DOI:
10.1039/C7RA01376K
(Paper)
RSC Adv., 2017,
7, 22133-22144
Delving into Zika virus structural dynamics – a closer look at NS3 helicase loop flexibility and its role in drug discovery†
Received
2nd February 2017
, Accepted 12th April 2017
First published on 20th April 2017
Abstract
The Zika virus has emerged as a pathogen of major health concern. The rapid spread of the virus has led to uproar in the medical domain as scientists frantically race to develop effective vaccines and small molecules to inhibit the virus. In the past year, there has been a flood of Zika knowledge published including its characteristics, transmission routes and its role in disease conditions such as microcephaly and Gullian–Barŕe syndrome. Targeted therapy against specific viral maturation proteins is necessary in halting the replication of the virus in the human host, thus decreasing host–host transmission. This prompted us to investigate the structural properties of the Zika NS3 helicase when bound to ATP-competitive inhibitor, NITD008. In this study, comparative molecular dynamic simulations were employed for APO and bound protein to demonstrate the molecular mechanism of the helicase. Results clearly revealed that NITD008-binding caused significant residue fluctuations at the P-loop compared to the rigid nature of the APO conformation. The NITD008-helicase complex also revealed residues 339–348 to transition from a 310-helix to a stable α-helix. These protein fluctuations were verified by investigation of dynamic cross correlation and principal component analysis. The fundamental dynamic analysis presented in this report is crucial in understanding Zika NS3 helicase function, thereby giving insights toward an inhibition mechanism. The information reported on the binding mode at the ATPase active site may also assist in designing effective inhibitors against this detrimental viral target.
1 Introduction
The re-emerging Zika virus (ZIKV) has evolved into a catastrophic epidemic over the past year, with scientific community announcing that the long-term effects associated with the virus will have to be dealt with in the decades to follow.1 The virus was declared an international public health emergency by the World Health Organization,2 based on growing evidence of the virus being linked with congenital neurological diseases such as Guillain–Barŕe, cranial nerve dysfunction and microcephaly.3,4 The ZIKV made its devastating re-appearance in Brazil and has now spread on a global scale, with an estimated 75 countries with reported mosquito-borne ZIKV transmission as of December 2016.5
Zika virus is an arthropod-borne Flavivirus initially discovered in the Zika forest area of Uganda in 1947.6 Of the Flavivirus genera, ZIKV is most closely related to the Spondweni virus from the Spondweni group; however, ZIKV shares structural similarities with other Flaviviruses, including Dengue virus and West Nile virus.7 The ZIKV genome is made up of structural proteins, being the capsid, precursor membrane and envelope form the viral particle and seven non-structural proteins, being NS1, NS2A, NS2B, NS3, NS4A, NS4B and NS5, which participate in the replication of the RNA genome, virion assembly and invasion of the innate immune system.8–10 In our previous review, we explicated on the key viral target proteins, including the multifunctional viral replication NS3 helicase protein.11 The ZIKV helicase comes from the superfamily helicases, SF2,12 with the inhibition of either one of the binding sites, the RNA-binding groove or the ATP-binding site (Fig. 1), leading to the virus becoming incapable of sufficient maturation and replication. The structural characteristics of the ZIKV NS3 protein includes three domains: domain I (residues 182–327), domain II (residues 328–480) and domain III (residues 481–617), as well as a P-loop (residues 196–203) which is located at the ATP-binding site of domain I.12,13
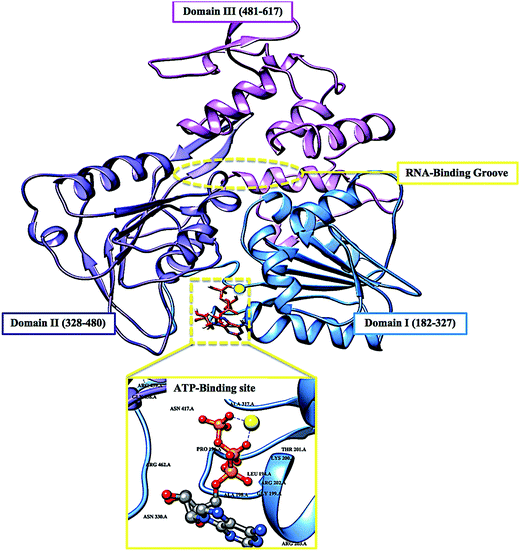 |
| Fig. 1 Cartoon and surface representation of the three domains of the ZIKV helicase and the two active-binding regions (yellow) that form profound hydrophobic cavities in the electrostatic surface area, allowing ATP and ssRNA to bind. | |
The co-crystallization of MnATP2− and RNA with ZIKV helicase, reported by Tian et al. (2016) and Cao et al. (2016), have paved the way to understanding the mechanism by which these substrates bind to the enzyme, initiating viral RNA replication.14,15 Despite the flood of integrated knowledge on ZIKV over the past year, the molecular and structural mechanism for helicase inhibition is yet to be established.12
Another battle being fought by researchers is the discovery of new modes of transmission of the virus, from initially being transmitted from vector to host, to now being inclusive of blood transfers from host to host as well as secondary sexual transmission.16–18 This has allowed for rapid diffusion of the virus between continents. In the plethora of strategic characteristics of the virus, its ability to target neuronal cells has been one of the most problematic tasks that pharmaceutical chemists have had to overcome.19–24 The design of ZIKV inhibitors will not only need to be target-specific, effective and have minimal toxicity, but it will also have to pass through the blood-brain-barrier.25
Although there are currently vaccine clinical trials under way, there are still no FDA approved small molecule inhibitors against the virus.26–30 This may be due to a number of reasons including time-consuming experimental testing of large libraries of compounds or minimal literature available on the functionality of the virus in host cells. These possible barriers have prompted us to utilize computational drug design tools, such as molecular dynamic (MD) simulations to explore the conformational landscape of this biological system's ATP-binding region. The crystallographic structures have revealed evidence of residue mobility, including the rotation of motor domains, however, the precise structural characteristics of the helicase upon small molecule binding, is yet to be determined.12,31–36
In this study we investigate the conformational changes at the ATP-binding region after a 130 ns MD simulation of the free enzyme state as well as a NITD008-bound complex.37 This study will be critical in understanding how the ZIKV NS3 helicase functions structurally, thus aiding in the design of effective, target-specific inhibitors.
2 Computational methods
2.1 System preparation
The ZIKV NS3 helicase in complex with ATP and a magnesium ion (PDB code: 5GJC)14 was obtained from RSCB Protein Data Bank.38 The 3-D structure of the experimental ZIKV inhibitor, NITD008, was obtained from PubChem39 and prepared on Molegro Molecular Viewer (MMV).40 In the ZIKV crystal structure of the ATP-bound helicase, residues A247-S253 were absent, thus the free enzyme (PDB code: 5JMT)13 was utilized in the docking of NITD008. Deng et al. (2016) reported conclusive in vivo evidence of the inhibition of ZIKV by NITD008. The compound is classified as an adenosine nucleoside analog that competitively inhibits ATP, thus sharing an active site.37
2.2 Molecular docking
Molecular docking is a conventional method in computational chemistry which is utilized in the prediction optimized geometric conformations of a ligand within an appropriate binding site.41 The molecular docking software utilized included Raccoon,42 Autodock Graphical user interface supplied by MGL tools43 and AutoDock Vina44 with default docking parameters. Prior to docking, Gasteiger charges were added to NITD008 and the non-polar hydrogen atoms were merged to carbon atoms. Water molecules were removed and polar hydrogen was added to the crystal structure of the NS3 helicase. NITD008 was then docked into the ATPase binding pocket of the NS3 helicase (by defining the grid box with spacing of 1 Å and size of 32 × 26 × 30 pointing in x, y and z directions). Due to the lack of experimental data describing ZIKV approved inhibitors, validation of molecular docking based on the lowest energy pose becomes unreliable.45 To overcome any experimental bias, the five best conformational poses, based on binding affinities (kcal mol−1), were subjected to MD simulations.
2.3 Molecular dynamic (MD) simulations
Molecular dynamic (MD) simulations provide a robust tool to explore the physical movements of atoms and molecules, thus providing insights on the dynamical evolution of biological systems. The MD simulation was performed using the GPU version of the PMEMD engine provided with the AMBER package, FF14SB variant of the AMBER force field46 was used to describe the protein.
ANTECHAMBER was used to generate atomic partial charges for the ligand by utilizing the restrained electrostatic potential (RESP) and the General Amber Force Field (GAFF) procedures. The leap module of AMBER 14 allowed for addition of hydrogen atoms, as well as Na+ and Cl− counter ions for neutralization to both the APO- and bound system.
Both systems were then suspended implicitly within an orthorhombic box of TIP3P water molecules such that all atoms were within 10 Å of any box edge.
An initial minimization of 2000 steps was carried out with an applied restraint potential of 500 kcal mol−1 Å−2 for both solutes, were performed for 1000 steps using a steepest descent method followed by a 1000 steps of conjugate gradients. An additional full minimization of 1000 steps was further carried out by conjugate gradient algorithm without restrain.
A gradual heating MD simulation from 0 K to 300 K was executed for 50 ps, such that the system maintained a fixed number of atoms and fixed volume, i.e., a canonical ensemble (NVT). The solutes within the system are imposed with a potential harmonic restraint of 10 kcal mol−1 Å−2 and collision frequency of 1.0 ps−1. Following heating, an equilibration estimating 500 ps of the each system was conducted; the operating temperature was kept constant at 300 K. Additional features such as a number of atoms and pressure where also kept constant mimicking an isobaric–isothermal ensemble (NPT). The systems pressure was maintained at 1 bar using the Berendsen barostat.
The total time for the MD simulation conducted was 130 ns. In each simulation the SHAKE algorithm was employed to constrict the bonds of hydrogen atoms. The step size of each simulation was 2 fs and an SPFP precision model was used. The simulations coincided with isobaric–isothermal ensemble (NPT), with randomized seeding, constant pressure of 1 bar maintained by the Berendsen barostat, a pressure-coupling constant of 2 ps, a temperature of 300 K and Langevin thermostat with collision frequency of 1.0 ps−2.
2.4 Post-dynamic analysis
The coordinates of the free enzyme and NITD008 complex were each saved every 1 ps and the trajectories were analyzed every 1 ps using PTRAJ, followed by analysis of RMSD, RMSF and radius of gyration using the CPPTRAJ module employed in AMBER 14 suit.
2.4.1 Binding free energy calculations. Binding free energy calculations is an important end point method that may elucidate on the mechanism of binding between a ligand and enzyme, including both enthalpic and enthropic contributions.47 To estimate the binding affinity of the docked systems, the free binding energy was calculated using the Molecular Mechanics/GB Surface Area method (MM/GBSA).48 Binding free energy was averaged over 15
000 snapshots extracted from the 130 ns trajectory. The free binding energy (ΔG) computed by this method for each molecular species (complex, ligand and receptor) can be represented as: |
ΔGbind = Gcomplex − Greceptor − Gligand
| (1) |
|
ΔGbind = Egas + Gsol − TS
| (2) |
|
Egas = Eint + EvdW + Eele
| (3) |
The term Egas denotes the gas-phase energy, which consist of the internal energy Eint; Coulomb energy Eele and the van der Waals energies EvdW. The Egas was directly estimated from the FF14SB force field terms. Solvation free energy, Gsol, was estimated from the energy contribution from the polar states, GGB and non-polar states, G. The non-polar solvation energy, SA. GSA, was determined from the solvent accessible surface area (SASA), using a water probe radius of 1.4 Å, whereas the polar solvation, GGB, contribution was estimated by solving the GB equation. S and T denote the total entropy of the solute and temperature respectively.
To obtain the contribution of each residue to the total binding free energy profile at the ATPase site, per-residue free energy decomposition was carried out at the atomic level for imperative residues using the MM/GBSA method in AMBER 14 suit.
The system displaying the most favorable binding interaction and energy contributions were subjected to further analysis.
2.4.2 Dynamic cross-correlation analysis (DCC). Dynamic cross correlation is a widespread method in MD simulations in which the correlation coefficients of motions between atoms of a protein may be quantified.49 The dynamic cross correlation between the residue-based fluctuations during simulation was calculated using the CPPTRAJ module incorporated in AMBER 14. The formula used to describe dynamic cross correlation is given below:
The cross-correlation coefficient (Cij) varies within a range of −1 to +1 of which the upper and lower limits correspond to a fully correlated and anti-correlated motion during the simulation process. Where, i and j stands for ith and jth residue respectively and Δri or Δrj represents displacement vectors correspond to ith and jth residue respectively. The generated dynamic cross correlation matrix was constructed in Origin software.
2.4.3 Principal component analysis (PCA). Principal component analysis (PCA) is a covariance-matrix-based mathematical technique that is able to demonstrate atomic displacement and the loop dynamics of a protein.50 Prior to processing the MD trajectories for PCA, the trajectories of the free enzyme (APO) and the NITD008-bound complex (complex) were stripped of solvent and ions using the PTRAJ module in AMBER 14. The stripped trajectories were then aligned against their corresponding fully minimized structures. PCA was performed for C-α atoms on 900 snapshots each. Using in-house scripts, the first two principal components were calculated and the covariance matrices were generated. The first two principal components (PC1 and PC2) generated from each trajectory were averaged for both the free-enzyme and NITD008-complex. The first two principal components (PC1 and PC2) were computed and a 2 × 2 covariance matrix were generated using Cartesian coordinates of Cα atoms. PC1 and PC2 correspond to first two eigenvectors of covariant matrices. Origin software51 was used to construct PCA plots.
3 Results and discussion
3.1 NITD008–NS3 helicase complex
3.1.1 Binding of NITD008 with ZIKV helicase. Research into ZIKV inhibitors has been minimal before 2016. However, NITD008, a Flavivirus adenosine analogue was evidenced, both in vitro and in vivo, to inhibit ZIKV replication. The adenosine nucleoside analogue competes with natural ATP substrates, which are incorporated into the growing RNA chain. By this substitution, NITD008 is incorporated into the RNA chain, thus terminating the RNA elongation and inhibiting ZIKV maturation.37Molecular docking has become a major computational tool that is used to predict the orientation of a ligand at a binding site on the receptor. Results from docking often display multiple predicted orientations of the ligand within the active pocket.52
In this study, NITD008 docked at the ATP-binding site in 6 favorable conformations (Fig. S2–S6†), with the highest binding-affinity being −8.2 kcal mol−1. Scoring functions often attempt to reproduce experimental binding affinities, but most software do not always yield the best prediction. Validation of the docked structure with experimentally known drugs was also not possible due to the lack of FDA inhibitors against ZIKV.45,53,54
In an attempt to improve the binding affinity prediction of NITD008, all 6 predicted complexes were subjected to 130 ns molecular dynamic simulations, allowing for more realistic receptor flexibility in an implicit solvent. Each complex was then analyzed using the accurate, MM/GBSA, free binding energy calculation to determine the most favorable pose of NITD008 at the NS3 ATPase active site.47,55–57
3.1.2 Free energy calculations. The total binding free energy for each of the 6 poses of the NITD008–NS3 helicase complex were calculated using the MM/GBSA approach to better understand the various energy contributions within the binding pocket and assess which binding pose would show the most favorable intermolecular interactions at the helicase active site. Per residue decomposition analysis was also assessed and the residue–ligand interaction network of each pose were depicted as “ligplot” maps (Fig. S2–S6†). Of the six systems, the pose with the highest docking score, −8.2 kcal mol−1, showed the most favorable free binding energy (−55.90 kcal mol−1) supported the molecular docking score, indicating a favorable structural pose of NITD008 at the binding site.The thermodynamic energy contribution of NITD008 to the total binding free energy of the complex surmounts to the stability of NITD008 in the ATP binding pocket and thus the stability of the complex during the simulation. Table 1 summarizes the free binding energy of the system taking into account the energies of the NS3 helicase and NITD008.
Table 1 Summary of free binding energy contributions to the NITD008–NS3 helicase system
|
Energy components (kcal mol−1) |
ΔEvdW |
ΔEelec |
ΔGgas |
ΔGsolv |
ΔGbind |
ZIKV helicase |
−3429.35 ± 30.09 |
−28 758.51 ± 159.37 |
−32 187.86 ± 155.05 |
−5121.93 ± 115.09 |
−37 309.79 ± 71.27 |
NITD008 |
−4.69 ± 0.85 |
18.12 ± 5.27 |
13.43 ± 5.28 |
−221.12 ± 3.35 |
−207.68 ± 3.72 |
Complex |
−37.71 ± 4.12 |
−382.94 ± 28.72 |
−420.64 ± 28.59 |
364.75 ± 22.80 |
−55.90 ± 7.71 |
Fig. 2 represents the residue interaction plot of NITD008 within the active site. The active site residues Gly199, Lys200 and Glu286 formed stable hydrogen bonds with highly electronegative oxygen atoms of NITD008. The residues pocketing NITD008 within the active site included Gly197, Ala198, Gly199, Lys200, Thr201, Arg202, Glu288, Gly415, Asn417 and Arg456.
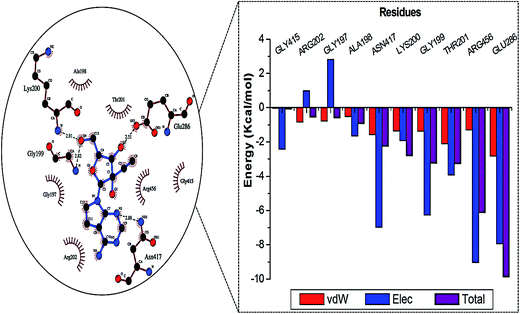 |
| Fig. 2 Energy contributions of the highest interacting residues at the ATPase active site. The residue ligand interaction network illustrates stabilizing hydrophobic interactions pocketing NITD008 at the active site. The highest energy contribution was a hydrogen bond interaction shared between Glu286 and the 3rd oxygen of the ribose component of NITD008. | |
It was also interesting to note that the most favorable NITD008-pose shared five active residues with the ATP-bound helicase reported by Tian et al. (2016). The crystal structure of the ATP-bound helicase showed Lys200 to stabilize the triphosphate of the ATP.14 The Lys200 of the NITD008-bound helicase showed a similar stabilizing hydrogen bond with the terminal hydroxyl group located on the ribose of NITD008.
Superimposition of NITD008-docked NS3 helicase with the ATP–NS3 helicase complex demonstrated both compounds to bind in a hydrophilic conformation despite the carbon and acetylene substitutions at N-7 of the purine and the 2′ position of the ribose, respectively (Fig. 3).
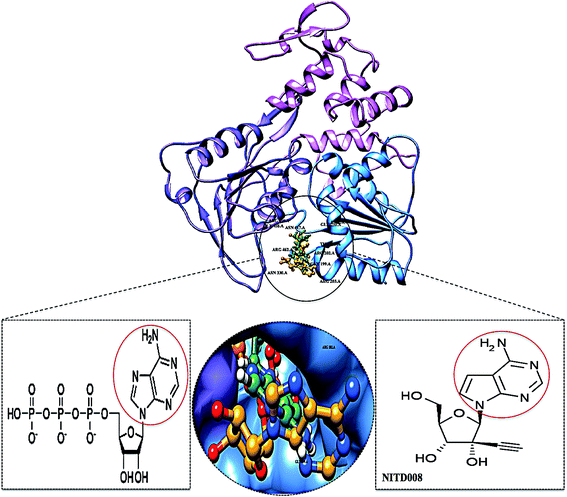 |
| Fig. 3 Superimposed conformation of structurally similar NITD008 and ATP docked at ATPase site of ZIKV NS3 helicase. | |
The structural similarities between NITD008 and ATP, as well as the active site residue interactions and accurate free-binding energy prompted the further analysis of NITD008-complex.
3.2 Systems stability
The length of a MD simulation is paramount when establishing insights into the structural dynamics of a biological system. With an extended simulation time, a system is able to reach convergence, thus becoming stable. To assure the equilibration of the simulation, the potential energy and temperature where monitored (Fig. S1†). The average potential energy (−145
774 kcal mol−1) was measured at 300 K, suggesting a stable conformation at this temperature.
3.2.1 Stability of NS3 helicase APO and bound system. The C-α backbone root mean square deviations (RMSD) were monitored throughout the 130 ns MD simulation for both the free (APO) enzyme and the complex. Both systems reached convergence after 60 ns (RMSD deviation < 2 Å). It can be noted that the C-α backbone atoms in both systems stabilized after a 40 ns time period, although, fluctuations in rigidity did increase during the 47–52 ns period in the NITD008 complex (Fig. 4). This could possibly be due to the occurrence of conformational changes because of the bond interactions taking place between NITD008 and the active site residues as seen in the per-residue energy decomposition.
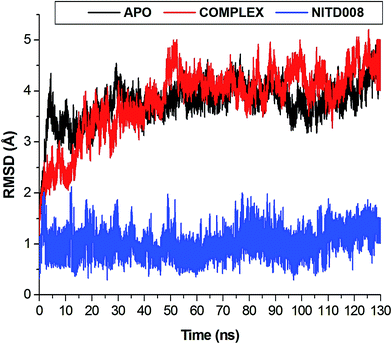 |
| Fig. 4 C-α backbone RMSD for NS3 helicase APO enzyme and NITD-complex conformation. The average C-α RMSD was calculated to be 3.62 Å and 3.77 Å, respectively. Increased fluctuations occurred at 47–52 ns in the NITD008-complex. | |
3.2.2 Conformational fluctuations of the NS3 helicase. To better understand the structural changes that may be occurring upon ligand binding, the root mean square fluctuation (RMSF) of the C-α atoms of each residue in the APO system and NITD008-complex were calculated. Fig. 5 clearly demonstrates greater flexibility of residues of the NITD008-complex when compared to the APO enzyme. Fluctuations take place between residues 198–204, which form distinct hydrophobic and hydrogen bond interactions with NIT008D at the active site. This region, the P-loop, is found in all Flavivirus helicases and has been shown to have flexibility during binding of ATP.14 The P-loop adopts structural modifications to accommodate the binding of ATP and Mn2+. This flexibility extends greatly in comparison to the APO enzyme, thus verifying ZIKV P-loop flexibility upon ligand-binding. Other fluctuations occurred in domain II, and I around the ATP-active site, at residues 244–248 and 325–348.
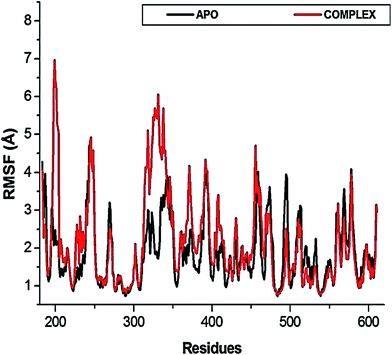 |
| Fig. 5 The RMSF of APO enzyme and NITD008-complex. The structural flexibility in domain I and II is highly attributed to the binding of NITD008 to the ATP-active site. This is substantiated by the average RMSF of the NITD008-complex (2.17 Å), which is significantly higher than that of the APO enzyme (1.90 Å). | |
3.2.3 Distribution of atoms around the NS3 helicase backbone. The radius of gyration around the C-α atoms can measure the shape and folding of NS3 helicase before and after NITD008 binding. The radius of gyration measures the distribution of atoms from the center of mass (COM), thus indicating how compact a system is. Both the APO (22.05 Å) and NITD008 (22.17 Å) showed very similar structural compactness, however, there was an atomic distribution in the NITD008-complex from 40–58 ns (Fig. 6). This correlates with the escalated instability of the complex at 47–52 ns demonstrated in the RMSD plot.
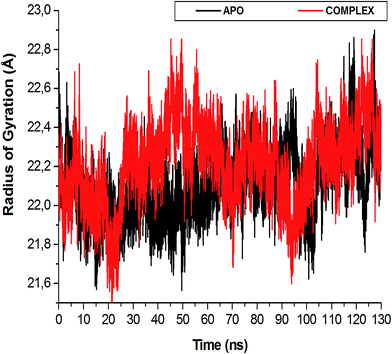 |
| Fig. 6 The radius of gyration (Rg) plot illustrating the difference in enzyme compactness of the NITD008-complex compared to the APO enzyme. | |
The flexibility calculated from the RMSD, RMSF and Rg encouraged us to explore the dynamic structural modifications of the NS3 helicase after NITD008 binding.
3.3 Investigation of the dynamic structural features ATP-active binding region
3.3.1 Loop flexibility and distance metrics. The ZIKV NS3 helicase is made up of three known flexible loops that are common to all Flaviviruses: the P-loop (residues 196–203), the RNA-binding loop (residues 244–255) and the β-hairpin loop (residues 431–444). These loops may vary in size depending on the type of virus; however, they all have the same fundamental structural flexibility. The RMSF plot demonstrated major fluctuations at the P-loop as well as the RNA-binding loop, the β-hairpin loop however, showed no significant conformational change compared to the APO enzyme. The plot also illustrated a flexible “325–338” region. Fig. 7 depicts three snapshots of the APO enzyme and NITD008-complex, taking at different intervals along the trajectory. Clear conformational shifts are illustrated along the trajectory in both APO and bound systems.
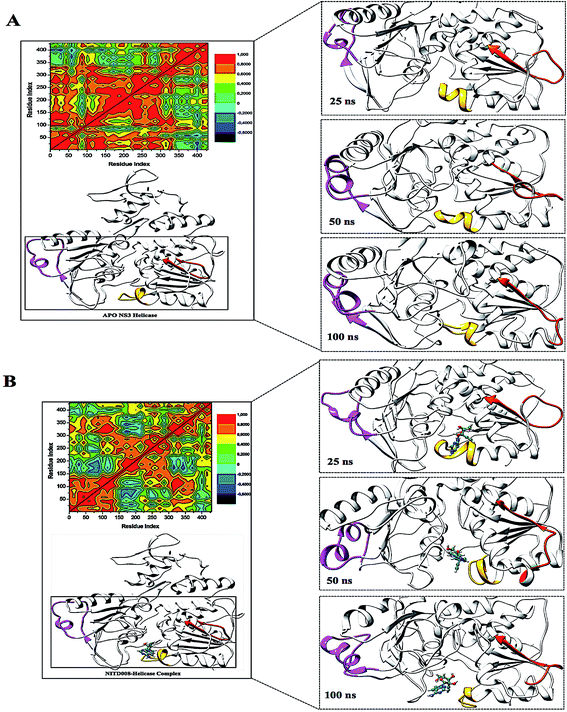 |
| Fig. 7 Structural flexibility of the P-loop (196–203), RNA-binding loop (244–255), and the 310 helix (339–348) along the trajectory. The RNA-binding loop (orange) showed the loop shifting down in the APO structure but an upward shift in the NITD008-helicase complex. The P-loop (yellow) shifted away from the active site in the bound complex but closed in on the active site when no ligand was present. In the APO structure, the helix-loop-helix stayed, with vibrational movement during the simulation, although, in the bound complex, the 310 helix (pink) was modified into a α-helix due to ligand motional shifts further into the hydrophobic pocket. | |
To further investigate the conformational changes of the NS3 helicase upon ligand binding, dynamic cross-correlation matrix (DCCM) analysis was performed at different conformational positions of the Cα backbone atoms of the free protein and ligand-bound complex. Highly correlated motions of residues are represented in the red to yellow regions, whereas, the negative/anti-correlated movements of residue Cα atoms are represented by blue-navy regions. It is evident from the correlation map that more globally correlated motion is observed in the case of the free protein, confirming conformational shifts after ligand binding. The latter residues of the NS3 helicase, being residues 500–600, displayed anti-correlated movements in both the APO and bound complex, supporting the residue fluctuations in Fig. 5. Fig. 7 also depicts anti-correlation motions at residues “340–390”, which may be explained by the snapshots, in which, the flexible region in the NITD008-bound complex was converted from a 310-helix to a α-helix.
The P-loop clearly illustrates that when NS3 helicase is in its APO form and exposed to a 130 ns simulation, the P-loop closes on the active site by uncoiling the α-helix at Arg203 to form part of the loop. The loop tip (Ala198) and the adjacent catalytic residue (Gly451) had an average distance of 9.71 Å compared to the NITD008-complex distance of 12.75 Å, whereby, as NITD008 becomes more stable at the active site and forms bond interactions, the P-loop is directed away from NITD008 and a larger catalytic space becomes available for the ligand as it forms stable hydrophobic interactions deeper within the hydrophobic pocket (Fig. 8).
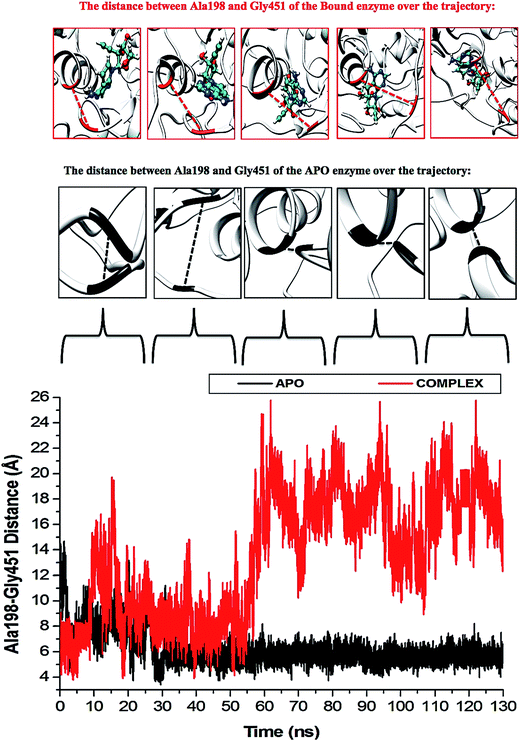 |
| Fig. 8 Residue fluctuations at the P-loop region. The APO enzyme illustrates closing of the loop at the active site due to a vacant hydrophobic pocket. Subsequent to ligand binding and the initiation of stabilizing hydrogen and hydrophobic bond interactions, the P-loop shifts down to accommodate the ligand, thus increasing the size of the hydrophobic pocket. | |
The “325–348” region demonstrates opposing conformational modifications between the APO and complex systems compared to that of the P-loop. The distance between the two catalytic residues from the loop tips; residue Ser324 and residue Asn448, measured for the APO and NITD008-complex was 6.34 Å and 8.34 Å, respectively (Fig. 9). The NITD008-complex had a greater distance between the residues due to the unraveling of 2 β-sheets found in domain II. This led to a “325–338” loop shift behind the active site and the “339–348” region being modified from a 310 helix to a α-helix (Fig. 7). The 310 helix conversion could be due to many reasons including changes in pH, interactions with other proteins and in this case, ligand binding. The ligand–protein interactions lead to distances between nitrogen and oxygen atoms from the protein backbone to fluctuate and as NITD008 moved further into the hydrophobic pocket, these fluctuations and hydrogen bond conversions caused the 310 helix to convert to an α-helix. These changes are important in illustrated the conformational fluctuations upon ligand binding.
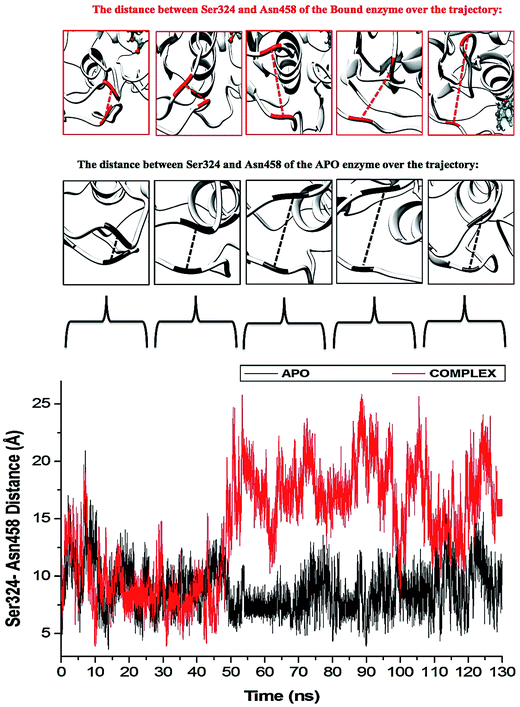 |
| Fig. 9 Residue fluctuations at the “325–348” region. The APO enzyme illustrates widening of the loops of the APO enzyme. The rear loop shifts down as the P-loop closes in on the active site. The largest fluctuation is seen after system stabilization at 40–60 ns. The NITD008-helicase enzyme shows instability in both loops throughout the simulations, although, there was no widening of the loops as the rear loop shifted back rather than downward movement seen in the APO system. | |
3.3.2 Principal component analysis. Conformational transitions of the free protein and NITD008-bound complex were characterized using PCA, a technique that has been widely employed to present experimentally detected conformational variations. Fig. 10 highlights the motional shifts across two principle components in the case of NITD008-bound and unbound NS3 helicase. It is evident that eigenvectors computed from the respective simulations varied immensely between the two systems, further elaborating on the dynamic conformational fluctuations from free to ligand-bound protein. The unbound system shows restricted structural motions of residue Cα atoms, whereby the NITD008-bound system shows a larger spatial occupancy, thus substantiating the rigidity of the unbound system. This corresponds with the stability of the systems, illustrating greater distribution of the atoms around the center of mass and the system stability deviations for the NITD008-bound system. Correlation from analysis of both the free and bound protein demonstrates structural loop flexibility after binding of NITD008 to the ATPase active site.
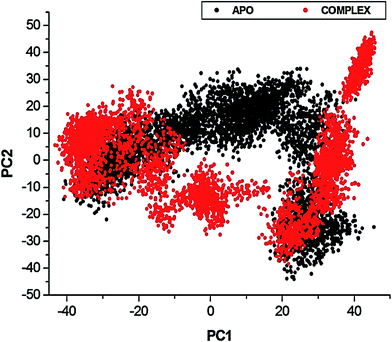 |
| Fig. 10 Projection of Eigen values of the Cα backbone, during 130 ns simulation, for APO and NITD008-bound conformations of NS3 helicase along the first two principal components. The X- and Y-axis, PC1 and PC2, respectively, represent a covariance matrix after elimination of eigenvectors (rotational movements). Each point between the single-directional motions represents a unique conformation during the simulation, whereby, similar structural conformations overlap in the graph. | |
4 Conclusion
The detailed MD analyses provided in this report demonstrate the structural alterations in ZIKV NS3 helicase loop flexibility subsequent to binding of potent inhibitor, NITD008.37 Molecular simulations revealed profound motional shifts of the ZIKV P-loop at the ATPase active site. This flexibility was revealed in the RMSF analysis and verified by graphical investigation of the loop at different time intervals during the simulation. Investigation into the dynamic cross-correlation of the unbound and bound systems as well as a plot of conformational poses along the first two principal components resulted in strongly significant structural flexibility of the NITD008–NS3 helicase system compared to the rigid unbound protein. The P-loop has demonstrates similar motional shifts in other Flaviviruses as well as in ZIKV, when natural substrate, ATP binds at the active site. The competitive inhibitor, NITD008, has been proven to effectively constrain ZIKV replication both in vitro and in vivo. Complex stability measured through the 130 ns simulation showed consistency of NITD008 at the ATPase active site and binding free energy calculations and residue–ligand networks revealed strong stabilizing hydrophobic and hydrogen bond interactions pocketing NITD008 in the active site. Further conformational changes were illustrated by the “325–338” loop shift behind the active site and the “339–348” region being modified from a fluctuating 310 helix to a more stable α-helix.
Crystallographic studies have identified the P-loop, specifically Lys200, to be critical in stabilizing the triphosphate moiety of an NTP, thus allowing flexibility upon ligand binding and activation.12–14 To augment these key findings, Lys200 showed strong hydrogen bonds with the NTP-analogue, NITD008. Other active-hotspot residues included P-loop residues: Gly197–Arg202, Ala198, Glu286, Gly415, Asn417 and Arg456. The insights demonstrating the above binding landscape of the ZIKV NS3 helicase will aid researchers in the identification of targeted-small molecule inhibitors through structure based drug design and to utilize pharmacophore models in screening for effective drugs with minimal toxicity.
Future experimental analysis is needed to fully understand these loop shifts toward inhibition of the enzyme as well as investigations into possible mutational resistance as seen in other Flavivirus helicase NTPase sites.
Acknowledgements
The authors acknowledge the National Research Foundation for their financial support (UID: 102103) and the Center for High Performance Computing (http://www.chpc.ac.za) for their computational resources.
Notes and references
- L. Broxmeyer and R. Kanjhan, Mod. Res. Inflammation, 2016, 5, 20–30 CrossRef.
- Centers for Disease Control, Centers Dis. Control Prev. Zika Virus Home, 2016, 1–12.
- S. A. Rasmussen, D. J. Jamieson, M. A. Honein and L. R. Petersen, N. Engl. J. Med., 2016, 374, 1981–1987 CrossRef CAS PubMed.
- A. M. Palomo, J. Publ. Health. Pol., 2016, 37, 133–135 CrossRef PubMed.
- WHO, Zika Situation Report, 2016, pp. 1–12 Search PubMed.
- O. Faye, C. C. M. Freire, A. Iamarino, O. Faye, J. V. C. de Oliveira, M. Diallo, P. M. Zanotto and A. A. Sall, PLoS Neglected Trop. Dis., 2014, 8, 1–10 Search PubMed.
- R. Tilak, S. Ray, V. W. Tilak and S. Mukherji, Medical Journal Armed Forces India, 2016, 72, 157–163 CrossRef PubMed.
- C. G. Noble, Y. L. Chen, H. Dong, F. Gu, S. P. Lim, W. Schul, Q. Y. Wang and P. Y. Shi, Antiviral Res., 2010, 85, 450–462 CrossRef CAS PubMed.
- M. Mahfuz, A. Khan, H. Al Mahmud, M. Hasan, A. Parvin, N. Rahman and S. M. B. Rahman, Indian J. Pharm. Biol. Res., 2014, 2, 44–57 Search PubMed.
- C. Zanluca, C. N. Duarte and D. Santos, Microbes Infect., 2016, 18, 295–301 CrossRef PubMed.
- P. Ramharack and M. E. S. Soliman, RSC Adv., 2016, 6, 68719–68731 RSC.
- R. Jain, J. Coloma, A. Garcia-Sastre and A. K. Aggarwal, Nat. Struct. Mol. Biol., 2016, 2, 1–4 Search PubMed.
- H. Tian, X. Ji, X. Yang, W. Xie, K. Yang, C. Chen, C. Wu, H. Chi, Z. Mu, Z. Wang and H. Yang, Protein Cell, 2016, 7, 450–454 CrossRef PubMed.
- H. Tian, X. Ji, X. Yang, Z. Zhang, Z. Lu, K. Yang, C. Chen, Q. Zhao, H. Chi, Z. Mu, W. Xie, Z. Wang, H. Lou, H. Yang and Z. Rao, Protein Cell, 2016, 7, 562–570 CrossRef CAS PubMed.
- X. Cao, Y. Li, X. Jin, Y. Li, F. Guo and T. Jin, Nucleic Acids Res., 2016, 44, 10505–10514 CAS.
- E. D'Ortenzio, S. Matheron, X. de Lamballerie, B. Hubert, G. Piorkowski, M. Maquart, D. Descamps, F. Damond, Y. Yazdanpanah and I. Leparc-Goffart, N. Engl. J. Med., 2016, 374, 2195–2198 CrossRef PubMed.
- A. C. Gourinat, O. O. Connor, E. Calvez, C. Goarant and M. Dupont-Rouzeyrol, Emerging Infect. Dis., 2015, 21, 84–86 CrossRef CAS PubMed.
- M. J. Turmel, M. J. P. Hubert, Y. M. V. Maquart, M. Guillou-Guillemette and I. Leparc-Goff, Lancet, 2016, 6736, 2501 CrossRef.
- A. R. Plourde and E. M. Bloch, Emerging Infect. Dis., 2016, 22, 1–15 CrossRef PubMed.
- J.-M. Anaya, C. Ramirez-Santana, I. Salgado-Castaneda, C. Chang, A. Ansari, M. E. Gershwin, R. Martines, J. Bhatnagar, M. Keating, L. Silva-Flannery, A. Muehlenbachs, J. Gary, C. Woods, A. Parker, B. Wakerley, A. Uncini, N. Yuki, J. Anaya, Y. Shoenfeld, A. Rojas-Villarraga, R. Levy, M. Dalakas, B. Wakerley, N. Yuki, S. Kivity, M. Arango, M. Ehrenfeld, O. Tehori, Y. Shoenfeld, J. Anaya, A. Denman, B. Rager-Zisman, T. Kolter, H. Willison, N. Yuki, R. Lardone, N. Yuki, F. Irazoqui, G. Nores, I. Kostovic, R. Ghiulai, M. Sarbu, Z. Vukelic, C. Ilie, A. Zamfir, T. Bell, E. Field, H. Narang, O. Faye, C. Freire, A. Iamarino, O. Faye, J. Oliveira, M. Diallo, A. Nahmias, S. Nahmias and D. Danielsson, BMC Med., 2016, 14, 1–3 CrossRef PubMed.
- N. L. Bayless, R. S. Greenberg, T. Swigut, J. Wysocka and C. A. Blish, Cell Host Microbe, 2016, 20, 423–428 CAS.
- D. Olagnier, M. Muscolini, C. B. Coyne, M. S. Diamond and J. Hiscott, DNA Cell Biol., 2016, 35, 367–372 CrossRef CAS PubMed.
- J. B. Brault, C. Khou, J. Basset, L. Coquand, V. Fraisier, M. P. Frenkiel, B. Goud, J. C. Manuguerra, N. Pardigon and A. D. Baffet, EBioMedicine, 2016, 10, 71–76 CrossRef PubMed.
- H. Li, L. Saucedo-Cuevas, J. A. Regla-Nava, G. Chai, N. Sheets, W. Tang, A. V. Terskikh, S. Shresta and J. G. Gleeson, Cell Stem Cell, 2016, 19, 593–598 CrossRef CAS PubMed.
- T. J. Nowakowski, A. A. Pollen, E. Di Lullo, C. Sandoval-Espinosa, M. Bershteyn and A. R. Kriegstein, Cell Stem Cell, 2016, 18, 591–596 CrossRef CAS PubMed.
- J. Cohen, Science, 2016, 351, 543–544 CrossRef CAS PubMed.
- E. Kim, G. Erdos, S. Huang, T. Kenniston, L. D. Falo and A. Gambotto, EBioMedicine, 2016, 13, 315–320 CrossRef PubMed.
- T. C. Pierson and B. S. Graham, Cell, 2016, 167, 625–631 CrossRef CAS PubMed.
- G. W. A. Dick, S. F. KIitchen and A. J. Haddow, Trans. R. Soc. Trop. Med. Hyg., 1969, 63, 708–737 CrossRef.
- R. W. Malone, J. Homan, M. V. Callahan, J. Glasspool-Malone, L. Damodaran, A. D. B. Schneider, R. Zimler, J. Talton, R. R. Cobb, I. Ruzic, J. Smith-Gagen, D. Janies, J. Wilson, D. Hone, S. Hone, S. Bavari, V. Soloveva and S. Weaver, PLoS Neglected Trop. Dis., 2016, 10, 1–26 Search PubMed.
- A. N. Hazin, A. Poretti, D. Di Cavalcanti Souza Cruz, M. Tenorio, A. van der Linden, L. J. Pena, C. Brito, L. H. V. Gil, D. de Barros Miranda-Filho, E. T. d. A. Marques, C. M. Turchi Martelli, J. G. B. Alves and T. A. Huisman, N. Engl. J. Med., 2016, 374, 2193–2195 CrossRef PubMed.
- N. Gruba, J. I. Rodriguez Martinez, R. Grzywa, M. Wysocka, M. Skorenski, M. Burmistrz, M. Lecka, A. Lesner, M. Sienczyk and K. Pyrc, FEBS Lett., 2016, 590, 3459–3468 CrossRef CAS PubMed.
- B. D. Cox, R. A. Stanton and R. F. Schinazi, Antiviral Chem. Chemother., 2016, 24, 118–126 CrossRef PubMed.
- J. Lei, G. Hansen, C. Nitsche, C. D. Klein, L. Zhang and R. Hilgenfeld, Science, 2016, 353, 503–505 CrossRef CAS PubMed.
- D. Luo, T. Xu, R. P. Watson, D. Scherer-Becker, A. Sampath, W. Jahnke, S. S. Yeong, C. H. Wang, S. P. Lim, A. Strongin, S. G. Vasudevan and J. Lescar, EMBO J., 2008, 27, 32090–33219 CrossRef PubMed.
- A. V. Chernov, S. A. Shiryaev, A. E. Aleshin, B. I. Ratnikov, J. W. Smith, R. C. Liddington and A. Y. Strongin, J. Biol. Chem., 2008, 283, 17270–17278 CrossRef CAS PubMed.
- Y. Q. Deng, N. N. Zhang, C. F. Li, M. Tian, J. N. Hao, X. P. Xie, P. Y. Shi and C. F. Qin, Open Forum Infect. Dis., 2016, 3, 1–4 Search PubMed.
- H. M. Berman, T. Battistuz, T. N. Bhat, W. F. Bluhm, E. Philip, K. Burkhardt, Z. Feng, G. L. Gilliland, L. Iype, S. Jain, P. Fagan, J. Marvin, D. Padilla, V. Ravichandran, N. Thanki, H. Weissig and J. D. Westbrook, Acta Crystallogr., Sect. D: Biol. Crystallogr., 2002, 58, 899–907 CrossRef.
- S. Kim, P. A. Thiessen, E. E. Bolton, J. Chen, G. Fu, A. Gindulyte, L. Han, J. He, S. He, B. A. Shoemaker, J. Wang, B. Yu, J. Zhang and S. H. Bryant, Nucleic Acids Res., 2016, 44, 1202–1213 CrossRef PubMed.
- S. Kusumaningrum, E. Budianto, S. Kosela, W. Sumaryono and F. Juniarti, J. Appl. Pharm. Sci., 2014, 4, 47–53 Search PubMed.
- Z. Yang, K. Lasker, D. Schneidman-Duhovny, B. Webb, C. C. Huang, E. F. Pettersen, T. D. Goddard, E. C. Meng, A. Sali and T. E. Ferrin, J. Struct. Biol., 2012, 179, 269–278 CrossRef CAS PubMed.
- S. Cosconati, S. Forli, A. L. Perryman, R. Harris, D. S. Goodsell and A. J. Olson, Expert Opin. Drug Discovery, 2010, 5, 597–607 CrossRef CAS PubMed.
- M. F. Sanner, Autodock4 and AutoDock-Tools4: automated docking with selective receptor flexibility, Scripps Res. Inst., 2008, vol. 26, pp. 1–12 Search PubMed.
- O. Trott and A. J. Olson, J. Comput. Chem., 2010, 31, 445–461 Search PubMed.
- N. Huang, B. K. Shoichet and J. J. Irwin, J. Med. Chem., 2012, 49, 6789–6801 CrossRef PubMed.
- P. C. Nair and J. O. Miners, In Silico Pharmacology, 2014, 2, 1–4 CrossRef PubMed.
- M. Ylilauri and O. T. Pentikäinen, J. Chem. Inf. Model., 2013, 53, 2626–2633 CrossRef CAS PubMed.
- T. Hou, J. Wang, Y. Li and W. Wang, J. Chem. Inf. Model., 2011, 51, 69–82 CrossRef CAS PubMed.
- V. Gosu and S. Choi, Sci. Rep., 2014, 4, 1–13 Search PubMed.
- A. M. Martinez and A. C. Kak, IEEE Trans. Pattern Anal. Mach. Intell., 2001, 23, 228–233 CrossRef.
- E. Seifert, J. Chem. Inf. Model., 2014, 54, 1552 CrossRef CAS PubMed.
- D. Ramirez and J. Caballero, Int. J. Mol. Sci., 2016, 17, 1–15 Search PubMed.
- X.-Y. Meng, H.-X. Zhang, M. Mezei and M. Cui, Curr. Comput.-Aided Drug Des., 2011, 7, 146–157 CrossRef CAS PubMed.
- L. G. Ferreira, R. N. Dos Santos, G. Oliva and A. D. Andricopulo, Molecular docking and structure-based drug design strategies, 2015, vol. 20 Search PubMed.
- P. A. Greenidge, C. Kramer, J. C. Mozziconacci and R. M. Wolf, J. Chem. Inf. Model., 2013, 53, 201–209 CrossRef CAS PubMed.
- J. M. Hayes and G. Archontis, Zika Virus outside Africa, InTech, 2011, pp. 171–190 Search PubMed.
- F. Godschalk, S. Genheden, P. Söderhjelm and U. Ryde, Phys. Chem. Chem. Phys., 2013, 15, 7731–7739 RSC.
Footnote |
† Electronic supplementary information (ESI) available. See DOI: 10.1039/c7ra01376k |
|
This journal is © The Royal Society of Chemistry 2017 |
Click here to see how this site uses Cookies. View our privacy policy here.