DOI:
10.1039/D0NA00279H
(Minireview)
Nanoscale Adv., 2020,
2, 3103-3114
Biologically interfaced nanoplasmonic sensors
Received
8th April 2020
, Accepted 26th June 2020
First published on 2nd July 2020
Abstract
Understanding biointerfacial processes is crucial in various fields across fundamental and applied biology, but performing quantitative studies via conventional characterization techniques remains challenging due to instrumentation as well as analytical complexities and limitations. In order to accelerate translational research and address current challenges in healthcare and medicine, there is an outstanding need to develop surface-sensitive technologies with advanced measurement capabilities. Along this line, nanoplasmonic sensing has emerged as a powerful tool to quantitatively study biointerfacial processes owing to its high spatial resolution at the nanoscale. Consequently, the development of robust biological interfacing strategies becomes imperative to maximize its characterization potential. This review will highlight and discuss the critical role of biological interfacing within the context of constructing nanoplasmonic sensing platforms for biointerfacial science applications. Apart from paving the way for the development of highly surface-sensitive characterization tools that will spur fundamental biological interaction studies and improve the overall understanding of biological processes, the basic principles behind biointerfacing strategies presented in this review are also applicable to other fields that involve an interface between an inorganic material and a biological system.
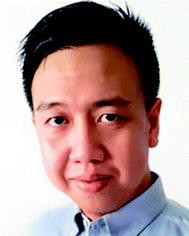 Abdul Rahim Ferhan | Abdul Rahim Ferhan is a Research Fellow in the Engineering in Translational Science Group at the Centre for Biomimetic Sensor Science of Nanyang Technological University (NTU). He received his B.Eng. degree in Chemical and Biomolecular Engineering and PhD degree in Biomedical Engineering from the School of Chemical and Biomedical Engineering, NTU. His research focuses on the development and application of novel nanoplasmonic sensing platforms for biomedical diagnostics and fundamental investigations into biomolecular interactions. |
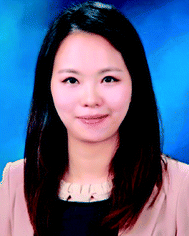 Bo Kyeong Yoon | Bo Kyeong Yoon is a Research Fellow in the Translational Nanobioscience group within the School of Chemical Engineering at Sungkyunkwan University (SKKU). She received a joint PhD degree from Nanyang Technological University and the University of Natural Resources and Life Sciences (BOKU) in Austria, where she studied Materials Science and Engineering along with Food Chemistry and Biotechnology. Her research focuses on engineering strategies to inhibit membrane-enclosed pathogens, such as enveloped viruses, and related lipid membrane-based biosensor technologies. |
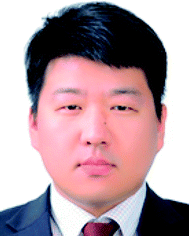 Won-Yong Jeon | Won-Yong Jeon is a Research Professor in the Translational Nanobioscience group within the School of Chemical Engineering at Sungkyunkwan University (SKKU). He received his B.S. and M.S. degrees in Chemistry and PhD degree in Nanobiomedical Science from Dankook University (DKU). His research focuses on the development and application of biosensing platforms for biomedical devices and electrochemical characterization of biomacromolecular interactions. |
 Nam-Joon Cho | Nam-Joon Cho is MRS-Singapore Chair Professor in the School of Materials Science and Engineering at Nanyang Technological University. A recipient of the National Research Foundation Fellowship in Singapore, he received his PhD degree in Chemical Engineering from Stanford University, and completed a postdoctoral fellowship at the Stanford University School of Medicine. His research focuses on engineering artificial lipid membrane and tissue platforms to probe biological systems, and to develop enhanced therapeutic and drug delivery options that more effectively target infectious diseases, inflammatory disorders, and cancer. |
Introduction
Understanding biointerfacial processes has broad implications across various biological-related fields.1–4 Against this backdrop, extensive research has been dedicated to unravel the mechanism behind a wide range of biomacromolecular interactions occurring at the solid–liquid interface.5–7 This has been accomplished with the aid of conventional surface-based characterization techniques such as surface plasmon resonance (SPR),8,9 attenuated total reflection Fourier-transform infrared (ATR-FTIR) spectroscopy,10,11 and atomic force microscopy (AFM).12,13 However, while these techniques are undoubtedly capable of extracting qualitative physicochemical insights related to a particular biointerfacial process of interest, they still present several limitations when performing real-time quantitative measurements due to instrumentation, data processing and analytical complexities.
In the SPR technique, measurements are typically conducted in the Kretcshmann configuration, which requires focusing a monochromatic polarized light beam onto a thin gold film through a glass prism.8 Biomolecular binding events on the gold film are tracked based on changes in intensity of the reflected light with respect to the angle of incidence. The technique is particularly sensitive to changes in bulk refractive index and the response signal is therefore susceptible to environmental perturbations. Likewise, in the ATR-FTIR technique, the chemical structure and conformation of biomolecules adsorbed onto the ATR crystal are determined based on interaction with an infrared (IR) beam.10 The instrument setup generally requires an IR source, a series of fixed and movable mirrors, a beam splitter, and an optically dense ATR crystal to achieve multiple internal reflections. While multiple internal reflections result in stronger IR interaction with the adsorbates, it can adversely affect the output signal due to significant light scattering. In addition, strong light absorption by water at certain regions of the IR spectrum (e.g., 3000–4000 cm−1) can also diminish the response signal. In the AFM technique, which is widely employed for direct visualization and height profiling of adsorbed biomolecules, the instrument setup generally comprises a laser source, a probe cantilever, a piezoelectric scanning unit, a photodetector, and a feedback controller.13 Aside from instrumentation complexity, one of its main limitations is the long acquisition time for each AFM image due the raster scanning process, resulting in poor temporal resolutions.
To overcome these limitations, nanoplasmonic sensing has recently emerged as a promising tool for biointerfacial science investigations.2,5,14 Besides simple instrumentation that promotes miniaturization and portability,15 nanoplasmonic sensing platforms offer exceptional surface sensitivity. While bulk sensitivity relates to the plasmonic response per refractive index change in the bulk medium, surface sensitivity relates to the plasmonic response per refractive index change in the immediate vicinity of the plasmonic transducer (e.g., due to a surface-bound molecular layer), and sensing platforms with high surface sensitivities facilitate the real-time tracking of biological processes with good spatiotemporal resolution. The prevalence of nanoplasmonic sensing platforms within the context of biointerfacial science has therefore prompted the development of robust biological interfacing strategies, which is imperative to maximize the characterization potential of nanoplasmonic sensors. Recently, it has been established that the measurement response from nanoplasmonic sensors is determined not only by the performance parameters of the sensing platform but also the type of surface modification and biointerfacing effected on the sensing surface.16 Biological interfaces should ideally anchor bioreceptors while warranting a high degree of detection specificity. This is achieved by minimizing the adsorption of non-target molecules on the surface, which in turn, along with optimal plasmonic properties of the transducer, contribute to a high specific signal response. Conventionally, some form of surface modification is usually necessary to effectively adhere a biologically active layer to the surface of the nanoplasmonic transducer (e.g., noble metal nanostructure).17,18 However, recent works have also demonstrated the possibility of directly introducing a biological interface without any surface modification.19,20
In this review, we discuss recent progress in the development of biologically interfaced nanoplasmonic sensors with emphasis on the latest strategies employed for surface modification and biological functionalization. We begin by describing the processes involved in the design and fabrication of nanoplasmonic architectures within the context of biological-related applications, highlighting notable design features that have been adopted to facilitate biological interfacing. We then review relevant surface modification and biological interfacing methodologies on nanoplasmonic architectures including the direct fabrication of biomimetic coatings in the form of supported lipid bilayers. Finally, we present a series of application examples in which biologically interfaced nanoplasmonic sensors have played a pivotal role in resolving biomacromolecular interactions at the nanoscale. We conclude by highlighting the vast potential of nanoplasmonic sensing in biointerfacial science and underscore the importance of continually developing biointerfaces that continue to push the analytical boundary of nanoplasmonic sensors.
Sensing platform design
Surface-based nanoplasmonic sensing platforms typically comprise of a sensor chip housed within a measurement chamber, which is connected to a visible-infrared light source and a spectrophotometer (Fig. 1). In laboratory setups, a white tungsten halogen lamp is typically utilized, and is connected to the measurement chamber and spectrophotometer via fibre optic cables. In portable systems, a light-emitting diode (LED) is commonly used in place of the tungsten halogen lamp, and is usually packaged with the sensor and spectrophotometer in a single compartment. Regardless of the setup configuration, the sensor chip represents the most crucial component of the sensing platform and there are several key considerations involved in its construction, especially for the purpose of biointerfacial investigations. Besides possessing extraordinary surface sensitivity and displaying high figures of merit (FoM), which is defined as the resonance shift divided by the resonance linewidth,21 the transducer nanostructures should be chemically stable and biologically inert, with strong adhesion to the support substrate.22 This is necessary to minimize bulk solution effects (i.e., arising from temperature, pH fluctuations, etc.) and ensure a stable signal readout with reduced noise, especially when performing measurements in the presence of complex biological solutions. In addition, the nanostructure configuration should allow unobstructed flow of the sample solution and without hampering the diffusion of target molecules to the sensing interface.23,24 This promotes good response sensitivity and high temporal resolution.
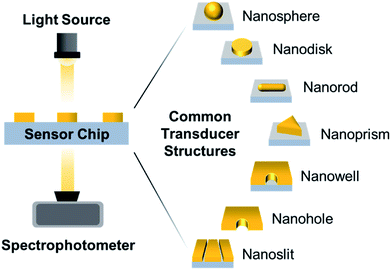 |
| Fig. 1 Overall schematic of the nanoplasmonic sensing platform in a typical transmission mode configuration with its primary components, accompanied by graphical representations of common transducer nanostructures (shown here as unit structures), which can either be randomly distributed or arranged in a periodic fashion on nanoplasmonic sensor chips. | |
Nanofabrication techniques
Advances in nanofabrication have established a series of lithographic and templating techniques that allow greater control over the construction of nanoplasmonic transducers.5,25–28 As a result, many efforts have focused on improving the surface sensitivity and FoM of the sensor by carefully modulating the transducer geometry, dimension and arrangement (e.g., random or periodic array). Recently established techniques that have gained popularity within the context of fabricating nanoplasmonic structures for biosensing applications include hole-mask colloidal lithography due to its relative simplicity in generating circular nanostructures29 (Fig. 2A), as well as template-stripping (following the fabrication of the template via nanoimprint lithography) for generating periodic nanocavity and nanohole arrays30 (Fig. 2B). Along this line, there is an emerging trend of employing DNA templating technologies to obtain nanostructures with nanometer precision.31,32 In the first demonstration, Shen et al. described a DNA-assisted lithography (DALI) method based on the combination of DNA origami and conventional lithography.31 Briefly, folded DNA origami structures with pre-programmed shapes were self-assembled on the support substrate and served as a template for the construction of well-defined metallic nanostructures. The primary advantage of DALI lies in the ability to program arbitrary and elaborate shapes, owing to the versatility of DNA origami. They demonstrated the method for the construction of three different metallic nanoshapes, which were found to present exceptional plasmonic properties.
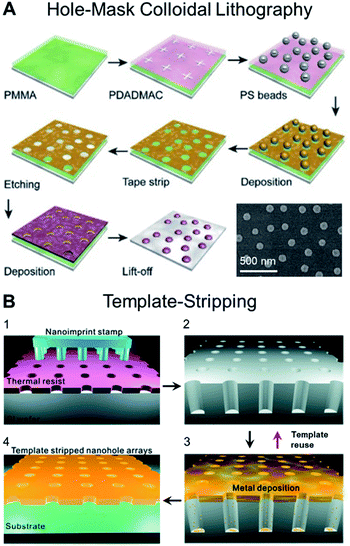 |
| Fig. 2 (A) Schematic illustrating the steps involved in producing plasmonic nanostructures following the hole-mask colloidal lithography technique, which enable the fabrication of uniform nanopatterns on wafer-scale samples. Reproduced with permission from ref. 29. Copyright (2019) Elsevier. (B) Schematic for the fabrication of large-area nanohole arrays following the template-stripping method. (1) Thermal resist layer spun on a Si wafer is imprinted with a nanoimprint stamp with circular post patterns; (2) Si wafer is subsequently etched to be a nanohole template with deep circular trenches. (3) Metal film is directionally deposited on the Si template. (4) Metal surface is coated with a thin layer of epoxy and covered with a glass slide. The Ag film is then peeled off of the template to reveal the smooth nanohole array made in the metal film. The Si template can be reused to make multiple identical samples. Reproduced with permission from ref. 30. Copyright (2011) American Chemical Society. | |
Nanostructure-performance relationship
Despite the progress in nanofabrication, predicting the overall performance of the sensor based on the properties of the nanoplasmonic transducer remains challenging. This process is largely iterative and involves several rounds of nanostructure synthesis, characterization and numerical simulations (e.g., finite-difference time-domain, FDTD). To address this limitation, recent efforts have shifted towards the utilization of machine learning, specifically deep learning, to establish the correlation between the physical properties, plasmonic properties and sensing performances of plasmonic nanostructures. For example, Malkiel et al. described a Deep Neural Network (DNN) trained with thousands of synthetic experiments.33 The novelty of the approach lies in the bidirectional architecture made up of two networks, namely the Geometry-Predicting-Network (GPN) and the Spectrum-Predicting-Network (SPN). The GPN predicts the geometry based on the spectra while the SPN performs the opposite. In a typical operation, the geometry predicted by the GPN is provided to the SPN to predict the spectrum. In a separate work, He et al. successfully employed DNN to predict far- and near-field optical properties of Au nanospheres, nanorods, and dimers (Fig. 3).34
 |
| Fig. 3 Structures of the machine learning model for prediction of optical properties and designing NPs. Far- and near-field optical data obtained from the FDTD simulations were used to train three different machine learning models: far-field spectra and structural information for (i) structure classification, far-field spectra and dimensions for (ii) the spectral DNN, and near-field enhancement maps and dimensions for (iii) the E-field DNN. After training, the machine learning models can be used to perform forward prediction and/or inverse design. The solid and dashed red arrows represent the forward prediction and the inverse design process, respectively. Reproduced with permission from ref. 34. Copyright (2019) Royal Society of Chemistry. | |
Indirect nanoplasmonic sensing
While conventional surface-based nanoplasmonic sensors typically comprise of bare nanoplasmonic transducers on a support substrate, the indirect nanoplasmonic sensing (INPS) concept was later introduced, in which the nanostructures are further coated with a thin conformal layer of dielectric material.35 This offers greater thermal, chemical and physical stability to the nanostructures as they are protected from the bulk environment and reduces the tendency for erosion and peel-off from the support substrate. More importantly, the INPS format greatly facilitates biological interfacing since the active sensing surface is now homogeneous and may comprise of biologically compatible materials such as silicon oxide and titanium oxide.
Recent progress in INPS have witnessed the construction of topographically flat nanoplasmonic sensors whereby the Au nanodisk transducers are embedded within the support substrate and coated with a flat layer of oxide material36,37 (Fig. 4A). This improves the flow of sample solution and ensures good diffusion of biomacromolecules to the sensing substrate. Further exploration of the INPS format involves various types of nanostructures and coating materials. In the latest development, Nugroho et al. described the construction of an array of vertically-stacked Ag nanodisks with different dimensions, separated by a thick layer of silicon oxide spacer, and conformally coated with a thin layer of silicon nitride38 (Fig. 4B). They achieved well-separated localized surface plasmon resonance (LSPR) modes exhibiting two resonance peaks that can be used for optical sensing in three dimensions (Fig. 4C). Separately, Zhao et al. demonstrated the fabrication of quasi-one-dimensional Au nanoribbons with a thin layer of indium oxide coating.39 The platform exhibits two sets of resonance peaks and dips with varying bulk and surface sensitivities, which was employed to distinguish the adsorption of biomolecules with different sizes.
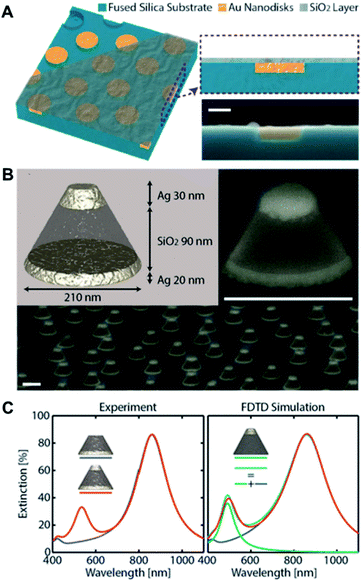 |
| Fig. 4 (A) Schematic depiction of the topographically flat nanoplasmonic sensor chip with embedded amorphous array of plasmonic Au nanodisks. The three-dimensional sketch shows the three “layers” of the device, i.e., the wells in the fused silica substrate, the Au nanodisks grown inside the wells, and the topographically flat SiO2 capping layer. Reproduced with permission from ref. 36. Copyright (2017) American Chemical Society. (B) Artist's rendition of the 3D nanoplasmonic sensor architecture. Two Ag nanodisks of different diameter and thickness optimized to maximize spectral separation of their LSPR are vertically separated by a thick SiO2 spacer layer. Tilted SEM image of a single and quasi-random array of a 3D sensor metasurface. Scale bars are 200 nm. (C) Experimental and FDTD-simulated optical extinction spectra of 3D sensors. Also shown are the extinction spectra of a sensor with only top disk (green) and sum of the extinction of the sensor with only bottom disk and sensor with only top disk (cyan). Reproduced with permission from ref. 38. Copyright (2020) American Chemical Society. | |
Biological interfacing strategies
The surface modification and biological interfacing of nanoplasmonic sensors serve various functions such as anchoring receptor biomolecules, reducing non-specific biomolecular interactions, mimicking biological systems, and tuning surface properties for a particular biological interaction of interest (e.g., by modulating surface charge or introducing specific functional groups). Undoubtedly, there are plenty of well-established surface modification and biological functionalization approaches to choose from, including, but not limited to, self-assembled monolayers (SAM),40–42 layer-by-layer (LbL) assemblies of alternately charged polymers,43–45 polymer brushes,46–48 and hydrogel coatings.49–51 While these approaches have been successfully adopted across a wide range of biosensing systems, and proven effective in hosting receptor biomolecules as well as preventing non-specific biomolecular interactions, not all are suitable for nanoplasmonic sensing.
Key considerations
There are several prerequisites for selecting a surface modification or biological interfacing strategy within the context of nanoplasmonic sensing. Firstly, it is important to recognize that nanoplasmonic sensors have effective sensing depths that are significantly lower than most commonly used surface-based measurement techniques.52,53 For example, the effective sensing depth, or penetration depth of the evanescent wave, in prism-based SPR is around 200 nm.54 Likewise, the effective sensing depth, or penetration depth of the shear wave, in QCM-D is around 60–250 nm – the exact value depends on the fundamental frequency of the quartz crystal, which is the lowest frequency at which the crystal can be excited to resonance.55 In contrast, the evanescent plasmonic field decay lengths in nanoplasmonic sensing platforms have been precisely determined using atomic layer deposition (ALD),56,57 and found to be in the order of 5–30 nm. Hence, the thickness of the biointerface should be below this range to ensure that biomolecular interactions occur well within the nanoplasmonic field and be adequately detected.58 In addition, the biointerfacing layer should not induce a huge change in refractive index in the immediate vicinity of the nanoplasmonic transducer (i.e., not optically dense). This is to maintain the resonance wavelength position and linewidth of the transducer and ensure that its surface sensitivity is preserved. The biointerface should also be robust to tolerate pH fluctuations and surface charge variations in the presence of complex biological solutions and withstand harsh flow conditions without delamination.
Lipid membrane models
Lipid membrane model systems represent an ideal candidate for the biological interfacing of nanoplasmonic sensors.59,60 They are largely planar with a uniform thickness of ∼5 nm, are not optically dense, and can be conformally coated on nanostructured surfaces to mimic key biophysical and architectural features of cellular membranes. Several methods have been established for the attachment of these biomimetic membranes to the sensor surface,61 which can be categorized into three main configurations namely hybrid bilayer membrane (HBM), tethered bilayer lipid membrane (tBLM), and supported lipid bilayer (SLB). Briefly, HBMs consist of a lipid monolayer formed over a self-assembled monolayer (e.g., alkanethiol) on a support substrate, while tBLMs consist of a lipid bilayer which is anchored to the underlying support substrate through a tethering unit. Both HBMs and tBLMs require an intermediate surface modification step. On the other hand, SLBs float solely on a thin hydration layer ∼1 nm above an unmodified support substrate.
On conventional nanoplasmonic sensors comprising of uncoated transducers, the fabrication of HBMs, tBLMs and SLBs is challenging due to different reasons. In the case of HBMs and tBLMs, the difference in material between the transducer and support substrate necessitates multiple surface modification steps. For SLBs, which is commonly fabricated via vesicle fusion, lipid vesicles do not rupture on the transducer material (i.e., Au and Ag), hence hampering the formation of a uniform layer on the sensor surface. The introduction of INPS enables the direct formation of SLBs on a silicon oxide overcoating, which induces the spontaneous rupture of lipid vesicles. Consequently, several works have successfully fabricated biologically interfaced nanoplasmonic sensors by fabricating SLBs on silicon oxide-coated substrates via vesicle fusion. Along this line, our group has performed a series of works using vesicles with different lipid compositions and under different treatments to understand the interaction of lipid bilayers with the underlying substrate.62–64
Novel lipid bilayer fabrication methods
Despite its popularity, there are several limitations associated with the vesicle fusion method.65 In particular, the spontaneous rupture of lipid vesicles upon adsorption can only be achieved on a limited selection of material surfaces.66 The incorporation of biological components also hinder the rupture process, which in turn restricts the formation of SLBs with realistic cell membrane compositions. Furthermore, the formation of high-quality SLBs require precise control over the size and uniformity of the extruded vesicles. These limitations motivated the development of the solvent-assisted lipid bilayer (SALB) method,67 which relies on the self-assembly of lipid molecules on the support substrate upon switching from an organic solvent to an aqueous buffer solution (Fig. 5A). The process is simple, quick and efficient, and feasible on a wide variety of material surfaces including Au and inorganic oxides (e.g., SiO2, TiO2 and Al2O3). Most importantly, it provides a bottom-up approach for the fabrication of realistic biomimetic platforms.
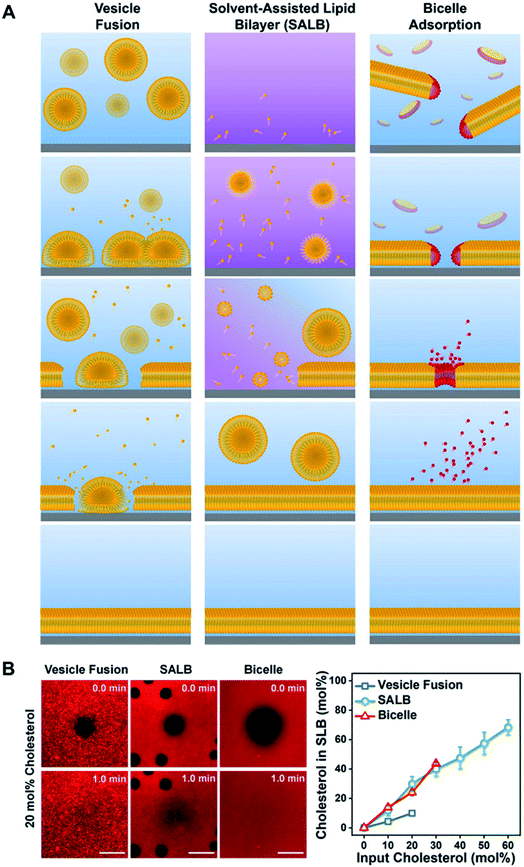 |
| Fig. 5 (A) Schematic comparison of the vesicle fusion, SALB, and bicelle methods to form supported lipid bilayers. (Left column) The vesicle fusion method involves vesicle adsorption, deformation, and eventual rupture on a solid surface. The SLB formation process critically depends on vesicle–substrate interactions, which must be sufficiently strong to induce rupture via vesicle–substrate and/or vesicle–vesicle interactions. Typically, this method works only on a narrow range of material surfaces, particularly silica-based ones. (Middle column) The SALB method is based on depositing long-chain phospholipids in a water-miscible organic solvent, followed by a solvent-exchange step with aqueous solution. Initially, the phospholipid molecules in organic solvent self-assemble into inverted micelles and/or remain in the monomeric state and attach to the surface in an equilibrium with bulk lipids. During the solvent-exchange step, the bulk liquid transitions from predominately organic solvent to fully aqueous solution. Consequently, phospholipid molecules within the system begin to form lamellar-phase structures, leading to SLB formation on the surface. (Right column) Bicelles contain long-chain (yellow) and short-chain (red) phospholipids and are disklike nanostructured assemblies in aqueous solution. If there is an attractive bicelle–surface interaction, then bicelles adsorb onto the surface and then can fuse with one another. Provided the total lipid concentration is sufficiently low, long-chain phospholipid molecules remain attached on the surface and form an SLB while short-chain phospholipid molecules leave the surface as monomers. The outcome is a complete SLB consisting of long-chain phospholipids, and the entire fabrication process occurs under aqueous conditions. Reproduced with permission from ref. 65. Copyright (2020) American Chemical Society. (B) In the left panel, fluorescence recovery after photobleaching (FRAP) images of SLBs formed at 20 mol% input cholesterol in each method. All scale bars are 20 μm. In the right panel, molar fraction of cholesterol in SLB as a function of input cholesterol fraction. Reproduced with permission from ref. 69. Copyright (2019) American Chemical Society. | |
More recently, our group has rigorously optimized and promoted another alternative approach for the fabrication of SLBs using lipid bicelles.68–70 Lipid bicelles are quasi-two-dimensional lamellar disks comprising of a mixture of long- and short-chain phospholipids.71 The bicelle-mediated formation of SLBs follow the adsorption and subsequent rupture of bicelles onto the support substrate, analogous to the vesicle fusion method. However, due to its relatively flat geometry and shorter residence times of the short-chain phospholipids, the quality of the resulting SLBs is significantly higher than those obtained via vesicle fusion. In addition, the use of bicelles instead of vesicles also offers greater possibility of biomolecular incorporation, including for cholesterol enrichment69 (Fig. 5B). Compared to the SALB method, the minimum required lipid concentration for the bicelle-mediated approach is 5–10 times lower. More importantly, it can be executed in a fully aqueous environment, which is advantageous for the incorporation of proteins.
Applications of biologically interfaced nanoplasmonic sensors
Assessing membrane–surface interactions
At the most fundamental level, biologically interfaced nanoplasmonic sensors have been used to quantitatively assess the influence of lipid composition on the interaction between lipid membranes and a contacting surface.39,62,72 Of note, the narrow effective sensing depth of the nanoplasmonic sensor has been exploited to study the effect of lipid charge on SLB spatial proximity to the underlying substrate.73 Differences in spatial proximity were resolved down to the sub-nanometer range. The study was later expanded to compare the interaction of lipid vesicles and SLBs on different oxides surfaces, with respect to changes in lipid composition74 (Fig. 6A and B). In a separate work, Limaj et al. employed an infrared plasmonic biosensor comprising of a silicon oxide-coated gold nanoantenna array to monitor in real time the kinetics of SLB formation by capturing the vibrational fingerprints of lipid molecules75 (Fig. 6C–E). Taken together, the series of insights from these works contribute towards achieving greater control over the SLB fabrication process, which is crucial in the development of realistic biological interfaces.
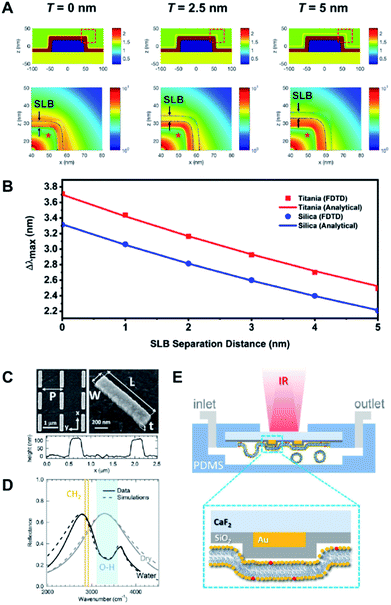 |
| Fig. 6 (A) Refractive index distributions (top) and FDTD simulations of the electric field distributions (bottom) for SLB-coated sensing platforms, with bilayer-substrate separation distances of 0, 2.5 and 5.0 nm. (B) Relationship between LSPR Δλmax shifts and SLB separation distance for titania- and silica-coated sensor surfaces according to FDTD simulation results and analytical calculations. Reproduced with permission from ref. 74. Copyright (2018) American Chemical Society. (C) SEM images and AFM profile of a typical gold nanoantenna array on CaF2 substrate with L = 1.05 μm, P = 1.37 μm, W = 0.2 μm, and t = 0.1 μm. (D) Experimental (solid lines) and simulated (dashed lines) reflectance for nanoantenna array (L = 1.05, P = 1.37 μm) in dry (gray) and aqueous (black) environment. The spectral regions associated with OH stretching (water) and CH2 symmetric and asymmetric stretching modes (lipids) are indicated by light blue-shaded region and orange lines, respectively. (E) Schematic illustration (not in scale) showing the fluidic chamber and experimental configuration during the measurements. Yellow and red phospholipid headgroups indicate the DOPC and Texas RED DHPE, respectively. Reproduced with permission from ref. 75. Copyright (2016) American Chemical Society. | |
Tracking biomolecular–membrane interactions
Biologically interfaced nanoplasmonic sensors have been successfully employed to investigate various biomolecular activities at the membrane interface involving proteins,76 peptides,77 and ionic liquids.78 Of note, the group of Sönnichsen explored the use of lipid membrane coated gold nanorods to study the molecular dynamics of an oscillating biological system using the MinDE protein from Escherichia coli (E. coli) as an example.79 They monitored the attachment and detachment of the protein from the lipid membrane for over an hour and extracted a precise wave profile exhibiting four phases. They also observed a strong influence of membrane composition and local curvature on the oscillation behaviour80 (Fig. 7A and B). Studies involving biomolecular interactions can also be performed on a chip-based format. For example, Zhu et al. integrated an antibody-conjugated nanoplasmonic barcode biosensor with a biomimetic microfluidic ‘adipose-tissue-on-chip’ platform to measure pro- and anti-inflammatory cytokines secreted by adipocytes and macrophages in a highly multiplexed fashion81 (Fig. 7C and D). This paves the way for the development of high-throughput measurement platforms, which can be adopted for clinical applications.
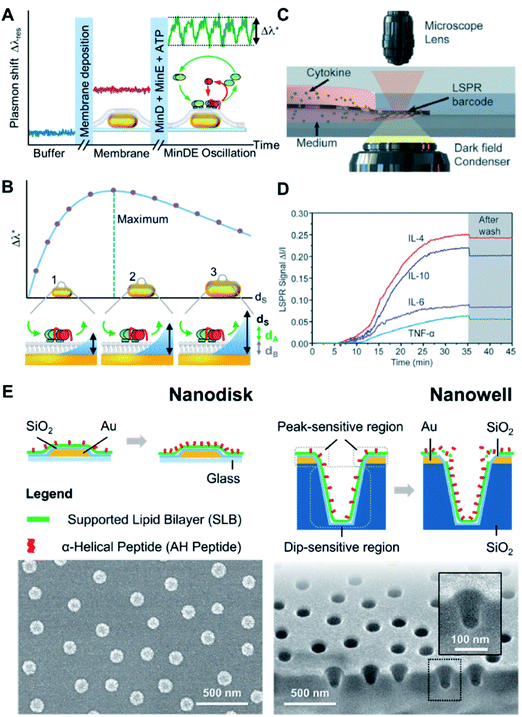 |
| Fig. 7 (A) The continuous monitoring of the peak shift generated from MinDE binding from of every nanoparticle as a function of time, starting in buffer (blue line). The formation of a supported membrane over the particles (pink line, center inset) and the transient adsorption of the MinDE protein system (green line, inset right side with MinD in green, MinE in red, the lipid membrane in gray, and the particles in gold) was observed. The wave amplitude Δλ* (dashed lines) is extracted for further analysis. (B) The sensing distance dS of the nanoparticle sensor was systematically changed by varying the size. The oscillation amplitude Δλ* shows a maximum when the sensor's sensing distance dS matches the thickness of the oscillating (dA) and static (dB) layers on top of the particle (inset center). If the particles' sensing distance (symbolized by the blue area) is smaller/larger than dA + dB, the observed oscillation amplitude Δλ* is reduced (inset left/right, respectively). Relationship between LSPR Δλmax shifts and SLB separation distance for titania- and silica-coated sensor surfaces according to FDTD simulation results and analytical calculations. Reproduced with permission from ref. 80. Copyright (2018) American Chemical Society. (C) Schematic of LSPR signal detection on a dark-field microscopy stage. (D) Real-time LSPR signals during the multiplexed cytokine detection. The gray area shows the LSPR signal after washing with PBS. Reproduced with permission from ref. 81. Copyright (2018) Royal Society of Chemistry. (E) Schematic illustrations of membrane–peptide interactions with supported lipid bilayers that are conformally coated on nanodisk and nanowell arrays (top). Accompanying SEM micrographs of the respective sensing platforms (bottom). Reproduced with permission from ref. 82. Copyright (2018) American Chemical Society. | |
Investigating influence of membrane curvature
An important aspect of biomolecular interactions that is often difficult to systematically study is the influence of membrane curvature. In this regard, advances in nanofabrication have permitted the construction of nanoplasmonic transducers with well-defined geometry, which can serve as templates to precisely control membrane curvature at the same time offering advanced sensing capabilities. Our group has investigated the curvature dependency of an amphiphatic α-helical (AH) peptide by introducing the peptide to SLBs fabricated on silicon oxide-coated gold nanodisk and gold nanowell arrays82 (Fig. 7E). In particular, the nanowells afforded SLBs with highly positive (i.e., on the rim) and negative (i.e., in the interior) curvatures, which would otherwise be difficult to achieve using a non-templated approach. Our study quantitatively confirmed the preference for AH peptide to bind and disrupt the membrane at positively curved membrane regions above a threshold concentration. Similarly, Emilsson et al. used nanowells with conformally coated SLBs to determine the preferential binding of the insulin receptor tyrosine kinase substrate protein (IRSp53) I-BAR domain to negatively curved membrane regions.83 In another work, the group employed plasmonic nanopores functionalized with nucleoporin domains to mimic the nuclear pore complex.84 They were able to track selective protein binding inside pores down to 50 nm in diameter.
Probing nanoparticle–membrane interactions
Besides biomolecular interactions, biologically interfaced nanoplasmonic sensors can also be utilized to study the interaction of inorganic nanoparticles with cellular membranes. For example, we introduced negatively-charged silica nanoparticles to an SLB-coated nanodisk array and discovered a slight variation in the nanoparticle–SLB interaction depending on the composition and effective charge of the SLB.85 The interaction was tracked in real-time and an uneventful, monotonic adsorption of the nanoparticles was observed on zwitterionic SLB. Interestingly, on positively charged SLB, lipid transfer from the SLB to the nanoparticle surface occurred shortly prior to adsorption. The lipid transfer process was detected by the transient change in spatial proximity of the lipid molecules, as indicated by a decrease in the LSPR-tracked nanoparticle adsorption rate. This work clearly demonstrates the benefit of high spatiotemporal resolution in identifying short-lived processes that would otherwise be missed on conventional surface-based measurement platforms.
Taken together, it is important to understand the relationship between nanostructure geometry and plasmonic property, and appreciate how certain geometries employed in combination with a range of coating materials and biological interfaces are beneficial for a particular set of applications (Table 1).
Table 1 Representative list of nanoplasmonic transducers used in recently developed biologically interfaced nanoplasmonic sensors, along with the respective plasmonic properties including bulk refractive index sensitivity (SB) and figure or merit (FoM), as well as the sensor applications
Nanoplasmonic structure, arrangement |
Coating material (active layer) |
Plasmonic feature, λ (nm) |
S
B (nm RIU−1) |
FoMa (RIU−1) |
Biological interface |
Demonstrated application |
Ref. |
The bulk FoM values were not provided in the original works and are therefore presented herein as estimated values based on the published extinction and scattering spectra.
This is a peak from a darkfield scattering spectrum; all other peaks and dips presented in this table are taken from extinction spectra based on measurements conducted in transmission configuration.
|
Au nanodisk, random ensemble |
SiO2 |
Peak, 720 |
110 |
2 |
SLB |
Assessing membrane–surface interactions |
74
|
Investigating effects of membrane curvature |
82
|
Probing nanoparticle–membrane interactions |
85
|
TiO2 |
Peak, 750 |
130 |
2 |
Lipid vesicles |
Assessing membrane–surface interactions |
74
|
Au nanoribbon, periodic array |
In2O3 |
Dip 1, 503 |
510 |
10 |
Lipid vesicles |
Assessing membrane–surface interactions |
39
|
Peak 1, 559 |
Not reported |
— |
Dip 2, 581 |
Not reported |
— |
Peak 2, 649 |
60 |
0.8 |
Au nanorod, single particle |
None |
Peakb, 670 |
183 |
3 |
SLB |
Tracking biomolecular–membrane interactions |
80
|
Au nanowell, random ensemble |
SiO2 |
Peak 1, 675 |
136 |
1 |
SLB |
Investigating effects of membrane curvature |
82
|
Dip 1, 835 |
220 |
1.2 |
Outlook
The successful implementation of surface-based nanoplasmonic sensing platforms to study a broad range of biointerfacial processes highlights the vast potential of biologically interfaced nanoplasmonic sensors as an advanced biophysical characterization tool. At the same time, the opportunity to enhance their quantitative performance and bioanalytical capability becomes limitless. In this regard, refinements in sensing platform design and nanoplasmonic transducer fabrication need to be accompanied by improvements in biological interfacing, and a concerted effort along both fronts rely upon a solid understanding of biophysicochemical interactions at the sensing interface.
Recent advances in nanofabrication technology have paved the way for the construction of plasmonic nanostructures with intricate geometries and using materials beyond the traditional gold and silver. The introduction of plasmonic transducers with exceptionally high aspect ratio, high curvature and sharp edges prompts the need to reassess the robustness of existing surface modification and biointerfacing strategies that have been effective on largely flat surfaces and of which may suffer from reduced adhesion and conformity on highly curved structures. There is also increasing demand to explore new surface chemistries to cater emerging plasmon-active materials such as aluminium,86–88 copper,89,90 graphene91–93 and highly-doped semiconductor nanocrystals.94–96 Along this line, the INPS configuration would be advantageous in conferring chemical stability, especially to materials that are reactive or prone to oxidation such as aluminium and copper.87,90 Conversely, the rapid formation of native oxides necessitates the INPS overcoating step to be performed as part of overall nanostructure fabrication process to ensure that conformal overlayers can be attained controllably on new materials without the formation of undesired intermediate oxide layers. Ultimately, it is important to ensure that biointerfacial processes occur within the region of high nanoplasmonic field intensity. Considering that it is challenging to experimentally measure the nanoplasmonic field intensity, systematic evaluation and optimization of biointerfacing on sensors with new geometries and materials therefore need to be supported by rigorous theoretical and simulation works. Such efforts would greatly facilitate the transference of previously established biointerfacing strategies to nanoplasmonic sensors with novel transducer configurations and expedite their utilization in biointerfacial science applications.
Meanwhile, the growing trend of fabricating supported lipid bilayers as biomimetic coatings without any form of surface modification has led to a paradigm shift in biosensor functionalization. While simplified model membrane coatings with various lipid compositions have been directly fabricated on nanoplasmonic sensors to probe their interactions with the underlying surface, such investigations have so far been limited to lipid bilayers with minimal biomacromolecular insertions and procedures for the construction of more realistic biomimetic coatings need to be established. The methods should preferably be universal and easily implemented across a wide range of metal and oxide surfaces such that various combinations of sensor configuration and model membrane can be adopted in a plug-and-play format. This would offer a wide selection of biologically interfaced sensors from which the most ideal option can be identified to study a particular biomacromolecular interaction of interest.
Regardless of the approach (i.e., with or without surface modification), it is crucial to understand the impact of the biological interface on the measurement response of the nanoplasmonic sensor, especially when research efforts are shifting toward the utilization of biologically interfaced nanosensors for more application-based studies. In other words, it becomes increasingly important to establish an analytical framework for interpreting nanoplasmonic responses arising from biomolecular interactions occurring at the sensing interface. These concerns need to be addressed through both experimental and simulation works, which can unravel and predict the influence of biointerfacing on the measurement response of the nanoplasmonic sensing platform. Such predictions can be further refined with the aid of artificial intelligence and deep learning techniques. With a significant knowledge database and understanding of interactions that occur across different facets of the sensing platform, we can attain greater control over its overall design process and continue to push the analytical boundary of biologically interfaced nanoplasmonic sensors.
Conflicts of interest
There are no conflicts to declare.
Acknowledgements
This work was supported by the National Research Foundation of Korea (NRF) grant funded by the Korean government (MSIT) (No. 2020R1C1C1005523). In addition, this work was supported by the Korea Research Fellowship Program through the National Research Foundation of Korea (NRF) funded by the Ministry of Science and ICT (2019H1D3A1A01070318).
References
- J. A. Jackman and N.-J. Cho, Biointerphases, 2012, 7, 18 CrossRef CAS PubMed
.
- E. Mauriz, P. Dey and L. M. Lechuga, Analyst, 2019, 144, 7105–7129 RSC
.
- K. Ariga, T. Mori and J. Li, Langmuir, 2019, 35, 3585–3599 CrossRef CAS PubMed
.
- A. R. Ferhan, J. A. Jackman, J. H. Park, N.-J. Cho and D.-H. Kim, Adv. Drug Delivery Rev., 2018, 125, 48–77 CrossRef CAS PubMed
.
- J. A. Jackman, A. R. Ferhan and N.-J. Cho, Chem. Soc. Rev., 2017, 46, 3615–3660 RSC
.
- W. D. Wilson, Science, 2002, 295, 2103 CrossRef CAS PubMed
.
- T.-H. Lee, D. J. Hirst, K. Kulkarni, M. P. Del Borgo and M.-I. Aguilar, Chem. Rev., 2018, 118, 5392–5487 CrossRef CAS PubMed
.
- H. H. Nguyen, J. Park, S. Kang and M. Kim, Sensors, 2015, 15, 10481–10510 CrossRef CAS PubMed
.
- D. Wang, F. J. Loo, J. Chen, Y. Yam, S.-C. Chen, H. He, K. S. Kong and P. H. Ho, Sensors, 2019, 19, 1266 CrossRef CAS PubMed
.
- S. G. Kazarian and K. L. A. Chan, Analyst, 2013, 138, 1940–1951 RSC
.
- H. J. Butler, P. M. Brennan, J. M. Cameron, D. Finlayson, M. G. Hegarty, M. D. Jenkinson, D. S. Palmer, B. R. Smith and M. J. Baker, Nat. Commun., 2019, 10, 4501 CrossRef PubMed
.
- N. Pavliček and L. Gross, Nat. Rev. Chem., 2017, 1, 0005 CrossRef
.
- Y. F. Dufrêne, T. Ando, R. Garcia, D. Alsteens, D. Martinez-Martin, A. Engel, C. Gerber and D. J. Müller, Nat. Nanotechnol., 2017, 12, 295–307 CrossRef PubMed
.
- J. A. Jackman, A. R. Ferhan and N.-J. Cho, Bull. Chem. Soc. Jpn., 2019, 92, 1404–1412 CrossRef CAS
.
- J.-F. Masson, Analyst, 2020, 145, 3776–3800 RSC
.
- S.-H. Oh and H. Altug, Nat. Commun., 2018, 9, 5263 CrossRef CAS PubMed
.
- W. Zhou, X. Gao, D. Liu and X. Chen, Chem. Rev., 2015, 115, 10575–10636 CrossRef CAS PubMed
.
- E. Petryayeva and U. J. Krull, Anal. Chim. Acta, 2011, 706, 8–24 CrossRef CAS PubMed
.
- G.-P. Nikoleli, P. D. Nikolelis, G. C. Siontorou, M.-T. Nikolelis and S. Karapetis, Membranes, 2018, 8, 108 CrossRef PubMed
.
- G.-P. Nikoleli, G. C. Siontorou, M.-T. Nikolelis, S. Bratakou and K. D. Bendos, Appl. Sci., 2019, 9, 1745 CrossRef CAS
.
- K. M. Mayer and J. H. Hafner, Chem. Rev., 2011, 111, 3828–3857 CrossRef CAS PubMed
.
- A. B. Dahlin, N. J. Wittenberg, F. Höök and S.-H. Oh, Nanophotonics, 2013, 2, 83–101 CAS
.
- B. Špačková, N. S. Lynn, J. Slabý, H. Šípová and J. Homola, ACS Photonics, 2018, 5, 1019–1025 CrossRef
.
- C. Escobedo, A. G. Brolo, R. Gordon and D. Sinton, Nano Lett., 2012, 12, 1592–1596 CrossRef CAS PubMed
.
- B. Malekian, K. Xiong, E. S. H. Kang, J. Andersson, G. Emilsson, M. Rommel, T. Sannomiya, M. P. Jonsson and A. Dahlin, Nanoscale Adv., 2019, 1, 4282–4289 RSC
.
- H. Xin, B. Namgung and L. P. Lee, Nat. Rev. Mater., 2018, 3, 228–243 CrossRef CAS
.
- N. C. Lindquist, P. Nagpal, K. M. McPeak, D. J. Norris and S.-H. Oh, Rep. Prog. Phys., 2012, 75, 036501 CrossRef PubMed
.
- C. Valsecchi and A. G. Brolo, Langmuir, 2013, 29, 5638–5649 CrossRef CAS PubMed
.
- L. Shao and J. Zheng, Appl. Mater. Today, 2019, 15, 6–17 CrossRef
.
- H. Im, S. H. Lee, N. J. Wittenberg, T. W. Johnson, N. C. Lindquist, P. Nagpal, D. J. Norris and S.-H. Oh, ACS Nano, 2011, 5, 6244–6253 CrossRef CAS PubMed
.
- B. Shen, V. Linko, K. Tapio, S. Pikker, T. Lemma, A. Gopinath, K. V. Gothelf, M. A. Kostiainen and J. J. Toppari, Sci. Adv., 2018, 4, eaap8978 CrossRef PubMed
.
- B. Shen, M. A. Kostiainen and V. Linko, Langmuir, 2018, 34, 14911–14920 CrossRef CAS PubMed
.
- I. Malkiel, M. Mrejen, A. Nagler, U. Arieli, L. Wolf and H. Suchowski, Light: Sci. Appl., 2018, 7, 60 CrossRef PubMed
.
- J. He, C. He, C. Zheng, Q. Wang and J. Ye, Nanoscale, 2019, 11, 17444–17459 RSC
.
- C. Langhammer, E. M. Larsson, B. Kasemo and I. Zorić, Nano Lett., 2010, 10, 3529–3538 CrossRef CAS PubMed
.
- F. A. A. Nugroho, R. Frost, T. J. Antosiewicz, J. Fritzsche, E. M. Larsson Langhammer and C. Langhammer, ACS Sens., 2017, 2, 119–127 CrossRef CAS PubMed
.
- J. Jose, L. R. Jordan, T. W. Johnson, S. H. Lee, N. J. Wittenberg and S.-H. Oh, Adv. Funct. Mater., 2013, 23, 2812–2820 CrossRef CAS
.
- F. A. A. Nugroho, D. Albinsson, T. J. Antosiewicz and C. Langhammer, ACS Nano, 2020, 14, 2345–2353 CrossRef CAS PubMed
.
- C. Zhao, X. Xu, A. R. Ferhan, N. Chiang, J. A. Jackman, Q. Yang, W. Liu, A. M. Andrews, N.-J. Cho and P. S. Weiss, Nano Lett., 2020, 20, 1747–1754 CrossRef CAS PubMed
.
- N. K. Chaki and K. Vijayamohanan, Biosens. Bioelectron., 2002, 17, 1–12 CrossRef CAS PubMed
.
- R. M. Nyquist, A. S. Eberhardt, L. A. Silks, Z. Li, X. Yang and B. I. Swanson, Langmuir, 2000, 16, 1793–1800 CrossRef CAS
.
- E. Reimhult and F. Höök, Sensors, 2015, 15, 1635–1675 CrossRef PubMed
.
- J. J. Richardson, J. Cui, M. Björnmalm, J. A. Braunger, H. Ejima and F. Caruso, Chem. Rev., 2016, 116, 14828–14867 CrossRef CAS PubMed
.
- P. T. Hammond, Adv. Mater., 2004, 16, 1271–1293 CrossRef CAS
.
- A. Baba, M.-K. Park, R. C. Advincula and W. Knoll, Langmuir, 2002, 18, 4648–4652 CrossRef CAS
.
- J. O. Zoppe, N. C. Ataman, P. Mocny, J. Wang, J. Moraes and H.-A. Klok, Chem. Rev., 2017, 117, 1105–1318 CrossRef CAS PubMed
.
- G. Emilsson, K. Xiong, Y. Sakiyama, B. Malekian, V. Ahlberg Gagnér, R. L. Schoch, R. Y. H. Lim and A. B. Dahlin, Nanoscale, 2018, 10, 4663–4669 RSC
.
- A. Hucknall, S. Rangarajan and A. Chilkoti, Adv. Mater., 2009, 21, 2441–2446 CrossRef CAS
.
- J. Tavakoli and Y. Tang, Polymers, 2017, 9, 364 CrossRef PubMed
.
- M. Toma, U. Jonas, A. Mateescu, W. Knoll and J. Dostalek, J. Phys. Chem. C, 2013, 117, 11705–11712 CrossRef CAS PubMed
.
- Y. Wang, C.-J. Huang, U. Jonas, T. Wei, J. Dostalek and W. Knoll, Biosens. Bioelectron., 2010, 25, 1663–1668 CrossRef CAS PubMed
.
- A. J. Haes, S. Zou, G. C. Schatz and R. P. Van Duyne, J. Phys. Chem. B, 2004, 108, 6961–6968 CrossRef CAS
.
- A. V. Whitney, J. W. Elam, S. Zou, A. V. Zinovev, P. C. Stair, G. C. Schatz and R. P. Van Duyne, J. Phys. Chem. B, 2005, 109, 20522–20528 CrossRef CAS PubMed
.
- J. M. Brockman, B. P. Nelson and R. M. Corn, Annu. Rev. Phys. Chem., 2000, 51, 41–63 CrossRef CAS PubMed
.
- M. C. Dixon, J. Biomol. Tech., 2008, 19, 151–158 Search PubMed
.
- H. Im, N. C. Lindquist, A. Lesuffleur and S.-H. Oh, ACS Nano, 2010, 4, 947–954 CrossRef CAS PubMed
.
- F. Mazzotta, T. W. Johnson, A. B. Dahlin, J. Shaver, S.-H. Oh and F. Höök, ACS Photonics, 2015, 2, 256–262 CrossRef CAS
.
- J. Li, J. Ye, C. Chen, Y. Li, N. Verellen, V. V. Moshchalkov, L. Lagae and P. Van Dorpe, ACS Photonics, 2015, 2, 425–431 CrossRef CAS
.
- A. Luchini and G. Vitiello, Front. Chem., 2019, 7, 343 CrossRef CAS PubMed
.
- C. Hélix-Nielsen, Membranes, 2018, 8, 44 CrossRef PubMed
.
- A. J. Jackman, W. Knoll and N.-J. Cho, Materials, 2012, 5, 2637–2657 CrossRef
.
- M. Dacic, J. A. Jackman, S. Yorulmaz, V. P. Zhdanov, B. Kasemo and N.-J. Cho, Langmuir, 2016, 32, 6486–6495 CrossRef CAS PubMed
.
- J. A. Jackman, S. R. Tabaei, Z. Zhao, S. Yorulmaz and N.-J. Cho, ACS Appl. Mater. Interfaces, 2015, 7, 959–968 CrossRef CAS PubMed
.
- J. A. Jackman, Z. Zhao, V. P. Zhdanov, C. W. Frank and N.-J. Cho, Langmuir, 2014, 30, 2152–2160 CrossRef CAS PubMed
.
- J. A. Jackman and N.-J. Cho, Langmuir, 2020, 36, 1387–1400 CrossRef CAS PubMed
.
- G. H. Zan, J. A. Jackman, S.-O. Kim and N.-J. Cho, Small, 2014, 10, 4828–4832 CrossRef CAS PubMed
.
- A. R. Ferhan, B. K. Yoon, S. Park, T. N. Sut, H. Chin, J. H. Park, J. A. Jackman and N.-J. Cho, Nat. Protoc., 2019, 14, 2091–2118 CrossRef CAS PubMed
.
- K. Kolahdouzan, J. A. Jackman, B. K. Yoon, M. C. Kim, M. S. Johal and N.-J. Cho, Langmuir, 2017, 33, 5052–5064 CrossRef CAS PubMed
.
- T. N. Sut, S. Park, Y. Choe and N.-J. Cho, Langmuir, 2019, 35, 15063–15070 CrossRef CAS PubMed
.
- T. N. Sut, J. A. Jackman and N.-J. Cho, Langmuir, 2019, 35, 8436–8444 CAS
.
- K. J. Glover, J. A. Whiles, G. Wu, N.-j. Yu, R. Deems, J. O. Struppe, R. E. Stark, E. A. Komives and R. R. Vold, Biophys. J., 2001, 81, 2163–2171 CrossRef CAS PubMed
.
- J. A. Jackman, S. Yorulmaz Avsar, A. R. Ferhan, D. Li, J. H. Park, V. P. Zhdanov and N.-J. Cho, Anal. Chem., 2017, 89, 1102–1109 CrossRef CAS PubMed
.
- A. R. Ferhan, J. A. Jackman and N.-J. Cho, Anal. Chem., 2017, 89, 4301–4308 CrossRef CAS PubMed
.
- A. R. Ferhan, B. Špačková, J. A. Jackman, G. J. Ma, T. N. Sut, J. Homola and N.-J. Cho, Anal. Chem., 2018, 90, 12503–12511 CrossRef CAS PubMed
.
- O. Limaj, D. Etezadi, N. J. Wittenberg, D. Rodrigo, D. Yoo, S.-H. Oh and H. Altug, Nano Lett., 2016, 16, 1502–1508 CrossRef CAS PubMed
.
- I. Bruzas, S. Unser, S. Yazdi, E. Ringe and L. Sagle, Anal. Chem., 2016, 88, 7968–7974 CrossRef CAS PubMed
.
- A. R. Ferhan, J. A. Jackman and N.-J. Cho, Anal. Chem., 2016, 88, 12524–12531 CrossRef CAS PubMed
.
- J. Witos, G. Russo, S.-K. Ruokonen and S. K. Wiedmer, Langmuir, 2017, 33, 1066–1076 CrossRef CAS PubMed
.
- C. Lambertz, A. Martos, A. Henkel, A. Neiser, T.-T. Kliesch, A. Janshoff, P. Schwille and C. Sönnichsen, Nano Lett., 2016, 16, 3540–3544 CrossRef CAS PubMed
.
- W. Ye, S. Celiksoy, A. Jakab, A. Khmelinskaia, T. Heermann, A. Raso, S. V. Wegner, G. Rivas, P. Schwille, R. Ahijado-Guzmán and C. Sönnichsen, J. Am. Chem. Soc., 2018, 140, 17901–17906 CrossRef CAS PubMed
.
- J. Zhu, J. He, M. Verano, A. T. Brimmo, A. Glia, M. A. Qasaimeh, P. Chen, J. O. Aleman and W. Chen, Lab Chip, 2018, 18, 3550–3560 RSC
.
- A. R. Ferhan, J. A. Jackman, B. Malekian, K. Xiong, G. Emilsson, S. Park, A. B. Dahlin and N.-J. Cho, Anal. Chem., 2018, 90, 7458–7466 CrossRef CAS PubMed
.
- G. Emilsson, E. Röder, B. Malekian, K. Xiong, J. Manzi, F.-C. Tsai, N.-J. Cho, M. Bally and A. Dahlin, Front. Chem., 2019, 7, 1 CrossRef CAS PubMed
.
- B. Malekian, R. L. Schoch, T. Robson, G. Ferrand -Drake del Castillo, K. Xiong, G. Emilsson, L. E. Kapinos, R. Y. H. Lim and A. Dahlin, Front. Chem., 2018, 6, 637 CrossRef CAS PubMed
.
- A. R. Ferhan, J. G. Ma, A. J. Jackman, N. T. Sut, H. J. Park and N.-J. Cho, Sensors, 2017, 17, 1484 CrossRef PubMed
.
- X. Zhu, G. M. Imran Hossain, M. George, A. Farhang, A. Cicek and A. A. Yanik, ACS Photonics, 2020, 7, 416–424 CrossRef CAS
.
- M. W. Knight, N. S. King, L. Liu, H. O. Everitt, P. Nordlander and N. J. Halas, ACS Nano, 2014, 8, 834–840 CrossRef CAS PubMed
.
- V. Liberman, K. Diest, C. W. Stull, M. T. Cook, D. M. Lennon, M. Rothschild and S. Schoeche, ACS Photonics, 2016, 3, 796–805 CrossRef CAS
.
- A. Böhme, F. Sterl, E. Kath, M. Ubl, V. Manninen and H. Giessen, ACS Photonics, 2019, 6, 1863–1868 CrossRef
.
- Y. V. Stebunov, D. I. Yakubovsky, D. Y. Fedyanin, A. V. Arsenin and V. S. Volkov, Langmuir, 2018, 34, 4681–4687 CrossRef CAS PubMed
.
- J. D. Cox and F. J. García de Abajo, Acc. Chem. Res., 2019, 52, 2536–2547 CrossRef CAS PubMed
.
- F. J. García de Abajo, ACS Photonics, 2014, 1, 135–152 CrossRef
.
- D. B. Farmer, P. Avouris, Y. Li, T. F. Heinz and S.-J. Han, ACS Photonics, 2016, 3, 553–557 CrossRef CAS
.
- A. Agrawal, S. H. Cho, O. Zandi, S. Ghosh, R. W. Johns and D. J. Milliron, Chem. Rev., 2018, 118, 3121–3207 CrossRef CAS PubMed
.
- Z. Liu, Y. Zhong, I. Shafei, S. Jeong, L. Wang, H. T. Nguyen, C.-J. Sun, T. Li, J. Chen, L. Chen, Y. Losovyj, X. Gao, W. Ma and X. Ye, Nano Lett., 2020, 20, 2821–2828 CrossRef CAS PubMed
.
- Z. Liu, Y. Zhong, I. Shafei, R. Borman, S. Jeong, J. Chen, Y. Losovyj, X. Gao, N. Li, Y. Du, E. Sarnello, T. Li, D. Su, W. Ma and X. Ye, Nat. Commun., 2019, 10, 1394 CrossRef PubMed
.
|
This journal is © The Royal Society of Chemistry 2020 |
Click here to see how this site uses Cookies. View our privacy policy here.