DOI:
10.1039/D2GC04418H
(Paper)
Green Chem., 2023,
25, 2640-2652
Influence of stabilisers on the catalytic activity of supported Au colloidal nanoparticles for the liquid phase oxidation of glucose to glucaric acid: understanding the catalyst performance from NMR relaxation and computational studies†
Received
22nd November 2022
, Accepted 17th March 2023
First published on 22nd March 2023
Abstract
Supported Au colloidal nanoparticles have been prepared in the presence of stabilising polymers, such as, PVA, PVP and PEG (polyvinylalcohol, polyvinylpyrrolidone, polyethylene glycol). The effect of the polymer to Au weight ratio was investigated, for the synthesis of Au nanoparticles with varying particle size and particle size distribution. By varying the polymer/Au wt/wt ratio, Au nanoparticles with mean diameters from 3 to 8 nm were synthesised. The synthesised Au catalysts were studied in the liquid phase oxidation of glucose to glucaric acid under alkaline conditions. We demonstrated that the choice of polymer and polymer to Au weight ratio, have an important influence in terms of catalytic activity and yield to glucaric acid. The highest yield to glucaric acid (22%) was obtained using Au–PVA catalysts. A strong deactivation was observed using Au catalysts. Further evaluation of the possible reasons for deactivation were investigated using experimental, computational and NMR relaxation studies.
1. Introduction
Glucaric acid (GA) is a high-value building block chemical that can be synthesised from biomass-derived molecules and has several important industrial applications.1,2 For example, GA can be commonly found in food ingredients, detergents, corrosion inhibitors, de-icing chemicals and biodegradable cleaners.3–6 Finally, there is a significant opportunity also in the production of new nylons and new types of hyperbranched polyesters.7
Sohst and Tollens reported one of the first chemical processes for D-GA production, based on the nitric acid oxidation of D-glucose (D-GLU) to D-GA.8 The first commercial plant for D-GA production used technology developed by Rivertop that consists of a one-pot oxidation process without NOx release.9 Current methods for GA production are based on this process and involve the chemical oxidation of D-GLU, frequently with nitric acid. Despite its commercial potential, large scale production of D-GA by nitric acid oxidation of D-GLU was hindered, primarily due to competing side reactions resulting in low conversion to D-GA (<50% yield). Furthermore, the highly exothermic character of the oxidation and the hazardous reagents used represent further issues with the large scale production of D-GA by this method. Therefore, research has become directed towards the development of more sustainable methods for the synthesis of D-GA.
TEMPO (2,2,6,6-tetramethyl-1-piperidinyloxy) and its 4-acetamido derivatives are some of the first alternative and commercially available nitroxide catalysts. Thus, GA, isolated as its monopotassium or disodium salt, can be synthesised from glucose in yields approaching 90%. Nonetheless, the drawbacks of this reaction are several, such as (i) further purification of salts obtained to GA, (ii) large quantities of waste generation and (iii) the cost of oxidants, making this process less sustainable and environmentally benign.10
1.1 Enzymatic processes
Enzymatic processes have also been used to selectively convert glucose (GLU) to GA. Su et al. optimized the process using modified E. coli, reducing the number of reaction steps and obtaining 5 g L−1 GA.11 However, these two approaches have two main drawbacks, namely: (i) difficult and expensive separation and purification steps are required, and (ii) long reaction times (3 days for a GA yield of 17%). Therefore, most current research on this process has focused on the development of heterogeneous catalysts, particularly, the utilisation of supported metal nanoparticles.
1.2 Investigation of supported monometallic and bimetallic nanoparticles
Supported monometallic and bimetallic nanoparticles (Au, Pt, Pd) have been widely investigated in the oxidation of alcohols and aldehydes over the last 30 years.12,13 Bimetallic catalysts have often been shown to surpass their monometallic counterparts in terms of activity, stability, selectivity and enhanced performance due to ensemble (structural) and electronic (ligand) effects.13 Additionally, monometallic catalysts have some drawbacks for this process, i.e. deactivation, leaching, and the use of a base (NaOH) for their activation, especially in the case of using monometallic Au nanoparticles.12,13 Delidovich et al. reported the synthesis of monometallic Pt and Pd nanoparticles supported on mesoporous graphite-like carbon. It was found that in the case of GLU oxidation with molecular oxygen at mild alkaline conditions, Pd/C catalysts with a mean particle size of 3.2 nm provided a substantially higher selectivity to gluconic acid (GO) than Pt/C regardless of the smaller mean particle size of Pt nanoparticles (∼1.1 nm). It was suggested that a higher tolerance of larger palladium particles towards the formation of strong surface Pd–O bonds leads to deactivation of the catalyst.14 Clearly, particle size control is key for the catalytic performance. Therefore, it is important to utilise preparation methods for the synthesis of nanoparticles with controlled particle size and in the metallic state. Liang et al. investigated a new preparation method, producing Pd NPs by atomic layer deposition (ALD) over alumina as the chosen support. The ALD method permits to avoid the large amounts of solvent required for the wet-chemical process. When the synthesised catalysts were tested in the GLU oxidation, 2.1% Pd/Al2O3 with mean Pd particle size of 3 nm showed a fast initial rate. After 360 min, it reached 66% conversion whilst 0.9% Pd/Al2O3 with a bimodal particle size distribution centered at 3 nm and 4.5 nm reached 62% conversion with good stability and no leaching phenomena.15 Hermans et al. worked with two different methods of synthesis (precipitation–reduction, denoted as PR, and urea-assisted sodium formate homogeneous deposition, denoted as HD) to prepare Pd nanoparticles supported on carbon black. They found that the optimal mean particle size of Pd for the reaction was 7 nm obtained by PR, giving the highest yield of 50% to GO amongst all the prepared catalysts. The authors concluded that the catalysts were deactivated by a gradual formation of oxygen layers on the Pd particles.16 To avoid the overoxidation problem, which is frequently reported with the use of Pd and Pt catalysts, the use of Au offers a potential solution.17,18 Zhang and Li synthesised Au nanoclusters stabilised with PET (phenylethanethiolate) and immobilised on activated carbon via a simple impregnation method.19 Au25(PET)18, Au38(PET)24, and Au144(PET)60 nanoclusters were synthesised with mean Au particle sizes of 1.3, 1.5, and 1.9 nm, respectively. The nanoclusters were then subjected to an annealing process (120–300 °C) to remove the thiolate ligand. Au25(PET)18 treated at 120 °C maintained the mean Au particle size. Au25(PET)18 treated at 150 and 300 °C increased their mean Au particle size to 3–7 and 8–15 nm, respectively. This was attributed mainly to the weak interaction between the Au nanoclusters and activated carbon supports. The nanoclusters treated at 300 °C gave the worst catalytic performance with only 37% GLU conversion. In contrast, the catalyst treated at 120 °C reached 94% GLU conversion. Finally, the authors investigated the size-dependence of nanoclusters. It was reported that GLU conversion increased with increasing core size and surface area of the Au nanoclusters (Au25(PET)18/AC < Au38(PET)24/AC < Au144(PET)60/AC).20 Wojcieszak et al. evaluated Au nanoparticles (4 nm) supported on CeO2 prepared using a microemulsion method with hydrous hydrazine as reductant in the production of glucuronic acid (an intermediate of GO oxidation to GA). The catalysts synthesised by the microemulsion method gave an 11% yield to glucuronic acid compared to 1% obtained with the catalyst prepared by sol immobilization Au(PVA)/CeO2. It was found that the preparation method has a key role in the catalyst activity, in particular, hydrazine treatment significantly increased the basicity of the catalyst.21 The pH of the reaction solution also plays an important role in the stability of the catalyst. Megias-Sayago et al. studied the effect of recycling Au-based catalysts in base-free conditions. Au nanoparticles, with a mean particle size between 4 and 9 nm were synthesized. All catalysts studied gave similar results and GLU conversion reached around 80% with a selectivity to GO of ∼100% at 40 °C. However, the catalytic activity decreased gradually after the 1st cycle. The initial catalytic performance was regained partially when the catalysts were treated with hot static air, water, or NaOH. It was reported that the important factor leading to the loss of activity is the leaching of Au. This was attributed to the chelating effect of the main major product, GO, as Au is sensitive to the strong absorption of aldehydes, ketones, enones, or compounds with β-dicarbonyl structure found in highly oxygenated species.22 The presence of base is critical for long-term usage of the catalysts used. Zhang et al. studied the effect of several bases using Pd nanoparticles supported on cellulose, a material known to be highly resistant, widely available, and biodegradable. Supported Pd metallic nanoparticles, obtained by the impregnation method, showed a mean particle size of 5 nm. This catalyst was tested in the liquid phase oxidation of GLU to GO using different bases (pyridine, NaOH, Na2CO3, NaHCO3, and Mg(OH)2), at room temperature. Na2CO3 and NaHCO3 resulted in high selectivity (∼90%) to GO with good reusability, while NaOH gave lower selectivity to GO (63%). The use of pyridine gave no GLU conversion. NaOH is a strong base that leads to the isomerisation or degradation of the GLU, in contrast to Na2CO3 and NaHCO3 which are milder bases.23
1.3 Mechanistic studies
From a mechanistic point of view the role of H2O2 and alkaline conditions was investigated. Rossi et al. investigated the reaction mechanism in the presence of Au nanoparticles and reported the formation of gluconate and hydrogen peroxide through a two-electron mechanism. The key point is represented by the electron-rich Au species, formed by the hydrated GLU anion with Au surface atoms, which is supposed to activate molecular oxygen by nucleophilic attack, assuming an efficient nucleophilic behaviour determined by the electronic properties of nanometric Au particles (<10 nm).24 Saliger et al. recently proposed the use of H2O2 as an oxidising agent in GLU oxidation under alkaline conditions. They found that oxygen and hydrogen peroxide comprise the same rate-determining step leading to similar activation energies (48 kJ mol−1 for H2O2 and 47 kJ mol−1 for O2 at 30–60 °C range). It was assumed that O2 formed by the decomposition of H2O2 is the effective oxidising agent.25 Alkaline conditions result in an increase of heterogeneous catalyst activity due to (i) the activation of GLU on the surface of Au nanoparticles and (ii) improved stabilisation of the Au nanoparticles, therefore preventing metal leaching in the reaction medium. Acidic conditions increase the selectivity to GO but significantly suppress the oxidation reaction.26 Efforts were made to avoid the use of basic conditions because of the promoting effect of hydroxyl groups in the isomerisation of GLU to fructose and mannose. Despite this effect, it was proven that –OH groups on the surface of Au sites are required for a high yield of GO.27 For the first step of the reaction there are several studies regarding the use of monometallic nanoparticles, however, for the synthesis of GA few works are published, as oxidation of GLU to GA is a more demanding process, especially using only monometallic nanoparticles. Lee et al. have tested several commercial catalysts, particularly Pt on different supports, such as SiO2, Al2O3, and carbon. Pt/C gave the best results and good stability after 5 cycles, with a TOF of 879 h−1 compared to195 h−1, and 102 h−1 for Pt/Al2O3, and Pt/SiO2 respectively. The study of the different experimental conditions underlines that the reactions under base-free and mild basic conditions give the best yield of GA as opposed to acidic and highly basic conditions as C–C bond cleavage of GA at basic pH results in low carbon chain carboxylic acids.28 Solmi et al. studied this reaction and the experimental reaction conditions (O2 pressure, base concentration, the quantity of catalyst and alloying Au with Bi in NPs) and concluded the need for a basic environment under milder conditions, lower reaction times and the possibility to carry out the reaction at higher metal molar ratios compared to other studies regarding Pt-based catalysts.29
In this paper, the objective of the presented work, is to investigate the influence of stabilisers,30–36 that are used for (i) the synthesis of preformed, supported, colloidal Au nanoparticles to control morphological properties and (ii) to study the catalytic performance of the synthesised catalysts for the selective oxidation of GLU to GA at mild reaction conditions as we have reported previously.29 The influence and impact of the stabilisers has been shown for a range of important catalytic applications, for example in coupling reactions,37,38 in liquid phase oxidation of alcohols and aldehydes,39–47 in the oxidative esterification of furfural,48 in gas phase oxidation reactions,49,50 in hydrogenation reactions,51–55 and in the selective oxidation of methane to methanol.56,57 We selected 3 specific stabilisers; PVA, PVP and PEG with differing structural properties. Therefore, each stabiliser can have a significant impact in terms of the synthesis of supported nanoparticles and subsequently, the catalytic activity.
2. Experimental
2.1 Materials
Tetra chloroauric(III) acid (Sigma Aldrich, 99.99%), poly(vinyl alcohol) (PVA, Sigma Aldrich, MW 13
000–23
000 g mol−1, hydrolysed 87–89%), polyvinylpyrrolidone (PVP, Sigma Aldrich, MW 29
000 g mol−1), polyethylenglycol (PEG, Sigma Aldrich, MW 8000 g mol−1), sodium borohydride (Sigma Aldrich, powder, ≥98.0%) and activated carbon SX1G (AC, Norit) were used for catalyst preparation. D-GLU (Alfa Aesar, >99%) and sodium hydroxide pellets (Sigma Aldrich) were used as reagents for catalytic tests. Gluconic acid sodium salt (Sigma Aldrich, 97%), glucaric acid potassium salt monohydrate (Sigma Aldrich, ≥98%), glyceric acid hemicalcium salt monohydrate (Sigma Aldrich, ≥97%), sodium mesoxalate monohydrate (Sigma Aldrich, ≥98%), oxalic acid dihydrate (Sigma Aldrich, ≥98%), tartronic acid (Sigma Aldrich, ≥97%), glycolic acid (Sigma Aldrich, 98%), arabinose (Sigma Aldrich, ≥98%), formic acid (Alfa Aesar, >95%), lactic acid (Alfa Aesar, 85%), and D-GLU were used as reference commercial compounds for HPLC analysis and quantification of reactants and products was carried out using external calibration method.
2.2 Synthesis of the catalysts
Supported Au catalysts were prepared following a sol immobilisation technique,47,48 to produce a catalyst with nominal metal loading of 1 wt% and by varying the polymer (stabiliser) and the polymer to Au weight ratio. For the preparation of 1 g of catalyst, the desired amount of HAuCl4·3H2O (0.021 g) was dissolved in 390 mL of distilled water (53.58 mg L−1 of Au precursor – 1.30 × 10−4 M Au). Then, the desired volume of polymer (PVA, PVP, PEG, aqueous solution of polymer was 0.101 g mL−1) was added as a stabilising agent. After an initial period of three minutes, the desired amount of fresh aqueous solution of NaBH4 (0.0096 g of sodium borohydride dissolved in 2.5 mL of water) was added to the solution under vigorous stirring (NaBH4
:
Au = 5
:
1 molar ratio) to obtain a red colloidal solution. After 30 minutes of stirring, 0.99 g of support (activated carbon) was added to the solution to immobilise the preformed Au colloidal nanoparticles. The pH of the solution was adjusted to 2 by the addition of concentrated sulfuric acid. The suspension was left stirring at room temperature for 1 h. The catalyst was filtered using a Büchner funnel with two filter papers. The slurry was then washed with distilled water until the washing water reached pH 7. The solid was dried overnight in a watch glass at room temperature. Finally, the solid was dried in the oven at 80 °C for 4 h.
2.3 Characterisation of catalysts
A number of the synthesised catalysts (Au–PVA, Au–PVP, Au–PEG) have been characterised previously,48 by means of UV-Vis spectroscopy, XRD, TEM and XPS and the experimental data especially for UV-Vis analysis and XRD with detailed description are presented in the ESI (Tables S1–S3, and Fig. S1–S4†). Transmission Electron Microscopy (TEM) analysis was performed to evaluate the mean Au particle size and the distribution of the Au nanoparticles presence on the surface of the support. It was carried out using a TEM/STEM FEI TECNAI F20 microscope operating at 200 keV. Samples were prepared by suspending in ethanol and treated by ultrasound for 15 min. A drop of the suspension was deposited on “quantifoil-carbon film” supported by a grid of Cu. The preparation was dried at 120 °C and analysed. To determine the nanoparticles average size and size distribution, the diameter of each sample was measured to a minimum of 400 nanoparticles. The XPS spectra were recorded with a Physical Electronic spectrometer (PHI Versa Probe II), using monochromatic Al Kα radiation (15 kV, 1486.6 eV) and a dual beam charge neutralizer for analyzing the core-level signals of the elements of interest. High-resolution spectra were recorded using a concentric hemispherical analyser with a constant pass energy value of 29.35 eV, irradiating an analysis area of 100 μm in diameter. The binding energy was determined with a precision of ±0.1 eV, using as reference the C 1s signal 284.5 eV. The pressure in the analysis chamber was kept below 5–10 Pa. The SmartSoft-VP 2.10.4.1 software was used for the acquisition of analysis data. A Shirley-type background was subtracted from the signals. The spectra that were recorded were analysed with Gauss-Lorentz type curves, to determine with greater precision, the binding energy of the atomic levels of the different elements. Atomic concentration percentages of the characteristic elements were determined considering the corresponding area sensitivity factor for the different measured spectral regions.
2.4 Glucose oxidation
The oxidation reaction experiments were performed in an autoclave batch reactor of 50 mL capacity.29 The experimental procedure of the catalytic test consists of the following steps: a reaction solution is prepared (0.79 g of GLU dissolved in 15 mL of distilled water) to obtain an aqueous solution of 5 wt% GLU (0.29 M). Then, 0.526 g (0.013 mol) of sodium hydroxide was added to obtain a molar ratio Glu
:
NaOH of 1
:
3 and the desired amount of catalyst was added. After adding the reagents and catalyst to the vessel, a magnetic stirrer bar was introduced to maintain the solution under stirring. The vessel was tightly closed, and the autoclave was purged with O2 (three times); then gaseous oxygen (as oxidant agent) was introduced (10 bar). The reactor was placed in contact with a heating mantle that allows it to reach the desired set-point temperature (60 °C) through feedback heating control with the thermocouple. The magnetic stirrer was switched on to guarantee the diffusion and homogeneity of the reagents. Once the temperature of the solution had reached 60 °C, the solution reaction was kept under these experimental conditions. At the end of the reaction time, the reactor mixture was cooled down by placing it on an ice bath for 10 minutes to quench the reaction. The reaction mixture was collected and centrifuged at 4500 rpm for 15 minutes to separate the liquid from the catalyst. The final liquid volume was measured and a sample was taken for subsequent analysis and storage. The catalyst was then left to dry at ambient conditions overnight. To identify and quantify all the chemical compounds present in the solution, high-performance liquid chromatography (HPLC) was used. The reaction mixture quantitative analyses were carried out using an Agilent 1260 Infinity Quaternary HPLC system. Analyses were performed using 0.0025 M sulfuric acid in ultra-pure water as eluent with a flow of 0.5 ml min−1. The injection system consisted of a six-way valve with an injection volume of 20 μl. Two Rezex ROA-H + (8%) 300 × 7.8 mm ion exclusion columns connected in series were used for the separation of products. A diode array detector (DAD) set to 202 nm was used to detect organic acids and a refractive index detector (RID) was used to detect monosaccharides. The column compartment was thermostated at 80 °C while the RID was kept at a constant temperature of 40 °C.
2.5 Computational studies
Small (2–3 nm) Au nanoparticles have been modelled using a (molecular) cluster approach involving the Au55 cluster, with size of ca. 1 nm. As starting models, five Au55 amorphous structures previously obtained by means of density functional tight-binding theory (DFT-B) have been considered.58 All initial structures were then optimised at the density functional theory (DFT) level using the hybrid B3LYP exchange–correlation functional,59–61 as implemented in the Gaussian16 package.62 On top of optimised Au55 structures, the adsorptions of GLU, GO and GA were studied. For the atoms of the adsorbates (H, C and O) the 6-31G** basis set was used,63 while the Stuttgart effective core potential have been used for Au atoms, accounting also for scalar relativistic effects.64 All calculations have been performed in vacuum, and final energies are evaluated using a larger basis set for lighter atoms, i.e. 6-311++G**,63 for H, C and O, and applying the counterpoise correction in order to account for the basis set superposition error.65 Density of electronic states (DOS) have been computed using the program Multifwn.66
2.6 NMR relaxation studies
A reference Au/AC catalyst powder was soaked in GA or GO solutions (0.6 M in water) for 2 days prior to analysis. The catalyst powder was then removed from the respective liquids and gently dried on a pre-soaked filter paper to remove excess liquid from the outer surface whilst avoiding removal of liquid from the internal pore structure. Following drying, the powder was transferred to a 5 mm NMR tube and tested using a T1–T2 NMR pulse sequence (Fig. 1) to determine spin–spin (T1) and spin–lattice (T2) relaxation times. The typical error for all T1–T2 measurements was approximately 3%.
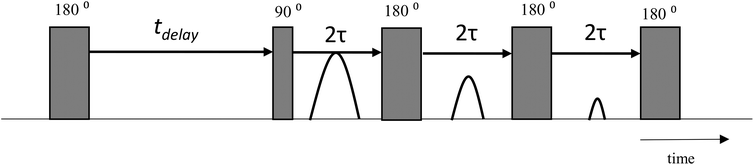 |
| Fig. 1
T
1–T2 NMR pulse sequence. The thin and thick vertical bars represent 90° and 180° radiofrequency (RF) pulses, respectively. T1 relaxation is encoded in the variable time tdelay. T2 relaxation is encoded in the train of n 180° pulses. A single data point is acquired at the centre of each echo time, τ. | |
After NMR analysis, the powder was removed from the NMR tubes and dried at 100 °C for 2 days to remove any water adsorbed to the catalyst surface leaving only the solute species (b.p. > 100 °C) adsorbed to the catalyst surface. After drying, the powder was again analysed using a 2D T1–T2 NMR pulse sequence. Following this, the catalyst powder was soaked in ethylene glycol for 2 days before being analysed again by 2D T1–T2 NMR. A full description of the soaking protocols is given in Fig. 2. The analysed samples are labelled in the form GX-sol, where GX (i.e. GA, GO) represents the sugar solution used for the original soaking and sol represents the secondary solvent used as a guest molecule after the drying protocol (i.e. ethylene glycol).
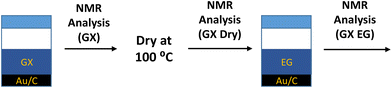 |
| Fig. 2 Soaking protocol used to prepare the Au/AC catalyst samples for the NMR analysis. | |
NMR experiments were performed in a Magritek SpinSolve benchtop NMR spectrometer operating at a 1H frequency of 43 MHz. For the NMR analysis, sixteen recovery delays were used, ranging from 1 ms to between 250–1000 ms. The echo train of the CPMG sequence was composed of between 320–1600 echoes dependent upon the sample acquired in a single shot with an echo spacing of 2τ = 1 ms. Each data set was acquired with 4 scans in approximately 2 min. Inversions were performed using a 2D fast Laplace inversion algorithm written in Matlab, as first implemented by Mitchell et al.67,68 All NMR relaxation measurements were performed at standard room temperature and pressure.
3. Results and discussion
3.1 Characterisation of the catalysts
A series of Au NPs were synthesised using different commercial polymers as stabilising agents (PVA, PVP, PEG), and for each of those, the effect of the amount of polymer was studied by varying the weight ratio polymer/Au from 0.3 to 2.4. The synthesised Au nanoparticles were immobilised on activated carbon, yielding the final catalysts, each labelled as “Au/AC_XXX_YYY”, where “XXX” represents the stabilizing polymer, and “YYY” is the /polymer/Au weight ratio. The synthesised catalysts are presented in Table 1.
Table 1 Synthesised Au catalysts, the polymer used as stabilising agent, the amount of polymer expressed as polymer
:
Au weight ratio and the resulting mean Au particle size
Samples |
Polymer |
Polymer : Au (wt/wt) |
Mean particle size of Au (nm) |
Au/AC_0 |
None |
0 |
7.9 ± 6.3 |
Au/AC_PVA_0.3 |
PVA |
0.3 |
4.3 ± 3.6 |
Au/AC_PVA_0.6 |
0.6 |
2.7± 1.6 |
Au/AC_PVA_1.2 |
1.2 |
2.6± 2.1 |
Au/AC_PVA_2.4 |
2.4 |
2.4± 1.2 |
Au/AC_PEG_0.3 |
PEG |
0.3 |
5.3 ± 2.0 |
Au/AC_PEG_0.6 |
0.6 |
5.6 ± 2.2 |
Au/AC_PEG_1.2 |
1.2 |
5.9 ± 2.3 |
Au/AC_PEG_2.4 |
2.4 |
6.4 ± 2.2 |
Au/AC_PVP_0.3 |
PVP |
0.3 |
5.5± 3.6 |
Au/AC_PVP_0.6 |
0.6 |
5.6 ± 3.9 |
Au/AC_PVP_1.2 |
1.2 |
7.4± 4.7 |
Au/AC_PVP_2.4 |
2.4 |
8.4 ± 4.9 |
The mean Au particle size and standard deviation values of the Au catalysts prepared are reported in Table 1. Fig. S5, S6 and S7† show TEM images and histograms for each catalyst. In the absence of stabiliser, Au/AC_0 displayed a very broad distribution of Au nanoparticles, with the formation of large Au nanoparticles and a mean Au particle size of 7.9 nm. When PVA was used as stabiliser, the PVA-Au-based samples showed a sharp decrease in the mean Au particle size up to a PVA/Au weight ratio of 0.6. A further increase of PVA/Au weight ratio beyond 0.6 did not affect the mean Au particle size. In the case of PEG- and PVP–Au-based nanoparticles a peculiar behaviour is seen. The smallest amount of stabilising agent (polymer/Au weight ratio of 0.3) led to a decrease in mean Au particle size whereas any further increase in the amount of polymer used led to an increase of mean Au particle size from 5 to 6–8 nm. It is evident from these results that by varying the stabiliser to Au weight ratio, the mean Au particle size can be tuned in the range 2–8 nm.
XPS analysis was carried out for the series of samples prepared, mainly focusing on investigating the surface exposure of Au and the Binding Energy (BE) values of Au. The results, reported in Table 2 and Fig. S8–S10,† showed that the values of BE for Au were in the range of 84.0–84.1 eV, suggesting the presence of metallic Au nanoparticles. The values obtained for Au on the surface showed that a general decrease in the amount of surface Au was observed when increasing the amount of each stabilising polymer. Moreover, a higher surface atomic ratio of Au/AC was observed in the case of using PVA as a stabiliser, indicating the higher amount of available Au surface sites for catalytic availability for the proposed reactions. Considering the effect of Au particle size, the highest Au surface exposure was observed with PVA/Au weight ratio of 0.3 and Au mean particle size of 4.3 nm.
Table 2 XPS Analysis for the synthesised catalysts
Samples |
BE Au [eV] |
Au on surface [at%] |
C on surface [at%] |
N on surface [at%] |
Surface atomic ratio Au/AC |
Au/AC_0 |
84.0 |
2.61 |
91.64 |
— |
0.028 |
Au/AC _PVA_0.3 |
84.1 |
3.48 |
87.52 |
— |
0.039 |
Au/AC_PVA_0.6 |
84.1 |
2.80 |
85.80 |
— |
0.033 |
Au/AC_PVA_1.2 |
84.1 |
2.40 |
82.55 |
— |
0.029 |
Au/AC_PVA_2.4 |
84.1 |
1.81 |
82.53 |
— |
0.022 |
Au/AC_PVP_0.3 |
84.0 |
1.43 |
90.94 |
2.1 |
0.016 |
Au/AC_PVP_0.6 |
84.0 |
1.17 |
88.69 |
3.25 |
0.013 |
Au/AC_PVP_1.2 |
84.0 |
0.15 |
90.81 |
2.9 |
0.0016 |
Au/AC_PVP_2.4 |
84.1 |
0.12 |
89.53 |
3.6 |
0.0013 |
Au/AC_PEG_0.3 |
84.1 |
0.84 |
94.35 |
— |
0.009 |
Au/AC_PEG_0.6 |
84.1 |
1.95 |
92.48 |
— |
0.021 |
Au/AC_PEG_1.2 |
84.1 |
1.52 |
93.36 |
— |
0.016 |
Au/AC_PEG_2.4 |
84.1 |
1.09 |
93.64 |
— |
0.012 |
Fig. S11† shows how the polymer to Au weight ratio, (amount of polymer used) can influence the Au availability on the surface. For all the polymers studied, a similar trend was obtained. Increasing the amount of polymer, the availability of Au on the catalyst surface decreased. This could be attributed to the fact that the stabilising agent can partially cover the surface of the metal nanoparticle and in some cases the mean Au particle size was increased as the amount of polymer to Au weight ratio increased.
Since Au particle size and availability of Au on the surface are correlated in Fig. 3, the two parameters were represented together. The comparison between the different samples showed a general trend where when the mean Au particle size decreased in the range of 3–4.5 nm there was an increase in the amount of Au present on the catalyst surface. When the mean Au nanoparticle size was over 5 nm and a stabiliser was used, the amount of Au on the catalyst surface decreased. However, it is clear that the nature of the polymer and the interaction of it on the metal surface affects the availability of the Au active sites. In particular, each polymer shows a peculiar trend. An increase in the amount of PVP used led to an increase of the mean Au particle size, but this is followed by a decrease of the amount of surface Au only up to a weight ratio of 1.2. PEG as a stabilising agent led to higher Au exposure than PVP, and the trend is similar. Higher amounts of polymer increased mean Au particle size and decreased surface Au percentage. PVA was proven to be more effective in reducing mean Au particle size, and higher amounts of PVA further decreased the average diameter of Au nanoparticles. However, simultaneously, it facilitated the further decrease of Au surface exposure, probably due to the higher coverage of the Au nanoparticles by the stabiliser.
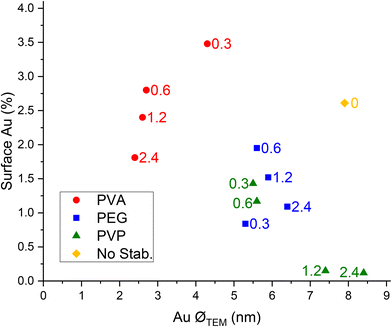 |
| Fig. 3 Correlation between the mean Au nanoparticle (NP) size and atomic percentage of Au on the surface for Au catalysts synthesised using PVA, PVP and PEG. | |
3.2 Catalytic tests
The catalytic performance of the synthesised samples was investigated for the liquid phase oxidation of GLU to GA under alkaline conditions. The reproducibility of the catalyst test protocols described in the Experimental section is shown in Fig. S12 and S13.†
3.2.1 Glucose oxidation.
The transformation of GLU to GO acid and GA under mild reaction conditions in liquid phase has been studied by several research groups.19,20,22–27 Although the transformation of GO has been achieved with high yield and activity using Au supported nanoparticles, the successful consecutive oxidation of GO to GA with yield above 40% is still a challenge using Au supported nanoparticles. The same series of catalysts were tested for the selective oxidation of GLU to GA, to compare the influence of stabiliser in terms of activity and yield to GO and GA and stability.
Fig. 4 shows the catalytic results of GLU oxidation as a function of PVA/Au weight ratio for the catalysts prepared. Increasing the PVA/Au weight ratio in the samples led to a slight increase in the GO amount (yield from 38% to 44%) and a slight decrease in the GA yield (from 22% to 17%), whereas the conversion was similar in all cases at around 90%. The lower GA yield observed can be attributed to the higher amount and presence of PVA (Au surface exposure) on the Au active sites that block the consecutive oxidation of GO to GA.
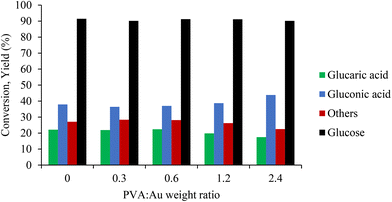 |
| Fig. 4 Catalytic screening of catalysts Au/AC PVA series for the selective oxidation of glucose GO, GA and other byproducts. Reaction conditions: 60 min, 60 °C, 1000 rpm, 10 bar O2, Glu : Au : NaOH molar ratio of 1000 : 1 : 3000. | |
Fig. S14† shows the catalytic data when PVP was used as the chosen stabiliser. As the PVP/Au weight ratio was increased, a small decrease in GA yield (from 20% to 16%) and a higher amount of GO (42% to 51%) was observed. Considering the characterisation data, with increase of PVP amount a lower Au surface exposure and the formation of larger Au nanoparticles was observed, therefore the decrease in yield observed to GA could be attributed to these two factors.
In the case of PEG–Au supported nanoparticles (Fig. S15†), an increase of PEG/Au weight ratio followed a peculiar trend. The GA yield increased from 4% to 20% when the amount of polymer varied from 0.3 to 0.6, but it was further decreased to 3% when the PEG was present in higher amount. The observed catalytic trend could be explained based on the fact that from the characterisation data, the highest Au surface exposure was with the sample that had PEG/Au weight ratio of 0.6 and smaller Au nanoparticles (5–6 nm), whereas with higher PEG/Au weight ratio the Au surface exposure is significant lower, and the presence of larger Au nanoparticles was observed (above 6 nm).
In Fig. 5, the yield of GA has been plotted as a function of Au surface exposure after 1 hour of reaction. The observed trend showed that the increase in the amount of Au on the surface did not significantly affect the GA yield, with a slight increase from 15% to 22%. One possible explanation is that after 1 hour of reaction, the catalyst deactivates because of a possible strong adsorption of the intermediates and products, especially of GA, on the catalyst surface. To support this hypothesis, in Fig. 6, the conversion of GLU and yield of product has been plotted as a function of reaction time. During the first 1 hour there is a progressive decrease of the GO yield and increase in the GA yield. However, after 1 hour of reaction the GA yield is constant, suggesting possible poisoning of the available active sites and, as a consequence, possible deactivation. For this reason, the catalytic tests were repeated with15 minutes of reaction to elucidate better the effect of the stabilisers in terms of catalytic performance at the initial stage of the reaction. After 15 minutes of the reaction, the catalytic trend for the series of the catalysts is clearer. In the case of the PVA–Au catalysts (Fig. S16†), the progressive increase of PVA amount was accompanied with a decrease in the catalytic activity. The conversion slightly decreased (from 88% to 83%), the GO yield increased from 51% to 62% and GA yield decreased from 16% to 8%.
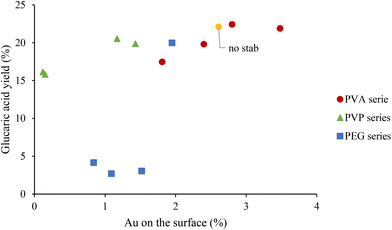 |
| Fig. 5 Correlation between the GA yield and atomic percentage of Au on the surface for PVA series, PVP series and PEG series at reaction time of 1 h. | |
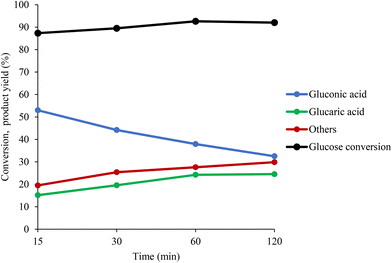 |
| Fig. 6 GLU conversion and formation of GO, GA and other byproducts as a function of reaction time. Reaction conditions: 60 °C, 1000 rpm, 10 bar O2, Glu : Au : NaOH molar ratio of 1000 : 1 : 3000. | |
The PEG–Au catalysts series (Fig. S17†) did not show a significant difference in terms of catalytic performance. The conversion and the GO yield are effectively constant at around 85% and 81%, respectively. The yield of GA was low (1–2%), and was not detected in the reaction mixture at high PEG/Au weight ratio. Finally, for the samples prepared with PVP (Fig. S18†) as stabiliser, the catalytic data showed a different trend in comparison to that obtained after 1 hour of reaction. In particular, Au/AC_PVP_0.3 is the only catalyst that showed a GA yield equal to 10%. It is evident that increasing the amount of PVP promoted a decrease of GA yield, increase of GO yield and a lower formation of byproducts was observed.
Fig. S19† shows the GA yield as a function of the Au atomic percentage on the surface. The amount of Au present on the PEG surface seems to not significantly affect GA production. Instead, the catalysts with the presence of PVA or PVP stabilisers showed the same trend. For example, when the Au surface exposure was higher, the GA yield followed an increase.
3.3 NMR relaxation studies
2D T1–T2 NMR relaxation measurements were used to investigate the deactivation of the Au/AC catalysts (using as a reference Au/AC_PVA_0.6) during the glucose oxidation reaction. NMR relaxation time measurements, particularly the determination of the T1/T2 ratio, have been used extensively in the past to determine the relative solid–liquid interaction strengths between solid pore surfaces and liquid guest molecules, such as reactants, solvents and reaction products, confined within the pore space of the solid material of interest.69–71 In brief, the greater the value of T1/T2, the stronger the interaction between the guest molecules and the surface. When internal field gradients are minimised by using a low magnetic field and low echo time values, the effect of molecular diffusion upon the relaxation time values obtained is removed,72 and eqn (1) has been found to hold true, whereby T1 and T2 represent the longitudinal and transverse relaxation times, respectively, and ρ1 and ρ2 represent the corresponding surface relaxivities.73 |  | (1) |
Previous work has shown that this ratio is related to surface adsorption and can be directly correlated to adsorption energies determined using temperature programmed desorption (TPD).74 As such, the T1/T2 ratio can find great use in the field of catalysis and surface science to quantify the strength of surface interactions taking place and explain phenomena such as solvent effects.75–78
The 2D T1–T2 correlation maps obtained can be seen in Fig. 7. The measured relaxation times and ratios are summarised in Tables S5 and S6.†
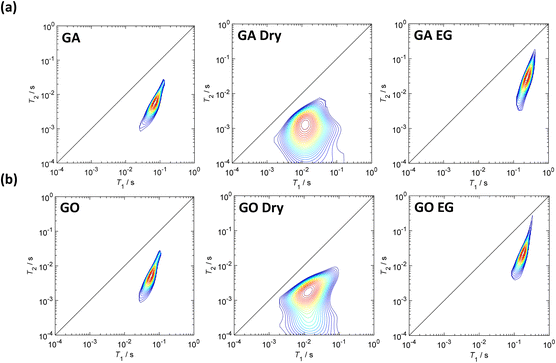 |
| Fig. 7 2D T1–T2 correlation plots obtained by soaking the Au/AC catalyst particles in (a) aqueous glucaric acid (GA) solution (0.6 M) and (b) aqueous gluconic acid (GO) solution (0.6 M). The soaked Au/AC particles were then dried at 100 °C for 24 hours and analysed again (GA Dry/GO Dry). The dried Au/AC particles were then soaked in ethylene glycol (EG) before analysis (GA/GO EG). | |
The relaxation time distributions in Fig. 7 show that the peak of the species present in the contour plots after the drying process is significantly broader than peaks from the other stages of the protocol. The broadening of the peak suggests that the species present may be of a solid-like nature (i.e. solid GA or GO adsorbed to the surface of the Au/AC catalyst). This, in turn, suggests that the drying treatment has removed all the water from the catalyst surface and left solid GA or GO upon the surface which is detected by low field NMR relaxation. The results shown in Tables S5 and S6† are represented graphically in Fig. 8 for ease of comparison between the measured T1/T2 values. Ethylene glycol imbibed within the pores of the fresh (Fig. S20†), non-soaked Au/AC catalyst (represented by the green bar) was used as a model guest molecule to investigate the effect of GA and GO adsorption to the Au/AC catalyst upon the ability of molecules with a relatively large amount of hydroxyl groups, such as a glucose, to adsorb to the Au/AC surface.
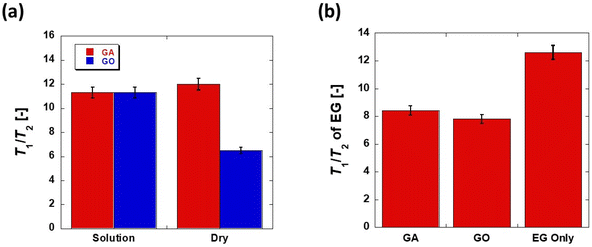 |
| Fig. 8 Measured T1/T2 values determined from the 2D T1–T2 correlation plots for (a) Au/AC catalyst soaked in aqueous acid solution (GA or GO in water, 0.6 M|) and after the drying stage of the protocol, denoted as solution and dry, respectively, and (b) ethylene glycol (EG) adsorbed over Au/AC after treatment with GA or GO aqueous solution and drying. EG only indicates the T1/T2 of ethylene glycol adsorbed over the fresh catalyst as reference. | |
From Fig. 8 it can be seen that T1/T2 values for the catalysts soaked in GA or GO aqueous solution then dried and soaked in EG are generally significantly lower than those for the catalysts soaked in aqueous GA or GO solution only. Indeed, the T1/T2 values for those soaked in GA or GO aqueous solution before drying and soaking in EG are also significantly lower than that obtained for EG only (from Fig. 8b) indicating that the initial adsorption of the glucose and acid solutions significantly impacts the subsequent adsorption of ethylene glycol. From the 2D T1–T2 correlation plots obtained (Fig. 7) it can be clearly seen that there is a species adsorbed to the Au/AC catalyst surface after drying at 100 °C which is significantly different to the adsorbed species seen after soaking in the aqueous acid solutions or ethylene glycol.
Analysis of the relaxation time distributions (Fig. 8) is used to determine the identity of this adsorbed species. The obtained T1/T2 ratio values (Fig. 8, Tables S5 and S6†) are statistically the same for the Au/AC catalyst soaked in both aqueous acid solutions and for the GA EG and GO EG samples. Intriguingly, the T1/T2 ratio values for the dry samples are significantly different, with GA Dry interacting with the catalyst surface much more strongly than GO Dry. This indicates that GA interacts with the catalyst surface much more strongly than GO most likely due to the additional carboxylic acid functionality present, which can hydrogen bond much more strongly to hydroxyl groups on the catalyst surface than the hydroxyl group of GO. When this adsorption data is compared to the reactivity data in Fig. 6, it is clear to see that the further oxidation of GO to GA is inhibited after 60 minutes reaction time as newly formed GA will adsorb strongly to active sites on the Au/AC catalyst surface. It is most likely that the exposed surface Au, blocks access of GO to the catalyst surface thereby inhibiting the consecutive oxidation process.
3.4 Computational results
Considering that experimental evidences indicate that smaller nanoparticles are generally more reactive than larger ones, here we built models of these nanoparticles based on previous global structure optimizations of Au55 clusters performed at the DFT-B level.58 Indeed, photoelectronic spectroscopy combined with DFT calculations,79 showed that anionic Aun clusters (with 53 < n < 65) have a distorted, irregular structure, due to relativistic effects that induce strong surface contractions analogous to bulk surface reconstructions. Au clusters of this size can be treated at DFT level, using hybrid exchange–correlation functionals, allowing for reliable estimates of adsorption energies of small molecules.80 Au clusters with low-symmetry structures are expected to exhibit higher reactivity relative to high-symmetry structures,81 since lower coordination of surface atoms should lead to catalytic reactivity increase. Thus, here we considered the five lowest-energy distorted structures obtained with DFT-B in the work of Van den Bossche,58 re-optimising them at B3LYP/6-31G* level. These optimisations confirmed the almost degeneracy of these five structures that are all within 0.8 kcal mol−1 difference in total energy, see Fig. S21.† The five different structures considered here feature very similar electronic structures, as evident from the computed DOSs (see Fig. S22 in the ESI†). In order to carry out subsequent adsorption studies, we selected the most stable amongst the five (almost degenerate) structures. Moreover, following the idea that the most reactive sites for the catalytic conversion of GLU are expected to be those with low-coordination, we focused our investigations of molecular adsorptions on the Au site with the lowest coordination within the considered cluster structure. Experimental evidence, shown above, suggest that deactivation of the catalyst after one hour of reaction is due to strong adsorption of one of the intermediates or products on the nanoparticle surface, which blocks the reactive sites. Therefore, the adsorption of the reagent GLU, the reaction intermediate GO and the main product GA has been investigated. We obtained adsorption energies of −10.5, −12.0 and −12.6 kcal mol−1 for GLU, GO and GA, respectively, at the B3LYP/6-311++G** level, see Fig. 9. Thus, the GO intermediate and the GA product appear to adsorb slightly more strongly than the reactant, in line with the experimentally observed poisoning of the nano-catalysts during the reaction and in agreement with the NMR relaxation studies. However, the small differences in adsorption energies strongly suggest that surface hydroxyl groups could be, as actually indicated by NMR studies reported above, the major contributors to the high stabilization of molecular species that feature more hydrogen bonding donors and acceptors than GLU.
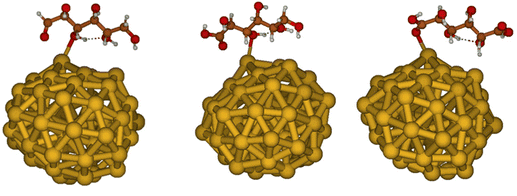 |
| Fig. 9 Adsorption of GLU (left), GO (center) and GA (right) on Au55 model of small Au nanoparticles. | |
As shown in Fig. 9, the shortest O–Au bond is found to be quite similar for all adsorbing molecules, being 2.53, 2.57 and 2.52 Å for GLU, GO and GA, respectively. For all three adsorbates we observed only one intramolecular hydrogen-bond in the optimised geometry in vacuum, suggesting that the intrinsic Au–adsorbate interactions do not provoke specific alterations of intramolecular hydrogen bonds. This outcome further suggests that hydroxyl surface groups could instead establish more hydrogen-bonding interactions with GLU than GO and GA, determining the overall poisoning of the nanoparticle during catalysis.
We then proceeded to study the adsorption energies of GLU, GO and GA on the nanoparticles in the presence of two OH− groups. We chose to proceed with the study with two OH− groups since the substantial difference between the reagent GLU and the reaction products (GO and GA) is in the two terminal groups of the molecules, associated with carboxylic groups that could form hydrogen bonds with hydroxyl surface species (Fig. 10).
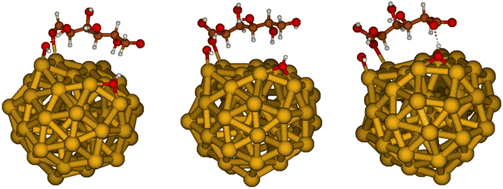 |
| Fig. 10 Adsorption of GLU (left), GO (center) and GA (right) on Au55 model of small Au nanoparticles with OH− groups. | |
In this case, the difference in the adsorption energy among the surface species is substantial: we obtained adsorption energies of −31.2, −40.7 and −44.3 kcal mol−1 for GLU, GO and GA, respectively, at the B3LYP/6-311++G** level. The presence of hydroxyl surface species alters the adsorption mode, having thus a primary effect on the covalent O–Au adsorbate/surface bond formed upon adsorption, which is found to be shorter (2.50 Å) in GO and GA than in GLU (2.54 Å). This explains the stronger adsorption of GO and GA on the nanoparticle surface with respect to GLU. Moreover, in the presence of OH− surface species, GA is bound more strongly than GO by 3.6 kcal mol−1, indicating that it is the most stable among the adsorbed species studied, in agreement with NMR studies. This stabilisation of GA versus GO surface species is due to an extra hydrogen bond between the carbonyl oxygen of the carboxylic group present in GA, and absent in GO, with the OH− adsorbed on the nanoparticle. In summary, we found that hydroxyl surface species could alter the adsorption mode by creating surface hydrogen bonds that make GA the strongest adsorbed species, in agreement with the experimental data.
4. Conclusions
The role of stabiliser (PVA, PEG, PVP) was investigated for the synthesis of preformed supported Au colloidal nanoparticles and the catalytic performance of the synthesised catalysts for the liquid phase oxidation of GLU oxidation to GA was studied. This reaction has been used as a “toolbox” for providing possible correlations between the role and the influence of stabiliser for tuning the Au active sites and controlling the activity, selectivity and stability of the catalysts for the model reaction. By varying the nature of the polymer and the polymer/Au wt/wt ratio, supported Au nanoparticles were synthesised with mean particle size in the range of 3–8 nm. We have shown that the influence of choice of polymer and tuning the polymer to Au weight ratio, have an important influence in terms of catalytic activity and yield to GA. When PVA was the chosen stabiliser, the highest yield to GA (22%) was obtained using Au–PVA catalysts.
Low-field NMR relaxation measurements indicate that GA interacts with the surface of the Au/AC catalyst more strongly than GO, one of the major intermediates. Moreover, computational studies showed that GA shows the strongest adsorption among GO and GLU on the surface of the Au active sites. This is as expected, as the presence of an additional carboxylic acid group in GA will allow for a greater degree of hydrogen bonding with hydroxyl groups on the catalyst surface. These results suggest that the catalyst deactivation over time is due to the build-up of strongly adsorbed GA molecules on the catalyst surface inhibiting adsorption of GO molecules and thereby inhibiting the further oxidation of GO to GA. Future studies for resolving the challenge of minimising deactivation on the supported Au catalysts could be in the direction of (i) synthesis of bimetallic and trimetallic nanoparticles with alloy and core–shell structure (Pd, Pt, Bi, Sn) for altering the electronic and especially the geometric properties of the active sites, (ii) nature of stabiliser by introducing heteroatoms such as P, N and S and varying the degree of hydrolysis for the polymer as well as the molecular weight and finally (iii) the influence and nature of support. Finally, the use of in situ/operando studies (EXAFS/XANES) will be fruitful for discovering the desired active and spectator sites in liquid phase processes.
Data availability
The data that supports the findings of this study is available within the article and the ESI.
Author contributions
Nikolaos Dimitratos, Carmine D'Agostino and Ivan Rivalta supervised the project and designed the experiments, Eleonora Monti and Alessia Ventimiglia carried out the synthesis of materials and catalytic testing; Elena Rodríguez-Aguado carried out the XPS experiments and helped in the interpretation; Ivan Rivalta and Alessia Ventimiglia carried out the computational studies and interpreted the data; Carmine D'Agostino and Luke Forster carried out the NMR relaxation studies and interpreted the data; Francesca Ospitali, Eleonora Monti and Juan Antonio Cecilia carried out the TEM and helped in the interpretation; Nikolaos Dimitratos, Carmine D'Agostino, Ivan Rivalta, Alessia Ventimiglia and Eleonora Monti, Stefania Albonetti, Tommaso Tabanelli, Fabrizio Cavani were involved in the writing and editing of the manuscript.
Conflicts of interest
The authors declare that they have no known competing financial interests or personal relationships that could have appeared to influence the work reported in this paper.
Acknowledgements
L. F. and C. D. would like to acknowledge the EPSRC (EP/V026089/1) for supporting their research activities. I. R. gratefully acknowledges the use of HPC resources of the “Pôle Scientifique de Modélisation Numérique” (PSMN) of the ENS-Lyon, France.
References
- M. Besson, P. Gallezot and C. Pinel, Chem. Rev., 2014, 114, 1827–1870 CrossRef CAS PubMed.
- A. Corma, S. Iborra and A. Velty, Chem. Rev., 2007, 107, 2411–2502 CrossRef CAS PubMed.
- R. D. Armstrong, B. M. Kariuki, D. W. Knight and G. J. Hutchings, Eur. J. Org. Chem., 2017, 6811–6814 CrossRef CAS PubMed.
-
K. Ahuja and S. Singh, Gluconic Acid Market Size Worth Over $80 mn by 2024, https://www.gminsights.com/pressrelease/gluconic-acid-market, (accessed October 2020).
- Glucaric Acid Market Size, Share & Trends Analysis By Product (Pure Glucaric Acid, D-Glucaric Acid-1,4-lactone), By Application (Food Ingredients, Detergents, Corrosion Inhibitors), and Segment Forecasts, 2017–2025, https://www.grandviewresearch.com/industry-analysis/glucaric-acid-market, (accessed September 2021).
- Z. Walaszek, J. Szemraj, M. Hanausek, A. K. Adams and U. Sherman, Nutr. Res., 1996, 16, 673–6815 CrossRef CAS.
- Q. Zhang, Z. Wan, I. K. M. Yu and D. C. W. Tsang, J. Cleaner Prod., 2021, 312, 127745 CrossRef CAS.
- O. Sohst and B. Tollens, Justus Liebigs Ann. Chem., 1888, 245, 1–27 CrossRef.
- N. Merbouh, J. F. Thaburet, M. Ibert, F. Marsais and J. M. Bobbitt, Carbohydr. Res., 2001, 336, 75–78 CrossRef CAS PubMed.
- T. S. Moon, S. H. Yoon, A. M. Lanza, J. D. Roy-Mayhew and K. L. J. Prather, Appl. Environ. Microbiol., 2009, 75, 589–595 CrossRef CAS PubMed.
- H. H. Su, Z. W. Guo, X. L. Wu, N. Li, P. Xu, M. H. Zong and W. Y. Lou, ChemSusChem, 2019, 12, 2278–2285 CrossRef CAS PubMed.
- N. Dimitratos, J. A. Lopez-Sanchez and G. J. Hutchings, Chem. Sci., 2012, 3, 20–44 RSC.
- M. Sankar, N. Dimitratos, P. J. Miedziak, P. P. Wells, C. J. Kiely and G. J. Hutchings, Chem. Soc. Rev., 2012, 41, 8099–8139 RSC.
- I. V. Delidovich, O. P. Taran, L. G. Matvienko, A. N. Simonov, I. L. Simakova, A. N. Bobrovskaya and V. N. Parmon, Catal. Lett., 2010, 140, 14–21 CrossRef CAS.
- X. Liang, L. B. Lyon, Y. B. Jiang and A. W. Weimer, J. Nanopart. Res., 2012, 14, 943 CrossRef.
- T. Haynes, V. Dubois and S. Hermans, Appl. Catal., A, 2017, 542, 47–54 CrossRef CAS.
- T. Mallat and A. Baiker, Chem. Rev., 2004, 104, 3037–3058 CrossRef CAS PubMed.
- S. E. Davis, M. S. Ide and R. J. Davis, Green Chem., 2013, 15, 17–45 RSC.
- J. Zhang, Z. Li, J. Huang, C. Liu, F. Hong, K. Zheng and G. Li, Nanoscale, 2017, 9, 16879–16886 RSC.
- C. Liu, J. Zhang, J. Huang, C. Zhang, F. Hong, Y. Zhou and M. Haruta, ChemSusChem, 2017, 10, 1976–1980 CrossRef CAS PubMed.
- R. Wojcieszak, I. M. Cuccovia, M. A. Silva and L. M. Rossi, J. Mol. Catal. A: Chem., 2016, 422, 35–42 CrossRef CAS.
- C. Megías-Sayago, L. F. Bobadilla, S. Ivanova, A. Penkova, M. A. Centeno and J. A. Odriozola, Catal. Today, 2018, 301, 72–77 CrossRef.
- X. Zhang, H. Shi, Q. Chi, X. Liu and L. Chen, Polym. Bull., 2020, 77, 1003–1014 CrossRef CAS.
- M. Comotti, C. Della Pina, E. Falletta and M. Rossi, Adv. Synth. Catal., 2006, 348, 313–316 CrossRef CAS.
- R. Saliger, N. Decker and U. Prüße, Appl. Catal., B, 2011, 102, 584–589 CrossRef CAS.
- P. Qi, S. Chen, J. Chen, J. Zheng, X. Zheng and Y. Yuan, ACS Catal., 2015, 5, 2659–2670 CrossRef CAS.
- C. Megías-Sayago, J. L. Santos, F. Ammari, M. Chenouf, S. Ivanova, M. A. Centeno and J. A. Odriozola, Catal. Today, 2018, 306, 183–190 CrossRef.
- J. Lee, B. Saha and D. G. Vlachos, Green Chem., 2016, 18, 3815–3822 RSC.
- S. Solmi, C. Morreale, F. Ospitali, S. Agnoli and F. Cavani, ChemCatChem, 2017, 9, 2797–2806 CrossRef CAS.
- C. D. Pina, E. Falletta, L. Prati and M. Rossi, Chem. Soc. Rev., 2008, 37, 2077–2095 RSC.
- K. Y. Lee, Y. W. Lee, J. H. Lee and S. W. Han, Colloids Surf., A, 2010, 372, 146–150 CrossRef CAS.
- C. J. Jia and F. Schüth, Phys. Chem. Chem. Phys., 2011, 13, 2457–2487 RSC.
- A. Villa, D. Wangb, G. M. Veith, F. Vindigni and L. Prati, Catal. Sci. Technol., 2013, 3, 3036–3041 RSC.
- R.-Y. Zhong, K.-Q. Sun, Y.-C. Hong and B.-Q. Xu, ACS Catal., 2014, 4, 3982–3993 CrossRef CAS.
- S. Campisi, M. Schiavoni, C. E. Chan-Thaw and A. Villa, Catalysts, 2016, 6, 185–205 CrossRef.
- L. M. Rossi, J. L. Fiorio, M. A. S. Garcia and C. P. Ferraz, Dalton Trans., 2018, 47, 5889–5915 RSC.
- Y. Li and M. A. El-Sayed, J. Phys. Chem. B, 2001, 105, 8938–8943 CrossRef CAS.
- H. Tsunoyama, H. Sakurai, N. Ichikuni, Y. Negishi and T. Tsukuda, Langmuir, 2004, 20, 11293–11296 CrossRef CAS PubMed.
- F. Porta, L. Prati, M. Rossi and G. Scari, J. Catal., 2002, 211, 464–469 CrossRef CAS.
- N. Dimitratos, A. Villa, C. L. Bianchi, L. Prati and M. Makkee, Appl. Catal., A, 2006, 311, 185–192 CrossRef CAS.
- A. S. K. Hashmi and G. J. Hutchings, Angew. Chem., Int. Ed., 2006, 45, 7896–7936 CrossRef PubMed.
- W. Hou, N. Dehm and R. Scott, J. Catal., 2008, 253, 22–27 CrossRef CAS.
- A. Villa, D. Wang, D. S. Su and L. Prati, ChemCatChem, 2009, 1, 510–514 CrossRef CAS.
- A. Villa, D. Wang, D. Su, G. M. Veith and L. Prati, Phys. Chem. Chem. Phys., 2010, 12, 2183–2189 RSC.
- S. M. Rogers, C. R. A. Catlow, C. E. Chan-Thaw, D. Gianolio, E. K. Gibson, A. L. Gould, N. Jian, A. J. Logsdail, R. E. Palmer, L. Prati, N. Dimitratos, A. Villa and P. P. Wells, ACS Catal., 2015, 5, 4377–4384 CrossRef CAS.
- N. Dimitratos, A. Villa, L. Prati, C. Hammond, C. E. Chan-Thaw, J. Cookson and P. T. Bishop, Appl. Catal., A, 2016, 514, 267–275 CrossRef CAS.
- E. Monti, A. Ventimiglia, C. A. G. Soto, F. Martelli, E. Rodríguez-Aguado, J. A. Cecilia, A. Sadier, F. Ospitali, T. Tabanelli, S. Albonetti, F. Cavani, R. Wojcieszak and N. Dimitratos, Catalysts, 2022, 12, 196 CrossRef CAS.
- E. Monti, A. Ventimiglia, C. A. G. Soto, F. Martelli, E. Rodríguez-Aguado, J. A. Cecilia, P. Maireles-Torres, F. Ospitali, T. Tabanelli, S. Albonetti, F. Cavani and N. Dimitratos, Mol. Catal., 2022, 528, 112438 CrossRef CAS.
- J. A. Lopez-Sanchez, N. Dimitratos, C. Hammond, G. L. Brett, L. Kesavan, S. White, P. Miedziak, R. Tiruvalam, R. L. Jenkins, A. F. Carley, D. Knight, C. J. Kiely and G. J. Hutchings, Nat. Chem., 2011, 3, 551–556 CrossRef CAS PubMed.
- N. Yang, S. Pattisson, M. Douthwaite, G. Zeng, H. Zhang, J. Ma and G. J. Hutchings, ACS Catal., 2021, 11, 11607–11615 CrossRef CAS.
- S. M. Ansar and C. L. Kitchens, ACS Catal., 2016, 6, 5553–5560 CrossRef CAS.
- S. Scurti, E. Monti, E. Rodríguez-Aguado, D. Caretti, J. A. Cecilia and N. Dimitratos, Nanomaterials, 2021, 11, 879–897 CrossRef CAS PubMed.
- S. R. Thomas, W. Yang, D. J. Morgan, T. E. Davies, J. J. Li, R. A. Fischer, J. Huang, N. Dimitratos and A. Casini, Chem. – Eur. J., 2022, 28, e202201575 CAS.
- S. Scurti, A. Allegri, F. Liuzzi, E. Rodríguez-Aguado, J. A. Cecilia, S. Albonetti, D. Caretti and N. Dimitratos, Catalysts, 2022, 12, 323 CrossRef CAS.
- S. Capelli, D. Motta, C. Evangelisti, N. Dimitratos, L. Prati, C. Pirola and A. Villa, Nanomaterials, 2020, 10, 505–522 CrossRef CAS PubMed.
- N. Agarwal, S. J. Freakley, R. U. McVicker, S. M. Althahban, N. Dimitratos, Q. He, D. J. Morgan, R. L. Jenkins, D. J. Willock, S. H. Taylor, C. J. Kiely and G. J. Hutchings, Science, 2017, 358, 223–227 CrossRef CAS PubMed.
- S. J. Freakley, N. Agarwal, R. U. McVicker, S. Althahban, R. J. Lewis, D. J. Morgan, N. Dimitratos, C. J. Kiely and G. J. Hutchings, Catal. Sci. Technol., 2020, 17, 5935–5944 RSC.
- M. Van den Bossche, J. Phys. Chem. A, 2019, 123, 3038–3045 CrossRef CAS PubMed.
-
Essentials of Computational Chemistry: Theories and Models, ed. C. J. Cramer, Wiley, New York, 2nd edn, 2002 Search PubMed.
- P. J. Stephens, F. J. Devlin, C. F. Chabalowski and M. J. Frisch, J. Phys. Chem., 1994, 98, 11623–11627 CrossRef CAS.
- A. D. McLean and G. S. Chandler, J. Chem. Phys., 1980, 72, 5639–5948 CrossRef CAS.
-
M. J. Frisch, G. W. Trucks, H. B. Schlegel, G. E. Scuseria, M. A. Robb, J. R. Cheeseman, G. Scalmani, V. Barone, G. A. Petersson, H. Nakatsuji, X. Li, M. Caricato, A. V. Marenich, J. Bloino, B. G. Janesko, R. Gomperts, B. Mennucci, H. P. Hratchian, J. V. Ortiz, A. F. Izmaylov, J. L. Sonnenberg, D. Williams-Young, F. Ding, F. Lipparini, F. Egidi, J. Goings, B. Peng, A. Petrone, T. Henderson, D. Ranasinghe, V. G. Zakrzewski, J. Gao, N. Rega, G. Zheng, W. Liang, M. Hada, M. Ehara, K. Toyota, R. Fukuda, J. Hasegawa, M. Ishida, T. Nakajima, Y. Honda, O. Kitao, H. Nakai, T. Vreven, K. Throssell, J. A. Montgomery, Jr., J. E. Peralta, F. Ogliaro, M. J. Bearpark, J. J. Heyd, E. N. Brothers, K. N. Kudin, V. N. Staroverov, T. A. Keith, R. Kobayashi, J. Normand, K. Raghavachari, A. P. Rendell, J. C. Burant, S. S. Iyengar, J. Tomasi, M. Cossi, J. M. Millam, M. Klene, C. Adamo, R. Cammi, J. W. Ochterski, R. L. Martin, K. Morokuma, O. Farkas, J. B. Foresman and D. J. Fox, Gaussian 16, Revision C.01, Gaussian, Inc., Wallingford CT, 2016 Search PubMed.
- A. Schäfer, H. Horn and R. Ahlrichs, J. Chem. Phys., 1992, 97, 2571–2577 CrossRef.
- B. P. Pritchard, D. Altarawy, B. Didier, T. D. Gibson and T. L. Windus, Chem. Inf. Model., 2019, 59, 4814–4820 CrossRef CAS PubMed.
- H. B. Jansen and P. Ros, Chem. Phys. Lett., 1969, 3, 140–143 CrossRef CAS.
- T. Lu and F. Chen, J. Comput. Chem., 2012, 33, 580–592 CrossRef CAS PubMed.
- N. Robinson, E. F. May and M. L. Johns, ACS Appl. Mater. Interfaces, 2021, 13, 54476–54485 CrossRef CAS PubMed.
- J. Mitchell, D. A. Graf von der Schulenburg, D. J. Holland, E. J. Fordham, M. L. Johns and L. F. Gladden, J. Magn. Reson., 2008, 193, 218–225 CrossRef CAS PubMed.
- G. Fillipini, F. Longobardo, L. Forster, A. Criado, G. Di Carmine, L. Nasi, C. D'Agostino, M. Melchionna, P. Fornasiero and M. Prato, Sci. Adv., 2020, 6, eabc9923 CrossRef PubMed.
- L. Forster, C. D'Agostino, M. Llosa-Tanco, V. Spallina, C. Brencio, F. Gallucci, M. Lindley, S. J. Haigh and D. A. Pacheco-Tanaka, Chem. Eng. J., 2021, 424, 139313 CrossRef.
- M. R. Barr, L. Forster, C. D'Agostino and R. Volpe, Appl. Surf. Sci., 2021, 571, 151253 CrossRef.
- J. Mitchell, T. C. Chandrasekera, M. L. Johns and L. F. Gladden, Phys. Rev., 2010, 81, 026101 CrossRef CAS.
- N. Robinson, P. Bräuer, A. P. E. York and C. D'Agostino, Phys. Chem. Chem. Phys., 2021, 23, 17752–17760 RSC.
- C. D'Agostino, J. Mitchell, M. D. Mantle and L. F. Gladden, Chem. – Eur. J., 2014, 20, 13009–13015 CrossRef PubMed.
- C. D'Agostino, G. L. Brett, P. J. Miedziak, D. W. Knight, G. J. Hutchings, L. F. Gladden and M. D. Mantle, Chem. – Eur. J., 2012, 18, 14426–14433 CrossRef PubMed.
- C. D'Agostino, M. R. Feaviour, G. L. Brett, J. Mitchell, A. P. E. York, G. J. Hutchings, M. D. Mantle and L. F. Gladden, Catal. Sci. Technol., 2016, 6, 7896–7901 RSC.
- C. D'Agostino, T. Kotionova, J. Mitchell, P. J. Miedziak, D. W. Knight, S. H. Taylor, G. J. Hutchings, L. F. Gladden and M. D. Mantle, Chem. – Eur. J., 2013, 19, 11725–11732 CrossRef PubMed.
- G. Di Carmine, F. Pesciaioli, S. Wang, A. Sinibaldi, G. Giorgianni, C. M. A. Parlett, A. Carlone and C. D'Agostino, ChemCatChem, 2022, 14, e202200405 CrossRef CAS.
- H. Häkkinen, M. Moseler, O. Kostko, N. Morgner, M. A. Hoffmann and B. V. Issendorff, Phys. Rev. Lett., 2004, 93, 093401 CrossRef PubMed.
- G. Mazzone, I. Rivalta, N. Russo and E. Sicilia, Phys. Chem. C, 2008, 112(15), 6073–6608 CrossRef CAS.
- W. Huang, M. Ji, C. D. Dong, X. Gu, L. M. Wang, X. G. Gong and L. S. Wang, ACS Nano, 2008, 2, 897–904 CrossRef CAS PubMed.
|
This journal is © The Royal Society of Chemistry 2023 |