Predicting the phytotoxic mechanism of action of LiCoO2 nanomaterials using a novel multiplexed algal cytological imaging (MACI) assay and machine learning†
Received
9th September 2023
, Accepted 2nd January 2024
First published on 9th January 2024
Abstract
Currently, there is a lack of knowledge of how complex metal oxide nanomaterials, like LiCoO2 (LCO) nanosheets, interact with eukaryotic green algae. Previously, LCO was reported to cause a number of physiological impacts to Raphidocelis subcapitata including endpoints related to growth, reproduction, pigment & lipid biosynthesis, and carbon biomass assimilation. Furthermore, LCO was proven to physically enter the cells, thus indicating the possibility for it to directly interact with key subcellular components. However, the mechanisms through which LCO interacts with these key subcellular components is still unknown. This study assesses the interactions of LCO at the biointerface of R. subcapitata using a novel multiplexed algal cytological imaging (MACI) assay and machine learning in order to predict its phytotoxic mechanism of action (MoA). Algal cells were exposed to varying concentrations of LCO, and their phenotypic profiles were compared to that of cells treated with reference chemicals which had already established MoAs. Hierarchical clustering and machine learning analyses indicated photosynthetic electron transport to be the most probable phytotoxic MoA of LCO. Additionally, single-cell chlorophyll fluorescence results demonstrated an increase in irreversibly oxidized photosystem II proteins. Lastly, LCO-treated cells were observed to have less nuclei/cell and less DNA content/nucleus when compared to non-treated cell controls.
Environmental significance
Complex metal oxide nanomaterials, like LiCoO2, are among some of the most widely produced nanomaterials in commerce, yet there is still little information on how they may interact with algae and other plant-type organisms. Furthermore, in general, there is a need for more non-targeted, high-throughput profiling assays that can quickly and effectively characterize nanomaterial mechanisms of action in environmentally relevant organisms, like algae. This study applies a novel phenotypic profiling approach for predicting the mechanisms through which nanomaterials, like LiCoO2, interact at the biointerface of plant-type organisms in a way that is quick, efficient, and cost-effective. Using this approach, it was found that the most probable mechanism of action of LiCoO2 in algae is that of photosynthetic electron transport inhibition.
|
Introduction
As the number of engineered nanomaterials found in the environment and commerce expands, understanding the breadth of their environmental consequences is a challenge we currently face. In particular, a class of nanomaterials we need more toxicological data on are complex metal oxide nanomaterials, like LiCoO2 (LCO) nanosheets due to their high volume of production and presence in commerce.1 LCO is one of the most commonly used cathode materials in rechargeable Li-ion batteries2 and can be found in a multitude of consumer electronics from computers and smart phones to high-end electric vehicles. As such, the annual production rate for LCO has skyrocketed to levels of environmental significance in recent years.1 What makes this matter particularly concerning, however, is that there is little to no infrastructure for recycling or for properly disposing of LIB's, nor is there any economic incentive to do so as it is cheaper to simply manufacture new battery materials.1 For example, it is currently estimated that less than 5% of all LIB's are being recycled, with the rest ending up in landfills or being disposed of in other mean of un-sustainable storage.3 As a result, this means that there is a high probability for LCO to be unintendedly released into the environment, thus posing an ecological risk. And what exacerbates this risk is that LCO contains high-valence metals with unique catalytic properties, high reactivity, and known inherent toxicity.4,5 Thus, understanding the impacts of LCO to a wide diversity of environmentally relevant organisms across multiple trophic levels is of paramount importance. Current research has been done to assess LCO's toxicological impacts and mechanism of action (MoA) in higher animal-type organisms. For example, Curtis et al., 20226 has reported LCO to cause a differential expression in electron transport and energy metabolism related genes across different fish and invertebrate species. Furthermore, Niemuth et al., 20207 has reported LCO to participate in redox reactions and alter the redox state of and Fe–S proteins, which are important for electron transport. However, our understanding of LCO's impacts to plant-type organisms is still limited.
In a previous study, LCO was reported to cause a number of physiological impacts to Raphidocelis subcapitata, such as reduced growth, altered pigment biosynthesis, and impaired photosynthetic productivity.8 Furthermore, LCO nanoparticles were proven to physically enter the algal cells, thus implying that they undergo direct interactions with key subcellular compartments.8 However, our understanding of the mechanisms governing these consequences is still unclear. Given that photosynthesis was impacted in algae and its reverse reaction, respiration, has also been reportedly impacted in higher animal species,6 it's likely that they may experience similar mechanistic disturbances. For example, key photosynthetic proteins like photosystem II (PSII), which facilitate electron flow in chloroplasts, could be impacted.
In order to assess the interactions of LCO at the biointerface of algal cells, a multiplexed algal cytological imaging (MACI) assay in combination with data mining and machine learning techniques can be used. MACI, as described in a previous study by Ostovich & Klaper, 2023,9 is a type of high-throughput phenotypic profiling assay which involves the use of fluorescence cytochemistry to visualize multiple subcellular structures within the cell, and automated high-content fluorescence imaging to take hundreds of snapshots of their morphology in a consistent manner. In general, high-content phenotypic profiling works off the principal that the morphology of a cell is very sensitive to environmental cues10 and that subtle, yet reproducible, alterations to subsets of morphological features can be used as a framework to characterize compound-specific interactions and predict MoAs of novel compounds when comparing their phenotypic profiles to that of compounds with established MoAs.11 Additionally, image-based profiling assays provide the advantage of speed and cost effectiveness when compared to transcriptomic- and proteomic-based profiling assays.12,13 However, MACI, in the context of eco- and phytotoxicological research, provides an additional benefit compared to other mainstream high-throughput phenotypic profiling assays, like Cell Painting,14 in that it relies on the fluorescent labeling of subcellular structures that are unique to the architecture of algal cells, as opposed to human/animal cells. This is beneficial in terms of phytotoxicological research as it would allow researchers to accurately characterize the MoAs that are unique to plant-type organisms, especially MoAs that target the chloroplast (not present in animal cells). Additionally, MACI could be particularly useful in terms of environmental relevance as plant-type organisms, like microalgae, on average, constitute the largest amount of biomass in the environment and drive environmental processes that affect ecosystem dynamics.15,16 Therefore data obtained from MACI could potentially be used to make predictions that are tied to larger ecosystem level consequences.
In this study, algal cells were exposed to LCO for 24 hours, and MACI was used to characterize subcellular changes in their phenotype with the goal of understanding LCO-algal interactions. R. subcapitata, was chosen as the model organism in this study as this particular algal species is a US EPA established model for environmental toxicology,17 as well as an important bioindicator species for monitoring water quality.18 In addition, it maintains its unicellular nature under stress, which is ideal for downstream segmentation in bioimage analyses. In comparison, other common types of microalgae, like Chlamydomonas spp. or Scenedesmus spp., could potentially form colonies or coenobia in response to environmental stressors,19,20 which would make it harder to distinguish individual cells from one another. Lastly, R. subcapitata was also chosen in order to make direct connections to previous algal-based LCO studies in the literature, which also used this algal species as a toxicological model organism.8 The phenotypic profiles of LCO-treated cells were compared to several reference compounds with established MoAs to predict the phytotoxic MoA of LCO. These reference compounds were chosen to represent MoAs that have been reported for other nanomaterials like membrane disruption,21 DNA damage,22 and more. The similarities between LCO and reference compound profiles were evaluated using hierarchical clustering based on Euclidean distance. An additional deep learning convolutional neural network (CNN) approach was also used to characterize the MoA experienced in individual cells as a means to predict LCO's phytotoxic MoA.
Materials and methods
LCO synthesis and characterization
LixCoO2 nanosheets were synthesized using techniques described in previous studies.8,23,24 18.2 MΩ cm−1 water was used for each step during the synthesis. A (Co(OH)2) precursor was prepared using a precipitation reaction between LiOH and Co(NO3)2·6H2O. 1 M Co(NO3)2·6H2O was added to a 0.1 M solution of LiOH, drop-by-drop. The precipitate was isolated and washed with 3 cycles of centrifugation for 5 min at 4696 g in order to isolate a pellet of particles, and then resuspended in water. Next, the supernatant was removed after washing and the solid product was dried in a vacuum oven at 30 °C overnight. The Co(OH)2 precursor was then lithiated to form LixCoO2 by adding 0.20 g Co(OH)2 particles to a molten salt flux of 6
:
4 molar ratio of LiNO3
:
LiOH at 200 °C in a PTFE container equipped with magnetic stirring in a silicone oil bath. The particles were heated and stirred in this molten salt flux for 30 min and the reaction was quenched with water. The precipitate was isolated and washed by 3 cycles of centrifugation for 5 min at 4696 g to isolate a pellet of particles, and were then resuspended in water. Then the product was isolated from the supernatant and dried in a vacuum oven at 30 °C overnight. The particles, which were digested in aqua regia, were analyzed using inductively coupled plasma – optical emission spectroscopy (ICP-OES) to yield a Li
:
Co ratio of 0.92
:
1. Surface area measurements, determined by nitrogen physisorption, yielded a surface area value of 125 m2 g−1. Individual LCO particles were imaged and sized using a FEI Tecnai T12 transition electron microscope (TEM). Only particles completely captured in each TEM micrograph were measured. Thickness was measured on particles that appeared very dark as this means they were viewed edge-on. Length of particles were measured if clearly defined endpoints were visible and if it could reasonably be assumed that it was a single particle as opposed to an aggregate. Dynamic light scattering (DLS) and zeta potential measurements of LCO suspensions in OECD 201 media were obtained with a Zetasizer Nano ZS Size Analyzer from Malvern PANalytical.
Algal cell culture
A stock culture of R. subcapitata, inoculated at 1 × 105 cells per mL, was grown in a 1 L Erlenmeyer flask and cultured in OECD 201 media.25 Cells were illuminated continuously in an incubator with a full spectrum T8 light bulb at a photon flux of 70 μE m−2 s−1. The stock culture was mixed with an orbital shaker at a speed of 111 rpm.
Exposure setup
This exposure was done to assess predict the phytotoxic MoA of LCO on R. subcapitata by comparing changes in the complex phenotype of LCO-treated cells to that of reference chemicals with established MoAs after 24 hours of exposure. Each reference chemical and their associated MoA are reported in Table 1, below.
Table 1 Reference chemicals with known MoAs
Chemical |
Mechanism of action |
Abbreviation |
Ref. |
Aclonifen |
Carotenoid biosynthesis inhibition |
CBI |
26
|
Carfentrazone |
Membrane disruption |
MD |
27
|
DCMU |
PSII photochemistry inhibition |
PPI |
28
|
Glufosinate |
N2 metabolism inhibition |
NMI |
29
|
H2O2 |
Oxidative stress |
OS |
30
|
Metolachlor |
Very-long-chain fatty acid synthesis inhibition |
VLCFASI |
31
|
MSMA |
OP uncoupler/e− transport inhibition |
OPU/e− TI |
32
|
Zeocin |
DNA damage |
DD |
33
|
An additional LCO exposure was done at 48 hours to better evaluate physiological endpoints such as nucleation state. Algae were exposed to one of four LCO concentrations or untreated control (0 μg mL−1, 0.01 μg mL−1, 0.1 μg mL−1, 1 μg mL−1, & 10 μg mL−1 LCO), an ion control that contained the concentration of lithium and cobalt ions that would be present in the algae media containing 10 μg mL−1 of LCO after 24 or 48 hours, depending on the exposure duration. In each treatment, 900 μL aliquots of algal stock culture were seeded into individual 1.5 mL microcentrifuge tubes after cells were growing exponentially. A stock suspension of LCO was constituted at 100 μg mL−1 in OECD 201 media. For this study, the OECD 201 media was made to be deficient in EDTA in order to prevent the mitigation of any metal-induced stress. This suspension was sonicated for 25 minutes before the addition to respective samples to break up any aggregated nanoparticles. Additionally, an ion solution made from LiOH and CoCl2·H2O, also constituted the OECD 201 media, was made at 10× the concentration of ion dissolution of 10 μg mL−1 of LCO after 24 and 48 hours, depending on the exposure duration. For each treatment, OECD 201 media, LCO suspension, or 10× ion solution was added to each 900 μL cell suspension at a final volume of 1 mL. The samples were then placed under full spectrum illumination, with tube lids open, at a photon flux of 70 μE m−2 s−1 for 24 hours. The 24 hour exposure duration was chosen for MoA prediction as this timepoint has been shown to better delineate initial phenotypic impacts,34 while the 48 hour exposure duration was chosen to examine physiological endpoints.
Single-cell chlorophyll fluorescence
At the conclusion of these exposures, a 50 μL aliquot from each sample was plated in to a well of a glass bottom 384 well plate (Cellvis, P384W-1.5H-N) and spun gently at 600 RPM for 1 minute to concentrate cells at the bottom of the well. Cells were not stained nor fixed with glutaraldehyde for this assay as to prevent interference with raw fluorescence. Cells were then dark adapted for 30 min to ensure PSII centers were in an open, or oxidized, state before imaging with an ImageXpress Micro XLS High-Content Screening System. For image acquisition, cells were visualized using a Cy5 filter (ex/em: 628/692) in a manner similar to that of a PAM Fluorometer, such that cells are exposed to a low intensity light beam followed by a saturating light beam to measure the minimal (F0) and maximal (Fm) chlorophyll fluorescence, respectively (Fig. 1) using the fluorescence cytochemistry parameters in Table 2. After acquiring images, bioimage analysis was used to measure the respective fluorescence intensities. Using the “mean_integrated_intensity” values for F0 and Fm, the variable fluorescence (Fv) and quantum efficiency of PSII (Fv/Fm) of individual cells were calculated using the following equation: |  | (1) |
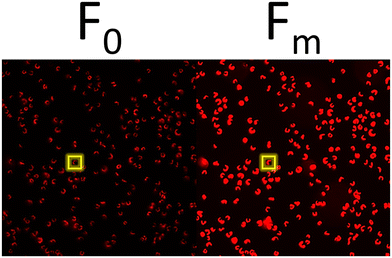 |
| Fig. 1 Representative fluorescence micrograph of single cells at an F0 and Fm state, respectively. | |
Table 2 Single-cell chlorophyll fluorescence cytochemistry parameters
Intensity |
Channel |
Excitation (nm) |
Emission (nm) |
Exposure time (nm) |
Minimal (F0) |
Cy5 |
628/40 |
692/40 |
35 ms |
Maximal (Fm) |
Cy5 |
628/40 |
692/40 |
270 ms |
Multiplexed algal cytological imaging (MACI) assay
The MACI assay was carried out as using methods described by Ostovich & Klaper, 2023.9 At the end of the exposure, 905 μL aliquots from each sample were transferred to sterile 1.5 mL microcentrifuge tubes. Commercially available fluorescent probes were used to stain nuclei and lipid droplets using NucBlue (Thermo Fisher, R37605) and BODIPY 505/515 (Thermo Fisher, D3921), respectively, and glutaraldehyde was used to fix the algal cells. After adding reagents, all reactions were incubated overnight, at 4 °C to minimize enzymatic degradation and maintain the integrity of the subcellular structures. After incubating reactions, cells were centrifuged at 4000×g for 5 min, washed 2× with 1× phosphate buffered saline (PBS), and resuspended in PBS. Cells from each sample were loaded into a well of a glass bottom 384 well plate (Cellvis, P384W-1.5H-N) at a seeding density of ∼2 × 103 cells per mm2 for optimal distribution of cells across the well surface. After loading cells, the well plate was then spun gently at 600 RPM for 1 minute to concentrate cells at the bottom of the well. Images were acquired at 9 sites per well with an ImageXpress Micro XLS High-Content Screening System with a 60× Plan Fluor 0.85 NA air immersion objective (Molecular Devices, 1-6300-0414), using the Cy5, GFP, and DAPI fluorescent channels to visualize the chloroplast, lipid droplets, and nuclei, respectively. To enhance image contrast and resolution, the digital confocal feature was used during image acquisition. For representative cell images with higher resolution, some images were also acquired with a 100× CFI L PLAN EPI CC 0.85 NA air immersion objective (Molecular Devices, 1-6300-0419).
Bioimage analysis
After acquiring images CellProfiler,35 was used for image pre-processing, object segmentation, and morphological feature extraction at the resolution of individual cells. Morphological features related to area, shape, intensity, and granularity of each subcellular structure were extracted, in addition to cytoplasmic intensity features to add more measurements for comparing the phenotypic profiles of LCO and reference chemical treatments, which were run on separate plates. These data were exported to a local SQLite database file and were then extracted using the RSQLite package in R.36
Phenotypic profiling – fingerprint analysis
Phenotypic response data was analyzed using the methods described by Ostovich & Klaper, 2023.9 The data was firstly processed by aggregating single-cell morphological feature measurements to per-image and then per-well values, which was done by taking the cell and image means, respectively. Secondly, well data from each compound and dose were then normalized to the non-treated cell control by computing a Z-score. In order to verify whether LCO elicited a change to the entire phenotypic profile of treated cells, a partial least squares-discriminant analysis (PLS-DA) was performed in R using the mixOmics package.37 Before feeding phenotypic response data into the PLS-DA models, an ANOVA was performed across all features for each reference chemical to remove any non-informative features with little variance (p-values > 0.05). Lastly, factor analysis was used to further reduce the dimensionality of phenotypic data vectors, and the fingerprints were subsequently compared to one another using hierarchical clustering based on Euclidean distance in R.
Phenotypic profiling – convolutional neural networks
In addition to fingerprint analysis, a CNN was also trained on a small subset of reference compound treated cells (∼9.3%) using the classifier module on CellProfiler Analyst (Ver 3.0).38 A separate bin was created for each reference chemical and the non-treated cell control in the classifier module, where around 1000 randomly fetched cells from each treatment were placed in each respective bin. For the non-treated cell control, cells form the reference chemical exposure and LCO exposure were both used to account for plate-to-plate and run-to-run variations. After training the CNN, it was used to score the entire experiment by classifying individual cells into predicted mechanistic classes, and computing enrichment scores for each sample as the logit area under the receiver operating characteristic curve. An ANOVA and a Tukey post hoc test was used to evaluate the significance of predicted mechanistic class enrichments for each treatment.
Statistical analysis
All statistical analyses were performed using R Studio.39,40 A Shapiro–Wilk test was used to verify normal distribution and a One-Way ANOVA was used to compare variance among group means, while a Tukey post hoc test was used for multiple comparisons. In each analysis, significant differences were determined with a 95% confidence interval.
Results and discussion
LCO characterization
The sizing of single LCO particles, using TEM micrographs (found in ESI†) suggested an average thickness and length of 5.54 ± 2.01 nm and 39.63 ± 17.35 nm, respectively. The zeta potential values for LCO suspended in OECD 201 media (found in ESI†) indicate that this nanomaterial is negatively charged between −17 and −20 mV in higher concentrations, and around −8 mV in the lowest concentration (0.01 μg mL−1). However, at all concentrations, the lower magnitude of the zeta potential (<|30| mV) suggests that LCO is not highly stable in suspension with OECD 201 media. Furthermore, the hydrodynamic diameter of LCO also generally increases with increasing concentration, thus also suggesting increasing aggregation of LCO nanoparticles with increasing concentration. These results generally coincide with LCO's behavior in other aqueous media, like moderately hard reconstituted water41 and Bold's modified freshwater solution,8 as well.
LCO significantly alters algal cell morphology after 24 hours
After exposing R. subcapitata with LCO for 24 hours, the cells were stained and imaged using the MACI protocol (Fig. 2a). In the representative fluorescence micrograph, the LCO-treated cells appear to be larger compared to the control cell, and also appear to have distinct levels of chlorophyll, TAG, and DNA content, which visually support the claim that LCO has an impact on cell morphology. A CellProfiler pipeline was used to convert the high-content image data into quantitative data where 570 unique, unbiased, morphological features of individual cells were used to generate phenotypic fingerprints of molecular interaction. In order to quantitatively verify if LCO treatment significantly alters algal cell morphology, a PLS-DA was used to analyze subtle changes in the complex phenotypic profile of LCO-treated cells with increasing concentration. Based on the PLS-DA response plot (Fig. 2b), LCO-treated cells display a significant separation between response groups, thereby indicating that LCO does elicit a significant, and measurable, change to cell morphology after 24 hours. Based on X-variate data, a significant separation from the control group can be seen at starting at the 0.1 μg mL−1 response group. Here, the ion control response group also exhibited a significant separation from the control group. However, the ion control response group, which represents the amount of Li+ and Co2+ ions released from 10 μg mL−1 of LCO after 24 hours, was also significantly different form the 10 μg mL−1 response group. Interestingly, this data suggests that while the ions do have somewhat of an impact on cell morphology, they are separate from nano-specific impacts.
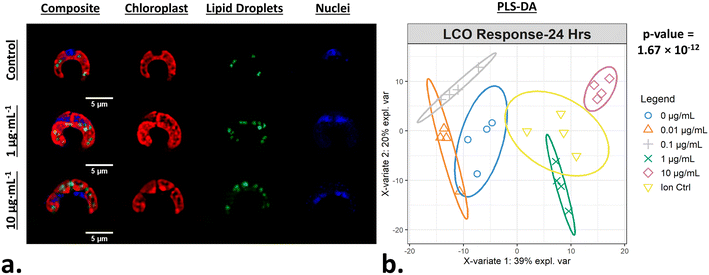 |
| Fig. 2 Phenotypic responses of algal cells when exposed to LCO. (a) Representative fluorescence micrograph of MACI labeling patterns in treated and non-treated algal cells. (b) A PLS-DA response plot graphically describes the change across complex morphological feature data with increasing concentration of LCO; ellipses represent 95% confidence intervals and p-values represent ANOVA statistics across the 1st latent variable between response groups. | |
Phenotypic profiles of LCO-treated cells compared to reference chemical-treated cells
In order to predict the phytotoxic MoA of LCO, the phenotypic profiles of LCO-treated cells were compared to reference chemical-treated cells with known MoAs. For this purpose, the 10 μM reference chemical data was compared to the 1 μg mL−1 LCO data, which is around 10 μM of LCO, to compare responses of similar concentrations.
After constructing the phenotypic fingerprints, an ANOVA was used to identify individual features that carry little information, which were removed from the analysis given a p-value > 0.05. Additionally, factor analysis was used to further reduce the dimensionality of the phenotypic data vectors down to 7 eigen features/factors in order to minimize redundant measurements while preserving variance. The phenotypic fingerprints across all replicates for each reference chemical and LCO treatment were hierarchically clustered based on Euclidean distance (Fig. 3). Euclidean distance is a data mining technique that measures how far apart two points are in a high-dimensional feature space,42 and can be calculated as the square root of the sum of squares between two vectors.43
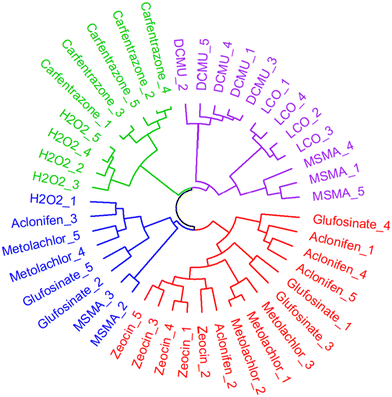 |
| Fig. 3 Dendrogram of hierarchically clustered LCO treated samples compared to reference chemical threated samples. Branches represent relative Euclidean distances between samples and nearest neighboring samples indicate the most similar phenotypic profiles to one-another. | |
The hierarchical clustering analysis was able to identify four main clusters. LCO-treated samples were clustered with DMCU and three of the MSMA-treated samples, thus indicating that their phenotypic profiles are most similar to one another. The MoAs of DMCU and MSMA are both related to electron transport inhibition in the chloroplast and mitochondrion, respectively.28,32 Based on the results of the hierarchical clustering analysis, we can deduce that the probable MoA of LCO is also likely related to electron transport inhibition. This would makes sense as LCO tends to target proteins and pathways that are involved in transport of electrons.7,6
Predicting the MoA of individual LCO-treated cells using convolutional neural networks
In addition to the hierarchical clustering analysis, a convolutional neural network was also used to classify individual LCO-treated cells into mechanistic classes. In CellProfiler Analyst, a CNN was trained on a small subset of randomly fetched cells from each treatment (∼9.3% of cells from the combined LCO and reference chemical exposures) using 50 × 50 neurons per layer. For the healthy cell class, the CNN was trained on cells form the non-treated cell controls of both the LCO and reference chemical exposures as a means to account for variations due to separate plates and separate runs. Based on the confusion matrix (Fig. 4), the CNN model was able to predict the correct mechanistic class across training cells with a moderately good classification accuracy of 73.82%. Once trained, the CNN model was used to score each cell in the LCO exposure, based on its individual phenotype, with a predicted mechanistic class, and then calculate enrichment scores for each sample.
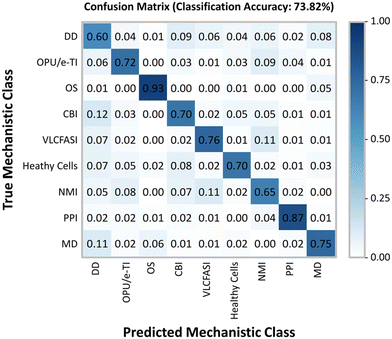 |
| Fig. 4 Convolutional neural network construction. A small subset of randomly fetched cells in each treatment from the reference chemical and LCO exposures were fed into a convolutional neural network model. Based on the training data, this CNN model yields a classification accuracy of 73.82% at correctly classifying cells by their true mechanistic class. | |
The average enrichment scores for the LCO exposure are visualized in a heatmap (Fig. 5) where values with asterisks (*) represent the mechanistic classes which are significantly enriched in each treatment when compared to the control. Based on these results, the CNN model predicted PSII photochemistry inhibition, or photosynthetic electron transport, as the most probable MoA of LCO with the highest and most significantly enriched scores. This was most notable in the 1 μg mL−1 and ion control treatments. These treatments also obtained high enrichment scores in the oxidative phosphorylation uncoupler/mitochondrial electron transport inhibition mechanistic class; however, the scores were not significantly different from the control due to larger deviations in the enrichment data. This data coincides with the hierarchical clustering data (Fig. 3) in that electron transport inhibition in general appears to be the most probable phytotoxic MoA of LCO, but the CNN data suggests that this disturbance is more prevalent in the photosynthetic pathway.
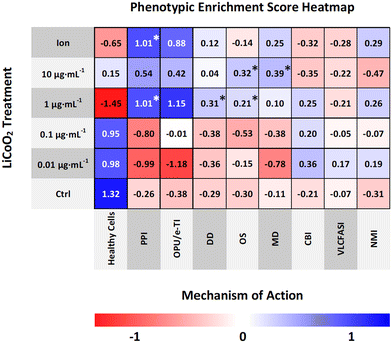 |
| Fig. 5 Phenotypic enrichment score heatmap. The CNN model classifies each cell across the entire LCO exposure with a predicted mechanistic class based on its phenotype. Enrichment scores for each mechanistic class are calculated in each sample. Heatmap values represent average treatment enrichment scores; values with asterisks (*) represent significantly enriched mechanistic classes compared to the control. | |
Part of the advantage of using a CNN classifier model for phenotypic profiling is the fact that it can identify rare objects,38 or smaller subpopulations of cells, in an otherwise heterogeneous cell population, that have distinct mechanistic classes that may go undetected with other phenotypic profiling methods that average across all cells. Interestingly, based off the enrichment data, there were also three other significantly enriched mechanistic classes found in much smaller subpopulations of cells. In the 1 μg mL−1 treatment, the DNA damage and oxidative stress mechanistic classes were also significantly enriched. Additionally, in the 10 μg mL−1 treatment, the oxidative stress and membrane disruption mechanistic classes were significantly enriched. These results may make sense as these mechanistic classes were only enriched in the highest concentrations of LCO, which are inherently more likely to have more cells undergoing a cytotoxic shock in which disturbances such as DNA damage, membrane disruption, and high levels of oxidative stress are experienced. However, it is important to reiterate here that the main MoA experienced by these cells is still largely photosynthetic electron transport inhibition as the other mechanistic classed were only indicated in a small percentage of cells.
Chlorophyll fluorescence markers indicate that LCO alters the oxidation state of PSII proteins
Chlorophyll fluorescence is a non-invasive way to determine relative amounts of chlorophyll content in plant-type organisms and to assess the overall efficiency of photochemistry.44,45 Several methods currently exist to make these sorts of measurements; pulse amplitude modulation (PAM) fluorometers, for example, are typically used to make these measurements in leaf tissue and algal cells. PAM's work by, first, exposing the sample to a pulse of low intensity light to get the minimal fluorescence, as most of the energy will enter photochemistry and only a small portion will be re-emitted as fluorescence signal. This is then followed by a pulse of saturating light to get the maximal fluorescence, which at this point, the PSII reaction centers will already be reduced and thus most of the excited energy will be re-emitted as fluorescence signal. In general, the minimal fluorescence is comparable to the levels of chlorophyll present in leaf and algal samples.44 Furthermore, by taking the ratio of minimal and maximal fluorescence, the quantum efficiency of PSII can be calculated,46 which is a good indicator of photochemistry efficiency.47 Here, this same concept was applied using high-content fluorescence microscopy for algal cells, and with this tool, the relative chlorophyll levels and quantum efficiencies of PSII evaluated for individual cells.
Fig. 6a displays measurements of single-cell chlorophyll fluorescence after being exposed to LCO for 48 hours; each red dot represents an individual cell. LCO treated cells had exhibited significant increases in chlorophyll content compared to that of the control (Fig. 6b). A quantum efficiency of PSII around 0.7 is considered healthy and/or normal for eukaryotic algal cells,48,49 so based on the data in Fig. 6c, even LCO treated cells are still within a normal range. Interesting, however, LCO treated cells exhibited a significant decrease in the quantum efficiency of PSII, compared to the control, in somewhat of a dose-dependent manner. In the context of the PSII biochemistry, this data would suggest that, in LCO treated cells, there is a higher fraction of damaged, or inactive, PSII reaction centers.50 This type of disturbance is typically due to the irreversible oxidation of the D1 and D2 proteins in the PSII complex, usually following the production of O2˙− and OH˙ radicals.51 These results, coincide with the hierarchical clustering (Fig. 3) and CNN (Fig. 5) analyses which predicted photosynthetic electron transport inhibition as the main phytotoxic MoA of LCO. Additionally, these results could help explain the reductions in the net production of carbon biomass reported in previous studies.8
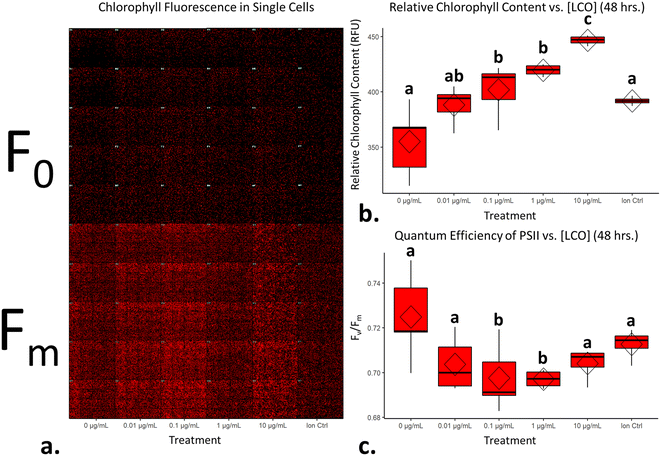 |
| Fig. 6 LCO significantly alters markers of chlorophyll fluorescence. (a) Representative montage micrograph of algal cells across all treatments at a state of minimal and maximal fluorescence after 48 hours of exposure to LCO; (b) relative chlorophyll content after 48 hours of exposure to LCO; (c) quantum efficiency of PSII. Diamonds on boxplots represent treatment means and significant differences were determined using a one-way ANOVA with a Tukey post hoc test for multiple comparisons; columns with different letters differ significantly (p < 0.05). | |
LCO significantly impacts the nucleation state and DNA content after 48 hours
In eukaryotic cells, the nucleus in an important subcellular compartment which houses the genetic material of the organism and is responsible for regulating gene expression and facilitating cellular division. Most green algae contain a unique multiple fission reproductive pattern in which one mother cell can divide into several daughter cells, depending on the environmental cues.19R. subcapitata, in particular, has the ability to divide into eight daughter cells,18 and measuring the number of nuclei per cell, as well as the relative amount of DNA content per nucleus, can be used to describe instances of cell cycle disruption/arrest.31,19,52 After exposing cells to LCO for 48 hours, cells were stained with NucBlue, in order to fluorescently label the DNA content within the algae. A CellProfiler pipeline was used the count the number of nuclei/cell, as well as measure the integrated intensity of each nuclei, which was taken as relative DNA content. On average, LCO-treated sample contained a higher percentage of cells with only one nucleus when compared to the non-treated samples (Fig. 7a). This affect increased in a dose-dependent manner, with significant differences from the control observed at 10 μg mL−1 LCO and the ion control. The opposite trend was observed for cells with more than one nucleus (i.e. 2 & 4 nuclei), which also changed in a dose-dependent manner, with significant differences from the control observed at 10 μg mL−1 LCO and the ion control. Looking only at the cells with one nucleus, LCO-treated cells had, on average, a lesser relative amount of DNA content when compared to the non-treated control cell (Fig. 7b). This was also observed in a dose-dependent manner, but with significant differences from the control at 0.1 μg mL−1 LCO, 10 μg mL−1 LCO, and the ion control. Interestingly, when assessing the Pearson correlation between the two trends, a strong negative correlation was observed (Fig. 7c). Together, these results indicate that, on average, LCO-treated cells exhibit an increased instance in the delay/arrest of cell cycle progression, specifically in the earlier stages of the cell cycle (stage 1/2) before first nuclear division.52 Based on these results, it may explain the instances of increased growth inhibition and biovolume of LCO-treated algal cells reported in previous studies.8 In this case, it may be possible that LCO had an impact on nuclear/cellular division through the impairment of photosynthetic electron transport.53 These results are similar to that of DCMU treated cells, which, on average, had been reported to have significantly less nuclei/cell when compared to non-treated control cells, and had appeared to have inhibited nuclear division.9 This would also be consistent with other photosynthetic organisms, like Euglena gracilis, in which similar impacts have been reported under impaired photosynthetic electron transport.53
 |
| Fig. 7 Nucleation state and relative DNA content in LCO-treated cells. (a) Percentage of cells with 1, 2, or 4 nuclei after 48 hours of exposure to LCO; (b) relative DNA content of single nucleated cells; (c) Pearson correlation between the percentage of single nucleated cells and their relative DNA content. Significant differences were determined using a one-way ANOVA with a Tukey post hoc for multiple comparisons; columns with different letters differ significantly (p < 0.05). Error bars represent SEM. | |
Conclusion
In this study, MACI and machine learning techniques were used to assess the interactions of LCO at the biointerface of R. subcapitata cells and to predict the phytotoxic MoA of LCO. Algal cells were exposed to varying concentrations of LCO, and their phenotypic profiles were compared to that of cells treated with reference chemicals with established MoAs. The described analyses predicted photosynthetic electron transport to be the most probable phytotoxic MoA of LCO, and single-cell chlorophyll fluorescence demonstrated an increase in irreversibly oxidized photosystem II proteins, thus fortifying the MACI assay prediction and coinciding with the impaired carbon biomass assimilation reported in previous work. Lastly, LCO-treated cells were observed to have less nuclei/cell and less DNA content/nucleus when compared to non-treated cell controls, which suggests an interference with cell cycle progression, also complementing the growth inhibition and biovolume data reported in previous work.
Conflicts of interest
There are no conflicts to declare.
Acknowledgements
This work was supported by the National Science Foundation under Grant No. CHE-2001611, the NSF Center for Sustainable Nanotechnology. The CSN is part of the Centers for Chemical Innovation Program.
References
- R. J. Hamers, Energy storage materials as emerging nano-contaminants, Chem. Res. Toxicol., 2020, 33(5), 1074–1081 Search PubMed.
- J. P. Brog, A. Crochet, J. Seydoux, M. J. D. Clift, B. Baichette, S. Maharajan, H. Barosova, P. Brodard, M. Spodaryk, A. Zütte, B. Rothen-Rutishauser, N. H. Kwon and K. M. Fromm, Characteristics and properties of nano-LiCoO 2 synthesized by pre-organized single source precursors : Li-ion diffusivity, electrochemistry and biological assessment, J. Nanobiotechnol., 2017, 1–23 Search PubMed.
- D. H. P. Kang, M. Chen and O. A. Ogunseitan, Potential environmental and human health impacts of rechargeable lithium batteries in electronic waste, Environ. Sci. Technol., 2013, 47(10), 5495–5503 CrossRef CAS PubMed.
- Z. Lu, H. Wang, D. Kong, K. Yan, P. Hsu, G. Zheng, H. Yao, Z. Liang, X. Sun and Y. Cui, Electrochemical tuning of layered lithium transition metal oxides for improvement of oxygen evolution reaction, Nat. Commun., 2014, 5, 1–7, DOI:10.1038/ncomms5345.
- N. Nitta, F. Wu, J. T. Lee and G. Yushin, Li-ion battery materials: present and future, Biochem. Pharmacol., 2015, 18(5), 252–264, DOI:10.1016/j.mattod.2014.10.040.
- B. J. Curtis, N. J. Niemuth, E. Bennett, A. Schmoldt, O. Mueller, A. A. Mohaimani, E. D. Laudadio, Y. Shen, J. C. White, R. J. Hamers and R. D. Klaper, Cross-species transcriptomic signatures identify mechanisms related to species sensitivity and common responses to nanomaterials, Nat. Nanotechnol., 2022, 17(6), 661–669 CrossRef CAS PubMed.
- N. J. Niemuth, Y. Zhang, A. A. Mohaimani, A. Schmoldt, E. D. Laudadio, R. J. Hamers and R. D. Klaper, Protein Fe-S Centers as a Molecular Target of Toxicity of a Complex Transition Metal Oxide Nanomaterial with Downstream Impacts on Metabolism and Growth, Environ. Sci. Technol., 2020, 54(23), 15257–15266 CrossRef CAS PubMed.
- E. Ostovich, A. Henke, C. Green, E. Laudadio, M. Spehlmann, N. Van Ee, J. Uertz and R. H. Hamers, Klaper R Physiological Impacts on Raphidocelis subcapitata in Response to Lithiated Cobalt Oxide Nanomaterials, Environ. Toxicol. Chem., 2023, 1–12 Search PubMed.
- E. Ostovich and R. Klaper, Using a novel multiplexed algal cytological imaging (MACI) assay and machine learning as a way to characterize complex phenotypes in plant-type organisms, Environ. Sci. Technol. Search PubMed in press.
- S. M. Gustafsdottir, V. Ljosa, K. L. Sokolnicki, J. A. Wilson, D. Walpita, M. M. Kemp, K. P. Seiler, H. A. Carrel, T. R. Golu, S. L. Schreiber, P. A. Clemons, A. E. Carpenter and A. F. Shamji, Multiplex cytological profiling assay to measure diverse, PLoS One, 2013, 8(12), 1–7 CrossRef PubMed.
- E. B. Svenningsen and T. B. Poulsen, Bioorganic & Medicinal Chemistry Establishing cell painting in a smaller chemical biology lab – A report from the frontier, Bioorg. Med. Chem., 2019, 27(12), 2609–2615, DOI:10.1016/j.bmc.2019.03.052.
- V. Ljosa, P. D. Caie, R. Horst, K. L. Sokolnicki, E. L. Jenkins, S. Daya, M. E. Roberts, T. R. Jones, S. Singh, A. Genovesio, P. A. Clemons, N. O. Carragher and A. E. Carpenter, Comparison of Methods for Image-Based Profiling of Cellular Morphological Responses to Small-Molecule Treatment, J. Biomol. Screening, 2013, 18(10), 1321–1329, DOI:10.1177/1087057113503553.
- Y. Feng, T. J. Mitchison, A. Bender, D. W. Young and J. A. Tallarico, Multi-parameter phenotypic profiling: Using cellular effects to characterize small-molecule compounds, Nat. Rev. Drug Discovery, 2009, 8(7), 567–578 CrossRef CAS PubMed.
- M. Bray, S. Singh, H. Han, C. T. Davis, B. Borgeson, C. Hartland, M. Kost-alimova, S. M. Gustafsdottir, C. C. Gibson and A. E. Carpenter, Cell Painting, a high-content image-based assay for morphological profiling using multiplexed fluorescent dyes, Nat. Protoc., 2016, 11(9), 1757–1774 CrossRef CAS PubMed.
- A. Boit and U. Gaedke, Benchmarking successional progress in a quantitative food web, PLoS One, 2014, 9(2), 1–25 CrossRef PubMed.
- C. Zhang, X. Chen, W. C. Chou and S. H. Ho, Phytotoxic effect and molecular mechanism induced by nanodiamonds towards aquatic Chlorella pyrenoidosa by integrating regular and transcriptomic analyses, Chemosphere, 2021, 270, 129473, DOI:10.1016/j.chemosphere.2020; 1-10.
-
U.S. Environmental Protection Agency, Ecological Effects Test Guidelines OCSPP 850.4500: Algal Toxicity, United States Environ Prot Agency, 2012, pp. 1–26 Search PubMed.
- T. Yamagishi, H. Yamaguchi, S. Suzuki, Y. Horie and N. Tatarazako, Cell reproductive patterns in the green alga Pseudokirchneriella subcapitata (=Selenastrum capricornutum) and their variations under exposure to the typical toxicants potassium dichromate and 3,5-DCP, PLoS One, 2017, 1–12 Search PubMed.
-
V. Zachleder, K. Bišová and M. Vítová, The Cell Cycle of Microalgae, in The Physiology of Microalgae, ed. M. Borowitzka, J. Beardall and J. Raven, Developments in Applied Phycology, Springer, Cham, 2016, vol. 6, pp. 3–46 Search PubMed.
- S. Roccuzzo, N. Couto, E. Karunakaran, R. V. Kapoore, T. O. Butler, J. Mukherjee, E. M. Hansson, A. P. Beckerman and J. Pandhal, Metabolic Insights Into Infochemicals Induced Colony Formation and Flocculation in Scenedesmus subspicatus Unraveled by Quantitative Proteomics, Front. Microbiol., 2020, 11, 1–17 CrossRef PubMed.
- T. Malina, E. Maršálková, K. Holá, J. Tuček, M. Scheibe, R. Zbořil and B. Maršálek, Toxicity of graphene oxide against algae and cyanobacteria: Nanoblade-morphology-induced mechanical injury and self-protection mechanism, Carbon, 2019, 155, 386–396 CrossRef CAS.
- Z. K. M. Al-Khazali and H. A. Alghanmi, Environmental Toxicity of Nano Iron Oxides (Fe2O3 NPs) on Algal Growth Klisinema persicum and Cellular DNA Damage Using Comet Assay, Egypt. J. Aquat. Biol. Fish., 2023, 27(1), 431–453 CrossRef.
- E. D. Laudadio, J. W. Bennett, C. M. Green, S. E. Mason and R. J. Hamers, Impact of Phosphate Adsorption on Complex Cobalt Oxide Nanoparticle Dispersibility in Aqueous Media, Environ. Sci. Technol., 2018, 52(17), 10186–10195 CrossRef CAS PubMed.
- E. D. Laudadio, P. Ilani-Kashkouli, C. M. Green, N. J. Kabengi and R. J. Hamers, Interaction of Phosphate with Lithium Cobalt Oxide Nanoparticles: A Combined Spectroscopic and Calorimetric Study, Langmuir, 2019, 35(50), 16640–16649 CrossRef CAS PubMed.
-
OECD, Test No. 201: Freshwater Alga and Cyanobacteria, Growth Inhibition Test. OECD Guidelines for Testing of Chemicals, Section 2, OECD Publishing Service, Paris, France, 2011, pp. 1–25 Search PubMed.
- A. C. Almeida, T. Gomes, K. Langford, K. V. Thomas and K. E. Tollefsen, Oxidative stress in the algae Chlamydomonas reinhardtii exposed to biocides, Aquat. Toxicol., 2017, 189, 50–59, DOI:10.1016/j.aquatox.2017.05.014.
- X. Li, S. L. Volrath, D. B. C. Nicholl, C. E. Chilcott, M. A. Johnson, E. R. Ward and M. D. Law, Development of Protoporphyrinogen Oxidase as an Efficient Selection Marker for Agrobacterium tumefaciens-Mediated Transformation of Maize, Plant Physiol., 2003, 133(2), 736–747 CrossRef CAS PubMed.
- L. Glauch and B. I. Escher, The Combined Algae Test for the Evaluation of Mixture Toxicity in Environmental Samples, Environ. Toxicol. Chem., 2020, 39(12), 2496–2508 CrossRef CAS PubMed.
- T. Nagai, Sensitivity differences among seven algal species to 12 herbicides with various modes of action, J. Pestic. Sci., 2019, 44(4), 225–232 CrossRef CAS PubMed.
- T. D. Geer, C. M. Kinley, K. J. Iwinski, A. J. Calomeni and J. H. Rodgers, Comparative toxicity of sodium carbonate peroxyhydrate to freshwater organisms, Ecotoxicol. Environ. Saf., 2016, 132, 202–211, DOI:10.1016/j.ecoenv.2016.05.037.
- M. D. Machado and E. V. Soares, Reproductive cycle progression arrest and modification of cell morphology (shape and biovolume) in the alga Pseudokirchneriella subcapitata exposed to metolachlor, Aquat. Toxicol., 2020, 222, 1–9, DOI:10.1016/j.aquatox.2020.105449.
- F. E. Dayan and M. L. d. M. Zaccaro, Chlorophyll fluorescence as a marker for herbicide mechanisms of action, Pestic. Biochem. Physiol., 2012, 102(3), 189–197, DOI:10.1016/j.pestbp.2012.01.005.
- M. Čížková, M. Slavková, M. Vítová, V. Zachleder and K. Bišová, Response of the green alga Chlamydomonas reinhardtii to the DNA damaging agent zeocin, Cells, 2019, 8(7), 1–15 CrossRef PubMed.
- J. Nyffeler, C. Willis, R. Lougee, A. Richard, K. Paul-friedman and J. A. Harrill, Bioactivity screening of environmental chemicals using imaging-based high-throughput phenotypic profiling, Toxicol. Appl. Pharmacol., 2020, 389, 1–19, DOI:10.1016/j.taap.2019.114876.
- D. R. Stirling, M. J. Swain-Bowden, A. M. Lucas, A. E. Carpenter, B. A. Cimini and A. Goodman, CellProfiler 4: improvements in speed, utility and usability, BMC Bioinf., 2021, 22(1), 1–11, DOI:10.1186/s12859-021-04344-9.
-
K. Müller, H. Wickham, D. A. James and S. Falcon, RSQLite: SQLite Interface for R, 2023, Available from: https://rsqlite.r-dbi.org, https://github.com/r-dbi/RSQLite Search PubMed.
- F. Rohart, B. Gautier, A. Singh and K.-A. Lê Cao, mixOmics: An R package for ‘omics feature selection and multiple data integration’, PLoS Comput. Biol., 2017, 13(11), 1–14, DOI:10.1371/journal.pcbi.1005752 , Available from: https://www.embase.com/search/results?subaction=viewrecord&from=exportamp;id=L619520782%0A.
- D. R. Stirling, A. E. Carpenter and B. A. Cimini, CellProfiler Analyst 3.0: accessible data exploration and machine learning for image analysis, Bioinformatics, 2021, 37(21), 3992–3994 CrossRef CAS PubMed.
-
R Core Team, R: A Language and Environment for Statistical Computing, Vienna, Austria, 2019 Search PubMed.
-
RStudio Team, RStudio: Integrated Development Environment for R, Boston, MA, 2020 Search PubMed.
- N. J. Niemuth, B. J. Curtis, M. N. Hang, M. J. Gallagher, D. H. Fairbrother, R. J. Hamers and R. D. Klaper, Next-Generation Complex Metal Oxide Nanomaterials Negatively Impact Growth and Development in the Benthic Invertebrate Chironomus riparius upon Settling, Environ. Sci. Technol., 2019, 53(7), 3860–3870 CrossRef CAS PubMed.
- J. C. Caicedo, S. Cooper, F. Heigwer, S. Warchal, P. Qiu, C. Molnar, A. S. Vasilevich, J. D. Barry, H. S. Bansal, O. Kraus, M. Wawer, L. Paavolainen, M. D. Herrmann, M. Rohban, J. Hung, H. Hennig, J. Concannon, I. Smith, P. A. Clemons, S. Singh, P. Rees, P. Horvath, R. G. Linington and A. E. Carpenter, Data-analysis strategies for image-based cell profiling, Nat. Methods, 2017, 14(9), 849–863 CrossRef CAS PubMed.
- D. P. P. Mesquita, J. P. P. Gomes, A. H. Souza Junior and J. S. Nobre, Euclidean distance estimation in incomplete datasets, Neurocomputing, 2017, 248, 11–18, DOI:10.1016/j.neucom.2016.12.081.
- H. Zhang, Y. Ge, X. Xie, A. Atefi, N. K. Wijewardane and S. Thapa, High throughput analysis of leaf chlorophyll content in sorghum using RGB, hyperspectral, and fluorescence imaging and sensor fusion, Plant Methods, 2022, 18(1), 1–17, DOI:10.1186/s13007-022-00892-0.
- K. Maxwell and G. N. Johnson, Chlorophyll fluorescence – A practical guide, J. Exp. Bot., 2000, 51(345), 659–668 CrossRef CAS.
- E. Weis and J. A. Berry, Quantum efficiency of Photosystem II in relation to ‘energy’-dependent quenching of chlorophyll fluorescence, Biochim. Biophys. Acta, Bioenerg., 1987, 894(2), 198–208 CrossRef CAS.
- A. A. Ghotbi-Ravandi, M. Sedighi, K. Aghaei and A. Mohtadi, Differential Changes in D1 Protein Content and Quantum Yield of Wild and Cultivated Barley Genotypes Caused by Moderate and Severe Drought Stress in Relation to Oxidative Stress, Plant Mol. Biol. Rep., 2021, 1–7 Search PubMed.
- R. M. Schuurmans, P. Van Alphen, J. M. Schuurmans, H. C. P. Matthijs and K. J. Hellingwerf, Comparison of the photosynthetic yield of cyanobacteria and green algae: Different methods give different answers, PLoS One, 2015, 10(9), 1–17 CrossRef PubMed.
- E. B. Young and J. Beardall, Photosynthetic function in Dunaliella tertiolecta (Chlorophyta) during a nitrogen starvation and recovery cycle, J. Phycol., 2003, 39(5), 897–905 CrossRef CAS.
- E. Janka, O. Körner, E. Rosenqvist and C. O. Ottosen, Using the quantum yields of photosystem II and the rate of net photosynthesis to monitor high irradiance and temperature stress in chrysanthemum (Dendranthema grandiflora), Plant Physiol. Biochem., 2015, 90, 14–22 CrossRef CAS PubMed.
- R. Kale, A. E. Hebert, L. K. Frankel, L. Sallans, T. M. Bricker and P. Pospíšil, Amino acid oxidation of the D1 and D2 proteins by oxygen radicals during photoinhibition of Photosystem II, Proc. Natl. Acad. Sci. U. S. A., 2017, 114(11), 2988–2993 CrossRef CAS PubMed.
- M. Hlavová, M. Čížková, M. Vítová, K. Bišová and V. M. Zachleder, DNA Damage during G2 Phase Does Not Affect Cell Cycle Progression of the Green Alga Scenedesmus quadricauda, PLoS One, 2011, 1–13 Search PubMed.
- M. Yee and J. C. Bartholomew, Effects of 3-(3,4-Dichlorophenyl)-1,1-Dimethylurea on the Cell Cycle in Euglena gracilis, Plant Physiol., 1989, 91, 1025–1029 CrossRef CAS PubMed.
|
This journal is © The Royal Society of Chemistry 2024 |
Click here to see how this site uses Cookies. View our privacy policy here.