DOI:
10.1039/D4FO01598C
(Paper)
Food Funct., 2024,
15, 8258-8273
N-Glycan profile of the cell membrane as a probe for lipopolysaccharide-induced microglial neuroinflammation uncovers the effects of common fatty acid supplementation†
Received
5th April 2024
, Accepted 30th June 2024
First published on 16th July 2024
Abstract
Altered N-glycosylation of proteins on the cell membrane is associated with several neurodegenerative diseases. Microglia are an ideal model for studying glycosylation and neuroinflammation, but whether aberrant N-glycosylation in microglia can be restored by diet remains unknown. Herein, we profiled the N-glycome, proteome, and glycoproteome of the human microglia following lipopolysaccharide (LPS) induction to probe the impact of dietary and gut microbe-derived fatty acids—oleic acid, lauric acid, palmitic acid, valeric acid, butyric acid, isobutyric acid, and propionic acid—on neuroinflammation using liquid chromatography-tandem mass spectrometry. LPS changed N-glycosylation in the microglial glycocalyx altering high mannose and sialofucosylated N-glycans, suggesting the dysregulation of mannosidases, fucosyltransferases, and sialyltransferases. The results were consistent as we observed the restoration effect of the fatty acids, especially oleic acid, on the LPS-treated microglia, specifically on the high mannose and sialofucosylated glycoforms of translocon-associated proteins, SSRA and SSRB along with the cell surface proteins, CD63 and CD166. In addition, proteomic analysis and in silico modeling substantiated the potential of fatty acids in reverting the effects of LPS on microglial N-glycosylation. Our results showed that N-glycosylation is likely affected by diet by restoring alterations following LPS challenge, which may then influence the disease state.
1. Introduction
N-Linked glycosylation is a common and important post-translational modification of proteins mainly through the addition of glycans to the amide group of asparagine residues in the consensus sequence, Asn-X (any amino acid except proline)-Ser/Thr.1 It is more prominent on cell surface proteins, secreted proteins or on intracellular organelle's membrane proteins such as Golgi, lysosome, and ER,2–4 which allow involvement in many cellular interactions implicated in a host of disease states and inflammation including cell-to-cell interactions and immunogenicity.5 In turn, it is a critical modification to the central nervous system (CNS) where alterations in the glycosylation affect neuroinflammation and may lead to neurodegenerative diseases over time.6,7 However, targeting glycoproteins in general and not just for neuroinflammation therapeutics remains underexploited.8 In Alzheimer's Disease (AD), for example, the N-glycome mapping of the elderly human brain was performed extensively across 11 brain regions showing the differential glycosylation of each region, where the trend was generally highly branched and highly sialofucosylated N-glycome with observed regional variations with regard to glycan types including high mannose and complex-type structures.9N-Acetylglucosaminyltransferases, which are responsible for highly branched multi-antennary N-glycan structures and key enzymes in glycoprotein biosynthesis, are also identified to be crucial in neuroinflammation with MGAT3 found to be increased in the brains of AD patients.10 In another study using the frontal cortex and hippocampus from AD patients, specific N-glycans including one containing a terminal galactose with sialofucosylated residues were reduced compared to that of non-AD subjects.11 Furthermore, proteins highly expressed in activated microglia were found to alter the glycosylation profiles in AD with ApoE, TREM2, and CD33.6,12–20 Considering that glycans and glycoproteins have been reported to play significant roles in neuroinflammatory cascades,21 there is paucity of the literature on glycoproteins being targeted for disease therapeutics.
Microglia, resident immune cells of the CNS, serve as an ideal model for studying the link between glycosylation and neuroinflammation, as they exhibit characteristic molecular changes including morphological differentiation, migration, phagocytosis, cytokine release, and inflammatory responses.22–24 Microglial activation has been identified as an important contributor to the pathological mechanisms of neuroinflammation in neurodegenerative diseases.22,24–26 The different functional states of microglia affecting their glycosylation at the different stages of chronic neuroinflammation is crucial to understanding the role of microglia in neurodegeneration. The activated microglia have been associated with several glycoenzymes, especially β1,4-galactosyltransferase,27–29 β1,4-galactosyltransferase V (β-1,4-GalT V),29 and β-1,4-GalT I.28 In a recent study, sialylation and desialylation carried out with sialyltransferases and sialidases, respectively, on the non-reducing termini of oligosaccharide chains to either glycoproteins or glycolipids were observed to affect the inflammatory activation of the microglia such that high sialylation of the neuronal cell surface inhibits microglial phagocytosis of the neurons via activation of Siglecs (sialic acid receptors), CD22 and CD33 or via binding inhibition of Toll-like receptor 4 (TLR4), complement receptor 3 (CR3), and other microglial receptors.30
Although accumulation of lipids is linked to neurodegenerative conditions,31,32 both dietary and gut microbe-derived fatty acids were interestingly found to modulate neuroinflammation through microglial activation.32–35 Fatty acids mainly from diet accumulate predominantly in the liver and adipose tissue where uptake from the plasma and de novo biosynthesis take place.36–39 While diet independently affects energy metabolism, the gut microbiome is also involved in energy harvest through the production of short-chain fatty acids by fermentation of dietary fiber.37,40–42 Fatty acids are transported across the blood brain barrier (BBB) either by passively diffusing across the endothelial membrane or transferring inside the endothelium via a transmembrane protein, and then once in the endothelium of the BBB, fatty acid shuttles through the cytosol by binding to fatty acid-binding proteins.37,38 Lipids and lipid metabolism are closely linked with microglia as they have receptors for lipid mediators involved in the control of microglial activation and inflammatory signaling.43,44 Microglia also express several lipid-related genes such as triggering receptors expressed on myeloid cells 2 (TREM2) which if mutated can lead to abnormalities in lipid metabolism and diseases like Alzheimer's disease.43,44 We raise the question whether food, especially fatty acids either directly consumed from diet or produced by gut microbes, can affect altered N-glycosylation in the microglia.
In this study, we performed an integrative LC-MS/MS-based pipeline starting with N-glycomics of in vitro neuroinflammation models using several glial cell types (astroglia, medulloblastoma and microglia) to explore effects of LPS on glial glycocalyx, followed by N-glycomic, proteomic and site-specific glycoproteomic profiling of the microglia. We then used these N-glycosylation characterization studies to probe the distinguishing profiles of neuroinflammation apart from non-neuroinflammation, which opens opportunity to assess therapeutics, specifically the effects of fatty acids such as oleic acid, lauric acid, palmitic acid, valeric acid, butyric acid, isobutyric acid and propionic acid on the N-glycome, proteome and glycoproteome of cell membranes from human microglial cells.
2. Materials and methods
2.1. Cell culture and LPS treatment
The cell lines, SVG p12 – CRL-8621 (also SVG, human brain fibroblast astroglia), DAOY – HTB-186 (human desmoplastic cerebellar medulloblastoma) and HMC3 – CRL-3304 (human microglial cell) were all obtained from the American Type Culture Collection (ATCC, VA, USA). Both DAOY and HMC3 were grown in ATCC Eagle's Minimum Essential Medium (EMEM), while SVG p12 was grown in ATCC RPMI-1640. Media were supplemented with 10% fetal bovine serum, 1% penicillin–streptomycin (Thermo Fisher Scientific, MA, USA), and 30 μM bovine serum albumin (1 g of lyophilized BSA, Sigma-Aldrich, MO, USA), which is used as a carrier to ensure FA dissolution. Cells were cultured in T75 flasks at 37 °C under 5% CO2, with the media changed every other day. Upon reaching 80% confluency, cells were treated with O55:B5 lipopolysaccharide (Sigma-Aldrich, USA) to a final concentration of 100 ng mL−1 and fatty acids with the following final concentration for the long/medium-chain at 100 μM and for the short-chain at 1000 μM, accordingly. Concentrations of LPS and fatty acids are based on the literature and validated by cell viability assays (ESI Fig. 1†).34,45–47 After 24 hours of incubation, cells were harvested for mass spectrometry-based analysis using a previously reported general protocol.2,48,49 Experiments were conducted with three biological replicates for each treatment.
2.2. Sample preparation for mass spectrometry analyses
Glycomic, proteomic and glycoproteomic analyses of the control and LPS- and fatty acid-treated HMC3 cell lines were performed following a previously developed protocol, which was optimized and described in Alvarez et al. (2022) and Li et al. (2020, 2019). Samples were prepared for N-glycan, peptide, and glycopeptide extraction from the cell surface membrane fractions before mass spectrometric analyses. The samples were kept on ice during all steps of preparation. During harvest, samples were washed twice with cold PBS buffer (Dulbecco's phosphate-buffered saline, Gibco) and were added with 1.2 mL of homogenization buffer (HB) containing 0.25 M sucrose, 20 mM HEPES-KOH (pH 7.4) and 1
:
100 protease inhibitor cocktail (EMD Millipore, Temecula, CA, USA). The sample was then lysed using a sonicator probe (QSonica, Newtown, CT, USA). Then, the samples were centrifuged at 2000g for 10 minutes at 4 °C using an Eppendorf microcentrifuge 5425 (Eppendorf, Enfield, CT, USA). The pellet containing cell debris and nucleus was discarded, and the supernatant was subsequently centrifuged at 42
000 rpm for 45 minutes at 4 °C to separate cell debris from biomolecules of interest, e.g. N-glycans, proteins and glycoproteins. Ultracentrifugation was performed using Sorvall WX100 + equipped with a Fiberlite F50L-24 × 1.5 fixed-angle rotor (Thermo Fisher Scientific, Waltham, MA, USA). The pellet obtained enriched in cell membrane proteins and glycoproteins was washed with 500 μL of 0.2 M Na2CO3 and centrifuged at 42
000 rpm for 45 minutes at 4 °C. Lastly, the subsequent pellet was washed with deionized water for 42
000 rpm for 45 minutes (4 °C) to wash out excess salts and contaminants. The membrane fractions obtained were stored at −20 °C until further analysis.
2.3.
N-Glycan release, PGC cleanup, and chip-QToF LC-MS/MS analysis
An aliquot of the extracted membrane fractions were treated with dithiothreitol (DTT) and glycerol-free peptide N-glycosidase F (PNGase F from New England Biolabs, MA, USA) and subsequently centrifuged to release the N-glycans into the supernatant. Prior to mass spectrometry analysis, N-glycans were desalted using porous graphitic carbon (PGC) SPE plates (Glygen Corp.), and then dried in vacuo using miVac (SP Scientific, PA, USA).
LC-MS/MS was performed using Chip/Q-ToF LC-MS/MS at 5 μL of injection. N-glycan profiles were obtained using an Agilent nanochip-QTOF (quadrupole time-of-flight)-MS mass spectrometer.48–50N-Glycan samples were reconstituted in 40 μL of water, and 5 μL of the resulting solution was used for injection into the nano-LC-MS/MS system. Separation was performed using an Agilent PGC-Chip II with a 40 nL enrichment and 43 mm × 75 μm analytical column (with a particle size of 5 μm) and a binary solvent system composed of mobile phase A (3% v/v acetonitrile and 0.1% v/v formic acid in water) and mobile phase B (90% v/v acetonitrile and 1% v/v formic acid in water). The gradient sequence for separation used was: 0–2.5 min, 1% B; 2.5–20 min, 16% B; 20–35 min, 58% B; 35–40 min, 100% B 40–50 min, 100% B; 50.01–65 min, 0% B with a flow rate of 0.3 μL min−1. Tandem MS spectra were acquired via collision-induced dissociation (CID), with spectra measured at 0.8 s per spectrum over a mass range of m/z 100 to 2000 in the positive ion mode.
N-Glycomic data were analyzed using Agilent MassHunter Qualitative Analysis (B.08.00), with database matching to an in-house glycan database at 10 ppm mass error. Quantification was also performed to determine percent relative abundances of each glycan types – high mannose, undecorated, fucosylated, sialylated, and sialofucosylated. Discovery of significantly different glycans was analysed using multiple t-tests at a false discovery rate (FDR) of 5%. Potential biosynthetic pathway and structure was annotated by database matching and literature analysis.
2.4. Protein digestion, peptide cleanup, glycopeptide enrichment, and nanoLC-Orbitrap LC-MS/MS analysis
After cell lysis and ultracentrifugation, samples were treated with 8 M urea and subsequently sonicated to resuspend the proteins and glycoproteins. Afterwards, dithiothreitol was added and the samples were incubated for 50 minutes at 55 °C. An alkylating agent, iodoacetamide (IAA), was also added and subsequently incubated for 20 minutes in the dark to alkylate the cysteine residues. To quench the reaction, 50 mM ammonium bicarbonate was added to each reaction. Finally, 10 μL of trypsin (with additional 10 μL of Glu-C in glycoproteomics) was added to each reaction and subsequently incubated at 37 °C for 18 hours to cleave the proteins into peptide fragments. After the incubation period, samples for proteomic analysis were cleaned up using C18 solid phase extraction (SPE) cartridges (Supelco DSC-18), while samples for glycoproteomic analysis were cleaned up using Hydrophilic Interaction Liquid Chromatography (HILIC) using iSPE HILIC cartridges (HILICON, 1 mL, 100 mg,). Prior to mass spectrometric analysis using a nanoLC paired with OrbiTrap, samples were dried and then dissolved in ultrapure water to make a 0.5 μg μL−1 concentration.
Digested peptides and glycopeptides in one (1) μL of the injected sample were separated using an Acclaim™ PepMap™ 100C18 LC Column (75 μm × 150 mm, particle size: 2 μm; Thermo Fisher Scientific) at a flow rate of 300 nL min−1, and then analyzed in an UltiMate™ WPS-3000RS nanoLC system coupled with an Orbitrap Fusion Lumos MS system (Thermo Fisher Scientific). Solvents A and B were prepared using water containing 0.1% formic acid and 80% acetonitrile containing 0.1% formic acid, respectively. MS spectra with a mass range of m/z 700–2000 for MS1 and m/z ≥120 for MS2 were collected at a rate of 1.5 s per spectrum in the positive ionization mode.
Glycoprotein and protein expression data were calculated from the extracted ion chromatogram-normalized peptide intensities. Glycoproteomic and proteomic mass spectrometry data were analyzed using ProteinMetrics's Byonic (v.3.5.0) for database matching and quantified using Byologic software (v.3.5.0). Specifically, glycoproteins were identified through filtering based on score >100 and Delta Mod score >10, and categorized based on the N-glycan type – high mannose, undecorated, fucosylated, sialylated and sialofucosylated. Differentially expressed proteins and glycoproteins were then analyzed using multiple t-tests at an FDR of 5%. Functional analysis was performed using the UniProt Knowledgebase, also UniProtKB (uniprot.org), and plotted using GraphPad prism (version 9.3.1).48
2.5.
In silico molecular docking and protein–protein modeling
3D crystal structure of the human TLR4-human MD-2 complex, 3FXI (PDB https://doi.org/10.2210/pdb3fxi/pdb), from Protein Data Bank was selected and loaded onto PyRx51 and docked against dietary fatty acid and gut–microbe derived fatty acids using the AutoDock VINA docking protocol.52 Binding against propionic acid (short-chain fatty acid) and palmitic acid (long chain fatty acid) were visualized using Discovery Studio™ (version 21.1.0.20298, Dassault Systemes, BIOVIA Corp., San Diego, CA, USA).
In silico modeling between SSRA, SSRB (translocon-associated protein subunit alpha and beta) and STT3B (dolichyl-diphosphooligosaccharide – protein glycosyltransferase subunit STT3B) was also carried out to predict the involvement of sialofucosylation in TRAP–OST protein complex interactions. The crystal structures of the selected proteins were downloaded from PDB (https://doi.org/10.2210/pdb8B6L/pdb). Select glycans from glycopeptides identified from glycoproteomic analysis were attached to SSRA, SSRB and STT3B using a CHARMM-GUI glycan modeler using a CHARMM36 force field for both proteins and carbohydrates.53 Using VMD, protein–protein interactions were visualized, and the number of interacting hydrogen bonds between the glycans and proteins was analyzed.54
2.6. Quantitative analysis of glycomic, proteomic and glycoproteomic data
N-Glycomic compositions were determined using Agilent MassHunter Qualitative Analysis (B.08.00) against an in-house database based on the human N-glycan biosynthetic pathway which allows classification into glycan types such as high mannose, undecorated, sialylated, fucosylated, and sialofucosylated. Quantification of N-glycans was acquired using relative abundance, wherein ion counts for observed glycan masses were integrated and then normalized to the summed ion counts of all glycans detected.
The mass spectrometry data for glycoproteomics and proteomics were analysed using ProteinMetrics's Byonic (v.3.5.0) and quantified using Byologic software (v.3.5.0). Proteins were compared against the human proteome database using a precursor mass tolerance of 20 ppm and fragment mass tolerance of 10 ppm. Glycoprotein and protein expression data were calculated based on the extracted ion count (XIC) area sum of top 3 most abundant peptides. Glycoform quantification was normalized to each protein's glycosite to generate the percentage occupancy of a particular glycoform.
Discovery of significantly different glycan types between LPS and control was initially analysed using multiple t-tests at a false discovery rate (FDR) of 5%. Significant N-glycans, proteins, and glycoproteins among all fatty acid-treated samples vs. LPS and control were then obtained using two-way ANOVA analysis with correction for multiple comparisons via Dunnett's statistical hypothesis testing and reporting multiplicity adjusted P value for each comparison at 95% confidence interval. For proteomics, log2fold-change equivalent to the relative abundance of protein in LPS treatment normalized to that of the control is plotted in a heatmap with proteins on the x-axis and samples on the y-axis. Volcano plot was generated by plotting −log
10 (p value) on the x-axis and log2fold-change on the y-axis. P values were obtained from comparing the control and LPS-treated samples using a multiple unpaired t-test with a single pooled SD for all samples and a two-stage linear step-up procedure of Benjamin, Krieger and Yekutieli. Statistics and plots were performed using GraphPad prism (version 9.3.1).
3. Results and discussion
3.1. LC-MS/MS characterization of glycosylation in microglia
More than 70% of proteins in the brain are glycosylated which makes protein glycosylation necessary for proper brain development and maintenance,9,19,55–58 wherein alterations in glycosylation may cause detrimental changes in the brain's function.10,11N-Glycosylation in human and mouse brains are reported to commonly have high mannose, and to be of fucosylated type and sialofucosylated type.9,55 The neurodegenerative phenotype can be mimicked using induction of LPS in cells and thus has been used as an in vitro model for Alzheimer's disease.30,59
While neuron dysfunction is a critical feature of cognitive decline, it is recognized that the non-neuronal types such as microglia and astrocytes also help in maintaining the proper brain function and in ensuring the homeostasis and survival of neurons.60,61 To investigate the effect of LPS on glial activation, we first started with a panel of three cell lines – SVG p12 (astroglia), HMC3 (microglia) and DAOY (medulloblastoma) – on which we conducted N-glycomic and glycoproteomic analyses (ESI Fig. 2†). The N-glycomic results showed significant changes with high mannose and sialofucosylated structures upon LPS treatment. We observed the greatest changes, especially in the glycoproteome of HMC3, hence we chose the microglial cell model for further analyses. Changes in microglial glycosylation have been found to have effects on neurodegeneration, such as the sialylation of the microglia is reported to attenuate the development of neurodegenerative disorders by inhibiting neuroinflammation and phagocytosis.30,62
Subsequently, we employed in vitro microglial cell models of LPS-induced neuroinflammation26,59,63–65 to explore the effects of dietary and gut microbiota-derived fatty acids. We co-treated microglial cells with LPS and a physiological dose of dietary fatty acid (oleic, palmitic, or lauric) or gut microbiota-derived fatty acid (valeric, isobutyric, butyric, or propionic) The LC-MS/MS workflow is depicted in Fig. 1. These fatty acids were selected due to their high abundances in diet and in gut microbial fermentation. After treatments, cells were harvested and prepared for analysis using nanoLC-QToF (for N-glycomics) and nLC-Orbitrap (for site-specific glycoproteomics). With the N-glycomic method, we quantified more than 300 unique N-glycan structures, while the site-specific glycoproteomic method quantified more than 2000 glycopeptides. Proteomics was also carried out to track the N-glycosylation pathways affected by LPS and the fatty acid treatments. We detected 19 proteins involved in N-glycan precursor synthesis and the attachment of N-glycan precursor using a label-free proteomics method (ESI Fig. 3†).
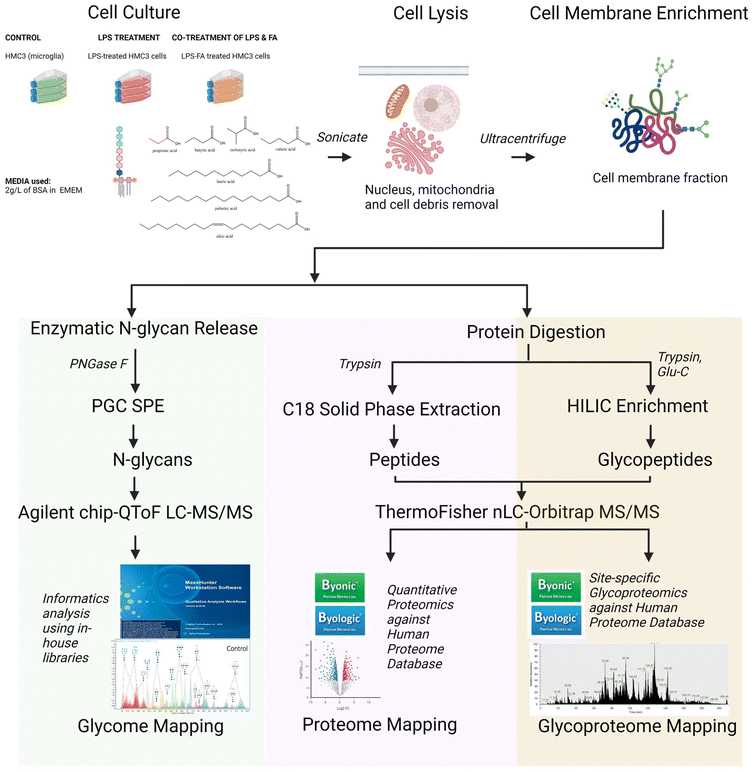 |
| Fig. 1 Overview of the workflow for the extensive LC-MS/MS based multi-omics analysis. The microglial cells (HMC3) were treated with LPS and fatty acids and were harvested after 24 hours (n = 3). The cells were lysed and ultracentrifuged to enrich macromolecules of interest. The steps for the N-glycomics, proteomics and site-specific glycoproteomics were outlined. LPS = lipopolysaccharide; FAs = fatty acids; BSA = bovine serum albumin; EMEM = Eagle's minimum essential medium; SPE = solid phase extraction; PGC = porous graphitic carbon; HILIC = hydrophilic interaction liquid chromatography. | |
3.2. LPS targets high mannose and sialofucosylated N-glycans in microglia
Upon treatment with LPS, we observed a drastic and significant decrease in high mannose N-glycans with a corresponding increase in the abundance of sialofucosylated N-glycans (Fig. 2a and b). Analysis of each individual N-glycan structure revealed that LPS treatment of microglial cells decreased the abundance of all high mannose type structures: Hex9HexNAc2, Hex8HexNAc2, Hex7HexNAc2, Hex6HexNAc2 and Hex5HexNAc2 (Fig. 2c). On the other hand, LPS treatment increased the abundance of specific sialofucosylated structures with varying degree of branching (bi-, tri-, and tetra-antennary) and varying degree of sialylation (mono- or bi-sialylated) (Fig. 2c). Tandem MS spectra of select differentially expressed N-glycans were mapped to confirm structures based on fragments, i.e. high mannose N-glycan structure, Hex9HexNAc2 (ESI Fig. 4a†), and sialofucosylated tetra-antennary glycan, Hex7HexNAc6Fuc1NeuAc2, which is composed of 2 isomers with either core fucose or terminal/antennary fucose residues (ESI Fig. 4b and c†). Therefore, LPS significantly alters the microglial glycocalyx, increasing the complex type of N-glycan. In the glycosylation pathway, high mannose structures represent early-stage N-glycans, typically added to nascent glycoproteins. Thus, decrease in high mannose is linked to dysregulated activities of mannosidases, enzymes responsible for trimming mannose residues during glycan maturation. On the other hand, increased sialofucosylation points towards enhanced addition of sialic and fucose residues to glycan structures. Sialofucosylated glycans are often found in mature glycoproteins on the cell surface. Therefore, the increase may be associated with hyperactive fucosyltransferases and sialyltransferases, which are enzymes involved in adding fucose and sialic acid, respectively. These results coincide with the N-glycan biosynthesis pathway, such that with increased activity of mannosidase, the pathway leads to creation of more complex-type N-glycans, wherein the N-glycan can be acted upon by several fucosyltransferase and sialyltransferase enzymes. The increase in fucosyltransferase activity following LPS treatment aligns with previous findings linking FUT8 (fucosyltransferase catalyzing core-fucosylation) activity to the regulation of AβO-induced microglial activation.66 This finding is consistent with a noticeable increase in core fucose levels observed during LPS treatment, a phenomenon detectable using our MS/MS fragmentation-based method and relative retention time analysis. As an example of probing the fucose position (either core or terminal/antennary) in N-glycans, we present herein sialofucosylated tetra-antennary N-glycan, Hex7HexNAc6Fuc1Sia1, which has an apparent increase in core fucosylation during neuroinflammation (ESI Fig. 5†).
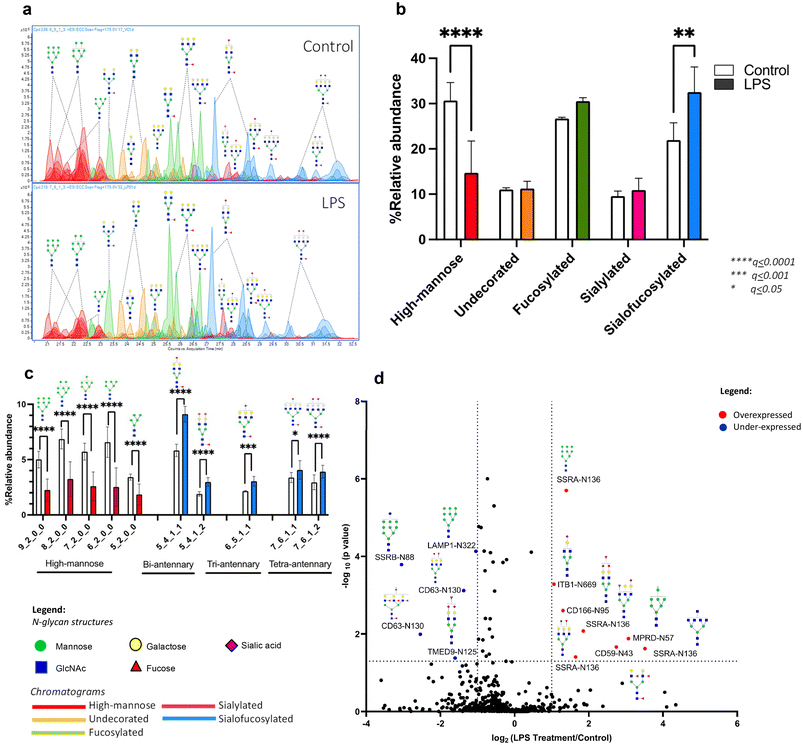 |
| Fig. 2 LPS-induced neuroinflammation changes microglial N-glycosylation. Changes observed after 24 h exposure of LPS in glycosylation using (a, b and c) N-glycomics analysis (n = 3), where representative chromatograms and bar graphs show that LPS significantly affects high-mannose and sialofucosylated N-glycans, and (d) site-specific glycoproteomics analysis (n = 3), where the volcano plot shows overexpressed (red) and underexpressed (blue) glycoforms. | |
We next determined the specificity of these changes in microglial glycosylation by LPS using site-specific glycoproteomics. Upon LPS treatment, we observed that 28 glycoproteins altered glycosylation in over 30 unique glycosites (Fig. 2d). We observed underexpression of SSRB (translocon-associated protein subunit beta) and LAMP1 (lysosome-associated membrane glycoprotein 1) high mannose glycoforms, and overexpression of CD166 (activated leukocyte cell adhesion molecule/ALCAM), SSRA (translocon-associated protein subunit alpha), and CD59 (protectin) sialofucosylated glycoforms. CD166 and CD59 glycoproteins are known to be important cell surface signaling molecules, while SSRA, SSRB, and LAMP1 are proteins involved in protein processing. The functions of these significantly different proteins have been previously shown to be affected by altered glycosylation in relation to inflammation.67–71 In particular, the underexpression of high mannose glycoforms indicate dysfunction in protein folding especially of SSRB and LAMP1 as a response to ER stress after injury.67–69 Microglial expression of CD166, a molecule activating cell leukocyte adhesion, and CD59, protecting cells from complement-mediated lysis are also found to be involved in promoting inflammatory activity during disease progression.70,71 The observed decrease in sialylation is consistent with the report that neuroinflammation can promote desialylation.30
3.3. Dietary and gut microbiota-derived fatty acids ameliorate the effect of LPS in microglial glycosylation
We further investigated whether our diet and the gut microbiota can alleviate the changes of LPS on microglia glycosylation. Diet-derived (oleic, palmitic and lauric) and gut microbiota-derived (valeric, butyric, isobutyric and propionic) fatty acids were co-treated with LPS into microglial cells, followed by N-glycomic and site-specific glycoproteomic analyses.
Comparing the effects of diet-derived fatty acids (oleic, palmitic and lauric), we observed a distinctive reversal of the LPS-induced effects by oleic acid both in terms of high mannose and sialofucosylated N-glycans (Fig. 3a). We analyzed each individual high mannose structure – Hex9HexNAc2, Hex8HexNAc2, Hex7HexNAc2, Hex6HexNAc2 and Hex5HexNAc2 – and found that oleic acid increased high mannose abundances to levels equivalent to that of the controls (Fig. 3b). The equivalent effect was observed in sialofucosylated N-glycans, wherein oleic acid co-treatment reduced the effect of LPS in bi-, tri-, and tetra-antennary sialofucosylated N-glycans to levels comparable to the control (Fig. 3c). Thus, among the diet-derived fatty acids studied, oleic acid was demonstrated the most pronounced ability to counteract the effects of LPS. Oleic acid has been previously reported to inhibit microglial nitric oxide production modulating the TLR2-mediated inflammatory response in microglia.72
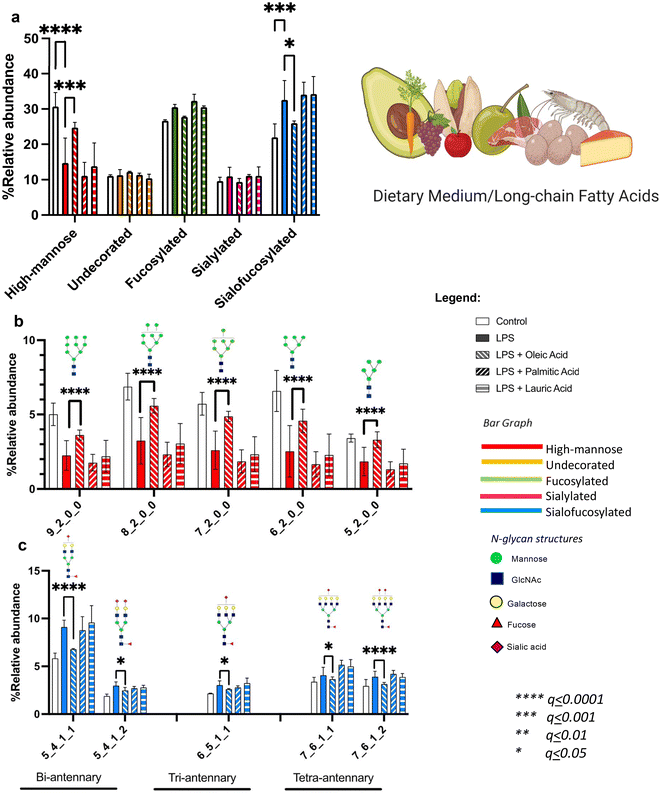 |
| Fig. 3
N-Glycome profiles of LPS-dietary fatty acid treated microglia. Microglia were co-treated with LPS and dietary fatty acids: oleic, palmitic, and lauric acids (n = 3). (a) % Relative abundance of glycan types shows that high mannose and sialofucosylated N-glycans were significantly affected by LPS. The specific (b) high mannose structures and (c) sialofucosylated structures are shown. | |
We also analyzed the effects of gut microbiota-derived fatty acids (valeric, butyric, isobutyric, and propionic). These compounds are known to be products of carbohydrate fermentation by bacteria in the human gut. In the co-treatment, we found that only isobutyric acid influenced the overall high mannose reduction by LPS (Fig. 4a). However, looking into each individual high mannose N-glycan showed that both isobutyric and propionic acids also alleviated LPS effects on specific structures (Fig. 4b). Isobutyric acid significantly increased the abundances of Hex9HexNAc2, Hex8HexNAc2, Hex7HexNAc2, Hex6HexNAc2, and Hex5HexNAc2, while propionic acid increased the amounts of Hex9HexNAc2, Hex8HexNAc2, Hex6HexNAc2, and Hex5HexNAc2. On the other hand, comparing the effects on sialofucosylated N-glycans showed that butyric, isobutyric, and propionic acids decreased the abundances of specific sialofucosylated structures (Fig. 4c). Butyric acid reverted the effects of LPS on the tetra-antennary Hex7HexNAc6Fuc1Sia1 and Hex7HexNAc6Fuc1Sia2N-glycans. Isobutyric acid reduced the amounts of bi-antennary (Hex5HexNAc4Fuc1Sia1 and Hex5HexNAc4Fuc1Sia2), tri-antennary (Hex6HexNAc5Fuc1Sia1), and tetra-antennary (Hex7HexNAc6Fuc1Sia1 and Hex7HexNAc6Fuc1Sia2) N-glycans. Lastly, propionic acid reduced the effects of LPS on bi- (Hex5HexNAc4Fuc1Sia1) and tri-antennary (Hex6HexNAc5Fuc1Sia1) N-glycans. From these results, the gut microbiota-derived fatty acids isobutyric, butyric, and propionic acids alleviated the effects of LPS in an N-glycan structure-specific manner. In general, short-chain fatty acids produced by the gut microbiome have been suggested to regulate microglial inflammation through the gut–brain axis, specifically affecting histone deacetylase (HDAC) activity and decreasing nuclear p65 NF-KB, which can inhibit inflammatory microglia following LPS stimulation.73
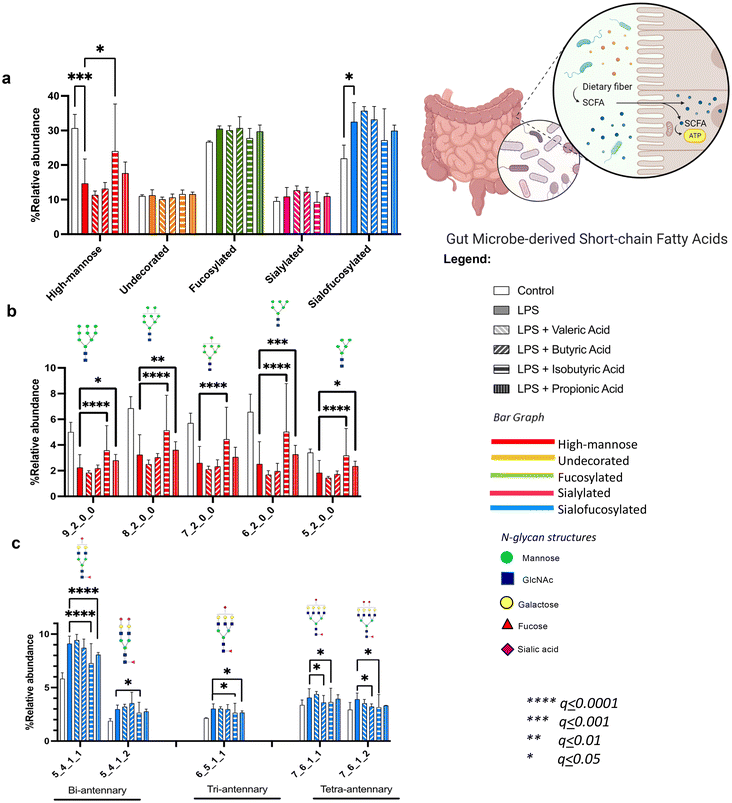 |
| Fig. 4
N-Glycome profiles of LPS-gut microbe-derived fatty acid treated microglia. Microglia were co-treated with LPS and gut microbe-derived fatty acids: valeric, butyric, isobutyric and propionic acids (n = 3). (a) % Relative abundance of glycan types show that high mannose and sialofucosylated N-glycans were significantly affected by LPS. The specific (b) high mannose structures and (c) sialofucosylated structures are shown. | |
3.4. Effects of LPS on N-glycosylation of specific glycoproteins are alleviated by dietary and gut microbiota-derived fatty acids
Based on the N-glycomic results, we found that both dietary and gut microbiota-derived fatty acids alleviate the effects of LPS on microglia glycosylation. Subsequently, we also determined the effects of LPS on specific glycoprotein, wherein it reduced the abundance of SSRB and LAMP1 high mannose glycoforms, while increasing the abundance of CD166, SSRA, and CD59 sialofucosylated glycoforms. We next determined whether the fatty acids alleviated the effects of LPS on these specific glycoproteins using glycoproteomic analysis.
With regard to the dietary fatty acids (oleic, palmitic and lauric), we found that both oleic and palmitic acids alleviated the effect of LPS on SSRB protein's high mannose N-glycan Hex9HexNAc2 (Fig. 5a). On the other hand, oleic acid reverted the effects of LPS on sialofucosylated N-glycans in CD166 (Hex5HexNAc4Fuc1Sia2) and SSRA (Hex6HexNAc5Fuc1Sia1, Hex5HexNAc5Fuc1Sia1) (Fig. 5b). Lauric acid was also able to reduce the levels of sialofucosylated N-glycan in CD166 (Hex5HexNAc4Fuc1Sia2).
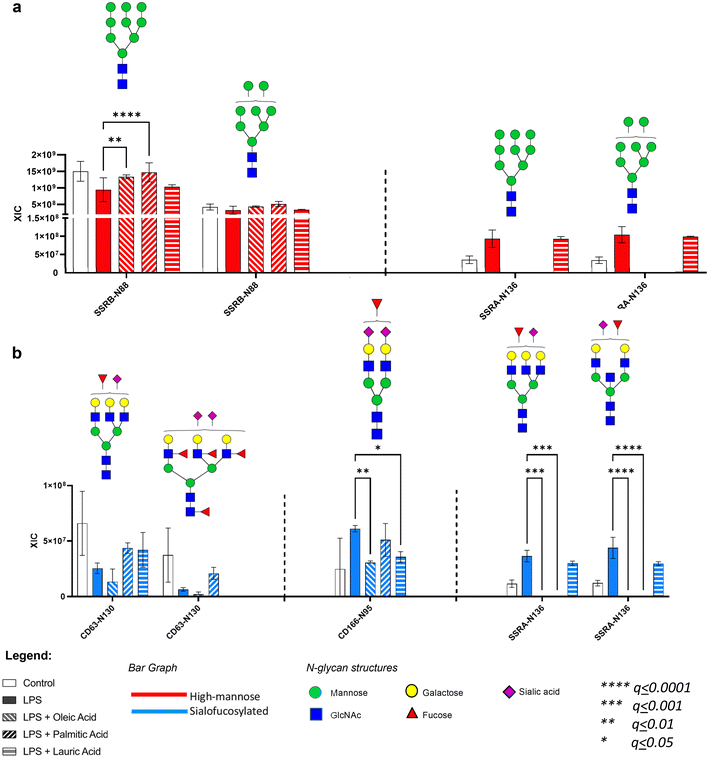 |
| Fig. 5
N-Glycoproteomic profiles of LPS-dietary fatty acid treated microglia. The changes of the microglial glycoproteome were also observed after 24 h treatment of LPS and dietary fatty acids: oleic, palmitic and lauric acids (n = 3). The extracted ion counts of specific glycoproteins with changing (a) high mannose structures and (b) sialofucosylated structures are shown. | |
Likewise, we also observed that gut microbe-derived fatty acids (valeric, butyric, isobutyric and propionic) ameliorated select LPS effects on microglia glycosylation. In terms of high mannose glycosylation, valeric acid reverted SSRB (Hex9HexNAc2, Hex8HexNAc2) glycosylation, while butyric acid reverted SSRA (Hex9HexNAc2 and Hex8HexNAc2) glycosylation (Fig. 6a). In terms of sialofucosylated species, isobutyric acid reverted the effects of LPS on CD63 (Hex6HexNAc5Fuc4Sia2), CD166 (Hex5HexNAc4Fuc1Sia2), and SSRA (Hex6HexNAc5Fuc1Sia1, Hex5HexNAc5Fuc1Sia1) glycosylation (Fig. 6b). Interestingly, all 4 gut microbe-derived fatty acids reversed the LPS effect on the sialofucosylated glycosylation of CD166 (Hex5HexNAc4Fuc1Sia2).
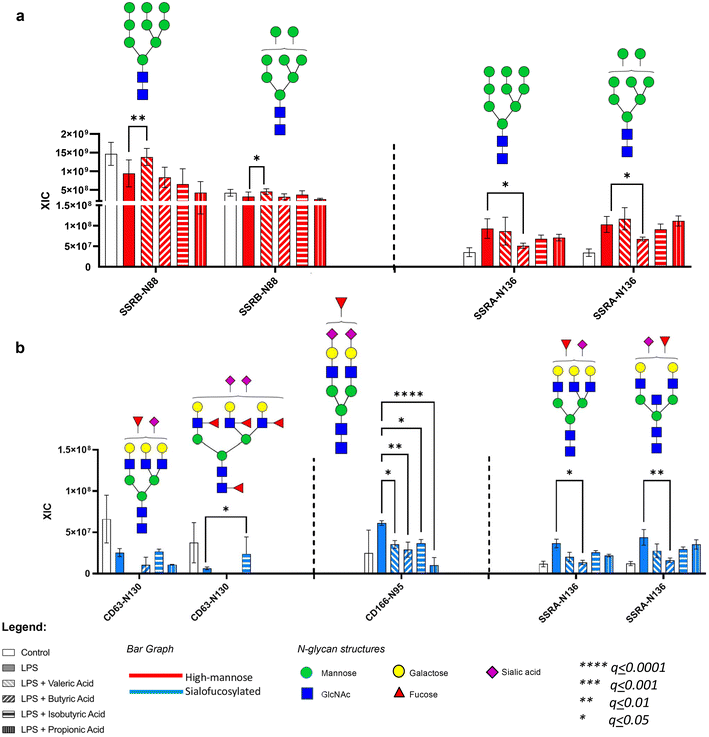 |
| Fig. 6
N-Glycoproteomic profiles of LPS-gut microbe-derived fatty acid treated microglia. The changes of the microglial glycoproteome were also observed after 24 h treatment of LPS and gut microbe-derived fatty acids: valeric, butyric, isobutyric and propionic acids (n = 3). The extracted ion counts of specific glycoproteins with changing (a) high mannose structures and (b) sialofucosylated structures are shown. | |
The glycoproteomic results highlight the observed effect of the fatty acids in the microglia specifically on the high mannose and sialofucosylated glycoforms of translocon-associated proteins (TRAP), which regulate the retention of ER resident proteins. It has been previously established that N-linked glycosylation is required for endoplasmic reticulum (ER) homeostasis,74–76 wherein the TRAP complex, composed of SSRA, SSRB, SSRC and SSRD subunits, was recently discovered through a model-based analysis of genome-wide CRISPR-Cas9 knockout (MAGECK) analysis, to be responsible in regulating the quality control of N-linked glycosylation during ER stress conditions in human cells (e.g. A549 and HEK-293 cells).69 Our study shows that the high mannose glycoforms of these TRAP proteins, especially SSRA and SSRB, are significantly decreased, while the sialofucosylated glycoforms are significantly increased in the presence of LPS. Interestingly, our results coincide with the previous finding that SSRA and SSRB were associated with BiP, an ER stress-induced chaperone which prevents SSRA and SSRB to function properly during excessive ER stress.69 Based on our results, we hypothesize that upon inducing ER stress, LPS alters SSRB and SSRA's N-glycosylation. We also showed through the significant site-specific effect of oleic (Hex9HexNAc2 – SSRB glycosite Asn88), palmitic (Hex9HexNAc2 - SSRB glycosite Asn88), valeric (Hex9HexNAc2 and Hex8HexNAc2 – SSRB glycosite Asn88) and butyric (Hex9HexNAc2, Hex8HexNAc2, Hex6HexNAc5Fuc1NeuAc1 and Hex5HexNAc5Fuc1NeuAc1 of SSRA glycosite Asn136) acids that select fatty acids can modulate high mannose and sialofucosylated glycoforms of SSRA and SSRB to counter the site-specific effects of LPS-induced ER stress in microglial cells. In addition, changes in sialofucosylation of the cluster of differentiation (CD) glycoproteins brought about by LPS were also observed specifically targeting CD63 and CD166, which are both considered important cell surface proteins that facilitate cell adhesion and migration during neuroinflammation.70,77 Both CD63 and CD166 have been identified as reliable indicators in plasma and serum samples related to cognitive decline and synaptic degeneration.70,77–79
3.5. Proteomics reveal reversal of the LPS effect by dietary and gut microbiota-derived fatty acids
Label-free proteomics analyses reveal the effect of LPS on the microglial glycocalyx, wherein it targets proteins involved in specific N-glycosylation pathways particularly, N-glycan precursor synthesis and attachment of N-glycan precursor. The heat map in ESI Fig. 3† shows the over- and underexpressed N-glycosylation-related proteins in different fatty acid supplementation following LPS induction. Interestingly, the fatty acids effectively counteract the effects of LPS on ALG5, a protein important in N-glycan precursor synthesis as well as on some components of oligosaccharyltransferase (OST), RPN1, RPN2 and STT3A. OST is a membrane protein complex critical in the first step in protein N-glycosylation, which initiates transfer of Glc3Man9GlcNAc2 from the lipid carrier dolichol-pyrophosphate to an asparagine residue within an Asn-X-Ser/Thr consensus motif (X being any amino acid except proline) in nascent polypeptide chains in the lumen of the endoplasmic reticulum.1 In intestinal inflammation, the underexpression of OST and its components is a marker of ER stress as a result of LPS stimulation.80,81 Previous studies have demonstrated that ER stress is a factor of inflammation mechanism by LPS, wherein ER-localized glycosyltransferases, which produce dolichol-linked precursor oligosaccharides such as ALG5 are also altered.82,83 While saturated fatty acids such as palmitic acid have been implicated in inducing prolonged ER stress causing inflammation, unsaturated fatty acids such as oleic acid and short-chain fatty acids may play important roles in the regulation of ER stress-induced inflammation in microglia.84,85
3.6.
In silico docking confirms that dietary fatty acids inhibit LPS
Based on our comprehensive analysis of both N-glycan and glycoprotein data, we observed a more pronounced alleviation of LPS-induced effects by dietary fatty acids compared to short-chain fatty acids derived from the gut microbiota. Looking into the mechanism of LPS, lipid A core of LPS molecules bind to the myeloid differentiation factor 2 (MD-2) protein hydrophobic pocket in order to enact its effect.86,87In silico molecular docking to the MD-2 receptor (known binding site of LPS) in immune cells showed that dietary fatty acids (long- and medium-chains) bind more than gut microbe-derived fatty acids (short-chain) as the former fatty acid lipid tail can interact with the protein's hydrophobic core with the fatty-acyl group exposed to the solvent surface (ESI Fig. 6†).
Notably, oleic acid demonstrated the most favorable binding energy among all tested fatty acids. Thus, the modeling results show that the dietary fatty acids may enact their alleviating effect by competing with LPS and blocking the binding site. Long chain fatty acids such as saturated palmitic acid and unsaturated arachidonic acid were previously shown to directly bind to MD-2 inducing responses to inflammation via an in vitro model and confirmed using RT-qPCR of tissues from an animal model.85,88 In contrast, the shorter gut microbe-derived fatty acids may enact their effect through a different pathway besides binding to the MD-2 receptor.
3.7.
In silico modeling shows that sialofucosylation is critical to the OST–TRAP interaction
We deduced the possibility of the interaction of the oligosaccharyl transferase (OST) complex and translocon-associated protein (TRAP) complex from our proteomic and glycoproteomic analyses (ESI Fig. 7a†). TRAP complex is found close to the OST complex, which interacts with both the translocon and the ribosome.89 To configure how the complexes interact, we performed an in silico analysis to show the binding between SSRA/SSRB, TRAP components and STT3B, catalytic subunit of the OST complex that catalyzes the first step in protein N-glycosylation (ESI Fig. 7b†). The modeling highlights the importance of sialofucosylation in the binding between SSRA and STT3B proteins, wherein the Hex5HexNAc5Fuc1NeuAc1 of glycopeptide FYIN136FTAL from SSRA is shown to be essential for the binding to occur.
4. Conclusion
This study utilized in vitro models of microglial activation induced by LPS and comprehensively characterized its impact using our N-glycomic and site-specific glycoproteomic platform coupled with proteomics and in silico analyses. While previous research has delved into the effects of LPS on protein expression, its influence on protein glycosylation remains largely unexplored. Our findings reveal that LPS-induced neuroinflammation leads to a decrease in high mannose N-glycosylation, while increasing the abundance of sialofucosylated N-glycans in microglial cells. In addition, we investigated the effects of both diet-derived fatty acids (oleic, palmitic and lauric) and those derived from the gut microbiota (valeric, isobutyric, butyric and propionic), demonstrating their capacity to alleviate the dysregulated glycosylation induced by LPS in microglial cells. This study sheds light on how fatty acids, whether through dietary intake or microbiota fermentation, can modulate the glycosylation patterns in a model of LPS-induced neuroinflammation in the brain. The discovery that oleic acid and select short-chain fatty acids relieve neuroinflammation by inhibiting inflammatory microglia via MD-2 receptor binding shows the dual role of fatty acids as medication for regulating inflammation and a source of nutrition. Further studies are warranted to explore the potentiality of decreasing the inflammatory state of different diseases through high intake of dietary fiber and olive oil.
Author contributions
Sheryl Joyce B. Grijaldo-Alvarez: writing – original draft, writing – review and editing, investigation, methodology, formal analysis, data curation, visualization, funding acquisition and conceptualization. Michael Russelle S. Alvarez: writing – review and editing, investigation, methodology, formal analysis, visualization and conceptualization. Ryan Lee Schindler: writing – review and editing, investigation, methodology and data curation. Armin Oloumi: writing – review and editing and methodology. Noah Hernandez: data curation. Tristan Seales: data curation. Jorge Gil C. Angeles: writing – review and editing. Ruel C. Nacario: writing – review and editing, supervision and conceptualization. Gladys C. Completo: writing – review and editing, supervision and conceptualization. Angela M. Zivkovic: writing – review and editing, supervision and conceptualization. J. Bruce German: writing – review and editing, supervision and conceptualization. Carlito B. Lebrilla: writing – review and editing, formal analysis, supervision, validation, conceptualization, funding acquisition and resources.
Data availability
Mass spectrometry data for glycomics, proteomics and glycoproteomics are deposited in the MassIVE Database (MassIVE ID: MSV000095120, https://doi.org/10.25345/C5PZ51Z26).
Conflicts of interest
All authors have no potential conflict of interest to declare.
Acknowledgements
Sheryl Joyce B. Grijaldo-Alvarez was granted a travel research award provided by the University of the Philippines through its COOPERATE program (Grant No.: OILCOOP-2021-02).
References
-
Essentials of Glycobiology, ed. A. Varki, R. D. Cummings, J. D. Esko, P. Stanley, G. W. Hart, M. Aebi, D. Mohnen, T. Kinoshita, N. H. Packer, J. H. Prestegard, R. L. Schnaar and P. H. Seeberger, Cold Spring Harbor Laboratory Press, Cold Spring Harbor, 4th edn, 2022 Search PubMed.
- Q. Li, Y. Xie, M. Wong and C. Lebrilla, Characterization of Cell Glycocalyx with Mass Spectrometry Methods, Cells, 2019, 8(8), 882, DOI:10.3390/cells8080882.
- M. Wong, G. Xu, M. Barboza, I. Maezawa, L.-W. Jin, A. Zivkovic and C. B. Lebrilla, Metabolic Flux Analysis of the Neural Cell Glycocalyx Reveals Differential Utilization of Monosaccharides, Glycobiology, 2020, 30(11), 859–871, DOI:10.1093/glycob/cwaa038.
- Q. Zhang, C. Ma, L.-S. Chin and L. Li, Integrative Glycoproteomics Reveals Protein N-Glycosylation Aberrations and Glycoproteomic Network Alterations in Alzheimer's Disease, Sci. Adv., 2020, 6(40), eabc5802, DOI:10.1126/sciadv.abc5802.
- C. B. Lebrilla and H. J. An, The Prospects of Glycanbiomarkers for the Diagnosis of Diseases, Mol. BioSyst., 2009, 5(1), 17–20, 10.1039/B811781K.
- H. Haukedal and K. K. Freude, Implications of Glycosylation in Alzheimer's Disease, Front. Neurosci., 2021, 14, 625348, DOI:10.3389/fnins.2020.625348.
- P. Pradeep, H. Kang and B. Lee, Glycosylation and Behavioral Symptoms in Neurological Disorders, Transl. Psychiatry, 2023, 13(1), 154, DOI:10.1038/s41398-023-02446-x.
- S. J. B. Grijaldo, M. R. S. Alvarez, F. M. Heralde, R. C. Nacario, C. B. Lebrilla, J. F. Rabajante and G. C. Completo, Integrating Computational Methods in Network Pharmacology and In Silico Screening to Uncover Multi-Targeting Phytochemicals against Aberrant Protein Glycosylation in Lung Cancer, ACS Omega, 2023, 8(23), 20303–20312, DOI:10.1021/acsomega.2c07542.
- J. Tena, I. Maezawa, M. Barboza, M. Wong, C. Zhu, M. R. Alvarez, L.-W. Jin, A. M. Zivkovic and C. B. Lebrilla, Regio-Specific N-Glycome and N-Glycoproteome Map of the Elderly Human Brain with and without Alzheimer’s Disease, Mol. Cell. Proteomics, 2022, 100427, DOI:10.1016/j.mcpro.2022.100427.
- K. Akasaka-Manya, H. Manya, Y. Sakurai, B. S. Wojczyk, Y. Kozutsumi, Y. Saito, N. Taniguchi, S. Murayama, S. L. Spitalnik and T. Endo, Protective Effect of N-Glycan Bisecting GlcNAc Residues on -Amyloid Production in Alzheimer's Disease, Glycobiology, 2010, 20(1), 99–106, DOI:10.1093/glycob/cwp152.
- S. Gaunitz, L. O. Tjernberg and S. Schedin–Weiss, The N–glycan Profile in Cortex and Hippocampus Is Altered in Alzheimer Disease, J. Neurochem., 2021, 159(2), 292–304, DOI:10.1111/jnc.15202.
- W.-T. Chen, A. Lu, K. Craessaerts, B. Pavie, C. Sala Frigerio, N. Corthout, X. Qian, J. Laláková, M. Kühnemund, I. Voytyuk, L. Wolfs, R. Mancuso, E. Salta, S. Balusu, A. Snellinx, S. Munck, A. Jurek, J. Fernandez Navarro, T. C. Saido, I. Huitinga, J. Lundeberg, M. Fiers and B. De Strooper, Spatial Transcriptomics and In Situ Sequencing to Study Alzheimer's Disease, Cell, 2020, 182(4), 976–991, DOI:10.1016/j.cell.2020.06.038.
- B. G. Cho, L. Veillon and Y. Mechref, N-Glycan, Profile of Cerebrospinal Fluids from Alzheimer's Disease Patients Using Liquid Chromatography with Mass Spectrometry, J. Proteome Res., 2019, 18(10), 3770–3779, DOI:10.1021/acs.jproteome.9b00504.
- J. Dorszewska, M. Prendecki, A. Oczkowska, M. Dezor and W. Kozubski, Molecular Basis of Familial and Sporadic Alzheimer's Disease, Curr. Alzheimer Res., 2016, 13(9), 952–963, DOI:10.2174/1567205013666160314150501.
- S. Estus, B. C. Shaw, N. Devanney, Y. Katsumata, E. E. Press and D. W. Fardo, Evaluation of CD33 as a Genetic Risk Factor for Alzheimer's Disease, Acta Neuropathol., 2019, 138(2), 187–199, DOI:10.1007/s00401-019-02000-4.
- R. Guerreiro and J. Bras, The Age Factor in Alzheimer's Disease, Genome Med., 2015, 7(1), 106, DOI:10.1186/s13073-015-0232-5.
- C. M. Karch and A. M. Goate, Alzheimer's Disease Risk Genes and Mechanisms of Disease Pathogenesis, Biol. Psychiatry, 2015, 77(1), 43–51, DOI:10.1016/j.biopsych.2014.05.006.
- P. Regan, P. L. McClean, T. Smyth and M. Doherty, Early Stage Glycosylation Biomarkers in Alzheimer's Disease, Medicines, 2019, 6(3), 92, DOI:10.3390/medicines6030092.
- J. Tena, X. Tang, Q. Zhou, D. Harvey, M. Barajas–Mendoza, L. Jin, I. Maezawa, A. M. Zivkovic and C. B. Lebrilla, Glycosylation Alterations in Serum of Alzheimer's Disease Patients Show Widespread Changes in N-glycosylation of Proteins Related to Immune Function, Inflammation, and Lipoprotein Metabolism, Alzheimer's Dementia, 2022, 14(1) DOI:10.1002/dad2.12309.
- J. Verheijen and K. Sleegers, Understanding Alzheimer Disease at the Interface between Genetics and Transcriptomics, Trends Genet., 2018, 34(6), 434–447, DOI:10.1016/j.tig.2018.02.007.
- A. L. Rebelo, M. T. Chevalier, L. Russo and A. Pandit, Role and Therapeutic Implications of Protein Glycosylation in Neuroinflammation, Trends Mol. Med., 2022, 28(4), 270–289, DOI:10.1016/j.molmed.2022.01.004.
- A. Deczkowska, H. Keren-Shaul, A. Weiner, M. Colonna, M. Schwartz and I. Amit, Disease-Associated Microglia: A Universal Immune Sensor of Neurodegeneration, Cell, 2018, 173(5), 1073–1081, DOI:10.1016/j.cell.2018.05.003.
- E. F. Garland, I. J. Hartnell and D. Boche, Microglia and Astrocyte Function and Communication: What Do We Know in Humans?, Front. Neurosci., 2022, 16, 824888, DOI:10.3389/fnins.2022.824888.
- A. K. Hodges, T. M. Piers, D. Collier, O. Cousins and J. M. Pocock, Pathways Linking Alzheimer's Disease Risk Genes Expressed Highly in Microglia, Neuroimmunol. Neuroinflammation, 2021, 8, 245, DOI:10.20517/2347-8659.2020.60.
- Z. Cai, M. D. Hussain and L.-J. Yan, Microglia, Neuroinflammation, and Beta-Amyloid Protein in Alzheimer's Disease, Int. J. Neurosci., 2014, 124(5), 307–321, DOI:10.3109/00207454.2013.833510.
- O. Sheppard, M. P. Coleman and C. S. Durrant, Lipopolysaccharide-Induced Neuroinflammation Induces Presynaptic Disruption through a Direct Action on Brain Tissue Involving Microglia-Derived Interleukin 1 Beta, J. Neuroinflammation, 2019, 16(1), 106, DOI:10.1186/s12974-019-1490-8.
- L. Han, D. Zhang, T. Tao, X. Sun, X. Liu, G. Zhu, Z. Xu, L. Zhu, Y. Zhang, W. Liu, K. Ke and A. Shen, The Role of N-Glycan Modification of TNFR1 in Inflammatory Microglia Activation, Glycoconjugate J., 2015, 32(9), 685–693, DOI:10.1007/s10719-015-9619-1.
- X. Liu, A. Li, Y. Ju, W. Liu, H. Shi, R. Hu, Z. Zhou and X. Sun, β4GalT1 Mediates PPARγ N-Glycosylation to Attenuate Microglia Inflammatory Activation, Inflammation, 2018, 41(4), 1424–1436, DOI:10.1007/s10753-018-0789-4.
- X. Wang, N. Shi, M. Hui, H. Jin, S. Gao, Q. Zhou, L. Zhang, M. Yan and H. Shen, The Impact of β-1,4-Galactosyltransferase V on Microglial Function, Front. Cell. Neurosci., 2021, 15, 723308, DOI:10.3389/fncel.2021.723308.
- M. Puigdellívol, D. H. Allendorf and G. C. Brown, Sialylation and Galectin-3 in Microglia-Mediated Neuroinflammation and Neurodegeneration, Front. Cell. Neurosci., 2020, 14, 162, DOI:10.3389/fncel.2020.00162.
- H. M. Melo, G. D. S. Seixas Da Silva, M. R. Sant'Ana, C. V. L. Teixeira, J. R. Clarke, V. S. Miya Coreixas, B. C. De Melo, J. T. S. Fortuna, L. Forny-Germano, J. H. Ledo, M. S. Oliveira, C. P. Figueiredo, R. Pardossi-Piquard, F. Checler, J. M. Delgado-García, A. Gruart, L. A. Velloso, M. L. F. Balthazar, D. E. Cintra, S. T. Ferreira and F. G. De Felice, Palmitate Is Increased in the Cerebrospinal Fluid of Humans with Obesity and Induces Memory Impairment in Mice via Pro-Inflammatory TNF-α, Cell Rep., 2020, 30(7), 2180–2194, DOI:10.1016/j.celrep.2020.01.072.
- M. B. Victor, N. Leary, X. Luna, H. S. Meharena, A. N. Scannail, P. L. Bozzelli, G. Samaan, M. H. Murdock, D. von Maydell, A. H. Effenberger, O. Cerit, H.-L. Wen, L. Liu, G. Welch, M. Bonner and L.-H. Tsai, Lipid Accumulation Induced by APOE4 Impairs Microglial Surveillance of Neuronal-Network Activity, Cell Stem Cell, 2022, 29(8), 1197–1212, DOI:10.1016/j.stem.2022.07.005.
- B. Chausse, P. A. Kakimoto, C. C. Caldeira-da-Silva, A. B. Chaves-Filho, M. Y. Yoshinaga, R. P. Da Silva, S. Miyamoto and A. J. Kowaltowski, Distinct Metabolic Patterns during Microglial Remodeling by Oleate and Palmitate, Biosci. Rep., 2019, 39(4), BSR20190072, DOI:10.1042/BSR20190072.
- T. J. Wenzel, E. J. Gates, A. L. Ranger and A. Klegeris, Short-Chain Fatty Acids (SCFAs) Alone or in Combination Regulate Select Immune Functions of Microglia-like Cells, Mol. Cell. Neurosci., 2020, 105, 103493, DOI:10.1016/j.mcn.2020.103493.
- E. Xu, C. Chen, J. Fu, L. Zhu, J. Shu, M. Jin, Y. Wang and X. Zong, Dietary Fatty Acids in Gut Health: Absorption, Metabolism and Function, Anim. Nutr., 2021, 7(4), 1337–1344, DOI:10.1016/j.aninu.2021.09.010.
- A. Hliwa, B. Ramos-Molina, D. Laski, A. Mika and T. Sledzinski, The Role of Fatty Acids in Non-Alcoholic Fatty Liver Disease Progression: An Update, Int. J. Mater. Sci., 2021, 22(13), 6900, DOI:10.3390/ijms22136900.
- M. Kazantzis and A. Stahl, Fatty Acid Transport Proteins, Implications in Physiology and Disease, Biochim. Biophys. Acta, Mol. Cell Biol. Lipids, 2012, 1821(5), 852–857, DOI:10.1016/j.bbalip.2011.09.010.
- F. Pifferi, B. Laurent and M. Plourde, Lipid Transport and Metabolism at the Blood-Brain Interface: Implications in Health and Disease, Front. Physiol., 2021, 12, 645646, DOI:10.3389/fphys.2021.645646.
- M. W. Wong, N. Braidy, A. Poljak, R. Pickford, M. Thambisetty and P. S. Sachdev, Dysregulation of Lipids in Alzheimer's Disease and Their Role as Potential Biomarkers, Alzheimer's Dementia, 2017, 13(7), 810–827, DOI:10.1016/j.jalz.2017.01.008.
- G. den Besten, K. van Eunen, A. K. Groen, K. Venema, D.-J. Reijngoud and B. M. Bakker, The Role of Short-Chain Fatty Acids in the Interplay between Diet, Gut Microbiota, and Host Energy Metabolism, J. Lipid Res., 2013, 54(9), 2325–2340, DOI:10.1194/jlr.R036012.
- J. Montenegro, A. M. Armet, B. P. Willing, E. C. Deehan, P. G. Fassini, J. F. Mota, J. Walter and C. M. Prado, Exploring the
Influence of Gut Microbiome on Energy Metabolism in Humans, Adv. Nutr., 2023, 14(4), 840–857, DOI:10.1016/j.advnut.2023.03.015.
- D. J. Morrison and T. Preston, Formation of Short Chain Fatty Acids by the Gut Microbiota and Their Impact on Human Metabolism, Gut Microbes, 2016, 7(3), 189–200, DOI:10.1080/19490976.2015.1134082.
- S. Yamamoto and T. Masuda, Lipid in Microglial Biology—from Material to Mediator, Inflammation Regener., 2023, 43(1), 38, DOI:10.1186/s41232-023-00289-z.
- B. A. Loving and K. D. Bruce, Lipid and Lipoprotein Metabolism in Microglia, Front. Physiol., 2020, 11, 393, DOI:10.3389/fphys.2020.00393.
- Z. Lu, S. Liu, M. F. Lopes-Virella and Z. Wang, LPS and Palmitic Acid Co-Upregulate Microglia Activation and Neuroinflammatory Response, Compr. Psychoneuroendocrinol., 2021, 6, 100048, DOI:10.1016/j.cpnec.2021.100048.
- T. V. Hung and T. Suzuki, Short-Chain Fatty Acids Suppress Inflammatory Reactions in Caco-2 Cells and Mouse Colons, J. Agric. Food Chem., 2018, 66(1), 108–117, DOI:10.1021/acs.jafc.7b04233.
- C. E. Storniolo, M. Cabral, M. A. Busquets, R. Martín-Venegas and J. J. Moreno, Dual Behavior of Long-Chain Fatty Acids and Their Cyclooxygenase/Lipoxygenase Metabolites on Human Intestinal Caco-2 Cell Growth, Front. Pharmacol., 2020, 11, 529976, DOI:10.3389/fphar.2020.529976.
- M. R. S. Alvarez, Q. Zhou, S. J. B. Grijaldo, C. B. Lebrilla, R. C. Nacario, F. M. Heralde, J. F. Rabajante and G. C. Completo, An Integrated Mass Spectrometry-Based Glycomics-Driven Glycoproteomics Analytical Platform to Functionally Characterize Glycosylation Inhibitors, Molecules, 2022, 27(12), 3834, DOI:10.3390/molecules27123834.
- Q. Li, Y. Xie, M. Wong, M. Barboza and C. B. Lebrilla, Comprehensive Structural Glycomic Characterization of the Glycocalyxes of Cells and Tissues, Nat. Protoc., 2020, 15(8), 2668–2704, DOI:10.1038/s41596-020-0350-4.
-
M. R. Alvarez, J. Tena, Q. Zhou, M. Cabanatan, S. Tomacas, L. M. Serrano, S. J. B. Grijaldo, J. Rabajante, M. T. Barzaga, N. Tan-Liu, F. I. Heralde, C. B. Lebrilla, G. C. Completo and R. Nacario, Glycan and Glycopeptide Serum Biomarkers in Filipino Lung Cancer Patients Identified Using LC-MS/MS, 2022 Search PubMed.
-
S. Dallakyan and A. J. Olson, Small-Molecule Library Screening by Docking with PyRx, in Chemical Biology, ed. J. E. Hempel, C. H. Williams and C. C. Hong, Methods in Molecular Biology, Springer New York, New York, NY, 2015, vol. 1263, pp. 243–250. DOI:10.1007/978-1-4939-2269-7_19.
- O. Trott and A. J. Olson, AutoDock Vina: Improving the Speed and Accuracy of Docking with a New Scoring Function, Efficient Optimization, and Multithreading, J. Comput. Chem., 2010, 31(2), 455–461, DOI:10.1002/jcc.21334.
- J. Huang, S. Rauscher, G. Nawrocki, T. Ran, M. Feig, B. L. De Groot, H. Grubmüller and A. D. MacKerell, CHARMM36m: An Improved Force Field for Folded and Intrinsically Disordered Proteins, Nat. Methods, 2017, 14(1), 71–73, DOI:10.1038/nmeth.4067.
- W. Humphrey, A. Dalke and K. Schulten, VMD: Visual Molecular Dynamics, J. Mol. Graphics, 1996, 14(1), 33–38, DOI:10.1016/0263-7855(96)00018-5.
- M. Barboza, K. Solakyildirim, T. A. Knotts, J. Luke, M. G. Gareau, H. E. Raybould and C. B. Lebrilla, Region-Specific Cell Membrane N-Glycome of Functional Mouse Brain Areas Revealed by nanoLC-MS Analysis, Mol. Cell. Proteomics, 2021, 20, 100130, DOI:10.1016/j.mcpro.2021.100130.
- P. Fang, J. Xie, S. Sang, L. Zhang, M. Liu, L. Yang, Y. Xu, G. Yan, J. Yao, X. Gao, W. Qian, Z. Wang, Y. Zhang, P. Yang and H. Shen, Multilayered N-Glycoproteome Profiling Reveals Highly Heterogeneous and Dysregulated Protein N-Glycosylation Related to Alzheimer's Disease, Anal. Chem., 2020, 92(1), 867–874, DOI:10.1021/acs.analchem.9b03555.
- Y. Kizuka and N. Taniguchi, Neural Functions of Bisecting GlcNAc, Glycoconjugate J., 2018, 35(4), 345–351, DOI:10.1007/s10719-018-9829-4.
- V. Sytnyk, I. Leshchyns'ka and M. Schachner, Neural Glycomics: The Sweet Side of Nervous System Functions, Cell. Mol. Life Sci., 2021, 78(1), 93–116, DOI:10.1007/s00018-020-03578-9.
- X. Zhan, B. Stamova and F. R. Sharp, Lipopolysaccharide Associates with Amyloid Plaques, Neurons and Oligodendrocytes in Alzheimer's Disease Brain: A Review, Front. Aging Neurosci., 2018, 10, 42, DOI:10.3389/fnagi.2018.00042.
- J. Penney, W. T. Ralvenius and L.-H. Tsai, Modeling Alzheimer's Disease with iPSC-Derived Brain Cells, Mol. Psychiatry, 2020, 25(1), 148–167, DOI:10.1038/s41380-019-0468-3.
- A. Slanzi, G. Iannoto, B. Rossi, E. Zenaro and G. Constantin, In Vitro Models of Neurodegenerative Diseases, Front. Cell Dev. Biol., 2020, 8, 328, DOI:10.3389/fcell.2020.00328.
- A. Sobue, O. Komine, Y. Hara, F. Endo, H. Mizoguchi, S. Watanabe, S. Murayama, T. Saito, T. C. Saido, N. Sahara, M. Higuchi, T. Ogi and K. Yamanaka, Microglial Gene Signature Reveals Loss of Homeostatic Microglia Associated with Neurodegeneration of Alzheimer's Disease, Acta Neuropathol. Commun., 2021, 9(1), 1, DOI:10.1186/s40478-020-01099-x.
- C. R. A. Batista, G. F. Gomes, E. Candelario-Jalil, B. L. Fiebich and A. C. P. de Oliveira, Lipopolysaccharide-Induced Neuroinflammation as a Bridge to Understand Neurodegeneration, Int. J. Mol. Sci., 2019, 20(9), 2293, DOI:10.3390/ijms20092293.
- A. Skrzypczak-Wiercioch and K. Sałat, Lipopolysaccharide-Induced Model of Neuroinflammation: Mechanisms of Action, Research Application and Future Directions for Its Use, Molecules, 2022, 27(17), 5481, DOI:10.3390/molecules27175481.
- J. Zhao, W. Bi, S. Xiao, X. Lan, X. Cheng, J. Zhang, D. Lu, W. Wei, Y. Wang, H. Li, Y. Fu and L. Zhu, Neuroinflammation Induced by Lipopolysaccharide Causes Cognitive Impairment in Mice, Sci. Rep., 2019, 9(1), 5790, DOI:10.1038/s41598-019-42286-8.
- L. Jin, J. Di Lucente, U. Ruiz Mendiola, X. Tang, A. M. Zivkovic, C. B. Lebrilla and I. Maezawa, The Role of FUT8 –catalyzed Core Fucosylation in Alzheimer's Amyloid–β Oligomer–induced Activation of Human Microglia, Glia, 2023, 71(5), 1346–1359, DOI:10.1002/glia.24345.
- Y. Jitsuhara, T. Toyoda, T. Itai and H. Yamaguchi, Chaperone-Like Functions of High-Mannose Type and Complex-Type N-Glycans and Their Molecular Basis, J. Biochem., 2002, 132(5), 803–811, DOI:10.1093/oxfordjournals.jbchem.a003290.
- A. Nakashima, S.-B. Cheng, T. Kusabiraki, K. Motomura, A. Aoki, A. Ushijima, Y. Ono, S. Tsuda, T. Shima, O. Yoshino, H. Sago, K. Matsumoto, S. Sharma and S. Saito, Endoplasmic Reticulum Stress Disrupts Lysosomal Homeostasis and Induces Blockade of Autophagic Flux in Human Trophoblasts, Sci. Rep., 2019, 9(1), 11466, DOI:10.1038/s41598-019-47607-5.
- C. Phoomak, W. Cui, T. J. Hayman, S.-H. Yu, P. Zhao, L. Wells, R. Steet and J. N. Contessa, The Translocon-Associated Protein (TRAP) Complex Regulates Quality Control of
N-Linked Glycosylation during ER Stress, Sci. Adv., 2021, 7(3), eabc6364, DOI:10.1126/sciadv.abc6364.
- R. Lyck, M.-A. Lécuyer, M. Abadier, C. B. Wyss, C. Matti, M. Rosito, G. Enzmann, T. Zeis, L. Michel, A. B. García Martín, F. Sallusto, F. Gosselet, U. Deutsch, J. A. Weiner, N. Schaeren-Wiemers, A. Prat and B. Engelhardt, ALCAM (CD166) Is Involved in Extravasation of Monocytes Rather than T Cells across the Blood–Brain Barrier, J. Cereb. Blood Flow Metab., 2017, 37(8), 2894–2909, DOI:10.1177/0271678X16678639.
- L. Wen, X. Yang, Z. Wu, S. Fu, Y. Zhan, Z. Chen, D. Bi and Y. Shen, The Complement Inhibitor CD59 Is Required for GABAergic Synaptic Transmission in the Dentate Gyrus, Cell Rep., 2023, 42(4), 112349, DOI:10.1016/j.celrep.2023.112349.
- A.-M. Howe, S. Burke, M. E. O'Reilly, F. C. McGillicuddy and D. A. Costello, Palmitic Acid and Oleic Acid Differently Modulate TLR2-Mediated Inflammatory Responses in Microglia and Macrophages, Mol. Neurobiol., 2022, 59(4), 2348–2362, DOI:10.1007/s12035-022-02756-z.
- M. E. Caetano-Silva, L. Rund, N. T. Hutchinson, J. A. Woods, A. J. Steelman and R. W. Johnson, Inhibition of Inflammatory Microglia by Dietary Fiber and Short-Chain Fatty Acids, Sci. Rep., 2023, 13(1), 2819, DOI:10.1038/s41598-022-27086-x.
- A. Helenius and M. Aebi, Roles of N-Linked Glycans in the Endoplasmic Reticulum, Annu. Rev. Biochem., 2004, 73(1), 1019–1049, DOI:10.1146/annurev.biochem.73.011303.073752.
- M. E. Losfeld, B. G. Ng, M. Kircher, K. J. Buckingham, E. H. Turner, A. Eroshkin, J. D. Smith, J. Shendure, D. A. Nickerson and M. J. Bamshad, University of Washington Center for Mendelian Genomics; Freeze, H. H. A New Congenital Disorder of Glycosylation Caused by a Mutation in SSR4, the Signal Sequence Receptor 4 Protein of the TRAP Complex, Hum. Mol. Genet., 2014, 23(6), 1602–1605, DOI:10.1093/hmg/ddt550.
- B. G. Ng, C. M. Lourenço, M. Losfeld, K. J. Buckingham, M. Kircher, D. A. Nickerson, J. Shendure and M. J. Bamshad, University of Washington Center for Mendelian Genomics; Freeze, H. H. Mutations in the Translocon–associated Protein Complex Subunit SSR3 Cause a Novel Congenital Disorder of Glycosylation, J. Inherited Metab. Dis., 2019, 42(5), 993–997, DOI:10.1002/jimd.12091.
- D. Kim, H. Nishida, S. Y. An, A. K. Shetty, T. J. Bartosh and D. J. Prockop, Chromatographically Isolated CD63+ CD81+ Extracellular Vesicles from Mesenchymal Stromal Cells Rescue Cognitive Impairments after TBI, Proc. Natl. Acad. Sci. U. S. A., 2016, 113(1), 170–175, DOI:10.1073/pnas.1522297113.
- J. Chen, A.-X. Dai, H.-L. Tang, C.-H. Lu, H.-X. Liu, T. Hou, Z.-J. Lu, N. Kong, X.-Y. Peng, K.-X. Lin, Z.-D. Zheng, S.-L. Xu, X.-F. Ying, X.-Y. Ji, H. Pan, J. Wu, X. Zeng and N.-L. Wei, Increase of ALCAM and VCAM-1 in the Plasma Predicts the Alzheimer's Disease, Front. Immunol., 2023, 13, 1097409, DOI:10.3389/fimmu.2022.1097409.
- M. Vaz, T. Soares Martins and A. G. Henriques, Extracellular Vesicles in the Study of Alzheimer's and Parkinson's Diseases: Methodologies Applied from Cells to Biofluids, J. Neurochem., 2022, 163(4), 266–309, DOI:10.1111/jnc.15697.
- Y. Wang, Q. Zhou, X. Zhang, Q. Qian, J. Xu, P. Ni and Y. Qian, Mild Endoplasmic Reticulum Stress Ameliorates Lipopolysaccharide-Induced Neuroinflammation and Cognitive Impairment via Regulation of Microglial Polarization, J. Neuroinflammation, 2017, 14(1), 233, DOI:10.1186/s12974-017-1002-7.
- D. B. Graham, A. Lefkovith, P. Deelen, N. De Klein, M. Varma, A. Boroughs, A. N. Desch, A. C. Y. Ng, G. Guzman, M. Schenone, C. P. Petersen, A. K. Bhan, M. A. Rivas, M. J. Daly, S. A. Carr, C. Wijmenga and R. J. Xavier, TMEM258 Is a Component of the Oligosaccharyltransferase Complex Controlling ER Stress and Intestinal Inflammation, Cell Rep., 2016, 17(11), 2955–2965, DOI:10.1016/j.celrep.2016.11.042.
- S. Tian, K. Muneeruddin, M. Y. Choi, L. Tao, R. H. Bhuiyan, Y. Ohmi, K. Furukawa, K. Furukawa, S. Boland, S. A. Shaffer, R. M. Adam and M. Dong, Genome-Wide CRISPR Screens for Shiga Toxins and Ricin Reveal Golgi Proteins Critical for Glycosylation, PLos Biol., 2018, 16(11), e2006951, DOI:10.1371/journal.pbio.2006951.
- H. Liu, J.-J. Yin, M.-M. Cao, G.-D. Liu, Y. Su and Y.-B. Li, Endoplasmic Reticulum Stress Induced by Lipopolysaccharide Is Involved in the Association between Inflammation and Autophagy in INS-1 Cells, Mol. Med. Rep., 2017, 16(5), 5787–5792, DOI:10.3892/mmr.2017.7350.
- Y. Wei, D. Wang, F. Topczewski and M. J. Pagliassotti, Saturated Fatty Acids Induce Endoplasmic Reticulum Stress and Apoptosis Independently of Ceramide in Liver Cells, Am. J. Physiol.: Endocrinol. Metab., 2006, 291(2), E275–E281, DOI:10.1152/ajpendo.00644.2005.
- Y. Wang, Y. Qian, Q. Fang, P. Zhong, W. Li, L. Wang, W. Fu, Y. Zhang, Z. Xu, X. Li and G. Liang, Saturated Palmitic Acid Induces Myocardial Inflammatory Injuries through Direct Binding to TLR4 Accessory Protein MD2, Nat. Commun., 2017, 8(1), 13997, DOI:10.1038/ncomms13997.
- B. S. Park, D. H. Song, H. M. Kim, B.-S. Choi, H. Lee and J.-O. Lee, The Structural Basis of Lipopolysaccharide Recognition by the TLR4−MD-2 Complex, Nature, 2009, 458(7242), 1191–1195, DOI:10.1038/nature07830.
- N. Maeshima and R. Fernandez, Recognition of Lipid A Variants by the TLR4-MD-2 Receptor Complex, Front. Cell. Infect. Microbiol., 2013, 3 DOI:10.3389/fcimb.2013.00003.
- Y. Zhang, H. Chen, W. Zhang, Y. Cai, P. Shan, D. Wu, B. Zhang, H. Liu, Z. A. Khan and G. Liang, Arachidonic Acid Inhibits Inflammatory Responses by Binding to Myeloid Differentiation Factor-2 (MD2) and Preventing MD2/Toll-like Receptor 4 Signaling Activation, Biochim. Biophys. Acta, Mol. Basis Dis., 2020, 1866(5), 165683, DOI:10.1016/j.bbadis.2020.165683.
- A. Russo, Understanding the Mammalian TRAP Complex Function(s), Open Biol., 2020, 10(5), 190244, DOI:10.1098/rsob.190244.
|
This journal is © The Royal Society of Chemistry 2024 |