DOI:
10.1039/D2DD00046F
(Communication)
Digital Discovery, 2022,
1, 755-762
Robotic cell assembly to accelerate battery research†
Received
26th May 2022
, Accepted 6th October 2022
First published on 12th October 2022
Abstract
Manual cell assembly confounds with research digitalization and reproducibility. Both are however needed for data-driven optimization of cell chemistries and charging protocols. Therefore, we present herein an automatic battery assembly system (AutoBASS) that is capable of assembling batches of up to 64 CR2023 cells. AutoBASS allows us to acquire large datasets on in-house developed chemistries and is herein demonstrated with LNO and Si@Graphite electrodes with a focus on formation and manufacturing data. The large dataset enables us to gain insights into the formation process through dQ/dV analysis and assess cell to cell variability. Exact robotic electrode placement provides a baseline for laboratory-scale manufacturing and reproducibility towards the accelerated translation of findings from the laboratory to the pilot plant scale.
Introduction
Optimization of active materials, electrolyte formulations, processing, and manufacturing of secondary batteries along the entire battery research chain1 is a capital-, material-, and time-intensive task.2 Consequently, there is only a limited number of data-driven3–5 studies in battery research on in-house assembled6i.e. non-commercially acquired cells. There is however a necessity for manufacturing larger numbers of cells in a reproducible manner7,8 for the investigation of chemistries before the pilot plant scale1i.e. when transitioning from the μg to g scale. To translate research between labs9 and to pilot production lines there is also a need to have unified ways of describing battery data.10 We, therefore, build the automatic battery assembly system (AutoBASS) as we see a pressing need to accurately and precisely assemble cells to provide a “fail fast”11 decision gate12,13 for new cell chemistries and protocols.14 The intention of is the proliferation of productive and reproducible coin cell manufacturing robots in small scale academic research. The intention is to build a bridge between singular man-made cells to pilot line production. AutoBASS is open source and agile enough that it provides an addition for verification and translation in an academic research context to large scale deployments i.e. its intention is to remove barriers for small batch upscaling instead of creating new ones.
Despite upscaling for demonstration purposes and optimization studies a recent study by Dechent et al.5 suggests that the minimum number of cells to study with models containing 1 or 3 parameters is 8 or 13 respectively to overcome cell-to-cell variability. Considering the many possible parameters that can be changed in the active and inactive materials2,15 a system for lab-scale cell assembly would therefore need to be able to produce large batches of cells with near or exceeding commercial cell reproducibility.5
Overall, there is very limited publicly available data on the cycling behavior of cells, let alone their manufacturing or formation cycle. Typically, either large batches of commercial cells are tested that lack data on formation or data is published5,14 on datasets containing less than 5–8 cells which are made manually.6 A short literature review yields that some emblematic papers in the field of data driven battery research consist of 48 manually assembled cells for coating optimization,6 and 45 commercial cells for early lifetime prediction.14 The entire field seems to be only having publicly available data on less than 500 cells in total.5 A laboratory manufacturing system, as presented herein, that can produce 64 cells in a day could significantly impact the field towards a complete closed-loop discovery cycle16,17 for cell chemistries and processes.
This robot resides at the top range of scale-up in our platform for accelerated electrochemical energy storage research (PLACES/R) as recently published by Stein et al.1 and, to the best of our knowledge, is the first robotic system of its kind for battery research. To foster proliferation of this approach and in as many labs as possible we publish the code and mechanical parts alongside this manuscript with a manual for assembly and operation. We demonstrate the utility of this setup on state-of-the-art Li-ion batteries consisting of LiNiO2 (LNO) cathodes, Si@Graphite composite (Si–C) anodes, and 1 M LiPF6 in 3
:
7 EC
:
EMC by weight solvent-cosolvent ratio electrolyte without additives. To minimize the influence of coating faults and inter-electrode sheet variation, electrodes (LNO from BASF, Si–C Cidetec) were coated at pilot plant coating lines and supplied through the BIG-MAP project. To the best of our knowledge this is the first open large-scale study of this state-of the-art materials combination. The thorough data and materials lineage tracking allows us to obtain FAIR18 guideline compliant dataset.
Methods
Materials preparation
The overall workflow of the herein presented automatic coin cell assembly robot focuses on the production process after electrode coating and electrolyte formulation. Though the system is principally amendable to manufacturing cells with different electrolyte mixtures19,20 and electrodes, the herein presented study uses the same electrolyte (1 M LiPF6 in 3
:
7 EC
:
EMC by weight formulated by Elyte, Germany) and electrodes throughout.
Prior to assembly, all sheet-like components need to be cut. For this we use a disc puncher (AOT Battery, China) that allows manual cutting of several hundred electrodes in an hour. Electrodes were not weighed on purpose to demonstrate the extremely low variability along the entire production process including electrode cutting.
The round anodes in this study were cut using a 15 mm diameter die, separators with a 16 mm die, and cathodes with a 14 mm die. Cathode sheets of LNO (LiNiO2) were supplied by BASF with a manufacturer specified areal loading of 3.1 mA h cm−2. Anode sheets of Si–C were supplied by CIDETEC with a manufacturer specified areal loading of 3.2 mA h cm−2. Active material synthesis, coating, and balancing of these electrodes was performed through the BIG-MAP project and is published in the dissemination project report (http://www.big-map.eu/dissemination/). All electrodes were manufactured in a dry room environment at the respective manufacturing sites. Electrodes were shipped in a sealed dry atmosphere and opened and cut inside a nitrogen filled glovebox. Transfer into the glovebox was performed by placing a small incision onto the sealed bag prior to evacuation in the antechamber. Separators (glass fiber) were additionally dried outside the glovebox at 75 °C overnight. The cut electrodes were placed manually into the corresponding trays shown in Fig. 1. Spacers are double stacked on the trays. All other coin cell parts (Pi-KEM, UK) were washed in an ultrasonic bath filled with isopropanol and then dried in an oven at 75 °C overnight. Placement of the parts in the respective trays was again performed inside the glovebox.
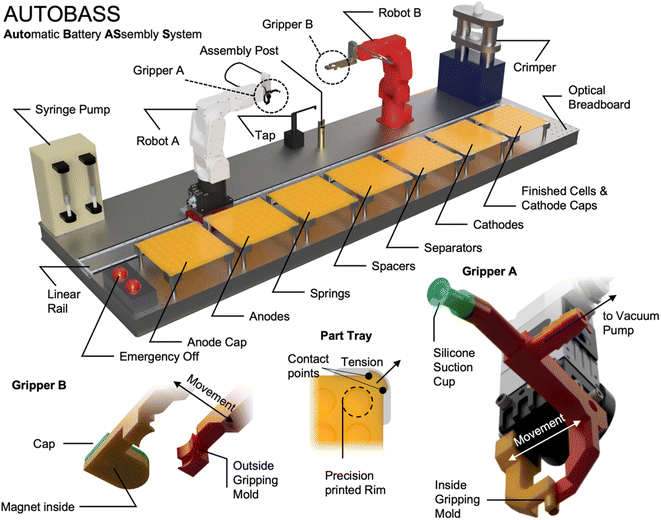 |
| Fig. 1 Schematic rendering of the automatic battery assembly system (AutoBASS) consisting of part trays for assembling CR2023 cells. These parts are namely: anode caps, anodes, springs, spacers, separators, cathodes, and cathode caps. Parts are picked from the trays and placed onto the assembly post by a soft silicone suction cup on gripper attached to robot A. Due to the chute-like shape of coin cell springs, they are picked and placed by a self-rectifying inside gripping mold on gripper A. The precise and accurate placement of parts is ensured by a tensioned and precision 3D printed tray. Cells are assembled with downfacing anode cap and flipped by gripper B which also transfers filled and assembled cells to the crimper. Removal from the crimper is achieved by magnetic pickup (spacers and springs are magnetic). Electrolyte is filled through a computer-controlled syringe pump with a rotating electrolyte tap. Rigid placement is ensured by an optical breadboard, long-range motion is achieved by a precision linear rail. A video of a cell assembly in the glovebox is shown in the ESI.† | |
Robotic CR2023 assembly
All cell components are picked up from trays as shown in Fig. 1 and placed on the assembly post by Robot A (Mecademic Meca500 Rev. 3). As the extent of the trays is much larger than the accessible area of Robot A it is mounted on a precision linear rail (Jenny Science Linax LXS 1800). Components are picked up either through vacuum or mechanic gripping depending on the kind of component as shown in the bottom right and left insets in Fig. 1.
During robot design, dozens of iterations of gripping strategies and method combinations were tested. Empirically we find that vacuum gripping works best for most components but the spring, which is also the recommended placement method by Murray et al.8 For the spring a special inner gripping mechanism was designed as shown in the bottom right of Fig. 1. This mechanism is also used for down tapping motions (see below). Separator static charging due to dry atmosphere vacuum gripping leading to component sticking needs to be compensated. We achieve this by placing Cu single sided adhesive tape into all separator holes in the tray and connecting the thus build metallic surface to ground.
The following description of the lengthy assembly procedure is shown with detailed pictures in the ESI† containing 46 photos of all relevant movement positions including a description on why these movements are executed in that way.
According to the standard procedure of coin cell assembly guidelines and others8 recommended by BIG-MAP, the assembly procedure is started with the anode case facing downwards, therefore, the anode cup is first picked up and placed outer face down on the post. Subsequently, a spacer and an anode are stacked onto each other. Through a computer-controlled syringe pump, connected to a 3D printed rotating electrolyte tap (self-build using a stepper motor and a 3D printed arm in which a PTFE hose is held) the first injection of 15.7 μL electrolyte is dispensed onto the anode. The second electrolyte injection of the same volume is then dispensed after placing of the separator. Images are taken after every placing the anode, separator, and cathode. The rest of the components (second spacer, spring, cathode cap) are stacked onto each other and the cell is closed. To ensure flush closure of the cell, first an alignment tapping motion by gripper B on robot B is performed. Then, using the spring gripper another tapping motion is performed to ensure a flush closing of the cell by a square tapping movement around the center. After this “flush pressing” and aligning, robot B picks up and flips the closed cell with gripper B to transfer it to the crimper. The crimper (MTI MSK-160E, China) is then being triggered by a microcontroller connected relay to start the crimping procedure. The pressure is set through an analog dial to 800 kg. After the crimping tool reverts to its homing position, robot B approaches the crimper again to pick up the finished cell from the die through a magnetic gripping mold on top of its gripper (see Fig. 1) finger to transfer it to the assembly post. By performing a sliding movement on top of the assembly post, the cell is dropped in the assembly post with the cathode cup facing up. Robot A picks up the cell and places the finished cell into the vacant position where the corresponding cathode cup was previously located. In the ESI† we supply a series of videos showing this procedure in detail.
Cycling procedure
We performed cycling of the cells according to the BIG-MAP guidelines which recommend 6 hours of wetting prior to formation. Through the exact timing and logging capabilities of the AutoBASS system we have a second exact tracking of wetting time. Cells were stored anode facing up before start of the formation, cycling was performed with cells being in a vertical position. Battery testing was performed using an Arbin battery tester with 64 channels in laboratory air. Formation was performed by three cycles at a C/20 rate for charge and discharge. In battery research, the current being used to charge/discharge an electrochemical cell is often expressed as a C-rate in order to normalize the charge/discharge rate in relative to its maximum capacity. A 1C rate indicates the amount of current under which a battery will be fully charged/discharged in 1 hour whereas under a C/20 rate it would be 20 hours. Therefore, for a battery with a capacity of 4.77 mA h, which is the theoretical capacity calculated from our material, 1C equates to a discharge current of 4.77 mA and C/20 would be 238.5 μA. The charging constant current (CC) step was stopped at 4.2 V and followed by a constant voltage (CV) step until a current of less than C/50 was observed. The fourth cycle was used to measure cell capacity after formation. Cycling was performed at 1C with a CV step until C/20 currents were observed. Discharging was performed at 1C.
Results and discussion
Assembly process
Placement of electrodes without active image feature recognition is comparably accurate as can be visually assessed in Fig. 2. Measurements of electrode placements are however complicated by the stark and sometimes weak contrast in color between the black anodes facing the camera, white separators wetted by electrolyte, and strongly reflective cathode foil. A facile method to measure the accuracy of electrode placement is taking a horizontal line through the image trying to assess the electrode-to-gasket distance. We find from taking the leftmost minima position to gasket distance variance, the precision of placing the anode is 120 μm, placing the separator is 190 μm, and for placing the cathode is 70 μm. The separators exhibit the largest variance as picking them up results in a slight deformation and sometimes static electrical charging. Dropping the separators then also introduces errors resulting in the fact that two were grossly misplaced (cell in row 1 column 1 and row 6 column 6 see Fig. 2c) and had to be manually readjusted.
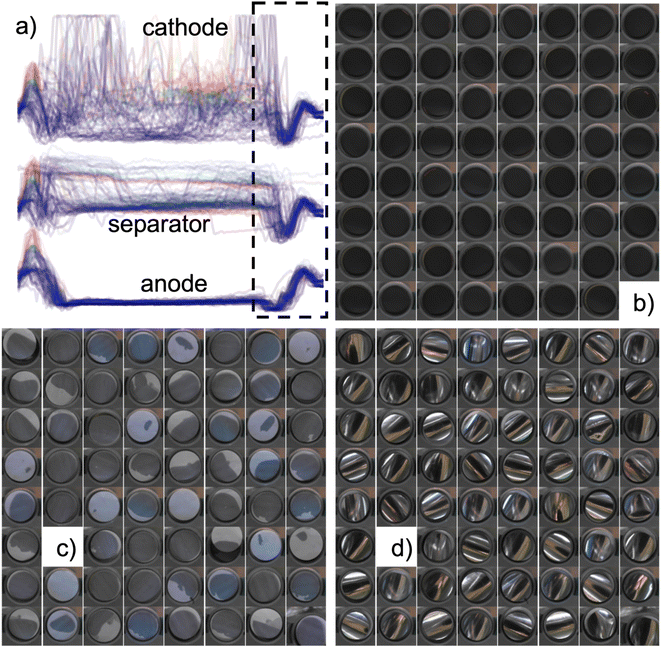 |
| Fig. 2 Analysis and pictures of the first batch of cells produced by AutoBASS. (a) RGB-pixel color values across the center line to assess placement accuracy of the electrodes. Because the anodes are black, there is little color difference to the anode cap. The white and wetted gray separators are well distinguishable to the background and the gasket. The back of the cathode is facing the camera and is highly reflective, but the gap is very well measurable. The variance of the left most minimum (beginning of gap) to the leftmost maximum color (gasket) is 0.12 mm for the anode, 0.19 mm for the separator, and 0.07 mm for the cathode. (b) shows the images after placing the anode, (c) separator (d) cathode. Some separators were greatly misplaced because they exhibited static charging and adhered to the suction cup resulting in a misplacement. Manual intervention was necessary for the two grossly misplaced separators in (c) top left and row 6 column 6. The figure labels are placed at pictures in which the camera failed. | |
It should be noted that the color variations visible in Fig. 2c and d are caused by the separator not being fully wetted and the reflective cathode backside mirroring the glovebox ceiling. The cathodes are all slightly curved due to the thick LNO coating causing the foil to slightly curl. It would be possible to determine the angular mismatch of the curling between anode and cathode, this is however beyond the scope of this manuscript.
Through the exact materials tracking of the entire assembly process from placement to testing, it is even possible to study the influence of wetting time onto some cell parameters. The time from closing the cell to starting the measurement is known with second accuracy. We find that there is no change in open circuit potential (OCP), where OCP refers to the potential between the battery terminals without any load applied. Open circuit potential depends on the battery state of charge, which increases with state of charge, from 3.75–11 h but that cells wetted >15 h exhibit a higher starting potential. All pictures and times measured during the assembly process are part of the accompanying datafile of this manuscript.
Cell-to-cell variation
Besides the different starting open circuit potentials of the two cell batches that were either rested for less or more than 15 hours there is no statistically significant correlation detectable between any of the measured assembly parameters (namely electrode or separator placement, image embeddings, wetting time) and electrochemical data (discharge capacity, dQ/dV data) in the three formation cycles as tested by the maximum information coefficient.21
In total 7 cells exhibited either a short circuit or other electrical issues (i.e. triggering of safety switches in the Arbin MitsPro 8 software). For a conservative assessment of failure rates, these tests were not resumed resulting in a failure rate of 10%. We believe this value to be of great importance as to the best of our knowledge there is no baseline available of how many faulty cells are assembled in laboratories.
The first three formation cycles are performed at a constant current charging with a C-rate of C/20 followed by a constant voltage step at 4.2 V at which the cell is held until the current reaches a C/50 rate. Discharging is performed using a constant current equivalent to a C/20 rate until 2.5 V. The discharge capacity calculated from bootstrapping analysis is 4.16 (4.12, 4.20) mA h with the values in brackets being the 90% confidence interval, the standard variation from bootstrapping is 0.144 mA h or expressed in relative terms 3.4%. From measured cells 90% fall within a 10% relative capacity variation in cycle 4. It should be stressed that all variations expressed herein are relating to the unnormalized discharge capacity i.e. electrodes were not weighted to assess the actual variability across the entire production process from electrode cutting to cell testing. We find that the distribution of Si-particles in the electrode varies to a great extent over the sheet as shown in the ESI† and is likely the main cause of the skewed capacity distribution.
Formation cycles
As is partially already evident from the histograms shown in Fig. 3 of the formation cycles, there is very little cell to cell variation. A method to study small deviations between cells and cycles are differential capacity curves as shown in Fig. 4. During the first formation cycle, as shown in the top left of Fig. 3 there is a significant differential charging variance. This would be indicative, if the peaks were shifted, of different resistances for certain intercalations, which does not seem to be the case. Instead, some peaks are significantly less pronounced or even absent for some cells. This could be indicative of some species on the surface of the electrodes (oxides, hydroxides, different Si-loading as shown in Fig. S1†) that react upon lithium de/intercalation. During the discharge (indicated by the arrows) there are virtually no differences but for a few outlier cells. The large variance during the first charge is likely due to an inhomogeneous distribution of Si-particles in the graphite electrode (see ESI Fig. S1†). The cathode material and likely the resulting cathode electrolyte interphase (CEI) appear to be very homogenous as can be seen in the virtually identical differential discharge capacity curves in Fig. 4. Besides 3 cells that show up in the dQ/dV plots as outliers there seems to be a bimodal distribution of dQ/dV shapes occurring in the second and third formation cycle as indicated by the dashed lines in Fig. 3. These likely correspond to the two qualitative Si-loading regiments as shown in Fig. S1.† There, the anodic shoulders for the first and second formation cycle exhibit some increased variance in the peaks around 3.4 V and in the fourth (capacity test) cycle the peak exhibits a broad variation. The bimodal distribution of dQ/dV curves is most prominently visible in the fourth circle as indicated by the dashed regions II and III. Since the bimodal curve shape distribution is visible on both the charge and discharge data, we believe that these minute variations highlight the necessity to produce larger arrays of cells as they would have otherwise likely not been captured. Comparing the herein acquired data to that found in literature on LNO the region showing increased variance that is denoted by the roman numeral I in Fig. 4 could be attributed to the H1 and H1 to H2 phase transition or more likely to the insertion into Si in the Si–C anode.22 Since there is some considerable variance in Si-particle loading (see Fig. S1†) across the anode sheets we believe this variance to be originating from the electrodes and not necessarily from assembly or randomness. The region marked by II and III is likely de/insertion into the H2 phase.23
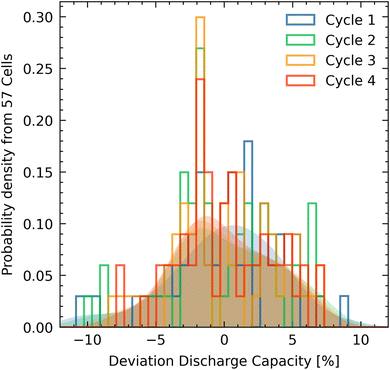 |
| Fig. 3 Distribution of discharge capacity deviation from the median for the respective cycle. Bootstrapping results in a mean of 4.16 (4.12, 4.20) mA h with the values in brackets being the 90% confidence interval, the standard variation from bootstrapping is 0.144 mA h. In the fourth cycle 90% of the cells are within a 10% error range and 60% are within a 5.9% error range. | |
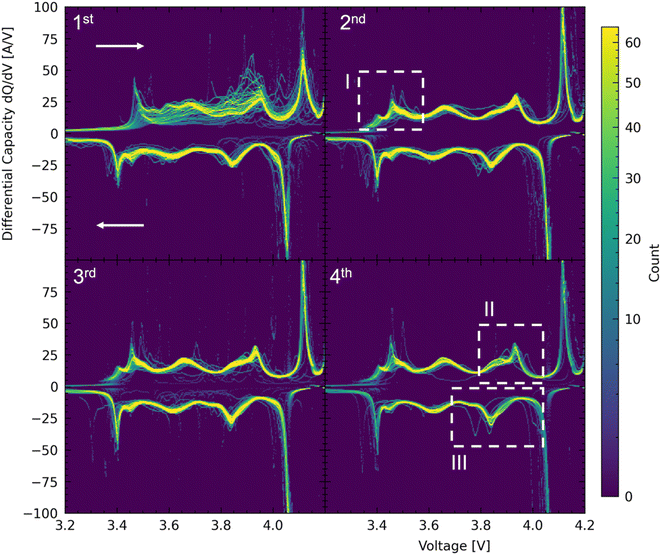 |
| Fig. 4 Differential charge and discharge curves for the first four cycles as hexbin plots. Charging and discharging was performed at a C-rate of C/20 until 4.2 V at which the cell was held until a current of C/50 was reached. Discharging was stopped at 2.5 V. The first charging cycle exhibits some significant variation that is not correlated to any of the available manufacturing data. Except for a few outlier cells all dQ/dV plots are virtually the same. The only region exhibiting some variation which is increasing in cycle 3 and 4 is marked with the roman numeral I. In the fourth cycle a bimodal distribution in the areas marked II and II becomes apparent. | |
Conclusion
Motivated by the necessity to produce larger batches of cells to overcome cell-to-cell variability and a critical need to translate results from the lab to pilot production lines we herein present, to the best of our knowledge, the first automatic coin cell assembly robot. Even without active control in electrode placements the accuracy is 0.07–0.2 mm which is better than any manual assembly in a glovebox could ever be. The variance in unnormalized capacity of the manufactured cells is found by bootstrapping to be only 3.4%.
We show that there is no measurable influence or correlation between our assembly process and the formation behavior of our cells and identify inhomogeneities in the anode foil to be the main cause of error. This robot can also act as a “fail early” decision gate for new concepts and cell chemistries due to its good reproducibility and comparably small amount of material necessary. With the employed method of electrode punching, we can obtain about 100 cells from as little as a 30 cm × 10 cm electrode sheet and gain insight into the statistical variation in cycling behavior very early in the materials discovery process.
We also use the herein manufactured batch to establish a conservative baseline for failed laboratory made cells, which is 10%. We believe to be able to reduce this failure rate even more by metallic vacuum grippers and active image recognition-based electrode placement in the future. The complete data and materials lineage tracking allows us to acquire a comprehensive dataset that can interrelate cell assembly and electrochemistry and believe this technology to be of great importance for the field especially with the transition towards post-Li ion batteries.24 All necessary software and mechanical parts besides bought components are published alongside this manuscript to foster the proliferation of AutoBASS to more laboratories with our hope to establish data driven battery research with new chemistries as greater pace and reproducibility.
Data availability
The software and mechanical parts to build and run AutoBASS can be found at https://github.com/Helge-Stein-Group/AutoBASS as well as in the ESI.† The dataset can be found in the ESI.† A brief description of the data structure can be found in the repository.
Conflicts of interest
There are no conflicts to declare.
Acknowledgements
This work contributes to the research performed at CELEST (Center for Electrochemical Energy Storage Ulm-Karlsruhe) and was partly funded by the Deutsche Forschungsgemeinschaft (DFG, German Research Foundation) under Germany's Excellence Strategy – EXC 2154 – project number 390874152. This project has also received funding from the European Union's Horizon 2020 research and innovation program under grant agreement no. 957189.
References
- H. S. Stein, A. Sanin, F. Rahmanian, B. Zhang, M. Vogler, J. K. Flowers, L. Fischer, S. Fuchs, N. Choudhary and L. Schroeder, From Materials Discovery to System Optimization by Integrating Combinatorial Electrochemistry and Data Science, Curr. Opin. Electrochem., 2022, 101053, DOI:10.1016/j.coelec.2022.101053
.
- J. Amici, P. Asinari, E. Ayerbe, P. Barboux, P. Bayle-Guillemaud, R. J. Behm, M. Berecibar, E. Berg, A. Bhowmik, S. Bodoardo, I. E. Castelli, I. Cekic-Laskovic, R. Christensen, S. Clark, R. Diehm, R. Dominko, M. Fichtner, A. A. Franco, A. Grimaud, N. Guillet, M. Hahlin, S. Hartmann, V. Heiries, K. Hermansson, A. Heuer, S. Jana, L. Jabbour, J. Kallo, A. Latz, H. Lorrmann, O. M. Løvvik, S. Lyonnard, M. Meeus, E. Paillard, S. Perraud, T. Placke, C. Punckt, O. Raccurt, J. Ruhland, E. Sheridan, H. Stein, J. Tarascon, V. Trapp, T. Vegge, M. Weil, W. Wenzel, M. Winter, A. Wolf and K. Edström, A Roadmap for Transforming Research to Invent the Batteries of the Future Designed within the European Large Scale Research Initiative BATTERY 2030+, Adv. Energy Mater., 2022, 2102785, DOI:10.1002/aenm.202102785
.
- J. Ling, M. Hutchinson, E. Antono, S. Paradiso and B. Meredig, High-Dimensional Materials and Process Optimization Using Data-Driven Experimental Design with Well-Calibrated Uncertainty Estimates, Integrating Materials and Manufacturing Innovation, 2017, 6(3), 207–217, DOI:10.1007/s40192-017-0098-z
.
- Z. Wang, Z. Sun, H. Yin, X. Liu, J. Wang, H. Zhao, C. H. Pang, T. Wu, S. Li, Z. Yin and X. Yu, Data-Driven Materials Innovation and Applications, Adv. Mater., 2022, 34, 2104113, DOI:10.1002/adma.202104113
.
- P. Dechent, S. Greenbank, F. Hildenbrand, S. Jbabdi, D. U. Sauer and D. A. Howey, Estimation of Li-Ion Degradation Test Sample Sizes Required to Understand Cell-to-Cell Variability**, Batteries Supercaps, 2021, 4(12), 1821–1829, DOI:10.1002/batt.202100148
.
- S. X. Drakopoulos, A. Gholamipour-Shirazi, P. MacDonald, R. C. Parini, C. D. Reynolds, D. L. Burnett, B. Pye, K. B. O'Regan, G. Wang, T. M. Whitehead, G. J. Conduit, A. Cazacu and E. Kendrick, Formulation and Manufacturing Optimization of Lithium-Ion Graphite-Based Electrodes via Machine Learning, Cell Rep. Phys. Sci., 2021, 2(12), 100683, DOI:10.1016/j.xcrp.2021.100683
.
- J. Hu, B. Wu, S. Chae, J. Lochala, Y. Bi and J. Xiao, Achieving Highly Reproducible Results in Graphite-Based Li-Ion Full Coin Cells, Joule, 2021, 5(5), 1011–1015, DOI:10.1016/j.joule.2021.03.016
.
- V. Murray, D. S. Hall and J. R. Dahn, A Guide to Full Coin Cell Making for Academic Researchers, J. Electrochem. Soc., 2019, 166(2), A329–A333, DOI:10.1149/2.1171902jes
.
-
I. E. Castelli, D. J. Arismendi-Arrieta, A. Bhowmik, I. Cekic-Laskovic, S. Clark, R. Dominko, E. Flores, J. Flowers, K. U. Frederiksen, J. Friis, A. Grimaud, K. V. Hansen, L. J. Hardwick, K. Hermansson, L. Königer, H. Lauritzen, F. L. Cras, H. Li, S. Lyonnard, H. Lorrmann, N. Marzari, L. Niedzicki, G. Pizzi, F. Rahmanian, H. Stein, M. Uhrin, W. Wenzel, M. Winter, C. Wölke and T. Vegge, Data Management Plans: The Importance of Data Management in the BIG-MAP Project, ArXiv210601616 Cond-Mat, 2021.
- S. Clark, F. L. Bleken, S. Stier, E. Flores, C. W. Andersen, M. Marcinek, A. Szczesna-Chrzan, M. Gaberscek, M. R. Palacin, M. Uhrin and J. Friis, Toward a Unified Description of Battery Data, Adv. Energy Mater., 2022, 12(17), 2102702, DOI:10.1002/aenm.202102702
.
- R. van de Krol and B. A. Parkinson, Perspectives on the Photoelectrochemical Storage of Solar Energy, MRS Energy & Sustainability, 2017, 4(1), 13, DOI:10.1557/mre.2017.15
.
- K. Alberi, M. B. Nardelli, A. Zakutayev, L. Mitas, S. Curtarolo, A. Jain, M. Fornari, N. Marzari, I. Takeuchi, M. L. Green, M. Kanatzidis, M. F. Toney, S. Butenko, B. Meredig, S. Lany, U. Kattner, A. Davydov, E. S. Toberer, V. Stevanovic, A. Walsh, N.-G. Park, A. Aspuru-Guzik, D. P. Tabor, J. Nelson, J. Murphy, A. Setlur, J. Gregoire, H. Li, R. Xiao, A. Ludwig, L. W. Martin, A. M. Rappe, S.-H. Wei and J. Perkins, The 2019 Materials by Design Roadmap, J. Phys. D: Appl. Phys., 2019, 52(1), 013001, DOI:10.1088/1361-6463/aad926
.
- A. Bhowmik, I. E. Castelli, J. M. Garcia-Lastra, P. B. Jørgensen, O. Winther and T. Vegge, A Perspective on Inverse Design of Battery Interphases Using Multi-Scale Modelling, Experiments and Generative Deep Learning, Energy Storage Mater., 2019, 21, 446–456, DOI:10.1016/j.ensm.2019.06.011
.
- P. M. Attia, A. Grover, N. Jin, K. A. Severson, T. M. Markov, Y.-H. Liao, M. H. Chen, B. Cheong, N. Perkins, Z. Yang, P. K. Herring, M. Aykol, S. J. Harris, R. D. Braatz, S. Ermon and W. C. Chueh, Closed-Loop Optimization of Fast-Charging Protocols for Batteries with Machine Learning, Nature, 2020, 578(7795), 397–402, DOI:10.1038/s41586-020-1994-5
.
- A. Benayad, D. Diddens, A. Heuer, A. N. Krishnamoorthy, M. Maiti, F. L. Cras, M. Legallais, F. Rahmanian, Y. Shin, H. Stein, M. Winter, C. Wölke, P. Yan and I. Cekic-Laskovic, High-Throughput Experimentation and Computational Freeway Lanes for Accelerated Battery Electrolyte and Interface Development Research, Adv. Energy Mater., 2021, 2102678, DOI:10.1002/aenm.202102678
.
- M. M. Flores-Leonar, L. M. Mejía-Mendoza, A. Aguilar-Granda, B. Sanchez-Lengeling, H. Tribukait, C. Amador-Bedolla and A. Aspuru-Guzik, Materials Acceleration Platforms: On the Way to Autonomous Experimentation, Curr. Opin. Green Sustainable Chem., 2020, 25, 100370, DOI:10.1016/j.cogsc.2020.100370
.
- F. Häse, L. M. Roch and A. Aspuru-Guzik, Next-Generation Experimentation with Self-Driving Laboratories, Trends Chem., 2019, 1(3), 282–291, DOI:10.1016/j.trechm.2019.02.007
.
- M. D. Wilkinson, M. Dumontier, I. J. Aalbersberg, G. Appleton, M. Axton, A. Baak, N. Blomberg, J.-W. Boiten, L. B. da Silva Santos, P. E. Bourne, J. Bouwman, A. J. Brookes, T. Clark, M. Crosas, I. Dillo, O. Dumon, S. Edmunds, C. T. Evelo, R. Finkers, A. Gonzalez-Beltran, A. J. G. Gray, P. Groth, C. Goble, J. S. Grethe, J. Heringa, P. A. C. t Hoen, R. Hooft, T. Kuhn, R. Kok, J. Kok, S. J. Lusher, M. E. Martone, A. Mons, A. L. Packer, B. Persson, P. Rocca-Serra, M. Roos, R. van Schaik, S.-A. Sansone, E. Schultes, T. Sengstag, T. Slater, G. Strawn, M. A. Swertz, M. Thompson, J. van der Lei, E. van Mulligen, J. Velterop, A. Waagmeester, P. Wittenburg, K. Wolstencroft, J. Zhao and B. Mons, The FAIR Guiding Principles for Scientific Data Management and Stewardship, Sci. Data, 2016, 3, 160018–160019, DOI:10.1038/sdata.2016.18
.
-
E. Flores, C. Wölke, P. Yan, M. Winter, T. Vegge, I. Cekic-Laskovic and A. Bhowmik, Learning the Laws of Lithium-Ion Electrolyte Transport Using Symbolic Regression, 2022, DOI: DOI:10.26434/chemrxiv-2022-nmmd4.
-
A. N. Krishnamoorthy, C. Wölke, D. Diddens, M. Maiti, Y. Mabrouk, P. Yan, M. Grünebaum, M. Winter, A. Heuer and I. Cekic-Laskovic, Data-Driven Analysis of High-Throughput Experiments on Liquid Battery Electrolyte Formulations: Unraveling the Impact of Composition on Conductivity, 2022, DOI: DOI:10.26434/chemrxiv-2022-vbl5d.
- D. N. Reshef, Y. A. Reshef, H. K. Finucane, S. R. Grossman, G. McVean, P. J. Turnbaugh, E. S. Lander, M. Mitzenmacher and P. C. Sabeti, Detecting Novel Associations in Large Data Sets, Science, 2011, 334(6062), 1518–1524, DOI:10.1126/science.1205438
.
- L. J. Krause, T. Brandt, V. L. Chevrier and L. D. Jensen, Surface Area Increase of Silicon Alloys in Li-Ion Full Cells Measured by Isothermal Heat Flow Calorimetry, J. Electrochem. Soc., 2017, 164(9), A2277–A2282, DOI:10.1149/2.0501712jes
.
- F. Wu, N. Liu, L. Chen, Y. Su, G. Tan, L. Bao, Q. Zhang, Y. Lu, J. Wang, S. Chen and J. Tan, Improving the Reversibility of the H2-H3 Phase Transitions for Layered Ni-Rich Oxide Cathode towards Retarded Structural Transition and Enhanced Cycle Stability, Nano Energy, 2019, 59, 50–57, DOI:10.1016/j.nanoen.2019.02.027
.
- R. Dugas, J. D. Forero-Saboya and A. Ponrouch, Methods and Protocols for Reliable Electrochemical Testing in Post-Li Batteries (Na, K, Mg, and Ca), Chem. Mater., 2019, 31(21), 8613–8628, DOI:10.1021/acs.chemmater.9b02776
.
|
This journal is © The Royal Society of Chemistry 2022 |
Click here to see how this site uses Cookies. View our privacy policy here.