DOI:
10.1039/C8AN01292J
(Paper)
Analyst, 2018,
143, 6103-6112
Characterization of inflammatory breast cancer: a vibrational microspectroscopy and imaging approach at the cellular and tissue level†
Received
13th July 2018
, Accepted 23rd October 2018
First published on 23rd November 2018
Abstract
Inflammatory breast cancer (IBC) has a poor prognosis because of the lack of specific biomarkers and its late diagnosis. An accurate and rapid diagnosis implemented early enough can significantly improve the disease outcome. Vibrational spectroscopy has proven to be useful for cell and tissue characterization based on the intrinsic molecular information. Here, we have applied infrared and Raman microspectroscopy and imaging to differentiate between non-IBC and IBC at both cell and tissue levels. Two human breast cancer cell lines (MDA-MB-231 and SUM-149), 20 breast cancer patients (10 non-IBC and 10 IBC), and 4 healthy volunteer biopsies were investigated. Fixed cells and tissues were analyzed by FTIR microspectroscopy and imaging, while live cells were studied by Raman microspectroscopy. Spectra were analyzed by hierarchical cluster analysis (HCA) and images by common k-means clustering algorithms. For both cell suspensions and single cells, FTIR spectroscopy showed sufficient high inter-group variability to delineate MDA-MB-231 and SUM-149 cell lines. Most significant differences were observed in the spectral regions of 1096–1108 and 1672–1692 cm−1. Analysis of live cells by Raman microspectroscopy gave also a good discrimination of these cell types. The most discriminant regions were 688–992, 1019–1114, 1217–1375 and 1516–1625 cm−1. Finally, k-means cluster analysis of FTIR images allowed delineating non-IBC from IBC tissues. This study demonstrates the potential of vibrational spectroscopy and imaging to discriminate between non-IBC and IBC at both cell and tissue levels.
1. Introduction
Inflammatory breast cancer (IBC) is one of the most lethal and fatal forms of breast cancer with a higher incidence in young women.1 It is characterized by a low survival rate in comparison with other breast cancer types.2 The majority of IBC patients have no well-defined mass on clinical examination and the first symptom is rapid breast enlargement and changes in the skin overlying the breast.2 Clinically, IBC is characterized by rapid onset, erythema, edema of the breast, and a “peau d'orange” appearance of breast skin. Moreover, at diagnosis, IBC patients are characterized by the presence of positive metastatic lymph nodes and up to 30% of IBC patients have metastasis at diagnosis.3 Nipple flattening, retraction, crusting, or blistering may be frequently apparent but not included as a diagnostic clinical feature of IBC.4 However, some conditions such as nonpuerperal bacteria mastitis may lead to late diagnosis and treatment of IBC.5 Pathologically, IBC is characterized by the presence of dermal and stromal tumor emboli. Lymphatic tumor emboli prevent drainage of the lymph fluid leading to swelling of the breast tissue and causing inflammation.6
Mammography and ultrasound are the common diagnostic and therapeutic monitoring imaging modalities of breast cancer.7 The main purpose of imaging in IBC is to determine the primary breast tumor, identify distant metastases, support image-guided biopsy, and optimally evaluate tumor response to neoadjuvant chemotherapy.4 However, mammography has a high rate of false-positives,8 or negative diagnostics.9 Other imaging modalities are more frequently used in breast cancer diagnosis such as magnetic resonance imaging (MRI, functional MRI), hybrid positron emission tomography/computed tomography (PET/CT, cone-beam breast CT) and 3-D ultrasound.4 However, these imaging modalities may not allow early diagnosis of IBC in some cases.
Many studies have tried to identify specific biological markers to differentiate between non-IBC and IBC conditions. For instance, E-cadherin is an epithelial marker and calcium dependent transmembrane glycoprotein that mediates epithelial cell–cell adhesion.10 IBC cells are characterized by overexpression of E-cadherin, which is essential for cell adherence and tumor embolus formation.11 In non-IBC, the loss of E-cadherin expression contributes to increased tumor proliferation and to the progression of metastasis and is associated with poor prognosis.12 DNA microarray studies showed gene expression differences between IBC and non-IBC and overexpression of toll-like receptors (TLR) in IBC tissues.13 Another study comparing MicroRNA (miRNA) expression profiles in non-IBC, IBC carcinoma tissues, and normal breast tissues found that IBC carcinoma tissues are characterized by five overexpressed miRNAs comprising miR-421, miR-486, miR-503, miR-720, and miR-1303.14 However, all previously discussed markers do not distinguish IBC from non-IBC and fail to explain the specific pathobiology of IBC. Late diagnosis of IBC due to the absence of any biological markers was associated with a low survival rate compared to other types of breast cancers.2 Therefore, there is room for new developments in order to characterize IBC and non-IBC cells and tissues.
Fourier-transform infrared (FTIR) and Raman vibrational spectroscopies have proven to be useful diagnostic tools for characterizing carcinoma cells and tissues based on their intrinsic molecular information.15–18 The obtained spectral data provide information on the structure and composition of characteristic biomolecules such as lipids, proteins, nucleic acids, and polysaccharides. Thus, vibrational signatures represent a complete “molecular fingerprint” of the sample and can be both qualitative and quantitative. Vibrational microspectroscopy/imaging is a highly sensitive method capable of probing cells and tissues at the micron level. It is a rapid, non-invasive and label-free approach,19 capable of detecting tumor heterogeneities, thus highlighting subtle intra-tumoral biological differences that may not be addressed through conventional histology approaches.20 A combination of spectroscopy with chemometrics tools like HCA,21,22 k-means (KM) clustering,23 and fuzzy c-means (FCM) clustering,24 could distinguish different cell types within the same tissue or even between different cancer cells in the same carcinoma tissue.
Previous studies have used FTIR and Raman spectroscopy for analyzing breast cancer cells and tissues. It has been reported that FTIR spectroscopy on isolated peripheral blood mononuclear cells (PBMCs) is potentially feasible. It is an efficient tool to differentiate between healthy and breast cancer patients, to detect early breast neoplasms and to reduce false positive results at screening.25 FTIR imaging was used to study the effect on three different breast cancer cell lines co-cultured with normal fibroblasts. The obtained spectral data easily discriminate between normal and co-cultured fibroblasts independent of their morphological changes.26 Recently, a simple model for cell type recognition using 2D-correlation analysis of FTIR images allowed the separation of the extracellular matrix (ECM) from carcinoma cells in breast cancer tissue.27 Raman confocal microscopy was used to study the impact of four different polyphenols (epigallocatechin gallate (EGCG), gallic acid, resveratrol and tannic acid) on the nucleus and cytoplasm of MCF-7 breast cancer cells. An increase in lipid content was observed after exposure to these polyphenols.28
FTIR imaging was applied on breast biopsies of different tumor histological grades to study the changes in the microenvironment surrounding the carcinomas.29 Differences were due to collagen bands at 1630 and 1640 cm−1, when the ECM near and far from the tumor was compared. Raman spectroscopy has also been proposed for the detection of tumor margins during breast cancer surgery, both in vivo and ex vivo.30,31 In addition, Raman spectroscopy was used to compare the spectra obtained from ductal carcinoma, surrounding inflammatory stroma and healthy breast tissue (lobules, ducts, stroma and fat).32 Furthermore, Raman spectroscopy was used in vivo to evaluate the biochemical changes pre- and post-photodynamic therapy in live rat breast tumors.33 In addition, Zhang and Barman employed spontaneous Raman spectroscopy for early detection and evaluation of cancer-colonized bone alterations in a mouse model system.34
The aim of the present study was to explore the potential of vibrational microspectroscopy/imaging, in combination with chemometric analytical methods for differentiating, on the one hand, between non-IBC and IBC cell lines and on the other hand, tissue sections from non-IBC and IBC patient biopsies.
2. Materials and methods
2.1. Cell lines
Two human breast cancer cell lines MDA-MB-231 (ATCC® HTB-26™), representing non-IBC, and SUM-149 representing IBC (kindly provided by the Cancer Biology Research Laboratory, Zoology department, Faculty of Science, Cairo University, Giza, Egypt), were used in this study. MDA-MB-231 cells were cultured in DMEM medium with 10% fetal bovine serum and 1% of penicillin/streptomycin antibiotic mixture. SUM-149 cells were cultured in HAM's F12 medium with 5% fetal bovine serum, 5 mM HEPES, 1 μg ml−1 hydrocortisone, 5 μg ml−1 insulin and 1% of penicillin/streptomycin antibiotic mixture. Both cell lines were incubated at 37 °C in 5% CO2.
2.2. High-throughput infrared analysis of cell suspensions
At 80% of confluency, cells were detached using 1% trypsin, washed with growth media supplemented with FBS, and then rewashed twice with PBS. The cells were then resuspended in sterile water and 5 μL of cell suspension (105 cells per mL) were deposited onto a 384-well silicon plate and left to air-dry. After 1 h drying at room temperature, the plate was placed in the high-throughput screening HTS-XT extension coupled to a Tensor 27 spectrometer (Bruker Optics GmbH, Ettlingen, Germany). Three independent cultures and 8 replicates per culture were analyzed. Measurements were performed in the transmission mode, at a spectral resolution of 4 cm−1 using 64 co-additions in the spectral range of 4000–400 cm−1.
2.3. FTIR imaging of single fixed cells
Two mL of cell suspension (105 cells per mL) were seeded on a calcium fluoride (CaF2) substrate (Crystran, Dorset, UK) and incubated for 24 h at 37 °C in 5% CO2. Cells were then fixed with 4% paraformaldehyde (PFA) for 20 min and washed three times with sterile distilled water, and then left to air-dry. The cells were analyzed with a FTIR imaging system (Spotlight 400, PerkinElmer, Courtaboeuf, France). The cells were selected after visual inspection and FTIR images were recorded in the transmission mode at a spatial resolution of 6.25 μm per pixel, and a spectral resolution of 4 cm−1 using 128 scans in the spectral range of 4000–800 cm−1. Three independent cultures were used and 8 cells per culture were analyzed.
2.4. Raman microspectroscopy of live cells
Two mL of cell suspension (105 cells per mL) were seeded on a CaF2 substrate and incubated for 24 h at 37 °C in 5% CO2. Cells were then rinsed three times with 0.9% sterilized NaCl and immersed in the same solution for Raman acquisition under live conditions. Live cells were analyzed with an Labram Raman microspectrometer (Horiba Jobin Yvon, Villeneuve d'Ascq, France) equipped with laser excitation at 660 nm, 950 lines per mm grating and using a confocal hole of 300 μm. The objective used on the microscope (Olympus BX40) was a 100× water immersion objective (NA: 1.0) giving a laser spot size of ∼1 μm. The laser power at the sample was 28 mW and the acquisition was performed in point mode in the spectral range of 600 to 1750 cm−1 with 1 accumulation of 45 s. Ten different individual cells were analyzed and for each cell, five Raman spectra were recorded from the cytoplasm.
2.5. Patient tissue samples
For patient inclusion in this study, Institutional Review Board (IRB) approval was obtained from the ethics committee of the Faculty of Medicine, Ain Shams University, Egypt (IRB#00006379). Each patient provided a signed consent form including approval for publication of results. Patients were clinically and histopathologically diagnosed as non-IBC and IBC as described before.35 A total of twenty breast cancer patients (10 non-IBC and 10 IBC), all triple negative (ER−, PR− and Her-2), and four healthy volunteers who had breast reduction surgery were enrolled.
2.6. FTIR imaging of formalin-fixed paraffin-embedded tissues
FTIR spectral images were acquired on formalin-fixed paraffin-embedded (FFPE) human breast tissues. To do so, three 5 μm thick sections were prepared. The first and third sections were stained with hematoxylin phloxin saffron solution (HPS) and underwent histopathological examination to define the normal and carcinoma outlines. The second tissue section was mounted on a CaF2 window for FTIR microspectroscopy/imaging analysis without any particular preparation, especially no chemical dewaxing. Images were acquired in transmission mode using the Spotlight 400 imaging system at a pixel size of 6.25 μm, and a spectral resolution of 4 cm−1 using 16 scans in the spectral range of 4000–800 cm−1. The workflow of the FTIR imaging and data analysis of the FFPE tissue sections is shown in Fig. 1.
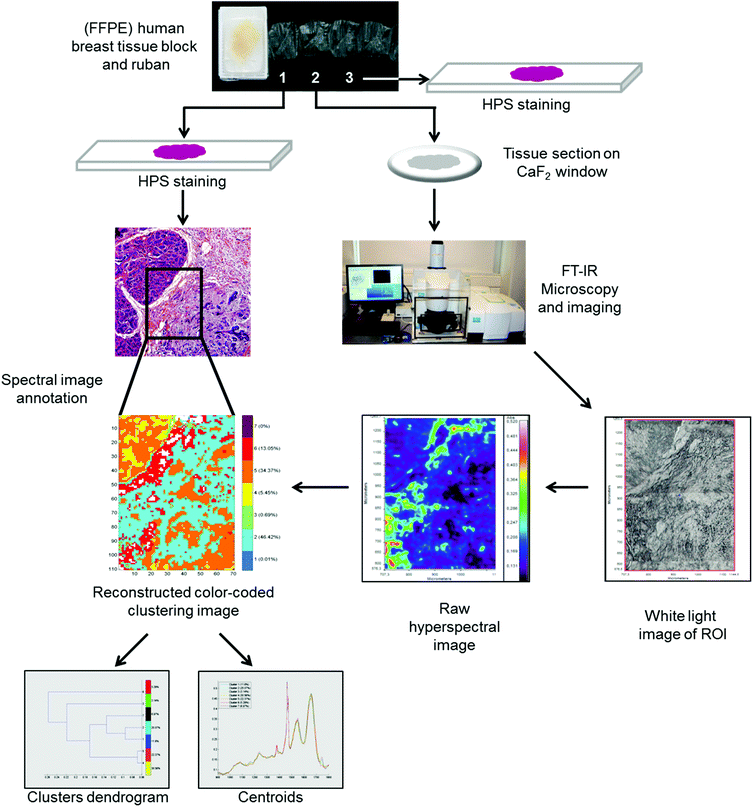 |
| Fig. 1 Workflow showing the FTIR spectral imaging analysis of FFPE breast tissue sections and analysis of FTIR images with common k-means clustering. | |
2.7. Preprocessing and processing of cell spectral data
2.7.1. HT-FTIR of cell suspensions.
Spectral data obtained from cell suspensions were baseline corrected (elastic function, 5 points) and their second derivative spectra computed and vector normalized to increase spectral differences and for comparing spectra with each other. Then, spectral data were processed by hierarchical cluster analysis (HCA) (Matlabsoftware) which is based on a distance calculation. The results of HCA analysis are represented in a tree figure called a dendrogram.
2.7.2. FTIR imaging of single fixed cells.
For single fixed cell imaging, the CaF2 substrate background was automatically subtracted from the recorded FTIR hyperspectral cell images. Spectra were obtained from the whole single cell and all of the obtained spectra of the same cell type were baseline corrected and their second derivative spectra computed and vector normalized. The processed spectra were analyzed by HCA analysis (OPUS 6.5 software, Bruker Optics GmbH, Ettlingen, Germany).
2.7.3. Raman microspectroscopy of single live cells.
Raman spectra were preprocessed using an in-house routine built in the Matlab software (MathWorks, Natick, MA, USA). First a set of calibration spectra (silicon, neon lamp, NIST, optics, dark current, CaF2 substrate) were recorded and these were subsequently used to process the raw Raman spectra. This procedure has been previously described in detail.36 The spectra were then smoothed using a Savitzky–Golay function with second order polynomial, baseline corrected, and vector normalized. Corrected spectra were processed with HCA analysis (Matlab software).
2.8. Preprocessing and processing of tissue spectral images
The recorded FTIR hyperspectral images of paraffin-embedded breast tissues exhibit both tissue biochemical information and paraffin bands (1378 cm−1 and 1467 cm−1) in the 900 to 1800 cm−1 spectral region. In our study, we did not perform a chemical dewaxing but images were digitally corrected for paraffin spectral contribution. This is achieved via an automated data processing method based on Extended Multiplicative Signal Correction (EMSC). The complete method has been previously described by Ly et al.37 For tissue classification, an unsupervised common k-means clustering method was used.38 In k-means, each spectrum belongs to a unique cluster and spectral images can be reconstructed for a rapid and simple visual analysis of clustering results. Apart from the cluster images, a dendrogram obtained by HCA showing the spectral distance between different k-means cluster centroids can be retrieved. All processed FTIR images were compared with adjacent HPS stained sections. Both EMSC and k-means clustering algorithms were implemented in Matlab Statistics Toolbox software.
2.9. Statistical analysis
Data were expressed as mean ± standard deviation (SD). The statistical difference between groups was assessed by Student's t-test and the Chi square test. P values <0.05 were considered to be statistically significant. All statistical analyses were performed by using SPSS 22.0 software (IBM Corporation, New York, USA).
3. Results and discussion
3.1. Clinical and pathological characteristics of non-IBC and IBC patients
Clinical and pathological characteristics of patients are described in Table 1. Statistical analysis showed that IBC patients were significantly younger with larger tumor sizes (p = 0.001 and 0.004, respectively) compared to non-IBC patients. Moreover, the status of lymph node metastasis and the incidence of lymphovascular invasion were significantly higher in IBC patients in comparison with non-IBC patients (p = 0.039 and 0.035, respectively).
Table 1 Clinical and pathological characteristics of non-IBC and IBC patients
Characteristic |
Non-IBC (n = 10) |
IBC (n = 10) |
P value |
Data are reported as means ± SD. Student's t-test. Chi square test. *Significant p value (p < 0.05). |
Age [year]
|
Range |
53–65 |
29–53 |
0.001*a |
Mean ± SD |
58.5 ± 4.1 |
42.1 ± 7.6 |
|
Tumor size [cm]
|
Mean ± SD |
3.4 ± 0.8 |
6 ± 2.3 |
0.004*b |
≤4 |
4 |
1 |
>4 |
6 |
9 |
|
Tumor grade
|
G1 |
2 |
0 |
0.289b |
G2 |
6 |
5 |
G3 |
2 |
4 |
G4 |
0 |
1 |
|
Axillary lymph node metastasis
|
0 |
4 |
0 |
0.039*b |
≤4 |
3 |
2 |
>4 |
3 |
8 |
|
Lymphovascular invasion
|
Negative |
3 |
8 |
0.035*b |
Positive |
7 |
2 |
3.2. Characterization of MDA-MB-231 and SUM-149 cell suspensions by high-throughput FTIR spectroscopy
Normalized mean FTIR spectra obtained from MDA-MB-231 and SUM-149 cells in suspension were compared. Visually, the spectral profiles of both cell types were quite similar in the 1800–800 cm−1 spectral range (Fig. 2A). In addition, the vector normalized second derivative spectra showed very minor modifications between both cell types in the 1200–800 cm−1 spectral range (saccharide absorption region) (Fig. 2B). The cell suspension spectra were analyzed by HCA. The computation was performed on all second derivative spectra (n = 8) for each cell suspension in the 1800–800 cm−1 spectral range. The results show that the eight replicate spectra of each cell suspension were grouped together in the same cluster and exhibited a high degree of homogeneity. This indicates a good reproducibility of the measurements and, therefore, a low intra-group variability. The inter-group variability was sufficiently high to distinguish the two cell types (Fig. 2C).
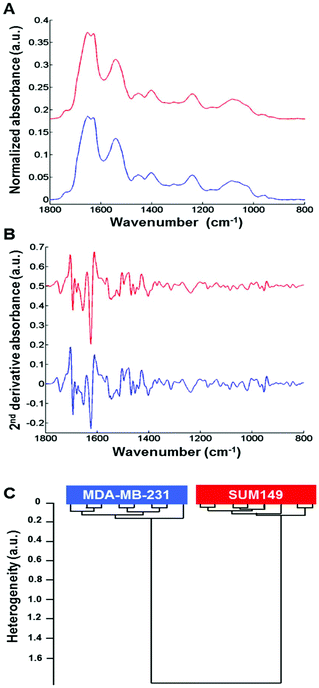 |
| Fig. 2 High-throughput FTIR spectra of MDA-MB-231 and SUM-149 cell suspensions. (A) Comparison between baseline corrected and normalized FTIR spectra obtained from MDA-MB-231 and SUM-149 cell suspensions in the spectral range of 1800–800 cm−1; (B) their second derivative counterparts; (C) HCA analysis of second derivative FTIR spectra. Spectra are offset for clarity. | |
3.3. Differentiating between non-IBC and IBC cells via fixed single cell FTIR imaging analysis
Fig. 3A shows on the left the white light images of MDA-MB-231 (top) and SUM-149 (bottom) single fixed cells and their corresponding FTIR images on the right. The mean spectrum of each cell was extracted from the dotted square, baseline corrected and vector normalized as displayed in Fig. 3B. The spectral profiles of both cell types were quite similar in the 1800–800 cm−1 spectral range. In order to enhance the differences, second derivative spectra were calculated and displayed some modifications between both cell types in the 1800–800 cm−1 spectral range as shown in Fig. 3C. HCA analysis was then performed on the second derivative spectra of each cell type in the 1800–800 cm−1 spectral range. The cluster analysis reveals two well distinct groups corresponding to each cell type (Fig. 3D). Furthermore, an intra-group low degree of heterogeneity and an inter-group high degree of heterogeneity were observed as with the cell suspension measurements. This separation was based on spectral differences in the following regions: 994–1072, 1096–1108, 1264–1352, 1386–1444, 1672–1692 and 1710–1743 cm−1. The assignment described in Table 2 is based on the previous work by Movasaghi et al.39 The effect of the media on the two cell lines was verified. MDA-MB-231 cells were grown in DMEM and HAM's F12 media and similarly for SUM-149 cells. HCA analyses show that the discrimination between the two cell lines is possible independent of the culture medium (see ESI, Figure ESI1†). In addition, their line morphology was observed in the two media. For both cell lines, the morphology was not affected by the medium (see ESI, Figure ESI2†). Finally, the cell growth kinetics of both cell lines in the two media demonstrate that SUM-149 cell growth is similar in DMEM and HAM's F12 media and a similar tendency is observed for the MDA-MB-231 cell line in the two media (see ESI, Figure ESI3†).
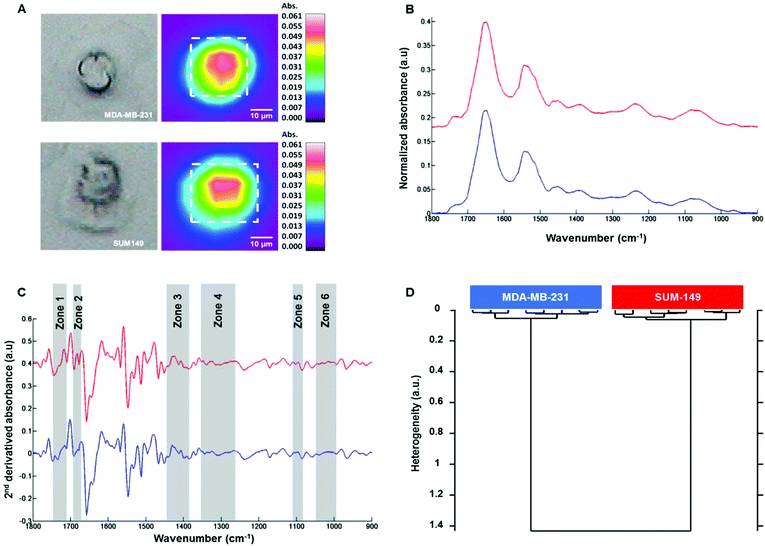 |
| Fig. 3 FTIR imaging of MDA-MB-231 and SUM-149 fixed single cells. (A) White light images of MDA-MB-231 (top left) and SUM-149 (bottom left) single fixed cells and their corresponding FTIR images on the right; (B) comparison between the mean spectrum of each cell (top: MDA-MB-231 and bottom: SUM-149) in the spectral range of 1800–800 cm−1 as extracted from the dotted square, then baseline corrected and vector normalized; (C) their corresponding second derivative spectra; (D) HCA analysis of second derivative FTIR spectra. Spectra are offset for clarity. | |
Table 2 Tentative assignment of FTIR bands based on the second derivative spectra of fixed MDA-MB-231 and SUM-149 cell types
Spectral range |
Frequency (cm−1) |
Assignment |
1 |
994–1072 |
RNA |
2 |
1096–1108 |
ν
as, νs CO–O–C, lipids |
3 |
1264–1352 |
Amide III, proteins |
4 |
1386–1444 |
ν COO−, δs CH3, lipids, proteins |
5 |
1672–1692 |
C O guanine deformation |
6 |
1710–1743 |
ν(C O), lipids (esters), νas (C O), RNA (esters) |
3.4. Characterization of MDA-MB-231 and SUM-149 live cells using Raman microspectroscopy
Raman microspectroscopy has the advantage of measuring single cells in 0.9% NaCl solution with very low interference from water molecules. The results described here were performed on live MDA-MB-231 and SUM-149 breast cancer cells. Data presented here are from cell cytoplasms (n = 10 cells; 5 spectra per cell for each cell line). Fig. 4A shows the comparison of the mean Raman spectra of MDA-MB-131 (top) and SUM-149 (bottom) cells. Several spectral differences can be observed in the regions of 688–992, 1019–1114, 1217–1375 and 1516–1625 cm−1 as depicted in Fig. 4A. The differences concern mainly proteins, nucleic acids and amino acids. The assignment described in Table 3 is based on the previous work by Movasaghi et al.40 HCA analysis was carried out on normalized spectra in the 600–1800 cm−1 spectral range and the results displayed in Fig. 4B show a good discrimination between non-IBC and IBC cells. For both HT-FTIR of cell populations and FTIR imaging of single fixed cells, a low intra-group variability was observed indicating a good reproducibility of the replicates. The inter-group variability was sufficiently high to delineate the two cell types.
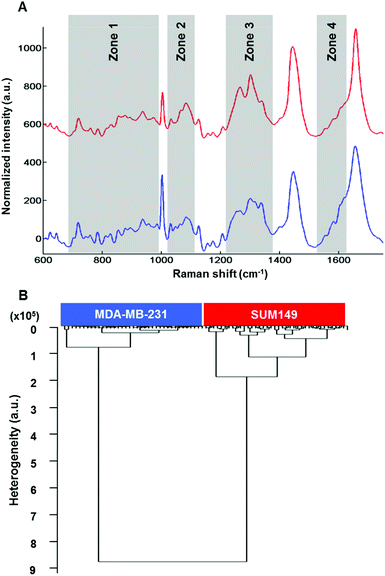 |
| Fig. 4 Raman microspectroscopy of MDA-MB-231 and SUM-149 single live cells. (A) Comparison between the mean spectrum of each cell (top: MDA-MB-231 and bottom: SUM-149) in the spectral range of 600–1800 cm−1, baseline corrected and vector normalized; (B) HCA analysis of Raman spectral data obtained from MDA-MB-231 and SUM-149 live cells in the spectral range of 1800–600 cm−1. Spectra are offset for clarity. | |
Table 3 Tentative assignment of Raman bands of live MDA-MB-231 and SUM-149 cell types
Spectral range |
Frequency (cm−1) |
Assignment |
1 |
688–992 |
DNA/RNA bases, tryptophan/tyrosine |
2 |
1000–1114 |
Phenylalanine, PO2− |
3 |
1217–1375 |
Amide III, lipids, nucleic acids (bases) |
4 |
1516–1625 |
C C bending mode |
Phenylalanine/tyrosine |
The intra-group and inter-group variabilities in single cells were comparable to those of the cell suspension data. Concerning Raman data, the intra-group variability in SUM-149 was found to be higher than that observed in FTIR analyses, where the cells were either dried or fixed. It can be hypothesised that the Raman data on live cells better reflect the heterogeneity within the cell population.
However, the two techniques provide different spatial resolutions and at the cellular level this can introduce a spectral variability.
Having demonstrated that non-IBC and IBC cells can be discriminated using vibrational spectroscopy, it would be interesting from a clinical perspective to evaluate the potential of the technique for differentiating non-IBC and IBC conditions at the tissue level.
3.5. Common k-means clustering of non-IBC and IBC tissue hyperspectral images
After digital dewaxing of the FTIR images of healthy and carcinoma breast tissue sections, a common k-means clustering was performed using 10 clusters. This method was applied to 20 breast cancer patients (10 non-IBC and 10 IBC) and 4 healthy volunteers who underwent breast reduction surgery. The reconstructed color-coded cluster images enabled the recovery of different histological features that allowed us to precisely localize breast tumors from other tissue components. An example of the k-means clustering results is shown in Fig. 5. Fig. 5A shows one healthy breast tissue on the left HPS-stained image and its corresponding k-means image on the right. In a similar way, Fig. 5B and C show two non-IBC and IBC cases, respectively. Annotation of each generated cluster was then performed with the help of a confirmed pathologist resulting in the following precise tissue characterization: breast carcinoma tissue was represented by clusters 2 and 10, breast adipose tissue by clusters 4 and 8, breast fibrous connective tissue by clusters 1, 5, 6, 7 and 9, and breast necrotic tissue by cluster 3. The outcome of k-means clustering can also be represented in the form of a dendrogram which shows the distance between the cluster centroids (Fig. 5D). All ten centroid spectra are shown in Fig. 5D.
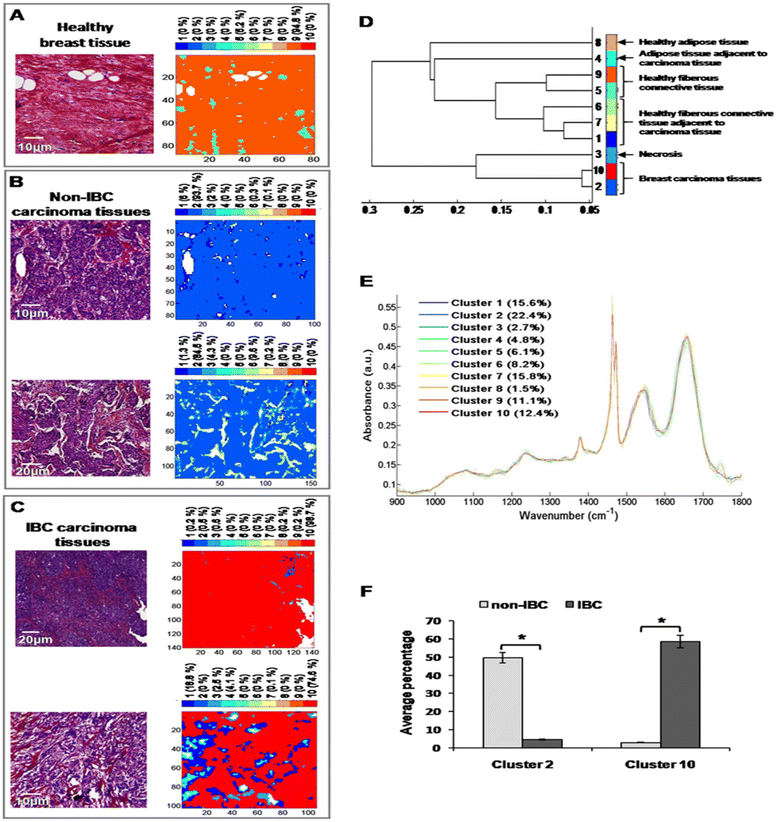 |
| Fig. 5 Common k-means clustering of FTIR images of non-IBC and IBC tissues. (A) Example of one healthy breast tissue: HPS stained image (left) and its corresponding k-means image (right); (B) example of two non-IBC cases: HPS-stained images (left) and their corresponding k-means images (right); (C) example of two IBC cases: HPS-stained images (left) and their corresponding k-means images (right); (D) dendrogram showing the grouping of ten clusters obtained after k-means; (E) comparison between ten centroid spectra; (F) histogram showing the average percentages of two clusters (2 and 10) representative of carcinoma in non-IBC and IBC tissues. *indicates a significant p value, as determined by the Chi square test. | |
Our results show that healthy adipose tissue and normal adipose tissue adjacent to carcinoma tissue obtained from both non-IBC and IBC patients were represented by two different clusters (cluster 8 and 4, respectively). Moreover, healthy and normal fibrous breast connective tissues adjacent to carcinoma tissues of both non-IBC and IBC patients were represented by five different clusters (clusters 5, 9 and 1, 6, 7, respectively) (Fig. 5D and E). An interesting finding is that the average percentage of carcinoma (cluster 2) was significantly higher in non-IBC (p < 0.05) compared to IBC carcinoma tissues. In contrast, the average percentage of carcinoma (cluster 10) was significantly higher in IBC (p < 0.05) compared to non-IBC carcinoma tissues. These results obtained from common k-means clustering showed that carcinoma tissues of non-IBC and IBC patients were represented by two different clusters (2 and 10, respectively) (Fig. 5F).
Vibrational microspectroscopy/imaging is an analytical tool with several obvious advantages in disease diagnosis. It has the ability to detect subtle molecular changes that precede any morphological changes. These observed spectroscopic changes are related to any modifications of the concentration and the conformational orientation of functional groups associated with proteins, lipids, nucleic acids and carbohydrates.41,42 The delay between diagnosis and treatment can be very long and distressing for patients and the cost is very substantial for health care. Vibrational spectroscopy, as a ‘spectral histological’ technique for cancer diagnosis and prognosis, can be used as a screening method to help histopathologists in their decision and thus shortening the delay time.43 Its potential for cell studies has been demonstrated for breast cancer cells. Recently, a strong correlation between gene expression patterns and FTIR spectral data has been demonstrated for thirteen breast cancer cell lines grown under 2D and 3D culture conditions.44 The same group has also shown that HTS-FTIR spectroscopy can classify numerous polyphenols according to the metabolic modifications they induced in the MDA-MB-231 cell line.45 Due to the late diagnosis of IBC, an accurate and rapid method is critically needed which will significantly improve the IBC outcome when implemented early enough.
In the present study, FTIR and Raman microspectroscopy and imaging were used for the first time to discriminate between non-IBC and IBC at the cell and tissue levels. Our results showed that both FTIR and Raman microspectroscopy were effective methods at the single cell level. However, since Raman measurements are amenable to live cell exploration, they reflect better the cell physiological status compared to fixed cell measurements. Recent studies have also demonstrated the potential of FTIR microspectroscopy for single live cell analysis. An increase in cellular level spatial resolution was achieved either by the high refractive index of a micro-ATR element46 or by the use of a synchrotron IR source.47 These advances are promising but require specific set-ups for live cell measurements with a proper control of the path length of the medium in which cells are measured. Thus, Raman microspectroscopy presents the advantages of a straightforward technique with subcellular spatial and axial resolutions. The FTIR imaging results obtained from breast carcinoma tissues after the application of the common k-means cluster algorithm showed that the reconstructed color-coded clustering images allowed the recovering of different histological structures, particularly to precisely localize tumoral areas and their normal counterparts. Furthermore, non-IBC and IBC carcinoma tissues, represented by two different clusters, could be differentiated and this is the first time that such results are reported. In contrast, we could not discriminate between non-IBC and IBC stromal breast tissues. In this case, it would be necessary to increase the spatial resolution and/or apply more advanced spectral image analysis methods. For instance, Patel and coll. used synchrotron-FTIR microspectroscopy to identify biomolecular changes associated with chronic oxidative stress in the mammary epithelium and stroma of breast tissues from healthy young women.48 Other modalities using FTIR imaging via a focal plane array detector could provide more insight into breast tissue analysis.49
4. Conclusions
In this study, we have applied vibrational spectroscopy and imaging to investigate non-inflammatory and inflammatory breast cancer at the cellular and tissue levels. IBC is one of the most lethal and fatal forms of breast cancer with a higher incidence in young women.
Differentiating between these two pathological conditions is crucial for patient therapy and management. Existing markers fail to explain the specific pathobiology of IBC and to distinguish IBC from non-IBC. Consequently, late diagnosis of IBC due to the absence of any biological markers is associated with a low survival rate compared to other types of breast cancer. Therefore, new approaches based on spectral analysis can be complementary to conventional methods based on immunohistochemistry. These methods have the advantages of being rapid, non-destructive, non-contact and label-free.
Our findings demonstrate that vibrational spectroscopy has the potential to discriminate between non-IBC and IBC at both the cell and tissue levels. This is an important aspect since inflammation is indicative of bad prognosis and its identification could be useful for patient therapy management.
Conflicts of interest
There are no conflicts to declare.
Acknowledgements
Financial support from the European Commission, H2020-MSCA-RISE-2014, funding scheme Marie Sklodovska-Curie, Research of Innovation Staff Exchange (RISE), GLYCANC ID 645756 is gratefully acknowledged. HTM is a recipient of this funding scheme. The authors thank the Reims PICT-IBiSA Platform for instrument facilities. Argyris Spyrou, PhD student, Uppsala University, Sweden, is acknowledged for his help in cell culture during the revision of the manuscript. Argyris Spyrou was a recipient of the French-Swedish Prize for young researchers funded by the French-Swedish Association for Research (AFSR).
References
- H. T. Mohamed, M. El-Shinawi, M. A. Nouh, A. R. Bashtar, E. T. Elsayed, R. J. Schneider and M. M. Mohamed, Front. Oncol., 2014, 4, 246 CrossRef PubMed.
- D. A.-R. Mona Mostafa Mohamed, S. F. Sabet and M. El-Shinawi, J. Adv. Res., 2013, 5, 12 Search PubMed.
- J. M. Walshe and S. M. Swain, Breast Dis., 2005, 22, 35–44 Search PubMed.
- F. M. Robertson, M. Bondy, W. Yang, H. Yamauchi, S. Wiggins, S. Kamrudin, S. Krishnamurthy, H. Le-Petross, L. Bidaut, A. N. Player, S. H. Barsky, W. A. Woodward, T. Buchholz, A. Lucci, N. T. Ueno and M. Cristofanilli, CA-Cancer J. Clin., 2010, 60, 351–375 CrossRef PubMed.
- F. Peters, A. Kießlich and V. Pahnke, Eur. J. Obstet. Gynecol. Reprod. Biol., 2002, 105, 59–63 CrossRef.
- S. B. Edge and C. C. Compton, Ann. Surg. Oncol., 2010, 17, 1471–1474 CrossRef PubMed.
- M. I. Nounou, F. ElAmrawy, N. Ahmed, K. Abdelraouf, S. Goda and H. Syed-Sha-Qhattal, Breast Cancer, 2015, 9s2, 17–34 Search PubMed.
- A. N. Tosteson, D. G. Fryback, C. S. Hammond, L. G. Hanna, M. R. Grove, M. Brown, Q. Wang, K. Lindfors and E. D. Pisano, JAMA Intern. Med., 2014, 174, 954–961 CrossRef PubMed.
- N. Ragu, P. Roger and P. Taourel, J. Radiol., 2004, 85, 2069–2070 CrossRef CAS PubMed.
- C. G. Kleer, K. L. van Golen, T. Braun and S. D. Merajver, Mod. Pathol., 2001, 14, 458–464 CrossRef CAS PubMed.
- Y. Gong, Semin. Oncol., 2008, 35, 33–40 CrossRef CAS PubMed.
- P. J. Kowalski, M. A. Rubin and C. G. Kleer, Breast Cancer Res., 2003, 5, R217–R222 CrossRef CAS PubMed.
- H. Yamauchi, W. A. Woodward, V. Valero, R. H. Alvarez, A. Lucci, T. A. Buchholz, T. Iwamoto, S. Krishnamurthy, W. Yang, J. M. Reuben, G. N. Hortobagyi and N. T. Ueno, Oncologist, 2012, 17, 891–899 CrossRef PubMed.
- F. Lerebours, G. Cizeron-Clairac, A. Susini, S. Vacher, E. Mouret-Fourme, C. Belichard, E. Brain, J. L. Alberini, F. Spyratos, R. Lidereau and I. Bieche, Int. J. Cancer, 2013, 133, 1614–1623 CrossRef CAS PubMed.
- C. Kendall, M. Isabelle, F. Bazant-Hegemark, J. Hutchings, L. Orr, J. Babrah, R. Baker and N. Stone, Analyst, 2009, 134, 1029–1045 RSC.
- L. Quaroni and T. Zlateva, Analyst, 2011, 136, 3219–3232 RSC.
- A. D. Meade, O. Howe, V. Unterreiner, G. D. Sockalingum, H. J. Byrne and F. M. Lyng, Faraday Discuss., 2016, 187, 213–234 RSC.
- J. Nallala, M. D. Diebold, C. Gobinet, O. Bouche, G. D. Sockalingum, O. Piot and M. Manfait, Analyst, 2014, 139, 4005–4015 RSC.
- H. T. Mohamed, V. Untereiner, G. D. Sockalingum and S. Brezillon, Glycoconjugate J., 2017, 34, 309–323 CrossRef CAS PubMed.
- D. Sebiskveradze, V. Vrabie, P. Jeannesson, O. Piot and M. Manfait, Diagn. Pathol., 2013, 8, S33 CrossRef.
- P. Lasch, W. Haensch, D. Naumann and M. Diem, Biochim. Biophys. Acta, 2004, 1688, 176–186 CrossRef CAS PubMed.
- G. Bellisola and C. Sorio, Am. J. Cancer Res., 2012, 2, 1–21 CAS.
- C. Pezzei, J. D. Pallua, G. Schaefer, C. Seifarth, V. Huck-Pezzei, L. K. Bittner, H. Klocker, G. Bartsch, G. K. Bonn and C. W. Huck, Mol. BioSyst., 2010, 6, 2287–2295 RSC.
- D. Sebiskveradze, V. Vrabie, C. Gobinet, A. Durlach, P. Bernard, E. Ly, M. Manfait, P. Jeannesson and O. Piot, Lab. Invest., 2011, 91, 799–811 CrossRef CAS PubMed.
- U. Zelig, E. Barlev, O. Bar, I. Gross, F. Flomen, S. Mordechai, J. Kapelushnik, I. Nathan, H. Kashtan, N. Wasserberg and O. Madhala-Givon, BMC Cancer, 2015, 15, 408 CrossRef PubMed.
- S. Kumar, T. S. Shabi and E. Goormaghtigh, PLoS One, 2014, 9, e111137 CrossRef PubMed.
- M. H. Ali, F. Rakib, K. Al-Saad, R. Al-Saady, F. M. Lyng and E. Goormaghtigh, J. Mol. Struct., 2018, 1163, 472–479 CrossRef CAS.
- A. Mignolet, B. R. Wood and E. Goormaghtigh, Analyst, 2018, 143, 258–269 RSC.
- S. Kumar, C. Desmedt, D. Larsimont, C. Sotiriou and E. Goormaghtigh, Analyst, 2013, 138, 4058–4065 RSC.
- A. S. Haka, Z. Volynskaya, J. A. Gardecki, J. Nazemi, R. Shenk, N. Wang, R. R. Dasari, M. Fitzmaurice and M. S. Feld, J. Biomed. Opt., 2009, 14, 054023 CrossRef PubMed.
- Y. Yang, F. Li, L. Gao, Z. Wang, M. J. Thrall, S. S. Shen, K. K. Wong and S. T. Wong, Biomed. Opt. Express, 2011, 2, 2160–2174 CrossRef PubMed.
- K. Kong, F. Zaabar, E. Rakha, I. Ellis, A. Koloydenko and I. Notingher, Phys. Med. Biol., 2014, 59, 6141–6152 CrossRef PubMed.
- T. Bhattacharjee, L. C. Fontana, L. Raniero and J. Ferreira-Strixino, J. Raman Spectrosc., 2018, 49, 786–791 CrossRef CAS.
- C. Zhang, P. T. Winnard Jr., S. Dasari, S. L. Kominsky, M. Doucet, S. Jayaraman, V. Raman and I. Barman, Chem. Sci., 2018, 9, 743–753 RSC.
- M. A. Nouh, M. M. Mohamed, M. El-Shinawi, M. A. Shaalan, D. Cavallo-Medved, H. M. Khaled and B. F. Sloane, J. Transl. Med., 2011, 9, 1 CrossRef CAS PubMed.
- F. Draux, C. Gobinet, J. Sule-Suso, A. Trussardi, M. Manfait, P. Jeannesson and G. D. Sockalingum, Anal. Bioanal. Chem., 2010, 397, 2727–2737 CrossRef CAS PubMed.
- E. Ly, O. Piot, R. Wolthuis, A. Durlach, P. Bernard and M. Manfait, Analyst, 2008, 133, 197–205 RSC.
- T. N. Nguyen, P. Jeannesson, A. Groh, O. Piot, D. Guenot and C. Gobinet, J. Biophotonics, 2016, 9, 521–532 CrossRef PubMed.
- Z. Movasaghi, S. Rehman and D. I. ur Rehman, Appl. Spectrosc. Rev., 2008, 43, 134–179 CrossRef CAS.
- Z. Movasaghi, S. Rehman and I. U. Rehman, Appl. Spectrosc. Rev., 2007, 42, 493–541 CrossRef CAS.
- M. J. Baker, J. l. Trevisan, P. Bassan, R. Bhargava, H. J. Butler, K. M. Dorling, P. R. Fielden, S. W. Fogarty, N. J. Fullwood and K. A. Heys, Nat. Protoc., 2014, 9, 1771 CrossRef CAS PubMed.
- H. J. Butler, L. Ashton, B. Bird, G. Cinque, K. Curtis, J. Dorney, K. Esmonde-White, N. J. Fullwood, B. Gardner and P. L. Martin-Hirsch, Nat. Protoc., 2016, 11, 664–687 CrossRef CAS PubMed.
- H. Barr, O. Old, M. Almond, C. Kendall, G. Rhys-Lloyd, G. Shetty, J. Hutchings and N. Stone, Mater. Today, 2015, 2, 890–893 CrossRef.
- M. Smolina and E. Goormaghtigh, Analyst, 2018, 143, 2520–2530 RSC.
- A. Mignolet, V. Mathieu and E. Goormaghtigh, Analyst, 2017, 142, 1244–1257 RSC.
- K. L. A. Chan and S. G. Kazarian, Chem. Soc. Rev., 2016, 45, 1850–1864 RSC.
- L. Quaroni, T. Zlateva, B. Sarafimov, H. W. Kreuzer, K. Wehbe, E. L. Hegg and G. Cinque, Biophys. Chem., 2014, 189, 40–48 CrossRef CAS PubMed.
- I. I. Patel, D. A. Shearer, S. W. Fogarty, N. J. Fullwood, L. Quaroni, F. L. Martin and J. Weisz, Cancer Biol. Ther., 2014, 15, 225–235 CrossRef CAS PubMed.
- I. Zawlik, E. Kaznowska, J. Cebulski, M. Kolodziej, J. Depciuch, J. Vongsvivut and M. Cholewa, Sci. Rep., 2016, 6, 37333 CrossRef CAS PubMed.
Footnotes |
† Electronic supplementary information (ESI) available. See DOI: 10.1039/c8an01292j |
‡ These authors contributed equally to this work. |
§ These authors contributed equally to this work and project supervision. |
|
This journal is © The Royal Society of Chemistry 2018 |
Click here to see how this site uses Cookies. View our privacy policy here.