DOI:
10.1039/D1RA05311F
(Paper)
RSC Adv., 2021,
11, 31062-31072
Discovery of 8-prenylnaringenin from hop (Humulus lupulus L.) as a potent monoacylglycerol lipase inhibitor for treatments of neuroinflammation and Alzheimer's disease†
Received
10th July 2021
, Accepted 15th September 2021
First published on 20th September 2021
Abstract
Monoacylglycerol lipase (MAGL), a serine hydrolase, converts endocannabinoid 2-arachidonoylglycerol (2-AG) to arachidonic acid (AA) and glycerol in the brain and plays a bidirectional role in controlling nueroinflammation. MAGL, involved in Alzheimer's and Parkinson's diseases, is a promising target for treatment of neurodegenerative disorders. However, the irreversible inhibitors of MAGL lead to the desensitization of CB1 receptors further impairing the benefits associated with the indirect CB1 stimulation. Therefore, development of potent reversible inhibitors from natural products (NPs) and traditional chinese medicines (TCMs) are safer and free from adverse side effects and feasible to avoid drawbacks which irreversible inhibitors cause. Here, we employed pharmacophore-based screening of drug candidates coupled with molecular docking, biochemical assay and Ligplot analyses to identify and characterize inhibitors targeting human MAGL (hMAGL). The built pharmacophore model, Phar-MAGL successfully identified inhibitors NP-2 (IC50 = 9.5 ± 1.2 μM), NP-5 (IC50 = 14.5 ± 1.3 μM), and NP-3 (IC50 = 15.2 ± 1.4 μM), which apparently attenuated the activities of hMAGL in vitro. The evident activities of the identified inhibitors against hMAGL showed that the pharmacophore model, Phar-MAGL is reliable and efficient in screening inhibitors against hMAGL. Our study successfully identified a natrual product inhibitor, NP-2 (8-PN), from the plant Humulus lupulus L. (hops) and its positive effects in neurogenesis and neurodifferentiation along with the evident inhibitory potency against hMAGL revealed the potential for further optimizing and developing into drugs to treat neuroinflammation, Alzheimer's and Parkinson's diseases.
Introduction
The evolutionarily protective system, inflammation, minimizes tissue damage and promotes healing through reducing infections and clearing antigens and cellular debris.1 However persistent inflammation in the central nervous system (CNS) causes pathologically chronic inflammatory disease.2 Especially, most neurological disorders (such as Alzheimer's, Huntington's, Parkinson's diseases, amyotrophic lateral sclerosis and neurodegeneration with brain iron accumulation (NBIA)) are correlated with the imbalanced neuronal homeostasis of the CNS.3,4 The produced proinflammatory chemokines and cytokines, the deterioration of the blood–brain barrier, and the activated immune cells are hallmarks of neuroinflammation.5,6 These increase neurodegeneration, risk of neuronal dysfunction, and may infiltrate protein and peripheral cells into CNS.7 Alzheimer's disease (AD), a neurodegenerative disorder, is characterized by neuroinflammation, deposition of amyloid plaques, loss of memory and cognitive function, associated with widespread neuronal death.8,9 Around 50 million people have dementia and nearly 10 million new cases occur every year (WHO). AD is the most common form of dementia contributing 60–70% of cases. However, very limited therapeutic agents are currently approved by Food and Drug Administration for treatment of AD.10,11 Thus, there is an urgent need to identify and develop new and novel therapeutics for the preventions and treatment of AD.
The monoacylglycerol lipase (MAGL) regulates the generation of arachidonic acid (AA) in the brain and terminates signaling of endocannabinoid 2-arachidonoylglycerol (2-AG).12,13 MAGL contributes in converting 2-AG to AA and glycerol, and 2-AG is responsible for 50% production of AA in the brain.14,15 The generation of AA is mainly catalyzed by phospholipase A2 (PLA2) in the periphery.16 AA is essential in the inflammatory response, acting as the precursor of multiple pathways and as signaling molecule of proinflammatory eicosanoids.17 Thus, MAGL play a bidirectional role in controlling brain inflammations through regulating arachidonate and endocannabinoid concentrations.18,19 Notably, inhibiting MAGL could attenuate the proinflammatory cytokine and eicosanoids in the brain20–25 as well as elevating 2-AG signaling at CB1/2 receptors.26 Additionally, pharmacological blockade and/or genetic deletion of MAGL reduces neuroinflammatory biomarkers (cytokines or gliosis) in mouse models of AD.27,28 Nevertheless, the major therapeutic treatment to relieve brain inflammation for now is the use of steroids.29 Corticosteroid medications are steroids used to reduce inflammation. The oral or intravenous intakes of steroids commonly resulted in life-threatening adverse effects, such as infection and adrenal crisis.30,31 Therefore, MAGL is now a significant and attractive target to develop new drug and agent for the treatment of diseases associated with neuroinflammation.
Nowadays, the determined crystal structure of MAGL provides evidence of enzyme–substrate interaction, giving insights to develop MAGL inhibitors.32,33 Generally, two types of MAGL inhibitors were reported: (i) irreversible inhibitors covalently binds to the active site of MAGL (ii) inhibitors reversibly binds into the catalytic pocket of MAGL.34 Among them, the chemically synthetic irreversible inhibitors are the majority for MAGL, such as NAM,35 Disulfiram,36 JZL184,37 KML29,38 SAR629,39 and CK37.40 Nevertheless, it has been suggested that the irreversible inhibition against MAGL leading to the desensitization of CB1 receptors further impairing the benefits associated to the indirect CB1 stimulation.12,41 Therefore, design and discovery of effectively reversible inhibitors are feasible alternatives to avoid the drawback which irreversible inhibitors of MAGL caused. Unlike the chemically synthetic inhibitors of MAGL, natural products (NPs)42,43 and traditional chinese medicines (TCMs)44 are safer and free from adverse side effects for new drug development. Especially, the TCMs is unique in Asia; remarkably different from the chemical drugs of Western countries. Many functions and applications of TCMs are still unraveling and discovering. Therefore, TCMs and NPs are of great potential in developing novel and potent MAGL inhibitors. This advantage together with the determined crystal structure of MAGL make it feasible to screen potent inhibitors from NPs and TCMs through computer-aided drug design (CADD).
In this context, we aim to identify, characterize, and develop new hMAGL inhibitors from NPs and TCMs by CADD coupled with biochemical examination and Ligplot analyses. We employed structure-based pharmacophore modeling and molecular docking to screen potential inhibitors against hMAGL. The built pharmacophore model, Phar-MAGL consisting of functionally essential features was used to screen inhibitor through ligand-pharmacophore mapping. The commonly identified 7 inhibitors by both molecular docking and pharmacophore screening were confirmed with inhibitory ability against hMAGL; especially natural product, NP-2 exhibited the most profound inhibitory potency (IC50 = 9.5 ± 1.2 μM). The observed results demonstrate that pharmacophore model (Phar-MAGL) is efficient and reliable in identifying potent hMAGL inhibitors. Furthermore, Ligplot analyses characterized and depicted the detailed molecular interactions of the identified inhibitors towards hMAGL, revealing the mode of action of hMAGL and NP-2. Our study successfully identified a reversible inhibitor (NP-2 and/or 8-PN) from the plant Humulus lupulus L. (hops). The positive effects in neurogenesis and neurodifferentiation along with the evident inhibitory potency against hMAGL indicate that NP-2 is of great potential for further optimizing and developing into drugs to treat neuroinflammation as well as AD.
Materials and methods
Natural products (NPs), traditional Chinese medicine (TCMs), and ligands preparations for computer-aided drug screening
A total of 68
285 compounds were retrieved from natural product databases, including 68
000 compounds from the InterBioScreen (IBS, http://www.ibscreen.com) diversity set, and 285 compounds from TCM (Taiwan Chinese medicine, http://www.nricm.edu.tw). The sketch molecules and prepare ligands modules implemented in Discovery Studio 3.5 (Accelrys Software, Inc., San Diego, CA, USA) were employed to generate 3D molecular structures of all compounds. The procedure of compound preparation for molecular docking and pharmacophore modeling was conducted by following steps: (i) two-dimensional (2D) structures were converted into three-dimensional (3D) structures, (ii) standard formal charges were calculated, and (iii) all hydrogen (not only polar hydrogens) atoms were added.
Receptor-ligand pharmacophore generation and pharmacophore-based inhibitor screening (ligand pharmacophore mapping)
Receptor-ligand pharmacophore modeling is used to identify the functionally important features, which are critical for ligands to interact with target proteins. Thus, we utilized the determined hMAGL-inhibitor complex structure (hMAGL-E3A (PDB ID: 6BQ0)) to build the pharmacophore model, containing the interactive features, for screening the hMAGL inhibitors. The receptor-ligand pharmacophore generation module of Discovery Studio 3.5 (Accelrys Software, Inc., San Diego, CA, USA) was employed to build the pharmacophore models. The hMAGL structure is served as the“Input Receptor”, and the structure of inhibitor, E3A was used as the“Input Ligand”, separately. The“Minimum Features” and“Maximum Features”were set to 4 and 10, respectively, and the“Maximum Pharmacophores”set was to 10. The“fast method” was applied for conformation generation with“rigid fitting method”. The rest parameters were set as default during the receptor-ligand pharmacophore generation process. Consequently, the built pharmacophores model was subjected to ligand-pharmacophore mapping (pharmacophore-based drug screening). All the molecules from NPs and TCMs (68
285 molecules) were fit to the built pharmacophore model, with the fitting method set to“flexible”and all other parameter were remain as default setting.
Preparation of protein structure for LibDock docking
Before performing the LibDock molecular docking, the protein structure of hMAGL (PDB ID: 6BQ0) was properly prepared. Firstly, the inhibitor E3A in the active site of hMAGL was selected when defining binding site from selection. Subsequently, the site sphere was built according to the selection and E3A was removed from the active site of hMAGL. The processed structure of hMAGL was further prepared by using “prepare protein” function implemented in Discovery Studio 3.5 (Accelrys Software, Inc., San Diego, CA, USA) and the following steps were performed. (i) Standardize atom names, insert missing atoms in residues and remove alternate conformations. (ii) Insert missing loop regions based on either SEQRES data or user specified loop definitions. (iii) Optimize short and medium size loop regions with the LOOPER algorithm. (iv) Minimize the remaining loop regions. (v) Calculate the pK and protonate the structure. All the remained parameters were set as default to prepared protein structure of hMAGL for LibDock molecular docking.
Structure-based molecular docking analysis was performed by using LibDock implemented in Discovery Studio 3.5 (Accelrys Software, Inc., San Diego, CA, USA) to identify the bioactive molecules from the NPs and TCMs databases. During the docking analysis, the protein structure of hMAGL (PBD ID: 6BQ0) were used to defined and edit the docking site. The inhibitor E3A in the active site of hMAGL was selected when defining binding site from selection and the protein structure of hMAGL was prepared as described in preparation of protein structure for LibDock docking. Subsequently, the site sphere was built according to the selection and employed for LibDock screening. The structure of hMAGL was used as “Input Receptor” and the prepared natural product database was used as “Input ligands”. About 68
285 natural products from NPs and TCMs databases were all docked in to the active site of hMAGL with “High Quality” for the “Docking Preferences” and “BEST” for the “Conformation Method”. The “Number of Hotspots” was set to “100” and the “Docking Tolerance” was set to “0.25”. As well, the “Smart Minimizer” as employed as the “Minimization Algorithm”. The remains were set as default. After the calculations, the most possible orientations and positions with the highest LibDockScore were selected and examined. The visualizations of molecular models are achieved by using PyMOL2.3.4 software (http://www.pymol.org).
Molecular dynamics simulations
The fitted poses of the identified inhibitors (NP-1 to NP-7) obtained from ligand-pharmacophore (Phar-MAGL) mapping were further subjected to molecular dynamics (MD) simulations. The hMAGL-inhibitor complexes obtained from ligand-pharmacophore mapping were firstly subjected to solvation (Discovery Studio 3.5) with orthorhombic cell shape under CHARMm forcefield. Consequently, the hMAGL-inhibitor complex was solvated with 6951 waters, 20 sodium atoms, and 18 chloride atoms. Furthermore, the solvated complex structure was subjected to Standard Dynamics Cascade (Discovery Studio 3.5) with default parameter setting. The final outputted conformation from MD simulation was selected and analyzed by ligplot.
Human MAGL assay
The inhibitory ability of identified natural compounds against hMAGL were determined according to the instructions provided within Cayman's assay kit (Cayman Chemical, Michigan, USA).45 The brief procedure of the assay was as follows: (i) 100% initial activity well was prepared by adding 150 μl of 1× assay buffer, 10 μl of hMAGL enzyme, and 10 μl of solvent to three wells. (ii) Background wells were prepared by adding 160 μl of 1× assay buffer and 10 μl solvent to three wells. (iii) Inhibitor wells were prepared by adding 150 μl of 1× assay buffer, 10 μl of MAGL enzyme, and 10 μl of inhibitor to the inhibitor wells. (iv) Mix the contents of the wells through pipetting and incubate at room temperature for 15 min. (v) Initiate the reactions by add 10 μl of MAGL substrate to all wells begin used, incubating for 10 min at room temperature. (vi) Read the absorbance at 405 nm. The inhibition% was determined using the following equations:
The IC50 of the inhibitors were determined through nonlinear regression fitting of the inhibition % versus the logarithm of the inhibitor concentrations by GraphPad Prim 6.
Results
Pharmacophore model generation
In this study, we strategically employed computer-aided drug design (CADD) coupling with in vitro biochemical examination to identified the potential hMAGL inhibitor from natural products (Fig. 1). CADD is with low cost and less time consuming, considerably accelerating the pace of investigations of inhibitors with specific biological activity. The in silico screening consists of two structure-based methods, i.e., pharmacophore modeling (receptor-ligand pharmacophore generation and ligand pharmacophore mapping) and molecular docking. The virtual high-throughput screening (molecular docking) is able to evaluate the potential biological activities of compounds based on their structural properties. To more precisely screen potent inhibitors against hMAGL, the functionally essential features responsible for the interactions between hMAGL and known inhibitors should be considered. The arrangement of essential features of a ligand which determines efficacious binding to a receptor can be described by using pharmacophores.46,47 The receptor-ligand pharmacophore generations create the molecular features through converting protein properties to reciprocal ligand space. This kind of protein structure-based pharmacophores (SBPs) can be used to explore protein-ligand interactions and to identify new ligands for specific proteins.48 Several studies have reported the successful use of SBPs for binding site similarity calculations, virtual screening, and lead optimizations.49–56 Especially, the pharmacophore-based lead identification technique is useful to screen novel compounds that meet the pharmacophore requirements and are of great potential to be biologically active. As well, pharmacophore-based lead identification is an effective filter to search for bioactive molecules and can reduce the number of compounds and costs in biophysical screenings. Receptor-ligand pharmacophore modeling is an advanced technique to probe the functional features essential for the interactions of the ligand (inhibitors) with the receptor (hMAGL).46,47,57–59 Meanwhile, the compound library consisting of 68
285 natural products were retrieved from IBS (68
000) and TCM (285) databases. To screen potent inhibitors by virtual high-throughput screening (molecular docking) and pharmacophore modeling, the structural information of hMAGL is critical. There are 9 complex structures of hMAGL bound with inhibitors deposited in protein data bank (PBD ID: 6BQ0, 3PE6, 4UUQ, 5ZUN, 7L4U, 7L4W, 7L50, 6AXI, and 7L4T). We superimposed these complex structures and found that the binding position and pose of inhibitor E3A in the active site of hMAGL was apparently different from the others (Fig. 2). Thus, we chose the complex structure of hMAGL-E3A (PDB ID: 6BQ0) to generate pharmacophore model by receptor-ligand pharmacophore generation (the combination of ligand-based and receptor-based pharmacophore generations). Consequently, the built pharmacophore model will be employed it to screen new and novel inhibitors which inhibit hMAGL with binding mode distinct from those of most majority of known inhibitors. The X-ray structure of hMAGL in complex with a Trifluoromethyl Glycol Carbamate (E3A) (PDB ID: 6BQ0) was used as a reference in this study. The complex structure shows that E3A forms significant hydrophilic and hydrophobic interactions to inhibit hMAGL. E3A binds into the active site of hMAGL mainly interacting with residues A51 and S122 by hydrogen bondings and contacting with residues G50, M123, G177, I179, L241 and C242 by hydrophobic interactions (Fig. 3A). Subsequently, we employed the complex structure of hMAGL-E3A to construct the pharmacophore model. The receptor-ligand pharmacophore generation uses the non-bond interactions between the input receptor and the input ligand to enumerate pharmacophores. The pharmacophores are ranked with a GFA (Genetic Function Approximation) model, and the top pharmacophores containing minimum features to maximum features are reported. During the construction, the structure of hMAGL was used as the receptor, and the entity of E3A was served as ligand to build the most possible and reliable pharmacophore model, containing the functionally essential features for screening hMAGL inhibitors. Consequently, pharmacophore model generated based on the E3A in complex with hMAGL was named Phar-MAGL as shown in Fig. 3B. Pharmacophore model, Phar-MAGL is mainly composed of one hydrogen-bond acceptor feature (green spheres), and three hydrophobic features (cyan spheres) Fig. 3C.
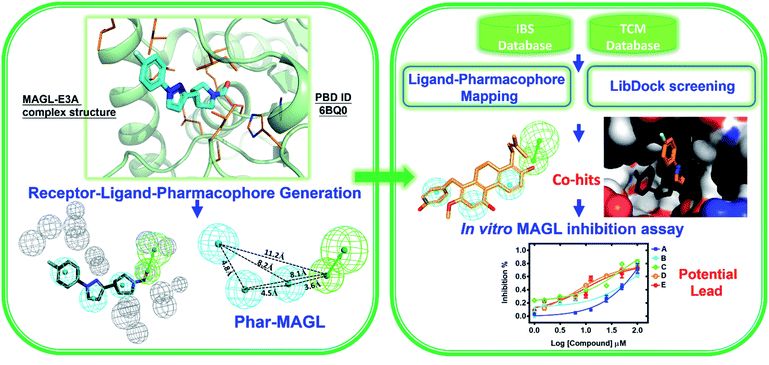 |
| Fig. 1 Flowchart of the combination of structure-based pharmacophore modelling and molecular docking couples with in vitro biochemical assay to identify potential inhibitors from natural product databases for human MAGL inhibition. | |
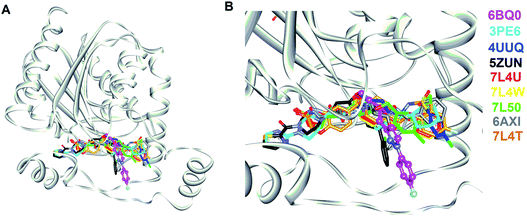 |
| Fig. 2 The complex structures of hMAGL with known inhibitors. (A) The superimposition of complex structures of hMAGL-inhibitor. (B) The amplified view of inhibitors binding into the active site of hMAGL. In (A) and (B), hMAGL was presented as ribbons (light-gray); the inhibitors shown as sticks were colored and labeled with PDB ID. | |
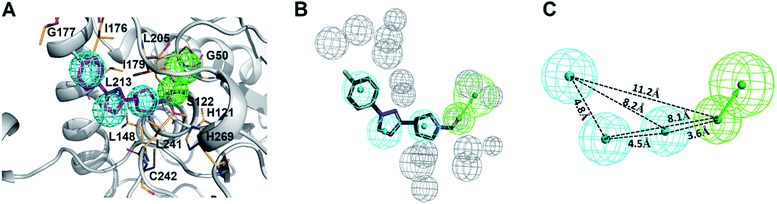 |
| Fig. 3 The construction of pharmacophore model, Phar-MAGL. (A) Receptor-ligand pharmacophore generation of Phar-MAGL was based on the complex structure of hMAGL-E3A (PDB ID: 6BQ0). The protein structure of hMAGL is shown in ribbon with active site residues presenting as light-orange sticks. E3A is shown in magenta stick. (B) The pharmacophore model Phar-MAGL. (C) Features at a specific distance correspond to the pharmacophore model, Phar-MAGL in all panels, the mesh spheres in cyan, green, and gray represent hydrophobic, hydrogen-bond acceptor features, and excluded volume, respectively). | |
Pharmacophore-based inhibitors screening
To comprehensively and systematically screen inhibitor against hMAGL, the functionally essential features of pharmacophore model, Phar-MAGL are considered. The pharmacophore model, Phar-MAGL was then employed to perform ligand-pharmacophore mapping to screen potential inhibitors from IBS and TCM databases. Ligand-pharmacophore mapping can be used to identify ligands that map to a pharmacophore, and aligns the ligands to the query. Ligands fitted with the pharmacophore were output with FitValues (the higher the fit score, the better the match), the measure of how well the ligand fits the pharmacophore. About 68
285 natural products were fitted to Phar-MAGL and the top-ranked hits are selected and compared with the hits from LibDock screening to isolate the commonly identified candidates. Ultimately, 7 potential candidates were selected and their poses fitted with Phar-MAGL were shown in Fig. 4. The hierarchy of the fitting values are NP-1 > NP-2 > NP-3 > NP-4 > NP-5 > NP-6 > NP-7.
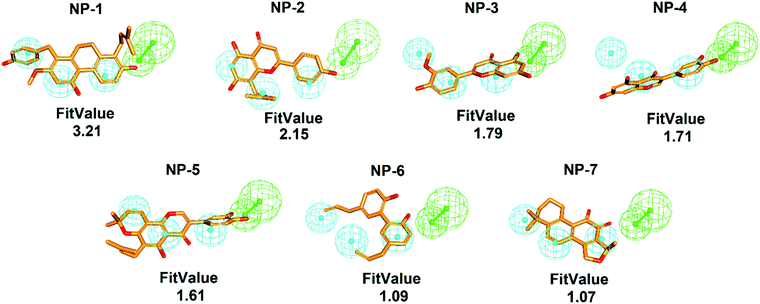 |
| Fig. 4 Pharmacophore-based inhibitor screening. The ligand-pharmacophore mapping results of the commonly identified 7 candidates NP-1 to NP-7. The chemical structures are shown in orange sticks (pharmacophore features are colored as follows: hydrogen-bond acceptor, green; hydrophobic group, cyan). | |
Virtual high-throughput screening (molecular docking) of inhibitors
In addition to pharmacophore screening, we also employed molecular docking to identify the possible inhibitors. The LibDock molecular docking of Discovery Studio 3.5 was used to screen bioactive inhibitors from IBS and TCM databases. LibDock is a high-throughput algorithm for docking ligands into the active site of a receptor.60–64 Ligand conformations are aligned to polar and apolar receptor interaction sites (hotspots) and the best scoring poses are reported. All the natural products (68
285 compounds) were docked into the active site of hMAGL (PBD ID: 6BQ0) following three steps: (i) calculates ligand conformations (ii) docks the conformations using LibDock (iii) minimizes docked poses using CHARMm. The resultant 7 candidates, commonly identified by pharmacophore screening, were selected and presented in Fig. 5. Consequently, the best docking conformation was chosen based on the best docking score of each compound. After that, the numbers of best docking conformations of all compounds were narrowed down—the molecular weight of compounds smaller than 250 Da or larger than 500 Da were eliminated. Subsequently, the Top100 candidates were compared with the result of ligand pharmacophore mapping. The hierarchy of LibDockScore is NP-1 > NP-5 > NP-3 > NP-2 > NP-4 > NP-7 > NP-6. These hits were subjected to human MAGL enzymatic assay to evaluate their inhibitory ability.
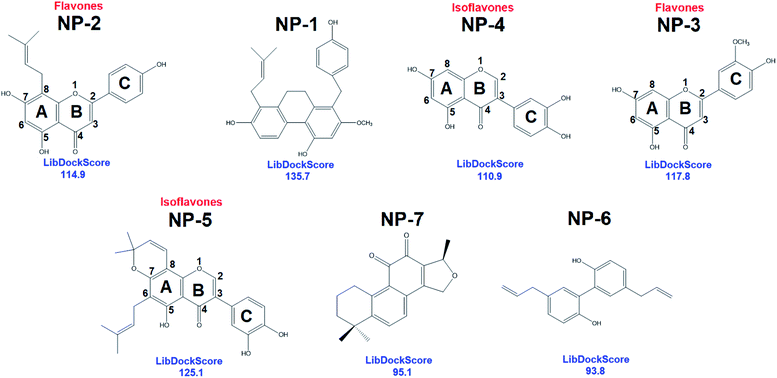 |
| Fig. 5 The 2D chemical structures with docking scores of the commonly identified inhibitors from LibDock molecular docking. | |
Inhibitory ability against human MAGL
The 7 potential candidates commonly identified by both LibDock docking and pharmacophore-base screening were further subjected to inhibition assay to evaluate their capabilities in attenuating the activity of MAGL. Firstly, the inhibitory efficacies of the 7 potential candidates were investigated at 10 μM compound concentration. The result showed that natural products NP-1, NP-2 and NP-3 displayed over 40% inhibition against hMAGL. While the inhibition% of NP-4, NP-5, NP-6, and NP-7 were less than 40% (Fig. 6). We further performed the inhibitory assay in a series of compound concentrations. The result demonstrated that all the 7 inhibitors exhibited the dose-dependent inhibition against hMAGL. The determined IC50 for NP-2, NP-5, NP-3, NP-1, NP-4, NP-7, and NP-6 are 9.5 ± 1.2, 14.5 ± 1.3, 15.2 ± 1.4, 29.7 ± 1.3, 65.5 ± 1.2, 97.2 ± 1.1, 98.1 ± 1.1 μM, respectively (Fig. 7).
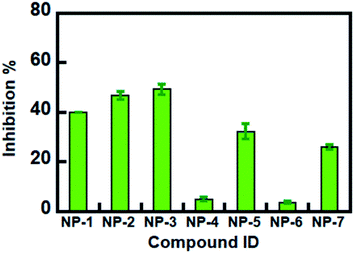 |
| Fig. 6 Inhibitory abilities of identified inhibitors against hMAGL at 10 μM. | |
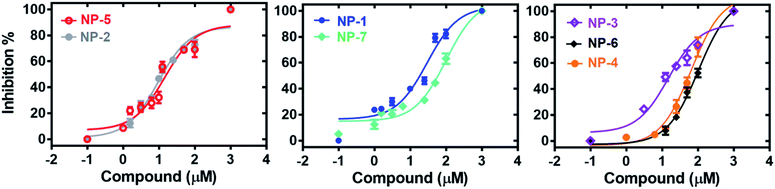 |
| Fig. 7 The dose-dependent inhibitions of the identified inhibitors against hMAGL. | |
Analyses of molecular interactions by ligplot
To better understand the structure–activity relationships of the identified inhibitors towards hMAGL, we employed Ligplot to analyze their detailed molecular interactions. The complex structures of hMAGL-inhibitor obtained from ligand-pharmacophore mapping were subjected to MD simulations (Fig. S3–S9†). The resultant complex structures of hMAGL-inhibitor from MD simulation were further analyzed by Ligplot. The results showed that NP-2 (IC50 = 9.5 ± 1.2 μM) and NP-5 (IC50 = 14.5 ± 1.3 μM) interacted to hMAGL with more hydrogen bonds and/or hydrophobic interactions, compared to those of NP1, NP-3, NP-4, NP-6, and NP-7 (Fig. 8, S1 and S2†). At the same time, these protein-ligand (hMAGL-inhibitors) interactions were also verified by analyses of intermolecular interactions module of Discovery Studio 3.5, and the results were consistent with that of Ligplot analyses as well as the PLIP web tool65 (Tables S1–S7†).
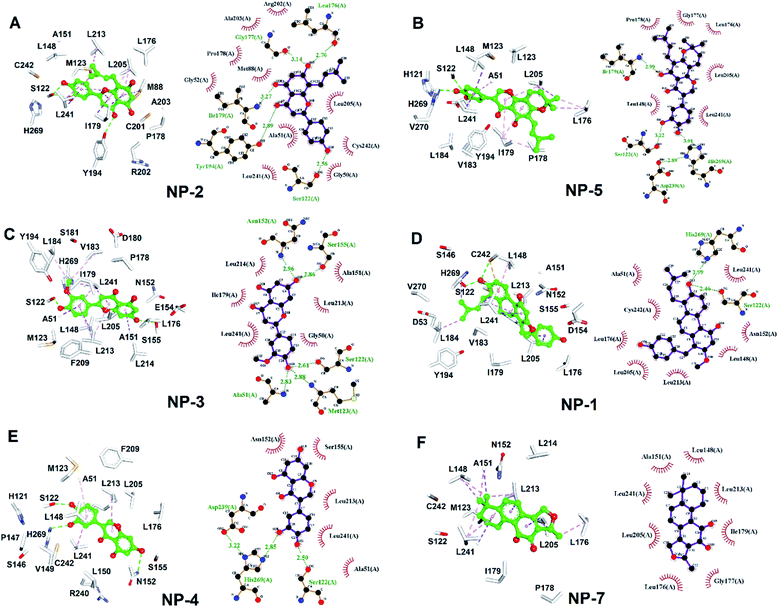 |
| Fig. 8 The molecular interactions of hMAGL-inhibitors. (A–F) The results of Ligplot analyses revealed the interactive networks of hMAGL-NP-1, hMAGL-NP-5, hMAGL-NP-3, hMAGL-NP-1, hMAGL-NP-4, and hMAGL-NP-7 complex structures. In panels (A–F), inhibitors were shown in ball-and-sticks (green); the active site residues were presented as white sticks. The dash lines indicate hydrogen-bond (green) and hydrophobic interactions (light-pink). | |
Discussion
MAGL, a serine hydrolase, catalyzes the hydrolysis of monoglycerides to glycerol. It degrades the 2AG into AA, thus linking the endocannabinoid and eicosanoid systems together.21,34 In addition, both genetic and pharmacologic studies have revealed the function of MAGL in regulating the endocannabinoid and eicosanoid signaling pathways.12,24,41,66 MAGL is highly expressed in brain, especially in astrocytes, microglia, oligodendrocytes and neurons.67,68 Numerous studies reported the therapeutic potential of MAGL inhibitors in disease models, such as anxiety,69 inflammation,70–72 pain,73 and neurodegenerative disorders.74,75 Moreover, MAGL was reported to involve in Alzheimer's and Parkinson's diseases because of its activity in generating the precursor (AA) of eicosanoids promoting neuroinflammation.76 Thus, MAGL is reported as a promising target for treatment of neurodegenerative diseases.27 However, most of the inhibitors against MAGL were characterized by an irreversible mode of action.34 The genetic deletion and chronic administration of irreversible MAGL inhibitors provokes negative effects in vivo. The irreversible inhibitors substantially abolished the activity of MAGL leading to a loss of therapeutic effects and chronic inactivation of MAGL in brain desensitized CB1 receptor.12,41,77 To address these problems, inhibitors characterized with reversible behavior is prefer to used, because they could keep the intact of endocannabinoid system. Therefore, in this study, discovery of reversible hMAGL inhibitors from NPs and TCMs shed lights on developing new and promising therapeutics which avoid the negative effects associated with irreversible inhibitors.
To screening the potential inhibitors from natural products, we employed receptor-ligand pharmacophore generation to build a bioactive pharmacophore model, Phar-MAGL on the basis of hMAGL-E3A complex structure. The built pharmacophore model contains functionally essential features interacting with hMAGL, consisting 1 hydrogen-bond acceptor and 3 hydrophobic features (Fig. 3), was then employed to screen inhibitors. To further test the effectiveness of Phar-MAGL in identifying hMAGL inhibitors, we conducted ligand-pharmacophore mapping to screen candidates from natural product databases. In addition, LibDock molecular docking were performed to screen bioactive candidates from the same compound library to explore the commonly identified inhibitors. The resultant candidates NP-2 showed strong inhibitory potency against hMAGL with IC50 = 9.5 ± 1.2 μM. The compound NP-5, NP-3, and NP-1 exerted moderate inhibitory efficacy; their IC50 values are 14.5 ± 1.3, 15.2 ± 1.4, and 29.7 ± 1.3 μM, individually. In contrast, inhibitors, NP-4, NP-7, and NP-6 were less potent in inhibiting hMAGL (IC50 is over 50 μM). It is noteworthy that NP-1, NP-2, NP-3, and NP-5 were almost all fitted well with the four functional features of Phar-MAGL, (Fig. 4) while NP-4, NP6, and NP7 partially overlapped with these features. This observation could explain the variations of these identified inhibitors in interfering the activity of hMAGL. Also, these results demonstrate the efficiency and reliability of Phar-MAGL in identifying the inhibitors against hMAGL. The complex structures of hMAGL-inhibitor obtained from ligand-pharmacophore mapping were subjected to molecular dynamic simulations and further analyzed by Ligplot to unveil their detailed molecular interactions. NP-2, the most potent inhibitor against hMAGL, interacts with residues S122, L176, I179, G177, and Y194 through hydrogen bondings and hydrophobically contacts with residues G50, A51, G52, M88, P178, R202, A203, L205, L241 and C242 (Fig. 8A and Table 1). Similarly, the inhibitors NP-5 and NP-3 exerted comparable inhibitory potency to that of NP-2. NP5 blocked the active site of hMAGL by contacting with residues S122, I179, D239, and H269 (hydrogen bond interactions) as well as L148, L176, G177, P178, L205, and L241 (hydrophobic interactions) (Fig. 8B and Table 1). NP-3 formed hydrogen bonds with residues A51, S122, M123, N152 and S155, also making hydrophobic contacts with residues G50, A15, I179, L213, L214, and L241 (Fig. 8C and Table 1). Also, moderate inhibitorNP-1 bound into the catalytic site of hMAGL with much less molecular interactions, compared to that of NP-2. The molecule NP-1 occupied the catalytic pocket of hMAGL with only 2 hydrogen bondings (to S122 and H269) and 8 hydrophobic interactions (to A51, L148, N152, L176, L205, L213, L241, and C242) (Fig. 8D and Table 1). On top of that, the less potent inhibitors NP-4, NP-7, and NP-6 can be also explained by their weak molecular interactions towards hMAGL. NP-4 bound to hMAGL with 3 hydrogen bonds (to S122, D239, and H269) and 5 hydrophobic interactions (to A51, N152, S155, L213, and L241) (Fig. 8E and Table 1). NP-7 hydrophobically interacted with residues L148, A151, L176, G177, I179, L205, L213, and L241 (Fig. 8F and Table 1). NP-6 blocked the active site by only interacting with residuesL148, L176, G177, I179, and L241 via hydrophobic contacts (Fig. S1† and Table 1). Notably, S122, D239, and H269 are the catalytic triad of hMAGL and C201, C208 and C242 were proposed to stabilize the active conformation of MAGL. Integrally, the inhibitors NP-1, NP-2, NP-3, NP-4, and NP-5 all formed hydrogen bonds with S122; NP-5 and NP-4 both formed hydrogen bonds with D239; NP-5 NP-1 and NP-4 formed hydrogen bonds with H269. Moreover, NP-2 and NP-1 showed interactions with C242 (Table 1). These key interactions could essentially contribute to the inhibitory capability of the identified inhibitors against hMAGL.
Table 1 Statistics of molecular interactions among the identified inhibitors with MAGLa
The symbol “*” denotes the residues of catalytic triads. The hydrogen bond and hydrophobic interactions are colored in pink and purple, respectively. |
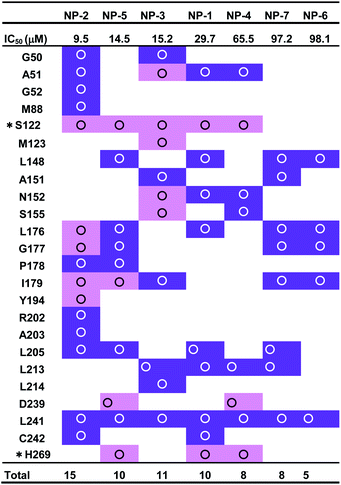 |
Interestingly, most of the identified inhibitors are flavonoids and its derivatives (Fig. 5). Flavonoids are a class of secondary metabolites with polyphenolic structures.78 These natural products are abundantly found in plant origin, such as bark, flowers, fruits, grains, roots, stems, tea, vegetables, and wine.79 It is reported that flavonoids are with anti-carcinogenic, anti-oxidant, anti-inflammatory, and anti-mutagenic effects and indispensable components in a variety of cosmetic, medicinal, nutraceutical, and pharmaceutical applications.79,80 Structurally, NP-2 (IC50 = 9.5 ± 1.2 μM) and NP-3 (IC50 = 15.2 ± 1.4 μM) classified as flavones which have a ketone in position 4 of the C ring and a double bond between position 2 and 3 (Fig. 4). Flavones are one of the important subgroups of flavonoids. In contrast, the structure of NP-4 (IC50 = 65.5 ± 1.2 μM) and NP-5 (IC50 = 14.5 ± 1.3 μM) are classified as the isoflavone, in which position 3 of the C ring is linked to the B ring (Fig. 4). Thus, based on our structural and functional analyses, we found that there could be a preference of the linkage of B and C rings in hMAGL inhibition. When flavonoids with B ring linked to the position 2 of C ring (flavones), their inhibitory ability against hMAGL outstand than those of their B ring linked to the position 3 of C ring (isoflavone). While the derivative of isoflavone NP-5, modified with extra functional groups, exhibited comparable/striking inhibitory capability to that of flavones. Accordingly, our findings unprecedentedly demonstrate the activity of flavonoids in inhibiting hMAGL, also implying its potential in developing drugs for the treatments of neurodegenerative disorders such as AD.
NP-2 (8-prenylnaringenin, 8-PN) is a natural product identified from the plant Humulus lupulus L (hops).81 Humulus lupulus L. are flowering plants of Cannabaceae family, native to NorthAmerica, Europe, and Western Asia.82 The value of Humulus lupulus L. (hops) in producing beer has been undisputed for centuries.83 Hops are abounding in phenolic compounds (mostly flavonoids) that are secondary metabolites.84 In addition, Humulus lupulus L. (hops) have used as traditional medicines to treat as antibacterial, antifungal agents and sleep disorders.85 Besides, the lupulin glands of hop cones excrete prenylated flavonoids which exhibit anticancer, anti-inflammatory, antiseptic, and antiplatelet activities.83 The 8-PN, found as a phytoestrogen in female Humulus lupulus L. (hops) cones, was more effective than the soya isoflavonoid genistein.86,87 In adult, the neurogenesis is partially controlled by sex hormones i.e., estradiol.88,89 It has been reported that estradiol boosts neurogenesis correlating with cognitive improvement.90,91 Moreover, estradiol can also ameliorate the neurodegenerative processes of Alzheimer's and Parkinson's diseases.91,92 It is noteworthy that the phytoestrogen 8-PN, with estradiol-like effects, exhibits an exceptional activity in binding to the α-estrogen receptor.93 As well, 8-PN was reported to positively control GABA-induced responses and its potential in neurodifferentiating has also been reported.94 Here, we demonstrated that 8-PN can interact with hMAGL and strongly reduced its activity (IC50 = 9.5 μM). MAGL is a promising therapeutic target for treatment of AD. The inhibition of hMAGL decreased neurodegeneration, prevented neuroinflammation, and improved long-term spatial learning and memory in AD animal.27 Taken together, the aforementioned demonstrated the great potential of the identified natural product inhibitor NP-2 (8-PN) to serve as a start point in optimizing and creating new and novel therapeutic agents for the treatments of neurodegenerative diseases, especially AD.
Conclusions
Conclusively, we performed pharmacophore-based approach coupled with molecular docking and biochemical assay to screen and characterize inhibitors targeting hMAGL. The built pharmacophore model, Phar-MAGL, comprehensively screened 68
285 natural products and identified compounds NP-2, NP-5, and NP-3 which apparently disrupted the activities of hMAGL. We demonstrated that NP-2 blocked the active site of hMAGL by mainly associating with residues S122, M123, and G177 via hydrogen bond interactions and hydrophobically contacts with residues A51, G52, M88, L148, P178, I179, R202, A203, G204, L205, and C242, which are keys for structure-based lead optimization against hMAGL protein. Besides, the evident activity of the identified inhibitors against hMAGL demonstrated the efficiency and reliability of pharmacophore, Phar-MAGL in identifying the inhibitors against MAGL activity. Also, the discovery of reversible inhibitor NP-2 (8-PN) from Hop (Humulus lupulus L.) is of great potential in developing new and promising therapeutics for treatments of neurodegenerative diseases.
Conflicts of interest
There are no conflicts to declare.
Acknowledgements
We thank to National Center for High-performance Computing (NCHC) for providing computational and storage resources. This study was supported by the Ministry of Science and Technology, Taiwan, ROC (109-2320-B-005-006-MY2) and Tung’s Taichung Metroharbor Hospital (TTMHH-NCHULS110004).
Notes and references
- L. Chen, H. Deng, H. Cui, J. Fang, Z. Zuo, J. Deng, Y. Li, X. Wang and L. Zhao, Oncotarget, 2018, 9, 7204–7218 CrossRef PubMed
. - H. Lassmann, Glia, 2020, 68, 830–844 CrossRef PubMed
. - P. Chen, M. R. Miah and M. Aschner, F1000Research, 2016, 5, 17 Search PubMed
. - B. Uttara, A. V. Singh, P. Zamboni and R. T. Mahajan, Curr. Neuropharmacol., 2009, 7, 65–74 CrossRef CAS PubMed
. - J. A. Smith, A. Das, S. K. Ray and N. L. Banik, Brain Res. Bull., 2012, 87, 10–20 CrossRef CAS PubMed
. - W. Y. Wang, M. S. Tan, J. T. Yu and L. Tan, Ann. Transl. Med., 2015, 3, 136 Search PubMed
. - R. M. Ransohoff, Science, 2016, 353, 777–783 CrossRef CAS PubMed
. - J. W. Kinney, S. M. Bemiller, A. S. Murtishaw, A. M. Leisgang, A. M. Salazar and B. T. Lamb, Alzheimer's Dementia, 2018, 4, 575–590 CrossRef PubMed
. - M. R. Minter, J. M. Taylor and P. J. Crack, J. Neurochem., 2016, 136, 457–474 CrossRef CAS PubMed
. - J. L. Cummings, T. Morstorf and K. Zhong, Alzheimer's Res. Ther., 2014, 6, 37 CrossRef PubMed
. - J. L. Cummings, G. Tong and C. Ballard, J. Alzheimer's Dis., 2019, 67, 779–794 Search PubMed
. - P. K. Chanda, Y. Gao, L. Mark, J. Btesh, B. W. Strassle, P. Lu, M. J. Piesla, M. Y. Zhang, B. Bingham, A. Uveges, D. Kowal, D. Garbe, E. V. Kouranova, R. H. Ring, B. Bates, M. N. Pangalos, J. D. Kennedy, G. T. Whiteside and T. A. Samad, Mol. Pharmacol., 2010, 78, 996–1003 CrossRef CAS PubMed
. - J. Z. Long, D. K. Nomura and B. F. Cravatt, Chem. Biol., 2009, 16, 744–753 CrossRef CAS PubMed
. - T. P. Dinh, T. F. Freund and D. Piomelli, Chem. Phys. Lipids, 2002, 121, 149–158 CrossRef CAS PubMed
. - D. K. Nomura, B. E. Morrison, J. L. Blankman, J. Z. Long, S. G. Kinsey, M. C. Marcondes, A. M. Ward, Y. K. Hahn, A. H. Lichtman, B. Conti and B. F. Cravatt, Science, 2011, 334, 809–813 CrossRef CAS PubMed
. - V. S. Hanna and E. A. A. Hafez, J. Adv. Res., 2018, 11, 23–32 CrossRef CAS PubMed
. - B. Wang, L. Wu, J. Chen, L. Dong, C. Chen, Z. Wen, J. Hu, I. Fleming and D. W. Wang, Signal Transduction Targeted Ther., 2021, 6, 94 CrossRef CAS PubMed
. - N. Barrie and N. Manolios, Eur. J. Rheumatol. Inflammation, 2017, 4, 210–218 CrossRef PubMed
. - C. Turcotte, F. Chouinard, J. S. Lefebvre and N. Flamand, J. Leukocyte Biol., 2015, 97, 1049–1070 CrossRef CAS PubMed
. - C. J. Fowler, Br. J. Pharmacol., 2012, 166, 1568–1585 CrossRef CAS PubMed
. - G. F. Grabner, R. Zimmermann, R. Schicho and U. Taschler, Pharmacol. Ther., 2017, 175, 35–46 CrossRef CAS PubMed
. - B. Ignatowska-Jankowska, J. L. Wilkerson, M. Mustafa, R. Abdullah, M. Niphakis, J. L. Wiley, B. F. Cravatt and A. H. Lichtman, J. Pharmacol. Exp. Ther., 2015, 353, 424–432 CrossRef CAS PubMed
. - S. G. Kinsey, L. E. Wise, D. Ramesh, R. Abdullah, D. E. Selley, B. F. Cravatt and A. H. Lichtman, J. Pharmacol. Exp. Ther., 2013, 345, 492–501 CrossRef CAS PubMed
. - M. M. Mulvihill and D. K. Nomura, Life Sci., 2013, 92, 492–497 CrossRef CAS PubMed
. - J. R. Savinainen, S. M. Saario and J. T. Laitinen, Acta Physiologica, 2012, 204, 267–276 CrossRef CAS PubMed
. - D. K. Nomura, C. S. Hudak, A. M. Ward, J. J. Burston, R. S. Issa, K. J. Fisher, M. E. Abood, J. L. Wiley, A. H. Lichtman and J. E. Casida, Bioorg. Med. Chem. Lett., 2008, 18, 5875–5878 CrossRef CAS PubMed
. - R. Chen, J. Zhang, Y. Wu, D. Wang, G. Feng, Y. P. Tang, Z. Teng and C. Chen, Cell Rep., 2012, 2, 1329–1339 CrossRef CAS PubMed
. - J. R. Piro, D. I. Benjamin, J. M. Duerr, Y. Pi, C. Gonzales, K. M. Wood, J. W. Schwartz, D. K. Nomura and T. A. Samad, Cell Rep., 2012, 1, 617–623 CrossRef CAS PubMed
. - S. Gundamraj and R. Hasbun, Front. Cell. Infect. Microbiol., 2020, 10, 592017 CrossRef PubMed
. - D. Grennan and S. Wang, JAMA, 2019, 322, 282 CrossRef PubMed
. - M. Yasir, A. Goyal, P. Bansal and S. Sonthalia, in StatPearls, Treasure Island (FL), 2021 Search PubMed
. - G. Labar, C. Bauvois, F. Borel, J. L. Ferrer, J. Wouters and D. M. Lambert, Chembiochem, 2010, 11, 218–227 CrossRef CAS PubMed
. - C. Schalk-Hihi, C. Schubert, R. Alexander, S. Bayoumy, J. C. Clemente, I. Deckman, R. L. DesJarlais, K. C. Dzordzorme, C. M. Flores, B. Grasberger, J. K. Kranz, F. Lewandowski, L. Liu, H. Ma, D. Maguire, M. J. Macielag, M. E. McDonnell, T. Mezzasalma Haarlander, R. Miller, C. Milligan, C. Reynolds and L. C. Kuo, Protein Sci., 2011, 20, 670–683 CrossRef CAS PubMed
. - H. Deng and W. Li, Acta Pharm. Sin. B, 2020, 10, 582–602 CrossRef CAS PubMed
. - N. Matuszak, G. G. Muccioli, G. Labar and D. M. Lambert, J. Med. Chem., 2009, 52, 7410–7420 CrossRef CAS PubMed
. - C. N. Kapanda, G. G. Muccioli, G. Labar, J. H. Poupaert and D. M. Lambert, J. Med. Chem., 2009, 52, 7310–7314 CrossRef CAS PubMed
. - G. Griebel, P. Pichat, S. Beeske, T. Leroy, N. Redon, A. Jacquet, D. Francon, L. Bert, L. Even, M. Lopez-Grancha, T. Tolstykh, F. Sun, Q. Yu, S. Brittain, H. Arlt, T. He, B. Zhang, D. Wiederschain, T. Bertrand, J. Houtmann, A. Rak, F. Vallee, N. Michot, F. Auge, V. Menet, O. E. Bergis, P. George, P. Avenet, V. Mikol, M. Didier and J. Escoubet, Sci. Rep., 2015, 5, 7642 CrossRef CAS PubMed
. - J. W. Chang, M. J. Niphakis, K. M. Lum, A. B. Cognetta 3rd, C. Wang, M. L. Matthews, S. Niessen, M. W. Buczynski, L. H. Parsons and B. F. Cravatt, Chem. Biol., 2012, 19, 579–588 CrossRef CAS PubMed
. - N. Aaltonen, J. R. Savinainen, C. R. Ribas, J. Ronkko, A. Kuusisto, J. Korhonen, D. Navia-Paldanius, J. Hayrinen, P. Takabe, H. Kasnanen, T. Pantsar, T. Laitinen, M. Lehtonen, S. Pasonen-Seppanen, A. Poso, T. Nevalainen and J. T. Laitinen, Chem. Biol., 2013, 20, 379–390 CrossRef CAS PubMed
. - C. N. Kapanda, J. Masquelier, G. Labar, G. G. Muccioli, J. H. Poupaert and D. M. Lambert, J. Med. Chem., 2012, 55, 5774–5783 CrossRef CAS PubMed
. - J. E. Schlosburg, J. L. Blankman, J. Z. Long, D. K. Nomura, B. Pan, S. G. Kinsey, P. T. Nguyen, D. Ramesh, L. Booker, J. J. Burston, E. A. Thomas, D. E. Selley, L. J. Sim-Selley, Q. S. Liu, A. H. Lichtman and B. F. Cravatt, Nat. Neurosci., 2010, 13, 1113–1119 CrossRef CAS PubMed
. - A. Abdel-Rahman, N. Anyangwe, L. Carlacci, S. Casper, R. P. Danam, E. Enongene, G. Erives, D. Fabricant, R. Gudi, C. J. Hilmas, F. Hines, P. Howard, D. Levy, Y. Lin, R. J. Moore, E. Pfeiler, T. S. Thurmond, S. Turujman and N. J. Walker, Toxicol. Sci., 2011, 123, 333–348 CrossRef CAS PubMed
. - J. D. McChesney, P. R. Health Sci. J., 2002, 21, 91–95 Search PubMed
. - S. W. Tang, W. H. Tang and B. E. Leonard, International Clinical Psychopharmacology, 2020, 35, 1–7 CrossRef PubMed
. - G. G. Muccioli, G. Labar and D. M. Lambert, Chembiochem, 2008, 9, 2704–2710 CrossRef CAS PubMed
. - X. Lu, H. Yang, Y. Chen, Q. Li, S. Y. He, X. Jiang, F. Feng, W. Qu and H. Sun, Curr. Pharm. Des., 2018, 24, 3424–3439 CrossRef CAS
. - S. Y. Yang, Drug Discovery Today, 2010, 15, 444–450 CrossRef CAS PubMed
. - S. Pirhadi, F. Shiri and J. B. Ghasemi, Curr. Top. Med. Chem., 2013, 13, 1036–1047 CrossRef CAS PubMed
. - S. A. Halim, S. Khan, A. Khan, A. Wadood, F. Mabood, J. Hussain and A. Al-Harrasi, Front. Chem., 2017, 5, 88 CrossRef PubMed
. - Y. C. Ma, B. Yang, X. Wang, L. Zhou, W. Y. Li, W. S. Liu, X. H. Lu, Z. H. Zheng, Y. Ma and R. L. Wang, J. Biomol. Struct. Dyn., 2020, 38, 4432–4448 CrossRef CAS PubMed
. - F. Opo, M. M. Rahman, F. Ahammad, I. Ahmed, M. A. Bhuiyan and A. M. Asiri, Sci. Rep., 2021, 11, 4049 CrossRef CAS PubMed
. - S. Pirhadi, T. Damghani, M. S. Avestan and S. Sharifi, J. Recept. Signal Transduction Res., 2020, 40, 357–364 CrossRef CAS PubMed
. - M. Sharma, N. Sharma, M. Muddassir, Q. I. Rahman, U. N. Dwivedi and S. Akhtar, J. Biomol. Struct. Dyn., 2021, 1–18, DOI:10.1080/07391102.2021.1936178
. - H. P. Sun, J. Zhu, F. H. Chen and Q. D. You, Mol. Inf., 2011, 30, 579–592 CrossRef CAS PubMed
. - K. R. Valasani, J. R. Vangavaragu, V. W. Day and S. S. Yan, J. Chem. Inf. Model., 2014, 54, 902–912 CrossRef CAS
. - Y. Zhou, S. Tang, T. Chen and M. M. Niu, Molecules, 2019, 24(23), 4258 CrossRef CAS PubMed
. - S. A. Khedkar, A. K. Malde, E. C. Coutinho and S. Srivastava, Med. Chem., 2007, 3, 187–197 CrossRef CAS PubMed
. - O. F. Guner, IDrugs, 2005, 8, 567–572 Search PubMed
. - Q. Gao, L. Yang and Y. Zhu, Curr. Comput.-Aided Drug Des., 2010, 6, 37–49 CrossRef CAS PubMed
. - S. N. Rao, M. S. Head, A. Kulkarni and J. M. LaLonde, J. Chem. Inf. Model., 2007, 47, 2159–2171 CrossRef CAS PubMed
. - D. J. Diller and R. Li, J. Med. Chem., 2003, 46, 4638–4647 CrossRef CAS PubMed
. - D. J. Diller and K. M. Merz Jr, Proteins, 2001, 43, 113–124 CrossRef CAS
. - D. B. Kitchen, H. Decornez, J. R. Furr and J. Bajorath, Nat. Rev. Drug Discovery, 2004, 3, 935–949 CrossRef CAS PubMed
. - S. Pal, V. Kumar, B. Kundu, D. Bhattacharya, N. Preethy, M. P. Reddy and A. Talukdar, Comput. Struct. Biotechnol. J., 2019, 17, 291–310 CrossRef CAS
. - M. F. Adasme, K. L. Linnemann, S. N. Bolz, F. Kaiser, S. Salentin, V. J. Haupt and M. Schroeder, Nucleic Acids Res., 2021, 49, W530–W534 CrossRef CAS PubMed
. - J. Z. Long, W. Li, L. Booker, J. J. Burston, S. G. Kinsey, J. E. Schlosburg, F. J. Pavon, A. M. Serrano, D. E. Selley, L. H. Parsons, A. H. Lichtman and B. F. Cravatt, Nat. Chem. Biol., 2009, 5, 37–44 CrossRef CAS PubMed
. - T. P. Dinh, D. Carpenter, F. M. Leslie, T. F. Freund, I. Katona, S. L. Sensi, S. Kathuria and D. Piomelli, Proc. Natl. Acad. Sci. U. S. A., 2002, 99, 10819–10824 CrossRef CAS PubMed
. - A. I. Gulyas, B. F. Cravatt, M. H. Bracey, T. P. Dinh, D. Piomelli, F. Boscia and T. F. Freund, European Journal of Neuroscience, 2004, 20, 441–458 CrossRef CAS PubMed
. - G. Bedse, R. J. Bluett, T. A. Patrick, N. K. Romness, A. D. Gaulden, P. J. Kingsley, N. Plath, L. J. Marnett and S. Patel, Transl. Psychiatry, 2018, 8, 92 CrossRef PubMed
. - D. Bridges, K. Ahmad and A. S. Rice, Br. J. Pharmacol., 2001, 133, 586–594 CrossRef CAS PubMed
. - M. M. Ibrahim, H. Deng, A. Zvonok, D. A. Cockayne, J. Kwan, H. P. Mata, T. W. Vanderah, J. Lai, F. Porreca, A. Makriyannis and T. P. Malan Jr, Proc. Natl. Acad. Sci. U. S. A., 2003, 100, 10529–10533 CrossRef CAS PubMed
. - S. G. Kinsey, D. K. Nomura, S. T. O'Neal, J. Z. Long, A. Mahadevan, B. F. Cravatt, J. R. Grider and A. H. Lichtman, J. Pharmacol. Exp. Ther., 2011, 338, 795–802 CrossRef CAS PubMed
. - S. Dvoracsko, A. Stefanucci, E. Novellino and A. Mollica, Future Med. Chem., 2015, 7, 2469–2483 CrossRef CAS PubMed
. - E. L. Scotter, M. E. Abood and M. Glass, Br. J. Pharmacol., 2010, 160, 480–498 CrossRef CAS PubMed
. - N. Pasquarelli, C. Porazik, H. Bayer, E. Buck, S. Schildknecht, P. Weydt, A. Witting and B. Ferger, Neurochem. Int., 2017, 110, 14–24 CrossRef CAS PubMed
. - C. K. Glass, K. Saijo, B. Winner, M. C. Marchetto and F. H. Gage, Cell, 2010, 140, 918–934 CrossRef CAS PubMed
. - A. Bernal-Chico, M. Canedo, A. Manterola, M. Victoria Sanchez-Gomez, A. Perez-Samartin, R. Rodriguez-Puertas, C. Matute and S. Mato, Glia, 2015, 63, 163–176 CrossRef PubMed
. - S. Kumar and A. K. Pandey, Sci. World J., 2013, 2013, 162750 Search PubMed
. - A. N. Panche, A. D. Diwan and S. R. Chandra, J. Nutr. Sci., 2016, 5, e47 CrossRef CAS PubMed
. - M. M. Juca, F. M. S. Cysne Filho, J. C. de Almeida, D. D. S. Mesquita, J. R. M. Barriga, K. C. F. Dias, T. M. Barbosa, L. C. Vasconcelos, L. Leal, J. E. Ribeiro and S. M. M. Vasconcelos, Nat. Prod. Res., 2020, 34, 692–705 CrossRef CAS PubMed
. - S. Possemiers, A. Heyerick, V. Robbens, D. De Keukeleire and W. Verstraete, J. Agric. Food Chem., 2005, 53, 6281–6288 CrossRef CAS PubMed
. - J. L. McCallum, M. H. Nabuurs, S. T. Gallant, C. W. Kirby and A. A. S. Mills, Front. Plant Sci., 2019, 10, 1438 CrossRef PubMed
. - T. Tronina, J. Poplonski and A. Bartmanska, Molecules, 2020, 25(18), 4201 CrossRef CAS PubMed
. - T. E. Ceremuga, L. A. Johnson, J. M. Adams-Henderson, S. McCall and D. Johnson, AANA Journal, 2013, 81, 193–198 Search PubMed
. - P. Zanoli and M. Zavatti, J. Ethnopharmacol., 2008, 116, 383–396 CrossRef CAS PubMed
. - K. Stulikova, M. Karabin, J. Nespor and P. Dostalek, Molecules, 2018, 23(3), 660 CrossRef PubMed
. - S. Milligan, J. Kalita, V. Pocock, A. Heyerick, L. De Cooman, H. Rong and D. De Keukeleire, Reproduction, 2002, 123, 235–242 CAS
. - C. Jorgensen and Z. Wang, Biomolecules, 2020, 10(8), 1151 CrossRef CAS PubMed
. - K. M. Hillerer, D. A. Slattery and B. Pletzer, Front. Neuroendocrinol., 2019, 55, 100796 CrossRef CAS PubMed
. - J. L. Spencer, E. M. Waters, R. D. Romeo, G. E. Wood, T. A. Milner and B. S. McEwen, Front. Neuroendocrinol., 2008, 29, 219–237 CrossRef CAS PubMed
. - F. A. Bustamante-Barrientos, M. Mendez-Ruette, A. Ortloff, P. Luz-Crawford, F. J. Rivera, C. D. Figueroa, L. Molina and L. F. Batiz, Front. Cell. Neurosci., 2021, 15, 636176 CrossRef CAS PubMed
. - E. Vegeto, V. Benedusi and A. Maggi, Front. Neuroendocrinol., 2008, 29, 507–519 CrossRef CAS PubMed
. - M. Bottner, J. Christoffel and W. Wuttke, J. Endocrinol., 2008, 198, 395–401 Search PubMed
. - A. Y. Benkherouf, S. L. Soini, M. Stompor and M. Uusi-Oukari, Eur. J. Pharmacol., 2019, 852, 34–41 CrossRef CAS PubMed
.
Footnotes |
† Electronic supplementary information (ESI) available. See DOI: 10.1039/d1ra05311f |
‡ These authors contributed equally to this work. |
|
This journal is © The Royal Society of Chemistry 2021 |
Click here to see how this site uses Cookies. View our privacy policy here.