Emerging investigator series: secondary organic aerosol formation from photooxidation of acyclic terpenes in an oxidation flow reactor†
Received
7th February 2024
, Accepted 5th May 2024
First published on 29th May 2024
Abstract
One major challenge in predicting secondary organic aerosol (SOA) formation in the atmosphere is incomplete representation of biogenic volatile organic compounds (BVOCs) emitted from plants, particularly those that are emitted as a result of stress – a condition that is becoming more frequent in a rapidly changing climate. One of the most common types of BVOCs emitted by plants in response to environmental stress are acyclic terpenes. In this work, SOA is generated from the photooxidation of acyclic terpenes in an oxidation flow reactor and compared to SOA production from a reference cyclic terpene – α-pinene. The acyclic terpenes used as SOA precursors included β-myrcene, β-ocimene, and linalool. Results showed that oxidation of all acyclic terpenes had lower SOA yields measured after 4 days photochemical age, in comparison to α-pinene. However, there was also evidence that the condensed organic products that formed, while a smaller amount overall, had a higher oligomeric content. In particular, β-ocimene SOA had higher oligomeric content than all the other chemical systems studied. SOA composition data from ultra-high performance liquid chromatography with electrospray ionization mass spectrometry (UHPLC-ESI-MS) was combined with mechanistic modeling using the Generator for Explicit Chemistry and Kinetics of Organics in the Atmosphere (GECKO-A) to explore chemical mechanisms that could lead to this oligomer formation. Calculations based on composition data suggested that β-ocimene SOA was more viscous with a higher glass transition temperature than other SOA generated from acyclic terpene oxidation. This was attributed to a higher oligomeric content compared to other SOA systems studied. These results contribute to novel chemical insights about SOA formation from acyclic terpenes and relevant chemistry processes, highlighting the importance of improving underrepresented biogenic SOA formation in chemical transport models.
Environmental significance
This research addresses uncertainties in the formation of atmospheric aerosol generated from the oxidation of volatile organic compounds released by terrestrial vegetation. In particular, it explores how this environmental process will be altered in a changing climate where increased frequency and severity of drought, heatwaves, and insect outbreaks has led to increased emissions of plant stress volatiles, such as acyclic terpenes, the chemistry of which are not accounted for in most global climate models. The work provides insight into the aerosol chemistry of acyclic terpenes, including information that can be used to improve model representation of this process.
|
Introduction
Secondary organic aerosol (SOA) contributes significantly to global organic aerosol mass loading,1,2 which affects atmospheric chemistry,3,4 climate change,5–7 and human health.8,9 The formation of SOA involves chemical reaction of volatile organic compounds (VOCs) with atmospheric oxidants10–12 leading to nucleation13–15 or condensation of oxidized reaction products onto pre-existing particles.16,17 Globally, the largest source of SOA is derived from biogenic volatile organic compounds (BVOCs) emitted by terrestrial plants, which comprise 90% of atmospheric VOCs.1,18,19 A comprehensive understanding of the formation, composition, and properties of biogenic SOA is crucial for predicting aerosol climate and health effects.
SOA formation from just a few BVOCs, generally those that are most abundant in forested areas, have been studied extensively in laboratory experiments, and those studies have formed the basis for model representations of the atmospheric chemistry of these compounds, including isoprene, α-pinene, β-pinene, and limonene. However, more than 1700 individual BVOCs have been identified and the most abundant BVOCs are not always the ones driving most of the reactivity.20 Their atmospheric reactivity varies by orders of magnitude due to diverse molecular structures, and this complex chemodiversity of BVOCs has been highlighted as a research challenge previously.21–23 For instance, ozonolysis of highly-reactive sesquiterpenes was one of the major sources of secondary products in a French maritime forest even though sesquiterpene abundance was ∼32 times lower than monoterpenes.24 Furthermore, in the Amazon Forest, acyclic monoterpenes including β-myrcene and β-ocimene contributed to nearly half of total reactivity of all monoterpenes but contributed to less than 20% of the total monoterpene emissions.20 The increased reactivity of acyclic terpenes compared to their cyclic counterparts is rooted in the fact that they contain a larger number of double bonds in their chemical structure, which highlights the potential impact of acyclic terpenes on changing aerosol chemistry. One major challenge in predicting SOA formation is to accurately represent the diverse BVOCs emitted from vegetation in the real atmosphere as well as their respective chemistry, creating a need for additional laboratory studies focused on highly reactive, but less studied, BVOCs such as acyclic terpenes.
BVOCs with particularly high reactivity are often emitted by plants under stressed conditions. Examples of common stressors include insect herbivory, drought, and air pollution.25,26 Stress BVOC composition and emission rates are influenced by several factors including, but not limited to, the type of stressor, the degree of damage, the presence of multiple interacting stressors,27,28 and the plant evolutionary history.29,30 These “stress” volatiles have important ecological functions for signaling within and between plants, and signaling to recruit natural enemies of plant herbivores.31 One class of highly reactive stress BVOCs commonly emitted from plants that can impact aerosol chemistry includes acyclic terpenes.25,29,32 For example, under aphid-stressed conditions, pine trees typically emit more acyclic monoterpenes and sesquiterpenes, such as β-ocimene and α-farnesene, respectively.33–36 In addition, β-ocimene and linalool are often identified as typical stress compounds in many plant species.37 In complex mixtures of plant BVOC emissions, increases in the contribution from acyclic terpenes is associated with reduced SOA yields.38,39 Acyclic terpenes in a mixture could also influence cloud formation processes, particle growth, and multiphase chemistry by generating SOA with lower hygroscopicity and increased liquid–liquid phase separation,.40 Therefore, plant stress emissions could have important implications for plant–aerosol interactions in a changing climate. However, detailed mechanistic insight into the aerosol chemistry of acyclic terpenes has not been provided in previous studies referenced here because those studies investigated complex mixtures of precursor volatiles, making it difficult to attribute changes in chemistry to the presence of any particular compound.
To date, only a few studies have systematically investigated the oxidation of acyclic terpenes in controlled laboratory experiments. Ozonolysis of single component acyclic terpene standards shows lower SOA mass yields compared to analogous experiments conducted with cyclic terpene standards, including α-pinene, limonene, and 3-carene.41,42 Ozonolysis of acyclic terpenes proceeds via ozone addition to the C
C double bonds and Criegee intermediates, similar to other terpenes.43 However, for acyclic compounds, this mechanism leads to fragmentation of the molecule. Another study looked at the oxidation of the acyclic terpene β-myrcene via OH radical, and demonstrated that this chemistry can regenerate peroxy radicals by isomerization reactions similar to isoprene and could therefore explain model-measurement discrepancies of OH and HO2 concentrations in recent field campaigns.44 This suggests acyclic terpene chemistry also plays an important role in controlling the atmosphere's radical budget. Further, SOA generated from acyclic terpene photooxidation has relatively higher O
:
C compared to other biogenic SOA precursors.45 The few studies that have focused on acyclic terpene chemistry all demonstrate that their role in atmospheric processes is quite different from the more typical cyclic terpene analogs, but no study has provided enough molecular detail on SOA composition to provide further insight into how this chemistry differs between the different acyclic versus cyclic chemical systems. To address this challenge, we investigated photooxidation of three common acyclic terpenes (β-myrcene, β-ocimene, and linalool) and compared their yields, composition, and physical properties to α-pinene SOA, which served as a reference compound. Composition data and mechanistic modeling were used to propose simplified chemical mechanisms leading to SOA formation from these chemical systems.
2 Methods
2.1 Experimental design
Three different acyclic terpenes including β-myrcene (Sigma-Aldrich Inc., 95%), β-ocimene (Sigma-Aldrich Inc., 90%) and linalool (Alfa Aesar Inc., 97%) were chosen for this study because they are commonly induced compounds after plant stress exposure.37,46 SOA was also generated with α-pinene (Acros Organics Inc., 98%). The molecular structures and reaction rate constants with OH and O3 for each of these acyclic terpenes are provided in Table 1. A schematic of the oxidation flow reactor (OFR; Aerodyne, Inc.) set-up used to generate SOA is shown in Fig. 1. Clean air was produced using a zero-air generator (Environics® Series 7000). The zero air was then humidified with a bubbler, and the dry and wet air were combined to maintain ∼55% relative humidity in the OFR. Flow rates were controlled with mass flow controllers (MFC). An aliquot of the VOC standard was placed in a sealed glass jar and introduced to the OFR by flowing dry clean air through the jar and into the OFR inlet. VOC mixing ratios were not measured at the inlet continuously, but this configuration produced stable particle mass in the OFR as measured with the scanning mobility particle sizer indicating that the VOC input was sufficiently stable as well. The total flow through the OFR was controlled by instrument sampling flows and supplemental vacuum flows at the OFR outlet, as shown in “part 1” and “part 2” in Fig. 1. The two slightly different set-ups were used for different experimental purposes where “part 1” was used for generating SOA yield curves and “part 2” was used for collecting filter samples for offline Orbitrap analysis. The ozone (O3) mixing ratio was continuously monitored (2B Technologies Inc., ozone monitor, Model 106-M) with a flow rate of 1 L min−1. Particle size distributions were measured with a scanning mobility particle sizer (SMPS: a custom-built blower/voltage box interfaced with a TSI DMA (model 3081) and a Brechtel CPC, with a 210Po radioactive neutralizer) with a sampling flow rate of 0.35 L min−1. Custom-built scrubbers were installed at the OFR outlet and filled with charcoal (Sigma-Aldrich Inc.) to remove both VOCs and O3. The scrubbers were designed for efficient aerosol transmission and built at the UCI machine shop. A scrubber was installed in front of the SMPS and in front of the filter holder for particle collection. An additional vacuum flow at the OFR outlet of 5.1 L min−1 was controlled with a mass flow controller (Alicat, Inc.). Therefore, the total flow through the OFR was maintained at 6.45 L min−1, with a corresponding residence time of 122 s. The VOC precursor concentration was adjusted using a dilution flow at the OFR inlet controlled with a MFC. The excess flow at the inlet was released through an exhaust line, where the exhaust flow ranged from 4.7 to 5.4 L min−1 depending on the dilution flow rate. For each experimental condition, duplicate VOC samples were collected onto stainless steel adsorbent cartridges (multibed Carbograph/Tenax TA; Markes International, Inc.) at the OFR inlet and outlet. The concentration of the VOC precursor was analyzed off-line with a thermo-desorption gas chromatograph mass spectrometer (TD-GC-MS, Markes International TD-100xr autosampler, Agilent GC 7890B, equipped with a 30 m, DB-5 column, and Agilent 5975 MS).
Table 1 Formulae, structures, and reaction rate constants for OH and O3 reacting with the acyclic terpene precursors selected in this study, as well as a benchmark terpene, α-pinene23,47–50
Compound |
Formula |
Structure |
Reaction rate constant |
K
OH (cm3 molecule−1 s−1) |
K
O3 (cm3 molecule−1 s−1) |
β-myrcene |
C10H16 |
|
3.34 × 10−10 |
4.44 × 10−16 |
β-ocimene |
C10H16 |
|
3.03 × 10−10 |
4.44 × 10−16 |
Linalool |
C10H18O |
|
1.70 × 10−10 |
4.30 × 10−16 |
α-pinene |
C10H16 |
|
5.23 × 10−11 |
1.07 × 10−16 |
The OFR used in this study is a 13 L aluminum cylinder equipped with two mercury lamps that have peak intensity at 185 nm and 254 nm. High oxidative capacity in the reactor can produce a photochemical age ranging from hours to days in a few minutes or less of real time. A thorough description of this OFR system can be found in previous reports.51–54 We mitigated and/or avoided unreasonable chemical conditions inside the OFR (i.e., non-tropospheric VOC photolysis, VOC ozonolysis, and elevated RO2–RO2 interactions) by running the reactor in OFR185 mode (with both 185 and 254 nm lamps on) while maintaining high relative humidity (RH) (>50%) and low VOC mixing ratio (<95 ppb) per recommendations in a review paper published on this topic.51 In OFR 185 mode, O3 is generated via photolysis of O2 (using the 185 nm lamp) from recombination of O(3P) with O2. Once formed, O3 is simultaneously photolyzed with the 254 nm lamp generating O(1D) radicals that further react with water vapor to form OH radicals. OH exposure in the OFR was calibrated using the decay of toluene (Alfa Aesar Inc., ≥ 99.5%) concentration under the same RH but with changing OFR lamp settings, similar to the SO2 decay calibration described in Lambe et al. (2015)52 Additional details related to the OH exposure calibration procedure and associated OFR conditions are provided in the Text S1 and Table S1,† respectively.
To better represent atmospheric conditions, understanding the fate of RO2 in the OFR is needed because an unrealistic RO2/HO2 ratio would skew radical chemistry by favoring RO2 and disfavoring HO2 radical reaction pathways. Schervish and Donahue. (2021)55 recommended an RO2/HO2 ratio less than 1 in order to maintain an atmospherically relevant fate of RO2, whereby it mainly reacts with HO2 as opposed to RO2 cross reactions. The OFR RO2 fate estimator v1.051,54 was used to simulate the fate of the RO2 radicals based on the OH reactivity and O3 mixing ratio in the particle filter collection experiments and the results are presented in Fig. S1.† The RO2/HO2 ratio ranged from 0.67 to 0.77 for all experiments in this study which suggests that the RO2 reaction with HO2 is the dominant reaction pathway compared to others, e.g., RO2 with OH, RO2 with RO2, and RO2 isomerization.
2.2 SOA generation and collection
A summary of the OFR conditions used in each experiment is shown in Table 2. We first characterized the SOA mass yields across different OH exposures to identify the OH exposure leading to the highest SOA yields. The OH exposure refers to the integration of OH radical concentration across the residence time of the OFR. In these experiments, the OH exposure ranged from 4.06 ×1011 to 1.1 × 1012 molecules s cm−3, corresponding to an equivalent atmospheric photochemical age of 3 to 9 days (assuming an ambient OH concentration of 1.5 × 106 molecules cm−3).56 This data product is useful for comparing oxidation behavior of the acyclic terpenes with other model SOA systems that have been reported previously, including α-pinene oxidation from Lambe et al. (2015)52 The OH exposure settings that produced the largest SOA yields were used for subsequent experiments described below.
Table 2 Summary of experimental conditions used in the OFR
Experiment |
Precursor |
VOC ranges (ppb) |
RH (%) |
O3a (ppm) |
O3 reported here is the mixing ratio at the outlet because the OFR was run in 185 mode where O3 is generated inside the OFR.
|
SOA mass yield versus OH exposure |
β-myrcene |
32 ± 5 |
55 ± 3 |
1.7 |
β-ocimene |
41 ± 5 |
58 ± 2 |
1.6 |
Linalool |
56 ± 12 |
60 ± 2 |
1.6 |
α-pinene |
81 ± 5 |
59 ± 3 |
1.8 |
SOA mass yield curve |
β-myrcene |
2–31 |
58 ± 4 |
2.2 |
β-ocimene |
4–69 |
60 ± 2 |
2.0 |
Linalool |
3–54 |
59 ± 2 |
1.8 |
SOA yields were calculated as the ratio of condensed organic aerosol mass formed (COA) to precursor gas reacted (ΔVOC) in the OFR. COA was calculated based on SMPS size distributions with an assumed density of 1.3 g cm−3, a reasonable density for biogenic SOA.57–59 However, because this value is quite uncertain, we use the range of literature values to define the uncertainty in the calculated yield (density range of 1.2 g cm−3 to 1.4 g cm−3) as lower and upper bounds. A minimum of five SMPS scans (with one scan referring to both an up and down scan) were averaged for each SOA mass loading measurement. ΔVOC was calculated from cartridge samples collected from the inlet only because all acyclic terpenes have been fully reacted inside the OFR when inlet mixing ratio is less than 400 ppb (see further detail in Text S2†).
For further comparison of the SOA yields obtained in this study for acyclic terpenes with previously studied biogenic SOA systems, SOA mass yield curves were generated across a range of condensed organic aerosol mass loadings. This data product has been commonly reported for different chemical systems, including biogenic SOA formed in an OFR.43,45–47 These mass yield curves were fitted using two different models to estimate the absorption partitioning parameters: the volatility basis set (VBS)60 and the two-product model.61,62 The VBS model is described with the following equation:
| 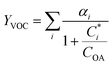 | (1) |
where
YVOC is the SOA mass yield for that VOC system;
COA denotes the mass concentration of organic aerosol;
αi is the mass-based stoichiometric yield for volatile product
i; and
C* is the saturation vapor concentration. In this study, five logarithmically spaced saturation vapor concentration bins from 0.1, 1, 10, 100 to 1000 μg m
−3 were used to capture the SOA mass yield under similar SOA mass loadings. The Odum two-product model is described with the following equation:
| 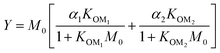 | (2) |
where
YVOC denotes the SOA mass yield for that VOC system;
M0 is the organic particle mass concentration (μg m
−3), and is equivalent to the term
COA in the VBS model;
KOMi is the partitioning coefficient of product
i, and
αi is the mass-based stoichiometric yield of product i.
2.3 High resolution mass spectrometry
For all acyclic terpene and α-pinene chemical systems, SOA samples were collected on Teflon filters (MilliporeSigma, Sigma Aldrich Inc., 0.2 μm PTFE membrane) at the OFR outlet. For each chemical system, three replicate experiments were performed, collecting one Teflon filter during each experiment. During SOA filter collection experiments, the O3 monitor was off and the sampling flow rate through the filter was 6.1 LPM using a vacuum pump. There were no residence time changes inside the OFR since the total flow was still maintained at 6.45 LPM, as shown in the OFR outlet “part 2” of Fig. 1. The detailed OH exposure and RO2/HO2 ratio for each type of SOA filter collection experiments are summarized in Table S2.† We acknowledge that some oligomerization could occur on the filter during particle collection with the long collection times required.63 However, this is a common method used for these types of experiments. For example, Nguyen et al. (2010)64 used a similar sample collection time when they investigated aerosol formation from isoprene ozonolysis with SOA mass loadings around 40 μg m−3. There is a trade-off between longer sample collection times and lower, more atmospherically relevant aerosol mass loadings, and our priority was to maintain low VOC mixing ratios in the OFR.
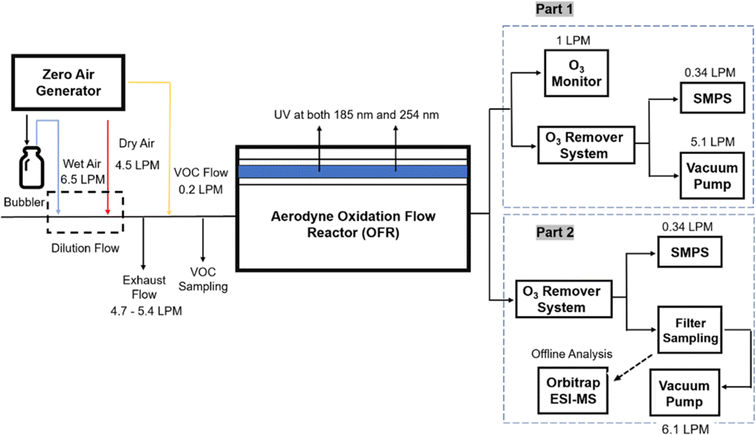 |
| Fig. 1 Schematic of the oxidation flow reactor. The experimental design used in this study which blue line, red line, and yellow line refer to wet air, dry air and VOC flow at the OFR inlet, respectively. Part 1 and part 2 at the OFR outlet are designed for different experiment purposes. | |
Filter sample preparation and extraction processes are described as follows. After finishing the collection, 4 mL acetonitrile (ACN) (optima LC-MS grade, Thermo Fisher Scientific) was added to a cleaned and dried extraction vial containing the sample filter cut into small pieces. The solution was then shaken for 15 min to extract the SOA from the filter using a toucher mixer shaker (Thermolyne Inc., Model M37615). Next, all filter pieces were removed from the vial with clean tweezers and the extract was evaporated to dryness using a vacuum solvent evaporator. Finally, the sample was reconstituted in 0.5 mL HPLC grade water (optima LC-MS grade, Thermo Fisher Scientific) as a final extraction solvent. These final extractions were analyzed immediately by ultra-performance liquid chromatography coupled to a high-resolution Q Exactive Plus Orbitrap mass spectrometer (Thermo scientific, USA). Three replicate analyses were performed for each extracted sample. Compound separations were achieved with using a Luna 1.6 μm Omega Polar C18 150 × 2.1 mm column (Phenomenex) fitted with a SecurityGuard ULTRA cartridge (porous polar C18, 2.1 mm; Phenomenex) maintained at 30 °C. Mobile phases is consisting of (A) 0.1% formic acid (optima LC-MS grade, Thermo Fisher Scientific) in LC-MS grade water (Fisher, Optima) and (B) 0.1% formic acid in LCMS grade acetonitrile (Fisher, Optima). Gradient elution was performed at a total flow rate of 300 μL min−1 with A/B mixture: starting as 5% B for 0–3 min, a linear gradient to 95% B from 3–14 min, 95% B for 14–16 min, linear gradient back to 5% B for 16–22 min. A heated electrospray ionization source was equipped with a Q Exactive Plus Orbitrap mass spectrometer (UPLC-HESI-HRMS; Thermo Scientific) with the following parameters: spray voltage of 2.50 kV (negative mode) and 3.50 kV (positive mode), capillary temperature of 320 °C (negative mode) and 325 °C (positive mode), auxiliary gas temperature of 320 °C, sheath gas flow rate of 35 (a.u.) and auxiliary gas flow rate of 10 (a.u.). Each sample extraction was analyzed for both in both positive (ESI (+)) and negative (ESI (−)) ion modes with the injection volume of 15 μL. In this study, a full scan data-dependent MS/MS (FS-ddMS2) approach was used with a scan range of m/z 100 to 750 with the mass resolving power of 140
000, and the top 7 most intensive ions from the adjacent full MS scan were performed in MS/MS scans.
The UHPLC-ESI-HRMS data were acquired and analyzed first using Xcalibur 4.2 software (Thermo Scientific), which was then processed using FreeStyle™ version 1.6.75.20 (Thermo Scientific). A similar procedure for HRMS data analysis is detailed on previous studies.65–67 Overall, three replicates and two blank samples (blank Teflon filter extraction) for each SOA sample were analyzed in both positive (ESI (+)) and negative (ESI (−)) ion modes. Peaks and their abundances were obtained using the Decon2LS software (https://omics.pnl.gov/software/decontools-decon2ls). The mass spectra shown in this study were all generated by integrating over the entire LC-MS run. Only ions that were observed in all three triplicate samples were included in the integrated mass spectrum. Additionally, ion signal intensity does not directly correlate with abundances without using authentic standards, which are not readily available from chemical suppliers. Consequently, the proportions shown here reflect only the signal intensities and not necessarily the relative abundances. Peaks containing 13C isotopes and originating from background were removed. Molecular formulae were assigned to each peak as CXHYOZ with an accuracy of ± 0.001 m/z units and constraining H/C to 0.30–2.25 and O/C to 0.00–2.30,40 where O/C denotes the oxygen to carbon ratio and H/C represents the ratio between hydron and carbon. All HRMS data hereafter are presented as formulae of the neutral SOA compounds combining both ionization modes. The adducts with H+ or Na+ for positive ions and deprotonation for negative ions were assumed to be the ionization mechanisms. The detailed compound elemental composition is performed by comparing O/C and carbon number of each SOA sample as described in the ESI.†
2.4 Volatility and viscosity prediction
The chemical properties of each SOA sample including volatility distribution and viscosity were estimated from the HRMS assigned molecular formulae with calculated oxidation state (OSC) and peak intensity. In brief, the volatility distribution of each SOA sample is determined based on the compositional parameterization developed by Li et al. (2016)68 which uses pure compound saturation mass concentration (C0) and elemental composition (the C, H and O number) to calculate SOA volatility. The viscosity and the glass transition temperature (Tg,i, defined as a temperature range where phase transition between an amorphous solid and semisolid states occurs) for each SOA was calculated following the methods from DeRieux et al. (2018)69 More details are given in the ESI supplemental materials (Text S3).†
2.5 Modeling gas-phase chemical mechanisms with GECKO-A
The photooxidation and ozonolysis reaction schemes of the three acyclic terpenes (β-myrcene, β-ocimene, and linalool) were modeled using the Generator for Explicit Chemistry and Kinetics of Organics in the Atmosphere (GECKO-A) model. A description of GECKO-A is given by Aumont et al. (2005)70 It is a modeling tool to generate nearly explicit gas-phase oxidation mechanisms for individual or multiple organic compounds under general atmospheric conditions.70–72 GECKO-A has been used previously to generate mechanisms for BVOC oxidation including α-pinene, limonene and camphene.73,74 The alignment of HRMS data with GECKO-A was used to generate possible gas-phase chemical mechanism for all acyclic terpenes. We did not include any matches between GECKO predictions and HRMS observations for hydroperoxides or peroxides because the analytical technique used in this study is not optimal for detecting those compounds.75
3 Results
3.1 SOA mass yield versus OH exposure
All acyclic terpenes reached a maximum SOA mass yield at a lower OH exposure than α-pinene (Fig. 2). For these experiments, the OH exposure ranged from 4.0 ×1011 to 1.1 × 1012 molecules s cm−3, which corresponds to an equivalent atmospheric photochemical age of 3 to 9 days, respectively, assuming an ambient OH concentration of 1.5 × 106 molecules cm−3.56 The OH exposure inflection points, where SOA mass yield transitions from a positive to a negative relationship with increasing OH exposure, were similar among all acyclic terpenes and occurred at lower OH exposure values compared to the reference cyclic compound, α-pinene. The inflection point indicates where the oxidation is shifting from functionalization to fragmentation dominated processes.52 At OH exposures below the inflection point, OH oxidation leads to reaction products that have more functional groups on the carbon backbone without reducing the number of carbons. The lower volatility of those gas-phase oxidation products promotes increased gas-particle partitioning. At OH exposures above the inflection point, OH oxidation increasingly breaks the main carbon backbone into smaller oxidation products, which have higher volatility and lower SOA yield. The highest SOA yield of β-myrcene, β-ocimene and linalool occurred at OH exposures of 4.3 × 1011, 4.1 ×1011 and 4.06 ×1011 molecules s cm−3, respectively. In addition, the α-pinene from this study exhibited maximum SOA yield at a slightly lower OH exposure point (5.14 ×1011) compared to the value from Lambe's study at 5.5 ×1011 molecules s cm−3, but this is within the uncertainty of the OH exposure calibration from the Lambe et al. (2015)52 study. Overall, the pattern of SOA yield curves for acyclic terpenes is expected because breaking a carbon–carbon bond on an acyclic compound will result in fragmentation of the carbon backbone while endocyclic carbon–carbon bond breaking would lead to ring-opening which maintains the original carbon backbone size. Therefore, our results suggest that photooxidation of acyclic terpenes is more prone to producing smaller, more volatile products via fragmentation reactions at lower OH exposures compared to many of the commonly studied cyclic terpene systems such as α-pinene. Another process that can contribute to lower yields is photolysis/photodegradation of the condensed organic material, leading to volatilization of the particle mass. This is unlikely to be a major loss process with the acyclic terpene SOA in this study because the photolysis lifetimes of biogenic SOA are typically on the order of hours in the absence of seed76 and the wavelengths of light using OFR185 mode do not efficiently photolyze OA.51
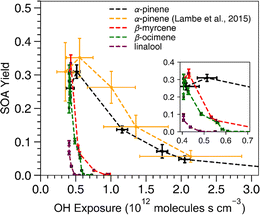 |
| Fig. 2 SOA mass yield versus OH exposure. The data from α-pinene experiments are from both this work (black) and Lambe et al. (2015)52 (yellow) for reference. The x-axis error bars represent standard deviations of OH exposures based on two replicate experiments for this study and were pulled from the Lambe et al. (2015)52 paper for their results. The error bars along the y-axis indicate standard deviations of the SOA mass yield calculated based on a particle density range of 1.2–1.4 g cm−3 to determine upper and lower bounds. | |
Of the acyclic terpenes, β-myrcene had the highest SOA mass yields at a maximum value of 0.33 ± 0.025, and it continued to generate SOA at higher OH exposures than the other acyclic terpenes. The SOA mass yield measured for β-myrcene in these experiments was consistent with a previously reported β-myrcene SOA mass yield value of 0.34 at 50 μg m−3 using similar OFR conditions.45 The highest SOA yield of α-pinene from the Lambe et al. (2015)52 study (0.35 ± 0.7) and our study (0.31 ± 0.02) was similar to β-myrcene (0.33 ± 0.025) and β-ocimene (0.31 ± 0.025). Linalool had the lowest SOA yield at 0.1 ± 0.001, possibly because it has fewer reactive alkene sites compared to the other acyclic terpenes.
3.2 SOA mass yield
SOA mass yields are commonly presented as a function of total condensed organic aerosol mass loading (COA) because these curves are used to develop fitting parameters for SOA models based on absorption-partitioning theory. To generate a similar plot, we conducted SOA experiments for each of the chemical systems using the OH exposure that produced the highest SOA yield (refer to Fig. 2). Thus, each of the curves presented in Fig. 3 represents an upper bound mass yield in the OFR under the conditions used in this study. The volatility basis set60 and the two-product61 absorption-partitioning models were used to fit the SOA yield data. The fitted parameters based on the VBS and 2-product models are summarized in Table 3 and the VBS fit is shown on Fig. 3 with the measured values.
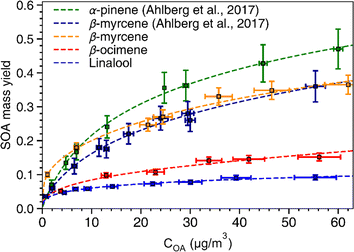 |
| Fig. 3 SOA mass yield curves. Yields of SOA for α-pinene, β-myrcene, β-ocimene, and linalool as a function of condensed organic aerosol (COA) mass concentration (μg m−3). The SOA yield data for α-pinene (green) and β-myrcene (dark blue) are from Ahlberg et al. (2017)45 The error bars along the y-axis indicate standard deviations of the SOA mass yield calculated based on a particle density range of 1.2–1.4 g cm−3. The fits shown in the figure were generated based on the volatility basis set60 model. | |
Table 3 Summary of fitted parameters for β-myrcene, β-ocimene and linalool using the two-product model and volatility-basis set (VBS) model
|
Two-product model |
Volatility-basis set model |
α
1
|
α
2
|
K
OM1
|
K
OM2
|
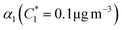
|
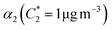
|
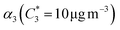
|
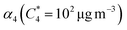
|
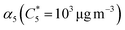
|
β-Ocimene |
0.08 |
12.3 |
0.27 |
8.8 × 10−5 |
0.022 |
0.011 |
0.058 |
6.445 × 10−4 |
1.088 |
β-Myrcene |
0.10 |
0.33 |
3.35 |
0.03 |
0.061 |
0.026 |
0.113 |
0.328 |
0.017 |
Linalool |
0.04 |
0.07 |
1.11 |
0.02 |
0.020 |
0.021 |
0.007 |
0.085 |
2.204 × 10−4 |
α-Pinene had the highest SOA mass yield compared to all acyclic terpenes (0.07–0.48) across the aerosol mass loading range, particularly at mass loadings above 10 μg m−3. Of the acyclic terpenes, β-myrcene (0.08–0.36) had the highest SOA yield, followed by β-ocimene (0.04–0.1) and linalool (0.04–0.08). The reason linalool has a particularly low SOA yield might be attributed to its lower reactivity with one less double bond compared to the other acyclic terpene systems. The experimental data were fitted with the VBS model using the parameters given in Table 3. For comparison, across the chemical systems at aerosol mass loadings of 10 μg m−3 (an atmospherically relevant aerosol mass concentration in a moderately polluted region1), the estimated SOA yields from the fits for α-pinene, β-myrcene, β-ocimene and linalool were 0.2, 0.18, 0.08, and 0.06, respectively. Interestingly, there is some disagreement between this study and the Ahlberg et al. (2017)45 report about the trend in α-pinene and β-myrcene SOA mass yield values at aerosol loadings below 10 μg m3; this study shows β-myrcene with slightly higher SOA mass yields than α-pinene at the smaller mass loadings, but the yield values measured in this study agree very well with the Ahlberg et al. (2017)45 study at higher mass loadings. The SOA yield for β-myrcene of 0.18 at 10 μg m−3 in this study agrees well with the value of 0.17 in Ahlberg et al. (2017).45 In OFRs, vapor wall losses are higher under conditions with a small condensational sink.77 This can reduce SOA yields, particularly at low mass loadings, so increased sensitivity of the system to vapor wall losses could potentially explain the differences in yield observed between this study and Ahlberg et al.45 The trend in SOA yields for acyclic terpenes from highest to lowest correspond well with a couple previous studies.76,84 However, we acknowledge that comparisons of SOA mass yields between chambers generally, including between OFR and smog chambers, should be approached cautiously because there are many variables that can influence yield. In OFRs, condensed phase chemistry that can produce less volatile material could be inhibited, such as oligomerization processes.51 If those processes are particularly inhibited in the acyclic terpene SOA systems, this could explain the yield trends. A non-exhaustive list of other variables that can influence SOA yield includes residence time (for any flow reactor approach), vapor wall loss, presence of seed, type of seed, humidity, radical chemistry, etc.
3.3 SOA chemical composition and properties
3.3.1 High-resolution mass spectrometry.
The high-resolution mass spectra of SOA formed from oxidation of α-pinene, β-myrcene, β-ocimene and linalool are shown in Fig. 4. Each acyclic terpene SOA system was plotted with the reference system, α-pinene, for comparison. The spectra include peaks originating from the combination of both positive (ESI (+)) and negative modes (ESI (−)) analyses, with the most abundant peaks for each SOA type labeled. At lower masses (<180 Da), both β-myrcene and β-ocimene SOA exhibit high signal intensity. At higher masses (>200 Da), β-myrcene had a much smaller contribution of peaks compared to α-pinene and β-ocimene. The contribution of peaks >280 Da in β-ocimene SOA is significantly higher than that observed in α-pinene and β-myrcene SOA at these larger masses. Most of the major peaks >280 Da were identified as C11 and C21 compounds. We did not observe a well-defined monomer versus oligomer region in α-pinene SOA, which has been presented previously in chamber experiment SOA.78 This is likely due to a much lower RO2/HO2 ratio in these OFR experiments compared to previous chamber experiments, which frequently require very high VOC mixing ratios to generate enough mass loadings for high resolution composition analysis (e.g., 500 ppb of reacted α-pinene in previous study85). High VOC mixing ratios can lead to elevated RO2–RO2 oligomerization reactions instead of favoring more atmospherically relevant RO2–HO2 reactions.79 Our RO2/HO2 ratio ranged from 0.67 to 0.77, within the recommended range for reproducing atmospherically relevant RO2 chemistry.55 Other than the differences in oligomer contributions, most of the major peaks observed in α-pinene SOA agree well with previous studies, including C8H12O5, C10H16O5, C9H14O3 and C8H12O2.78,80–82 There was substantial overlap in the most abundant peaks in α-pinene SOA, β-myrcene SOA, and β-ocimene SOA, including C8H12O5, C8H12O2, C9H14O3, C7H8O3, and C7H10O2. While these peaks all have the same exact mass, they are very likely structural isomers with different formation mechanisms. This will be discussed in more detail based on GECKO-A model simulations in Section 3.3.2. Interestingly, SOA chemical complexity varied between the different systems. This is shown by plotting the cumulative normalized intensities versus the number of peaks required to reach that cumulative intensity (Fig. S2†). Over 500 different peaks contributed to 80% of cumulative normalized intensity for α-pinene and β-myrcene SOA. In comparison, just 170 peaks contributed to 80% of cumulative intensity for linalool demonstrating lower chemical diversity, while over 800 peaks contributed to 80% of cumulative intensity for β-ocimene SOA demonstrating higher chemical diversity.
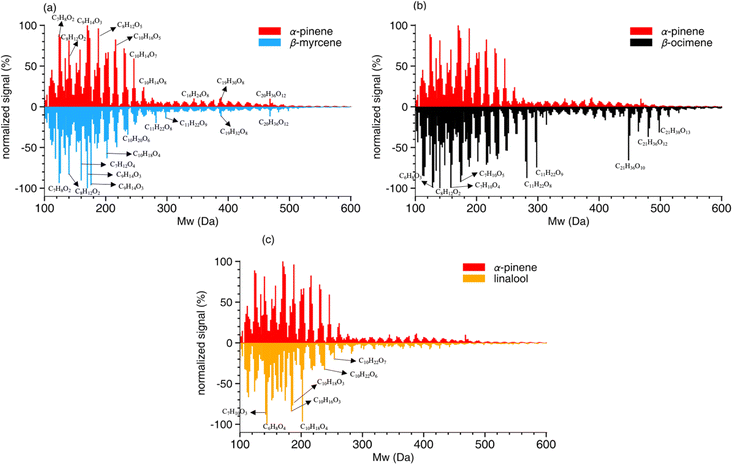 |
| Fig. 4 SOA mass spectra. High-resolution mass spectra of SOA formed from photooxidation of α-pinene compared to the mass spectra of SOA formed from (a) β-myrcene, (b) β-ocimene, and (c) linalool in an OFR. Spectra from both ESI (+) and ESI (−) modes were combined, and the x-axis represents the neutral mass. The signal for each peak is scaled to the normalized intensity. | |
A summary of the chemical composition from all SOA samples is provided in Table 4. The monomer and oligomer compounds are defined as the peaks that have C4–C10 and C11–C20 carbon numbers, respectively, since all VOCs included in this study are C10 compounds and any compound with more than 10 carbons would necessitate carbon addition. β-Ocimene SOA had the highest contribution of oligomers (31.4%) while α-pinene and β-myrcene SOA had similar contribution of oligomers at 23.9% and 25.5%, respectively, and linalool SOA had the lowest oligomer contribution at 19.7%. Interestingly, a significant oligomeric contribution in β-ocimene SOA is clearly derived from C11 compounds (24%) and C21 compounds (9%) that were not observed in the other SOA types. Linalool SOA exhibited the smallest degree of fragmentation with C10 compounds contributing up to 31% of the monomeric signal. In terms of oxygen distribution for monomers and oligomers, linalool SOA had the lowest oxygen-to-carbon (O/C) ratio of 0.51. The α-pinene and β-myrcene SOA had similar oxidation levels with O/C ratio of 0.56, and β-ocimene had the highest O/C ratio of 0.61.
Table 4 Summary of SOA composition. The contribution of different molecular formulae identified in SOA mass spectra based on high resolution mass spectrometry; the color axis indicates the relative contribution to the total for each category indicated in the left column and is based on the relative abundance of signal intensity
3.3.2 GECKO-A modeling.
GECKO mechanistic modeling was used to explore the possible chemical mechanisms of acyclic terpene oxidation. The first four generations of reaction products generated by GECKO-A for all acyclic terpenes included in this study are shown in the ESI (Fig. S3–S5†), and several identified peaks in the UHPLC-ESI-HRMS spectra that have the same exact mass as chemical structures predicted by GECKO-A are provided in Table S3.† It is important to note that many of the peaks could have multiple structural isomers and we cannot confirm the exact structures with the MS data. The reaction of OH radicals with terpenes is mainly initiated by the addition of OH to the C
C double bond or, to a lesser extent, by hydrogen abstraction. A larger fraction of C9 carbonyl compounds is formed from β-myrcene photooxidation owing to its two terminal double bonds compared to β-ocimene. Alkyl radicals are formed from this initial step and rapidly react with O2 to generate peroxy radicals (RO2). The fate of the RO2 radical depends on the environmental conditions, including the RO2/HO2 ratio.83–85 They can combine with HO2, OH, or another RO2 to form stable products.54 At RO2/HO2 ratios larger than one, RO2 + RO2 interactions are enhanced relative to ambient atmospheric conditions. At values less than one, RO2 + HO2 interactions are promoted, better representing the chemical interactions that occur in Earth's atmosphere. The RO2/HO2 ratios estimated in these experiments ranged from 0.67–0.77 (see Methods section 2.1). Based on the OFR RO2 Fate Estimator v1.0.,51 approximately 89.4% of β-myrcene oxidation products can be attributed to the RO2/HO2 reaction, while less than 1% of oxidation products result from RO2/RO2 reaction.
The composition of β-ocimene SOA was less straightforward to explain. A significant fraction of the signal in β-ocimene SOA was attributed to C11 and C21 compounds which did not contribute appreciably to the total signal in the other SOA types in this study. We attributed the C11 and C21 compounds to oligomers; all SOA precursors were C10 compounds and therefore the formation of C11 and C21 compounds requires the combination of two smaller compounds. One possible β-ocimene chemical mechanism that could generate the C11 signals in the mass spectrum was developed by synthesizing GECKO-A predictions with UHPLC-ESI-HRMS data (Fig. 5). It was of particular interest that these C11 peaks were prominent only in the ocimene SOA and not in any other SOA types in this study. Furthermore, GECKO only predicted five-carbon and six-carbon products from ocimene oxidation and not any of the other SOA precursors (Fig. S3–S5†). It is possible that the GECKO pathways that produced the five-carbon and six-carbon products unique to the ocimene system were also the pathways leading to the prominent C11 compounds. Another observation of note is the C11 compounds were highly oxygenated molecules (HOMs) with chemical formulae, C11H20–22O8–9. The presence of these HOMs suggested a possible autooxidation mechanism formed via hydroperoxide intermediates.83 One of the five-carbon peroxy radical intermediates predicted by GECKO would serve as a likely candidate for autooxidation because the alkyl radical formed after a hydrogen shift would be located in a stabilized allylic position (simplified mechanism shown in Fig. 5). This autooxidation mechanism could produce two HOM products, C5H10O5 and C5H10O6. It is challenging to confirm the presence of these peaks with the MS data because the analytical method used here is not particularly sensitive to organic peroxides and they are known to fragment easily during ionization with ESI.75,86,87 However, multifunctionalized peroxides have been observed with ESI in positive mode previously, so it is possible the instrument would be capable of detecting these structures.88,89 We observed structures in positive mode that could match C5H10O5 and C5H10O6 or a fragment that lost an HOOH group (C5H8O4).
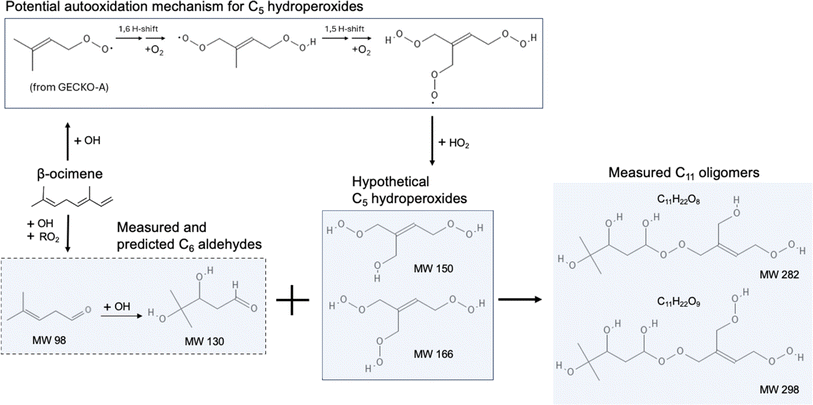 |
| Fig. 5 One possible reaction mechanism that could generate C11 oligomers from β-ocimene oxidation. The C6 aldehydes in the shaded box with a dashed outline are predicted by GECKO-A modeling and have matching neutral masses in the ESI HRMS spectrum. | |
Once in the condensed phase, hydroperoxides can combine with aldehydes to generate peroxyhemiacetal dimers85 which would also only be detectable in positive mode. In fact, in a study comparing various accretion reactions between compounds including hydroperoxide, hydroxyl, carboxyl, aldehyde, and ketone functional groups, the reaction of hydroperoxides and aldehydes to form peroxyhemiacetals were the most favorable even without the presence of an acid catalyst.90 In addition, Hall and Johnston91 indicated that under low-NOx conditions, because of the dominant fate of RO2 reacting with HO2, hydroperoxides are likely to be formed and further react with aldehydes which subsequently contributes to peroxyhemiacetal production, thereby lowering SOA volatility. The C11H20O8–9 structures were only observed in negative mode so they cannot be peroxyhemiacetals. However, the C11H22O8–9 structures were present in multiple LC peaks in both positive and negative mode. This means there were a few different compounds contributing to this signal in the integrated mass spectrum, some of which could be peroxyhemiacetals. One six-carbon aldehyde predicted by GECKO is C6H12O3, formed via multi-generation OH oxidation. This could combine with the five-carbon HOMs to generate peroxyhemiacetals with the formulae C11H22O8 and C11H22O9 (Fig. 5). Unfortunately, the exact structures of the C11 HOMs cannot be confirmed with the MS/MS data collected during these experiments. Additionally, this mechanism could only account for the signal observed in positive mode so there is still some unexplained chemistry leading to other C11 peaks observed in negative mode. One alternate explanation for the presence of oligomers is the long filter collection time required (see Methods section); others have published results showing that condensed phase chemistry can occur on filters themselves.63 However, this cannot explain why the C11 and C21 oligomers are only observed in β-ocimene SOA and not in all SOA systems in this study. The prominent C21 peaks in the ocimene SOA mass spectrum could be the product of these C11 compounds combining with one of the many C10 products and intermediates that would form in this chemical system. Recent studies by Kenseth et al.92,93 proposed a possible mechanism for the production of oligomeric esters formed from hydroperoxides in the particle phase that could potentially contribute to these C21 peaks, but could not explain the production of the C11 HOMs. There are too many possible C10 + C11 combinations to pinpoint a particular mechanism here, but further exploration of ocimene oxidation mechanisms would be a natural follow-up to this study.
Some of the products shown in Table S4† with matching exact masses in the mass spectra are only predicted via ozonolysis even though we did not expect significant ozonolysis chemistry to occur in the OFR based on our calculations of VOC reaction rates via OH and ozonolysis. The fate of each VOC reacting with OH and O3 was estimated using reaction rate constants of β-myrcene, β-ocimene, and linalool (kmyrcene–OH/O3, kocimene–OH/O3, and klinalool–OH/O3), which are summarized in Table 1. Results are reported in Table S4.† For all the experimental conditions shown in Table S2,† the fate of β-myrcene, β-ocimene, and linalool should primarily be OH oxidation with more than 98% expected to react with OH. However, the SOA composition data clearly showed evidence of ozonolysis oxidation products. For example, C8 compounds contributed 12.2% and 10.2% to the total signal for β-myrcene and β-ocimene SOA, respectively, even though these products are only predicted to form via ozonolysis and not OH oxidation (note: these percentages are different from Table 4 which shows the percent contribution to total monomer signal, not the % contribution to total signal). One possible explanation could be that ozonolysis occurred on the Teflon filter itself as the SOA samples were collected, downstream of the OFR. While several measures were implemented to prevent this from occurring (including installation of two custom-built ozone scrubbers upstream of the filter assembly), there was still ∼20 ppb O3 remaining downstream of the scrubbers. With an SOA filter collection time of 15 hours, 20 ppb O3 could very well lead to chemistry on the filter outside of the OFR. This is one of the challenges when using an OFR system where ozone mixing ratios are very high, often in the tens of ppm. Therefore, the ozonolysis chemistry shown in the simplified mechanism may not have occurred within the OFR itself and we cannot exclude this possibility.
3.3.3 SOA volatility, and viscosity.
Volatility and viscosity of SOA components were estimated based on the molecular formulae identified in the high-resolution mass spectra. This is displayed as the glass transition temperature (Tg) versus saturation mass concentration (C0) for each molecular formula, with the color of the markers representing the oxygen-to-carbon, or O
:
C, ratio (Fig. 6). C0 is calculated based on eqn (S2 in the ESI)† and is divided into four volatility categories including extremely low volatility organic compounds (ELVOC; C0 < 3 × 10−4 μg m−3), low-volatility organic compounds (LVOC; 3 × 10−4 < C0 < 0.3 μg m−3), semi-volatile organic compounds (SVOC; 0.3 < C0 < 300 μg m−3), and intermediate volatility organic compounds (IVOC; 300 < C0 < 3 × 106 μg m−3).94 Many compounds fall in the IVOC region for α-pinene,β-myrcene, and especially linalool SOA. The elemental composition (e.g., O
:
C ratio and number of carbons, or nC) and volatility distribution for each SOA system is presented in Fig. S6.† Among these three SOA systems, α-pinene has a relatively larger contribution of compounds in the lower volatility region, followed by β-myrcene and linalool SOA. A much larger contribution of oxidation products in the ELVOC and LVOC regions are identified in β-ocimene SOA compared to all the other SOA types. Most of the ELVOC and LVOC compounds are C11 and C21 compounds, which are absent in the other SOA systems investigated in this study. The production of highly oxygenated organic molecules (HOMs) of ELVOC from auto-oxidation processes has been shown to significantly contribute to SOA formation.15,95 It is possible that acyclic monoterpenes could also undergo auto-oxidation and be contributing to some of the ELVOCs observed in this study. However, the absence of auto-oxidation mechanisms in GECKO-A makes it difficult to assess HOM production in this case.
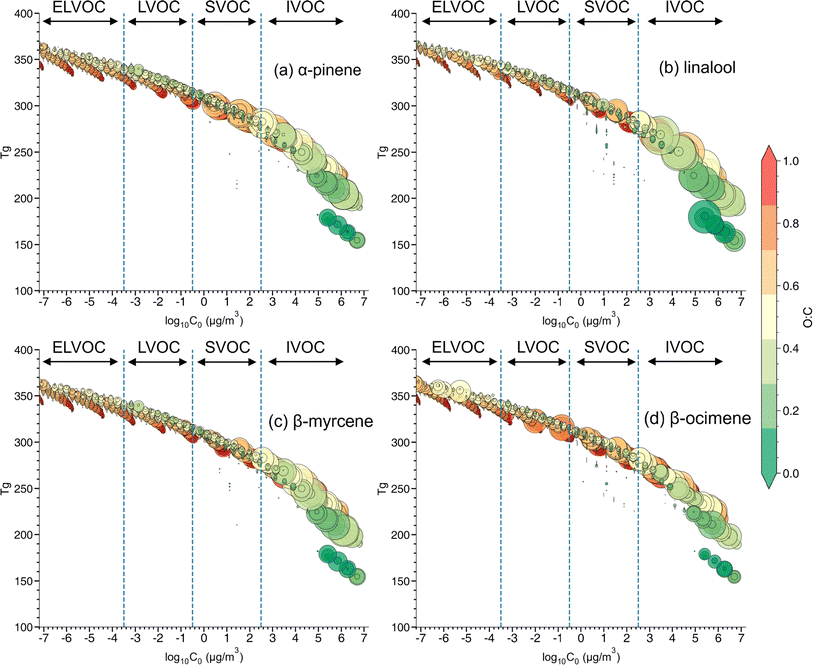 |
| Fig. 6 Predicted glass transition temperature (Tg) as a function of saturation mass concentration (C0) of individual compounds derived from the SOA mass spectra from (a) α-pinene, (b) linalool, (c) β-myrcene, and (d) β-ocimene SOA samples. The marker size represents normalized intensity of each peak to the total signal and color bar indicates oxygen-to-carbon (O : C) ratio. | |
The estimated glass transition temperature (Tg) for each peak decreases as C0 increases, as expected.40,94 The overall value of Tg for each type of SOA is estimated by integrating the individual Tg values for each molecular formula observed in that SOA system. The lower volatility oxidation products identified in β-ocimene SOA led to the highest Tg (263.6 K), followed by α-pinene (258.8 K), β-myrcene (255.3 K), and linalool SOA (245.2 K). The estimated particle viscosity values are 680 Pa s, 310 Pa s, 181 Pa s, and 42.7 Pa s for β-ocimene, α-pinene, β-myrcene, and linalool SOA, respectively, with the highest viscosity in β-ocimene SOA driven by oligomers. Under relatively high RH (>70%) and typical ambient temperatures (>295 K) during a field campaign at a rural site in southeastern US, where SOA is mainly formed by oxidation of isoprene and monoterpenes, the viscosity of ambient organic-dominated particles were often less than 100 Pa s, exhibiting mostly a liquid phase behavior. While at lower RH (<50%), the viscosity value of ambient organic-dominated particles was higher than 100 Pa s,96 values typical for a non-liquid phase. The lower Tg value due to the relatively lower MW oxidation products, could result in lower viscosity at similar RH and temperature range.69 Our results demonstrated that linalool SOA is less viscous than β-myrcene, β-ocimene and α-pinene SOA, suggesting more liquid-like particles in the OFR. The variation in viscosity between acyclic terpene SOA types in this study highlights that lumping all acyclic terpenes together could lead to inaccurate predictions of SOA properties. Compared with other studies using the same parameterization for estimating viscosity, the particle viscosity for α-pinene SOA at RH around 50% is much lower in this study than the value of 103 to 104 Pa s reported by DeRieux et al. (2018).69 This difference in reports could be attributed to the difference of RO2/HO2 ratio between the environmental smog chamber used in the previous study and the OFR used in this study. Higher RO2/HO2 ratios in the OFR prevents suppresses RO2 + RO2 fate which would contribute to elevated oligomerization reactions, lower volatility oxidation products, higher glass transition temperatures, and thus higher viscosity in chamber experiments.
4 Conclusion
We conducted several OFR experiments to investigate SOA formation, chemical composition, and physical properties from photooxidation of acyclic terpenes, including β-myrcene, β-ocimene, and linalool. Results for α-pinene are also provided as a benchmark reference system for comparison. Plant emissions of acyclic terpenes are commonly induced by adverse conditions leading to plant stress – conditions that are increasing in frequency and intensity in a changing climate. The result showed that all acyclic terpenes were prone to fragmentation reactions at relatively low OH exposures, leading to lower SOA mass yields compared to α-pinene at a comparable OH exposure. Higher abundance of acyclic terpenes in future climate conditions would warrant more explicit absorption-partitioning treatment of acyclic terpene oxidation products to improve predictions of SOA formation and properties. We observed significant contributions of C11 and C21 oligomers in β-ocimene SOA and it remains unclear why similar oligomerization reactions were not observed in the other acyclic terpene systems. Combining UHPLC-ESI-HRMS data with oxidation schemes from GECKO-A model simulations, we proposed one possible chemical mechanisms that could contribute to some of the C11 compounds. There appears to be something unique about the SOA chemistry associated with β-ocimene oxidation and thus additional investigations focused on measuring gas-phase reaction products directly and leveraging additional analytical techniques to probe oligomer chemistry in the condensed phase, particularly those that can target organic peroxide analysis, would be a natural follow-up to this study. Estimated volatility and viscosity of the different SOA systems in this study indicated that changes in BVOC emission profiles under stressed conditions could alter ambient SOA chemical composition and physical properties. In particular, β-ocimene SOA was more similar to α-pinene SOA than β-myrcene SOA in its volatility distribution and viscosity, which has not been highlighted in any previous studies. This demonstrates substantial variation even between different acyclic terpene SOA systems. One limitation of the current study is that the primary pathway for SOA production was through nucleation (e.g. no seed particles were used). However, there are many other pathways to SOA formation in the atmosphere that were outside the scope of this study to explore in detail. For example, it is possible that oxidation products derived from these acyclic BVOCs could undergo multiphase chemistry within pre-existing wet acidic aerosols, as shown for IEPOX SOA formation in the eastern U. S.97 Future studies should explore the potential role of acid-catalyzed multiphase chemistry associated with these non-isoprene acyclic BVOCs. Given the projected increases in plant stress conditions in a rapidly changing climate, the contribution of acyclic terpenes to the atmospheric aerosol budget may increase and need to be included in chemical transport models. However, SOA chemistry could vary strongly for some acyclic terpenes, thus lumping these compounds into a single VOC group may be insufficient to accurately predict how these changes will alter climate-relevant SOA properties.
Author contributions
CF designed the experimental study and helped with data analysis and interpretation. SG performed the OFR experiments and analyzed OFR data and HRMS data. SG wrote the manuscript. VP helped with running samples on the Orbitrap and analyzing the MS data. The manuscript was edited by FK, VP, and CF.
Conflicts of interest
There are no conflicts of interest to declare.
Acknowledgements
This work was supported by National Science Foundation AGS program (Award #2035125). Funding for the Orbitrap instrument used in this paper was provided by NSF MRI (Chemistry; Award #1920242). CF acknowledges U See I Write, a faculty writing initiative at the University of California, Irvine, for providing protected writing time during which a substantial portion of this manuscript was written.
References
- M. Hallquist, J. C. Wenger, U. Baltensperger, Y. Rudich, D. Simpson, M. Claeys, J. Dommen, N. M. Donahue, C. George, A. H. Goldstein, J. F. Hamilton, H. Herrmann, T. Hoffmann, Y. Iinuma, M. Jang, M. E. Jenkin, J. L. Jimenez, A. Kiendler-Scharr, W. Maenhaut, G. McFiggans, T. F. Mentel, A. Monod, A. S. H. Prevot, J. H. Seinfeld, J. D. Surratt, R. Szmigielski and J. Wildt, Atmos. Chem. Phys., 2009, 82 Search PubMed.
- M. Shrivastava, C. D. Cappa, J. Fan, A. H. Goldstein, A. B. Guenther, J. L. Jimenez, C. Kuang, A. Laskin, S. T. Martin, N. L. Ng, T. Petaja, J. R. Pierce, P. J. Rasch, P. Roldin, J. H. Seinfeld, J. Shilling, J. N. Smith, J. A. Thornton, R. Volkamer, J. Wang, D. R. Worsnop, R. A. Zaveri, A. Zelenyuk and Q. Zhang, Rev. Geophys., 2017, 55, 509–559 CrossRef.
- J. P. D. Abbatt, A. K. Y. Lee and J. A. Thornton, Chem. Soc. Rev., 2012, 41, 6555 RSC.
- M. Shiraiwa, M. Ammann, T. Koop and U. Pöschl, Proc. Natl. Acad. Sci. U. S. A., 2011, 108, 11003–11008 CrossRef CAS PubMed.
-
IPCC, Climate Change 2014: Synthesis Report. Contribution of Working Groups I, II and III to the Fifth Assessment Report of the Intergovernmental Panel on Climate Change, ed. R. K. Pachauri and L. A. Meyer, IPCC, Geneva, Switzerland, p. 151 Search PubMed.
- N. Mahowald, D. S. Ward, S. Kloster, M. G. Flanner, C. L. Heald, N. G. Heavens, P. G. Hess, J.-F. Lamarque and P. Y. Chuang, Annu. Rev. Environ. Resour., 2011, 36, 45–74 CrossRef.
-
National Academies of Sciences Engineering and Medicine, The Future of Atmospheric Chemistry Research: Remembering Yesterday, Understanding Today, Anticipating Tomorrow, The National Academies Press, Washington, DC, 2016 Search PubMed.
- U. Baltensperger, J. Dommen, M. R. Alfarra, J. Duplissy, K. Gaeggeler, A. Metzger, M. C. Facchini, S. Decesari, E. Finessi, C. Reinnig, M. Schott, J. Warnke, T. Hoffmann, B. Klatzer, H. Puxbaum, M. Geiser, M. Savi, D. Lang, M. Kalberer and T. Geiser, J. Aerosol Med. Pulm. Drug Deliv., 2008, 21, 145–154 CrossRef CAS PubMed.
- J. Lelieveld, J. S. Evans, M. Fnais, D. Giannadaki and A. Pozzer, Nature, 2015, 525, 367–371 CrossRef CAS PubMed.
- J. L. Jimenez, M. R. Canagaratna, N. M. Donahue, A. S. H. Prevot, Q. Zhang, J. H. Kroll, P. F. DeCarlo, J. D. Allan, H. Coe, N. L. Ng, A. C. Aiken, K. S. Docherty, I. M. Ulbrich, A. P. Grieshop, A. L. Robinson, J. Duplissy, J. D. Smith, K. R. Wilson, V. A. Lanz, C. Hueglin, Y. L. Sun, J. Tian, A. Laaksonen, T. Raatikainen, J. Rautiainen, P. Vaattovaara, M. Ehn, M. Kulmala, J. M. Tomlinson, D. R. Collins, M. J. Cubison, E. J. Dunlea, J. A. Huffman, T. B. Onasch, M. R. Alfarra, P. I. Williams, K. Bower, Y. Kondo, J. Schneider, F. Drewnick, S. Borrmann, S. Weimer, K. Demerjian, D. Salcedo, L. Cottrell, R. Griffin, A. Takami, T. Miyoshi, S. Hatakeyama, A. Shimono, J. Y. Sun, Y. M. Zhang, K. Dzepina, J. R. Kimmel, D. Sueper, J. T. Jayne, S. C. Herndon, A. M. Trimborn, L. R. Williams, E. C. Wood, A. M. Middlebrook, C. E. Kolb, U. Baltensperger and D. R. Worsnop, Science, 2009, 326, 1525–1529 CrossRef CAS PubMed.
- A. L. Robinson, N. M. Donahue, M. K. Shrivastava, E. A. Weitkamp, A. M. Sage, A. P. Grieshop, T. E. Lane, J. R. Pierce and S. N. Pandis, Science, 2007, 315, 1259–1262 CrossRef CAS PubMed.
- T. Kurtén, K. Tiusanen, P. Roldin, M. Rissanen, J.-N. Luy, M. Boy, M. Ehn and N. Donahue, J. Phys. Chem. A, 2016, 120, 2569–2582 CrossRef PubMed.
- M. Kulmala, J. Kontkanen, H. Junninen, K. Lehtipalo, H. E. Manninen, T. Nieminen, T. Petäjä, M. Sipilä, S. Schobesberger, P. Rantala, A. Franchin, T. Jokinen, E. Järvinen, M. Äijälä, J. Kangasluoma, J. Hakala, P. P. Aalto, P. Paasonen, J. Mikkilä, J. Vanhanen, J. Aalto, H. Hakola, U. Makkonen, T. Ruuskanen, R. L. Mauldin, J. Duplissy, H. Vehkamäki, J. Bäck, A. Kortelainen, I. Riipinen, T. Kurtén, M. V. Johnston, J. N. Smith, M. Ehn, T. F. Mentel, K. E. J. Lehtinen, A. Laaksonen, V.-M. Kerminen and D. R. Worsnop, Science, 2013, 339, 943–946 CrossRef CAS PubMed.
- J. Tröstl, W. K. Chuang, H. Gordon, M. Heinritzi, C. Yan, U. Molteni, L. Ahlm, C. Frege, F. Bianchi, R. Wagner, M. Simon, K. Lehtipalo, C. Williamson, J. S. Craven, J. Duplissy, A. Adamov, J. Almeida, A.-K. Bernhammer, M. Breitenlechner, S. Brilke, A. Dias, S. Ehrhart, R. C. Flagan, A. Franchin, C. Fuchs, R. Guida, M. Gysel, A. Hansel, C. R. Hoyle, T. Jokinen, H. Junninen, J. Kangasluoma, H. Keskinen, J. Kim, M. Krapf, A. Kürten, A. Laaksonen, M. Lawler, M. Leiminger, S. Mathot, O. Möhler, T. Nieminen, A. Onnela, T. Petäjä, F. M. Piel, P. Miettinen, M. P. Rissanen, L. Rondo, N. Sarnela, S. Schobesberger, K. Sengupta, M. Sipilä, J. N. Smith, G. Steiner, A. Tomè, A. Virtanen, A. C. Wagner, E. Weingartner, D. Wimmer, P. M. Winkler, P. Ye, K. S. Carslaw, J. Curtius, J. Dommen, J. Kirkby, M. Kulmala, I. Riipinen, D. R. Worsnop, N. M. Donahue and U. Baltensperger, Nature, 2016, 533, 527–531 CrossRef PubMed.
- M. Ehn, J. A. Thornton, E. Kleist, M. Sipilä, H. Junninen, I. Pullinen, M. Springer, F. Rubach, R. Tillmann, B. Lee, F. Lopez-Hilfiker, S. Andres, I.-H. Acir, M. Rissanen, T. Jokinen, S. Schobesberger, J. Kangasluoma, J. Kontkanen, T. Nieminen, T. Kurtén, L. B. Nielsen, S. Jørgensen, H. G. Kjaergaard, M. Canagaratna, M. D. Maso, T. Berndt, T. Petäjä, A. Wahner, V.-M. Kerminen, M. Kulmala, D. R. Worsnop, J. Wildt and T. F. Mentel, Nature, 2014, 506, 476–479 CrossRef CAS PubMed.
- T. Jokinen, T. Berndt, R. Makkonen, V.-M. Kerminen, H. Junninen, P. Paasonen, F. Stratmann, H. Herrmann, A. B. Guenther, D. R. Worsnop, M. Kulmala, M. Ehn and M. Sipilä, Proc. Natl. Acad. Sci. U. S. A., 2015, 112, 7123–7128 CrossRef CAS PubMed.
- M. Shiraiwa and J. H. Seinfeld, Geophys. Res. Lett., 2012, 39, 2012GL054008 CrossRef.
- A. Guenther, T. Karl, P. Harley, C. Wiedinmyer, P. I. Palmer and C. Geron, Atmos. Chem. Phys., 2006, 30 Search PubMed.
- A. B. Guenther, X. Jiang, C. L. Heald, T. Sakulyanontvittaya, T. Duhl, L. K. Emmons and X. Wang, Geosci. Model Dev., 2012, 5, 1471–1492 CrossRef.
- A. B. Jardine, K. J. Jardine, J. D. Fuentes, S. T. Martin, G. Martins, F. Durgante, V. Carneiro, N. Higuchi, A. O. Manzi and J. Q. Chambers, Geophys. Res. Lett., 2015, 42, 1576–1583 CrossRef CAS.
- N. Dudareva, F. Negre, D. A. Nagegowda and I. Orlova, Crit. Rev. Plant Sci., 2006, 25, 417–440 CrossRef CAS.
- R. Atkinson and J. Arey, Acc. Chem. Res., 1998, 31, 574–583 CrossRef CAS.
- R. Atkinson and J. Arey, Chem. Rev., 2003, 103, 4605–4638 CrossRef CAS PubMed.
- K. Mermet, E. Perraudin, S. Dusanter, S. Sauvage, T. Léonardis, P.-M. Flaud, S. Bsaibes, J. Kammer, V. Michoud, A. Gratien, M. Cirtog, M. Al Ajami, F. Truong, S. Batut, C. Hecquet, J.-F. Doussin, C. Schoemaecker, V. Gros, N. Locoge and E. Villenave, Sci. Total Environ., 2021, 756, 144129 CrossRef CAS PubMed.
- Ü. Niinemets, Trends Plant Sci., 2010, 15, 145–153 CrossRef PubMed.
- Ü. Niinemets, For. Ecol. Manage., 2010, 260, 1623–1639 CrossRef.
- J. K. Holopainen and J. Gershenzon, Trends Plant Sci., 2010, 15, 176–184 CrossRef CAS PubMed.
- J. D. Blande, J. K. Holopainen and Ü. Niinemets, Plant, Cell Environ., 2014, 37, 1892–1904 CrossRef CAS PubMed.
- Ü. Niinemets, A. Kännaste and L. Copolovici, Front. Plant Sci., 2013, 4, 262 Search PubMed.
- C. L. Faiola, B. T. Jobson and T. M. VanReken, Biogeosciences, 2015, 12, 527–547 CrossRef.
- J. K. Holopainen and J. D. Blande, Front. Plant Sci., 2013, 4 DOI:10.3389/fpls.2013.00185.
- F. Loreto and J.-P. Schnitzler, Trends Plant Sci., 2010, 15, 154–166 CrossRef CAS PubMed.
- C. L. Faiola, I. Pullinen, A. Buchholz, F. Khalaj, A. Ylisirniö, E. Kari, P. Miettinen, J. K. Holopainen, M. Kivimäenpää, S. Schobesberger, T. Yli-Juuti and A. Virtanen, ACS Earth Space Chem., 2019, 3, 1756–1772 CrossRef CAS PubMed.
- J. Holopainen, Trends Plant Sci., 2004, 9, 529–533 CrossRef CAS PubMed.
- M. Kivimäenpää, A. B. Babalola, J. Joutsensaari and J. K. Holopainen, Forests, 2020, 11, 573 CrossRef.
- J. Engelberth, H. T. Alborn, E. A. Schmelz and J. H. Tumlinson, Proc. Natl. Acad. Sci. U. S. A., 2004, 101, 1781–1785 CrossRef CAS PubMed.
- C. Wu, I. Pullinen, S. Andres, A. Kiendler-Scharr, E. Kleist, A. Wahner, J. Wildt and T. F. Mentel, 13C labelling study of constitutive and stress-induced terpenoidemissions from Norway spruce and Scots pine, Biogeochemistry, 2017, 1–29 Search PubMed.
- F. Khalaj, A. Rivas-Ubach, C. R. Anderton, S. China, K. Mooney and C. L. Faiola, ACS Earth Space Chem., 2021, 5, 1242–1253 CrossRef CAS.
- A. Ylisirniö, A. Buchholz, C. Mohr, Z. Li, L. Barreira, A. Lambe, C. Faiola, E. Kari, T. Yli-Juuti, S. A. Nizkorodov, D. R. Worsnop, A. Virtanen and S. Schobesberger, Atmos. Chem. Phys., 2020, 20, 5629–5644 CrossRef.
- N. R. Smith, G. V. Crescenzo, Y. Huang, A. P. S. Hettiyadura, K. Siemens, Y. Li, C. L. Faiola, A. Laskin, M. Shiraiwa, A. K. Bertram and S. A. Nizkorodov, Environ. Sci.: Atmos., 2021, 1, 140–153 CAS.
- N. L. Ng, J. H. Kroll, M. D. Keywood, R. Bahreini, V. Varutbangkul, R. C. Flagan, J. H. Seinfeld, A. Lee and A. H. Goldstein, Environ. Sci. Technol., 2006, 40, 2283–2297 CrossRef CAS PubMed.
- O. Böge, A. Mutzel, Y. Iinuma, P. Yli-Pirilä, A. Kahnt, J. Joutsensaari and H. Herrmann, Atmos. Environ., 2013, 79, 553–560 CrossRef.
- P. Deng, L. Wang and L. Wang, J. Phys. Chem. A, 2018, 122, 3013–3020 CrossRef CAS PubMed.
- Z. Tan, L. Hantschke, M. Kaminski, I.-H. Acir, B. Bohn, C. Cho, H.-P. Dorn, X. Li, A. Novelli, S. Nehr, F. Rohrer, R. Tillmann, R. Wegener, A. Hofzumahaus, A. Kiendler-Scharr, A. Wahner and H. Fuchs, Atmos. Chem. Phys., 2021, 21, 16067–16091 CrossRef CAS.
- E. Ahlberg, J. Falk, A. Eriksson, T. Holst, W. H. Brune, A. Kristensson, P. Roldin and B. Svenningsson, Atmos. Environ., 2017, 161, 210–220 CrossRef CAS.
- H. Hakola, V. Tarvainen, A. P. Praplan, K. Jaars, M. Hemmilä, M. Kulmala, J. Bäck and H. Hellén, Atmos. Chem. Phys., 2017, 17, 3357–3370 CrossRef CAS.
- D. Kim, P. S. Stevens and R. A. Hites, J. Phys. Chem. A, 2011, 115, 500–506 CrossRef PubMed.
-
U. S. EPA, Estimation Programs Interface Suite™ for Microsoft® Windows, V 4.11 or Insert Version Used. United States Environmental Protection Agency, Washington, DC, USA. 2023 Search PubMed.
- F. Bernard, V. Daële, A. Mellouki and H. Sidebottom, J. Phys. Chem. A, 2012, 116, 6113–6126 CrossRef CAS PubMed.
- R. Atkinson and J. Arey, Atmos. Environ., 2003, 37, 197–219 CrossRef.
- Z. Peng and J. L. Jimenez, Chem. Soc. Rev., 2020, 49, 2570–2616 RSC.
- A. T. Lambe, P. S. Chhabra, T. B. Onasch, W. H. Brune, J. F. Hunter, J. H. Kroll, M. J. Cummings, J. F. Brogan, Y. Parmar, D. R. Worsnop, C. E. Kolb and P. Davidovits, Atmos. Chem. Phys., 2015, 15, 3063–3075 CrossRef CAS.
- A. T. Lambe, T. B. Onasch, P. Massoli, D. R. Croasdale, J. P. Wright, A. T. Ahern, L. R. Williams, D. R. Worsnop, W. H. Brune and P. Davidovits, Atmos. Chem. Phys., 2011, 11, 8913–8928 CrossRef CAS.
- Z. Peng, J. Lee-Taylor, J. J. Orlando, G. S. Tyndall and J. L. Jimenez, Atmos. Chem. Phys., 2019, 19, 813–834 CrossRef CAS.
- M. Schervish and N. M. Donahue, Environ. Sci.: Atmos., 2021, 1, 79–92 CAS.
- J. Mao, X. Ren, W. H. Brune, J. R. Olson, J. H. Crawford, A. Fried, L. G. Huey, R. C. Cohen, B. Heikes, H. B. Singh, D. R. Blake, G. W. Sachse, G. S. Diskin, S. R. Hall and R. E. Shetter, Atmos. Chem. Phys., 2009, 11 Search PubMed.
- Q. G. J. Malloy, S. Nakao, L. Qi, R. Austin, C. Stothers, H. Hagino and D. R. Cocker, Aerosol Sci. Technol., 2009, 43, 673–678 CrossRef CAS.
- S. Nakao, P. Tang, X. Tang, C. H. Clark, L. Qi, E. Seo, A. Asa-Awuku and D. Cocker, Atmos. Environ., 2013, 68, 273–277 CrossRef CAS.
- C. L. Faiola, A. Buchholz, E. Kari, P. Yli-Pirilä, J. K. Holopainen, M. Kivimäenpää, P. Miettinen, D. R. Worsnop, K. E. J. Lehtinen, A. B. Guenther and A. Virtanen, Sci. Rep., 2018, 8, 3053 CrossRef CAS PubMed.
- N. M. Donahue, A. L. Robinson, C. O. Stanier and S. N. Pandis, Environ. Sci. Technol., 2006, 40, 2635–2643 CrossRef CAS PubMed.
- J. R. Odum, T. Hoffmann, F. Bowman, D. Collins, R. C. Flagan and J. H. Seinfeld, Environ. Sci. Technol., 1996, 30, 2580–2585 CrossRef CAS.
- J. F. Pankow, Atmos. Environ., 1994, 28(2), 185–188 CrossRef CAS.
- J. Zhou, E. A. Bruns, P. Zotter, G. Stefenelli, A. S. H. Prévôt, U. Baltensperger, I. El-Haddad and J. Dommen, Atmos. Meas. Tech., 2018, 11, 65–80 CrossRef CAS.
- T. B. Nguyen, A. P. Bateman, D. L. Bones, S. A. Nizkorodov, J. Laskin and A. Laskin, Atmos. Environ., 2010, 44, 1032–1042 CrossRef CAS.
- D. E. Romonosky, A. Laskin, J. Laskin and S. A. Nizkorodov, J. Phys. Chem. A, 2015, 119, 2594–2606 CrossRef CAS PubMed.
- S. A. Nizkorodov, J. Laskin and A. Laskin, Phys. Chem. Chem. Phys., 2011, 13, 3612 RSC.
- L. T. Fleming, P. Lin, A. Laskin, J. Laskin, R. Weltman, R. D. Edwards, N. K. Arora, A. Yadav, S. Meinardi, D. R. Blake, A. Pillarisetti, K. R. Smith and S. A. Nizkorodov, Atmos. Chem. Phys., 2018, 18, 2461–2480 CrossRef CAS.
- Y. Li, U. Pöschl and M. Shiraiwa, Atmos. Chem. Phys., 2016, 16, 3327–3344 CrossRef CAS.
- W.-S. W. DeRieux, Y. Li, P. Lin, J. Laskin, A. Laskin, A. K. Bertram, S. A. Nizkorodov and M. Shiraiwa, Atmos. Chem. Phys., 2018, 18, 6331–6351 CrossRef.
- B. Aumont, S. Szopa and S. Madronich, Atmos. Chem. Phys., 2005, 21 Search PubMed.
- B. Aumont, R. Valorso, C. Mouchel-Vallon, M. Camredon, J. Lee-Taylor and S. Madronich, Atmos. Chem. Phys., 2012, 12, 7577–7589 CrossRef CAS.
- M. Camredon, B. Aumont, J. Lee-Taylor and S. Madronich, Atmos. Chem. Phys., 2007, 12 Search PubMed.
- I. K. Afreh, B. Aumont, M. Camredon and K. C. Barsanti, Atmos. Chem. Phys., 2021, 21, 11467–11487 CrossRef CAS.
- Q. Li, J. Jiang, I. K. Afreh, K. C. Barsanti and D. R. Cocker III, Atmos. Chem. Phys., 2022, 22, 3131–3147 CrossRef CAS.
- Y. Qin, V. Perraud, B. J. Finlayson-Pitts and L. M. Wingen, Environ. Sci. Technol., 2023, 57, 14260–14268 CrossRef CAS PubMed.
- D. E. Romonosky, N. N. Ali, M. N. Saiduddin, M. Wu, H. J. Julie Lee, P. K. Aiona and S. A. Nizkorodov, Atmos. Environ., 2016, 130, 172–179 CrossRef CAS.
- B. B. Palm, P. Campuzano-Jost, A. M. Ortega, D. A. Day, L. Kaser, W. Jud, T. Karl, A. Hansel, J. F. Hunter, E. S. Cross, J. H. Kroll, Z. Peng, W. H. Brune and J. L. Jimenez, Atmos. Chem. Phys., 2016, 16, 2943–2970 CrossRef CAS.
- D. E. Romonosky, Y. Li, M. Shiraiwa, A. Laskin, J. Laskin and S. A. Nizkorodov, J. Phys. Chem. A, 2017, 121, 1298–1309 CrossRef CAS PubMed.
- G. S. Tyndall, R. A. Cox, C. Granier, R. Lesclaux, G. K. Moortgat, M. J. Pilling, A. R. Ravishankara and T. J. Wallington, J. Geophys. Res., 2001, 106, 12157–12182 CrossRef CAS.
- I. Kourtchev, J.-F. Doussin, C. Giorio, B. Mahon, E. M. Wilson, N. Maurin, E. Pangui, D. S. Venables, J. C. Wenger and M. Kalberer, Atmos. Chem. Phys., 2015, 15, 5683–5695 CrossRef CAS.
- K. Kristensen, T. Cui, H. Zhang, A. Gold, M. Glasius and J. D. Surratt, Atmos. Chem. Phys., 2014, 14, 4201–4218 CrossRef.
- L. Jia and Y. Xu, Sci. Total Environ., 2020, 738, 139831 CrossRef CAS PubMed.
- F. Bianchi, T. Kurtén, M. Riva, C. Mohr, M. P. Rissanen, P. Roldin, T. Berndt, J. D. Crounse, P. O. Wennberg, T. F. Mentel, J. Wildt, H. Junninen, T. Jokinen, M. Kulmala, D. R. Worsnop, J. A. Thornton, N. Donahue, H. G. Kjaergaard and M. Ehn, Chem. Rev., 2019, 119, 3472–3509 CrossRef CAS PubMed.
- F. Jorand, A. Heiss, K. Sahetchian, L. Kerhoas and J. Einhorn, Faraday Trans., 1996, 92, 4167 RSC.
- J. D. Crounse, L. B. Nielsen, S. Jørgensen, H. G. Kjaergaard and P. O. Wennberg, J. Phys. Chem. Lett., 2013, 4, 3513–3520 CrossRef CAS.
- M.-C. Reinnig, L. Müller, J. Warnke and T. Hoffmann, Anal. Bioanal. Chem., 2008, 391, 171–182 CrossRef CAS PubMed.
- B. Witkowski and T. Gierczak, J. Mass Spectrom., 2013, 48, 79–88 CrossRef CAS PubMed.
- Y. B. Lim and B. J. Turpin, Atmos. Chem. Phys., 2015, 15, 12867–12877 CrossRef CAS.
- F. Khan, K. Kwapiszewska, Y. Zhang, Y. Chen, A. T. Lambe, A. Kołodziejczyk, N. Jalal, K. Rudzinski, A. Martínez-Romero, R. C. Fry, J. D. Surratt and R. Szmigielski, Chem. Res. Toxicol., 2021, 34, 817–832 Search PubMed.
- H. K. Maben and P. J. Ziemann, Environ. Sci.: Processes Impacts, 2023, 25(2), 214–228 RSC.
- W. A. Hall and M. V. Johnston, J. Am. Soc. Mass Spectrom., 2012, 23, 1097–1108 CrossRef CAS PubMed.
- C. M. Kenseth, Y. Huang, R. Zhao, N. F. Dalleska, J. C. Hethcox, B. M. Stoltz and J. H. Seinfeld, Proc. Natl. Acad. Sci. U. S. A., 2018, 115, 8301–8306 CrossRef CAS PubMed.
- C. M. Kenseth, N. J. Hafeman, S. P. Rezgui, J. Chen, Y. Huang, N. F. Dalleska, H. G. Kjaergaard, B. M. Stoltz, J. H. Seinfeld and P. O. Wennberg, Science, 2023, 382, 787–792 CrossRef CAS PubMed.
- Y. Li, D. A. Day, H. Stark, J. L. Jimenez and M. Shiraiwa, Atmos. Chem. Phys., 2020, 20, 8103–8122 CrossRef CAS.
- M. Ehn, T. Berndt, J. Wildt and T. Mentel, Int. J. Chem. Kinet., 2017, 49, 821–831 CrossRef CAS.
- A. Pajunoja, W. Hu, Y. J. Leong, N. F. Taylor, P. Miettinen, B. B. Palm, S. Mikkonen, D. R. Collins, J. L. Jimenez and A. Virtanen, Atmos. Chem. Phys., 2016, 16, 11163–11176 CrossRef CAS.
- J. D. Surratt, M. Lewandowski, J. H. Offenberg, M. Jaoui, T. E. Kleindienst, E. O. Edney and J. H. Seinfeld, Environ. Sci. Technol., 2007, 41, 5363–5369 CrossRef CAS PubMed.
|
This journal is © The Royal Society of Chemistry 2024 |