DOI:
10.1039/D2LC00486K
(Communication)
Lab Chip, 2022,
22, 2938-2943
Selection and characterisation of bioreceptors to develop nanoparticle-based lateral-flow immunoassays in the context of the SARS-CoV-2 outbreak†
Received
27th May 2022
, Accepted 10th June 2022
First published on 29th July 2022
Abstract
This manuscript aims at raising the attention of the scientific community to the need for better characterised bioreceptors for fast development of point-of-care diagnostic devices able to support mass frequency testing. Particularly, we present the difficulties encountered in finding suitable antibodies for the development of a lateral flow assay for detecting the nucleoprotein of SARS-CoV-2.
Introduction
The COVID-19 pandemic1–3 has shown the importance of developing reliable yet easy-to-use, cheap, fast, and portable diagnostic devices to support mass testing.4–10 Diagnostic testing is fundamental for a rapid screening of the population, to identify and track positive cases (i.e. both symptomatic and asymptomatic individuals), and immunity assessment.11,12 As suggested by the World Health Organization (WHO), in order to meet such a high demand of testing, countries have been relying on lateral flow assays (LFAs).13 Indeed, such molecular sensing platforms allow achieving the rapid (<30 min), low-cost (5 USD), and single-step detection of the COVID-19 biomarkers.14 Moreover, LFAs are an evolving platform with constantly improving sensitivity.15–17 Our group recently provided a protocol describing the fabrication of a LFA to detect human IgG.18 This is a generalisable protocol that can be easily adapted to other targets, such as the SARS-CoV-2 virus, only by changing the bioreceptors (e.g. antibodies or aptamers). However, the selection of suitable bioreceptors for the detection of SARS-CoV-2 unveiled several experimental hurdles, which we want to share with the community. More specifically, we want to raise attention towards the importance of comprehensive characterisation of bioreceptors (in this case, antibodies) before their implementation into LFAs. Many studies compare the performance of commercial SARS-CoV-2 diagnostic kits,19–26 but to the best of our knowledge, only one recent work has focused on the technical challenges behind bioreceptor selection.27
The COVID-19 pandemic represents a unique situation due to the scarcity of antibodies against SARS-CoV-2 antigens at the beginning of the outbreak (from December 2019 to May 2020). During this period, the research community made an important effort in the characterisation process to select suitable antibodies to rapidly face the fast spread of the virus. The main goal of this characterisation is to understand whether the selected antibodies exhibit the required binding properties to work in a LFA platform. Specifically, antibodies have to display: (1) stability, in order to work under variable environmental conditions (temperature, humidity, pressure) and support long-term storage; (2) fast binding kinetics, due to the short time window for the bioreceptor–analyte interaction in the LFA assay (in the range of seconds to a few minutes); (3) strong binding affinity, as we want the signal to remain stable while and after the assay takes place.18 Unfortunately, besides already identified technical problems associated with antibodies such as batch to batch differences,28–31 suppliers do not provide enough characterisation of important binding parameters (e.g. binding and kinetic constants), and they test antibodies using only standard laboratory procedures (e.g., ELISA, western blot). A parallel approach could be the estimation of these parameters through thermodynamics, binding, and kinetics studies, but this requires resources, time, and facilities that private companies may not be willing to implement.18 This lack of experimental data does not allow developers to pursue a rational selection of antibodies forcing them into a trial-and-error approach. Since this can hide many technical challenges, here we present a study which describes how to proceed in such circumstances with experimental tools that are more available and can be performed by any laboratory, always keeping in mind that the final goal is the development of a colorimetric LFA to be implemented in COVID-19 diagnostics, and considering that similar scenarios can occur in the future.
Results and discussion
Following our fabrication protocol for LFA,18 we characterised antibodies for their adaptation as bioreceptors in an AuNP-based LFA as they were released in the market. Specifically, among the antigens of SARS-CoV-2, we decided to target the nucleoprotein since it is highly abundant in the virion.32,33 From April 2020 to February 2021, we purchased 17 commercial anti-nucleoprotein antibodies from different companies (see Table S1†). The criteria for the selection were: (1) cost, (2) antibody concentration (over 1 mg mL−1), (3) delivery time, and (4) animal host used for its production. Since the recognition event in LFAs is based on the formation of the classic immune-sandwich complex (Fig. S3†), we screened antibodies through two phases: (1) ELISA, to quickly check antibody binding performance (Fig. 1) and (2) the half-stick format, to check their compatibility with the conditions encountered in a LFA (i.e., under a constant flow in a nitrocellulose membrane) (Fig. 2). Firstly, we performed ELISA tests to identify the antibody combinations with the best binding performance (Fig. 1A). In order to carry out these measurements, we followed a previously reported ELISA protocol.34,35 The experimental criteria to identify such antibodies were similar to the standard for evaluating antibody titre by the chessboard titration method, which allows assessing two variables simultaneously: antibody couples and the presence/absence of nucleoprotein.36 Specifically, in the absence of the target (i.e. blank), the background signal has to display a value lower than 0.2 a.u. (OD Blank), while in the presence of a saturated concentration of nucleoprotein (100 ng mL−1), the produced signal should be higher than 1.0 a.u. (OD Positive). Surprisingly, we found that out of 80 tested combinations, only 10 met these two criteria (Fig. 1B). Such a low success rate (12.5%) is a consequence of the high cross-reactivity between antibodies (in most cases, a blank signal as high as the positive can be observed (Fig. 1C)) and their low affinity for the target or slow kinetics (for antibody couples whose positive signals were lower than 1.0 a.u.) (Fig. 1D and E). It should be noted that all but one antibodies were publicised to work in ELISA and that none of them were part of a pre-validated matched pair.
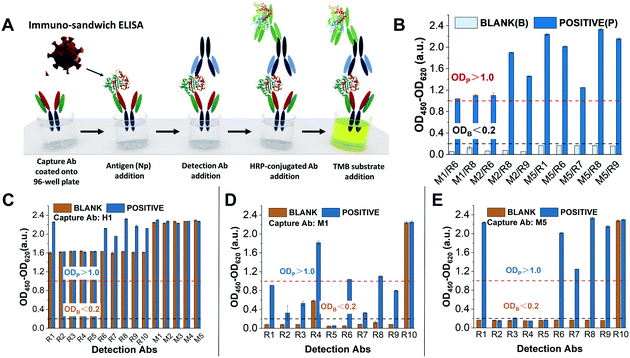 |
| Fig. 1 ELISA tests were performed to screen antibody combinations with the best binding performance against the nucleoprotein (N protein) of SARS-CoV-2. (A) Schematic of the immune-sandwich ELISA procedure. (B) Antibody combinations with the best ELISA performance. The nucleoprotein concentrations of the blank and positive solutions are 0 ng mL−1 and 100 ng mL−1, respectively. Antibody combinations that meet the performance requirements should have the following conditions: the OD value of the blank nucleoprotein solution is less than 0.2 a.u. and the OD value of the positive nucleoprotein solution is greater than 1.0 a.u.27 (C–E) Each individual graph shows the ELISA test results of the same capture antibodies (coated on ELISA plate wells) combined with multiple detection antibodies. (C) Capture Abs H1 and detection Abs R1–R10 and M1/M5. None of the antibody combinations qualified because the OD value of the blanks was much greater than 0.2, which means high cross-reactivity and poor specificity; (D) capture Abs M1 and detection Abs R1–R1. Only M1/R6 and M1/R8 were qualified with OD blank <0.2 and OD positive >1.0 while the rest were not qualified due to OD blank >0.2 or OD positive <1.0; and (E) capture Abs M5 and detection Abs R1/R10. Couples M5/R1, M5/R6, M5/R7, M5/R8 and M5/R9 were satisfactory, especially M5/R1 and M5/R8, and the others were not qualified due to OD blank >0.2 (M5/R10) or OD positive <1.0. | |
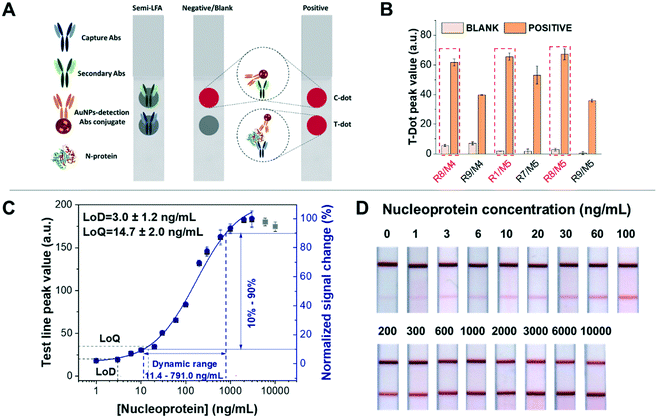 |
| Fig. 2 Dot test for screening out antibody combinations and calibration curve of detection of nucleoprotein spiked in artificial saliva based on half-stick LFA. (A) Schematic representation of the dot test based on half-stick LFA: anti-nucleoprotein Abs (capture Abs) and secondary Abs were manually dropped on the nitrocellulose membrane as the test dot and control dot, respectively. When the half-stick is tested with a blank sample, only the control dot (or line) is visible, demonstrating that the assay functioned properly. If the sample contains nucleoproteins, both control and test dots (or lines) are visible, and the intensity of the latter will depend on the analyte concentration. (B) All antibody combinations with good performance in the dot test (easy to distinguish between blank and positive samples with the naked eye). R1/M5, R8/M4 and R8/M5 elicit the best response. (C) Calibration curve of the half-stick LFA with R8/M5 antibodies. The data were fitted to a four-parameter logistic curve (blue line). The fitted curve (obtained using Origin 2018 32-bit and presented as value ± standard error) corresponds to the following equation: y = start + (end − start) × x^n/(k^n + x^n), with start = 1.04 ± 1.09, end = 112.12 ± 10.21, k = 169.28 ± 44.06 and n = 0.90 ± 0.12. The reduced χ2 = 2.58, R2 = 1.00 and the adjusted R2 = 0.99 Grey points were beyond the curve range due to the saturation of bioreceptor binding sites. The dynamic range of the half-sticks is from 11.4 ng mL−1 to 791.0 ng mL−1, calculated from the signal change from 10% to 90%, and the graph was obtained by analysing at least three (n = 3) independent LFAs for each target concentration. The fitting curve, LoD, LoQ, and dynamic range fully were acquired by the protocol from Parolo et al.18 (D) Half-stick LFA for detection of nucleoprotein, responding to increasing concentrations of the nucleoprotein. | |
Among the 10 working antibody combinations, we selected the 5 combinations of capture and detection antibodies (M2/R8, M5/R1, M5/R6, M5/R8 and M5/R9) with the best performance in order to move on to the half-stick characterisation. To do this, we used dotted half-sticks rather than full LFA strips because they are faster to prepare (taking into account the number of antibody combinations) and smaller reagent amounts are required.18,37 In order to identify the antibodies with the best binding performance in the nitrocellulose membrane, we established that the blank's signal should be <5.0 a.u. and the positive sample's signal >30.0 a.u. These values approximately correspond with the dynamic range obtained in a provided protocol for human IgG detection.18 With these criteria, only 6 couples of antibodies showed a suitable binding performance in the half-stick format (Fig. 2B). We hypothesise that the shorter time of incubation/recognition and the absence of washing steps in half-stick dot tests compared to ELISAs are the causes of the decrease in the number of antibody couples compatible with a paper-based platform.
To demonstrate the feasibility of the half-stick assay for further experimentation with clinical samples, we tested it using artificial saliva, taking into account that COVID-19 diagnosis is possible using such a kind of sample.4,38 The dot test results showed that three antibody couples (R1/M5, R8/M4 and R8/M5) had similar test line peak values for the detection of 100 ng mL−1 nucleoprotein (Fig. 2B). Then, preliminary calibration curves of R1/M5, R8/M4 and R8/M5 were obtained after testing the sensors with increasing concentrations of nucleoprotein (0, 3, 10, 30, 100, 300, 1000, and 3000 ng mL−1) (Fig. S5†). Fitting the curves with a four-logistic parameter equation, we calculated the EC50, that is, the half maximal concentration of nucleoprotein that elicits a response halfway between the baseline and saturation signal. Given the immune-sandwich nature of the system, EC50 is a good indicator of affinity. The R8/M5 couple displays the lowest EC50 value (134.7 ng mL−1), while the EC50 values for R8/M4 and R1/M5 are 197.9 ng mL−1 and 337.1 ng mL−1 respectively (Fig. S5 and Table S4†). Moreover, R8/M5 showed the best sensitivity for nucleoprotein detection, as observed from the steeper slope in the calibration curves (Fig. S5A and B†). Consequently, antibody couple R8/M5 was selected for the further development of a nucleoprotein LFA sensor. A half-stick was prepared by dispensing the R8 antibody on the test line (TL) and the secondary antibodies (anti-mouse IgG) on the control line (CL), while the antibody nucleoprotein M5 was conjugated to AuNPs. In order to characterise the sensor response, we challenged it using nucleoprotein-spiked artificial saliva samples covering a nucleoprotein concentration range between 1 ng mL−1 (21.2 pM) and 10 μg mL−1 (0.2 μM) (Fig. 2C). Through the analysis of the obtained half-sticks, we calculated a limit of detection (LoD) of 3.0 ± 1.2 ng mL−1, limit of quantification (LoQ) of 14.7 ± 2.0 ng mL−1, and a useful dynamic range of 11.4–791.0 ng mL−1 (Fig. 2C). The test line signals obtained using nucleoprotein concentrations higher than 10 ng mL−1 were clearly seen by the naked eye (Fig. 2D). In addition, the recovery of nucleoprotein samples ranged from 83.0 ± 2.0% to 116.7 ± 16.7%, demonstrating the accuracy of the test (Table S2†). The analytical performance of the described half-sticks is comparable to that of a full SARS-CoV-2 nucleoprotein LFA recently published for the detection of the nucleoprotein.39 To further support our results, we found another recently published study by Cate et al., in which 1021 anti-nucleoprotein antibodies were tested in LFA, taking advantage of an automatized, high-throughput robotic system,27 and the couple of antibodies we independently selected has been found among the top performing couples.
Finally, to make stronger our message that knowing beforehand the binding behaviour of bioreceptors would speed up the development of diagnostic tests, we studied the binding constant of three couples of antibodies selected according to their performance in the preliminary steps, which goes as follows. One of them worked in ELISA but did not in half-stick (R8/M2), while the other two couples did work in both types of assays (R8/M4 and R8/M5). R8/M2 showed an EC50 at least 72% higher than those of the other two couples (R8/M4 and R8/M5) and also a higher working range, thus indicating a lower affinity, which is not suitable for LFAs (Table S3 and Fig. S4†).
Conclusions
Despite the successful implementation of the LFA development protocol,18 the significant amount of invested economic resources (∼25
000 €), personnel, and time (over 10 months) to identify working antibodies is alarming. We understand that the majority of the antibodies are validated for a few specific applications, generally traditional laboratory-bound techniques (e.g. ELISA, western blot). However, the COVID-19 pandemic has demonstrated that we cannot rely exclusively on long (hours) and cumbersome (multistep) diagnostic techniques to effectively diagnose infectious diseases because we need sensing platforms able to support massive (or high-frequency) testing. Therefore, we urge antibody producers and distributors to consider the implementation of more extensive characterisation of their products, which would allow researchers to make better-informed purchases. At the same time, the integration of new antibodies into point-of-care devices would also be faster. The availability of information such as the binding kinetics of bioreceptors could lead researchers towards purchasing antibodies that better suit their platform's needs, e.g. antibodies with fast binding kinetics for a LFA, which features a short receptor–analyte interaction time. We realise that longer bioreceptor characterisation implies higher costs for the company (e.g. new instrumentation, delayed commercial availability). Nonetheless, we truly believe that researchers would rather buy more expensive, but well characterised antibodies than cheaper but poorly characterised ones. This in turn would optimise the time and economic resources required for the development of a point-of-care diagnostic device, speeding up its placement in the market.
Author contributions
LH: conceptualisation, investigation, formal analysis, software, and writing – original draft. EC: conceptualisation, investigation, and writing – original draft. CFC: investigation and writing – review and editing. CP: methodology, data curation, supervision, and writing – review and editing. AI: supervision and writing – review and editing. RA: software, data curation, and supervision. LR: supervision and writing – review and editing. AM: project administration, funding acquisition, and supervision.
Conflicts of interest
There are no conflicts to declare.
Acknowledgements
We acknowledge Consejo Superior de Investigaciones Científicas (CSIC) for the project “COVID19-122” granted in the call “Nuevas ayudas extraordinarias a proyectos de investigación en el marco de las medidas urgentes extraordinarias para hacer frente al impacto económico y social del COVID-19 (Ayudas CSIC-COVID-19)”. We acknowledge also the MICROB-PREDICT project that has received funding from the European Union's Horizon 2020 research and innovation programme under grant agreement No. 825694. Financial support from the EU Graphene Flagship Core 3 Project (No. 881603) is also acknowledged. This article reflects only the authors' view, and the European Commission is not responsible for any use that may be made of the information it contains. ICN2 is funded by the CERCA programme/Generalitat de Catalunya. ICN2 is supported by the Severo Ochoa Centres of Excellence programme, funded by the Spanish Research Agency (AEI, grant no. SEV-2017-0706). E. C. acknowledges Ministerio de Ciencia e Innovación of Spain and Fondo Social Europeo for the Fellowship PRE2018-084856 awarded under the call ‘Ayudas para contratos predoctorales para la formación de doctores, Subprograma Estatal de Formación del Programa Estatal de Promoción del Talento y su Empleabilidad en I+D+i’, under the framework of ‘Plan Estatal de Investigación Científica y Técnica y de Innovación 2017–2020’. L. H. acknowledges the China Scholarship Council. L. H., E. C. and C. F.-C. acknowledge the Autonomous University of Barcelona (UAB) for their support. C. P. (ISGlobal) also acknowledges support from the Spanish Ministry of Science and Innovation and State Research Agency through the “Centro de Excelencia Severo Ochoa 2019–2023” Program (CEX2018-000806-S), and support from the Generalitat de Catalunya through the CERCA Program. A. I. was supported by a PROBIST postdoctoral fellowship funded by the European Research Council (Marie Skłodowska-Curie grant agreement No. 754510).
Notes and references
- F. Wu, S. Zhao, B. Yu, Y. M. Chen, W. Wang, Z. G. Song, Y. Hu, Z. W. Tao, J. H. Tian, Y. Y. Pei, M. L. Yuan, Y. L. Zhang, F. H. Dai, Y. Liu, Q. M. Wang, J. J. Zheng, L. Xu, E. C. Holmes and Y. Z. Zhang, Nature, 2020, 579, 265–269 CrossRef CAS PubMed.
- P. Zhou, X. Yang, X. Wang, B. Hu, L. Zhang, W. Zhang, H. Guo, R. Jiang, M. Liu, Y. Chen, X. Shen, X. Wang, F. Zhan, Y. Wang, G. Xiao and Z. Shi, Nature, 2020, 579, 270–273 CrossRef CAS PubMed.
- J. Lan, J. Ge, J. Yu, S. Shan, H. Zhou, S. Fan and Q. Zhang, Nature, 2020, 581, 215–220 CrossRef CAS PubMed.
- M. Nagura-Ikeda, K. Imai, S. Tabata, K. Miyoshi, N. Murahara, T. Mizuno, M. Horiuchi, K. Kato, Y. Imoto, M. Iwata, S. Mimura, T. Ito, K. Tamura and Y. Kato, J. Clin. Microbiol., 2020, 58, 1–9 CrossRef PubMed.
- C. R. Wells, J. P. Townsend, A. Pandey, S. M. Moghadas, G. Krieger, B. Singer, R. H. McDonald, M. C. Fitzpatrick and A. P. Galvani, Nat. Commun., 2021, 12, 1–9 CrossRef PubMed.
- G. Guglielmi, Nature, 2020, 585, 496 CrossRef CAS PubMed.
- T. R. Mercer and M. Salit, Nat. Rev. Genet., 2021, 22, 415–422 CrossRef CAS PubMed.
- G. Rosati, A. Idili, C. Parolo, C. Fuentes-Chust, E. Calucho, L. Hu, C. D. C. C. E. Silva, L. Rivas, E. P. Nguyen, J. F. Bergua, R. Alvárez-Diduk, J. Muñoz, C. Junot, O. Penon, D. Monferrer, E. Delamarche, A. Merkoçi, A. G. Rosati, A. Idili, C. Parolo, C. Fuentes, E. Calucho, L. Hu and C. D. C. Castro, ACS Nano, 2021, 15, 17137–17149 CrossRef CAS PubMed.
- A. Idili, C. Parolo, R. Alvarez-Diduk and A. Merkoçi, ACS Sens., 2021, 6, 3093–3101 CrossRef CAS PubMed.
- D. B. Larremore, B. Wilder, E. Lester, S. Shehata, J. M. Burke, J. A. Hay, M. Tambe, M. J. Mina and R. Parker, Sci. Adv., 2021, 7, eabd5393 CrossRef CAS PubMed.
- L. J. Carter, L. V. Garner, J. W. Smoot, Y. Li, Q. Zhou, C. J. Saveson, J. M. Sasso, A. C. Gregg, D. J. Soares, T. R. Beskid, S. R. Jervey and C. Liu, ACS Cent. Sci., 2020, 591–605 CrossRef CAS PubMed.
- O. Vandenberg, D. Martiny, O. Rochas, A. van Belkum and Z. Kozlakidis, Nat. Rev. Microbiol., 2021, 19, 171–183 CrossRef CAS PubMed.
- World Health Organization (WHO), Antigen-detection in the diagnosis of SARS-CoV-2 infection using rapid immunoassays Interim guidance, 11 September 2020, 2020.
- G. Guglielmi, Nature, 2021, 590, 202–205 CrossRef CAS PubMed.
- B. S. B. S. Miller, L. Bezinge, H. D. H. D. Gliddon, D. Da Huang, G. Dold, E. R. Gray, J. Heaney, P. J. Dobson, E. Nastouli, J. J. L. L. Morton and R. A. McKendry, Nature, 2020, 587, 588–593 CrossRef CAS PubMed.
- A. Sena-Torralba, R. Alvarez-Diduk, C. Parolo, H. Torné-Morató, A. Müller and A. Merkoçi, Anal. Chem., 2021, 93, 3112–3212 CrossRef CAS PubMed.
- A. Sena-Torralba, H. Torné-Morató, C. Parolo, S. Ranjbar, M. A. Farahmand Nejad, R. Álvarez-Diduk, A. Idili, M. R. Hormozi-Nezhad and A. Merkoçi, Adv. Mater. Technol., 2022, 2101450 CrossRef.
- C. Parolo, A. Sena-Torralba, J. F. Bergua, E. Calucho, C. Fuentes-Chust, L. Hu, L. Rivas, R. Álvarez-Diduk, E. P. Nguyen, S. Cinti, D. Quesada-González and A. Merkoçi, Nat. Protoc., 2020, 15, 3788–3816 CrossRef CAS PubMed.
- V. Haselmann, M. Kittel, C. Gerhards, M. Thiaucourt, R. Eichner, V. Costina and M. Neumaier, Clin. Chim. Acta, 2020, 510, 73–78 CrossRef CAS PubMed.
- S. Mahapatra and P. Chandra, Biosens. Bioelectron., 2020, 165, 112361 CrossRef CAS PubMed.
- C. L. Charlton, J. N. Kanji, K. Johal, A. Bailey, S. S. Plitt, C. Macdonald, A. Kunst, E. Buss, L. E. Burnes, K. Fonseca, B. M. Berenger, K. Schnabl and J. Hu, J. Clin. Microbiol., 2020, 58, e01361–20 CrossRef CAS PubMed.
- M. Martínez, D. Navalpotro, N. Tormo, R. Olmos, M. Moreno, M. Dolores, O. Mochón and C. Gimeno, J. Clin. Virol., 2020, 129, 104529 CrossRef PubMed.
- C. Schnurra, N. Reiners, R. Biemann, T. Kaiser, H. Trawinski and C. Jassoy, J. Clin. Virol., 2020, 129, 104544 CrossRef CAS PubMed.
- A. Krüttgen, C. G. Cornelissen, M. Dreher, M. Hornef, M. Imöhl and M. Kleines, J. Clin. Virol., 2020, 128, 104394 CrossRef PubMed.
- K. Y. L. Chua, S. Vogrin, I. Bittar, J. H. Horvath, H. Wimaleswaran, J. A. Trubiano, N. E. Holmes and Q. Lam, Pathology, 2020, 52, 778–782 CrossRef CAS PubMed.
- M.-A. Trabaud, V. Icard, M.-P. Milon, A. Bal, B. Lina and V. Escuret, J. Clin. Virol., 2020, 132, 104613 CrossRef CAS PubMed.
- D. M. Cate, J. D. Bishop, H. V. Hsieh, V. A. Glukhova, L. F. Alonzo, H. G. Hermansky, B. Barrios-Lopez, B. D. Grant, C. E. Anderson, E. Spencer, S. Kuhn, R. Gallagher, R. Rivera, C. Bennett, S. A. Byrnes, J. T. Connelly, P. K. Dewan, D. S. Boyle, B. H. Weigl and K. P. Nichols, ACS Omega, 2021, 6, 25116–25123 CrossRef CAS PubMed.
- M. Baker, Nature, 2015, 521, 274–276 CrossRef CAS PubMed.
- A. Bradbury and A. Plückthun, Nature, 2015, 518, 27–29 CrossRef CAS PubMed.
- J. R. Couchman, J. Histochem. Cytochem., 2009, 57, 7–8 CrossRef CAS PubMed.
- C. B. Saper, J. Comp. Neurol., 2005, 493, 477–478 CrossRef PubMed.
- J. Cubuk, J. J. Alston, J. J. Incicco, S. Singh, M. D. Stuchell-Brereton, M. D. Ward, M. I. Zimmerman, N. Vithani, D. Griffith, J. A. Wagoner, G. R. Bowman, K. B. Hall, A. Soranno and A. S. Holehouse, Nat. Commun., 2021, 12, 1–17 CrossRef PubMed.
- W. Zeng, G. Liu, H. Ma, D. Zhao, Y. Yang, M. Liu, A. Mohammed, C. Zhao, Y. Yang, J. Xie, C. Ding, X. Ma, J. Weng, Y. Gao, H. He and T. Jin, Biochem. Biophys. Res. Commun., 2020, 527, 618–623 CrossRef CAS PubMed.
- E. Morales-Narváez, H. Montón, A. Fomicheva and A. Merkoçi, Anal. Chem., 2012, 84, 6821–6827 CrossRef PubMed.
- J. F. Bergua, R. Álvarez-Diduk, A. Idili, C. Parolo, M. Maymó, L. Hu and A. Merkoçi, Anal. Chem., 2022, 94, 1271–1285 CrossRef CAS PubMed.
- China National Intellectual Property Administration, CN102876634A, 2012, 1–21.
- H. V. Hsieh, J. L. Dantzler and B. H. Weigl, Diagnostics, 2017, 7, 29 CrossRef CAS PubMed.
- D. Shan, J. M. Johnson, S. C. Fernandes, H. Suib, S. Hwang, D. Wuel, M. Mendes, M. Holdridge, E. M. Burke, K. Beauregard, Y. Zhang, M. Cleary, S. Xu, X. Yao, P. P. Patel, T. Plavina, D. H. Wilson, L. Chang, K. M. Kaiser, J. Nattermann, S. V. Schmidt, E. Latz, K. Hrusovsky, D. Mattoon and A. J. Ball, Nat. Commun., 2021, 12, 1–8 CrossRef PubMed.
- B. D. Grant, C. E. Anderson, J. R. Williford, L. F. Alonzo, V. A. Glukhova, D. S. Boyle, B. H. Weigl and K. P. Nichols, Anal. Chem., 2020, 92, 11305–11309 CrossRef CAS PubMed.
Footnotes |
† Electronic supplementary information (ESI) available: Extended materials and methods, bioreceptor selection and characterisation data (PDF). See DOI: https://doi.org/10.1039/d2lc00486k |
‡ These authors contributed equally. |
|
This journal is © The Royal Society of Chemistry 2022 |