Evaluation of reverse osmosis drinking water treatment of riverbank filtrate using bioanalytical tools and non-target screening†
Received
27th August 2019
, Accepted 15th October 2019
First published on 18th October 2019
Abstract
Stand-alone reverse osmosis (RO) has been proposed to produce high-quality drinking water from raw riverbank filtrate impacted by anthropogenic activities. To evaluate RO efficacy in removing organic micropollutants, biological analyses were combined with non-target screening using high-resolution mass spectrometry and open cheminformatics tools. The bank filtrate induced xenobiotic metabolism mediated by the aryl hydrocarbon receptor AhR, adaptive stress response mediated by the transcription factor Nrf2 and genotoxicity in the Ames-fluctuation test. These effects were absent in the RO permeate (product water), indicating the removal of bioactive micropollutants by RO membranes. In the water samples, 49 potentially toxic compounds were tentatively identified with the in silico fragmentation tool MetFrag using the US Environmental Protection Agency CompTox Chemicals Dashboard database. 5 compounds were confirmed with reference standards and 16 were tentatively identified with high confidence based on similarities to accurate mass spectra in open libraries. The bioactivity data of the confirmed chemicals indicated that 2,6-dichlorobenzamide and bentazone in water samples can contribute to the activation of AhR and oxidative stress response, respectively. The bioactivity data of 7 compounds tentatively identified with high confidence indicated that these structures can contribute to the induction of such effects. This study showed that riverbank filtration followed by RO could produce drinking water free of the investigated toxic effects.
Water impact
Effects triggered by mixtures of organic micropollutants detected in a raw riverbank filtrate were removed by stand-alone reverse osmosis drinking water treatment. Potentially toxic contaminants were characterised by non-target screening of high-resolution mass spectrometry data using open cheminformatics and an openly accessible chemical database with bioactivity metadata, broadening the scope of the qualitative screening beyond just target compounds.
|
1. Introduction
Natural drinking water sources are ubiquitously contaminated with polar organic micropollutants and their transformation products (TPs).1–4 The chemical mixtures that threaten the quality of source waters and drinking water can vary widely, including persistent and pseudo-persistent, i.e. continuously emitted, mobile hydrophilic compounds.5 As the potential adverse effects on human health are not fully understood,6,7 it is preferred to maximise micropollutant removal from drinking water and to efficiently, comprehensively evaluate its quality.
Reverse osmosis (RO) has shown great potential for removing organic micropollutants from a variety of water matrices.8–10 RO uses semi-permeable membranes to separate solutes from water molecules under the driving force of an externally applied pressure.11 Chemical passage through RO membranes follows a solution–diffusion mechanism,12 with solvent and solutes independently transported to the permeate side along their transmembrane chemical potential gradient. Diffusion of organics is mainly hindered by the compound size and influenced by the charge and hydrophobicity of solutes and membranes.12,13 As the baseline mechanism behind chemical removal by RO is physical separation, by-products are not expected unless the membrane integrity is compromised or the feed water is disinfected.13 Although RO is considered as an energy intensive step when incorporated in conventional treatment trains,14 stand-alone RO applications to produce potable water from natural waters requiring minimum pre-treatment have emerged, representing a new scenario to achieve excellent removal of harmful chemicals and waterborne pathogens with low operational costs and environmental impact.15
In the Netherlands, RO has been proposed as a single-step treatment to produce high-quality drinking water from riverbank filtrate. Riverbank filtration (RBF) is an energy-efficient process that occurs naturally or can be induced to increase source water quality in catchment areas impacted by anthropogenic activities.16–20 RBF can attenuate micropollutant concentrations as a result of biodegradation and sorption phenomena taking place mostly in the hyporheic zone21,22 and to a lesser extent in the aquifer.23 The fate of polar organics largely depends on the biogeochemical conditions of RBF systems and on the physicochemical properties of compounds.19 Typically, sorption is effective in retaining non-polar, moderately hydrophobic compounds and cationic compounds by hydrophobic and electrostatic interaction mechanisms, respectively, whereas neutral hydrophilic substances and anionic organics can pass through the hyporheic zone, remaining unchanged if they are not biodegraded.16,18
To comprehensively assess water quality, a combination of chemical analysis and effect-based methods (EBMs) has been proposed.24,25 EBMs relying on low-complexity in vivo or cell-based in vitro bioanalytical tools with specific endpoints can be employed to evaluate the adverse effects of (organic) chemicals,26 emphasising mixture effects of water samples rather than single components.27 EBMs focussing on genotoxicity and cytotoxicity emerged in the 1970s,28,29 whereas reporter gene assays were introduced in the 1990s.30 Nowadays, EBMs are being increasingly integrated in routine applications to evaluate toxicity pathways with biological endpoints relevant for water quality. Sensitive test batteries covering specific and non-specific modes of actions are employed, including bioassays representative of receptor-mediated endocrine disruption, metabolism of xenobiotics and adaptive stress response indicated as minimum requirements.31
Dissolved polar organics are typically characterised by liquid-chromatography coupled to tandem mass spectrometry (LC-MS/MS). The capabilities of recent high-resolution MS (HRMS) have set the basis for suspect screening and non-target screening (NTS), i.e. methodologies to elucidate the structures of unknown ions by tentative annotation of accurate mass full-scan spectra (HRMS1) and tandem mass spectra (HRMS2) without the need for reference standards in advance of measurement.32–34 Suspect screening deals with the tentative annotation of compounds expected to occur in the samples. Typically, suspect chemicals have known structures, and in some cases known fragmentation behaviour and chromatographic retention time. Meanwhile, NTS deals with the elucidation of structures for which a priori information of their occurrence in a sample is not available. State-of-the art NTS uses the high-throughput performance of open cheminformatics tools such as MetFrag and SIRIUS,35,36in silico fragmenters that query a chemical database, e.g. PubChem,37 to retrieve candidate structures. These are then scored on the basis of the fit of the in silico-generated MS fragments to the experimental HRMS2 data and, in some cases, on selected metadata associated with candidate structures. This approach has shown potential for increasing the chemical identification success rate.38 However, identification with large databases such as PubChem can result in many thousands of candidates, which can be challenging to interpret in high throughput use cases. The U.S. Environmental Protection Agency (EPA) hosts the CompTox Chemicals Dashboard,39 an open database with high-quality, structure-curated data of ∼875
000 substances.40 The structures deposited in the Dashboard are linked to human and ecological hazard data from various sources, including in vitro bioactivity data from ToxCast and Tox21 high-throughput screening programmes,41,42 predicted exposure data from the ExpoCast project,43 and a variety of high-interest environmental lists of chemicals. A valuable and so far unique feature of the Dashboard is the accessibility to MS-ready form structures.44 The Dashboard is downloadable, giving the possibility of being used as the local database in MetFrag (or other applications). Because of its health- and environment-relevant metadata, the Dashboard is a valuable tool for NTS of environmental contaminants with potential toxic effects.45
The aim of this study was to evaluate the application of RO as a stand-alone treatment step to produce high quality drinking water from a raw riverbank filtrate that originated from the Lower Rhine in the Netherlands, using the biological and chemical methods mentioned above. The Rhine catchment area, despite regulatory actions and mitigation measures that substantially improved its ecological status,46 remains contaminated with anthropogenic organic micropollutants,7,47,48 so their removal from the river water by RBF and RO requires continuous monitoring. We adopted a combined approach relying on (i) EBMs representative of endocrine disruption, xenobiotic metabolism, adaptive stress response and genotoxicity relevant for human health and (ii) NTS of LC-HRMS/MS data using open cheminformatics tools in connection with the EPA CompTox Chemicals Dashboard. The bioassay test battery provided a broad coverage of modes of action and represented toxicity pathways relevant for human health known to be triggered by micropollutants in environmental water samples.24,31,49 To our knowledge, this is the first effect-based monitoring study of a RO drinking water treatment plant fed with raw natural freshwater where potentially toxic compounds were characterised by state-of-the-art NTS with open cheminformatics approaches.
2. Materials and methods
2.1. Full-scale RO treatment plant and sampling
The full-scale RO system was operated for research purposes in the premises of an actual drinking water treatment plant located in the Dutch municipality of Woerden. The system consisted of a three-stage filtration series equipped with ten ESPA2-LD-4040 membrane modules (Hydranautics, Oceanside, CA) in a 6
:
3
:
1 configuration. ESPA2 is a thin-film composite with an active layer of cross-linked aromatic polyamide,50 currently considered the commercial standard RO membrane. The molecular weight cut-off (MWCO) values for this membrane range between 100 and 200 Da.51–53 It is noteworthy that RO membranes are considered non-porous and thus the MWCO principle may not be applicable since solute–membrane affinity interactions influence compound removal rather than only compound size.13 Each step was equipped with flow meters to monitor the feed water, permeate and concentrate lines. The RO system was fed with an actual drinking water source at ≈9 m3 h−1 consisting of raw anaerobic riverbank filtrate with an average travel time of 30 years and freshly abstracted on site. The RO system was set at 70% productivity, resulting in a permeate flow of ≈6.3 m3 h−1 and implying that 30% of the feed water was discarded as RO concentrate. Feed water, RO permeate and RO concentrate samples (n = 4) from the same water package were collected in one sampling event. As the quality of the RBF and the conditions of RO are stable throughout the sampling period, no variations were expected. The samples were taken from faucets built on the system, transferred to 10 L polypropylene bottles and stored in the dark at 2 °C for 12 days before enrichment by solid-phase extraction (SPE). From these samples, aliquots of different volumes and a number of replicates were taken to comply with different enrichment protocols as indicated in section 2.2 and in the ESI† S-1.
2.2. Sample enrichment by solid-phase extraction
To comply with pre-established extraction protocols and avoid problems with the biological and chemical analyses, three enrichment procedures relying on a hydrophilic–lipophilic balance (HLB) sorbent material with solid-phase extraction (SPE) Oasis cartridges by Waters (Etten-Leur, the Netherlands) were used: one for the reporter gene assays, one for the Ames tests and one for chemical analysis, respectively. Details on the different procedures are given in the ESI† section S-1. The enrichment protocols differed by the sample load and composition of the elution solvent. Although this inconsistency may be a limitation, the same broad range of organic compounds is expected to be covered by the three procedures as (i) there were no differences in the pH of the water samples and wash solvents and (ii) organic eluents of comparable polarity were used in all cases. The SPE enrichment factor for the reporter gene assay procedure was 1000×, that for the Ames test was 10
000× and that for chemical analysis was 100× (taking into account the dilution in ultrapure water for the extracts to be compatible with the chromatographic mobile phase used for chemical analysis).
2.3. Bioanalysis
2.3.1.
In vitro reporter gene assays.
In vitro nuclear receptor reporter gene assays, representative of seven endpoints, were used to evaluate specific and non-specific toxicity. In these assays, chemicals with receptor affinity (i.e., ligands) cause a ligand–receptor complex to translocate into the nucleus, where expression of a reporter gene is induced by binding of the complex to a receptor-specific response element on the DNA.26 Endocrine disruption was assessed with a hormone receptor test battery consisting of four cell lines expressing the human estrogen receptor alpha (ERα-GeneBLAzer), the rat androgen receptor (AR-GeneBLAzer), the human glucocorticoid receptor (GR-GeneBLAzer) and the human progesterone receptor (PR-GeneBLAzer), respectively. For these bioassays, ligand–receptor binding induced expression of a reporter gene encoding the enzyme β-lactamase. Further details including experimental procedures for activation of the nuclear receptor and cytotoxicity are described in the literature.54,55 Induction of xenobiotic metabolism was evaluated with two bioassays. The first assay was based on the rat cell line H4L1.1c4 expressing the aryl hydrocarbon receptor containing a chemical-activated luciferase reporter gene (AhR-CALUX). This assay is sensitive to compounds exhibiting dioxin-like activity, which induce the transcription of metabolic enzymes, e.g. the cytochrome P450, that can convert AhR ligands to reactive intermediates.56 Further details including the procedure adopted for the AhR assay can be found in the literature.49,54 The second bioassay to assess the xenobiotic metabolism was based on the human cell line HEK 293H expressing the peroxisome proliferator-activated receptor gamma (PPARγ-GeneBLAzer) with a reporter gene encoding for β-lactamase and followed a previously described procedure.49 This assay is representative of the induction of enzymes responsible for glucose, lipid and fatty acid metabolism. The adaptive stress response was evaluated with a methodology described by Escher et al.57 based on AREc32,58 a stable antioxidant response element-driven Nrf2 reporter gene cell line derived from the human breast cancer MCF7 cells with the addition of a luciferase gene. Activation of the oxidative stress response in AREc32 can be triggered by electrophilic chemicals and reactive oxygen species.57,58
The GeneBLAzer cell lines were purchased from Thermo Fisher (Schwerte, Germany), AREc32 cells were obtained via a material transfer agreement from C. Roland Wolf, Cancer Research UK, and AhR-CALUX cells were obtained via a material transfer agreement from Michael Denison, UC Davis, USA.
All sample concentrations were expressed in units of relative enrichment factor (REF), which takes into account the SPE enrichment factor and the dilution factor in the bioassay.31 The maximum REF used in this study was 100, i.e. the highest enrichment factor in the bioassays was 100 times higher than in the water samples. This could be accomplished by evaporating an aliquot of the extracts in a glass vial and re-solubilising the dried extract in bioassay medium, so that the reporter gene assays did not contain any solvent. For all assays, cell viability was assessed by a cell imaging method.59 To ensure that cytotoxicity would not mask the observed effects, all concentrations above the inhibitory concentration IC10 causing 10% cytotoxicity were not included in the concentration–response curves of the activation. For hormone receptor-mediated effects and xenobiotic metabolism, the concentrations (in REF) causing 10% of the maximum effect (EC10) were derived. For the adaptive stress response, there is no maximum effect, so the concentration causing an induction ratio of 1.5 (ECIR1.5) was derived instead. All data were evaluated using linear concentration–effect curves as outlined in detail recently.60
2.3.2. Ames fluctuation assays.
The Ames-fluctuation test based on genetically modified Salmonella typhimurium strains TA98 and TA100 was performed to assess the potential of water samples to induce frame-shift mutations and base-pair substitution, respectively.29 The bacterial strains, culture media, and S9 liver enzymes from phenobarbital/β-naphthoflavone-exposed rats were purchased from Xenometrix GmbH (Allschwil, Switzerland). The test was performed as reported previously with minor modifications.61 These modifications included the Salmonella typhimurium strains (TA100 was used here instead of TAmix) and the data treatment (chi-square test was used here instead of a cumulative binomial distribution). Concentrated water samples and procedure controls were tested in duplicate with and without S9 enzyme mix, in two independent experiments. The solvent control (DMSO) and positive controls (in DMSO) were tested in triplicate. The positive controls were: 20 μg mL−1 4-nitroquinoline N-oxide (4-NQO) and 5 μg L−1 2-aminoanthracene (2-AA) for strains TA98 − S9 and TA98 + S9, respectively; 12.5 μg mL−1 nitrofurantoin (NF) and 20 μg mL−1 2-AA for strains TA100 − S9 and TA100 + S9, respectively. 4-NQO and NF were purchased from Sigma-Aldrich (Zwijndrecht, the Netherlands), whereas 2-AA was purchased from Boom (Meppel, the Netherlands).
The REF of the water extracts (in DMSO) in the Ames test was 200, resulting from diluting 6 μL aliquots in a final volume of 300 μl assay medium. Results were expressed as the number of cell culture wells in which a colour change of a pH indicator in the medium was observed. The solvent controls were valid if ≤10 wells showed a colour change of the pH indicator. The positive controls were valid if ≥25 wells showed a colour change of the pH indicator. A chi-square-test was used to determine statistically significant differences (p < 0.05). Test conditions were compared to solvent and SPE blanks (procedure controls) for potential false positive results. Samples were considered mutagenic if a statistically significant response was repeated within independent experiments under at least one of the test conditions.
2.4. Chemical analysis followed by non-target screening
The SPE extracts were analysed with an ultrahigh-performance LC system (Nexera Shimadzu, Den Bosch, the Netherlands) coupled to a maXis 4G high resolution quadrupole time-of-flight HRMS (q-ToF/HRMS) upgraded with a HD collision cell and equipped with an electrospray ionisation source (Bruker Daltonics, Leiderdorp, the Netherlands). Further details on the LC-HRMS method are given in the ESI† (S-2).
NTS of HRMS data was entirely performed with the software patRoon executed within the R statistical environment.62,63patRoon is a comprehensive platform that combines openly available cheminformatics tools for NTS and selected vendor software. Further documentation is available on the GitHub repository.62 The raw LC-HRMS analysis files were converted to a centroided mzML format by using an algorithm available in the HRMS system vendor software Data Analysis (Bruker Daltonics, Wormer, the Netherlands). Processing of the non-target features, i.e. peak-picking, grouping and retention time (tR) alignment, was performed using the OpenMS algorithm within patRoon.64 An absolute intensity threshold of 10
000 was considered for peak picking. Feature groups were defined as unique m/z (comprehensive of carbon isotope signals) and tR pairs occurring in the different sample matrices. A tolerance window of 5 ppm mass accuracy and 20 s tR was considered. Only features present in all replicates and with intensities at least five times higher than in the procedural blanks were subjected to further processing. Protonated ([M + H]+) and deprotonated ([M − H]−) ions were considered for post processing of positive and negative electrospray ionisation mode datasets, respectively. The best molecular formula fitting precursor and product ions was calculated using the GenForm algorithm.65 The MetFrag approach was chosen for tentative annotation of the non-target features.36 Candidate structures with a neutral monoisotopic mass within ±5 ppm of that of the adjusted non-target ions were retrieved from the EPA CompTox Chemicals Dashboard, which was used as the local database (May 2018 version, approx. 760
000 chemicals).66 The structures were fragmented in silico and the fragments were fitted to the experimental HRMS2 spectra using MetFrag. The candidate structures were scored based on the following scoring terms: (i) FragScore: fit of the in silico fragments to the experimental HRMS2 spectra; (ii) MetFusionScore: spectral similarities to the MassBank of North America (MoNA, https://mona.fiehnlab.ucdavis.edu/spectra/search) built within MetFrag with the MetFusion approach;67,68 (iii) individualMoNAscore: spectral similarity by candidate structure InChIKey lookup in MoNA; (iv) ExpoCast: median exposure prediction (in mg per kg body weight per day);43 (v) ToxCastPercentActive: percentage of active hit calls in the ToxCast database; (vi) pubMedReferences: number of literature references in PubMed; (vii) DataSources: data sources on the Dashboard; (viii) CPDatCount: number of consumer products based on the EPA's Chemicals and Products database.69 These eight scoring terms were individually normalised by the highest value found among the proposed candidates and an equal weighting of 1 was used. An additional score of 1 was added for hits in the following lists: (i) SUSDAT: merged list of >40
000 structures from the NORMAN Suspect List Exchange (https://www.norman-network.com/nds/SLE/); (ii) MASSBANK: list of NORMAN compounds on the European MassBank (https://massbank.eu/); (iii) TOXSL21: list of substances included in the TOXSL21 programme; (iv) ToxCast: list of substances included in the ToxCast programme. Finally, a formula score was assigned to candidate structures for which consensus between formulas derived by MetFrag and calculated by GenForm was reached. The formula consensus approach was adopted as GenForm performs an algebraic calculation of the best formula fitting precursor and fragment ions' accurate masses, whereas MetFrag finds the best candidate structure matching the (de)protonated monoisotopic mass used as query, de facto back-calculating formulas of the in silico fragments. Therefore, the two approaches are complementary and their combination can enhance spectra interpretation.
As the main aim of this NTS was to identify, with the highest possible confidence, micropollutants that could have contributed to the observed effects in the bioassays, prioritisation of the tentatively annotated features involved filtering out candidate structures that were not present in the MASSBANK list or for which an individual MoNA score could not be assigned. Evaluation of the results included visual assessment of chromatographic peaks and plots of de-noised HRMS2 spectra, as well as inspection of the MetFrag scores. All tentatively annotated structures were assigned identification confidence levels based on the scale proposed by Schymanski et al.70 Whenever possible, this process was aided by calculation of spectral similarity to records in MoNA or MassBank with the R package OrgMassSpecR.71 Spectral matches were reviewed manually by at least three co-authors for plausibility before a level 2a (accurate mass spectral library match) or level 3 (tentative candidate) annotation was assigned in the final results.
3. Results and discussion
3.1. Reporter gene assays
Only the AhR-CALUX and AREc32 bioassays showed activity, while none of the hormone receptor-mediated effects were induced by the feed water and the RO samples. Concentration–effect curves limited to the assays that showed sufficient activity to allow the derivation of EC10 or ECIR1.5 are provided in the ESI† (S-3) and inhibitory concentrations for cytotoxicity (IC10) and effect concentrations for reporter gene activation (EC10 and ECIR1.5) of individual samples are reported in Table S-4.1.† The cytotoxic concentrations and effect concentrations of the active samples only, i.e. RO feed water (ROF) and RO concentrate (ROC) are plotted in Fig. 1.
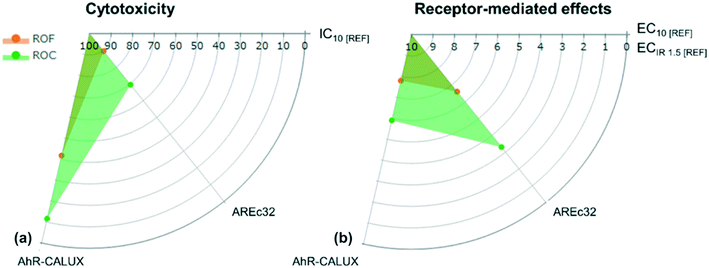 |
| Fig. 1 Radar plots of cytotoxicity (panel a) and receptor-mediated effects (panel b) expressed as IC10 and EC10 and ECIR1.5 in units of REF, respectively, depicting the gene reporter assays where effects were induced. The RO permeate was not plotted for graphic purposes, as it did not induce cytotoxicity nor effects up to REF 100. ROF = reverse osmosis feed, i.e. riverbank filtrate; ROC = reverse osmosis concentrate. | |
The lack of induction of hormone receptor-mediated effects could be rationalised based on the chemistry of the agonists of these receptors in relation to the investigated water matrices. Hormones, despite featuring polar functional groups along their structures, are mostly hydrophobic and thus they are expected to be retained in RBF systems by sorption phenomena.72 Compounds other than hormones have shown the ability to induce androgenic and estrogenic effects,49 thus either such chemicals were not present in the bank filtrate (RO feed water) or they occurred at non-active concentrations within the tested REF range. A recent study observed that RBF could not fully remove estrogenic activity,73 nevertheless in that study a bank filtrate having a travel time of ≈20 days was tested, whereas in our case the travel time of the RBF was on average 30 years. We assumed that a much longer travel time could have maximised hormone removal or dilution to undetectable concentrations.
For ROF, the average IC10 was ≈42 REF in the AhR assay, whereas in the AREc32 assay the IC10 was ≈89 REF. This indicated that the ROF needed to be enriched 42 and 89 times in order to cause a 10% decrease in the viability of the AREc32 and AhR cell lines, respectively. While the IC10 values of ROF were lower in AhR by a factor of 2 compared to AREc32, the greatest difference was observed when the cells were exposed to ROC. In this case, an IC10 of ≈12 REF was quantified for the AhR cell line, whereas for AREc32 the IC10 was ≈70 REF. In line with a previous literature report,57 the AREc32 cell line was more robust and less prone to disturbance by non-specific toxicity. In all cases, the ROP was not cytotoxic within the tested REF range, except in one ambiguous case discussed later in this section, where also receptor-mediated effects were induced. Overall, our results indicated that the ROP was not cytotoxic within the tested REF range up to REF 100.
RO samples and SPE procedural blanks induced xenobiotic metabolism mediated by the AhR. Procedural blanks were active with an average EC10 of ≈72 REF, whereas the ROP samples displayed an average EC10 of ≈69 REF. As these EC10 values were similar, the activity of the ROP was attributed to impurities enriched during sample preparation and not to micropollutants that were able to pass through the RO membranes. EC10 values of ≈8 REF and ≈6 REF were quantified for ROF and ROC, respectively, indicating similar bioactivity of these matrices at low enrichment factors. These results highlight the importance of applying robust barriers against organic micropollutants during drinking water treatment and our study indicates that RO filtration is a suitable barrier to remove potential precursors of carcinogenic compounds. A recent study on groundwater impacted by sewage exfiltration found that deep aquifers used as negative controls were equally active to water from shallow groundwater wells in AhR, ERα and GR assays,74 indicating that some micropollutants caused effects at levels below the limit of detection of their analytical methods. This highlights the importance of obtaining adequate controls and blank samples as well as the ability to discern between the sensitivity of the bioassays and that of the detector used for targeted chemical analysis. In the cited study, the same results were obtained for ERα and GR, whereas in our study neither estrogenic nor glucocorticoid activities were observed.
The toxicity pathway representative of oxidative stress response was induced by ROF and ROC, with ECIR1.5 values ≈6.6 REF and ≈3.3 REF, respectively. Procedural blanks and ROP samples were not active, except for a single ROP replicate, which gave ambiguous results and caused ≈10% reduction in cell viability with a very wide standard error at REF ≈ 100. This sample induced the Nrf2 factor with an ECIR1.5 of ≈60 REF. This effect resulted from an unclear interference, as the remaining three replicates did not induce oxidative stress.
Escher et al.57 used the reporter gene assay AREc32 to investigate water recycling in an Australian advanced water treatment plant (AWTP), which included RO filtration in the treatment train.57 ROF and ROC from that AWTP displayed higher effects with ECIR1.5 of 0.89 REF for ROF and 0.38 REF for ROC, which corresponds to higher activity compared to our samples. This was not surprising as in their case RO was applied to a wastewater pre-treated with ultrafiltration, a membrane process effective against macromolecules of molecular weight ≥1 kDa,75 thus not suitable against micropollutants, whose size usually does not exceed 300–400 Da. Consequently, it is conceivable that the ROF in the Australian AWTP had a higher loading of chemicals.
3.2. Ames tests
The results of the Ames-fluctuation tests for S. typhimurium strains TA98 and TA100 with and without the S9 mix are summarised in Table 1, with plots given in the ESI† (S-5). ROF was genotoxic to strain TA98 − S9, indicating mutagenicity of micropollutants occurring in the bank filtrate non-mediated by the S9 enzyme mix. One ROF replicate induced genotoxicity in strain TA98 + S9, indicating that enzyme-mediated chemical activation resulted in frame-shift mutations in the genome of this particular strain. However, we consider ROF to be non-genotoxic under this condition given the disagreement between replicate tests. Additionally, under condition TA98 + S9 (and TA100 + S9), a decrease of ≈25% viability compared to the control was observed when the strain was exposed to ROF, indicating the non-specific cytotoxicity of organic components enriched from the bank filtrate that may have resulted in false negative results. In all these cases, genotoxic compounds were removed by RO as exposure to ROP extracts did not result in S. typhimurium revertants. For condition TA100 − S9, the genotoxicity of ROF was observed in both experiments; however, this result might be a false positive one given the mutagenic effects induced by one of the procedural blanks while negative controls were not mutagenic. One of the replicate ROP samples was also genotoxic to strain TA100 − S9; however, the effect could not be replicated and may result from impurities introduced during the extraction procedure. It was concluded that while direct genotoxic potential may be present in ROF, ROP was not mutagenic under any of the test conditions. A supporting literature report indicating the mutagenicity of groundwater to S. typhimurium strain TA98 without the S9 enzyme mix was found,76 although in that study the activity was attributed to natural compounds and not to anthropogenic pollutants. Another study on drinking water prepared from Dutch groundwater found that, when present, mutagenic activity was predominantly indirect for strain TA98, i.e. without S9, and that in some cases even drinking water was mutagenic to strain TA98 − S9.77
Table 1 Ames test results of RO samples
|
ROF [REF 200] |
ROP [REF 200] |
Test conditions |
Viability (%) |
Genotoxicity |
Viability (%) |
Genotoxicity |
ROF = RO feed water (riverbank filtrate); ROP = RO permeate; REF = relative enrichment factor; the genotoxicity results of two independent experiments are reported: + = genotoxic; − = non-genotoxic.
One out of two procedural blanks was genotoxic in one replicate experiment, but negative controls were not.
One out of two procedural blanks was genotoxic in one replicate experiment, but negative controls were not.
|
TA98 (−S9) |
122 ± 1 |
Positive (++) |
130 ± 15 |
Negative (−−) |
TA98 (+S9) |
75 ± 20 |
Negative (−+) |
75 ± 19 |
Negative (−−) |
TA100 (−S9) |
107 ± 1 |
Positive (++)a |
110 ± 6 |
Negative (−+)b |
TA100 (+S9) |
75 ± 1 |
Negative (−−) |
93 ± 16 |
Negative (−−) |
3.3. Non-target screening
An overview of the features detected in the ROF (bank filtrate), ROC and ROP is provided in Fig. 2.
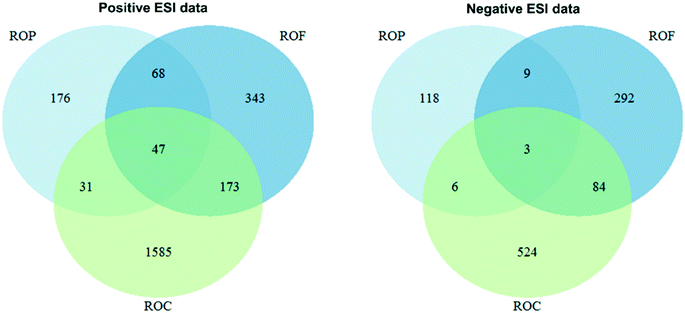 |
| Fig. 2 Venn diagrams of non-target features in samples from the RO drinking water treatment plant detected in positive (left) and negative (right) electrospray ionisation (ESI) datasets. ROF: RO feed water; ROP: RO permeate; ROC: RO concentrate. | |
In total, 2423 and 1036 features were detected in positive and negative electrospray ionisation, respectively, and considered for post processing. The distribution of positive and negative features among the RO water matrices was overall comparable in numbers except for ROC, in which 1836 and 617 positive and negative features were detected, respectively. In general, a higher number of features was expected in ROC as in this matrix the concentrations of solutes would reach levels up to 3.3 times higher than in ROF assuming near-full rejection by RO. The lower number of negative features in ROC might result from ion suppression caused by dissolved organic matter, naturally occurring in this bank filtrate at concentrations around 7–8 mg L−1 and that might have been carried through the extraction to some extent.78 In addition, negative electrospray ionisation efficiency might have been suppressed by acetic acid added to the LC mobile phase as a modifier. Lastly, as excellent rejection of inorganic ions can be achieved by RO,50 different adducts could have been formed in the ROC samples analysed in positive electrospray ionosation mode, possibly explaining the higher number of positive features in this matrix. As shown in Fig. 2, only about 2/3 and 1/3 of the features detected in ROF were also found in the positive and negative ionisation ROC data, respectively. This might result from matrix effects, such as ion suppression, which might have affected both ionisation and extraction efficiency in ROC. Additionally, in ROC we encountered some instances in which early eluting features fell out of the 20 s tolerance window used to group features across water matrices, resulting in a given m/z being assigned to two different feature groups and thus not overlapping between ROF and ROC. This behaviour was not investigated further as these features were nonetheless considered for tentative identification if they complied with the prioritisation criteria. Based on the physicochemical properties behind incomplete chemical removal by RO, it could be assumed that most features detected in ROP, which were overall comparable between the positive and negative datasets, were either small and hydrophilic uncharged compounds, small cationic compounds or uncharged (moderately) hydrophobic compounds exhibiting polar groups ionisable by HRMS.13 Features occurring only in ROP might have been undetectable elsewhere due to matrix effects or some of them might have even leached from the RO system. An overview of the m/z values and retention time of the features detected in the different water matrices is provided in the ESI† (Fig. S-6.1).
Among the detected features, 1528 positive and 833 negative ions from all sample matrices were assigned to tentative structures by MetFrag. In the positive data, 53 tentatively annotated structures were present in the MassBank list, 24 of which were similar to spectra in MoNA. Additionally, 13 structures not present in the MassBank list were similar to records in MoNA. In the negative data, 28 candidate structures were similar to records in MoNA, 2 of which were also present in the MassBank list. All other structures were not found in spectral libraries and did not have associated bioactivity metadata. The InChIKey identifiers of candidates that exhibited chromatograms of good quality and plausible HRMS2 annotation and that would likely ionise in electrospray ionisation-HRMS analysis (e.g., neutral polar and ionic organics) were used to query MoNA and the European MassBank. Similarities to relevant spectra were calculated. This approach resulted in the tentative identification of 25 and 24 candidate structures in the positive and negative data, respectively. Analysis of reference standards led to confirmation (level 1 identification) of 2,6-dichlorobenzamide, phenazone and trimethyl phosphate in the positive electrospray ionisation data, whereas bentazone and acesulfame were confirmed in the negative electrospray ionisation data. Supporting spectral library evidence, shown in the ESI† (S-8) and indicated here in parentheses next to the compound name, was found for 16 structures. In the positive data, 2-phenylethylamine (Fig. S-8.1†), benzisothiazolinone (Fig. S-8.4†), diethyl phosphate (Fig. S-8.5†), diphenylphosphinic acid (Fig. S-8.9†), and triphenylphosphine oxide (Fig. S-8.10†) were assigned identification confidence level 2a, the highest possible without reference standards. Anthranilic acid (Fig. S-8.2†), 4-hydroxybenzoic acid (Fig. S-8.3†) and fusaric acid (Fig. S-8.6†) could not be identified with confidence higher than level 3 despite the good match with library spectra, as other isomers could not be ruled out. In the case of the triazine TPs 2-hydroxysimazine (Fig. S-8.7†) and 2-hydroxyatrazine (Fig. S-8.8†), level 3 was assigned despite good spectral similarity due to (quasi-)isobaric interferences in the experimental HRMS2 data. In the negative data, acamprosate (Fig. S-8.13†), saccharin (Fig. S-8.14†) and mecoprop (Fig. S-8.16†) were assigned level 2a, whereas catechol (Fig. S-8.11†), mandelic acid (Fig. S-8.12†) and 2-naphthalenesulfonic acid (Fig. S-8.15†) could not be assigned a higher level than 3 as other isomers could not be ruled out. All level 2a were assigned based on matching spectra available on MoNA or MassBank, except diphenylphosphinic acid and saccharin, for which spectra measured in-house were used instead. For compounds identified as level 3 with supporting library spectra, it is important to stress the benefits of establishing a harmonised LC method for NTS in order to use a retention index, which could have increased confidence in the identification of isomers. The chemicals (tentatively) identified with the highest confidence having bioactivity metadata matching the endpoints covered by the bioassay test battery are listed in Table 2. In the ESI† (S-7) the complete lists of (tentatively) identified structures in the positive (Table S-7.1†) and negative electrospray ionisation datasets (Table S-7.2†) are provided.
Table 2 List of (tentatively) identified structures, their identification confidence level (ICL), relevant bioactivity metadata and sample matrix in which they were detected
Compound |
DTXSIDa |
Formula |
Class |
ESI modeb |
ICLc |
Endpoints with AC50d (μM) |
ToxCast active (%) |
Sample matrixe |
Unique identifiers of substances deposited in the EPA CompTox Chemicals Dashboard. The DTXSIDs can be used to access bioactivity data. For example, for benzisothiazolinone, visit the following URL: https://comptox.epa.gov/dashboard/dsstoxdb/results?search=DTXSID5032523#bioactivity.
ESI = electrospray ionisation; detected adduct: + = [M + H]+; − = [M − H]−.
Identification confidence level.70
Data from the EPA CompTox Chemicals Dashboard, limited to the reporter gene assays that were similar to those included in the test battery used for this study. AC50: active concentration in μM causing 50% of the effects.
Sample matrix in which the compound was (tentatively) identified. ROF: reverse osmosis feed water (riverbank filtrate); ROC: reverse osmosis concentrate; ROP: reverse osmosis permeate;1 supporting library evidence found, but insufficient to rule out other isomers;2 reference spectrum previously measured in-house.
|
Benzisothiazolinone |
DTXSID5032523 |
C7H5NOS |
Herbicide |
+ |
2a |
Nrf2 induction (5.8) |
30.6 |
ROF, ROC, ROP |
2,6-Dichlorobenzamide |
DTXSID7022170 |
C7H5Cl2NO |
Herbicide metabolite |
+ |
1 |
AhR induction (60.6) |
1.8 |
ROF, ROC |
4-Hydroxybenzoic acid |
DTXSID3026647 |
C7H6O3 |
Natural and industrial |
+/− |
31 |
AhR induction (49.2); ERα induction (57.2) |
1.3 |
ROF, ROC |
Triphenylphosphine oxide |
DTXSID2022121 |
C18H15OP |
Industrial |
+ |
2a |
Nrf2 induction (40.3) |
1.8 |
ROF, ROC, ROP |
Acamprosate |
DTXSID6047529 |
C5H11NO4S |
Pharmaceutical |
− |
2a |
Nrf2 induction (43.6) |
1.8 |
ROF, ROC |
Bentazone |
DTXSID0023901 |
C10H12N2O3S |
Herbicide |
− |
1 |
Nrf2 induction (32.1) |
3.3 |
ROF, ROC |
Catechol |
DTXSID3020257 |
C6H6O2 |
Natural and industrial |
− |
31 |
Nrf2 induction (12.4); AhR induction (57.2); ERα induction (71–84) |
14.1 |
ROF, ROC |
Mecoprop |
DTXSID9024194 |
C10H11ClO3 |
Herbicide |
− |
2a |
AhR induction (30.3); PPARγ induction (85.3) |
0.6 |
ROF, ROC |
Naphthalene-2-sulfonic acid |
DTXSID5044788 |
C10H8O3S |
Industrial |
− |
31 |
AhR induction (40.3) |
2.0 |
ROF, ROC |
Saccharin |
DTXSID5021251 |
C7H5NO3S |
Sweetener |
− |
2a2 |
AhR induction (43.4) |
1.3 |
ROF, ROC |
3.4. Bioactivity of the (tentatively) identified micropollutants
ToxCast data in the EPA Chemicals Dashboard indicated that 2,6-dichlorobenzamide (BAM) activated a similar AhR bioassay with an AC50 (active concentration causing 50% of the effects) of 60.6 μM. Based on a concentration of 39 ± 2 ng L−1 quantified in a bank filtrate from the same RBF system that was fed to the full-scale RO treatment plant,79 BAM can make only a minor contribution to the activation of AhR observed in the present work. As chlorobenzamides are potentially mutagenic,80,81 BAM might have contributed to the genotoxicity characterised in ROF with the Ames tests. This chemical was not detected in the ROP, which is in line with previous studies from our group,53 where BAM displayed less than 1% passage in a pilot-scale RO drinking water treatment.
Amongst the compounds tentatively identified with supporting library evidence, ToxCast data showed that 4-hydroxybenzoic acid, catechol, mecoprop, naphthalene-2-sulfonic acid and saccharin (all detected in ROF and ROC) can activate similar assays based on the AhR gene reporter. Based on the acid dissociation constant (pKa) of 4-hydroxybenzoic acid (pKa = 4.6), mecoprop (pKa = 3.7) and naphthalene-2-sulfonic (pKa < 1), these chemicals would occur in ROF as dissociated acids as the pH value of this water matrix is ≈7, additionally supporting their occurrence in the bank filtrate16 and their lack of detection in the ROP.13 Mecoprop was identified with the highest possible confidence without a reference standard, i.e. level 2a, based on matching spectral records on MoNA and the presence of distinctive isotopic peaks in both HRMS1 and HRMS2 experimental data. ToxCast data indicated that mecoprop elicited effects in a PPARγ assay with an AC50 nearly 3 times higher, thus less toxic, than that of AhR. Although we did not measure the environmental concentrations of micropollutants, it would be plausible that mecoprop would not occur at levels high enough to induce PPARγ-mediated effects. This compound is a household herbicide that has been frequently detected in European WWTP effluents at concentrations up to 2.2 μg L−1.82 Mecoprop is not retained by RBF systems, leaving biodegradation as the sole option of attenuation. Although evidence of degradation in oxic RBF systems exists,83 mecoprop is persistent under anoxic conditions.84 Its lack of detection in the ROP is in line with the high removal efficiency by RO reported in the literature, which was higher than 97%.85 Mecoprop was found to be non-mutagenic to S. typhimurium strains TA98 and TA100 with and without the S9 enzyme.86 Saccharin is an artificial sweetener ubiquitously detected along with acesulfame (confirmed in ROF and ROC), both indicators of the impact of domestic wastewater on natural waters as they are added in high amounts to food and beverages.87 Because these sweeteners occur in an anionic form at pH values of natural waters, they have high mobility potential in the sub-surface.88 Their negative charge can explain their detection in the RBF system and their lack of detection in the RO permeate. The latter is in line with literature data, which reported more than 90% removal by RO for both compounds.53,89 ToxCast data indicated that saccharin induced effects in an AhR assay with an AC50 of 43.4 μM, whereas data for acesulfame were not found. Both sweeteners were not genotoxic to the S. typhimurium strain TA100 with and without the S9 enzyme.90
ToxCast data for bentazone indicated its ability to induce the Nrf2 transcription factor with an AC50 of 32.1 μM. In line with literature data,53,85 this chemical is well removed by RO as it was not detected in the ROP. Bentazone was identified in 32% of European groundwater and is currently approved for use in the EU.2 Bentazone was not mutagenic to the S. typhimurium strains TA98 and TA100 with and without the S9 enzyme mix.86 Among the tentatively identified chemicals, bioactivity data of benzisothiazolinone, acamprosate, catechol and triphenylphosphine oxide showed that these chemicals can induce the Nrf2 transcription factor. Benzisothiazolinone was the tentatively identified compound with the lowest AC50 (5.82 μM in Nrf2 assay) and the highest ToxCast percent active bioassays (31%). In a previous study with the AhR-CALUX assay used here, this chemical was not active below cytotoxic concentrations.49 Although this biocide is removed by wastewater sludge,91 indications of high groundwater contamination potential were found,92 further supporting its tentative identification in the RBF system. Triphenylphosphine oxide is a persistent and toxic industrial chemical released into surface waters via wastewater effluents.93 A monitoring study on groundwater from various sources in the Netherlands found that triphenylphosphine oxide was more frequently detected in bank filtrate and confined groundwater, corroborating its tentative identification in the RO feed water.94 Acamprosate is the active ingredient of a pharmaceutical product to treat alcohol dependence, so far not detected in the environment, but indicated as a potential drinking water contaminant.95 This chemical is anionic at any natural pH value, it is not metabolised by the human body and thus it is excreted unchanged following therapeutic administration.96 This suggests that acamprosate may be released into surface waters via domestic wastewater effluents and may pass through the riverbank, reaching groundwater and exhibiting mobility in the sub-surface if not biodegraded. Given the lack of further environmentally relevant information, its inclusion in future suspect screenings is recommended.
It is noteworthy that although neither effects nor genotoxicity were observed for the ROP, benzisothiazolinone, trimethyl phosphate and triphenylphosphine oxide were the only (tentatively) identified compounds in the RO permeate. Benzisothiazolinone (151.0092 Da), trimethyl phosphate (140.0238 Da) and triphenylphosphine oxide (278.0861 Da) are compounds whose physicochemical properties confer critical behaviour in RO filtration. Benzisothiazolinone has a pKa of 9.5, and thus occurred as a neutral species in ROF, whereas trimethyl phosphate is always uncharged as its structure has no atoms that can be ionised. Benzisothiazolinone has a predicted log octanol–water partition coefficient (log
Kow) of 1.02, whereas trimethyl phosphate has an experimental log
Kow of −0.65. Thus, both chemicals are hydrophilic, exhibit no affinity for the aromatic polyamide of which the separation layer of RO membranes is made and remain dissolved in water, being able to pass through the RO membranes due to their small size. Triphenylphosphine oxide, instead, is also uncharged, but exhibits a log
Kow of 2.83. Despite its larger size, this relatively hydrophobic chemical displays affinity for the aromatic polyamide active layer and likely undergoes adsorption followed by solution–diffusion, resulting in breaking through the permeate side. Based on ToxCast data, it can be assumed that the concentrations of benzisothiazolinone and triphenylphosphine oxide were too low to trigger oxidative stress even after enrichment of the ROP samples. Nevertheless, as these chemicals were not fully removed, they should be quantitatively monitored in RO drinking water treatment processes as higher feed water concentrations might result in potentially toxic concentrations in the ROP.
4. Conclusions
RO filtration directly applied to a raw riverbank filtrate in full-scale drinking water treatment was capable of producing potable water that did not induce any detectable adverse effects in the applied EBM battery. Toxicity pathways representative of xenobiotic metabolism, adaptive stress response and genotoxicity were activated by enriched bank filtrate. For the gene reporter assays, it would take no more than 6- to 8-fold concentration of this ROF to induce cellular toxicity pathways. The possible role of RBF in attenuating endocrine disrupting compounds was shown based on the lack of hormone receptor-mediated effects observed when RO feed water was tested. The water investigated in this study originated from anthropogenically impacted surface waters (i.e., the Lower Rhine), and the suitability of RBF as a drinking water pre-treatment seems confirmed. The bioanalytical tools used in this study indicated that RO is highly effective in removing chemicals that can induce specific and non-specific potentially toxic effects. Applying non-target screening relying on open cheminformatics tools and on an openly accessible chemical database aided the (tentative) identification of these micropollutants, while health-relevant chemical metadata could explain the biological activity observed with effect-based methods for a subset of (tentatively) identified structures. Further confirmation activities and quantification to link chemical and bioassay results will be the scope of follow-up work. As for quantification of compound concentrations in water samples, a complete validation study of the SPE method should be conducted for all investigated matrices to obtain recovery values, which are currently unknown. Testing the individual chemicals with a new test bioassay battery covering the same endpoints investigated in this study would then be necessary to confidently determine the contribution of each confirmed structure to the total observed effects. The tentatively identified structures may be monitored actively in future studies, for which reference standards should be obtained for higher confidence. Overall, the identification confidence and success rate could be improved further in the future by increasing the number of accurate mass spectra deposited in open libraries. Although the approach undertaken in this study is not meant to replace the use of reference compounds in both biological and chemical analyses, it demonstrates the potential of the employed methods to generate useful, real-world data about drinking water quality, increasing the knowledge about the occurrence of chemicals in the environment and their behaviour in drinking water treatment. Additionally, the potential of elucidating chemical structures behind biological activities by non-target screening can be useful to derive cause–effect relationships.
Conflicts of interest
There are no conflicts of interest to declare.
Acknowledgements
The work at IBED, University of Amsterdam and part of the work at KWR Watercycle Research Institute were financially supported by the drinking water company Oasen (Gouda, the Netherlands) via the ECROS project. The work at UFZ Leipzig, Eawag and part of the work at KWR Watercycle Research Institute were supported by the EU FP7 project SOLUTIONS (grant number 603437). The work at LCSB (University of Luxembourg) was supported by the FNR (grant number 12341006). Evgeni Alaminov at Oasen is acknowledged for facilitating the sampling at the De Hooge Boom drinking water treatment plant in Woerden, the Netherlands. The robotic platforms, on which the reporter gene assays were performed, are part of the major infrastructure initiative CITEPro (Chemicals in the Terrestrial Environment Profiler) funded by the Helmholtz Association. Astrid Reus (KWR Watercycle Research Institute) is acknowledged for providing additional information on the Ames testing procedure. The two anonymous reviewers are acknowledged for their useful comments.
References
- R. P. Schwarzenbach, B. I. Escher, K. Fenner, T. B. Hofstetter, C. A. Johnson and U. von Gunten,
et al., The Challenge of Micropollutants in Aquatic Systems, Science, 2006, 313(5790), 1072–1077 CrossRef CAS . Available from: http://science.sciencemag.org/content/313/5790/1072.abstract.
- R. Loos, G. Locoro, S. Comero, S. Contini, D. Schwesig and F. Werres,
et al., Pan-European survey on the occurrence of selected polar organic persistent pollutants in ground water, Water Res., 2010, 44(14), 4115–4126 CrossRef CAS.
- D. W. Kolpin, E. T. Furlong, M. T. Meyer, E. M. Thurman, S. D. Zaugg and L. B. Barber,
et al., Pharmaceuticals, Hormones, and Other Organic Wastewater Contaminants in U.S. Streams, 1999−2000: A National Reconnaissance, Environ. Sci. Technol., 2002, 36(6), 1202–1211, DOI:10.1021/es011055j.
- E. T. Furlong, A. L. Batt, S. T. Glassmeyer, M. C. Noriega, D. W. Kolpin and H. Mash,
et al., Nationwide reconnaissance of contaminants of emerging concern in source and treated drinking waters of the United States: Pharmaceuticals, Sci. Total Environ., 2017, 579, 1629–1642 CrossRef CAS PubMed . Available from: http://www.sciencedirect.com/science/article/pii/S0048969716305551.
- T. Reemtsma, U. Berger, H. P. H. Arp, H. Gallard, T. P. Knepper and M. Neumann,
et al., Mind the Gap: Persistent and Mobile Organic Compounds—Water Contaminants That Slip Through, Environ. Sci. Technol., 2016, 50(19), 10308–10315, DOI:10.1021/acs.est.6b03338.
- M. Schriks, M. B. Heringa, M. M. E. van der Kooi, P. de Voogt and A. P. van Wezel, Toxicological relevance of emerging contaminants for drinking water quality, Water Res., 2010, 44(2), 461–476 CrossRef CAS . Available from: http://www.sciencedirect.com/science/article/pii/S0043135409005429.
- W. Brack, R. Altenburger, G. Schüürmann, M. Krauss, D. López Herráez and J. van Gils,
et al., The SOLUTIONS project: Challenges and responses for present and future emerging pollutants in land and water resources management, Sci. Total Environ., 2015, 503, 22–31 CrossRef.
- J. Radjenović, M. Petrović, F. Ventura and D. Barceló, Rejection of pharmaceuticals in nanofiltration and reverse osmosis membrane drinking water treatment, Water Res., 2008, 42(14), 3601–3610 CrossRef.
- B. I. Escher, M. Lawrence, M. Macova, J. F. Mueller, Y. Poussade and C. Robillot,
et al., Evaluation of Contaminant Removal of Reverse Osmosis and Advanced Oxidation in Full-Scale Operation by Combining Passive Sampling with Chemical Analysis and Bioanalytical Tools, Environ. Sci. Technol., 2011, 45(12), 5387–5394, DOI:10.1021/es201153k.
- T. Fujioka, S. J. Khan, Y. Poussade, J. E. Drewes and L. D. Nghiem, N-nitrosamine removal by reverse osmosis for indirect potable water reuse – A critical review based on observations from laboratory-, pilot- and full-scale studies, Sep. Purif. Technol., 2012, 98, 503–515 CrossRef CAS.
- R. J. Petersen, Composite reverse osmosis and nanofiltration membranes, J. Membr. Sci., 1993, 83(1), 81–150 CrossRef CAS . Available from: http://www.sciencedirect.com/science/article/pii/037673889380014O.
- J. Wang, D. S. Dlamini, A. K. Mishra, M. T. M. Pendergast, M. C. Y. Wong and B. B. Mamba,
et al., A critical review of transport through osmotic membranes, J. Membr. Sci., 2014, 454, 516–537 CrossRef CAS . Available from: http://linkinghub.elsevier.com/retrieve/pii/S0376738813009873.
- C. Bellona, J. E. Drewes, P. Xu and G. Amy, Factors affecting the rejection of organic solutes during NF/RO treatment—a literature review, Water Res., 2004, 38(12), 2795–2809 CrossRef CAS.
- M. Garfí, E. Cadena, D. Sanchez-Ramos and I. Ferrer, Life cycle assessment of drinking water: comparing
conventional water treatment, reverse osmosis and mineral water in glass and plastic bottles, J. Cleaner Prod., 2016, 137, 997–1003 CrossRef.
-
W. G. J. Van der Meer, “Het Drinkwaterbedrijf van de Toekomst?” [Internet], Inaugural lecture at the TU Delft, The Netherlands, 2013. Available from: http://resolver.tudelft.nl/uuid:7844ed04-f447-4b01-ac42-d3c665050f81 Search PubMed.
- J. Hollender, J. Rothardt, D. Radny, M. Loos, J. Epting and P. Huggenberger,
et al., Comprehensive micropollutant screening using LC-HRMS/MS at three riverbank filtration sites to assess natural attenuation and potential implications for human health, Water Res., 2018, 1, 100007 Search PubMed . Available from: http://www.sciencedirect.com/science/article/pii/S2589914718300070.
- C. Hoppe-Jones, G. Oldham and J. E. Drewes, Attenuation of total organic carbon and unregulated trace organic chemicals in U.S. riverbank filtration systems, Water Res., 2010, 44(15), 4643–4659 CrossRef CAS . Available from: https://www.sciencedirect.com/science/article/pii/S0043135410003994?via%3Dihub.
- S. Huntscha, D. M. Rodriguez Velosa, M. H. Schroth and J. Hollender, Degradation of polar organic micropollutants during riverbank filtration: Complementary results from spatiotemporal sampling and push-pull tests, Environ. Sci. Technol., 2013, 47(20), 11512–11521 CrossRef CAS.
- N. Tufenkji, J. N. Ryan and M. Elimelech, Peer Reviewed: The Promise of Bank Filtration, Environ. Sci. Technol., 2002, 36(21), 422A–428A, DOI:10.1021/es022441j.
- D. A. Umar, M. F. Ramli, A. Z. Aris, W. N. A. Sulaiman, N. U. Kura and A. I. Tukur, An overview assessment of the effectiveness and global popularity of some methods used in measuring riverbank filtration, J. Hydrol., 2017, 550, 497–515 CrossRef CAS . Available from: https://www.sciencedirect.com/science/article/pii/S0022169417303086.
- C. Bertelkamp, J. Reungoat, E. R. Cornelissen, N. Singhal, J. Reynisson and A. J. Cabo,
et al., Sorption and biodegradation of organic micropollutants during river bank filtration: A laboratory column study, Water Res., 2014, 52, 231–241 CrossRef CAS . Available from: https://www.sciencedirect.com/science/article/pii/S0043135413008944.
- C. Bertelkamp, A. R. D. Verliefde, K. Schoutteten, L. Vanhaecke, J. Vanden Bussche and N. Singhal,
et al. The effect of redox conditions and adaptation time on organic micropollutant removal during river bank filtration: A laboratory-scale column study, Sci. Total Environ., 2016, 544, 309–318 CrossRef CAS . Available from: https://www.sciencedirect.com/science/article/pii/S0048969715310160.
- C. K. Schmidt, F. T. Lange and H.-J. Brauch, Characteristics and evaluation of natural attenuation processes for organic micropollutant removal during riverbank filtration, Water Sci. Technol.: Water Supply, 2007, 7(3), 1–7 CAS.
- W. Brack, S. A. Aissa, T. Backhaus, V. Dulio, B. I. Escher and M. Faust,
et al., Effect-based methods are key. The European Collaborative Project SOLUTIONS recommends integrating effect-based methods for diagnosis and monitoring of water quality, Environ. Sci. Eur., 2019, 31(1), 10, DOI:10.1186/s12302-019-0192-2.
- R. Altenburger, W. Brack, R. M. Burgess, W. Busch, B. I. Escher and A. Focks,
et al., Future water quality monitoring: improving the balance between exposure and toxicity assessments of real-world pollutant mixtures, Environ. Sci. Eur., 2019, 31(1), 12, DOI:10.1186/s12302-019-0193-1.
-
B. Escher and F. Leusch, Bioanalytical Tools in Water Quality Assessment, IWA Publishing, 2012 Search PubMed.
- A.-S. Wernersson, M. Carere, C. Maggi, P. Tusil, P. Soldan and A. James,
et al., The European technical report on aquatic effect-based monitoring tools under the water framework directive, Environ. Sci. Eur., 2015, 27(1), 7 CrossRef.
- J. C. Chang, P. B. Taylor and F. R. Leach, Use of the Microtox®assay system for environmental samples, Bull. Environ. Contam. Toxicol., 1981, 26(1), 150–156 CrossRef CAS.
- B. Ames, J. Mccann and E. Yamasaki, Methods for detecting carcinogens and mutagens with the Salmonella mammalian microsome mutagenicity test, Mutat. Res., 1975, 31, 347–364 CAS . Available from: https://ci.nii.ac.jp/naid/10007377646/en/.
- S. Jobling, M. Nolan, C. R. Tyler, G. Brighty and J. P. Sumpter, Widespread Sexual Disruption in Wild Fish, Environ. Sci. Technol., 1998, 32(17), 2498–2506, DOI:10.1021/es9710870.
- B. I. Escher, M. Allinson, R. Altenburger, P. A. Bain, P. Balaguer and W. Busch,
et al., Benchmarking Organic Micropollutants in Wastewater, Recycled Water and Drinking Water with In Vitro Bioassays, Environ. Sci. Technol., 2014, 48(3), 1940–1956, DOI:10.1021/es403899t.
- M. Krauss, H. Singer and J. Hollender, LC–high resolution MS in environmental analysis: from target screening to the identification of unknowns, Anal. Bioanal. Chem., 2010, 397(3), 943–951, DOI:10.1007/s00216-010-3608-9.
- E. L. Schymanski, H. P. Singer, P. Longrée, M. Loos, M. Ruff and M. A. Stravs,
et al., Strategies to Characterize Polar Organic Contamination in Wastewater: Exploring the Capability of High Resolution Mass Spectrometry, Environ. Sci. Technol., 2014, 48(3), 1811–1818, DOI:10.1021/es4044374.
- J. Hollender, E. L. Schymanski, H. P. Singer and P. L. Ferguson, Nontarget Screening with High Resolution Mass Spectrometry in the Environment: Ready to Go?, Environ. Sci. Technol., 2017, 51(20), 11505–11512, DOI:10.1021/acs.est.7b02184.
- K. Dührkop, K. Scheubert and S. Böcker, Molecular formula identification with SIRIUS, Metabolites, 2013, 3(2), 506–516 CrossRef PubMed.
- C. Ruttkies, E. L. Schymanski, S. Wolf, J. Hollender and S. Neumann, MetFrag relaunched: incorporating strategies beyond in silico fragmentation, Aust. J. Chem., 2016, 8, 3, DOI:10.1186/s13321-016-0115-9.
- A. Gindulyte, B. A. Shoemaker, B. Yu, G. Fu, J. He and J. Zhang,
et al., PubChem Substance and Compound databases, Nucleic Acids Res., 2015, 44(D1), D1202–D1213, DOI:10.1093/nar/gkv951.
- E. L. Schymanski, C. Ruttkies, M. Krauss, C. Brouard, T. Kind and K. Dührkop,
et al., Critical Assessment of Small Molecule Identification 2016, automated methods, J. Cheminf., 2017, 9(1), 22, DOI:10.1186/s13321-017-0207-1.
- A. J. Williams, C. M. Grulke, J. Edwards, A. D. McEachran, K. Mansouri and N. C. Baker,
et al., The CompTox Chemistry Dashboard: a community data resource for environmental chemistry, Aust. J. Chem., 2017, 9(1), 61, DOI:10.1186/s13321-017-0247-6.
- A. M. Richard and C. R. Williams, Distributed structure-searchable toxicity (DSSTox) public database network: a proposal, Mutat. Res., Fundam. Mol. Mech. Mutagen., 2002, 499(1), 27–52 CrossRef CAS . Available from: http://www.sciencedirect.com/science/article/pii/S0027510701002895.
- R. Kavlock, K. Chandler, K. Houck, S. Hunter, R. Judson and N. Kleinstreuer,
et al., Update on EPA's ToxCast Program: Providing High Throughput Decision Support Tools for Chemical Risk Management, Chem. Res. Toxicol., 2012, 25(7), 1287–1302, DOI:10.1021/tx3000939.
- R. R. Tice, C. P. Austin, R. J. Kavlock and J. R. Bucher, Improving the Human Hazard Characterization of Chemicals: A Tox21 Update, Environ. Health Perspect., 2013, 121(7), 756–765, DOI:10.1289/ehp.1205784.
- J. F. Wambaugh, R. W. Setzer, D. M. Reif, S. Gangwal, J. Mitchell-Blackwood and J. A. Arnot,
et al., High-Throughput Models for Exposure-Based Chemical Prioritization in the ExpoCast Project, Environ. Sci. Technol., 2013, 47(15), 8479–8488, DOI:10.1021/es400482g.
- A. D. McEachran, K. Mansouri, C. Grulke, E. L. Schymanski, C. Ruttkies and A. J. Williams, “MS-Ready” structures for non-targeted high-resolution mass spectrometry screening studies, Aust. J. Chem., 2018, 10(1), 45 Search PubMed . Available from: https://www.ncbi.nlm.nih.gov/pubmed/30167882.
- A. D. McEachran, J. R. Sobus and A. J. Williams, Identifying known unknowns using the US EPA's CompTox Chemistry Dashboard, Anal. Bioanal. Chem., 2017, 409(7), 1729–1735, DOI:10.1007/s00216-016-0139-z.
- M. Verweij, The remarkable restoration of the Rhine: plural rationalities in regional water politics, Water Int., 2017, 42(2), 207–221 CrossRef.
- J. Hollender, M. Bourgin, K. B. Fenner, P. Longree, C. S. McArdell and C. Moschet,
et al., Exploring the Behaviour of Emerging Contaminants in the Water Cycle using the Capabilities of High Resolution Mass Spectrometry, Chimia, 2014, 68(11), 793–798 CrossRef CAS PubMed.
- M. Ruff, M. S. Mueller, M. Loos and H. P. Singer, Quantitative target and systematic non-target analysis of polar organic micro-pollutants along the river Rhine using high-resolution mass-spectrometry – Identification of unknown sources and compounds, Water Res., 2015, 87, 145–154 CrossRef CAS.
- P. A. Neale, R. Altenburger, S. Aït-Aïssa, F. Brion, W. Busch and G. de Aragão Umbuzeiro,
et al., Development of a bioanalytical test battery for water quality monitoring: Fingerprinting identified micropollutants and their contribution to effects in surface water, Water Res., 2017, 123, 734–750 CrossRef CAS . Available from: http://www.sciencedirect.com/science/article/pii/S0043135417305894.
- K. P. Lee, T. C. Arnot and D. Mattia, A review of reverse osmosis membrane materials for desalination—Development to date and future potential, J. Membr. Sci., 2011, 370(1–2), 1–22 CrossRef CAS . Available from: https://www.sciencedirect.com/science/article/pii/S0376738810010045.
- T. Fujioka, S. J. Khan, J. A. McDonald and L. D. Nghiem, Validating the rejection of trace organic chemicals by reverse osmosis membranes using a pilot-scale system, Desalination, 2015, 358, 18–26 CrossRef CAS . Available from: http://linkinghub.elsevier.com/retrieve/pii/S0011916414006365.
- V. Yangali-Quintanilla, S. K. Maeng, T. Fujioka, M. Kennedy and G. Amy, Proposing nanofiltration as acceptable barrier for organic contaminants in water reuse, J. Membr. Sci., 2010, 362(1), 334–345 CrossRef CAS.
- V. Albergamo, B. Blankert, E. R. Cornelissen, B. Hofs, W.-J. Knibbe and W. van der Meer,
et al., Removal of polar organic micropollutants by pilot-scale reverse osmosis drinking water treatment, Water Res., 2019, 148, 535–545 CrossRef CAS . Available from: http://www.sciencedirect.com/science/article/pii/S0043135418307449.
- J. Nivala, P. A. Neale, T. Haasis, S. Kahl, M. König and R. A. Müller,
et al., Application of cell-based bioassays to evaluate treatment efficacy of conventional and intensified treatment wetlands, Environ. Sci.: Water Res. Technol., 2018, 4(2), 206–217, 10.1039/C7EW00341B.
- M. König, B. I. Escher, P. A. Neale, M. Krauss, K. Hilscherová and J. Novák,
et al., Impact of untreated wastewater on a major European river evaluated with a combination of in vitro bioassays and chemical analysis, Environ. Pollut., 2017, 220, 1220–1230 CrossRef PubMed . Available from: http://www.sciencedirect.com/science/article/pii/S0269749116307758.
- P. A. Neale and B. I. Escher, In vitro bioassays to assess drinking water quality, Current Opinion in Environmental Science & Health, 2018, 7, 1–7 Search PubMed . Available from: http://www.sciencedirect.com/science/article/pii/S24685844183003944.
- B. I. Escher, M. Dutt, E. Maylin, J. Y. M. Tang, S. Toze and C. R. Wolf,
et al., Water quality assessment using the AREc32 reporter gene assay indicative of the oxidative stress response pathway, J. Environ. Monit., 2012, 14(11), 2877–2885 RSC.
- X. J. Wang, J. D. Hayes and C. R. Wolf, Generation of a stable antioxidant response element--driven reporter gene cell line and its use to show redox-dependent activation of Nrf2 by cancer chemotherapeutic agents, Cancer Res., 2006, 66(22), 10983–10994 CrossRef CAS PubMed.
- B. I. Escher, L. Glauch, M. König, P. Mayer and R. Schlichting, Baseline Toxicity and Volatility Cutoff in Reporter Gene Assays Used for High-Throughput Screening, Chem. Res. Toxicol., 2019, 32(8), 1646–1655 Search PubMed.
- B. I. Escher, P. A. Neale and D. L. Villeneuve, The advantages of linear concentration-response curves for in vitro bioassays with environmental samples, Environ. Toxicol. Chem., 2018, 37(9), 2273–2280 CrossRef CAS PubMed.
- M. B. Heringa, D. J. H. Harmsen, E. F. Beerendonk, A. A. Reus, C. A. M. Krul and D. H. Metz,
et al., Formation and removal of genotoxic activity during UV/H2O2--GAC treatment of drinking water, Water Res., 2011, 45(1), 366–374 CrossRef CAS.
-
R. Helmus, patRoon R package, 2018. Available from: https://github.com/rickhelmus/patRoon Search PubMed.
-
R Core Team, R: A Language and Environment for Statistical Computing. Found Stat Comput Vienna, Austria URL https://wwwR-project.org/, 2017 Search PubMed.
- H. L. Röst, T. Sachsenberg, S. Aiche, C. Bielow, H. Weisser and F. Aicheler,
et al., OpenMS: a flexible open-source software platform for mass spectrometry data analysis, Nat. Methods, 2016, 13, 741, DOI:10.1038/nmeth.3959.
- M. Meringer, S. Reinker, J. Zhang and A. Muller, MS/MS data improves automated determination of molecular formulas by mass spectrometry, MATCH, 2011, 65(2), 259–290 CAS.
-
EPA's National Center for Computational Toxicology, CompTox Chemicals Dashboard Metadata Files for Integration with MetFrag. figshare. Dataset, 2018.
-
Mass Bank of North America (MoNA), The Fiehn laboratory at UC Davis. Available from: http://mona.fiehnlab.ucdavis.edu/ Search PubMed.
- M. Gerlich and S. Neumann, MetFusion: integration of compound identification strategies, J. Mass Spectrom., 2013, 48(3), 291–298, DOI:10.1002/jms.3123.
- K. L. Dionisio, K. Phillips, P. S. Price, C. M. Grulke, A. Williams and D. Biryol,
et al., The Chemical and Products Database, a resource for exposure-relevant data on chemicals in consumer products, Sci. Data, 2018, 5, 180125, DOI:10.1038/sdata.2018.125.
- E. L. Schymanski, J. Jeon, R. Gulde, K. Fenner, M. Ruff and H. P. Singer,
et al., Identifying Small Molecules via High Resolution Mass Spectrometry: Communicating Confidence, Environ. Sci. Technol., 2014, 48(4), 2097–2098, DOI:10.1021/es5002105.
-
N. Dodder, K. Mullen and M. N. Dodder, Package OrgMassSpecR, 2017 Search PubMed.
- M. J. Benotti, R. Song, D. Wilson and S. A. Snyder, Removal of pharmaceuticals and endocrine disrupting compounds through pilot-and full-scale riverbank filtration, Water Sci. Technol.: Water Supply, 2012, 12, 11–23 CAS.
- J. Plutzer, P. Avar, D. Keresztes, Z. Sári, I. Kiss-Szarvák and M. Vargha,
et al., Investigation of estrogen activity in the raw and treated waters of riverbank infiltration using a yeast estrogen screen and chemical analysis, J. Water Health, 2018, 16(4), 635–645, DOI:10.2166/wh.2018.049.
- D. G. Lee, P. R. Roehrdanz, M. Feraud, J. Ervin, T. Anumol and A. Jia,
et al., Wastewater compounds in urban shallow groundwater wells correspond to exfiltration probabilities of nearby sewers, Water Res., 2015, 85, 467–475 CrossRef CAS . Available from: http://www.sciencedirect.com/science/article/pii/S0043135415301998.
- C. Li, Y. Ma, H. Li and G. Peng, A convenient method for the determination of molecular weight cut-off of ultrafiltration membranes, Chin. J. Chem. Eng., 2017, 25(1), 62–67 CrossRef CAS . Available from: http://www.sciencedirect.com/science/article/pii/S100495411630297X.
- T. Haider, R. Sommer, S. Knasmüller, P. Eckl, W. Pribil and A. Cabaj,
et al., Genotoxic response of Austrian groundwater samples treated under standardized UV (254 nm) disinfection conditions in a combination of three different bioassays, Water Res., 2002, 36(1), 25–32 CrossRef CAS PubMed.
- H. J. Kool, C. F. Van Kreyl and S. Persad, Mutagenic activity in groundwater in relation to mobilization of organic mutagens in soil, Sci. Total Environ., 1989, 84, 185–199 CrossRef CAS PubMed . Available from: http://www.sciencedirect.com/science/article/pii/0048969789903823.
- N. Cullum, C. K. Meng and P. Zavitsanos, Effect of Sample Matrix on Suppression of Ionization in Water Samples Using LC-ESI-MS, Agilent Technologies publication, 2004, 1–10 Search PubMed.
- V. Albergamo, R. Helmus and P. de Voogt, Direct injection analysis of polar micropollutants in natural drinking water sources with biphenyl liquid chromatography coupled to high-resolution time-of-flight mass spectrometry, J. Chromatogr. A, 2018, 1596, 53–61 CrossRef . Available from: http://www.sciencedirect.com/science/article/pii/S0021967318308926.
- L. Guoguang, J. Xiangning and X. Xiaobai, Photodegradation of 1-(2-chlorobenzoyl)-3-(4-chlorophenyl) urea in different media and toxicity of its reaction products, J. Agric. Food Chem., 2001, 49(5), 2359–2362 CrossRef CAS PubMed.
- M. S. Holtze, H. C. B. Hansen, R. K. Juhler, J. Sørensen and J. Aamand, Microbial degradation pathways of the herbicide dichlobenil in soils with different history of dichlobenil-exposure, Environ. Pollut., 2007, 148(1), 343–351 CrossRef CAS PubMed.
- R. Loos, R. Carvalho, D. C. António, S. Comero, G. Locoro and S. Tavazzi,
et al., EU-wide monitoring survey on emerging polar organic contaminants in wastewater treatment plant effluents, Water Res., 2013, 47(17), 6475–6487 CrossRef CAS PubMed.
-
S. Huntscha, D. M. R. Velosa, M. H. Schroth and J. Hollender, Degradation of Polar Organic Micropollutants during Riverbank Filtration: Complementary Results from Spatiotemporal Sampling and Push–Pull Tests, 2013 Oct 2. Available from: http://pubs.acs.org/doi/abs/10.1021/es401802z#.V6SAOYMS6w8.mendeley Search PubMed.
- G. M. Williams, I. Harrison, C. A. Carlick and O. Crowley, Changes in enantiomeric fraction as evidence of natural attenuation of mecoprop in a limestone aquifer, J. Contam. Hydrol., 2003, 64(3), 253–267 CrossRef CAS PubMed . Available from: http://www.sciencedirect.com/science/article/pii/S0169772202002061.
- F. S. Kegel, B. M. Rietman and A. R. D. Verliefde, Reverse osmosis followed by activated carbon filtration for efficient removal of organic micropollutants from river bank filtrate, Water Sci. Technol., 2010, 61(10), 2603–2610 CrossRef CAS.
-
D. Brkic, S. Gasic, A. Vértesi, V. Karan and N. Neskovic, Genotoxicity of GAL-57 Herbicide in Salmonella typhimurium and Escherichia coli, 2015 Search PubMed.
- F. T. Lange, M. Scheurer and H.-J. Brauch, Artificial sweeteners---a recently recognized class of emerging environmental contaminants: a review, Anal. Bioanal. Chem., 2012, 403(9), 2503–2518, DOI:10.1007/s00216-012-5892-z.
- I. J. Buerge, H.-R. Buser, M. Kahle, M. D. Müller and T. Poiger, Ubiquitous Occurrence of the Artificial Sweetener Acesulfame in the Aquatic Environment: An Ideal Chemical Marker of Domestic Wastewater in Groundwater, Environ. Sci. Technol., 2009, 43(12), 4381–4385, DOI:10.1021/es900126x.
- R. Ling, L. Yu, T. P. T. Pham, J. Shao, J. P. Chen and M. Reinhard, The tolerance of a thin-film composite polyamide reverse osmosis membrane to hydrogen peroxide exposure, J. Membr. Sci., 2017, 524, 529–536 CrossRef CAS . Available from: http://www.sciencedirect.com/science/article/pii/S0376738816313953.
- S. Ghoshal and A. Mukherjee, Genotoxicity Testing of Low-Calorie Sweeteners: Aspartame, Acesulfame-K, and Saccharin AU - Bandyopadhyay, Atrayee, Drug Chem. Toxicol., 2008, 31(4), 447–457, DOI:10.1080/01480540802390270.
- A. Wick, O. Marincas, Z. Moldovan and T. A. Ternes, Sorption of biocides, triazine and phenylurea herbicides, and UV-filters onto secondary sludge, Water Res., 2011, 45(12), 3638–3652 CrossRef CAS PubMed . Available from: http://www.sciencedirect.com/science/article/pii/S0043135411001990.
- M. Surgan, M. Condon and C. Cox, Pesticide Risk Indicators: Unidentified Inert Ingredients Compromise Their Integrity and Utility, Environ. Manage., 2010, 45(4), 834–841, DOI:10.1007/s00267-009-9382-9.
- R. J. Emery, M. Papadaki, L. M. Freitas dos Santos and D. Mantzavinos, Extent of sonochemical degradation and change of toxicity of a pharmaceutical precursor (triphenylphosphine oxide) in water as a function of treatment conditions, Environ. Int., 2005, 31(2), 207–211 CrossRef CAS PubMed . Available from: http://www.sciencedirect.com/science/article/pii/S0160412004001680.
- T. L. ter Laak, L. M. Puijker, J. A. van Leerdam, K. J. Raat, A. Kolkman and P. de Voogt,
et al., Broad target chemical screening approach used as tool for rapid assessment of groundwater quality, Sci. Total Environ., 2012, 427, 308–313 CrossRef.
- C. Babua, B. Sreenivasa Rao, K. V. N. Suresh Reddy and N. B. Development, Validation of HPLC Assay Method for the Acamprosate Ca in Commercial Tablets, Indian J. Adv. Chem. Sci., 2013, 2(1), 46–49 Search PubMed.
- M. I. Wilde and A. J. Wagstaff, Acamprosate, Drugs, 1997, 53(6), 1038–1053, DOI:10.2165/00003495-199753060-00008.
Footnote |
† Electronic supplementary information (ESI) available. See DOI: 10.1039/c9ew00741e |
|
This journal is © The Royal Society of Chemistry 2020 |