DOI:
10.1039/C3RA46861E
(Paper)
RSC Adv., 2014,
4, 6702-6709
Exploring structural requirements of leads for improving activity and selectivity against CDK5/p25 in Alzheimer's disease: an in silico approach†
Received
20th November 2013
, Accepted 2nd January 2014
First published on 6th January 2014
Abstract
A congeneric series of 224 cyclin-dependant kinase 5/p25 (CDK5/p25) inhibitors was exploited to understand the structural requirements for improving activity against CDK5/p25 and selectivity over CDK2. The CDK5/p25 enzyme complex plays a significant role in the formation of neurofibrillary tangles in Alzheimer's disease. In the present study, 2D-quantitative structure–activity relationship (2D-QSAR), group or fragment based QSAR (G-QSAR), and quantitative activity–activity relationship (QAAR) models were developed and validated with satisfactory performance as evidenced from statistical metrics, indicating the reliability and robustness of the models. The 2D-QSAR and G-QSAR models explore the structural requirements for improving activity, while the QAAR model facilitates the better understanding of features required for selectivity of the inhibitors. The docking study further provides information regarding the key active site residues and structural features important for proper binding in the active site of the CDK5/p25 complex.
1. Introduction
Alzheimer's disease (AD) is a lethal neurological disorder with neurobehavioral associated symptoms due to progressive degeneration of various parts of the brain. It gradually destroys learning skills, thinking, memory and finally the ability to carry out basic activities of daily living (ADLs). The root cause as well as treatment of AD is unknown, while several hypotheses exist such as the cholinergic hypothesis, amyloid hypothesis, tau hypothesis etc. These hypotheses are based on the two major hallmarks of AD that are hyperphosphorylation of the tau protein (leading to formation of neurofibrillary tangles) and amyloid plaques production.1 The hyperphosphorylation of the tau proteins is a result of deregulation in several protein kinases such as cyclin-dependant kinase 5 (CDK5),2 casein kinase 1 (CK1),3 glycogen synthase kinase 3 (GSK3)4 etc. Thus, these kinases are a promising class of potential targets for the treatment of AD.
Cyclin-dependant kinases (CDKs) are the group of enzymes that control the events of the cell cycle and also participate in apoptosis. The main biological function of CDKs is cell cycle regulation; however certain CDKs (i.e. CDK5) are involved in controlling cell differentiation in neuronal cells instead of cell cycle control. CDK5 is expressed in post-mitotic cells of the central nervous system and it plays a vital role during neuronal differentiation. It is a unique member of the family since it requires association with p35 activator for activation instead of cyclins.5,6 The CDK5 activity is restricted to neurons, since the activator p35 is neuronal-specific. The deregulation of CDK5 activity has been concerned in several neurogenerative diseases including AD. This deregulation is induced by the proteolytic cleavage of p35 by calpain, a calcium-dependent cysteine protease, forming the active fragment p25, which is observed to be accumulated in the brain of AD patients.7 It is believed, although the precise mechanism is unclear, that the catalytic activity of CDK5/p25 is significantly higher than the CDK5/p35, and this hyperactive CDK5/p25 complex causing hyperphosphorylation of tau protein is considered to be responsible in neuronal cell toxicity.8
One of the imposing challenges in designing drugs against any kinase is selectivity. In this case, achieving selectivity against CDK5 is an important issue. The selectivity issue of CDK5 inhibitors over CDK2 has been reported in the literature.9–11
The quantitative structure–activity relationship (QSAR), group-based QSAR (G-QSAR) and quantitative activity–activity relationship (QAAR) techniques are very useful for exploring variations in the properties and its relationship with activity/selectivity of the compounds. These techniques are widely used to predict activity/selectivity of unknown compounds, once the relationship is well defined in terms of mathematical equation and validated by means of various validation parameters.
In this study, 2D-QSAR, G-QSAR and QAAR techniques are performed on a series of CDK5/p25 inhibitors to explore structural requirements for CDK5/p25 inhibition considering both the issues i.e. activity against CDK5/p25 as well as selectivity over CDK2. The structural features required for binding to CDK5 specifically was studied using molecular docking approach.
2. Materials and methods
2.1 Dataset
The activity values of the congeneric series of 224 4-amino-5-methyl-4H-1,2,4-triazole-3-thiol derivatives against CDK5/p25 were collected from the literature10,11 (all 224 structures are provided as a .sdf file in ESI†). These values were measured using the same experimental procedure (scintillation proximity assay) by the same research group (Shiradkar et al.) for all the compounds. The activity values of all the compounds expressed as IC50 values (nM) were converted to pIC50 values (nM) for 2D-QSAR, G-QSAR and QAAR studies. For QAAR studies, 18 out of 224 compounds were employed whose activity values against CDK2 were reported (all 18 structures are provided separately as a .sdf file in ESI†). The difference of CDK5 and CDK2 pIC50 (nM) values were considered as the dependent variable for the development of the QAAR model. All the structures were drawn using ChemDraw Ultra software version 5.0.12 In case of G-QSAR modeling, the fragmentation of the molecules is a prerequisite step to designate substitution sites. The common scaffold and the substitution sites are shown in Fig. 1. R1, R2 and R3 are the substitution sites of the congeneric series under study.
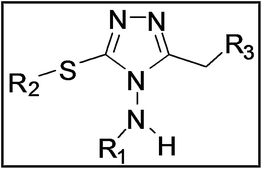 |
| Fig. 1 Common scaffold and the substitution sites employed in the G-QSAR study. | |
2.2 Descriptor calculation
For developing the 2D-QSAR model, a pool of 387 descriptors was calculated using Cerius 2 version 4.10,13 PaDEL-Descriptor version 2.11 (ref. 14) and Dragon 6 software.15 Different classes of descriptors were calculated which include electrotopological state keys, electronic, spatial, structural, thermodynamic, topological, constitutional, functional group counts and extended topochemical atom (ETA) indices. In G-QSAR, a relationship between activity and descriptor calculated for each molecular fragment at assigned substitution sites (R1, R2 and R3) is derived. Therefore, a total of 318 descriptors were calculated for all three groups/substitution sites using VLifeMDS version 3,16 which consist of physiochemical, atom-type based and interaction descriptors. All descriptors including those computed for 2D-QSAR as well as G-QSAR were employed for the QAAR study. After calculating respective descriptors, data pre-treatment was done to remove constant (variance < 0.0001) and inter-correlated (r = 1) descriptors using a software tool (DTC_dataPreTreatment) developed by us and available at http://dtclab.webs.com/software-tools.
2.3 Dataset splitting
The dataset for 2D-QSAR was divided into 160 training and 64 test set compounds based on k-means clustering method17 using SPSS 9.0.18 The k-means clustering technique assists to classify similar compounds into a cluster. The test set compounds were then selected from each cluster randomly. In this way, the training and test sets consist of the entire chemical diversity present in the dataset. Same division of the data set was utilized for G-QSAR. Since a limited number of compounds were available for the QAAR study, all 18 compounds were employed in the training set for model development.
2.4 Development of models
In the present study, three different types of models were built: (a) 2D-QSAR, to develop relationship between activity and molecular properties i.e. descriptors, (b) G-QSAR, to develop relationship between activity and descriptors calculated for each molecular fragment at assigned substitution sites, (c) QAAR, to develop relationship between selectivity of CDK5 inhibitors over CDK2 and descriptors. Here, we have built models exploiting different chemometric techniques like stepwise multiple linear regression (stepwise MLR), partial least squares (PLS),19 genetic function approximation (GFA),20 GFA spline, genetic PLS (G/PLS) using Cerius 2 version 4.10. For validation of the models, different statistical metrics were employed, which includes internal, external, overall validation parameters and Y-randomization test using online software tool partially developed by the present group and available at http://aptsoftware.co.in/DTCMLRWeb/index.jsp. The validation parameters computed to check the quality of models were correlation coefficient (R2), adjusted R2(Ra2), standard error of estimate (SEE), leave-one-out cross-validated correlation coefficient (Q2), both scaled and unscaled versions of novel rm2 metrics21 such as
and Δrm2(training) for internal validation,
and Δrm2(test) for external validation,
and Δrm2(overall) for overall validation, Rpred2 (external validation), and cRp2 (ref. 22) based on Y-randomization results. In Y-randomization test, the activity values of the training set compounds were randomly shuffled keeping the descriptor matrix unchanged and new models were built based on the shuffled activity values. For a robust model, the squared average correlation coefficient of the randomized models (average Rr2) must be significantly lower than R2 of the non-random (original) model and also cRp2 must be greater than 0.5. To verify the robustness and predictivity of the QAAR model, more stringent Leave-Many-Out (LMO) cross validation was performed on the training set. All three models were also evaluated for model acceptability criteria's suggested by Golbraikh and Tropsha.23,24 According to the acceptance criteria, a model must follow the following conditions:
(i) Q2 > 0.5
(ii) r2 > 0.6
(iii) |r02 − r′02| < 0.3
(iv) (r2 − r02)/r2 < 0.1 and 0.85 ≤ k ≤ 1.15 or (r2 − r′02)/r2 < 0.1 and 0.85 ≤ k′ ≤ 1.15
2.5 Molecular docking analysis
Docking analysis was performed to predict the binding orientation and interaction patterns of the CDK5 inhibitors which may possibly play an important role in understanding the activity and selectivity profiles of the inhibitors. The docking was carried out using GLIDE 6 module in Maestro Software 9.3.25 The 3-D crystal structure of CDK5/p25 complex with 1.9 resolution was downloaded from RCSB Protein Data Bank (PDB id: 4AU8).26 The protein preparation of the downloaded PDB was carried out using Protein Preparation Wizard to correct errors like steric clashes, missing loops, missing atom names, picking alternate conformations which may be present in the protein crystallographic data. Hydrogen atoms were added to the crystal structure, water molecules were removed except in the active site and the protein was minimized using OPLS2005 force field. The 224 CDK inhibitors were prepared using LigPrep module. Co-crystal ligand was isolated from the protein and prepared, since it was used to set the docking protocol. For ligand preparation, the 3D structures of all the compounds (2D structures were converted to 3D using OpenBabel software27) were processed to correct their protonated state and all possible conformation of each compound was generated. For setting the docking protocol, the grid box was generated around the co-crystal ligand with size of 15 Å and other default parameters were employed. Standard Precision (SP) scoring mode and all other default settings were used for carrying out docking. An additional docking validation was also performed to verify the set protocol by carrying out docking of a known ligand (co-crystal ligand, PDB id: 1UNH) with reported interactions28 with same protein i.e. CDK5/p25 complex. The set protocol was then followed to perform docking on 224 CDK5 inhibitors.
3. Result and discussion
3.1 QSAR models
The statistically significant models for 2D-QSAR, G-QSAR and QAAR models were obtained using GFA linear technique, which are mentioned below (eqn (1)–(3)) and the values of the corresponding validation metrics for respective models are summarized in Table S1 (provided as ESI†).
2D-QSAR model. |
pICCDK550 nM = 7.65 (±0.0969) + 0.504 (±0.371)S_aasN + 0.518 (±0.06)Atype_N_69 + 0.0017 (±0.00057)D/Dtr05 − 2.465 (±0.537)Atype_C_43 + 0.026 (±0.0051)S_sCl
| (1) |
Ntraining = 160; R2 = 0.726, Ra2 = 0.717, SEE = 0.255, Q2 = 0.700, SDEP = 0.262,
, Δrm2(training) = 0.207;Ntest = 64; Rpred2 = 0.675,
, Δrm2(test) = 0.168,
, Δrm2(overall) = 0.197.
Eqn (1) represents the best 2D-QSAR model obtained using GFA linear technique and the selected descriptors suggested different structural requirements for improving activity. The standard errors of regression coefficients are shown within parentheses. Ntraining and Ntest are the number of compounds in training and test sets which were used to develop the model and to validate the developed model, respectively. The predictive ability of the 2D-QSAR model was found to be significant, since Q2 = 0.700 (Leave-One-Out method), Rpred2 = 0.675,
, and Δrm2(test) = 0.168. All other validation parameters were also found to be with statistically significant values confirming the reliability of the model. The Y-randomization results (average Rr2 = 0.029, and average Qr2 = −0.046 for 50 randomly generated models) showed that the developed model is robust with cRp2 = 0.712. The model also passes the Golbraikh and Tropsha model acceptance criteria (all respective parameters are shown in Table 1).
Table 1 Results of 2D-QSAR and G-QSAR model according to Golbraikh and Tropsha'srecommended criteria
Parameters |
2D-QSAR model |
G-QSAR model |
Remarks |
Q2 |
0.70 |
0.686 |
Passed |
r2 |
0.677 |
0.696 |
Passed |
|r02 − r′02| |
0.1024 |
0.099 |
Passed |
(r2 − r02)/r2 and k or (r2 − r′02)/r2 and k′ |
0.004 and 1.001 or 0.154 and 0.997 |
0.0015 and 0.99 or 0.144 and 0.99 |
Passed |
G-QSAR model. |
pICCDK550 nM = 6.621 (±0.1009) + 0.136 (±0.058)R3_SsClE_index − 16.22 (±0.91)R2_chi5chain + 0.469 (±0.065)R2_SsNH2count − 0.126 (±0.033)R1_k1alpha + 1.376 (±0.276)R1_chi3Cluster
| (2) |
Ntraining = 160; R2 = 0.716, Ra2 = 0.706, SEE = 0.260, Q2 = 0.686, SDEP = 0.268,
, Δrm2(training) = 0.214;Ntest = 64; Rpred2 = 0.695,
, Δrm2(test) = 0.188,
, Δrm2(overall) = 0.207.
Eqn (2) represents the best G-QSAR model achieved using the GFA linear technique and it consists of descriptors which suggested structural requirement at specific locations (R1, R2 and R3 positions shown in Fig. 1) in the molecules to improve the activity. The predictive ability of G-QSAR model was also found to be significant based on Q2 = 0.686, Rpred2 = 0.695,
, and Δrm2(test) = 0.188 values. All other validation parameters along with Y-randomization test results (average Rr2 = 0.033, average Qr2 = −0.044 for 50 randomly generated models, cRp2 = 0.70) were found to be statistically significant confirming the reliability of the model. Like 2D-QSAR model, this model also passes the Golbraikh and Tropsha model acceptance criteria (Table 1).
QAAR model. |
pICCDK5–CDK250 nM = −7.133 (±1.24) + 3.8522 (±0.419)Jurs_FPSA_2 − 4.39 (±0.549)R2_chi3Cluster + 5.65 (±1.43)R3_ElectronegativityCount
| (3) |
Ntraining = 18; R2 = 0.860, Ra2 = 0.830, SEE = 0.246, Q2 = 0.787, SDEP = 0.268,
, Δrm2(training) = 0.102.Eqn (3) corresponds to the best QAAR model achieved using the GFA linear technique. This model helps in understanding the structural requirements to attain or improve selectivity over CDK2. The internal parameters such as Q2 = 0.790,
, Δrm2(training) = 0.102 and LMO results (as shown in Table 2) indicate the better predictive potential of the developed QAAR model. The Y randomization results (average Rr2 = 0.173, average Qr2 = −0.405 for 50 randomly generated models, cRp2 = 0.78) further confirm the robustness of the QAAR model.
Table 2 Leave-Many-Out (LMO) cross-validation results for the QAAR model
Na |
Q2 |
SDEP |
N – number of compounds left in each cycle. |
2 |
0.772 |
0.276 |
3 |
0.789 |
0.265 |
4 |
0.762 |
0.282 |
5 |
0.800 |
0.259 |
6 |
0.698 |
0.318 |
The contributions of descriptors found in 2D-QSAR, G-QSAR and QAAR models (eqn (1)–(3)) are discussed below and compared with docking results for better explanation.
3.2 Docking studies
Molecular docking studies were performed to find essential active site residues playing role in activity and/or selectivity of the compounds against CDK5/p25 enzyme (PDB id: 4AU8). It also explores the orientation of the inhibitors and interaction patterns, which suggest the role of various functional groups present. The RMSD value of co-crystal ligand after re-docking was found to be less than 1 (i.e., 0.435) and it also reproduced the interaction present in the co-crystal protein (PDB id: 4AU8). The additional validation test which involved docking of a known ligand (co-crystal ligand, PDB id: 1UNH) with CDK/p25 complex also gives satisfactory results, since the top docking pose of this ligand has shown same reported interactions28 (a snapshot shown in Fig. 2). Thus, the same docking protocol was used to dock the 224 CDK5/p25 inhibitors. Docking studies revealed that the CDK5 inhibitors were found to be interacting with the active site residues like Cys 83, Asp 84, Lys 89, Ile 10, Glu 12, Glu 81, Lys 20, Lys 88, Phe 80, Lys 9, Lys 89, Gln 130, Glu 8, Asp 144, Asn 131, Phe 82, Gln 85.
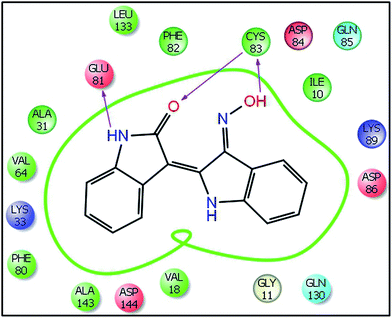 |
| Fig. 2 Top docking pose of a known ligand (co-crystal ligand, PDB id: 1UNH) used for validation of the set docking protocol (with Glide gscore = −8.333). | |
3.3 Discussion on contribution of descriptors of the 2D-QSAR and G-QSAR models to the activity
The contributions of descriptors selected in G-QSAR and 2D-QSAR models are discussed here and wherever possible, we have tried to justify it using the docking results. The compound names, activity values (observed, calculated and LOO predicted) and docking scores are provided in ESI (Tables S2–S4†).
R3-SsClE-index (G-QSAR model) is an electrotopological state index, which carries information concerning the topology of an atom and the electronic interactions due to all other atoms in the molecule. This descriptor shows a positive contribution to the activity. Here, R3-SsClE-index corresponds to the number of chlorine connected with one single bond at position R3. It suggests that a chlorine atom present at R3 position improves the activity. It is in accordance with the S_sCl descriptor (2D-QSAR model), also a type of electrotopological state indices, which designates sum of electrotopological state values of chlorine atoms with single bond. This can be observed in compounds C7a and C7b, where decrease in the value of R3-SsClE-index due to removal of chlorine at R3 results in decrease in activity as well as docking score (Fig. 3).
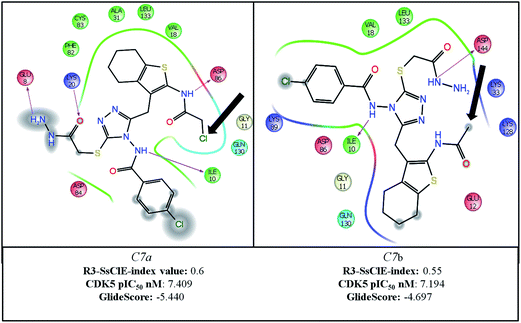 |
| Fig. 3 The best docked conformations of the compound C7a and C7b within the binding sites of the CDK5 enzyme. The black arrow points the site of substitution being discussed. (Snapshot taken using Maestro Software 9.3.) | |
The R2-Chi5chain (G-QSAR model) descriptor indicates a retention index for 5 member ring at position R2. This descriptor shows a negative and highest contribution to the activity. All the compounds having 5 member 1,2,4-triazole ring present at R2 position shows poor activity (pIC50 nM < 6). Hence, this descriptor effectively differentiates all the compounds with pIC50 < 6. It is in accordance with the descriptor D/Dtr05 (2D-QSAR model), which is a distance/detour ring index of order 5. The docking analysis showed that presence of five member ring affects interactions and hence docking score. This can be observed in compounds C9e and C10e as shown in Fig. 4.
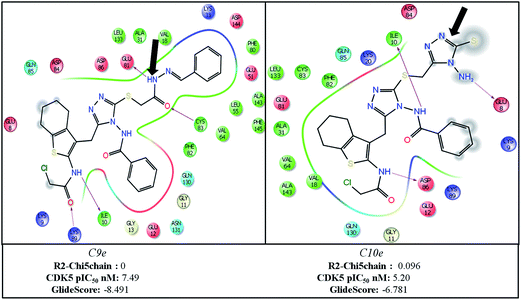 |
| Fig. 4 The best docked conformations of the compound C9e and C10e within the binding sites of the CDK5 enzyme. | |
The R2-SsNH2count (G-QSAR model) is an electrotopological state index descriptor showing positive contribution to the activity. It defines the total number of –NH2 groups connected with one single bond at R2 position. It effectively differentiates, along with the R2-Chi5chain descriptor, compounds in active and less active (pIC50 nM < 6). Compounds (e.g., B6a) with –NH2 group, but without 5 membered 1,2,4-triazole ring at R2 position shows higher activity and those compounds having –NH2 group along with 5 membered 1,2,4-triazole ring (e.g., B9a) or without –NH2 group (e.g., B4a) show less activity. It is in accordance with the descriptor Atype_N_69 (2D-QSAR model), which designates the presence of atom type ‘N’ as seen in these fragments: Ar–NH2 and X–NH2 (‘Ar’ represents aromatic groups and ‘X’ represents any heteroatoms). From the docking studies, it is observed that –NH2 group plays role in hydrogen bonding with Asp 144, Gln 130 (active site residues). In presence of the ring structure, these interactions may get affected. For example, changes in the interactions, docking score and activity values with changes in R2 group are seen in compounds B4a, B9a and B6a (Fig. 5).
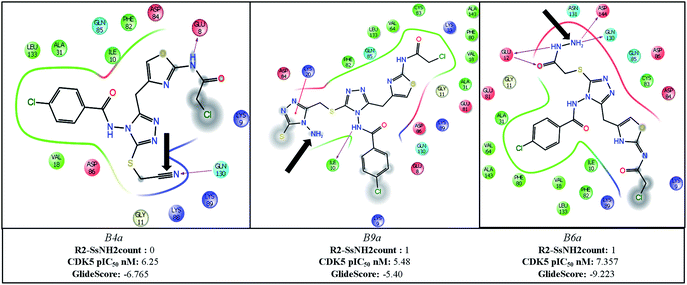 |
| Fig. 5 The best docked conformations of the compound B4a, B9a, and B6a within the binding sites of the CDK5/p25 enzyme complex. | |
The R1-chi3Cluster (G-QSAR model) descriptor designates the amount of branching, ring structure present and flexibility at position R1. It shows a positive contribution to the CDK5 activity suggesting that the presence of ring structure or branching at R1 contributes positively to the activity. This can be exemplified in case of compound C9i, when the phenyl group is replaced with methyl group (C9e) at R1 position, the activity value decreases from 7.494 to 6.473 (pIC50 nM). The R1-k1alpha (G-QSAR model) descriptor signifies first alpha modified shape index. It shows a negative contribution to the activity. The contribution of this descriptor to the response is marginal. Atype_C_43 (2D-QSAR study) descriptor indicates number of carbon atoms of specified type, i.e., X--CR+++X (‘X’ represents any heteroatom, R represents any group linked through carbon, ‘--' represents aromatic bonds as in benzene or delocalized bonds such as the N–O bond in a nitro group and ‘+++’ represents aromatic single bonds). It shows a negative and highest contribution to the activity according to the 2D-QSAR model. It effectively differentiates compounds into active and less active (pIC50 nM < 6) classes with values 1 and 2 respectively. S_aasN (2D-QSAR model) is a type of electrotopological state index, which shows a positive contribution to the activity. Here, S_aasN indicates nitrogen with two aromatic and a single bonds. The contribution is marginal showing an increase in activity with increase in S_aasN value upto 2. Further increase shows decrease in activity as observed in case of compounds (C10a–C10l) bearing five membered 1,2,4-triazole ring at R2 position which leads to decrease in activity (negative contribution of R2-Chi5chain descriptor).
3.4 Contribution of descriptors of the QAAR model to selectivity
The Jurs-FPSA-2 descriptor signifies the fractional positively charged partial surface area. It shows a positive contribution to the selectivity, which indicates that molecules with larger partial positive surface areas and larger partial positive charges will show higher selectivity. This can be exemplified by comparing Jurs-FPSA-2 and selectivity values of compounds B8a and B8b. The increase in Jurs-FPSA-2, due to removal of chlorine group at R3 position, results in increase in selectivity value from 0.085 to 1.232.
The R2-chi3Cluster descriptor designates the amount of branching and ring structure present and flexibility at position R2. It shows a negative contribution to the selectivity. Here it can be seen that if a ring structure, or higher degree of branching is present at position R2, it increases chi3Cluster value and decreases selectivity. In the docking studies, it is seen that presence of such structures affects interaction with Asp 144, Glu 12 and Gln 130 residues. It can be concluded that an increase in branching or presence of ring structure at R2 position decreases selectivity (example shown in Fig. 6).
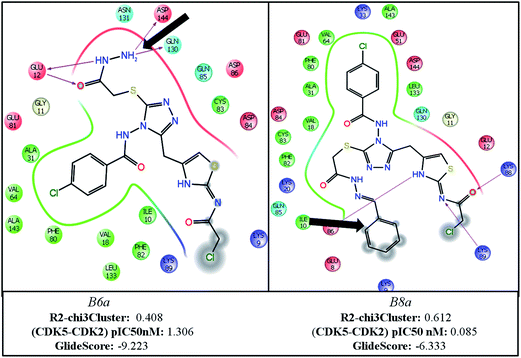 |
| Fig. 6 The best docked conformations of the compound B6a and B8a within the binding sites of the CDK5/p25 enzyme complex. | |
The R3-ElectronegativityCount descriptor is a measure of electronegativity. It shows a positive contribution to the selectivity. It is observed that even a little change in descriptor value affects selectivity. Removal of chlorine at R3 group reduces R3-ElectronegativityCount value from 0.597 to 0.55, resulting in decrease in selectivity (from 1.312 to 0.963) as well as activity (suggested by positive contribution of R3-SsClE-index descriptor selected in the G-QSAR model) and docking score (example as shown in Fig. 3).
In total, there are 224 structures and it was not easy to draw any conclusion regarding structure–activity relationships (SAR) from visual inspection of 2D structures and experimental activities. From the results of QSAR studies, the understanding of SAR of this series of compounds becomes clearer, and specific conclusions can be made. Once the SAR is interpreted from the QSAR models, it is then confirmed with docking studies. We may not focus on the specific features and their interaction patterns directly from docking studies without prior information about SAR. Also the reported QSAR models well explain the SAR and they pass all the requisite validation criteria, which prove their predictive ability and hence they are useful for predicting activity/selectivity of similar class of compounds.
4. Conclusions
This study presents three QSAR models (2D-QSAR, G-QSAR and QAAR) and molecular docking studies for investigating structural requirements to attain improved activity as well as selectivity against CDK5/p25. The best QSAR models were evolved from the GFA linear technique and were validated by means of internal, external and overall validation tests. All the dataset compounds were docked in the active site of the CDK5/p25 enzyme (PDB id: 4AU8) using the protocol, which was set by docking the co-crystal ligand. After considering all the developed models and docking analyses, it can be concluded that (see Fig. 7):
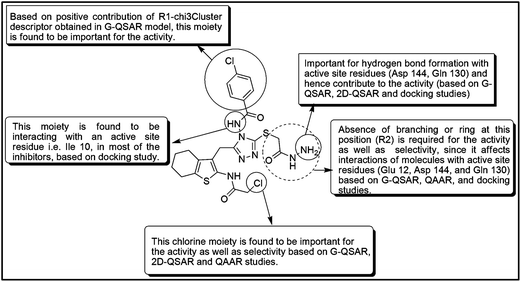 |
| Fig. 7 Summary of the mechanistic interpretation of 2D-QSAR, G-QSAR, QAAR and docking studies for CDK5/p25 inhibitors using compound 7a (one of the most active compounds). | |
(1) Presence of branching or ring structure at R2 position affects the activity as well as selectivity, since it hinders interactions of molecules with active site residues (Glu 12, Asp 144, and Gln 130). This conclusion is based on G-QSAR, QAAR, and docking studies.
(2) Presence of –NH2 group at R2 position is important for the activity, since it plays a significant role in interaction with active site residues (Asp 144, and Gln 130); this conclusion is based on G-QSAR, 2D-QSAR and docking studies.
(3) A chlorine atom at R3 is found to be important for the activity as well as selectivity based on G-QSAR, 2D-QSAR and QAAR studies.
(4) Presence of a ring structure like 4-chloro-benzyl group at R1 position is required for the activity based on the positive contribution of the R1-chi3Cluster descriptor as obtained in the G-QSAR model. Hence a ring or branching structure at R1 position is important for the activity.
(5) Presence of a –NH– fragment is found to be essential, since it interacts with an active site residue (Ile 10) as seen in most of the compounds. The Ile 10 residue was earlier found to be an essential residue responsible for biological activity based on molecular dynamics study.29
To design new molecules with improved activity/selectivity, one has to consider the above requisite structural features, while trying with recommended different scaffolds (for e.g., different ring structures or branched structures at R1 position) at various positions on similar backbones and then can predict the activity or selectivity values using reported QSAR models. Further, the newly designed molecules can be docked to the CDK5/p25 enzyme to notice the changes in interactions with active site residues and docking scores due to newly added structural features. Further, the developed models can be used as efficient query tools for screening of potent and selective CDK5/p25 inhibitors. This study offers an understanding of the essential structural features or properties of the molecules for proper binding in the active site of the CDK5/p25 enzyme.
Acknowledgements
The authors are thankful to the Department of Biotechnology (DBT), Government of India, New Delhi for the financial assistance.
References
- S. E. Arnold, B. T. Hyman, J. Flory, A. R. Damasio and G. W. Van Hoesen, The topographical and neuroanatomical distribution of neurofibrillary tangles and neuritic plaques in the cerebral cortex of patients with Alzheimer's disease, Cereb. Cortex, 1991, 1, 103–116 CrossRef CAS.
- G. N. Patrick, L. Zukerberg, M. Nikolic, S. de La Monte, P. Dikkes and L.-H. Tsai, Conversion of p35 to p25 deregulates Cdk5 activity and promotes neurodegeneration, Nature, 1999, 402, 615–622 CrossRef CAS PubMed.
- J. Kuret, G. S. Johnson, D. Cha, E. R. Christenson, A. J. DeMaggio and M. F. Hoekstra, Casein Kinase 1 Is Tightly Associated with Paired-Helical Filaments Isolated from Alzheimer's Disease Brain, J. Neurochem., 1997, 69, 2506–2515 CrossRef CAS.
- D. P. Hanger, K. Hughes, J. R. Woodgett, J.-P. Brion and B. H. Anderton, Glycogen synthase kinase-3 induces Alzheimer's disease-like phosphorylation of tau: generation of paired helical filament epitopes and neuronal localisation of the kinase, Neurosci. Lett., 1992, 147, 58–62 CrossRef CAS.
- M. Otyepka, B. Iveta, K. Zdeněk and K. Jaroslav, Different mechanisms of CDK5 and CDK2 activation as revealed by CDK5/p25 and CDK2/cyclin A dynamics, J. Biol. Chem., 2006, 281, 7271–7281 CrossRef CAS PubMed.
- D. O. Morgan, Cyclin-dependent kinases: engines, clocks, and microprocessors, Annu. Rev. Cell Dev. Biol., 1997, 13, 261–291 CrossRef CAS PubMed.
- M. Lee, Y. T. Kwon, M. Li, J. Peng, R. M. Friedlander and L.-H. Tsai, Neurotoxicity induces cleavage of p35 to p25 by calpain, Nature, 2000, 405, 360–364 CrossRef CAS PubMed.
- J. C. Cruz and L.-H. Tsai, A Jekyll and Hyde kinase: roles for Cdk5 in brain development and disease, Curr. Opin. Neurobiol., 2004, 14, 390–394 CrossRef CAS PubMed.
- J. Sridhar, N. Akula and N. Pattabiraman, Selectivity and potency of cyclin-dependent kinase inhibitors, AAPS J., 2006, 8, E204–E221 CrossRef CAS PubMed.
- M. R. Shiradkar, K. C. Akula, V. Dasari, V. Baru, B. Chiningiri, S. Gandhi and R. Kaur, Clubbed thiazoles by MAOS: a novel approach to cyclin-dependent kinase 5/p25 inhibitors as a potential treatment for Alzheimer's disease, Bioorg. Med. Chem., 2007, 15, 2601–2610 CrossRef CAS PubMed.
- M. Shiradkar, J. Thomas, V. Kanase and R. Dighe, Studying synergism of methyl linked cyclohexyl thiophenes with triazole: Synthesis and their cdk5/p25 inhibition activity, Eur. J. Med. Chem., 2011, 46, 2066–2074 CrossRef CAS PubMed.
- ChemDraw Ultra 5.0, Cambridge Soft Corporation, Cambridge, USA, 1999 Search PubMed.
- Cerius2 Version 4.10., Accelrys, Inc., San Diego, CA, 2005 Search PubMed.
- C. W. Yap, PaDEL-descriptor: An open source software to calculate molecular descriptors and fingerprints, J. Comput. Chem., 2011, 32, 1466–1474 CrossRef CAS PubMed.
- R. Todeschini, V. Consonni, A. Mauri and M. Pavan, DRAGON-Software for the calculation of molecular descriptors version 6, 2004.
- M. D. S. Vlife, software package, version 3.0, supplied by VLifescience Technologies Pvt Ltd, Pune, 2008.
- J. T. Leonard and K. Roy, On selection of training and test sets for the development of predictive QSAR models, QSAR Comb. Sci., 2006, 25, 235–251 CAS.
- J. N. i. Marija, S. Inc, SPSS professional statistics 9.0, 1994.
- P. Geladi and B. R. Kowalski, Partial least-squares regression: a tutorial, Anal. Chim. Acta, 1986, 185, 1–17 CrossRef CAS.
- D. Rogers and A. J. Hopfinger, Application of genetic function approximation to quantitative structure–activity relationships and quantitative structure–property relationships, J. Chem. Inf. Comput. Sci., 1994, 34, 854–866 CrossRef CAS.
- K. Roy, P. Chakraborty, I. Mitra, P. K. Ojha, S. Kar and R. N. Das, Some case studies on application of rm2 metrics for judging quality of quantitative structure–activity relationship predictions: Emphasis on scaling of response data, J. Comput. Chem., 2013, 34, 1071–1082 CrossRef CAS PubMed.
- I. Mitra, A. Saha and K. Roy, Exploring quantitative structure–activity relationship studies of antioxidant phenolic compounds obtained from traditional Chinese medicinal plants, Mol. Simul., 2010, 36, 1067–1079 CrossRef CAS.
- A. Golbraikh and A. Tropsha, Beware of q2!, J. Mol. Graphics Modell., 2002, 20, 269–276 CrossRef CAS.
- A. Tropsha, Best practices for QSAR model development, validation, and exploitation, Mol. Inf., 2010, 29, 476–488 CrossRef CAS.
- Glide, version 6.0, Schrödinger, LLC, New York, NY, 2013 Search PubMed.
- J. Malmstrom, J. Viklund, C. Slivo, A. Costa, M. Maudet, C. Sandelin, G. Hiller, L.-L. Olsson, A. Aagaard and S. Geschwindner, Synthesis and structure–activity relationship of 4-(1,3-benzothiazol-2-yl)-thiophene-2-sulfonamides as cyclin-dependent kinase 5 (cdk5)/p25 inhibitors, Bioorg. Med. Chem. Lett., 2012, 12, 5919–5923 CrossRef PubMed.
- N. M. O'Boyle, M. Banck, C. A. James, C. Morley, T. Vandermeersch and G. R. Hutchison, Open Babel: An open chemical toolbox, J. Cheminf., 2011, 3, 1–14 Search PubMed.
- M. Mapelli, L. Massimiliano, C. Crovace, M. A. Seeliger, L. Tsai, L. Meijer and A. Musacchio, Mechanism of CDK5/p25 Binding by CDK Inhibitors, J. Med. Chem., 2005, 48, 671–679 CrossRef CAS PubMed.
- W. Wang, X. Cao, X. Zhu and Y. Gu, Molecular dynamic simulations give insight into the mechanism of binding between 2-aminothiazole inhibitors and CDK5, J. Mol. Model., 2013, 19, 2635–2645 CrossRef CAS PubMed.
Footnotes |
† Electronic supplementary information (ESI) available: Table S1 contains summary (list of descriptors and values of various statistical and validation metrics) of the reported QSAR and QAAR models. Tables S2, S3 and S4 list the results of 2D-QSAR, G-QSAR and QAAR models (compound names, descriptor values, activity values (observed, calculated and LOO predicted) and docking scores) respectively. The structures of all 224 compounds considered in the G-QSAR and 2D-QSAR models are provided in a .sdf file (224cdk5ExploitH.sdf). The structures of 18 compounds considered in the QAAR model are provided in another .sdf file (18cdk5.sdf). See DOI: 10.1039/c3ra46861e |
‡ Presently at Manchester Institute of Biotechnology, Manchester M1 7DN, UK. |
|
This journal is © The Royal Society of Chemistry 2014 |
Click here to see how this site uses Cookies. View our privacy policy here.