eliminates mechanical cell disruption. The oil phase is sent to a solvent distillation column to recover a majority of the hexane, considering stripping reboiler duty in overall process heat balances, leaving the raw algal oil, which is sent on to hydrotreating to produce renewable diesel (RD). Finally, the residual material remaining after extraction is sent to AD. All process and cost assumptions associated with solvent extraction, solvent recovery, and the AD/CHP systems are consistent with earlier published work.5,6 However, a caveat on the modeled AD step is that the present pathway model removes a significant fraction of non-lipid biomass by way of carbohydrate hydrolysis and fermentation, thus reducing the carbon-to-nitrogen (C/N) ratio, which could reduce the efficiency of the AD process due to N inhibition,41 but a specific limit is not well quantified for algal biomass residues. For cursory modeling purposes, no adjustments are made here to AD operating parameters or fractional yields (further details for TEA process modeling assumptions are included in ESI Table 2†).
3. Results
3.1. Algal biomass composition and theoretical conversion yields
The data shown in Table 1 list the biomass composition for the samples used and represent high-protein, high-carbohydrate and high-lipid materials (reflecting an early, mid and late harvesting stage respectively) for two strains, Scenedesmus and Chlorella. For the mid and late-stage harvests, two sets of pretreatment experiments were included to assess the repeatability of the pretreatment and extraction data, both used blended and individual harvest biomass samples. The high carbohydrate biomass contains both storage and structural carbohydrates, and in particular refers to the accumulation of starch at a time in the culture's growth before lipids substantially accumulate.23 Interestingly, though the composition differs between the two strains, the total carbohydrate content is relatively similar; the difference lies in the starch fraction and structural composition of those carbohydrates. Similar lipid contents were measured between the two strains at the respective conditions, though overall protein content in Chlorella was higher than in Scenedesmus. The detailed compositional analysis data show that glucose concentration exceeds 40% of the biomass in combination with high FAME content (up to 40%) and thus forms a promising biofuels feedstock. In the flat-panel photobioreactor, batch-type cultivation configuration presented in the methods section, biomass productivity typically ranged between 0.2 and 0.4 g L−1 day−1, which varied by season and environmental conditions. An overall average productivity of 0.28 ± 0.08 g L−1 day−1 was measured for Scenedesmus and 0.23 ± 0.08 g L−1 day−1 for Chlorella for 20 and 11 outdoor cultures respectively (J. McGowen, ASU, unpublished data) and are comparable with previously published data.21 The combination of biomass productivity with the compositional analysis indicates that for the early harvest, the productivity of carbohydrates and lipids (at 40% of the biomass each) can reach up to 0.11 g L−1 day−1 for Scenedesmus and 0.09 g L−1 day−1 for Chlorella (Table 1).
Theoretical conversion of carbohydrates and lipids to fuels for two strains was calculated based on the composition data shown in Table 1. Using the format shown in Table 2, a direct comparison to other biofuels feedstocks can be made as the fuel yields are presented on a BTU energy basis and then converted to gallon gasoline equivalent (GGE) per ton biomass, which can be considered a benchmark fuel yield unit.42,43 We have calculated theoretical ethanol and hydrocarbon yields based on literature conversion factors of 51 wt% (glucose-to-ethanol metabolic limit) and 78 wt% (FAME-to-hydrocarbon).5,44 The caveat with this dataset is that 100% extraction and conversion efficiencies are assumed and no losses are built in the theoretical conversion projections. The data shown in Table 2 illustrate that the theoretical fuel yields are highly dependent on the composition of the original biomass. Namely, the lowest overall theoretical yields occurs in the early harvest biomass, and the highest in the late harvest biomass, for both strains. The maximum biofuel potential can be found in the late harvest Scenedesmus biomass, at 143 GGE per dry ton biomass, followed by late harvest Chlorella at 128 GGE per ton. For comparison, terrestrial lignocellulosic biomass feedstocks amenable to fermentation pathways such as corn stover may contain on the order of 60% fermentable carbohydrates (C5 and C6 sugars),40,45 which corresponds to a theoretical limit of 104 gallons ethanol or 68 GGE per ton biomass.40 Algal biomass thus has a higher potential summative biofuel yield compared to typical terrestrial feedstocks. Algae also compare favorably to traditional feedstock conversion pathways, e.g. 76 GGE per ton for corn starch to ethanol.46 Alternatively, heterotrophic cultivation of oleaginous yeast, e.g. Lipomyces or Yarrowia sp., can produce biomass with up to 60% lipids,47–49 which would equate to an up to 153 GGE per ton biomass using the calculations described here. On a side note, the majority of industrial microorganisms do not readily metabolize pentose sugars (5-carbon sugars i.e. xylose, arabinose), which contribute significantly to terrestrial biomass, with resulting penalties on ethanol (or other bio-based product) yields.50 Algae offer another key advantage to terrestrial biomass feedstocks in this regard, as typical algal species contain very little pentose (C5) carbohydrates.15
Table 2 Theoretical conversion yields based on the measured biomass composition for two strains at three different harvest times (early, mid and late), based on conversion calculations detailed in ref. 5 and 40
|
Scenedesmus
|
Chlorella
|
Early |
Mid |
Late |
Early |
Mid |
Late |
51 wt% glucose-to-ethanol conversion metabolic yield using Saccharomyces cerevisiae fermentation.
65.8 vol% ethanol-to-gasoline conversion (heating value equivalent).
78 wt% FAME-to-hydrocarbon conversion, % DW = percent dry weight.
|
Total carbohydrates (% DW) |
24 |
48 |
39 |
12 |
42 |
27 |
Glucose/mannose (% DW) |
21 |
46 |
38 |
6 |
37 |
24 |
Ethanol (% DW)a |
11 |
24 |
19 |
3 |
19 |
12 |
Ethanol (gal per ton) |
32 |
72 |
59 |
9 |
57 |
37 |
Gasoline equivalent (gal per ton)b |
21 |
47 |
39 |
6 |
37 |
24 |
Btu equivalent (×103) |
2478 |
5481 |
4476 |
678 |
4344 |
2787 |
|
Fatty acids (FAME) (% DW) |
7 |
27 |
41 |
13 |
22 |
41 |
Hydrocarbon (% DW)c |
5 |
21 |
32 |
10 |
17 |
32 |
Diesel equivalent (gal per ton) |
16 |
64 |
99 |
31 |
53 |
98 |
Btu equivalent (×103) |
1959 |
7865 |
12 139 |
3858 |
6559 |
12 021 |
|
Total fuel energy (×103 Btu) |
4432 |
13 344 |
16 624 |
4545 |
10 902 |
14 813 |
Total gasoline equivalent (GGE per ton) |
38 |
115 |
143 |
39 |
94 |
128 |
3.2. Optimization of acid-catalyzed conversion of algal biomass
We set up an extraction and conversion process at the small scale using microwave pretreatments to allow for high-throughput experimental design and exploration of conversion conditions relevant to larger-scale processes, which were implemented for the fermentation studies described later. We determined that the data obtained using the microwave reactor at the 4 mL scale, while perhaps not perfectly scalable due to reactor geometry, solids loading and mixing regimes, are a satisfactory surrogate for data from larger scale reactors and could provide both boundary conditions for subsequent experiments as well as evidence on the utility of this approach. The pretreated samples were extracted with hexane as a representative solvent for a commercially relevant solvent system. Three fractions are generated after centrifugation, a hexane-extracted lipid fraction, a liquor or aqueous stream containing the soluble sugars for fermentation and a solid residue fraction, enriched in proteins. For each of the triplicate experiments, the fermentable carbohydrates were measured in the aqueous fraction and lipids were measured as FAMEs in the hexane extractable lipid fraction as well as in the residual biomass. The relatively small amount of ash detected in the biomass (<7%) is assumed to solubilize in the aqueous phase during the hydrolysis process. For the initial investigation, we established a fractional factorial design of pretreatment condition parameters; acid concentration, time and temperature. The results are not shown, but are the subject of a follow on manuscript dealing with a highly detailed parametric investigation of pretreatment effectiveness and biomass integration. Based on the exploratory quantitative data, we decided to focus our process sequence optimization work around the pretreatment conditions of 2% acid (w/w), at 145 °C and 1 min reaction time.
3.2.1. Process sequence comparison.
A side-by-side comparison of two different process pathways allowed us to evaluate the effectiveness of bioconversion as a like-for-like comparison of the observed yields. A first pathway was mimicked in a scenario referred to as “extraction prior to pretreatment”, where the first extraction step reflects a baseline model lipid extraction scenario similar to our previously established techno-economic base case with elimination of the mechanical disruption step (i.e. direct extraction of wet algal biomass using hexane solvent). As an alternative, we also looked at the conversion efficiency and respective carbohydrate and lipid process yields from acid hydrolysis of whole biomass slurries followed by extraction of the lipids, in a scenario referred to as “pretreatment prior to extraction”. This latter process reflects a chemical rather than physical biomass disruption step that can make the algae more amenable to subsequent lipid extraction, though a possible drawback to this approach was the potential for degradation of lipids and loss of carbohydrates during pretreatment.
Representative samples of aqueous algal biomass before and after conversion before any separation or extraction are shown in the micrographs in Fig. 1. Significant morphological changes can be observed in the biomass and cell residue after conversion, which we interpret to be due to complete disruption of the algal cells. Distinct oil droplets are visibly associated with residues for all six biomass samples. Some structural differences appear between the two different strains, Chlorella and Scenedesmus, with larger and less integrated droplets in the solid residue for Scenedesmus, and more entrained droplets for Chlorella.
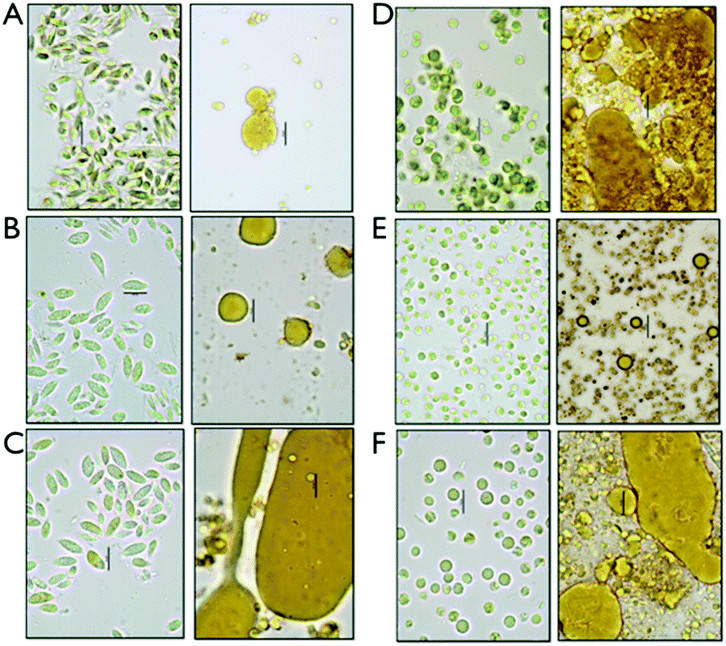 |
| Fig. 1 Illustration of morphological changes of cellular structure of the algae after pretreatment (R) relative to the original biomass (L) for each double panel. (A) Early harvest Scenedesmus (Sd), (B) mid harvest Sd, (C) late Sd; (C) early Chlorella (Cv), (E) mid harvest Cv., (F) late harvest Cv. | |
The quantitative determination of monosaccharides other than glucose by HPLC is often problematic for microalgal carbohydrates due to severe co-elution and uncertain quantification of additional non-carbohydrate components, oligosaccharides and amino acids released during the pretreatment process.15 For the purpose of carbohydrate hydrolysis measurements and because glucans made up the majority of the structural carbohydrates (Table 1), we only took glucose concentration in the liquors into account and used this as a proxy for release and hydrolysis of biomass carbohydrates (Table 3).
Table 3 Quantitative lipid and carbohydrate release before and after a conversion process, expressed as a fraction of whole biomass FAME or carbohydrates respectively, extractable lipids and FAME, and non-extractable FAME expressed as fraction of whole biomass. Each value of lipid extractability is the mean ± stdev of triplicate pretreatment or control experiments. Glucose in liquor = glucose measured in hydrolysates liquors after acid pretreatment, before and after extraction expressed on a biomass dry weight basis. Glucose release = % glucose released relative to whole biomass glucose content
|
Scenedesmus
|
Chlorella
|
Early |
Mid |
Late |
Early |
Mid |
Late |
Extraction – pretreatment |
FAME in biomass (% DW) |
6.8 |
24.4 |
35.1 |
11.6 |
20.8 |
35.0 |
FAME in extract (% DW) |
0.9 ± 0.0 |
1.9 ± 0.3 |
2.0 ± 0.1 |
1.7 ± 0.2 |
0.3 ± 0.0 |
1.3 ± 0.3 |
FAME extractability (%) |
13.7 ± 0.6 |
7.6 ± 1.4 |
5.8 ± 0.4 |
14.2 ± 1.5 |
1.5 ± 0.2 |
3.8 ± 0.7 |
Glucose in biomass (% DW) |
13.7 |
34.8 |
26.0 |
4.8 |
36.7 |
23.6 |
Glucose in liquor (% DW) |
12.9 |
29.0 |
24.4 |
3.0 |
31.3 |
20.1 |
Glucose release (%) |
94.0 |
83.2 |
93.9 |
62.5 |
85.3 |
85.2 |
|
Pretreatment – extraction |
FAME in biomass (% DW) |
6.8 |
25.6 |
35.1 |
12.45 |
21.36 |
35.1 |
FAME in extract (% DW) |
5.3 ± 0.4 |
23.6 ± 0.4 |
27.12 ± 0.55 |
5.1 ± 0.3 |
4.7 ± 0.4 |
18.0 ± 0.1 |
FAME extractability (%) |
78.4 ± 5.3 |
92.5 ± 1.5 |
77.3 ± 1.6 |
40.7 ± 2.2 |
22.2 ± 2 |
51.2 ± 0.3 |
Glucose in biomass (% DW) |
13.7 |
34.8 |
26.0 |
4.8 |
36.7 |
23.6 |
Glucose in liquor (% DW) |
10.6 |
25.4 |
18.6 |
3.3 |
29.8 |
17.9 |
Glucose release (%) |
77.4 |
73.1 |
71.7 |
68.8 |
81.2 |
75.8 |
Comparing the glucose release data from the small-scale microwave experiments, we achieved high levels of hydrolysis of the glucan present in the respective whole biomass samples. The glucose release as a fraction of the respective biomass carbohydrate composition was between 72 and 94% for Scenedesmus and 63 to 86% for Chlorella (Table 3). A higher relative recovery was measured on the samples that were first extracted and then pretreated (83–94%). Although glucose recovery is not complete, these data supported our process concept and encouraged us to perform further evaluation and optimization. Earlier published reports already demonstrated high yields of carbohydrates after an acid hydrolysis conversion of algal biomass,25,26,51,52 the results presented here are valuable and unique because of the use of two different strains with varied biomass composition used for comparison.
We also investigated the fate of lipids during the conversion process. Our initial concern regarding the potential degradation of fatty acids in a hot acid aqueous environment was addressed by measuring the recovery or mass balance of fatty acids (as FAME) in each of the three fractions (hexane-extracted lipid fraction, liquor or aqueous stream and solid residue). The FAME content in each fraction was normalized relative to the respective biomass concentration in the experiment and compared to the total FAME content in the original biomass (Table 3). A control experiment was included to provide a baseline by which to compare the recovery after acid pretreatment and to estimate reproducibility of the replicate pretreatment reactions, which was found to be around 5% relative standard deviation (RSD).
Overall comparison of the FAME mass balance (defined as the sum of the extractable fatty acids and the residual fatty acids in the biomass after extraction) for Chlorella and Scenedesmus was between 86 and 96%, and 71 and 87% respectively (data not shown). We observed a lower extractability of the Chlorella samples, indicating a level of fatty acid losses that is accelerated after pretreatment.14 This distinction in extraction between Chlorella and Scenedesmus biomass will be reported in future work. A second important parameter for the down select is the extractability of lipids after pretreatment. For the two process scenarios we measured the lipids that can be extracted using hexane. The gravimetric extraction yields as well as the fatty acids in the extracts were calculated and normalized for the amount of biomass that entered the small-scale reactions and both reflect the respective process yields. A summary of the extractable lipids and extractable FAME data is shown in Table 3. Large differences between Scenedesmus and Chlorella are apparent; although the whole biomass lipid content is comparable, the extractable fraction for Chlorella is much lower; the majority of the fatty acids (i.e. 49–78%) are associated with the residue for Chlorella, whereas after extraction of Scenedesmus pretreated slurries, only 10–23% of the fatty acids are left behind in the residue. This parameter is important and contributes highly to the decision for downselecting to one strain and harvest condition for scale-up. There are several hypotheses to explain the low level of extractable lipids in Chlorella; (i) lipids are physically entrapped in residual biomass, (ii) polarity of the lipids is too high to be soluble in hexane and thus lipids stay behind with the residual biomass, (iii) a pretreatment side reaction has caused chemical interaction of lipids to cell wall residue. All three hypotheses are currently being investigated and additional routes to increase the extraction efficiency are being studied.
3.2.2. Process scale up and fermentation of pretreatment liquors.
To specifically investigate the fermentability of the sugars in the aqueous liquors, we scaled up the pretreatment to ∼1 kg scale in a ZipperClave reactor and conducted bench-scale flask-fermentations. We used the optimized conditions identified in the small-scale reaction experiments. No additional saccharifying enzymes were added to the hydrolysate liquor prior to testing in fermentations conducted in small shake flasks using both Saccharomyces cerevisiae D5A (yeast) and Zymomonas mobilis 8b (bacteria). Z. mobilis was included in the fermentation experiments to test the general utility of the liquor in a fermentation process.38 The fermentation profiles for each strain are shown in Fig. 2 for S. cerevisiae and Z. mobilis, in terms of process yields. Process yields in this context are calculated as the ethanol concentration measured during fermentation relative to the theoretically calculated ethanol concentration from the measured sugar concentration (using a 51% theoretical conversion of glucose to ethanol). While the highest ethanol yield was achieved in the early harvested Chlorella using S. cerevisiae fermentations (not taking the >100% yields observed with the late harvested Chlorella into account), the actual ethanol concentrations were lowest for the early-harvested biomass for both strains and fermentative organisms, as the carbohydrate content was the lowest for these conditions (Table 4). The ethanol yields for S. cerevisiae fermentation achieved over 80% yield, for both strains, and all harvest scenarios. The late-harvested Chlorella supported >100% ethanol yield for S. cerevisiae fermentation, which is most likely due to additional, unidentified fermentable carbohydrates or oligomeric forms of fermentable carbohydrates that are present in the liquors but not measured. The results that are shown in Table 4 are calculated based on the fermentability of monosaccharides released, in particular glucose, xylose and mannose, and measured using a standard HPLC technique. Higher than 100% yields of ethanol fermentation are usually attributed to analytical challenges associated with the full characterization of complex mixtures of mono- and oligo-saccharides. For example, in this case the presence of fermentable sugars in the liquors that were not identified or quantified by HPLC but are fermentable by S. cerevisiae, but not by Zymomonas. Based on published literature, C6 monosaccharides (e.g. glucose, fructose, sucrose, mannose, …) are fermentable by Saccharomyces53 and glucose, xylose, fructose and sucrose is fermentable by Zymomonas.38,54 One explanation for the overproduction of ethanol in the late-harvest Chlorella is that partially hydrolyzed starch may be present in the liquors, a substrate that is fermentable by Saccharomyces and not by Zymomonas.53 This explanation is supported by starch being much more prominent the late harvests of Chlorella relative to Scenedesmus (Table 1). The original fermentation data were collected based on triplicate experiments, with close reproducibility between the replicate cultures. Ethanol yields resulting from the Z. mobilis fermentation achieved higher yields in the mid and late-harvested Scenedesmus 82.9% and 90.3%, respectively compared to the mid and late-harvested Chlorella biomass (77.1% and 78.7% respectively).
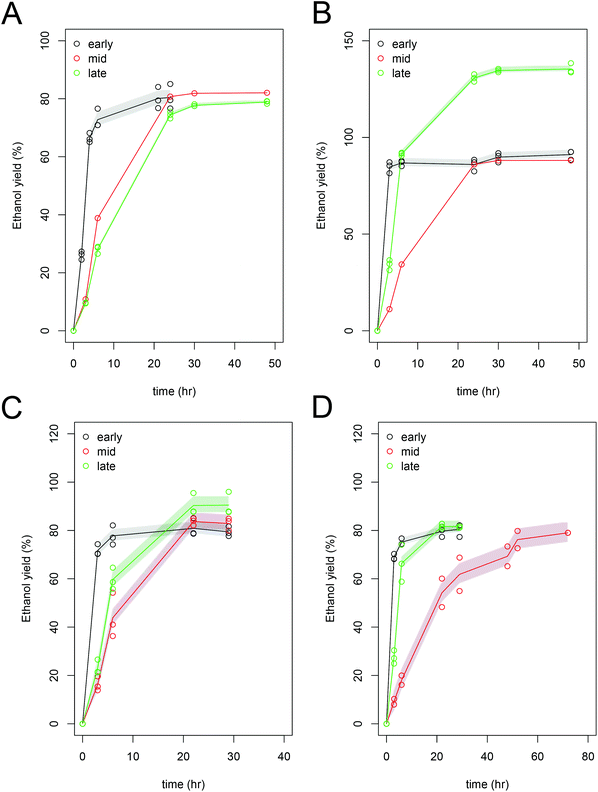 |
| Fig. 2 Fermentation of hydrolysate liquors (shown as yield ethanol (% of theoretical yields) from Scenedesmus (A,C) or Chlorella (B,D) fermentation experiments with either Saccharomyces cerevisiae D5A (A–B) or Zymomonas mobilis 8b (C–D). Shaded areas connect the standard deviation of triplicate fermentation experiments. | |
Table 4 Ethanol concentration and yield from fermentation of algal sugars using S. cerevisiae D5A and Z. mobilis 8b as the fermentation organisms
|
Scenedesmus
|
Chlorella
|
Early |
Mid |
Late |
Early |
Mid |
Late |
Measured ethanol yields of >100% may reflect fermentation of additional carbohydrates beside glucose and mannose, which was not accounted for in the theoretical calculations. The values for carbohydrate and ethanol concentrations are shown as the mean ± stdev of triplicate fermentation experiments.
|
S. cerevisiae D5A |
Carbohydrates in liquor (g L−1) |
16.30 ± 0.50 |
62.48 ± 0.40 |
62.04 ± 0.10 |
5.90 ± 0.10 |
44.60 |
21.07 ± 0.30 |
5-HMF (g L−1) |
0.67 ± 0.01 |
0.91 ± 0.26 |
1.47 ± 0.01 |
0.18 ± 0.01 |
1.68 |
0.99 ± 0.01 |
Ethanol concentration (g L−1) |
6.70 ± 0.20 |
26.14 ± 0 |
25.45 ± 0.10 |
2.73 ± 0.10 |
20.05 |
14.62 ± 0.30 |
Ethanol productivity (g L−1 day−1) |
6.68 ± 0.08 |
13.10 |
12.9 ± 0.1 |
1.4 ± 0.0 |
10 |
7.3 ± 0.2 |
Ethanol yield (%)a |
80.3 |
82.0 |
80.4 |
91.1 |
88.1 |
135.4 |
|
Z mobilis 8b |
Carbohydrates in liquor (g L−1) |
15.28 ± 1.5 |
47.42 ± 0.3 |
41.31 ± 1.8 |
7.84 ± 0.4 |
44.59 ± 0.3 |
22.4 ± 0.2 |
5-HMF (g L−1) |
0.42 ± 0.02 |
1.1 ± 0.0 |
1.32 ± 0.05 |
0.2 ± 0 |
1.61 ± 0.02 |
0.93 ± 0.01 |
Ethanol concentration (g L−1) |
6.20 ± 0.75 |
20.05 ± 0.62 |
19.03 ± 0.13 |
3.22 ± 0.28 |
17.94 ± 0.14 |
9.32 ± 0.11 |
Ethanol productivity (g L−1 day−1) |
5.17 ± 0.63 |
16.71 ± 0.51 |
15.86 ± 0.11 |
2.69 ± 0.23 |
14.95 ± 0.12 |
7.76 ± 0.09 |
Ethanol yield (%)a |
77.1 |
82.89 |
90.31 |
73.8 |
77.05 |
78.7 |
While it appears from the data presented in Fig. 2A, that the mid and late harvest Scenedesmus have similar ethanol yield, the absolute ethanol productivity in the mid-harvest Scenedesmus is higher due a higher carbohydrate concentration in the initial biomass (Table 4). For further detailed development of this process and optimization of fermentative pathways, the implementation of improved carbohydrate analytical methods will be essential to truly quantify the value of the biomass and characterize the kinetics of fermentation. Fermentation of the mid-harvested Chlorella biomass by Z. mobilis required additional time (a total of 50 hours) to reach the maximum ethanol yield compared to the early- and late-harvested biomass, which could be related to potential inhibitors resulting from pretreatment, such as hydroxymethylfurfural (HMF), which had the highest concentration in the late harvested Chlorella biomass (1.9 g L−1). Fermentation of carbohydrates to ethanol occurred in less than 24 hours (in most cases, the fermentation was completed between 6 and 21 hours) for most of the cultivation regimes, fermentation organisms, and algal strains. The final ethanol concentrations were highest in the S. cerevisiae fermentations for the mid and late harvest samples. The slower rates of fermentation performance or apparent toxicity was observed at these hydrolysate concentrations for either organism across all three cultivation regimes and the measured ethanol yields were close to the theoretically predicted yields39 (Table 4). For each strain, we expressed performance, cultivation regime and fermentation organism by normalizing yield against the pure sugar control (glucose) for both fermentation experiments; averaging 94.3% ± 1.0 (data not shown).
Furfural, derived from temperature-induced degradation of C5 sugars and a potential toxin for fermentation, was not present in any of these hydrolysates. 5-Hydroxy-methyl furfural is a degradation product of C6 sugars, and is a common inhibitor of ethanol fermentations with cellulosic sugars. We measured concentrations of 0.9 to 1.9 g L−1 in the algal hydrolysates (Table 4), which is similar to the concentrations detected in cellulosic biomass hydrolysates.55,56
3.3. Demonstrated process yields
The compiled data obtained at the gram and kilogram scales indicate strain and growth condition differences in the demonstrated yields, based on integrating the lipid extractability and sugar fermentation data from the combined experiments illustrated in Tables 3 and 4. Based on these data, reflecting actual measured extractability and glucose release from pretreatment, the mid harvest Scenedesmus biomass case yielded the highest overall combined biofuels potential per ton biomass (97 GGE per ton) as extrapolated from the observed experimental data, and thus was selected as the basis for techno-economic analysis to begin evaluating the implications for a scaled-up commercial process relative to established approaches focused only on lipid extraction.
3.4. Techno-economic analysis of new process sequence
To frame the analysis for TEA modeling, a case is evaluated based on currently observed experimental values, as well as another case based on reasonable projected improvements in conversion process conditions and yields towards future goals. Such improvements are assumed to be made in the acid pretreatment, fermentation, and lipid extraction steps, while anaerobic digestion (AD) operational and yield assumptions are maintained fixed for consistency with the harmonization baseline5 and underlying literature data.57–59 As discussed in the methods section and parameters summarized in ESI Table 2,† all TEA cases and modeled yields are based on the mid-harvest Scenedesmus basis, given its promising experimental and theoretical maximum fuel yields (Table 2). It bears clarification that the yields (GGE per ton) shown in ESI Table 2† are lower than the bench-scale experimentally observed yields (97 GGE per ton), primarily driven by a lower modeled ethanol yield due to additional processing losses incurred throughout the integrated commercial-scale process model, such as soluble sugar losses associated with the solid-liquid separation step (25%), sugar diversion to ethanol-fermenting organism inoculum propagation (10%), and assumed contamination losses in a commercial process (3%). Additionally, the TEA modeling framework applied here was based on the previously published harmonization baseline and associated base case algal cultivation productivity of 13.2 g m−2 day−1 (AFDW basis), which calculates out to a biomass production cost of $$1050 per ton.60 We further extrapolate the analysis for an “improved” conversion case out to increased cultivation productivity scenarios of 30 and 50 g m−2 day−1 (which would translate to approximately $$660 per ton and $$530 per ton, respectively, based on extrapolating from the above-referenced harmonization benchmark process5 while leaving all other feedstock cultivation and processing parameters unchanged).
Fig. 3 presents the results of the TEA for the modeled minimum fuel selling price in 2011-year $s compared to fuel yields, for each scenario considered. Both MFSP and yields are based on total fuel yield (renewable diesel plus ethanol, where applicable) translated to a GGE basis according to product heating values.42 The relative breakdown between ethanol and diesel yields is shown in ESI Table 2† for the “baseline” and “improved” conversion scenarios.
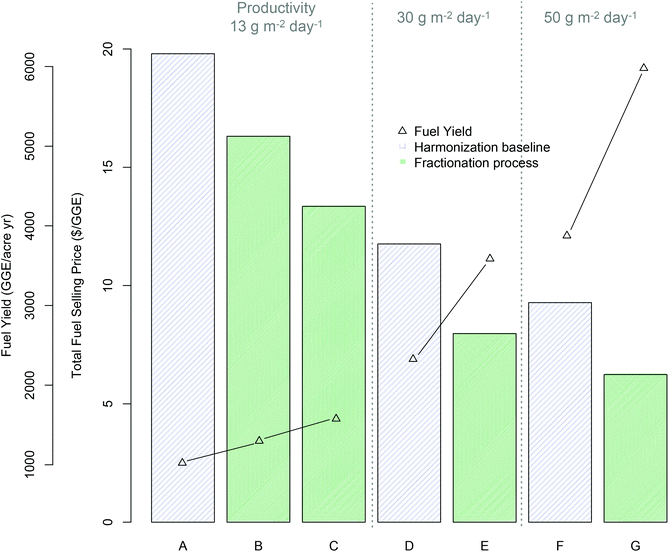 |
| Fig. 3 Economics of fractionation process technology pathway (all cases based on mid-harvest Scenedesmus biomass) relative to benchmark lipid extraction based on TEA modeling results for minimum fuel selling price (MFSP) and total fuel yield per cultivation acre (GGE per acre per year); (A) harmonization baseline5 (13.2 g m−2 day−1 cultivation productivity): (B) fractionation “baseline” process assumptions (see ESI Table 2†) (13.2 g m−2 day−1 productivity), (C) fractionation “improved” process assumptions (ESI Table 2†) (13.2 g m−2 day−1 productivity), (D–E) harmonization baseline and improved fractionation respectively with improved productivity (30 g m−2 day−1), (F–G) harmonization baseline and improved fractionation respectively with 50 g m−2 day−1 productivity. | |
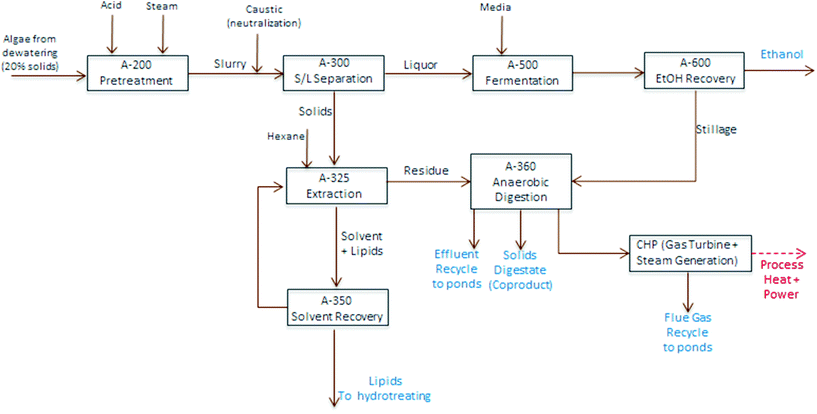 |
| Fig. 4 Block flow diagram schematic of algae fractionation process model utilized for TEA modeling purposes. | |
TEA results for the base case algal cultivation productivity of 13.2 g m−2 day−1 show promising economic potential for this technology pathway, with an 18% improvement (reduction) in MFSP based on currently observed experimental results relative to the lipid-focused benchmark $($16.31/GGE versus $$19.80/GGE respectively), or a 33% improvement for the theoretical “improved” conversion case $($13.35/GGE) (Fig. 3, scenario A–C). This improvement is driven in large part by the substantial increase in total fuel yield, at 27% increase for the “baseline experimental” case relative to the benchmark (1299 versus 1023 GGE per acre-year respectively), or 54% increase for the “improved” case (1577 GGE per acre-year). Extrapolating further to concomitantly increased algal cultivation productivity combined with the “improved” conversion case, the present technology pathway shows the potential to maintain an approximate 33% improvement in MFSP relative to the harmonization baseline technology at either 30 g m−2 day−1 or 50 g m−2 day−1 algal productivity; $$7.97/GGE versus $$11.76/GGE (Fig. 3D and E) and $$6.24/GGE versus $$9.28/GGE (Fig. 3F and G) for the respective productivity scenarios. This can be associated with a 54% increase in total fuel yields; 3587 versus 2326 GGE per acre-year and 5979 versus 3876 GGE per acre-year for the productivity scenarios shown in Fig. 3. It is important to note that no other upstream parameters are improved here, such as switching to lower-cost cultivation practices (for example removing pond liners) or reducing dewatering costs to reflect alternative dewatering techniques. These would contribute to further reductions in biomass production costs beyond the calculated value of $$530 per ton noted above based simply on the highest assumed areal productivity (50 g m−2 day−1). Thus, the resulting cost estimates for the “future” case scenarios do not represent the absolute best-case costs that may be achieved, but provide a consistent means for comparison of new technologies relative to benchmarks.
While the models evaluated here leave room for further refinement as additional data is collected and process understanding is established, our analysis suggests that the processing pathway associated with the fractionation approach described here holds potential for increasing yields and thereby reducing costs, relative to standard lipid extraction and conversion of biomass residues to lower-value co-products such as biogas (via AD). As a point of reference, the US Department of Energy (DOE) maintains a Multi-Year Program Plan (MYPP)60 document, which describes a starting baseline of roughly 1050 gal per acre per year of raw algal oil intermediate, based on 13.2 g m−2 day−1 algal productivity and 25% lipid content, focused only on extraction of lipids. This could be translated using the information provided to roughly 56 GGE of algal oil intermediate per dry ton of algal biomass cultivated or 53 GGE per dry ton for upgraded renewable diesel after processing through a hydrotreater.5 For further reference, published values for a number of terrestrial biomass-derived biofuel technologies include 76 GGE per ton for corn starch to ethanol based on published operating yields,46 52 GGE per ton for biochemical ethanol from corn stover,40 63 GGE per ton for thermochemical ethanol from woody biomass61 and 45 GGE per ton for biomass-to-diesel via biological (fermentative) conversion of sugars,50 and up to 153 GGE per ton for oleaginous yeast biomass with up to 60% lipids, based on published oil content data.47,48 These fuel yield values are compared to the demonstrated and theoretical values from the algae fractionation pathway described here, namely 97 and 115–143 GGE per ton respectively, where the former (experimentally calculated) value is higher than the modeled value of 70 GGE per ton for the experimental baseline case, due to additional processing losses incurred in the integrated commercial-scale model as a caveat discussed above.
These yield comparisons highlight the potential for a viable path towards ultimately meeting aggressive yield targets required to sustain economics. Indeed, the ultimate year 2022 yield goal of roughly 5300 gal per acre per year of raw algal oil established in the above-cited DOE MYPP document60 would require aggressive gains in algal cultivation performance to either 50 g m−2 day−1 productivity at 30% lipid content, or vice-versa to 30 g m−2 day−1 and 50% lipid content, when focused on lipids alone. However, if the late harvest Scenedesmus scenario shown above could ultimately be improved to achieve a yield of 129 GGE per ton (90% of theoretical), achieving the same target of 5300 gal per acre per year would require a productivity near 28 g m−2 day−1 without any differences in algal composition (Table 1), thus reducing the burden on algal growth performance required to achieve final yield targets. We also highlight a considerable increase in overall energetic yield of the combined process (using fuel BTU as the metric) relative to the baseline extraction process, while still leaving a residue for anaerobic digestion to drive the heating and powering of the plant and to enable recycle of nutrients back to the cultivation step. The energy yields, in our case used as the metric for conversion efficiency, are also critical drivers for sustainability and life cycle metrics of a process, which, in the data we present, indicate at least a doubling of the relative to the baseline process.6 By virtue of this increased energetic yield, we anticipate improvements in overall process sustainability, particularly in the areas of energy balances and greenhouse gas emissions profiles, but this remains to be demonstrated with a thorough life cycle analysis study beyond the scope of this paper.
4. Conclusions
We have evaluated two algal strains cultivated under conditions that accumulate high levels of protein, lipid or carbohydrates. Using data for compositional analysis, lipid extraction, pretreatment and fermentation, we identified Scenedesmus, grown under conditions to accumulate significant levels of carbohydrates and lipids (mid harvest) as a target biomass source to move forward for a demonstration of our novel fraction process with demonstrated total fuel yields amounting to 97 GGE per ton biomass accounting for a calculated 33% reduction in the baseline fuel cost. The process described here provides a new route to valorizing algal biomass components and a potentially viable route for algal biofuels development with high efficiency and clean product-streams demonstrated for wet biomass extraction. Such an approach may offer more co-product flexibility than for example a hydrothermal liquefaction model, which converts the whole biomass rather than fractionates to selective constituents, and thus negating the ability to pursue higher-value co-product components native to the starting biomass. We chose to evaluate the conversion to ethanol to demonstrate the fermentability of algal sugars and to keep this work within the framework of biofuels to allow us to easily add the contributions of two products based on a common metric (GGE per ton). We are presenting this manuscript also as a fractionation approach to algal biofuels and bioproducts, by keeping the fractions available for individual component upgrading. Because of our institutional research focus on bioenergy, we focused the application on biofuels development; however, this technology can also find applications in the bioproducts realm, and the areas of food and feed ingredient R&D or high value applications in the bioplastics, or carbon fiber.
Acknowledgements
We gratefully acknowledge Holly Smith and Joseph Shekiro. Drs. John McGowen and Thomas Dempster (AzCATI, ASU, Mesa, AZ) provided the biomass. This work was supported by the U.S. Department of Energy under contract no. DE-AC36-08-GO28308 with the National Renewable Energy as part of the BioEnergy Technology Office (BETO) task #1.3.4.300, 1.3.1.200 and 1.3.4.201, and as part of the Sustainable Algal Biofuels Consortium project, funded under DOE Award # DE-EE0003372.
References
- J. C. Quinn, K. Catton, N. Wagner and T. H. Bradley, BioEnergy Res., 2011, 5, 49–60 CrossRef.
- R. Davis, A. Aden and P. T. Pienkos, Appl. Energy, 2011, 88, 3524–3531 CrossRef PubMed.
- P. J. L. B. Williams and L. M. L. Laurens, Energy Environ. Sci., 2010, 3, 554–590 CAS.
- L. Lardon, A. Hélias and B. Sialve, Environ. Sci. Technol., 2009, 43, 6475–6481 CrossRef CAS.
-
R. Davis, D. Fishman, E. D. Frank and M. S. Wigmosta, Renewable Diesel from Algal Lipids: An Integrated Baseline for Cost, Emissions, and Resource Potential from a Harmonized Model, http://www.nrel.gov/docs/fy12osti/55431.pdf, Golden, CO, 2012 Search PubMed.
- R. E. Davis, D. B. Fishman, E. D. Frank, M. C. Johnson, S. B. Jones, C. M. Kinchin, R. L. Skaggs, E. R. Venteris and M. S. Wigmosta, Environ. Sci. Technol., 2014, 48, 6035–6042 CrossRef CAS PubMed.
- P. M. Foley, E. S. Beach and J. B. Zimmerman, Green Chem., 2011, 13, 1399 RSC.
- P. Anastas and J. Zimmerman, Environ. Sci. Technol., 2003, 37, 94A–101A CrossRef.
- R. E. Teixeira, Green Chem., 2012, 14, 419 RSC.
- C. F. Murphy and D. T. Allen, Environ. Sci. Technol., 2011, 45, 5861–5868 CrossRef CAS PubMed.
- A. F. Clarens, H. Nassau, E. P. Resurreccion, M. a. White and L. M. Colosi, Environ. Sci. Technol., 2011, 45, 7554–7560 CrossRef CAS PubMed.
- P. Biller and a. B. Ross, Bioresour. Technol., 2011, 102, 215–225 CrossRef CAS PubMed.
- D. C. Elliott, T. R. Hart, A. J. Schmidt, G. G. Neuenschwander, L. J. Rotness, M. V. Olarte, A. H. Zacher, K. O. Albrecht, R. T. Hallen and J. E. Holladay, Algal Res., 2013, 2, 445–454 CrossRef PubMed.
- S. Changi, M. Zhu and P. E. Savage, ChemSusChem, 2012, 5, 1743–1757 CAS.
- D. Templeton, M. Quinn, S. Van Wychen, D. Hyman and L. M. L. Laurens, J. Chromatogr., A, 2012, 1270, 225–234 CrossRef CAS PubMed.
- M. M. Rebolloso-Fuentes, a. Navarro-Pérez, F. García-Camacho, J. J. Ramos-Miras and J. L. Guil-Guerrero, J. Agric. Food Chem., 2001, 49, 2966–2972 CrossRef CAS PubMed.
- R. H. Wijffels, M. J. Barbosa and M. H. M. Eppink, Biofuels Bioprod. Biorefin. Biofpr, 2010, 4, 287–295 CrossRef CAS.
- Q. C. Doan, N. R. Moheimani, A. J. Mastrangelo and D. M. Lewis, Biomass Bioenergy, 2012, 46, 79–88 CrossRef CAS PubMed.
- V. H. Work, R. Radakovits, R. E. Jinkerson, J. E. Meuser, L. G. Elliott, D. J. Vinyard, L. M. L. Laurens, G. C. Dismukes and M. C. Posewitz, Eukaryotic Cell, 2010, 9, 1251–1261 CrossRef CAS PubMed.
- Q. Hu, M. Sommerfeld, E. Jarvis, M. Ghirardi, M. Posewitz, M. Seibert and A. Darzins, Plant J., 2008, 54, 621–639 CrossRef CAS PubMed.
- P. E. Zemke, M. R. Sommerfeld and Q. Hu, Appl. Microbiol. Biotechnol., 2013, 97, 5645–5655 CrossRef CAS PubMed.
- L. Wang, Y. Li, M. Sommerfeld and Q. Hu, Bioresour. Technol., 2013, 129, 289–295 CrossRef CAS PubMed.
- L. M. L. Laurens, S. Van Wychen, J. P. McAllister, S. Arrowsmith, T. a. Dempster, J. McGowen and P. T. Pienkos, Anal. Biochem., 2014, 452, 86–95 CrossRef CAS PubMed.
- R. Harun, M. K. Danquah and G. M. Forde, J. Chem. Technol. Biotechnol., 2010, 85, 199–203 CAS.
- J. R. Miranda, P. C. Passarinho and L. Gouveia, Bioresour. Technol., 2012, 104, 342–348 CrossRef CAS PubMed.
- S.-H. Ho, S.-W. Huang, C.-Y. Chen, T. Hasunuma, A. Kondo and J.-S. Chang, Bioresour. Technol., 2013, 135, 191–198 CrossRef CAS PubMed.
- L. Laurens, M. Quinn, S. Van Wychen, D. Templeton and E. J. Wolfrum, Anal. Bioanal. Chem., 2012, 403, 167–178 CrossRef CAS PubMed.
-
S. Van Wychen and L. M. L. Laurens, Determination of Total Solids and Ash in Algal Biomass - Laboratory Analytical Procedure (LAP), http://www.nrel.gov/docs/fy14osti/60956.pdf, Golden, CO, 2013 Search PubMed.
-
S. Van Wychen and L. M. L. Laurens, Determination of Total Carbohydrates in Algal Biomass - Laboratory Analytical Procedure (LAP), http://www.nrel.gov/docs/fy14osti/60957.pdf, Golden, CO, 2013 Search PubMed.
-
L. M. L. Laurens, Summative Mass Analysis of Algal Biomass – Integration of Analytical Procedures Laboratory Analytical Procedure (LAP), http://www.nrel.gov/docs/fy14osti/60943.pdf, Golden, CO, 2013 Search PubMed.
-
S. Van Wychen and L. M. L. Laurens, Determination of Total Lipids as Fatty Acid Methyl Esters (FAME) by in situ Transesterification - Laboratory Analytical Procedure (LAP), http://www.nrel.gov/docs/fy14osti/60958.pdf, 2013 Search PubMed.
- J. Mossé, J. Agric. Food Chem., 1990, 18–24 CrossRef.
-
http://www.megazyme.com/downloads/en/data/K-TSTA.pdf, 2011.
- M. Balat and H. Balat, Appl. Energy, 2009, 86, 2273–2282 CrossRef CAS PubMed.
- N. D. Weiss, N. J. Nagle, M. P. Tucker and R. T. Elander, Appl. Biochem. Biotechnol., 2009, 155, 418–428 CrossRef CAS PubMed.
- D. D. Spindler, C. E. Wyman, A. Mohagheghi and K. Grohmann, Appl. Biochem. Biotechnol., 1988, 17, 279–293 CrossRef CAS.
- N. Dowe, Methods Mol. Biol., 2009, 581, 233–245 CAS.
- M. Zhang, C. Eddy, K. Deanda, M. Finkelstein and S. Pictaggio, Science, 1995, 267, 240–243 CrossRef CAS PubMed.
- T. D. Ranatunga, J. Jervls, R. F. Helm, J. D. Mcmillan and C. Hatzis, Appl. Biochem. Biotechnol., 1997, 67, 185 CrossRef CAS.
-
D. Humbird, R. Davis, L. Tao, C. Kinchin, D. Hsu and A. Aden, Process Design and Economics for Biochemical Conversion of Lignocellulosic Biomass to Ethanol, Golden, CO, 2011 Search PubMed.
- S. Schwede, A. Kowalczyk, M. Gerber and R. Span, Bioresour. Technol., 2013, 148, 428–435 CrossRef CAS PubMed.
-
Lower and Higher Heating Values of Fuels - DOE Hydrogen Analysis Resource Center, http://hydrogen.pnl.gov/cocoon/morf/projects/hydrogen/datasheets/lower_and_higher_heating_values.xls, 2008.
-
Fuel Properties Comparison by Alternative Fuels Data Center, http://www.afdc.energy.gov/fuels/fuel_comparison_chart.pdf, 2013.
- M. Balat and H. Balat, Appl. Energy, 2009, 86, 2273–2282 CrossRef CAS PubMed.
- D. W. Templeton, C. J. Scarlata, J. B. Sluiter and E. J. Wolfrum, J. Agric. Food Chem., 2010, 58, 9054–9062 CrossRef CAS PubMed.
-
H. Shapouri, M. Salassi and N. J. Fairbanks, The Economic Feasibility of Ethanol
Production from Sugar in the United States, Baton Rouge, LA, 2006 Search PubMed.
- J. M. Ageitos, J. A. Vallejo, P. Veiga-Crespo and T. G. Villa, Appl. Microbiol. Biotechnol., 2011, 90, 1219–1227 CrossRef CAS PubMed.
- I. R. Sitepu, L. a. Garay, R. Sestric, D. Levin, D. E. Block, J. Bruce German and K. L. Boundy-Mills, Biotechnol. Adv., 2014, 32, 1336–1360 CrossRef CAS PubMed.
- H. Wei, W. Wang, J. M. Yarbrough, J. O. Baker, L. Laurens, S. Van Wychen, X. Chen, L. E. Taylor, Q. Xu, M. E. Himmel and M. Zhang, PLoS One, 2013, 8, e71068 CAS.
-
R. Davis, L. Tao, E. C. D. Tan, M. J. Biddy, G. T. Beckham and C. Scarlata, Process Design and Economics for the Conversion of Lignocellulosic Biomass to Hydrocarbons: Dilute-Acid and Enzymatic Deconstruction of Biomass to Sugars and Biological Conversion of Sugars to Hydrocarbons, Golden, CO, 2013 Search PubMed.
- R. Harun and M. K. Danquah, Process Biochem., 2011, 46, 304–309 CrossRef CAS PubMed.
- O. K. Lee, a. L. Kim, D. H. Seong, C. G. Lee, Y. T. Jung, J. W. Lee and E. Y. Lee, Bioresour. Technol., 2013, 132, 197–201 CrossRef CAS PubMed.
-
J. A. Barnett, R. W. Payne and D. Yarrow, Yeasts: Characteristics and Identification, Cambridge University Press, Cambridge, UK, 3rd edn, 2000 Search PubMed.
- J. Swings and J. De Ley, Bacteriol. Rev., 1977, 41, 1 CAS.
- E. Palmqvist and B. Hahn-Hagerdal, Bioresour. Technol., 2000, 74, 25–33 CrossRef CAS.
- J. Shekiro Iii, E. M. Kuhn, N. J. Nagle, M. P. Tucker, R. T. Elander and D. J. Schell, Biotechnol. Biofuels, 2014, 7, 23 CrossRef PubMed.
-
R. W. Harris and M. J. J. Cullinane, Process Design and Cost Estimating Algorithms for the Computer Assisted Procedure for Design and Evaluation of Wastewater Treatment Systems, 1982.
-
G. Tchobanoglous, F. L. Burton and H. D. Stensel, Wastewater Engineering: Treatment and Reuse, McGraw-Hill Education, Boston, 2003 Search PubMed.
-
L. K. Wang, Biosolids Treatment Processes, Humana, Totowa, NJ, 2007 Search PubMed.
- MYPP-US Department of Energy, http://www1.eere.energy.gov/bioenergy/pdfs/mypp_may_2013.pdf, 2013.
-
A. Dutta, M. Talmadge, J. Hensley, M. Worley, D. Dudgeon, D. Barton, P. Groenendijk, D. Ferrari, B. Stears, E. M. Searcy, C. T. Wright and J. R. Hess, Process Design and Economics for Conversion of Lignocellulosic Biomass to Ethanol - Thermochemical Pathway by Indirect Gasification and Mixed Alcohol Synthesis, Golden, CO, 2011 Search PubMed.
Footnote |
† Electronic supplementary information (ESI) available. See DOI: 10.1039/c4gc01612b |
|
This journal is © The Royal Society of Chemistry 2015 |
Click here to see how this site uses Cookies. View our privacy policy here.