DOI:
10.1039/D1NR03691B
(Paper)
Nanoscale, 2021,
13, 17340-17349
A digital SERS sensing platform using 3D nanolaminate plasmonic crystals coupled with Au nanoparticles for accurate quantitative detection of dopamine†
Received
8th June 2021
, Accepted 7th September 2021
First published on 7th September 2021
Abstract
We report a digital surface-enhanced Raman spectroscopy (SERS) sensing platform using the arrays of 3D nanolaminate plasmonic crystals (NLPC) coupled with Au nanoparticles and digital (on/off) SERS signal analysis for the accurate quantitative detection of dopamine (DA) at ultralow concentrations. 3D NLPC SERS substrates were fabricated to support the optically dense arrays of vertically-stacked multi-nanogap hotspots and combined with Raman tag-conjugated Au nanoparticles for NLPC-based dual-recognition structures. We demonstrate that the 3D NLPC-based dual-recognition structures including Au nanoparticle-induced additional hotspots can enable more effective SERS enhancement through the molecular recognition of DA. For the accurate quantification of DA at ultralow concentrations, we conducted digital SERS analysis to reduce stochastic signal variation due to various microscopic effects, including molecular orientation/position variation and the spatial distribution of nanoparticle-coupled hotspots. The digital SERS analysis allowed the SERS mapping results from the DA-specific dual-recognition structures to be converted into binary “On/Off” states; the number of “On” events was directly correlated with low-abundance DA molecules down to 1 pM. Therefore, the digital SERS platform using the 3D NLPC-based dual-recognition structures coupled with Au nanoparticles and digital SERS signal analysis can be used not only for the ultrasensitive, accurate, and quantitative determination of DA, but also for the practical and rapid analysis of various molecules on nanostructured surfaces.
Introduction
Dopamine (DA) is one of the most important neurotransmitters, playing critical roles in various physiological and brain activities.1 DA has been considered a crucial biomarker in medical diagnostics because abnormal DA levels have been observed in patients suffering from diverse neurological disorders such as Parkinson's disease, schizophrenia, attention deficit hyperactivity disorder, Huntington's disease, and epilepsy.2–4 For example, patients with Parkinson's disease show a significantly lower level of DA and other catecholamines in their cerebrospinal fluids (CSF).5 In addition to its neural communication role, DA is also involved in immunoregulation, modulating the metabolism and the immune system.6 Therefore, the quantitative determination of DA is crucial, and the need for a highly sensitive and accurate DA sensing platform has increased greatly.
Although various DA detection modalities have been developed, including electrochemistry,7,8 chromatography,9,10 chemiluminescence,11,12 fluorescence,13–15 surface plasmon resonance,16 and plasmonic colorimetry,17,18 the majority of DA sensing platforms can only reliably measure DA down to the nanomolar range, which is not sufficient for the diagnosis of neurological disorders requiring a sensitivity down to the tens of picomolar range.5 Recently, several sensors have demonstrated improved sensitivity down to 1 pM.8,12,16 Of these, electrochemical sensors are one of the most well-established techniques due to their high sensitivity and rapid detection.8,12 However, this approach suffers from low selectivity because of the presence of electroactive interferents in extracellular fluids such as ascorbic acid and uric acid, which are oxidized by a very similar potential to that for DA.
Surface-enhanced Raman spectroscopy (SERS) has emerged as a promising biochemical detection technique, providing molecular fingerprint information with a rapid, non-destructive, and ultrasensitive detection capability down to the single-molecule level.19–27 SERS exploits the surface plasmon enhancement of both the excitation and inelastic Raman scattering processes of molecules at plasmonic hotspots, dramatically amplifying intrinsically weak Raman scattering signals by several orders of magnitude.19 Although various label-free SERS platforms have been used to detect DA in extracellular physiological fluids, the label-free SERS approach still faces significant challenges in diagnostic applications due to its lack of specificity and low sensitivity.28–32 For mitigating these issues, sample pre-treatment and chemical functionalization have been used to selectively extract DA from extracellular physiological fluids and specifically capture DA.33–35 Despite the notable improvement, reliable DA sensing using label-free SERS still remains at the nanomolar level because DA molecules have a weak Raman scattering cross-section.36 Furthermore, the poorly resolved peaks make rigorous quantitative analysis more challenging. As an alternative approach, label-based SERS sensing using Raman tags has been reported for DA detection, using, for example, complementary DNA combined with aptamers or immunoassays.37,38 Although picomolar levels of DA have been successfully detected, DNA hybridization is costly and time-consuming and has a low throughput, making it less efficient for diagnostic applications. In addition, nanoparticle aggregation in colloidal solutions generally causes poor reproducibility, and destructive chemical processes after the capture of DA may lead to inaccurate quantification of the target molecules.20
For SERS-based sensing, including both label-free and label-based SERS, reliable quantitative SERS analysis of target analytes at ultralow concentrations has been challenging because of the stochastic nature involved in SERS-based sensing.39–41 The stochastic property, originating from the spatial non-uniformity of hotspot distributions and temporal molecule adsorption dynamics, leads to fluctuations in the SERS intensity. Although capturing target molecules via suitable chemical functionalization can mitigate the dynamic movement of molecules in the vicinity of SERS hotspots, the measured SERS intensity is still subject to variation in the molecular position and orientation, reducing the accuracy of a quantitative readout. Therefore, a method that can reduce signal variation is required for the more reliable quantitative determination of target molecules.
Herein, we present a digital SERS sensing platform using the arrays of 3D nanolaminate plasmonic crystals (NLPC) coupled with Au nanoparticles (AuNPs) and digital (on/off) SERS signal analysis for the accurate quantitative detection of DA, down to 1 pM. The arrays of 3D NLPC as a scalable 3D SERS substrate consist of optically dense arrays of 3D nanogap hotspots based on vertically stacked metal–insulator–metal (MIM) nanostructures, which can independently provide substantial local field enhancement under near-infrared (NIR) excitation. To specifically target DA using a dual-recognition-based sandwich structure, we employ two different reagents that can readily bind to two terminals (diol and amine) of DA molecules: 3,3′-dithiodipropionic acid di(N-hydroxysuccinimide ester) (DSP) for 3D NLPC SERS substrates and 3-mercaptophenylboronic acid (3-MPBA) for AuNPs. Both the DSP-functionalized 3D NLPC SERS substrate and the 3-MPBA-modified AuNPs are exploited to create additional hotspots by binding the 3-MPBA-AuNPs to the existing nanogap hotspots of the 3D NLPC SERS substrate in the presence of DA molecules to induce further SERS enhancement, thus enabling highly sensitive SERS sensing. For the accurate quantitative determination of DA, we perform digital SERS analysis to reduce the signal fluctuations from different microscopic sources by converting the SERS intensities into binary “On/Off” responses. Recently, a proof-of-concept of the digital SERS assay has been reported using two common non-resonant molecular probes with multivariate resolution curve fitting.42 Compared to the absolute SERS intensity, the digitalization of molecule adsorption events shows more accurate quantitative SERS analysis. In this work, this digital SERS analysis shows that the count of the dual-recognition events over SERS mapping images is proportional to the DA concentration. Furthermore, the soft lithography-based fabrication technique enables the wafer-scale and cost-effective mass production of the 3D NLPC SERS substrate. In addition, the all-gold-based substrates offer long-term storage stability, and NIR excitation reduces autofluorescence from other molecules in extracellular physiological fluids.20 Therefore, the digital SERS platform using the 3D NLPC-based dual-recognition structures coupled with AuNPs and digital SERS signal analysis can be used as an ultrasensitive and practical analytical tool for the accurate quantitative determination of the target molecules on nanostructured surfaces.
Experimental section
Materials and reagents
3,3′-Dithiodipropionic acid di(N-hydroxysuccinimide ester) (DSP), dimethyl sulfoxide (DMSO), and sodium hydroxide (NaOH) were purchased from Sigma Aldrich (St. Louis, MO, USA). 3-Hydroxytyramine (DA) hydrochloride and 3-mercaptophenylboronic acid (3-MPBA) were obtained from TCI (Tokyo, Japan). Different concentrations of DA solution were prepared with artificial CSF (aCSF) to mimic clinical samples. The aCSF at pH 7.4 was prepared by following the protocol from Abcam (https://www.abcam.com/protocols/brain-slice-electrophysiology-video-protocol). Colloidal AuNPs with a diameter of 10 nm were purchased from BBI Solutions (Crumlin, UK). Deionized (DI) water with a resistivity of 18.3 MΩ-cm was used in the experiments.
Fabrication of 3D NLPC SERS substrates
Fig. 1A illustrates the fabrication process for a 3D NLPC SERS substrate. To create periodic nanopillar arrays, we first prepared a composite polydimethylsiloxane (PDMS) stamp patterned with a nanohole array (diameter = 150 nm, periodicity = 400 nm, and height = 400 nm) by soft lithography.43 The composite PDMS stamp was replicated from a nanopillar-patterned silicon master, prepared initially by laser interference lithography. It has two layers of PDMS, where the bottom layer is a stiffer one called hard-PDMS. The hard-PDMS was spin-coated onto the silane-coated silicon master and cured for 10 minutes at 60 °C. Liquid PDMS was then poured on the hard-PDMS and cured for 4 hours. UV-curable polyurethane (PU) (NOA61, Norland Product Inc., USA) on a 3′′ silicon wafer was solidified against the PDMS stamp via UV curing for 10 min and subsequent heat curing in a convection oven at 80 °C overnight. To create the multiple MIM building blocks on the nanopillar arrays, we deposited four 30 nm-thick Au layers and three SiO2 layers with thicknesses of 12, 14, and 16 nm (from bottom to top), respectively, in an alternating way using an electron-beam evaporator (PVD250, Kurt J. Lesker Company, USA). The thicknesses of the metal and dielectric layers were determined by considering two factors: (i) to allow the placement of 10 nm diameter AuNPs inside the nanogaps and (ii) to generate multiple plasmon resonances from the visible to the NIR range, which was based on our previous studies that the thickness of the dielectric layer in nanolaminated structures dictates the individual plasmon resonance wavelength. Specifically, the plasmon resonance wavelength blue shifts as the insulator thickness increases due to the weakened coupling between two metal nanodisks, and we used three different thicknesses for the SiO2 layers to avoid the anti-crossing behavior.44 As adhesion layers, a 1 nm-thick Cr layer was deposited onto the polymer pillar arrays before the alternating MIM deposition, and a 1 nm-thick Ti layer was deposited on every metal–dielectric interface. We chose Ti based on its compatibility with the subsequent wet-etching process using a buffered oxide etchant (BOE). To open the embedded nanogaps, we used 10
:
1 BOE (Transene Inc., USA) for 10 s to partially etch the SiO2 layers so that the molecules and AuNPs could access the nanogap hotspot regions. The opening of dielectric nanogap hotspots in vertically stacked MIM nanostructures has been reported to increase the SERS enhancement factor by up to one order of magnitude.45,46Fig. 1B shows a photographic image of a 3D NLPC SERS substrate on a 3′′ silicon wafer, highlighting its large size and the vivid light diffraction pattern from the uniform distribution of periodic MIM nanopillar arrays. The periodic structure was further confirmed using a top-view scanning electron microscopy (SEM) (FEI Helios 600 Nanolab Dual-beam, USA) image of the 3D NLPC SERS substrate in Fig. 1C. The soft-lithography-based fabrication allows for the cost-effective and high-throughput mass production of wafer-scale 3D NLPC SERS substrates, which is desirable for biological and clinical applications.
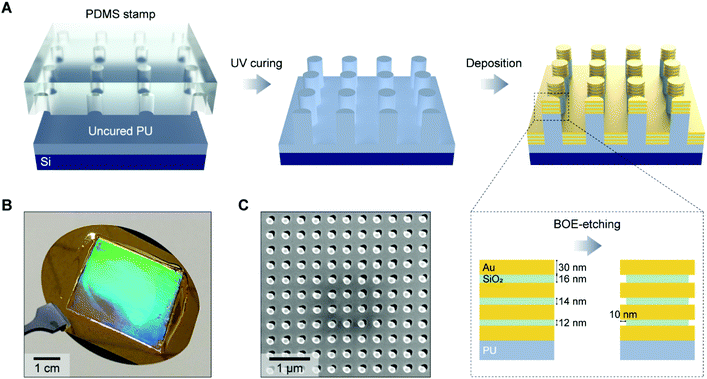 |
| Fig. 1 Fabrication of 3D nanolaminate plasmonic crystals (NLPC) as a SERS substrate for dopamine (DA) sensing. (A) Schematic illustration of the fabrication process for a 3D NLPC SERS substrate. (B) Photographic and (C) top-view SEM images of a 3D NLPC SERS substrate. | |
Preparation of 3D NLPC-based dual-recognition structures for DA detection
To produce 3-MPBA-modified AuNPs, 30 μL of 1 mM 3-MPBA (dissolved in 0.2 M NaOH) was added to 1 mL of 10 nm AuNPs (1 OD), and the mixture was allowed to react for 1 h at room temperature. For the removal of unbound 3-MPBA, 3-MPBA-modified AuNPs (3-MPBA-AuNPs) were collected by centrifugation at 15
000 rpm for 10 min and then rinsed three times by resuspension of 3-MPBA-AuNPs in DI water. The 3D NLPC substrates were then functionalized with 1 mL of 5 mM DSP in DMSO for 100 min, followed by washing with DMSO and DI water for 5 min, respectively, to remove residual DSP. To prepare 3D NLPC SERS substrates with the dual-recognition-based sandwich structures for DA detection, DSP-functionalized NLPC (DSP-NLPC) substrates were incubated with different concentrations of DA in aCSF at pH 7.4 (1 mL) for 1 h at room temperature, and then the substrates were rinsed in DI water for 5 min to remove unbound DA molecules. Finally, the DA-bound DSP-NLPC substrates were incubated with 200 μL of 3-MPBA-AuNPs for 1 h, followed by washing with DI water for 5 min to remove unbound 3-MPBA-AuNPs, thus reducing the possibility of false-positive signals.
SERS measurements
A confocal Raman microscope (DXR2, Thermo Scientific, USA) equipped with a 780 nm diode laser was used for SERS measurements. Before the measurement, the instrumental calibration was verified using the silicon peak at 520 cm−1. SERS signals were obtained using 1 mW of laser power through a 50× objective lens (NA = 0.75) for an acquisition time of 1 s, repeated twice. All of the measured data were baseline-corrected using the embedded software OMNIC.
3D finite-difference time-domain simulation
To calculate the near-field optical properties of the 3D NLPC, we performed the finite-domain time-difference (FDTD) simulations (FDTD solution, Lumerical Inc.). A uniform mesh size of 1 nm (x, y, and z directions) was used. The optical constants of gold were taken from the study of Johnson and Christy. The Bloch boundary condition was used in the x- and y-directions with a periodicity of 400 nm, and the perfectly matched layer boundary condition was used in the z-direction.
Results and discussion
Characterization of 3D NLPC SERS substrates
The 3D NLPC substrate consisting of vertically stacked MIM building blocks can exhibit a multiresonant optical property due to the plasmon hybridization of multiple nanolocalized modes.45–51 Compared to conventional SERS substrates, which can support a spectrally tuned single localized surface plasmon mode near the excitation laser wavelength, the broadband multiresonant response from the visible to the NIR range allows for versatile multiband SERS operation that can be adapted to application-specific demands.
We evaluated the SERS performance of the 3D NLPC substrate by measuring the 3-MPBA molecules on the NLPC using two different excitation wavelengths of 633 and 780 nm. For the SERS intensity of 3-MPBA at 994 cm−1, 780 nm excitation shows a relatively higher performance compared to 633 nm excitation (Fig. 2A). To corroborate our experimental observation, we investigated the near-field optical properties of the NLPC using FDTD simulations. The spatial distributions of the calculated electric field intensity (|E|2) show that the stronger local electric fields are concentrated at the nanogaps and the sidewalls of the metal nanodisks under an excitation wavelength of 780 nm compared to 633 nm despite the highly localized electric fields in the three nanogaps at both wavelengths (Fig. 2B). More specifically, the 3D NLPC exhibits a maximum |E|2 of 287 at 780 nm, higher than that at 633 nm (|E|2 of 112).
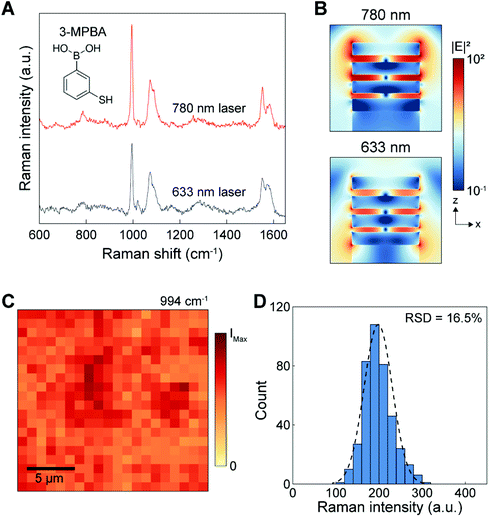 |
| Fig. 2 Performance characterization of 3D NLPC SERS substrates. (A) SERS spectra of 3-MPBA molecules functionalized on NLPC substrates at excitation wavelengths of 633 and 780 nm. (B) Calculated electric field intensity distribution maps at excitation wavelengths of 633 and 780 nm using the FDTD method. (C) SERS mapping image over an area of 20 μm × 20 μm with 20 × 20 pixels using the intensity of the 3-MPBA peak at 994 cm−1. (D) Statistical distribution of the Raman intensity converted from the SERS mapping image shown in (C). | |
On the basis of the higher SERS intensity at 780 nm, we used the excitation wavelength of 780 nm for further investigation of NLPC-based SERS sensing. In addition, SERS measurements under NIR excitation can allow a weaker autofluorescence background and lower phototoxicity for biological and clinical samples.20 To assess the uniformity of 3D NLPC SERS substrates, we obtained a SERS mapping image using the intensity of the 3-MPBA peak at 994 cm−1. The mapping image shows a uniform distribution of SERS intensities from 400 pixels over a large area of 20 μm × 20 μm (Fig. 2C). The histogram plot displays that the intensity distribution fits a normal distribution curve, indicating that the SERS hotspot spatial distribution has good uniformity (Fig. 2D). The relative standard deviation (RSD) of the NLPC was 16.5%, better than various commercially available SERS substrates.52 Furthermore, we did not observe any contamination-induced peaks from the intrinsic device spectrum (Fig. S1†).
3D NLPC-based DA detection
The dual-recognition assay, which has a sandwich-type structure, can be adopted in SERS sensing to allow the quantitative detection of target analytes with reduced non-specific binding. In conventional SERS-based dual-recognition methods, plasmonic nanogap hotspots are generated from binding events where two affinity reagents link the target molecule between two metal surfaces.53–56 Following a similar approach with certain improvements, we combined Raman tag-conjugated AuNPs (diameter = 10 nm) with 3D NLPC SERS substrates consisting of arrays of vertically stacked multi-nanogap hotspots. This NLPC-based sensing platform can produce additional hotspots through the binding of 3-MPBA-AuNPs to the existing nanogap hotspots of the 3D NLPC SERS substrate in the presence of DA molecules.
Fig. 3A presents the molecular sensing scheme used to implement the NLPC-based dual-recognition assay for DA detection. DA molecules can bind to the DSP-functionalized NLPC substrate through the formation of an amide bond via the reaction between N-hydroxysuccinimide ester and amine. The 3-MPBA-modified AuNPs, where 3-MPBA molecules act as Raman tags, are bound to DA molecules through the interaction between boronic acid and the diol terminal group. A label-free SERS sensing approach exhibits a poor signal-to-noise level for the detection of DA (Fig. 3B). Once a solution containing DA molecules is introduced to the NLPC SERS substrates with the dual-recognition functionality, two types of signal levels can be obtained as “On” (Fig. 3D) and “Off” (Fig. 3C) depending on the presence or absence of DA molecules from a single unit cell perspective. Therefore, this approach can be used for the quantitative detection of DA molecules.
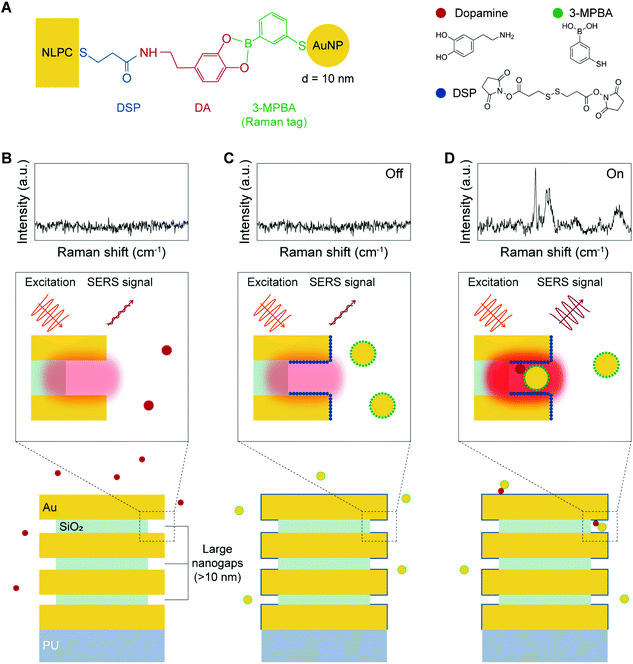 |
| Fig. 3 Digital SERS sensing platform using the 3D NLPC-based dual-recognition structure coupled with AuNPs for quantitative DA detection. (A) Molecular sensing scheme for the specific targeting of DA via a 3D NLPC-based dual-recognition structure. (B) Label-free SERS sensing. DA-specific dual-recognition structure, producing (C) an “Off” state in the absence of DA and (D) an “On” state in the presence of DA. | |
To investigate the SERS activity of 3D NLPC substrates, we first attempted to detect DA molecules on the 3D NLPC substrates using a label-free approach (Fig. 4A). The label-free SERS measurement of 1 mM DA on the 3D NLPC produced multiple broad and spectrally overlapping Raman peaks at ∼1180 cm−1 for ρ(CH2), 1380 cm−1 for v(19b), 1525 cm−1 for v(19a), and ∼1586 cm−1 for v(8a), which is in good agreement with the previous study.57 Here, ρ and v denote rocking and stretching modes, respectively. For the same concentration of DA (1 mM) on the DSP-functionalized NLPC substrate, the Raman spectrum exhibited a ∼1.3 times higher peak intensity compared to the label-free SERS sensing at 1525 cm−1, indicating the more efficient binding of DA to the NLPC substrate via DSP-mediated capture. Remarkably, the ratio between the two characteristic peaks for DA at 1380 and 1525 cm−1 changed, possibly because the DA molecular orientation relative to the local optical field in the hotspots was modulated by the DA binding onto the DSP-functionalized NLPC substrate.58,59 Interestingly, we did not observe any distinct Raman peaks for DSP in the measured spectra, which can be attributed to Raman-inactive asymmetric dipolar stretching modes, including N-hydroxysuccinimide and COOH vibrational modes as the main components of DSP.55,60 For lower DA concentrations, including 1 μM and 1 nM, the Raman intensities were significantly reduced to the noise level because of the weak Raman cross-section of the DA molecules. However, we still observed the SERS profile of DA with broad peaks (Fig. S2†).
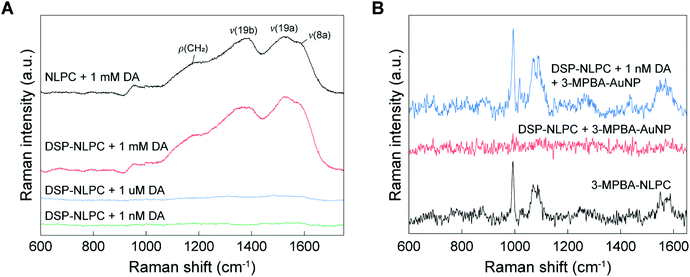 |
| Fig. 4 3D NLPC-based DA detection using label-free and Raman tag-labeled SERS sensing. (A) Raman spectra for DA on the NLPC substrates functionalized with and without DSP for label-free SERS sensing at different DA concentrations. (B) Raman spectrum for the DA-specific dual-recognition structure at 1 nM DA, compared with configurations without DA and/or Raman tag-conjugated AuNPs. | |
To examine the utility of the NLPC-based dual-recognition structures for DA detection, we compared the Raman spectrum of the DA-specific dual-recognition structures with a reference case and a configuration without the target molecules (DA) (Fig. 4B). As a reference, we first measured the Raman spectrum of a self-assembled monolayer of 3-MPBA on the 3D NLPC SERS substrate without DA molecules and additional AuNPs (black). In the configuration of the 3D NLPC substrate with the DA-specific dual-recognition structures for 1 nM DA (blue), the Raman spectrum clearly exhibited the main characteristic peaks of 3-MPBA at 994 and 1071 cm−1, similar to the reference case even with a long distance from the substrate due to the dual-recognition structures. Although the strength of the local electric field significantly decreases with the increase of the distance, new hotspots induced by AuNPs can provide further enhancement. In contrast, the Raman spectrum of the DSP-functionalized NLPC substrate with 3-MPBA-modified AuNPs (red) did not have any peaks associated with either DSP or 3-MPBA because the 3-MPBA-AuNPs could not bind to the substrate in the absence of DA molecules. SEM images show the AuNPs attached to the 3D NLPC SERS substrate through the dual-recognition method (Fig. S3†). These results indicate that the introduction of AuNPs to 3D NLPC SERS substrates can generate additional hotspots with stronger SERS enhancement and the 3D NLPC-based dual-recognition structures coupled with AuNPs are effective in the detection of DA.
Digital SERS sensing for the accurate quantitative determination of DA
To evaluate the quantitative DA sensing capability of the 3D NLPC-based dual-recognition structures coupled with AuNPs, we performed SERS mapping for the 3D NLPC substrate with the DA-specific dual-recognition structures using different concentrations of DA from 1 pM to 1 nM. Fig. 5A shows the SERS mapping images using the intensity of the 3-MPBA peak at 994 cm−1 for the different DA concentrations, and each mapping image was obtained from an area of 20 μm × 20 μm with 20 × 20 pixels. As the DA concentration decreased, there was a gradual decrease in the total number of pixels with a higher Raman intensity depicted as orange and red dots. The sum and average of the SERS intensity from 400 pixels also exhibited the same decreasing trend with a decrease in the DA concentration (Fig. S2†). Fig. 5B shows four representative “On” spectra obtained from the 3D NLPC-based dual-recognition structures coupled with AuNPs, indicating the presence of DA at four different positions and the representative “Off” spectrum in the absence of DA. Remarkably, the four “On” spectra revealed different spectral profiles for 3-MPBA in terms of intensity, peak ratio, and the number of peaks. This spot-to-spot variation in the “On” spectral profiles can originate from several different factors. First, the SERS intensity of a specific vibrational mode strongly depends on the direction between the transition dipole moment and the local optical field; thus variation in the molecule orientation at plasmonic hotspots can lead to a significant difference in the relative peak intensity based on the surface selection rules.58,59 Second, variation in the molecular orientation can be higher for 3D nanostructured SERS substrates with multiple hotspots than for 2D planar metal surfaces. Furthermore, a dual-recognition structure involving two binding connections can have a higher degree of molecular orientation variation than the case with one binding connection. Third, the spatial distribution of the AuNPs relative to the single unit cell of the 3D NLPC substrate can cause different degrees of SERS enhancement. For an excitation laser focus spot with a diameter of ca. 500 nm, every pixel in the SERS mapping image contains at least more than a single unit cell of the 3D NLPC SERS substrate (with a periodicity of 400 nm). AuNPs can be placed at different positions, for example, in the nanogaps or on the top surface of the metal nanodisks when 3-MPBA-AuNP binds to the DA molecules. This spatial variation in AuNPs can lead to different SERS intensities for the detection of DA molecules at ultralow concentrations. In addition to these microscopic factors, SERS substrates can exhibit intrinsic inhomogeneity in their hotspot distribution, caused by nanoscale geometrical variation.21 Because of the various microscopic factors, the conventional analog method, which correlates the sum or average of the measured SERS intensity with the concentration of target analytes, can lead to an inaccurate quantitative SERS analysis with differences between the measured signals and the actual DA concentration of the sample.
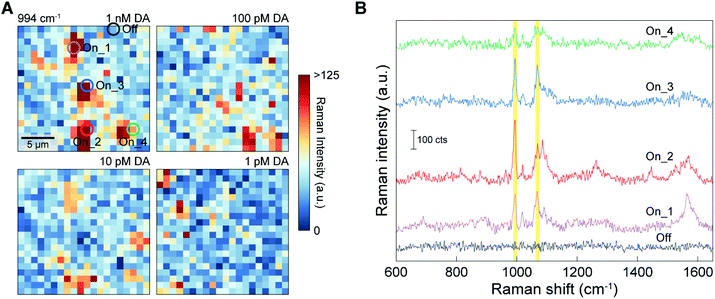 |
| Fig. 5 SERS mapping results from the 3D NLPC-based DA-specific dual-recognition structures coupled with AuNPs using different concentrations of DA. (A) SERS mapping images using the intensity of the 3-MPBA peak at 994 cm−1 for different DA concentrations. (B) Raman spectra of four different “On” sites and one “Off” site. The origins of the spectra are depicted as circles in (A). | |
Digital assays have been used to allow the quantitative determination of target analytes for biomedical applications, including enzyme-linked immunosorbent assay (ELISA) and polymerase chain reaction (PCR).61 The availability of digital assays for SERS measurements was recently demonstrated by highly accurate SERS analysis at ultralow concentrations.42 For the accurate quantitative determination of target analytes by digital SERS sensing, spatial SERS mapping can satisfy the requirement for a sufficient amount of data to minimize errors. We thus conducted digital SERS analysis to reduce signal variation stemming from the random molecular position and orientation and their increased complexity with the use of 3D nanostructures and the dual-recognition structures. For the SERS mapping images obtained from the 3D NLPC-based dual-recognition structures coupled with AuNPs (Fig. 5A), we converted the SERS mapping results into binary signals by assigning an “On” or “Off” state to the intensities if they were above or below a pre-determined threshold, respectively. The threshold value of 60 CCD counts was determined by three times higher than the average noise level (Fig. S5†).
Fig. 6A shows a digitized version of the SERS mapping images using the peak at 994 cm−1 for different DA concentrations, where the grey “On” pixels indicate the presence of DA and the white “Off” pixels represent null events. The digital images reveal the locations of DA binding sites on the 3D NLPC SERS substrates via the dual-recognition assay and can thus allow an accurate quantitative determination of DA molecules on the surfaces. The total number of “On” pixels from each mapping image was plotted as a function of the DA concentration (Fig. 6B). As the DA concentration increased from 1 pM to 1 nM, there was a clear increase in the total number of “On” pixels. To corroborate this digital SERS-based detection using the NLPC-based dual-recognition structures, we analyzed the additional characteristic peak for 3-MPBA at 1071 cm−1. The number of “On” events was also proportional to the DA concentration, which is consistent with the results for the peak at 994 cm−1. Accordingly, the digital “On” counts within the SERS mapping images are clearly correlated with low-abundance DA molecules. A high DA specificity was observed by comparing the digital counts for 1 nM of DA and other molecules including neurotransmitters that do not have diol and amine simultaneously (L-tyrosine, L-phenylalanine, and epinephrine) and catechol which has a chemical structure similar to DA (Fig. S6†). Furthermore, device-to-device reproducibility was verified with the average recovery rate of 93.7% (Table S1†) using a linear regression model derived from Fig. 6B (Fig. S7†). These results indicate that digital SERS signal analysis can significantly reduce the effect of multiple sources of signal variation that would otherwise lead to the inaccurate quantification of target analytes using the conventional analog method. Therefore, this digital SERS platform using the 3D NLPC-based dual-recognition structures coupled with AuNPs and the digital SERS signal analysis can be used effectively for the highly sensitive, accurate, and quantitative determination of DA molecules on nanostructured surfaces. Table S2† provides a list of previous SERS-based DA sensing techniques compared to this work.
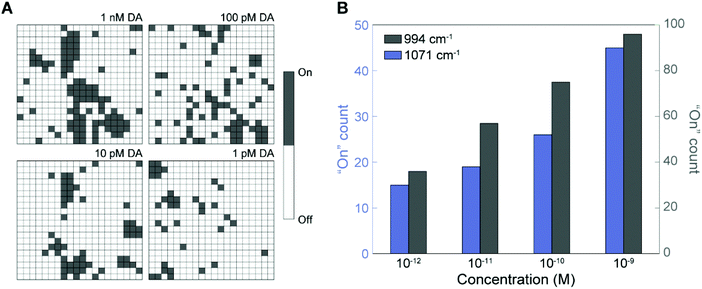 |
| Fig. 6 Digital SERS sensing for the quantitative determination of DA. (A) Digital mapping images with different DA concentrations converted from those in Fig. 5A. (B) Histogram of the total number of “On” spectra using two main peaks for 3-MPBA at 994 and 1071 cm−1 (the yellow lines in Fig. 5B). | |
Conclusions
We demonstrated a new type of digital SERS sensing platform using 3D NLPC-based dual-recognition structures coupled with AuNPs and digital SERS signal analysis for the accurate quantitative determination of low-abundance DA molecules. To develop this platform, we used a top-down high-throughput and cost-effective fabrication method to produce 3D NLPC SERS substrates consisting of vertically stacked multi-nanogap hotspots and then introduced Raman tag-conjugated AuNPs to the substrates for more effective SERS enhancement and selective DA detection. For the accurate quantitative determination of target DA molecules, we conducted digital SERS signal analysis to reduce the signal variation originating from the different microscopic sources, including random molecular orientation, the random spatial distribution of AuNP-induced hotspots, and the intrinsic inhomogeneity of SERS substrates, by converting the SERS intensities into “On” or “Off” states. Accordingly, the digital SERS method can reliably quantify target analytes with a lower variation compared to conventional intensity-based SERS analysis. The digital SERS analysis showed that the digital “On” counts in the SERS mapping images were proportional to the DA concentration, thus allowing the quantitative measurement of DA concentrations down to 1 pM. Therefore, the proposed 3D NLPC-based digital SERS platform can be used not only as an effective and practical tool for highly sensitive and quantitative DA detection, but also as a promising and rapid analytical tool for various molecules.
Author contributions
Wonil Nam: data curation, formal analysis, investigation, methodology, software, validation, visualization, writing – original draft, and writing – review & editing. Wansun Kim: data curation, investigation, and validation. Wei Zhou: conceptualization, funding acquisition, resources, supervision, and writing – review & editing. Eun-Ah You: conceptualization, funding acquisition, investigation, project administration, resources, supervision, and writing – review & editing.
Conflicts of interest
There are no conflicts to declare.
Acknowledgements
This work was supported by the Air Force Office of Scientific Research (AFOSR) Young Investigator Award FA9550-18-1-0328, the Characterization Platform for Advanced Materials funded by the Korea Research Institute of Standards and Science (KRISS-2021-GP2021-0011), and the Industrial Innovation Foundation Construction Program (P0014175) funded by the Ministry of Trade, Industry & Energy (MOTIE, Korea).
Notes and references
- R. A. Wise, Nat. Rev. Neurosci., 2004, 5, 483–494 CrossRef CAS PubMed.
- J. D. Berke, Nat. Neurosci., 2018, 21, 787–793 CrossRef CAS PubMed.
- R. de la Fuente-Fernandez, T. J. Ruth, V. Sossi, M. Schulzer, D. B. Calne and A. J. Stoessl, Science, 2001, 293, 1164–1166 CrossRef CAS PubMed.
- A. Zhang, J. L. Neumeyer and R. J. Baldessarini, Chem. Rev., 2007, 107, 274–302 CrossRef CAS PubMed.
- D. S. Goldstein, C. Holmes and Y. Sharabi, Brain, 2012, 135, 1900–1913 CrossRef PubMed.
- C. Sarkar, B. Basu, D. Chakroborty, P. S. Dasgupta and S. Basu, Brain, Behav., Immun., 2010, 24, 525–528 CrossRef CAS PubMed.
- T. Palomaki, E. Peltola, S. Sainio, N. Wester, O. Pitkanen, K. Kordas, J. Koskinen and T. Laurila, Biosens. Bioelectron., 2018, 118, 23–30 CrossRef CAS PubMed.
- S. Verma, P. Arya, A. Singh, J. Kaswan, A. Shukla, H. R. Kushwaha, S. Gupta and S. P. Singh, Biosens. Bioelectron., 2020, 165, 112347 CrossRef CAS PubMed.
- V. Carrera, E. Sabater, E. Vilanova and M. A. Sogorb, J. Chromatogr. B: Anal. Technol. Biomed. Life Sci., 2007, 847, 88–94 CrossRef CAS PubMed.
- H. Gu, E. L. Varner, S. R. Groskreutz, A. C. Michael and S. G. Weber, Anal. Chem., 2015, 87, 6088–6094 CrossRef CAS PubMed.
- X. Xu, H. Shi, L. Ma, W. Kang and S. Li, Luminescence, 2011, 26, 93–100 CrossRef CAS PubMed.
- L. Zhang, Y. Cheng, J. Lei, Y. Liu, Q. Hao and H. Ju, Anal. Chem., 2013, 85, 8001–8007 CrossRef CAS PubMed.
- A. Yildirim and M. Bayindir, Anal. Chem., 2014, 86, 5508–5512 CrossRef CAS PubMed.
- J. Zhao, L. Zhao, C. Lan and S. Zhao, Sens. Actuators, B, 2016, 223, 246–251 CrossRef CAS.
- S. Kruss, D. P. Salem, L. Vukovic, B. Lima, E. Vander Ende, E. S. Boyden and M. S. Strano, Proc. Natl. Acad. Sci. U. S. A., 2017, 114, 1789–1794 CrossRef CAS.
- A. Pathak and B. D. Gupta, Biosens. Bioelectron., 2019, 133, 205–214 CrossRef CAS PubMed.
- J. J. Feng, H. Guo, Y. F. Li, Y. H. Wang, W. Y. Chen and A. J. Wang, ACS Appl. Mater. Interfaces, 2013, 5, 1226–1231 CrossRef CAS PubMed.
- D. Wen, W. Liu, A. K. Herrmann, D. Haubold, M. Holzschuh, F. Simon and A. Eychmuller, Small, 2016, 12, 2439–2442 CrossRef CAS.
- S.-Y. Ding, J. Yi, J.-F. Li, B. Ren, D.-Y. Wu, R. Panneerselvam and Z.-Q. Tian, Nat. Rev. Mater., 2016, 1, 16021 CrossRef CAS.
- C. Zong, M. Xu, L. J. Xu, T. Wei, X. Ma, X. S. Zheng, R. Hu and B. Ren, Chem. Rev., 2018, 118, 4946–4980 CrossRef CAS PubMed.
- Y. Fang, N. H. Seong and D. D. Dlott, Science, 2008, 321, 388–392 CrossRef CAS PubMed.
- A. Garg, W. Nam and W. Zhou, ACS Appl. Mater. Interfaces, 2020, 12, 56290–56299 CrossRef CAS PubMed.
- L. Zhang, C. Jiang and Z. Zhang, Nanoscale, 2013, 5, 3773–3779 RSC.
- H. Zhang, M. Liu, F. Zhou, D. Liu, G. Liu, G. Duan, W. Cai and Y. Li, Small, 2015, 11, 844–853 CrossRef CAS PubMed.
- J. Zhang, L. He, P. Chen, C. Tian, J. Wang, B. Liu, C. Jiang and Z. Zhang, Nanoscale, 2017, 9, 1599–1606 RSC.
- J. Zhang, L. He, X. Zhang, J. Wang, L. Yang, B. Liu, C. Jiang and Z. Zhang, Sens. Actuators, B, 2017, 253, 839–845 CrossRef CAS.
- T. Zhang, Y. Sun, L. Hang, H. Li, G. Liu, X. Zhang, X. Lyu, W. Cai and Y. Li, ACS Appl. Mater. Interfaces, 2018, 10, 9792–9801 CrossRef CAS PubMed.
- J. Lu, C. Xu, H. Nan, Q. Zhu, F. Qin, A. G. Manohari, M. Wei, Z. Zhu, Z. Shi and Z. Ni, Appl. Phys. Lett., 2016, 109, 073701 CrossRef.
- X. Yu, X. He, T. Yang, L. Zhao, Q. Chen, S. Zhang, J. Chen and J. Xu, Int. J. Nanomed., 2018, 13, 2337–2347 CrossRef CAS PubMed.
- P. Wang, M. Xia, O. Liang, K. Sun, A. F. Cipriano, T. Schroeder, H. Liu and Y. H. Xie, Anal. Chem., 2015, 87, 10255–10261 CrossRef CAS PubMed.
- Y. Luo, L. Ma, X. Zhang, A. Liang and Z. Jiang, Nanoscale Res. Lett., 2015, 10, 937 Search PubMed.
- B. Lin, J. Chen, P. Kannan, Y. Zeng, B. Qiu, L. Guo and Z. Lin, Mikrochim. Acta, 2019, 186, 260 CrossRef PubMed.
- M. Kaya and M. Volkan, Anal. Chem., 2012, 84, 7729–7735 CrossRef CAS PubMed.
- V. D. Phung, W. S. Jung, T. A. Nguyen, J. H. Kim and S. W. Lee, Nanoscale, 2018, 10, 22493–22503 RSC.
- M. L. B. Figueiredo, C. S. Martin, L. N. Furini, R. J. G. Rubira, A. Batagin-Neto, P. Alessio and C. J. L. Constantino, Appl. Surf. Sci., 2020, 522, 146466 CrossRef CAS.
- P. Li, B. Zhou, X. Cao, X. Tang, L. Yang, L. Hu and J. Liu, Chemistry, 2017, 23, 14278–14285 CrossRef CAS.
- J. H. An, D. K. Choi, K. J. Lee and J. W. Choi, Biosens. Bioelectron., 2015, 67, 739–746 CrossRef CAS PubMed.
- L. Tang, S. Li, F. Han, L. Liu, L. Xu, W. Ma, H. Kuang, A. Li, L. Wang and C. Xu, Biosens. Bioelectron., 2015, 71, 7–12 CrossRef CAS PubMed.
- N. C. Lindquist, C. D. L. de Albuquerque, R. G. Sobral-Filho, I. Paci and A. G. Brolo, Nat. Nanotechnol., 2019, 14, 981–987 CrossRef CAS PubMed.
- H. K. Choi, K. S. Lee, H. H. Shin, J. J. Koo, G. J. Yeon and Z. H. Kim, Acc. Chem. Res., 2019, 52, 3008–3017 CrossRef CAS PubMed.
- D. P. Dos Santos, M. L. A. Temperini and A. G. Brolo, Acc. Chem. Res., 2019, 52, 456–464 CrossRef CAS PubMed.
- C. D. L. de Albuquerque, R. G. Sobral-Filho, R. J. Poppi and A. G. Brolo, Anal. Chem., 2018, 90, 1248–1254 CrossRef CAS PubMed.
- D. Qin, Y. Xia and G. M. Whitesides, Nat. Protoc., 2010, 5, 491–502 CrossRef CAS PubMed.
- J. Song and W. Zhou, Nano Lett., 2018, 18, 4409–4416 CrossRef CAS PubMed.
- W. Nam, X. Ren, S. A. S. Tali, P. Ghassemi, I. Kim, M. Agah and W. Zhou, Nano Lett., 2019, 19, 7273–7281 CrossRef CAS PubMed.
- J. Song, W. Nam and W. Zhou, Adv. Mater. Technol., 2019, 4, 1800689 CrossRef.
- W. Nam, Y. Zhao, J. Song, S. Ali Safiabadi Tali, S. Kang, W. Zhu, H. J. Lezec, A. Agrawal, P. J. Vikesland and W. Zhou, J. Phys. Chem. Lett., 2020, 11, 9543–9551 CrossRef CAS PubMed.
- J. Song, W. Cheng, M. Nie, X. He, W. Nam, J. Cheng and W. Zhou, ACS Nano, 2020, 14, 9521–9531 CrossRef CAS PubMed.
- X. Ren, W. Nam, P. Ghassemi, J. S. Strobl, I. Kim, W. Zhou and M. Agah, Microsyst. Nanoeng., 2020, 6, 47 CrossRef CAS PubMed.
- W. Nam, X. Ren, I. Kim, J. Strobl, M. Agah and W. Zhou, Anal. Chem., 2021, 93, 4601–4610 CrossRef CAS PubMed.
- W. Nam, J. Song, S. A. Safiabadi Tali, H. J. Lezec, A. Agrawal and W. Zhou, ACS Appl. Nano Mater., 2021, 4, 3175–3184 CrossRef CAS.
- Y. Liu, Y. Zhang, M. Tardivel, M. Lequeux, X. Chen, W. Liu, J. Huang, H. Tian, Q. Liu, G. Huang, R. Gillibert, M. L. de la Chapelle and W. Fu, Plasmonics, 2019, 15, 743–752 CrossRef.
- T. T. Chuong, A. Pallaoro, C. A. Chaves, Z. Li, J. Lee, M. Eisenstein, G. D. Stucky, M. Moskovits and H. T. Soh, Proc. Natl. Acad. Sci. U. S. A., 2017, 114, 9056–9061 CrossRef CAS PubMed.
- X. Gu, M. J. Trujillo, J. E. Olson and J. P. Camden, Annu. Rev. Anal. Chem., 2018, 11, 147–169 CrossRef CAS PubMed.
- K. Zhang, Y. Liu, Y. Wang, R. Zhang, J. Liu, J. Wei, H. Qian, K. Qian, R. Chen and B. Liu, ACS Appl. Mater. Interfaces, 2018, 10, 15388–15394 CrossRef CAS PubMed.
- I. Khalil, W. A. Yehye, N. M. Julkapli, S. Rahmati, A. A. Sina, W. J. Basirun and M. R. Johan, Biosens. Bioelectron., 2019, 131, 214–223 CrossRef CAS PubMed.
- O. Jha, T. K. Yadav and R. A. Yadav, Spectrochim.
Acta, Part A, 2018, 189, 473–484 CrossRef CAS PubMed.
- M. Moskovits, J. Chem. Phys., 1982, 77, 4408–4416 CrossRef CAS.
- E. C. Le Ru, S. A. Meyer, C. Artur, P. G. Etchegoin, J. Grand, P. Lang and F. Maurel, Chem. Commun., 2011, 47, 3903–3905 RSC.
- C. Y. Lim, N. A. Owens, R. D. Wampler, Y. Ying, J. H. Granger, M. D. Porter, M. Takahashi and K. Shimazu, Langmuir, 2014, 30, 12868–12878 CrossRef CAS PubMed.
- Y. Zhang and H. Noji, Anal. Chem., 2017, 89, 92–101 CrossRef CAS PubMed.
Footnote |
† Electronic supplementary information (ESI) available. See DOI: 10.1039/d1nr03691b |
|
This journal is © The Royal Society of Chemistry 2021 |