DOI:
10.1039/D3CE00757J
(Highlight)
CrystEngComm, 2023,
25, 6388-6404
Advances in protein solubility and thermodynamics: quantification, instrumentation, and perspectives
Received
28th July 2023
, Accepted 10th October 2023
First published on 11th October 2023
Abstract
While protein crystallization has broadly been applied to determine the 3D structure of proteins, it has recently drawn great attention to replace the traditional downstream processing for protein-based biopharmaceuticals due to its advantages in stability, storage, and delivery. However, establishing the crystallization conditions of a protein remains a challenge because of the limited understanding of the underlying phenomena. This highlight provides a critical review of the advanced experimental approaches to measure thermodynamic parameters (e.g. solubility) that can help in establishing the necessary conditions to perform a protein crystallization trial. Firstly, methods and techniques to assess protein crystallizability and solution quality are presented. Next, methodologies to measure the main thermodynamic parameters are revised (with the respective advantages, limitations, and studied proteins). Later, protocols and set-ups (with a focus on microfluidic devices) used to quantify solubility parameters are highlighted (involved apparatus capabilities, solubility screening details, and studied proteins). Lastly, future directions and outlook of this critical review are approached by covering new trends in the research field.
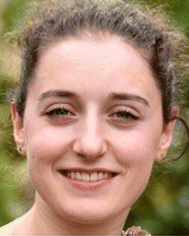 Joana Ferreira | Joana Ferreira is a post-doctoral researcher at the Transport Phenomena Research Centre (CEFT) of the Faculty of Engineering of the University of Porto (FEUP). After a master's in chemical engineering at FEUP, she started a joined PhD thesis at KU Leuven and FEUP. Her PhD work was focused on the study of protein crystallization in droplet-based microfluidics. Her research interests in crystal engineering rely on multidisciplinary perspectives to solve problems through the combination of experimental, numerical, and analytical approaches. Her work was recently highlighted in the virtual issue on Women Researchers at the Forefront of Crystal Engineering of the Crystal Growth & Design journal. |
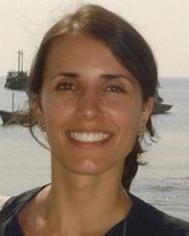 Filipa Castro | Filipa Castro is an assistant researcher at the Centre of Biological Engineering (CEB) of the University of Minho. She received her PhD thesis from the University of Minho in Bioengineering through the MIT-Portugal programme. The work was done in collaboration with the University of Minho, the Faculty of Engineering of the University of Porto (FEUP) and the Massachusetts Institute of Technology (MIT). After two post-doctoral fellowships, she joined as a junior researcher the Supramolecular Assemblies group from the Laboratory for Process Engineering, Environment, Biotechnology and Energy (LEPABE) of FEUP, where she deepened her knowledge on crystallization/precipitation science and multiphase flow reactors. She has been studying both fundamentals and applications of crystallization/precipitation science, in particular protein crystallization with applications to both health issues and downstream processing. |
1. Introduction
Protein crystallization has had an extraordinary impact on the determination of 3D protein structures through X-ray crystallography.1,2 Over 85% of the protein structures deposited in the Protein Data Bank (PDB) are from crystal-based structural methods.3 Although other techniques have emerged (e.g. Cryo-EM, NMR), X-ray crystallography remains the predominant method to obtain information on protein structures.4 The information obtained is used for understanding disease mechanisms, identifying drug targets, and optimizing pharmaceutical drug design.5 Moreover, the pharmaceutical industry has increased efforts for the development of protein crystal-based therapeutics. On the one hand, crystallization can represent a cost-effective alternative to the conventional chromatographic steps in the downstream processing of therapeutic proteins.6 On the other hand, protein crystals offer advantages in terms of formulation and drug delivery.7,8 The outstanding impact of protein crystallization over the last decades can be seen from the high number and exponential increase on the number of publications in this research field as shown in Fig. 1.
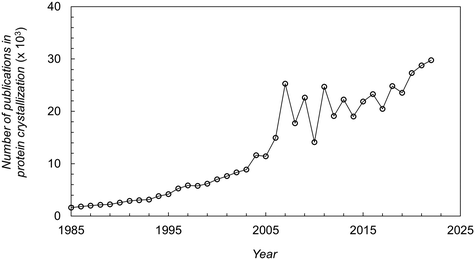 |
| Fig. 1 Number of publications (papers, books, chapters, etc.) in protein crystallization reported in the literature over the last decades.16 | |
Regardless of the ultimate purpose, the first step consists in establishing the protein crystallization conditions.9 This is a challenging task due to the size, complexity and dynamic behaviour of proteins, as well as the intricacy of all the occurring interactions (i.e. protein–protein interactions, protein–solvent interactions, etc.).10 Additionally, the unavailability of generalized strategies to crystallize proteins makes the process of identifying crystallization conditions time consuming, fastidious, and costly. Despite the advances of protein screening methods [i.e. high-throughput crystallization screening (HTS)], the task of finding crystallization conditions for a specific protein still largely relies on empirical results rather than on theorical perspectives.6 A significant number of experiments, where numerous chemical and physical parameters are tested, are mostly conducted. Moreover, it must be done on a recurring basis for each protein under study. According to Newman et al. (2012),11 only 0.2% of individual crystallization screening conditions performed in HTS laboratory yield a crystal.
Considering the limited understanding of the underlying phenomena, limited guidance is available to systematically identify conditions that may lead to protein crystals. Added to this is the lack of consistency in the reported crystallization conditions, and the limited quantitative experimental data available in the literature. For the crystallization process to take place, the protein must be crystallizable, the quality of the protein solution must be high, and the supersaturation must be achieved.12 Therefore, the measurement of parameters related to the protein itself (i.e. amino acid sequence, folding, etc.) and the protein solution (i.e. purity, homogeneity, etc.), as well as the protein thermodynamics (i.e. solubility) system under study is a critical step towards a more rational approach for the establishment of protein crystallization conditions. This rational approach will enable researchers to enhance the crystallization success rate and process controllability, thus drastically reducing screening time and associated costs. In terms of industrial relevance, this will also benefit drug development and crystal-based therapeutics.
Solubility is probably the most important parameter when studying a solid compound since it governs the crystallization process13 and is a prerequisite to any meaningful crystallization measurement.14 Saridakis & Chayen (2003)15 stated that the solubility curve derivation requires at least two conditions (one of which is typically the protein concentration) within a time range around 3–4 weeks. As far as we know, this highlight merges, for the first time, a critical review of the main experimental methodologies and analytical models, as well as applied protocols and instrumentation to determine protein solubility and other thermodynamic parameters that assess protein–protein interactions. This contribution will serve as a guideline for young researchers and academics to identify thermodynamically possible conditions for crystallizing proteins. The covered methods and techniques are focused on soluble proteins.
2. Protein crystallizability
The protein itself and the protein solution are the most important variables when attempting to perform a crystallization trial.12,17 Concerning the ability of a protein to bind to partners, the molecular surface structure (e.g. hydrophobicity, charge, amino acid residues) dictates the molecular interactions.10 According to Derewenda (2010),18 on the one hand, the surface properties must enable the dissolution of the protein to reach supersaturation, and on the other hand, mediate viable intermolecular contacts. Further, the “quality” of the protein solution is critical to increase the chances of yielding crystals. In general, pure, stable, soluble and monodisperse (i.e. uniform population of molecular species) proteins in high concentrations (5–15 mg mL−1) are easier to crystallize.19
2.1. Protein ability to crystallize
The ability of a protein to self-assemble into a crystal is the first requirement for protein crystallization.12,20 This is specific for each protein under a particular set of conditions and is related to its amino acid sequence and structure. Protein–protein interactions promoting crystal contacts are mainly governed by hydrogen bonds, hydrophobic interactions/van der Waals and electrostatic bonds (Fig. 2). Nevertheless, those interactions are complex, particularly due to protein anisotropy (i.e. non-spherical shape, non-uniform charge distribution, rough local topography, and heterogeneous functionality on the protein surface) (Fig. 3), and it is difficult to determine the contribution from each type of interaction.21,22 One approach to predict the intermolecular interactions of a protein that are likely to be crystal contacts is to use information from protein structure. There are three major techniques to determine the structure of a protein: X-Ray crystallography (XRD), nuclear magnetic resonance (NMR), and electron microscopy (EM). However, knowledge of the crystallization conditions is required.
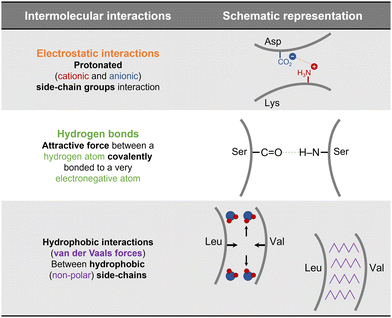 |
| Fig. 2 Schematic representation of the main protein–protein interactions involved in crystal contacts with examples of amino acids residues that can be involved in each of those.17,21,23 | |
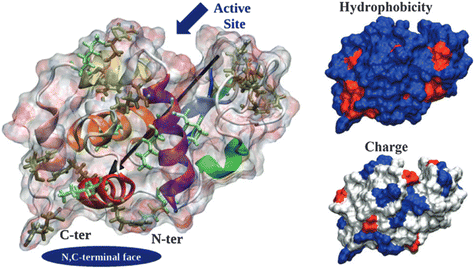 |
| Fig. 3 Hen egg-with lysozyme (HEWL) structure. Protein surface is shown as a ghost surface colored by partial charge with protein secondary structure elements indicated as a cartoon and colored: red – α-helix A, orange – α-helix B, purple – α-helix C, yellow – α-helix D, pink – C-terminal α-helix 310 from domain α, green – middle α-helix 310 from domain β, blue – sheet β1, cyan – sheet β2, gray – sheet β3, and white – other structures including loops, turns and β-bridges. Arg and Lys residues are shown as licorice and colored in tan and light green, respectively. The black arrow indicates the protein dipole moment. Protein termini, N,C-terminal face and active site location are shown by navy objects and annotated. Figures on the right-hand side show the distribution of charged (positive – blue, negative – red, and neutral – white) and hydrophilic (hydrophilic – blue and hydrophobic – red) residues. [Reproduced from ref. 24 with permission from The Royal Society of Chemistry]. | |
Derewenda (2010)18 reported two main criteria that proteins must meet to crystallize: ability to dissolve and ability to enable crystal contacts. The molecular surface of the protein should allow adequate solubility to achieve the requisite supersaturation levels. The residues exposed on the protein surface should promote the compatibility with the environment under study.25 For instance, polar residues on a protein surface promote the protein's solubility in a polar aqueous solvent. As illustrated in Fig. 3, HEWL has a rather hydrophilic surface which contributes to HEWL's solubility in water. Concerning crystal contacts, studies have related protein surface properties to crystallization propensity.26–30 Price et al. (2010)30 analyzed the relationship between the frequency of each amino acid, mean hydrophobicity, mean sidechain entropy, total and net electrostatic charge, isoelectric point (pI), the fraction of disordered residues and chain length, and successful protein crystallization (Fig. 4). The results showed that the frequency of certain amino acids such as Ala and Phe was positively correlated to a successful crystallization outcome, while Lys and Glu negatively correlated.30 Furthermore, it was verified that mean hydrophobicity promoted crystallization, while mean sidechain entropy, fractional positive or negative charge and disordered regions tended to hinder crystallization.30 In terms of protein pI and chain length, both demonstrated bimodal effects, with the rate of success initially increasing and later decreasing with increasing parameter values. Examples of proteins that are easy targets for crystallization are soluble and globular proteins (e.g. lysozyme, insulin). Globular proteins consist of a hydrophobic core surrounded by a hydrophilic external surface which interacts with water (Fig. 3). In the specific case of lysozyme, the polar character of Asp and Glu residues is responsible for hydrogen bonding involved in crystal contacts.31
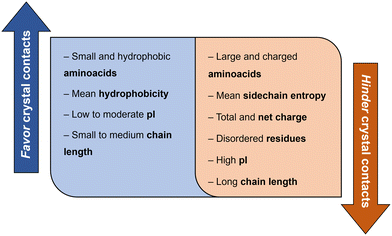 |
| Fig. 4 Correlation between protein surface properties favoring and hindering crystal contacts towards a successful crystallization trial.30 | |
Overall, studies have suggested that crystal contacts correspond to non-randomly selected regions of the protein surface.10 Therefore, it should be possible to control the protein–protein interactions involved in crystal contacts by tuning the solution conditions or by mutating certain surface residues.23
2.2. Protein solution quality
The purity, homogeneity, and stability of the protein solution considerably impact the likelihood of success in crystal formation.12 There are plenty of possibilities concerning the assessment of the protein solution “quality”.32 Herein, an overview of the most common techniques is presented (Table 1). Firstly, it is important to characterize the purity of the protein solution that should be as pure as possible (minimum of 90%). This is routinely achieved by sodium dodecyl sulfate polyacrylamide gel electrophoresis (SDS-PAGE), which separates proteins based on their molecular weight. Samples as low as 100 ng of protein can be detected in a few hours.32 Besides the purity of the protein solution, homogeneity and stability should also be checked. Dynamic light scattering (DLS) allows simultaneous determination of the monodispersity of the species of interest and the presence of soluble high-order assemblies and aggregates.33 When performed over time and/or at different temperatures, the stability of the protein solution in different buffers can also be tested. Likewise, the thermal stability of the protein in different buffers and in the presence of different ligands can be evaluated by differential scanning fluorometry (DSF).32 In addition, information on parameters such as protein activity, integrity and folding can be used to assess the quality of the protein solution.32,34 Activity assays are target-specific and have the additional benefit of measuring the fraction of active protein in a purified sample. Mass spectroscopy (MS) can be used to get detailed information about the protein primary structure, and thus on protein integrity.32 Regarding protein folding, it can be checked by NMR spectroscopy.35
Table 1 Overview of the important steps in the assessment of protein solution quality
Analysis type |
Measured parameter |
First-line methods |
Ref. |
Protein solution quality
|
Purity |
Separate and distinguish the protein of interest from other proteins (SDS-PAGE) |
32
|
Homogeneity |
Determine the monodispersity of the species of interest and the presence of aggregates (DLS) |
33
|
Stability |
Evaluate the stability over time (DLS) and the thermal stability (DFS) of the solution |
32
|
Additional analysis
|
Activity |
Measure the active and total concentration (UV-Vis spectroscopy) |
32
|
Integrity |
Analyze protein primary structure (MS) |
32
|
Folding |
Detect the folded and unfolded state (NMR) |
35
|
3. Measurement of the solubilized protein concentration in solution
Crystallization requires the creation of a supersaturated state for nucleation and subsequent protein crystal growth,36i.e. the quantity of the macromolecule present in solution must be higher than the solubility limit. The driving force of crystallization from solution (Δμ) arises thus from the difference between the chemical potential of the protein molecules in the supersaturated (μ) and saturated (μs) states as exhibited by eqn (1)36 | Δμ = μ − μs = RgT(ln a − ln as), | (1) |
where Rg is the gas constant, and T the absolute temperature. a and as are the protein activity in solution and the protein activity in solution in equilibrium with the crystals, respectively. Thus, this driving force can be expressed as a ratio of activities, which can be related to the concentration as defined by eqn (2)36 |  | (2) |
where γ and γeq are the activity coefficient and activity coefficient at equilibrium, respectively. C is the molar protein concentration in solution, while Ceq is the molar protein concentration in solution at equilibrium (i.e. solubility). The ratio of activity coefficients in the supersaturated and saturated solutions (γ/γeq) is often assumed to be equal to 1 (γ/γeq ≈ 1). Thereby, the driving force for crystallization can be expressed as the supersaturation ratio (S)36 |  | (3) |
Estimated values of supersaturation rely on the protein solubility and concentration measurements in solution. The main methods employed to measure protein solubility are presented in Table 3 and Fig. 5. Concerning the measurement of the protein concentration in solution, Table 2 compiles the principal methodologies used. UV-Vis spectroscopy at 280 nm is the most widely employed technique.32 The method allows for direct measurement of protein concentration with no further reagents needed to be added and total recovery of the protein sample. However, its application is limited to cases where the extinction coefficient of the protein under study is known or calculated from its amino acid composition.37 Additionally, the protein of interest must contain a known amount of tryptophans and tyrosines, since UV absorption at 280 nm is predominately from those aromatic amino acids.32,38 Dye-binding assays such as biuret [e.g. bicinchoninic acid (BCA), Lowry],39 colorimetric (e.g. Bradford),38 and fluorescent40 methods (Table 2) can be employed. Those assays involve the addition of a component that will directly or indirectly alter the color of the protein solution, whose intensity is proportional to the content of protein in the sample. Fourier transform infrared spectroscopy (FTIR)41 is another technique that can be used to quantify protein concentration. From the FTIR spectrum, it is possible to determine the concentration of amine bonds from amide band I and II, and then calculate the protein concentration. Limitations include the equipment requisites (e.g. minimal and maximal concentrations) and the incompatibility of several amine-containing buffers (e.g. HEPES, Tris) or additives (e.g. EDTA).
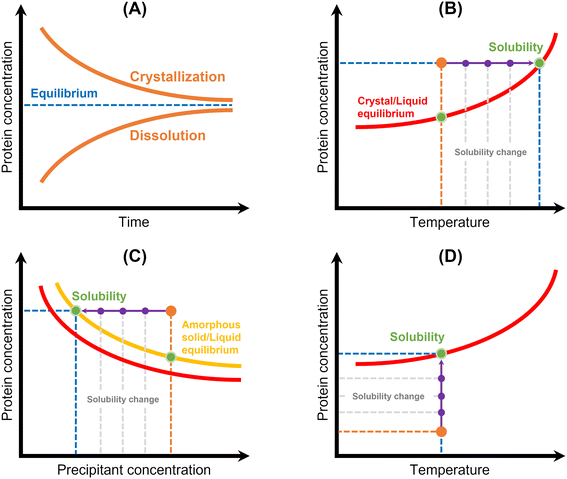 |
| Fig. 5 Schematic representation of the principles of the main methods used for protein solubility measurement: (A) equilibrium method, (B) temperature variation, (C) precipitation method, and (D) lyophilized protein addition and ultrafiltration/centrifugation. Red lines correspond to the crystal/liquid equilibrium curves, yellow line corresponds to the amorphous solid/liquid equilibrium curve, and protein concentration is represented in orange. Solubility is represented by a green spot, while solubility and/or protein concentration changes are represented by a purple arrow.12 | |
Table 2 Main methodologies to measure the protein concentration in solution and respective studied proteins
Methodology |
Advantages |
Limitations |
Studied protein |
Ref. |
Note: *the referred paper is a review. |
UV-Vis spectroscopy
|
– Simple |
– Depends on the composition of proteins' amino acids |
– BSA and IgG mAb |
42
|
Direct measurement of protein concentration |
– Fast |
– Limited application |
– Insulin |
43
|
– Lysozyme |
44–46
|
22* |
Biuret methods
|
– Compatibility with most surfactants |
– Incompatibility with certain substances (e.g. components that reduce copper, reducing agents) |
– Serum total protein |
39
|
Protein-copper chelation and secondary detection of reduced copper (BCA, Lowry) |
– No dependency on the composition of amino acids of the protein |
Colorimetric dye methods
|
– Fast |
– Incompatibility with surfactants |
– BSA and IgG mAb |
38
|
Protein–dye binding and direct detection of the color change (Bradford) |
– Compatibility with most components from the crystallization mixture |
– Dependency on the composition of amino acids |
– Insulin |
43
|
– Ref2NM, MAGOH, WW34, Y14, OFR57, and TAP |
47
|
Fluorescence dye methods
|
– High sensitivity |
– Specialized equipment |
– Proteins from tissue lysates |
40
|
Protein–dye binding and direct detection of increase in fluorescence ssociated with the bound dye |
– Low compatibility with certain components from the crystallization mixture (e.g. detergents, solvent) |
FTIR
|
– No dependency on the composition of amino acids of the protein |
– Equipment specificities |
– Proteins from raw milk |
41
|
Determination of the concentration of amine bonds |
– Incompatibility with buffers and additive containing amine |
4. Solubility measurement methodologies
Protein solubility is defined as the concentration of soluble protein that is in equilibrium with its crystal form under specific chemical and physical conditions (e.g. pH, temperature, additives).21 In fact, it is crucial to establish reliable protein solubility data in each system to design crystallization assays. Trevino et al. (2008)48 reviewed the different methodologies for measuring protein solubility, which include thermodynamic and apparent solubility experimentation. Thermodynamic solubility is defined as the concentration of soluble protein that is in equilibrium with a crystalline solid phase, while apparent solubility is defined as the concentration of soluble protein that is in equilibrium with an amorphous solid phase.
4.1. Thermodynamic solubility
Thermodynamic solubility is measured through equilibration of a protein solution with a crystalline solid phase42,48–58 (Table 3 and Fig. 5). The solubility is then equal to the protein concentration in the equilibrated solution, which can be assayed by a suitable analytical technique (see Table 2). Two approaches have been used:48 (1) dissolve crystals into an undersaturated solution until saturation is reached or (2) expose crystals to a supersaturated solution and allow crystal growth to bring the system to equilibrium (Fig. 5A). However, this methodology requires that crystallization conditions of the target protein must be known, and measurements can be laborious and time-consuming. Efforts have been made to reduce the required time and sample amount: Pusey & Gernert (1988)52 and later Cacioppo et al. (1991)53 developed a technique based upon maximization of the area for solute transfer, and minimization of the required solution volume to be brought to equilibrium. Nakazato et al. (2004)54 observed concentration gradients around a lysozyme crystal via two-beam interferometry identifying dissolution or growth conditions. The authors derived the solubility curve of lysozyme in function of precipitant in two days using 7 mg of protein. More recently, Adawy et al. (2013)59 employed phase shifting interferometry coupled to an image processing software program to study the concentration gradients developed around a lysozyme crystal during its growth and/or dissolution. Haire & Blow (2001)55 reported the measurement of protein solubility through a spin filter method, where speed and simplicity of the method stand out, but around 20 mg of protein per filter is required.
Table 3 Summary of the main methodologies used to measure protein solubilities (thermodynamic and apparent) and respective studied proteins
Methodology |
Advantages |
Limitations |
Studied protein |
Ref. |
Note: *the referred papers are reviews or focused on methodologies. |
Thermodynamic solubility
|
Equilibrium
|
– Widely accepted |
– Time-consuming |
– ETI and glucose isomerase |
49
|
Protein crystals are either grown or dissolved until equilibrium is reached |
– Accurate |
– Protein crystals are required |
– Insulin |
43, 75 and 76 |
– Lysozyme |
46, 49, 50, 52–56, 58 and 77 |
– Ribonuclease A and bacteriorhodopsin |
50
|
– Thaumatin |
57
|
48 and 51* |
Temperature variation
|
– Fast |
– Protein crystals are required |
– Equine serum albumin |
62
|
Temperature of a crystalline suspension is slowly increased until a clear solution is achieved |
– Automation feasibility |
– Heating rate |
– Glucose isomerase |
63
|
– Hemoglobin |
61
|
– Lysozyme |
60, 62 and 63 |
Apparent solubility
|
Precipitation
|
– Fast |
– Low accuracy due do phase transition control |
– α-Chymotrypsin, human serum albumin, RNase Sa, α-lactalbumin, fibrinogen, and ovalbumin |
68
|
Protein solution is mixed with a precipitant to induce precipitation |
– Protein crystals are not required |
– Alcohol dehydrogenases and D-serine dehydratase |
64
|
– Bovine serum albumin |
66
|
– ETI and glucose isomerase |
49
|
– FC fusion protein and single domain antibody construct |
65
|
– Insulin |
78
|
– Lysozyme |
44, 45, 49, 50, 53, 60, 68 and 78 |
– mAbs |
65–67
|
48* |
Dissolution of lyophilized protein
|
– Protein crystals are not required |
– Low accuracy due to highly concentrated protein solution and lyophilized protein composition |
– Lysozyme, zein, and casein |
79
|
Lyophilized protein (powder) is slowly added to the solvent until saturation is reached |
– Protein crystals are not required |
– Low accuracy due to highly concentrated protein solution |
– Lysozyme, zein, and casein |
48* |
Ultrafiltration/centrifugation
|
79
|
Protein is separated and concentrated from the rest of the solution based on the size |
48* |
Methodologies based on temperature variation42,60–62 have also been described (Table 3 and Fig. 5B). They are based on the determination of the temperature at which a crystalline suspension becomes a clear solution during heating with a certain rate. Consequently, it consists in establishing “a clear point”, which can be determined by light scattering,61 image analysis42,60,63 or refractive index.62 Ferreira et al. (2020)60 implemented an approach based on the temperature variation method to measure the solubility of lysozyme. First, the authors formed protein crystals in small crystallization drops by cooling. Then, temperature was increased, and an excess of saturated protein solution was added to the drop. Temperature was slowly increased until crystal dissolution was observed by optical microscopy. A similar methodology was employed by van Driessche et al. (2009).63 The authors measured the in situ growth and dissolution of crystal surfaces with a laser confocal differential interference contrast microscope to determine the equilibrium temperature of glucose isomerase and lysozyme. In both cases,60,63 equilibrium temperatures were determined within a short-range time and using a low amount of sample. However, this methodology is probably limited to model proteins (or well-known proteins), where it is necessary to know a priori the crystallization conditions and have some clues on the solubility of the system under study. Furthermore, the high costs and low accessibility of the instrumentation used in63 as well as the expertise required to design a cell with high optical access and with only a few crystals should be noted. Feeling-Taylor et al. (1999)61 reported a miniaturized optical scintillation technique (∼100 μL), where temperature dependence of solubility for human hemoglobin was measured. Firstly, crystals are formed and detected by light scattering. Then, dissolution of the crystals is promoted by temperature increases.
4.2. Apparent solubility
Owing to both the experimental time and the sample amount required to measure the thermodynamic solubility, more readily methods have been employed. Precipitation has been frequently used to measure protein solubility44,45,48,49,60,64–68 (Table 3 and Fig. 5). This methodology consists in inducing protein precipitation through the addition of polymers (e.g. PEG) or salts (e.g. ammonium sulphate) and then measuring the concentration of protein in solution using an analytical technique (see Table 3) (Fig. 5C). Some studies reported turbidity measurements as a primary detection for the formation of a precipitate.66,67 Precipitation allows faster solubility measurements, but only apparent solubility can be determined. The amorphous phase has different characteristics than the crystalline phase, and the control of the phase transition is rather difficult. For instance, protein solutions containing PEG can originate different protein phase behaviors (precipitates, crystals, or liquid–liquid phase separation) depending on the PEG concentration69 (Fig. 5C). Liquid–liquid phase separation is commonly observed because of the high supersaturations required for crystallizing proteins.70 Further, solubility values in the presence of an amorphous solid depend on the initial protein concentration, whereas solubility values in the presence of a crystalline solid phase do not.71 In fact, it is known that amorphous solids are significantly more soluble than their crystalline counterparts.72 Ferreira et al. (2020)60 used both precipitation and crystallization methods to measure lysozyme solubility and verified significant differences. The authors mentioned that structurally different lysozyme aggregates were probably produced during precipitation. Hofmann et al. (2018)65 verified that PEG-induced precipitation was not adequate to predict the solubility of a single-domain antibody construct. Possible explanations included conformational changes of the protein, volume exclusion effects of PEG and high protein concentration, which led to specific protein phase behavior when compared to diluted protein solutions.
The addition of lyophilized protein to solvent and concentration of a protein solution by ultrafiltration have also been employed48,68 (Table 3 and Fig. 5). Both methods require that the concentration of protein in solution be increased until saturation is reached (Fig. 5D). However, it can be difficult to carry out with highly soluble proteins, once gel-like or supersaturated solutions may form,68 hampering the determination of solubility accurately. In addition, lyophilized protein contains additional components such as water and salt, which can significantly impact solubility measurement.73,74
5. Measurement of other thermodynamic parameters that quantify protein binding
The second virial coefficient (B22), enthalpy (ΔH), and entropy (ΔS) are thermodynamic parameters enabling the measurement of the binding ability of a given protein in solution. Protein interactions are typically expressed in terms of the second virial coefficient,80 while the measurement of enthalpy and entropy changes provides a quantitative description of the forces governing molecular associations.81
5.1. Protein–protein interaction measurement – second viral coefficient
The second virial coefficient (B22) provides a measure of the protein–protein interactions, including contributions from excluded volume, electrostatic factors (attractive and repulsive) and hydrophobic interactions, which has been extensively reviewed by Wilson & Delucas (2014).80 For relatively weak interactions, B22 quantifies the deviation of a dilute solution from thermodynamic ideality. Thus, the osmotic virial coefficient (Π) can be defined by eqn (4)82,83 | 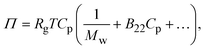 | (4) |
where Cp is the protein mass concentration and Mw the protein molecular weight. Considering protein molecules as spheres, the second virial coefficient can be expressed by eqn (5)82,83 | 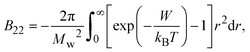 | (5) |
where W is the potential mean force and kB the Boltzmann constant, while r corresponds to the radial direction. B22 reflects the deviation degree of the osmotic pressure of a protein solution from an ideal solution, i.e. when B22 = 0. Therefore, positive and negative values of B22 indicate predominantly repulsive and attractive interactions, respectively. Following this approach, the determination of B22 can be useful for the selection of solution conditions favorable for protein aggregation (thus, crystallization propensity) or stable protein solution.80,84 Moreover, studies have shown the correlation between B22 and solubility for different proteins.84–86B22 can be experimentally determined using static light scattering (SLS),87 self-interaction chromatography (SIC),88 size exclusion chromatography (SEC),89 membrane osmometry (MO),84,90 and sedimentation velocity analytical ultracentrifugation (SV-AUC)91–93 (Table 4). For the definition of a crystallization window, MO and SV-AUC are not practical owing to the time and the protein amount required. Also, MO is prone to experimental issues, such as membrane fouling and adsorption. Despite the advantage size separation, SV-AUC may be one of the lowest throughput methods.
Table 4 Main equations and techniques to experimentally determine B22 and the respective studied proteins
Technique |
Equations & techniques |
Advantages |
Limitations |
Studied protein |
Ref. |
Notes: *the referred papers are reviews. Kc is the optical constant, Rθ the excess Rayleigh ratio at scattering angle θ, (dn/dCp) the refractive index increment for the protein/solvent pair, n the refractive index of the solvent, NA the Avogadro's number, and λ the wavelength of the laser beam. BHS is the protein excluded volume, k′ the chromatographic capacity factor, ϕ the surface area of the protein-modified particles that is available to the mobile phase protein, ρ the number of covalently immobilized protein molecules per unit of surface area of the bare chromatography particles, Vr the volume required to elute the interacting mobile phase protein, and V0 the volume required to elute a non-interacting species (neutral marker) of equivalent size. |
SLS
|
where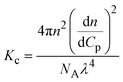 |
– Diagnostic of solution conditions that lead to crystallization |
– Protein amount and time required |
– Equine serum albumin and thaumatin |
87
|
– High sensitivity to the presence of aggregates |
– Insulin |
76
|
– Low compatibility with multicomponent mixtures |
– Lysozyme |
87 and 94 |
– mAb anti-CD20 |
83 and 95 |
SIC
|
where |
– High sensitivity |
– Protein immobilization required |
– Catalase, concanavalin A, and lactoferrin |
88
|
– High compatibility with multicomponent mixtures |
– Impurities/additives may interfere with protein binding sites |
– Lysozyme |
88 and 97 |
– Possibility of automation and miniaturization |
80 and 96* |
Other techniques
|
– Size exclusion chromatography (SEC) |
– Lysozyme |
84, 89, 91 and 92 |
– Membrane osmometry (MO) |
– mAbs |
80 and 90–92 |
– Sedimentation velocity analytical ultracentrifugation (SV-AUC) |
– Ovalbumin |
84
|
SLS has been most widely used to determine B22. Measurement of B22via SLS has been valuable to define a crystallization window.87,94 However, negative values of B22 denoting attractive interaction do not guarantee the formation of protein crystals. Pantuso et al. (2020)95 studied the aggregation mechanism of the monoclonal antibody anti-CD20 and verified no correlation between B22 and protein aggregation propensity. It is important to note that according to B22 description, it would be expected to apply at low protein concentrations, while crystallization experiments are usually conducted at high protein concentrations. Under such conditions, protein phase behavior can differ from that observed in dilute solutions due to crowding effects, deviation from thermodynamic ideality and higher-order interactions. Besides this aspect, multicomponent solutions are usually used to crystallize proteins, where the contribution of the interactions between protein and salt or polymers must be considered. On the other hand, the formation of a crystal requires protein–protein contacts that are correctly positioned to form an ordered crystalline lattice, and those contacts do not occur randomly but require selection of the binding partner.30,42 Constraints related to the measurement of B22 by SLS, such as the protein amount and time required, as well as the challenges associated with multicomponent systems, led to the exploration of other techniques.80,88
SIC is based on the assumption that increased attraction between the injected mobile-phase protein and the covalently, but randomly bound protein will result in an increase in the solution volume required to elute the injected protein from the column80,88,96,97 (Table 4). Valente et al. (2005)97 demonstrated that SIC provides a valid approach to measure B22 for lysozyme self-interaction as a function of several cosolvents. Tessier et al. (2003)96 verified a quantitative agreement between B22 measured by SIC and SLS for both lysozyme and chymotrypsinogen over a wide range of pH and ionic strengths. Nevertheless, experimental concerns can be related to the need for protein immobilization, and the fact that the impurities/additives present in solution may bind the immobilized protein sites.
Ruppert et al. (2001)84 derived an empirical relation between B22 and solubility. The authors verified a good agreement between the model and the experimental data for different experimental conditions (i.e. protein type and concentration, salt type and concentration, temperature, and pH) for the low-solubility range (up to 30 mg mL−1). A correlation between B22 and solubility was also observed by Guo et al. (1999)86 for lysozyme and ovalbumin under various solvent conditions. More recently, Link & Heng (2022)76 observed a 5-fold increase in insulin solubility with pH increase from 6.0 to 6.7, but SLS measurements showed no significant alteration of B22. Both B22 and solubility are strongly affected by solution parameters such as pH and ionic strength, or precipitating type/concentration, which could explain such differences.
5.2. Thermodynamic definition – Gibbs free energy
The change in Gibbs free energy (ΔG) depends on the enthalpic (ΔH) and entropic (ΔS) contributions as defined by eqn (6)81where ΔH can be regarded as a reflection of the nature of intermolecular contacts and hydration, while ΔS is related to the change in the number of possible conformations of a molecule.98 ΔH has mostly been assessed indirectly from solubility data (indirect methods) but it can be directly measured by microcalorimetry (direct methods). Regarding ΔS, it can only be determined indirectly from solubility data (indirect methods).81
5.2.1. Direct methods.
During a first-order phase transition, a thermodynamic system releases or absorbs latent heat and crystallization is not an exception. Upon protein crystallization, the binding energy of the protein molecules is released as heat.81 The exothermal signal of the crystallizing protein solution can be recorded from calorimetry experiments to experimentally determine the crystallization enthalpy (ΔH).81 This methodology is combined with spectrophotometric measurement of protein concentration in solution to assess the fraction of crystallized protein (ΔCpV) (Table 5). There are few reports on the measurement of ΔH due to experimental difficulties. For instance, it is important to ensure that the onset and offset of the crystallization process is neither too fast compared to the initial thermalization of the sample and instrument nor too slow to allow for a significant signal. Besides, the value of ΔH is typically less than 100 kJ mol−1,43,81,99 which is difficult to measure. Recently, Hentschel et al. (2021)81 measured the ΔH of lysozyme solutions by combining microcalorimetry with UV-Vis spectroscopy. The authors verified that the measured values agree with the ones determined by the van 't Hoff equation based on solubility data.
Table 5 Methods to determine enthalpic variables (ΔH and ΔS) and the respective studied proteins
|
Technique |
Equation |
Studied protein & thermodynamic values |
Ref. |
Note:
Q is the heat released upon crystallization, ΔP the differential microcalorimetric power signal, V the sample volume, and ti and tf the initial and final times, respectively. |
Direct
|
Calorimetry
|
|
– Lysozyme |
81
|
|
ΔH ≈ 65 kJ mol−1 |
Indirect
|
van't Hoff analysis of solubility data
|
|
– Chymotrypsinogen A |
90
|
ΔH ∈ [−79.8, −27.2] kJ mol−1 |
– Glucose isomerase |
63
|
ΔH ∈ [−174, −144] kJ mol−1 |
ΔS ∈ [−462, −370] J mol−1 K−1 |
– Hemoglobin |
61
|
ΔH = 155 kJ mol−1 |
– Insulin |
43
|
ΔH ∈ [−55, −20] kJ mol−1 |
ΔS ∈ [−110, −35] J mol−1 K−1 |
– Orthorhombic lysozyme |
45
|
ΔH = 22 kJ mol−1 |
– Tetragonal lysozyme |
45, 63, 81, 90, 93 and 100 |
ΔH ∈ [−129, −31] kJ mol−1 |
ΔS ∈ [−10, 241] J mol−1 K−1 |
5.2.2. Indirect methods.
ΔH and ΔS can be determined indirectly based on the van 't Hoff analysis of solubility data81 (Table 5). For simplicity, the relationship between the equilibrium constant and temperature can be represented by the van 't Hoff equation, which is derived from the relationship between equilibrium constants and Gibbs free energy as expressed by eqn (7)43,81,98 |  | (7) |
where K is the equilibrium constant, which can be represented by eqn (8)37,73 | 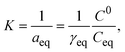 | (8) |
where C0 is the molar protein concentration in a hypothetical solution standard state (1 M). By combining eqn (7) and (8) and assuming a solution close to ideality (γeq ≈ 1), eqn (9) can be derived | 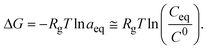 | (9) |
6. Instrumentation for solubility measurements
After revising the employed methodologies and techniques for protein solubility measurements, this section introduces the platforms and protocols that are currently available in the literature for this purpose. In fact, the reported literature is especially focused on APIs (active pharmaceutical ingredients) (e.g. paracetamol, adipic acid). However, the increasing interest in proteins has contributed to the appearance of several protein-oriented devices. Stura et al. (1992)101 designed a simple standard screen (footprint) for the comparison of protein solubilities, which was aided by SDS-PAGE and isoelectric focusing data to monitor and relate crystallization results to biochemical analysis. This screening strategy revealed a high success rate in crystal production for both proteins (e.g. glycoproteins) and more complex biological systems. The set-up consisted of a temperature controlled multiwell sitting-drop vapor diffusion tray. Later, Santesson et al. (2003)64 followed the Stura footprinting screening strategy for D-serine dehydratase.
6.1. Thermodynamic solubility experimentation
During the last decades, several protocols were reported in the literature by covering distinct protocols and set-ups. Pusey & Gernert (1988)52 developed a column packed with protein microcrystals, which was fed by a solution below and above the solubility limit to enable dissolution or crystal growth, respectively. The authors were able to measure lysozyme solubility within a time frame of 24 h using a column volume between 1 and 5 mL. Later, the methodology was improved by Cacioppo et al. (1991),53 where the required column volume was reduced to a range between 75 and 900 μL.
Haire & Blow (2002)55 used a spin filter method to measure protein solubility. It consisted of equilibrating a crystal slurry with protein solution and then centrifuging through a filter to assay the protein concentration. More recently, Nakazato et al. (2004)54 developed a dialysis cell for both direct optical measurement of protein concentration and observation of concentration gradients around a crystal via two-beam interferometry. Chen et al. (2005)57 reported one of the first droplet-based microfluidic devices for crystallizing proteins. However, the authors measured the solubility of thaumatin in Eppendorf tubes by both dissolution of protein crystals and crystallization of thaumatin solution. Table 6 summarizes the reported works where thermodynamic solubility experiments were performed.
Table 6 Summary of the reported experimental protocols and respective platforms for thermodynamic solubility experimentation and studied proteins
Apparatus capabilities and associated techniques |
Solubility screening details |
Studied protein |
Ref. |
– Eppendorf tubes at fixed temperature– Frequent gentle mixing procedure – Nanodrop UV-Vis spectrophotometer for protein concentration measurements |
– Increase in solubility as a function of pH– Solubility independency of the zinc salt type |
– Insulin |
76
|
|
– Temperature-controlled vials– HPLC analysis for protein concentration measurements |
– Amino acids increase solubility (arginine)– Amino acids do not alter solubility (leucine and glycine) |
– Insulin |
75
|
|
– Set-up consists of drop jacketed glass cell– UV-Vis spectrophotometer for protein concentration measurements |
– Polymeric additives induce entropic variations while the structural integrity is preserved– Enthalpic variations induced by pH and ionic strength – Enhanced chemical activity evidenced by lower solubility values |
– Lysozyme |
60
|
|
– Novel approach using laser confocal differential interference contrast microscopy (LCM-DIM) in a temperature-controlled stage |
– Fast and precise determination of solubility in function of temperature– Determination of thermodynamic parameters (enthalpy and entropy) – Derivation of solubility curves |
– Glucose isomerase– Lysozyme |
63
|
|
– Microdialysis crystallization method– Crystal quality characterized by the Wilson plot method |
– Determination of solubility curves at variable pH and precipitation solution concentration ranges– Crystal quality influenced by pH |
– Lysozyme |
56
|
|
– Eppendorf tubes at fixed temperature– UV-Vis spectrophotometer for protein concentration measurements |
– Significant solubility differences under different precipitant solutions |
– Thaumatin |
57
|
|
– Batch technique with temperature-controlled vials– UV-Vis spectrophotometer and Bradford reagent for proteinconcentration measurements |
– Temperature, and enthalpy and entropy dependence of solubility at varying solution composition and temperature |
– Insulin |
43
|
|
– Automated microbatch method for initial screening– Hanging-drop vapor diffusion set-up – Decoupling nucleation and growth stages |
– Derivation of solubility curves |
– Trypsin– C-Phycocyanin |
15
|
|
– Miniature column solubility apparatus– Recrystallization and redissolution by dialysis – SDS-PAGE for protein species identification |
– Wide range of temperature, pH, and sodium chloride solution concentration– Rapid determination of solubility curves – Solubility increases in function of temperature – pH has a varied and unpredictable effect on solubility |
– Lysozyme |
46
|
|
– Miniaturized scintillation arrangement with integrated temperature control– X-ray crystallography for structural characterization |
– Determination of solubility in function of temperature– Estimation of thermodynamic parameters (enthalpy) |
– Hemoglobin |
61
|
|
– Novel technique consisting of a Michelson interferometer and a temperature controlled-stage– High accuracy and time saving protocol |
– Applicable to solubility measurements of a metastable phase– Solubility curves for tetragonal and orthorhombic crystal forms |
– Lysozyme |
77
|
|
– Batch set-up– Hanging-drop vapor diffusion set-up – Diffractometer for crystal lattices characterization |
– Slight solubility differences in H2O and D2O, possibly because of differences between H and D bonds |
– Lysozyme |
58
|
|
– Easy to use semi-micro column apparatus– Optimized set-up configuration – Eliminates the time factor when deriving protein phase diagrams |
– Rapid determination of solubility curves– Solubility increase of different crystal forms with decreasing salt concentration and increasing temperature |
– Lysozyme |
53
|
|
– Automated microbatch system– Complete sample mixing – Minimal evaporation – Application specific software – Low operation cost |
– Speed and ease of operation, and simplicity– Determination of broad protein solubility properties |
– Lysozyme– Glucose isomerase – ETI |
49
|
6.2. Apparent solubility experimentation
Guilloteau et al. (1992)44 revealed that information about solubility data allows the determination of optimum supersaturation conditions, thus contributing to a better experimental reproducibility. Moreover, the authors concluded that the temperature influence on the protein (lysozyme) solubility and crystal form was modulated by the salt nature. Table 7 summarizes the reported works where apparent solubility experiments were performed.
Table 7 Summary of the reported experimental protocols and respective platforms for apparent solubility experimentation and studied proteins
Apparatus capabilities and associated techniques |
Solubility screening details |
Studied protein |
Ref. |
– Set-up consists of drop jacketed glass cell– UV-Vis spectrophotometer for protein concentration measurements |
– Polymeric additives induce entropic variations while the structural integrity is preserved– Enthalpic variations induced by pH and ionic strength – Enhanced chemical activity evidenced by lower solubility values |
– Lysozyme |
60
|
|
– In-house acoustically levitated drops set-up– Commercial kits from Hampton research in standard vapor diffusion experiments for crystallization trials – Right-angle light scattering for protein concentration measurements |
– Several crystallizing agents are tested– Concentration measurements of all components in the drop at any time during the experiment – Derivation of solubility curves followed by crystallization condition optimization |
– Alcohol dehydrogenase– D-Serine dehydratase |
64
|
|
– Thermal denaturation experiments performed on AVIV spectrophotometers |
– Increased negative surface charge strongly correlates with increased solubility– No correlation between positive surface charge and solubility |
– α-Chymotrypsin– Lysozyme – Serum albumin – RNase Sa – Ovalbumin – α-Lactalbumin – Fibrinogen |
68
|
|
– Novel method using a high-throughput screening approach (robotic liquid handling section)– Photometric turbidity measurements and in-line concentration measurements – Controlled concentration of the solution while constantly analyzing the solution state |
– Determination of process relevant solubility limits– Analysis of relevant kinetic effects (buffer systems) |
– Lysozyme– Insulin |
78
|
|
– UV-Vis spectrophotometer for protein concentration measurements– Diffractometer for crystal lattice characterization |
– Solubility curve measurements– Slight influence of the cation nature on the solubility – Solubility and crystal form are affected differently by temperature changes – Salt nature determines the crystal form |
– Lysozyme |
44
|
|
– Automated microbatch system– Complete sample mixing – Minimal evaporation – Application specific software – Low operation cost |
– Speed and ease of operation, and simplicity– Determination of broad solubility properties |
– Lysozyme– Glucose isomerase – ETI |
49
|
|
– Batch set-up in polystyrene test tubes– Ultrafiltration crystallization method at controlled temperature – UV-Vis spectrophotometer for protein concentration measurements |
– Solubility examined as a function of temperature, pH, and salt concentration– Negative crystallization enthalpy for tetragonal crystal form – Positive crystallization enthalpy for orthorhombic crystal form |
– Lysozyme |
45
|
6.3. Solubility screening in microfluidic devices
The conventional protein crystallization techniques such as vapor diffusion (hanging-drop or sitting-drop), microbatch, etc. rely on testing many potential crystallization conditions, while consuming large amounts of the protein of interest, without any theoretical background guidance. Therefore, microfluidic devices constitute a high-throughput methodology to systematically screen crystallization conditions and, simultaneously, limit the protein volume consumption. Moreover, microfluidics allows complex protocols to be carried out on a single chip (Lab-on-a-Chip devices) for fluid-handling and processing. These devices offer attractive advantages over conventional macroscale instruments, such as shorter operation times, higher mixing and heat transfer efficiencies, lower energy consumption, etc.102,103 Sommer & Larsen (2005)104 highlighted that the developed protocol based on tailor-made microbatch crystallization screening resulted in around 50% crystallization probability per experiment for the studied proteins (SERCA and UMP kinase). Most of the reported works in microfluidic devices consist in implementing the microbatch technique, but this is not the only implemented traditional crystallization technique. Leng & Salmon (2009)13 revised the existent microfluidic devices for crystallization applications more focused on lab-on-a-chip instrumentation for protein crystallization condition screening. More recently, Candoni et al. (2019)105 published a review paper highlighting microfluidic devices developed by the research team for solubility and crystallization experimentation, mostly covering APIs (e.g. paracetamol, sulfathiazole, glyclazide) and a few protein examples (e.g. lysozyme, rasburicase, QR2). Microfluidic devices were also applied for measuring thermodynamic parameters as reported elsewhere.100,106,107Table 8 and Fig. 6 present an overview of the reported microfluidic devices used to screen protein solubility conditions.
Table 8 Summary of the reported microfluidic devices for protein solubility screening and respective studied proteins
Crystallization technique |
Device capabilities |
Solubility screening details |
Studied protein |
Ref. |
Microbatch
|
– Versatility aspect: on-chip dialysis and in situ X-ray diffraction |
– Micro-dialysis method |
– Lysozyme |
108
|
– Screening and optimization through concentration and temperature control |
– Crystallization and dissolution experiments based on phase behaviors |
– Insulin |
– Tailoring crystal size, number, and quality |
– IspE |
Microbatch (dispersed microdroplets)
|
– Chemical library for non-specialists in microfluidics |
– Directly solubilizing powder (only for APIs) |
– Lysozyme |
105
|
– Screening and optimization through concentration and temperature control |
– Derivation of solubility curves |
– Rasburicase |
– Concentration measurements via in-line UV-Vis |
– QR2 |
– Crystals characterization via X-ray diffraction |
– etc. |
Reverse vapor diffusion
|
– Drop state is determined at any point in time |
– Evaporation-based method |
– Lysozyme |
50
|
– Efficient method to traverse phase space along a known path in the phase diagram |
– Determination of solubility boundaries |
– Ribonuclease A – bacteriorhodopsin |
– Possibility to decouple nucleation and growth events to enhance crystal size and quality |
Microbatch
|
– Tailor-made crystallization screening |
– Complete screening of phase behaviors |
– SERCA |
104
|
– High probability of yielding crystallization hits |
– UMP kinase |
Microbatch
|
– Fully automated |
– Complete mapping of phase behaviors |
– Lysozyme |
109
|
– Formulation chip: rapidly generation of complex mixtures |
– Xylanase |
– Design of maximum likelihood crystallization trials |
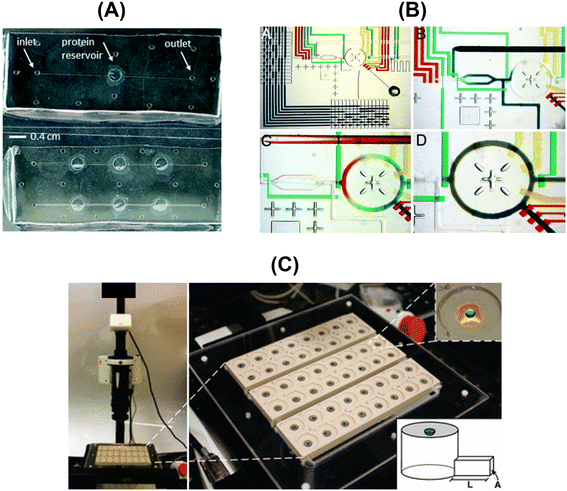 |
| Fig. 6 Overview of the designed and fabricated microfluidic platforms for protein solubility measurements: (A) top-view of the microfluidic chips embedding the dialysis membrane for the on-chip crystallization of proteins and in situ X-ray measurements [adapted from ref. 108 with permission from The Royal Society of Chemistry], (B) optical micrographs showing the combinatorial mixing using a microfluidic formulator. [Adapted from ref. 109 with permission from the Proceedings of the National Academy of Sciences of the United States of America (copyright (2004) National Academy of Sciences, U.S.A.)], and (C) photograph of the automated data acquisition set-up: three 16-compartment evaporation-based crystallization platforms [adapted from ref. 50 (https://pubs.acs.org/doi/full/10.1021/jp911780z). Further permission related to the material excerpted should be directed to the American Chemical Society]. | |
Outlook and future perspectives
This highlight critically reviews the advances in experimental approaches to measure thermodynamic parameters able to assess protein solubility and, consequently, to enhance crystal formation propensity. Initially, protein interactions and protein solution quality are highlighted as the main variables to successfully crystallize a protein, which reveals the importance of being characterized before conducting any assay. Secondly, within the methodologies to measure protein solubilities (thermodynamic and apparent), efforts have been directed towards the reduction of the required sample amount and time. In what concerns the assessment of intermolecular interactions, discrepancies between second virial coefficient measurements and protein aggregation rates have been frequently observed. Lastly, experimental protocols and platforms are revised in terms of apparatus capabilities, experimental techniques, and studied proteins. Microfluidic devices offer unequalled conditions to conduct protein solubility experiments and are capable of answering both questions: sample amount and time.
Despite the significant developments in this research field, there is still room for improvement. In this context, one can learn from the strategies applied to small organic molecules (e.g. APIs), even though protein and small organic molecules have distinct behaviors. Traditional and advanced procedures and instrumentation commonly used to measure small organic molecules' solubility can be adapted to experimentally determine protein solubility. The solvent addition method consists in adding a solvent dropwise, at a constant temperature, to a known crystalline suspension until full dissolution of the material is observed. Reus et al. (2015)110 applied the solvent addition method in a multi-reactor crystallizer (Crystal16) for the measurement of p-hydroxybenzoic acid solubility. The authors determined a “clear point” through turbidity measurements, video recording, and FTIR concentration measurements. The results showed that the obtained solubility values agreed with the equilibrium method. This could represent an alternative to the equilibrium method as it enables more expeditious solubility measurements. Nevertheless, crystallization conditions of the protein under study must be known, and operating parameters such as the required volume and accuracy of the detection technique must be considered. Another important aspect to mention is the equipment used, Crystal16. It is a well-known commercialized apparatus to measure, not only the solubility, but also to screen a phase diagram. The system consists of a micro-vial set-up with an integrated transmissivity technology from Technobis Crystallization Systems.111 The theoretical understanding behind the equipment was initially introduced by ter Hoorst's group.112 More recently, Peybernès et al. (2018)73 performed in situ solubility measurements of organic compounds directly from powder using small amounts of material (30 mg) and a time frame of a few hours. The authors developed a microfluidic set-up where the solvent flows through the powder bed blocked by a filter. At the outlet of the filter, due to the dissolution of the powder, the solution is saturated.
Regardless of all the experimental advances and employed methodologies and platforms, the task of measuring protein solubility can still be a costly and time-consuming process, frequently characterized by low success rates. Furthermore, the methods described along the highlight are often not readily amenable for high-throughput screening. Therefore, sequence-based computational tools have been used to predict protein solubility.113,114 However, these tools suffer from relatively low prediction accuracy and limited applicability for various classes of proteins, mostly only covering model proteins (e.g. lysozyme, thaumatin, insulin). Accuracy can be improved by using more advanced algorithms and incorporating additional information. For instance, protein 3D structure information can be used to provide more geometric information of each amino acid residual.115 It is also worth mentioning some computational tools (e.g. OBScore, ParCrys, CrystalP2, XtalPred, PPCPred, SCMCRYS, SVMCRYS, PredPPCrys I & II, Crysalis I & II) that focus on feature extraction of protein sequences to predict crystallization propensity.2,116,117 Considerable developments are rapidly emerging alongside the recent advances in Artificial Intelligence (AI),118–121 which includes deep-learning models such as DeepSoluE,115 ProteinBERT,122 DSResSol,123 PON-Sol2,124 and DeepSol.125
Author contributions
Joana Ferreira: conceptualization, methodology, investigation, writing – original draft. Filipa Castro: conceptualization, methodology, investigation, writing – original draft.
Conflicts of interest
There are no conflicts to declare.
Acknowledgements
J. Ferreira acknowledges funding from CEFT under FCT/MCTES (PIDDAC) through a postdoctoral scholarship. F. Castro acknowledges FCT CEEC Individual contract (2022.06818.CEECIND). This work was financially supported by: HealthyWaters (NORTE-01-0145-FEDER-000069), supported by Norte Portugal Regional Operational Programme (NORTE 2020), under the PORTUGAL 2020 Partnership Agreement, through the European Regional Development Fund (ERDF); and LA/P/0045/2020 (ALiCE), UIDB/00532/2020 and UIDP/00532/2020 (CEFT), funded by national funds through FCT/MCTES (PIDDAC).
References
- H. M. Berman, J. Westbrook, Z. Feng, G. Gilliland, T. N. Bhat, H. Weissig, I. N. Shindyalov and P. E. Bourne, Nucleic Acids Res., 2000, 28, 235–242 CrossRef CAS PubMed.
- C. Jin, Z. Shi, C. Kang, K. Lin and H. Zhang, Int. J. Mol. Sci., 2022, 23, 1–12 CAS.
- PDB data distribution by experimental method and molecular type, https://www.rcsb.org/stats/summary, (accessed 14 July 2023).
- S. C. Shoemaker and N. Ando, Biochemistry, 2018, 57, 277–285 CrossRef CAS.
- L. Maveyraud and L. Mourey, Molecules, 2020, 25, 1–18 CrossRef PubMed.
- R. dos Santos, A. L. Carvalho and A. C. A. Roque, Biotechnol. Adv., 2017, 35, 41–50 CrossRef CAS PubMed.
- S. Puhl, L. Meinel and O. Germershaus, Asian J. Pharm. Sci., 2016, 11, 469–477 CrossRef.
- D. Hekmat, D. Hebel and D. Weuster-Botz, Chem. Eng. Technol., 2008, 31, 911–916 CrossRef CAS.
-
E. K. Lee and W. Kim, in Isolation and Purification of Proteins, ed. R. Hatti-Kaul and B. Mattiasson, CRC Press, New York, Basel, 2003, pp. 1–43 Search PubMed.
- C. N. Nanev, Prog. Cryst. Growth Charact. Mater., 2020, 66, 1–23 CrossRef.
- J. Newman, E. E. Bolton, J. Müller-Dieckmann, V. J. Fazio, D. T. Gallagher, D. Lovell, J. R. Luft, T. S. Peat, D. Ratcliffe, R. A. Sayle, E. H. Snell, K. Taylor, P. Vallotton, S. Velanker and F. Von Delft, Acta Crystallogr., Sect. F: Struct. Biol. Cryst. Commun., 2012, 68, 253–258 CrossRef CAS PubMed.
- B. Rupp, Acta Crystallogr., Sect. F: Struct. Biol. Commun., 2015, 71, 247–260 CrossRef CAS PubMed.
- J. Leng and J.-B. Salmon, Lab Chip, 2009, 9, 24–34 RSC.
- S. Black, L. Dang, C. Liu and H. Wei, Org. Process Res. Dev., 2013, 17, 486–492 CrossRef CAS.
- E. Saridakis and N. E. Chayen, Biophys. J., 2003, 84, 1218–1222 CrossRef CAS PubMed.
- Dimensions AI, https://app.dimensions.ai/discover/publication, (accessed 14 July 2023).
- A. McPherson and J. A. Gavira, Acta Crystallogr., Sect. F: Struct. Biol. Commun., 2014, 70, 2–20 CrossRef CAS PubMed.
- Z. S. Derewenda, Acta Crystallogr., Sect. D: Biol. Crystallogr., 2010, 66, 604–615 CrossRef CAS PubMed.
-
W. K. Ranatunga, D. Su and M. F. Romero, in Molecular Life Sciences, ed. R. D. Wells, J. S. Bond, J. Klinman and B. S. S. Masters, Springer, New York, NY, 2018, pp. 841–848 Search PubMed.
- J. J. Mcmanus, P. Charbonneau, E. Zaccarelli and N. Asherie, Curr. Opin. Colloid Interface Sci., 2016, 22, 73–79 CrossRef CAS.
-
A. Ducruix and R. Giegé, Crystallization of Nucleic Acids and Proteins – A Practical Approach, Oxford University Press, 1999, pp. 1–435 Search PubMed.
- L. J. Quang, S. I. Sandler and A. M. Lenhoff, J. Chem. Theory Comput., 2014, 10, 835–845 CrossRef CAS PubMed.
- D. Fusco, J. J. Headd, A. De Simone, J. Wang and P. Charbonneau, Soft Matter, 2014, 10, 290–302 RSC.
- K. Kubiak-Ossowska, M. Cwieka, A. Kaczynska, B. Jachimska and P. A. Mulheran, Phys. Chem. Chem. Phys., 2015, 17, 24070–24077 RSC.
- M. Ptak-Kaczor, M. Banach, K. Stapor, P. Fabian, L. Konieczny and I. Roterman, Int. J. Mol. Sci., 2021, 22, 1–18 Search PubMed.
- J. A. Carver, A. B. Grosas, H. Ecroyd and R. A. Quinlan, Cell Stress Chaperones, 2017, 22, 627–638 CrossRef CAS PubMed.
- F. L. V. Gray, M. J. Murai, J. Grembecka and T. Cierpicki, Protein Sci., 2012, 21, 1954–1960 CrossRef CAS PubMed.
-
Z. S. Derewenda and A. Godzik, The “sticky patch” model of crystallization and modification of proteins for enhanced crystallizability, 2017, vol. 1607, pp. 77–115 Search PubMed.
- M. Cieślik and Z. S. Derewenda, Acta Crystallogr., Sect. D: Biol. Crystallogr., 2009, 65, 500–509 CrossRef PubMed.
- W. N. Price, Y. Chen, S. K. Handelman, H. Neely, R. Karlin, R. Nair, J. Liu, M. Baran, S. N. Tong, F. Forouhar, S. S. Swaminathan, R. Xiao, J. R. Luft, A. Lauricella, G. T. Detitta, B. Rost, G. T. Montelione and J. F. Hunt, Nat. Biotechnol., 2010, 27, 51–57 CrossRef PubMed.
- M. Buck, J. Boyd, C. Redfield, C. M. Dobson, D. A. MacKenzie, D. J. Jeenes and D. B. Archer, Biochemistry, 1995, 34, 4041–4055 CrossRef CAS PubMed.
- B. Raynal, P. Lenormand, B. Baron, S. Hoos and P. England, Microb. Cell Fact., 2010, 13, 1–10 Search PubMed.
- U. Nobbmann, M. Connah, B. Fish, P. Varley, C. Gee, S. Mulot, J. Chen, L. Zhou, Y. Lu, F. Sheng, J. Yi and S. E. Harding, Biotechnol. Genet. Eng. Rev., 2007, 24, 117–128 CrossRef CAS PubMed.
- Y. Wang, N. van Oosterwijk, A. M. Ali, A. Adawy, A. L. Anindya, A. S. S. Dömling and M. R. Groves, Sci. Rep., 2017, 7, 1–10 CrossRef PubMed.
- M. Dreydoppel, J. Balbach and U. Weininger, J. Biomol. NMR, 2022, 76, 3–15 CrossRef CAS PubMed.
-
J. W. Mullin, Crystallization, Oxford, United Kingdom, 4th edn, 2001, pp. 1–594 Search PubMed.
- C. N. Pace, F. Vajdos, L. Fee, G. Grimsley and T. Gray, Protein Sci., 1995, 4, 2411–2423 CrossRef CAS PubMed.
-
J. E. Noble, in Methods in Enzymology, Edited by Press, Academic, 2014, vol. 536, pp. 17–26 Search PubMed.
- K. Zheng, L. Wu, Z. He, B. Yang and Y. Yang, Measurement, 2017, 112, 16–21 CrossRef.
- J. R. Wiśniewski and F. Z. Gaugaz, Anal. Chem., 2015, 87, 4110–4116 CrossRef PubMed.
- Y. Etzion, R. Linker, U. Cogan and I. Shmulevich, J. Dairy Sci., 2004, 87, 2779–2788 CrossRef CAS PubMed.
- T. Detoisien, M. Forite, P. Taulelle, J. Teston, D. Colson, J. P. Klein and S. Veesler, Org. Process Res. Dev., 2009, 13, 1338–1342 CrossRef CAS.
- L. Bergeron, L. F. Filobelo, O. Galkin and P. G. Vekilov, Biophys. J., 2003, 85, 3935–3942 CrossRef CAS PubMed.
- J. P. Guilloteau, M. M. Riès-Kautt and A. F. Ducruix, J. Cryst. Growth, 1992, 122, 223–230 CrossRef CAS.
- S. B. Howard, P. J. Twigg, J. K. Baird and E. J. Meehan, J. Cryst. Growth, 1988, 90, 94–104 CrossRef CAS.
- E. L. Forsythe, R. A. Judge and M. L. Pusey, J. Chem. Eng. Data, 1999, 44, 637–640 CrossRef CAS.
- A. P. Golovanov, G. M. Hautbergue, S. A. Wilson and L. Y. Lian, J. Am. Chem. Soc., 2004, 126, 8933–8939 CrossRef CAS PubMed.
- S. R. Trevino, J. M. Scholtz and C. N. Pace, J. Pharm. Sci., 2008, 97, 4155–4166 CrossRef CAS PubMed.
- N. E. Chayen, P. D. Shaw Stewart, D. L. Maeder and D. M. Blow, J. Appl. Crystallogr., 1990, 23, 297–302 CrossRef CAS.
- S. Talreja, S. L. Perry, S. Guha, V. Bhamidi, C. F. Zukoski and P. J. A. Kenis, J. Phys. Chem. B, 2010, 114, 4432–4441 CrossRef CAS PubMed.
- N. Asherie, Methods, 2004, 34, 266–272 CrossRef CAS PubMed.
- M. L. Pusey and K. Gernert, J. Cryst. Growth, 1988, 88, 419–424 CrossRef CAS.
- E. Cacioppo, S. Munson and M. L. Pusey, J. Cryst. Growth, 1991, 110, 66–71 CrossRef CAS.
- K. Nakazato, T. Homma and T. Tomo, J. Synchrotron Radiat., 2004, 11, 34–37 CrossRef CAS PubMed.
- L. F. Haire and D. M. Blow, J. Cryst. Growth, 2001, 232, 17–20 CrossRef CAS.
- W. Iwai, D. Yagi, T. Ishikawa, Y. Ohnishi, I. Tanaka and N. Niimura, J. Synchrotron Radiat., 2008, 15, 312–315 CrossRef CAS PubMed.
- D. L. Chen, G. J. Gerdts and R. F. Ismagilov, J. Am. Chem. Soc., 2005, 127, 9672–9673 CrossRef CAS PubMed.
- I. Broutin, M. Riès-Kautt and A. Ducruix, J. Appl. Crystallogr., 1995, 28, 614–617 CrossRef CAS.
- A. Adawy, K. Marks, W. J. de Grip, W. J. P. van Enckevort and E. Vlieg, CrystEngComm, 2013, 15, 2275–2286 RSC.
- C. Ferreira, M. F. Pinto, S. Macedo-Ribeiro, P. J. B. Pereira, F. A. Rocha and P. M. Martins, Phys. Chem. Chem. Phys., 2020, 22, 16143–16149 RSC.
- A. R. Feeling-Taylor, R. Michael Banish, R. E. Hirsch and P. G. Vekilov, Rev. Sci. Instrum., 1999, 70, 2845–2849 CrossRef CAS.
- R. J. Gray, W. B. Hou, A. B. Kudryavtsev and L. J. DeLucas, J. Cryst. Growth, 2001, 232, 10–16 CrossRef CAS.
- A. E. S. van Driessche, J. A. Gavira, L. D. Patiño Lopez and F. Otalora, J. Cryst. Growth, 2009, 311, 3479–3484 CrossRef CAS.
- S. Santesson, E. S. Cedergren-Zeppezauer, T. Johansson, T. Laurell, J. Nilsson and S. Nilsson, Anal. Chem., 2003, 75, 1733–1740 CrossRef CAS PubMed.
- M. Hofmann, M. Winzer, C. Weber and H. Gieseler, J. Pharm. Pharmacol., 2018, 70, 648–654 CrossRef CAS PubMed.
- M. Oeller, P. Sormanni and M. Vendruscolo, Sci. Rep., 2021, 11, 1–10 CrossRef PubMed.
- Q. Chai, J. Shih, C. Weldon, S. Phan and B. E. Jones, mAbs, 2019, 11, 747–756 CrossRef CAS PubMed.
- R. M. Kramer, V. R. Shende, N. Motl, C. N. Pace and J. M. Scholtz, Biophys. J., 2012, 102, 1907–1915 CrossRef CAS PubMed.
- I. R. M. Juckes, Biochim. Biophys. Acta, 1971, 229, 535–546 CrossRef CAS PubMed.
- M. C. R. Heijna, W. J. P. van Enckevort and E. Vlieg, Phys. Rev. E: Stat., Nonlinear, Soft Matter Phys., 2007, 76, 1–7 CrossRef PubMed.
- Y.-C. Shih, J. M. Prausnitz and H. W. Blanch, Biotechnol. Bioeng., 1992, 40, 1155–1164 CrossRef CAS PubMed.
- B. C. Hancock and M. Parks, Pharm. Res., 2000, 17, 397–404 CrossRef CAS PubMed.
- G. Peybernès, R. Grossier, F. Villard, P. Letellier, M. Lagaize, N. Candoni and S. Veesler, Org. Process Res. Dev., 2018, 22, 1856–1860 CrossRef.
- G. Peybernès, R. Grossier, F. Villard, P. Letellier, N. Candoni and S. Veesler, Cryst. Growth Des., 2020, 20, 3882–3887 CrossRef.
- F. J. Link and J. Y. Y. Heng, CrystEngComm, 2021, 23, 3951–3960 RSC.
- F. J. Link and J. Y. Y. Heng, Cryst. Growth Des., 2022, 22, 3024–3033 CrossRef CAS PubMed.
- G. Sazaki, K. Kurihara, T. Nakada, S. Miyashita and H. Komatsu, J. Cryst. Growth, 1996, 169, 355–360 CrossRef CAS.
- M. Wiendahl, C. Völker, I. Husemann, J. Krarup, A. Staby, S. Scholl and J. Hubbuch, Chem. Eng. Sci., 2009, 64, 3778–3788 CrossRef CAS.
- D. Wei, M. Wang, H. Wang, G. Liu, J. Fang and Y. Jiang, ACS Omega, 2022, 7, 31338–31347 CrossRef CAS PubMed.
- W. W. Wilson and L. J. Delucas, Acta Crystallogr., Sect. F: Struct. Biol. Commun., 2014, 70, 543–554 CrossRef CAS PubMed.
- L. Hentschel, J. Hansen, S. U. Egelhaaf and F. Platten, Phys. Chem. Chem. Phys., 2021, 23, 2686–2696 RSC.
- B. L. Neal, D. Asthagiri, O. D. Velev, A. M. Lenhoff and E. W. Kaler, J. Cryst. Growth, 1999, 196, 377–387 CrossRef CAS.
- M. Dieterle, T. Blaschke and H. Hasse, Z. Phys. Chem., 2013, 227, 333–343 CrossRef CAS.
- S. Ruppert, S. I. Sandler and A. M. Lenhoff, Biotechnol. Prog., 2001, 17, 182–187 CrossRef CAS PubMed.
- C. M. Mehta, E. T. White and J. D. Litster, Biotechnol. Prog., 2012, 28, 163–170 CrossRef CAS PubMed.
- B. Guo, S. Kao, H. McDonald, A. Asanov, L. L. Combs and W. W. Wilson, J. Cryst. Growth, 1999, 196, 424–433 CrossRef CAS.
- W. W. Wilson, J. Struct. Biol., 2003, 142, 56–65 CrossRef PubMed.
- A. Quigley and D. R. Williams, Eur. J. Pharm. Biopharm., 2015, 96, 282–290 CrossRef CAS PubMed.
- A. Adawy and M. R. Groves, Crystals, 2017, 7, 1–10 CrossRef.
- E. Binabaji, S. Rao and A. L. Zydney, Biotechnol. Bioeng., 2014, 111, 529–536 CrossRef CAS PubMed.
- S. K. Chaturvedi and P. Schuck, AAPS J., 2019, 21, 1–9 CrossRef PubMed.
- A. Saluja, R. M. Fesinmeyer, S. Hogan, D. N. Brems and Y. R. Gokarn, Biophys. J., 2010, 99, 2657–2665 CrossRef CAS PubMed.
- K. K. Arthur, J. P. Cabirelson, B. S. Kendrick and M. R. Stoner, J. Pharm. Sci., 2009, 98, 3522–3539 CrossRef CAS PubMed.
- Y. Liu, X. Wang and C. B. Ching, Cryst. Growth Des., 2010, 10, 548–558 CrossRef CAS.
- E. Pantuso, T. F. Mastropietro, M. L. Briuglia, C. J. J. Gerard, E. Curcio, J. H. ter Horst, F. P. Nicoletta and G. Di Profio, Sci. Rep., 2020, 10, 1–14 CrossRef PubMed.
- P. M. Tessier and A. M. Lenhoff, Curr. Opin. Biotechnol., 2003, 14, 512–516 CrossRef CAS PubMed.
- J. J. Valente, K. S. Verma, M. C. Manning, W. W. Wilson and C. S. Henry, Biophys. J., 2005, 89, 4211–4218 CrossRef CAS PubMed.
- Z. S. Derewenda and P. G. Vekilov, Acta Crystallogr., Sect. D: Biol. Crystallogr., 2006, 62, 116–124 CrossRef PubMed.
- C.-B. Lu, J. Wang and X.-J. Ching, Cryst. Growth Des., 2003, 3, 83–87 CrossRef.
- J. Ferreira, F. Castro, F. Rocha and S. Kuhn, Chem. Eng. Sci., 2018, 191, 232–244 CrossRef CAS.
- E. A. Stura, G. R. Nemerow and I. A. Wilson, J. Cryst. Growth, 1992, 122, 273–285 CrossRef CAS.
- G. M. Whitesides, Nature, 2006, 442, 368–373 CrossRef CAS PubMed.
- S. Haeberle and R. Zengerle, Lab Chip, 2007, 7, 1094–1110 RSC.
- M. O. A. Sommer and S. Larsen, J. Synchrotron Radiat., 2005, 12, 779–785 CrossRef CAS PubMed.
- N. Candoni, R. Grossier, M. Lagaize and S. Veesler, Annu. Rev. Chem. Biomol. Eng., 2019, 10, 59–83 CrossRef PubMed.
- S. Maosoongnern, V. Diaz Borbon, A. E. Flood and J. Ulrich, Ind. Eng. Chem. Res., 2012, 51, 15251–15257 CrossRef CAS.
- M. Ildefonso, N. Candoni and S. Veesler, Cryst. Growth Des., 2011, 11, 1527–1530 CrossRef CAS.
- N. Junius, S. Jaho, Y. Sallaz-Damaz, F. Borel, J. B. Salmon and M. Budayova-Spano, Lab Chip, 2020, 20, 296–310 RSC.
- C. L. Hansen, M. O. A. Sommer and S. R. Quake, Proc. Natl. Acad. Sci. U. S. A., 2004, 101, 14431–14436 CrossRef CAS PubMed.
- M. A. Reus, A. E. D. M. Van Der Heijden and J. H. Ter Horst, Org. Process Res. Dev., 2015, 19, 1004–1011 CrossRef CAS.
- Technobis Group, Technobis Crystallization Systems, https://www.crystallizationsystems.com/applications/solubility/, (accessed 22 June 2023).
- J. H. ter Horst, M. A. Deij and P. W. Cains, Cryst. Growth Des., 2009, 9, 1531–1537 CrossRef CAS.
- M. Oeller, R. Kang, R. Bell, H. Ausserwöger, P. Sormanni and M. Vendruscolo, Briefings Bioinf., 2023, 24, 1–7 CAS.
- M. Hebditch, M. A. Carballo-Amador, S. Charonis, R. Curtis and J. Warwicker, Bioinformatics, 2017, 33, 3098–3100 CrossRef CAS PubMed.
- C. Wang and Q. Zou, BMC Biol., 2023, 21, 1–11 CrossRef PubMed.
- A. Elbasir, R. Mall, K. Kunji, R. Rawi, Z. Islam, G. Y. Chuang, P. R. Kolatkar and H. Bensmail, Bioinformatics, 2020, 36, 1429–1438 CrossRef CAS PubMed.
- H. Wang, L. Feng, G. I. Webb, L. Kurgan, J. Song and D. Lin, Briefings Bioinf., 2018, 19, 838–852 CrossRef CAS PubMed.
-
K. Suzuki, K. Sakakibara, M. Nakamura, S. Shinoda and Y. Asano, in Proceedings of the International Conference on Machine Learning and Cybernetics, IEEE Computer Society, 2022, vol. 2022-September, pp. 237–241 Search PubMed.
- J. Chen, S. Zheng, H. Zhao and Y. Yang, J. Cheminf., 2021, 13, 1–10 CAS.
- X. Han, W. Ning, X. Ma, X. Wang and K. Zhou, Metab. Eng. Commun., 2020, 11, 1–9 Search PubMed.
- X. Han, X. Wang and K. Zhou, Bioinformatics, 2019, 35, 4640–4646 CrossRef CAS PubMed.
- N. Brandes, D. Ofer, Y. Peleg, N. Rappoport and M. Linial, Bioinformatics, 2022, 38, 2102–2110 CrossRef CAS PubMed.
- M. Madani, K. Lin and A. Tarakanova, Int. J. Mol. Sci., 2021, 22, 1–20 CrossRef PubMed.
- Y. Yang, L. Zeng and M. Vihinen, Int. J. Mol. Sci., 2021, 22, 1–15 Search PubMed.
- S. Khurana, R. Rawi, K. Kunji, G.-Y. Chuang, H. Bensmail and R. Mall, Bioinformatics, 2018, 34, 2605–2613 CrossRef CAS PubMed.
|
This journal is © The Royal Society of Chemistry 2023 |