DOI:
10.1039/C9MT00141G
(Paper)
Metallomics, 2019,
11, 1716-1728
Preclinical studies on metal based anticancer drugs as enabled by integrated metallomics and metabolomics†
Received
29th May 2019
, Accepted 29th August 2019
First published on 29th August 2019
Abstract
Resistance development is a major obstacle for platinum-based chemotherapy, with the anticancer drug oxaliplatin being no exception. Acquired resistance is often associated with altered drug accumulation. In this work we introduce a novel -omics workflow enabling the parallel study of platinum drug uptake and its distribution between nucleus/protein and small molecule fraction along with metabolic changes after different treatment time points. This integrated metallomics/metabolomics approach is facilitated by a tailored sample preparation workflow suitable for preclinical studies on adherent cancer cell models. Inductively coupled plasma mass spectrometry monitors the platinum drug, while the metabolomics tool-set is provided by hydrophilic interaction liquid chromatography combined with high-resolution Orbitrap mass spectrometry. The implemented method covers biochemical key pathways of cancer cell metabolism as shown by a panel of >130 metabolite standards. Furthermore, the addition of yeast-based 13C-enriched internal standards upon extraction enabled a novel targeted/untargeted analysis strategy. In this study we used our method to compare an oxaliplatin sensitive human colon cancer cell line (HCT116) and its corresponding resistant model. In the acquired oxaliplatin resistant cells distinct differences in oxaliplatin accumulation correlated with differences in metabolomic rearrangements. Using this multi-omics approach for platinum-treated samples facilitates the generation of novel hypotheses regarding the susceptibility and resistance towards oxaliplatin.
Introduction
Already in the 1930s, Warburg and his contemporaries reported the concept of aerobic glycolysis in cancer. They highlighted the fundamental differences in the central metabolic pathways between healthy and malignant tissues.1 While for many years cancer research focused on genomic changes and oncogenes,2–4 links between metabolism and cancer only recently re-emerged as an exciting area of investigation.5 Indeed, dynamic changes of biological systems can be tracked by measuring the metabolome. Furthermore, molecular signatures are extremely powerful for hypothesis generation, offering novel insights, e.g., in the crosstalk of tumor cells with the tumor microenvironment6 or in the drug mode of action.7,8 Metabolome profiling of drug-exposed cell cultures in vitro offers the unique opportunity to identify potential weaknesses in cellular drug responses, allowing correlations of biochemical pathways and/or genetic polymorphisms with drug susceptibility.3,9
To date, only a few metabolomic studies exist in the field of metal-based anticancer agents, despite the fact that platinum-based therapies are among the most frequently clinically applied anticancer therapies.10 The amount of registered clinical studies involving metal-based anticancer drugs (novel compounds and combination therapies) is constantly high with increasing numbers over the last few years,11,12 such as successful combinations with immunotherapies in recent years.13 As for many drugs, including the clinically approved platinum compounds, it is not fully defined how the drug is taken up by the malignant tissues.14,15 A clear-cut mechanism remains to be elucidated even after years of clinical practice. Intrinsic and acquired platinum resistance are a major obstacle jeopardizing successful therapy.16–19 For the clinically approved platinum drugs, DNA is the established molecular target.11,15 However, the fact that only 1% of the platinum reaches the nucleus strongly suggests additional important cellular interactions.20 Recently, oxaliplatin was found to kill cancer cells by inducing ribosome biogenesis stress rather than by engaging a DNA damage response.21
The few metabolomic studies investigating platinum drugs (1) address the role of metabolism in chemosensitivity of tumor cells towards platinum compounds,22 (2) investigate metabolomics as a predictive tool,23,24 (3) used metabolomics to discriminate isogenic sensitive and resistant cell types19,25,26 and (4) proposed metabolomics as a tool-set for early detection of nephrotoxicity.27 By correlating biochemical pathways (measured by transcriptomics and metabolomics) from the NCI60 cell line panel with tumor cell chemosensitivity, it was found that pathways including the tricarboxylic acid (TCA) cycle, pyruvate metabolism, lipoprotein uptake and nucleotide synthesis (salvage pathways and de novo synthesis) were specific for sensitivity towards platinum compounds.28,29
Overall metabolomic platforms and procedures are still not as mature as other -omic technologies.4 There is a need for new methods and technologies as analytical protocols have often to be adapted to the specific applications to try to cope with the intrinsic challenges of analysis such as metabolome coverage, analytical throughput and accuracy.30 In this work we propose the combination of metabolomics and metallomics as a novel powerful tool-set in preclinical metallodrug research to address the metal uptake and cellular drug distribution within the same sample. This unique combination ultimately allows assessment of metabolome perturbations exerted by the metallodrugs and correlation of the corresponding biochemical pathways with drug accumulation and/or cytotoxicity. Complementary use of elemental inductively coupled plasma mass spectrometry (ICP-MS) and high-resolution molecular mass spectrometry (Orbitrap-MS) together with the developed sample preparation enabled the study of drug uptake, drug distribution between nucleus/protein and small molecule fraction and metabolic signatures upon drug exposure from one cell culture sample. Sample preparation in metabolomics is highly demanding25,31 and absolutely key when acquiring a snap-shot of the highly dynamic metabolome, particularly with regards to the handling time. Several preparation protocols have been established to simultaneously measure proteomics and metabolomics,32–34 but the combination of metal analysis and metabolomics has not been addressed so far. In the past, it has been shown that the choice of chromatography was key for the selectivity, throughput and metabolome coverage of the analysis.35,36 In this work we implemented hydrophilic interaction liquid chromatography (HILIC) for the retention of polar metabolites.37–45 Finally, 13C internal standardization based on yeast standards25,35,43,46,47 was employed at the extraction stage.
Together with high-resolution mass spectrometry this strategy allows (1) improvement of the identification task in untargeted metabolomics, an approach coined as credentialing,48 and (2) merging of targeted quantification and untargeted analysis in one workflow.25 With this method targeted relative quantification between different sample groups was facilitated without compromising untargeted data evaluation.25 To test the novel metabolomics/metallomics strategy in a preclinical setting we investigated metabolic changes and drug accumulation in an oxaliplatin sensitive and resistant cell model over time.
Experimental
Reagents, chemicals and standards
The metabolite standards were purchased from Sigma-Aldrich or Fluka (Vienna, Austria) except for malic acid which was purchased from Merck (Vienna, Austria). A multistandard stock solution of 100 μM was prepared in water and used as a quality control (QC) of 10 μM. A fully 13C labeled yeast extract of Pichia pastoris (2 billion cells) from ISOtopic solutions e.U., (Vienna, Austria) was reconstituted in 2 mL of water and added in the same amount to the samples. The final dilution of the internal standard for the measurement was 1
:
10. As shown in previous studies,25,35,43,46,47 the yeast based material provided a 13C isotopic enrichment of >98.8% for primary metabolites resulting in a non-interfering isotopologue pattern with the natural compounds. The platinum standard (1002 ± 6 μg mL−1) was purchased from Inorganic Ventures (Christiansburg, Virginia, USA). Media and reagents for cell culture were purchased from Sigma-Aldrich (Vienna, Austria) and all materials such as dishes, plates and flasks were purchased from StarLab (Hamburg, Germany) unless indicated otherwise.
Cell culture and cytotoxicity tests
The human colon cancer line HCT116, acquired from ATCC (American Type Culture Collection, USA), and the oxaliplatin resistant subline (established as described elsewhere49) were grown as adherent monolayer cultures in McCoy's 5A medium (Sigma-Aldrich) supplemented with 10% heat-inactivated fetal bovine serum (BioWest) and 4 mM L-glutamine (Sigma-Aldrich) without antibiotics at 37 °C (StarLab) under a humidified atmosphere containing 5% CO2. HCT116 cells were seeded at a density of 5 × 103 viable cells per well into 96-well microculture plates (StarLab) 24 h prior to the treatment. Stock solutions of oxaliplatin were prepared in McCoy's 5A medium and diluted stepwise. Cells were incubated for 24 h with the indicated drug concentrations. Cell viability was measured using resazurin sodium salt (Sigma-Aldrich) (440 μM in PBS (Sigma-Aldrich)). After 4 h at 37 °C the fluorimetric measurement of the formed resorufin was performed with a Synergy HT reader (BioTek) (excitation wavelength at 530 nm, emission wavelength at 590 nm). The concentration–effect curves from three independent experiments were averaged. An average IC50 value of 35 ± 6 μM after 24 h of incubation ensured that a concentration of 5 μM oxaliplatin was adequate (sub-cytotoxic) for the following preparations (see Fig. S1 for the concentration–effect curves, ESI†).
Preparation of cell extracts
5 × 105 cells per well were seeded of each cell line in a 6-well plate in 3 mL per well (N = 3 for 4 h and 8 h and N = 6 for 24 h experiments). After 24 h, 1 mL of the control medium or oxaliplatin-containing (final concentration 5 μM) medium was added. Plates were incubated for 4 h, 8 h or 24 h. After incubation, the medium was removed, and the wells were washed once with 4 mL of PBS. Then, liquid nitrogen was added to quench the metabolism in the cells and the samples were stored at −80 °C until analysis. Upon extraction, 50 μL internal standard at room temperature and 1 mL ice-cold methanol (80% methanol, 20% water, v/v) were added. The cells were scraped into the extraction solvent and transferred to an Eppendorf tube. The cell scraper and the wells were washed two times with 475 μL extraction solvent to reach a final volume of 2 mL extract. After thorough mixing and centrifugation (20
000 RCF, 5 min, 4 °C), the metabolome cell extract was separated from the precipitate which contained the nucleus and proteins (denoted as precipitate) and transferred into a fresh Eppendorf tube. The purity of the fractions was not assessed.50 From the metabolome cell extract, an aliquot of 200 μL was transferred into a HPLC vial with an insert for evaporation and reconstitution in 50 μL ACN (50% ACN, 50% water, v/v) leading to a pre-concentration factor of 4. Additionally, a pooled sample was prepared by mixing aliquots (10 μL) of all the pre-concentrated samples. Another aliquot (200 μL) of the metabolome cell extract was used for platinum quantification by flow injection (FI)-ICP-MS. The precipitate was dissolved in 1 mL of 0.05 M NaOH and mixed thoroughly to ensure proper dissolving. For metallomics, 900 μL of the dissolved precipitate was evaporated by a SpeedVac and 300 μL HCl 6 M was added, thoroughly vortexed, transferred to reaction vessels and heated at 100 °C for 24 h for the hydrolysis of the proteins. After the hydrolysis of the proteins, the HCl was removed by evaporation. The dry samples were finally re-dissolved in 200 μL H2O for platinum quantification using FI-ICP-MS. A scheme summarizing the sample preparation is shown in Fig. 1.
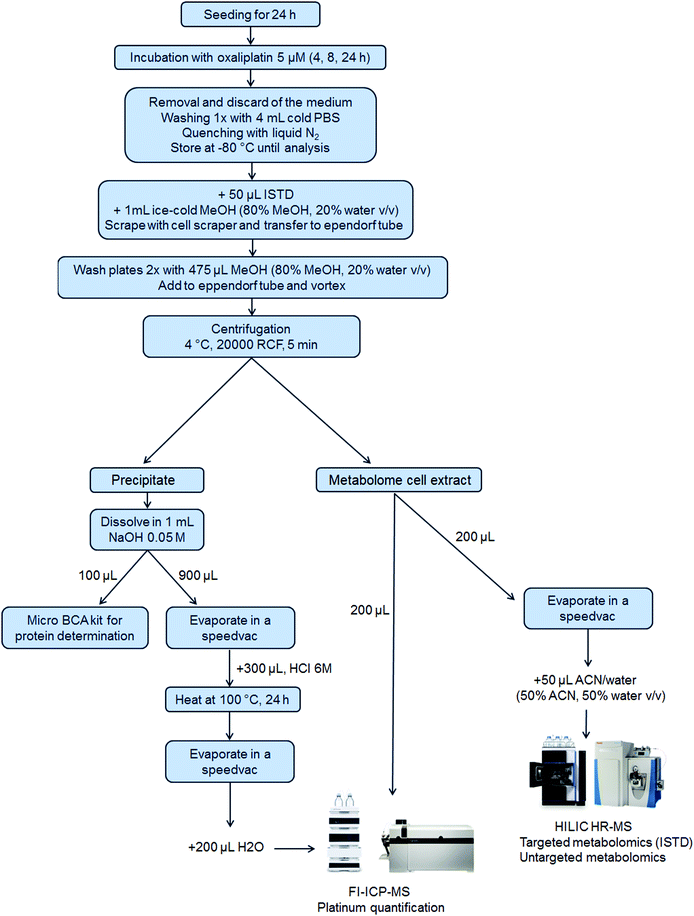 |
| Fig. 1 A scheme of integrated metallomics/metabolomics – from sample preparation to analysis. The precipitate was employed for the protein determination and platinum quantification, the latter requiring protein hydrolysis before injection on the FI-ICP-MS system. In parallel to the analysis of the precipitate, two aliquots of the metabolome cell extract were analyzed: one was pre-concentrated by evaporation, re-suspended in ACN/water (1 : 1, v/v), and injected into the HILIC HR-MS system for metabolomic analysis whereas the other one was directly injected into the FI-ICP-MS system for platinum analysis. | |
Determination of protein content for normalization of adherent cell cultures
The protein concentration was assessed from the precipitate dissolved in 0.05 M NaOH (100 μL). For this purpose, the commercially available micro BCA protein assay kit (Thermo Fisher Scientific, Pierce Biotechnology, Rockford, USA) was employed. In order to validate this approach for normalization purposes, different number of cells were seeded in 6-well plates and extracted as described before, prior to protein quantification. A standard curve with BSA was used for calibration (0.5–20 μg mL−1).
FI-ICP-MS
Platinum uptake was quantified by FI-ICP-MS. Briefly, a HPLC Agilent 1260 infinity Bio-inert HPLC (Waldbronn, Germany) was coupled to a Triple Quadrupole ICP-MS Agilent 8800 (Agilent Technologies, Tokyo, Japan) with the Agilent MassHunter software package (Work-station Software, Version C.01.03, 2016) for data treatment. The chromatographic and ICP-MS conditions are summarized in Table S1 (ESI†).
HILIC-HR-MS
HILIC-HR-MS was employed for metabolomic analysis. Briefly, a Vanquish UHPLC (Thermo Scientific) was coupled to a high field Thermo Scientific™ Q Exactive HF™ quadrupole-Orbitrap mass spectrometer equipped with an ESI source. The separation was conducted on an ACQUITY® UPLC BEH Amide column 2.1 × 100 mm, 1.7 μM (Waters Corp., Milford, MA) with trifunctional amide groups bonded to an ethylene bridged hybrid substrate as a stationary phase and a sub-2 μm particle. The column was operated at 250 μL min−1 and at 40 °C. The injection volume was 5 μL. Mobile phase A consisted of 50 mM HCOONH4 in water, pH = 4.0. Mobile phase B consisted of 80% ACN and 20% water with 50 mM HCOONH4. The chromatographic run started at 100% B (2 min) followed by a linear gradient to 50% B (6 min). Then, it was held at 50% B for 2 min and re-equilibrated at 100% B for 5 min. The parameters for the full mass scan (Full-MS), ESI-source (ESI) and untargeted fragmentation approach (ddMS2) are summarized in Table S2 (ESI†). The samples were randomized to minimize batch variations. The targeted data evaluation was performed using TraceFinderTM 4.1 from Thermo Scientific™ with internal standardization. Thermo Scientific™ Compound Discoverer™ 3.0 was employed for the untargeted data processing. This software combines feature detection with statistical data evaluation. For the detection of unknown features at the MS1 level a minimum peak intensity of 10
000 with a mass tolerance of 3 ppm and a minimum number of 2 isotopes were used. For identification, an mzCloud search was performed with 5 ppm mass tolerance and a threshold for compound annotation of 70.
Results and discussions
To achieve an integrated metallomics/metabolomics workflow (Fig. 1) several key steps required optimization and/or novel analytical developments. The following, describes the methodological aspects of this study.
Sample preparation
A sample preparation method based on simultaneous direct scraping and extraction adopted from metabolomic workflows (Dettmer et al.31 and Schwaiger et al.25) was implemented in this work. As internal standard 13C-enriched Pichia pastoris was added upon extraction. The subsequent centrifugation step resulted in two sample fractions, (1) denoted as the metabolome cell extract containing primarily small molecules and (2) the precipitate which includes the nucleus and proteins insoluble in methanol.
Normalization strategy
The application of normalization strategies is an established approach to ensure comparability and improved biological repeatability of quantitative studies addressing either different cell types or different cultivation time points. A typical metabolomics experiment relates either relative or absolute concentration changes to cell number, protein amount or dry/wet weight.51,52 The same holds true for quantitative drug uptake studies. In platinum accumulation studies of adherent cell cultures, cells are often trypsinized to consequently normalize the platinum content to total cell number. As trypsinization introduces significant alterations to the metabolome,31,42,53 protein content was used for normalization in this study. For this purpose, the protocol of a commercially available protein assay was adapted to adherent cell cultures omitting the precipitation step of the kit by directly dissolving the precipitate for protein quantification. An independent validation experiment assessing cell number and protein content showed the validity of the normalization method. As can be observed in Fig. S2 and Table S3 (ESI†) a linear calibration of cell number versus protein content could be achieved. The working range was at low μg mL−1 level resulting in biological repeatability of typically 10%.
Metabolomics enabled by HR-MS
A standard panel consisting of >130 metabolites, including amino acids, sugars, sugar phosphates, organic acids, nucleotides and cofactors and coenzymes served for selection of chromatographic separation. The selection covered pathways key in cancer metabolism,53,54 such as glycolysis, TCA cycle, energy metabolism, amino acid synthesis, fatty acid synthesis, purine and pyrimidine pathways. In this work, a HILIC separation based on a sub-2 μm stationary phase with trifunctional amide groups bonded to an ethylene bridged hybrid substrate was implemented. Compared to chromatographic separation on a mixed mode stationary phase in targeted metabolomics of a 10 μM multicomponent standard, the selected HILIC phase provided higher metabolite coverage (see Fig. 2 bar chart and pie chart of recovered metabolite mix). The detailed information about the chromatographic methods is shown in Table S4 (ESI†). Based on the retention time distribution (see violin plots Fig. S3, ESI†) and ionization coverage, pH 4 was selected as an optimum separation condition. Detailed information on separated metabolites, retention times, ionization modes and separation of critical isomers is summarized in the ESI† (Fig. S4, S5 and Tables S5–S7). Repeated injections of a quality control 10 μM multi-metabolite standard over >25 hours revealed good technical repeatability (see Table S8, ESI†). Using the sub-2 μm HILIC phase, most standard peaks revealed a full width at half maximum (FWHM) <10 s (see Table S9, ESI†). 13C enriched standards derived from the labeled yeast extract were added to the cancer cells during sample preparation with the aim of improving biological repeatability of sample preparation and subsequent HILIC analysis. This isotopically enriched biomass has been successfully used in numerous metabolomic studies addressing targeted absolute quantification35,43,46,47 and novel workflows merging targeted and untargeted analysis.25,55 It was shown that the addition of internal standard did not hamper the untargeted data evaluation, but enabled simultaneous absolute quantification of a targeted panel.25 In this work, we implemented the internal standardization strategy for the first time with the aim of improving biological repeatability of relative quantification based on high-resolution mass spectrometry. Fig. 3a displays metabolites according to compound classes and numbers as found up-to-date in 13C-enriched Pichia pastoris.25,35,43,46,47,55Fig. 3b shows the actual number of metabolites recovered in the cell extracts in targeted analysis using the here established protocols and available standards. Fig. 3c shows the number of metabolites recovered in yeast cell extracts in targeted analysis using the here established protocols and available standards. Overall >60 metabolites could be internally standardized upon sample extraction improving technical and biological repeatability (see Fig. 4a and b respectively).
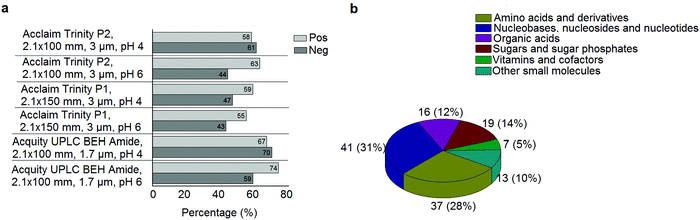 |
| Fig. 2 (a) Percentage of metabolites found in a 10 μM multicomponent standard with the different HILIC columns at pH 4 and pH 6 in positive and negative mode. When combining positive and negative mode, the UPLC BEH Amide column at pH 4 showed the highest coverage. (b) The number of metabolites and their classes (combining positive and negative mode) as found in a 10 μM multicomponent standard of >130 metabolites with the UPLC BEH Amide column at pH 4. | |
 |
| Fig. 3 The number of metabolites and their classes (combining a positive and negative mode) as found (a) up to date in 13C-enriched Pichia pastoris,25,35,43,46,47,55 (b) in a sensitive control HCT116 cell extract measured with the UPLC BEH Amide column at pH 4 and (c) in 13C-enriched Pichia pastoris added in sensitive control HCT116 prior to cell extraction and measured with the UPLC BEH Amide column at pH 4. | |
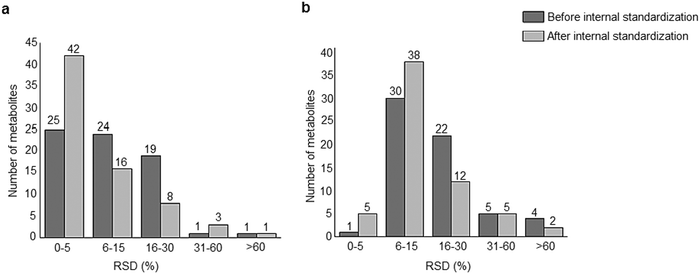 |
| Fig. 4 Repeatability of the HILIC-HR-MS approach combining positive and negative mode of (a) a pooled cell extract (N = 3 biological replicates) with and without internal standardization. The internal standardization improved the technical repeatability. (b) A sensitive line control cell extract (N = 6 biological replicates) with and without internal standardization. The internal standard was added prior to the extraction and it improved the biological repeatability. | |
Metallomics enabled by FI-ICP-MS
Flow injection combined with ICP-MS detection enabled absolute quantification of metal based anticancer drugs in the metabolome cell extract omitting mineralization steps. The metal in the precipitate, resembling the protein and nucleus associated fraction, was quantified after mineralization. In this study, we focus on the third generation platinum compound oxaliplatin knowing that this compound shows in vitro and in vivo antitumor activity against colorectal cancer.56 The recovery of intact oxaliplatin in the extraction solvent was excellent (100 ± 7%, N = 3). However, the preclinical application of sub-IC50 concentrations of oxaliplatin resulted in cytosolic concentration levels ranging below 1.5 μg L−1 (as assessed by the total platinum concentration) which hampered elemental speciation approaches (typical LOD of HILIC-ICP-MS ideally compatible with the extraction solvent is 3 μg L−1 platinum), while reversed phase approaches required removal of the organic solvent, a step which altered the elemental speciation of the metallodrug.57
The study case – addressing metabolic perturbation exerted by oxaliplatin in sensitive versus resistant cancer cell models of human colon cancer
More than a decade ago, oxaliplatin was approved as a standard treatment of advanced metastatic colorectal carcinoma in a combination with 5-fluorouracil and leucovorin (FOLFOX).58 Despite being in the clinic since 2004, several key aspects regarding its mode of action are still open and are distinctly different from cisplatin. The ultimate target of both drugs is DNA.16,17 Despite forming the same types of inter- and intrastrand DNA cross-links, the drug's reactivity towards DNA is different with oxaliplatin being less reactive than cisplatin. It is believed that the DACH ligand of oxaliplatin, in contrast to the amino groups of cisplatin, is responsible for its differential recognition by the mismatch repair machinery and therefore leads to different activity spectrum. The promise that oxaliplatin would have low-cross resistance to cisplatin and attack cisplatin resistant tumors was found to be partially true and was limited to special cases such as in combination therapy against colon cancer.59,60 Moreover, the activity of oxaliplatin is, in contrast to cisplatin, strictly depending on an immunoproficient background.61
In this work, the novel integrated metallomics/metabolomics workflow was applied in a preclinical study on oxaliplatin resistance. More specifically, we investigated the human colon cancer HCT-116 cell model with a sub-line of acquired resistance. The resistant cell model was generated by pulsed-exposure of HCT-116 cells to increasing concentrations of oxaliplatin. As compared to the parental cell model, the resistant cell models displayed more than 10-fold resistance against oxaliplatin.62 The workflow enabled addressing drug uptake and distribution between nucleus/protein fraction and cytosolic low molar mass fraction together with metabolic perturbation upon different exposure times.
Metallomics
Fig. 5 shows the drug accumulation data obtained by ICP-MS based metallomics (LOD of 0.04 μg L−1 of Pt, 3σ criterion and RSDs of 1–24% and 16–45% for the precipitate and the metabolome cell extract respectively). While in the sensitive HCT116 cell line the precipitate concentration of platinum increased over time (to ∼10 μg Pt per g protein), the metabolome cell extract remained rather constant (∼15–18 μg Pt per g protein). Within the first 4 h the platinum accumulation data were comparable for the sensitive and resistant cell models. Drug accumulation was reduced after 8 h of incubation, as previously shown.62 In contrast to the parental cell line, the resistant model showed constant low nucleus/protein concentration, while the metabolome cell extract was decreasing. Of note, to date there is no clear-cut uptake mechanism for oxaliplatin established. Passive diffusion and transporter-mediated uptake mechanisms are discussed.63,64 The comparative drug accumulation data of Fig. 4, points towards predominant passive diffusion uptake for the first 4 hours. Differential active efflux transport mechanisms might be observed after 8 h of exposure between the parental and the resistant cell line. In fact, several studies linked overexpression of transporters to therapy outcome or resistance, however knowledge is still fragmentary.63,64 The influx transporters involve copper transporter (CTR) proteins and organic cation transporters (OCTs). The export of platinum drugs has been linked to efflux pumps such as ATP binding cassette (ABC) multidrug transporters, copper-transporting P-type adenosine triphosphatases (ATPases), and multidrug and toxin extrusion (MATE) proteins.65
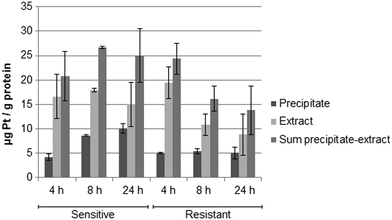 |
| Fig. 5 Total platinum concentration normalized to the protein content in the precipitate (nucleus/proteins), in the metabolome cell extract and in the sum of both of the sensitive and resistant cancer line HCT116 upon incubation with oxaliplatin 5 μM (N = 3 biological replicates for 4 and 8 h, N = 6 biological replicates for 24 h). | |
Metabolomics
The metabolomics experiments revealed that the parental and resistant HCT-116 cell lines exhibited a distinctly different metabotype from the start as evidenced by multivariate statistics (see heat-map and principal component analysis Fig. 6 and Fig. S6–S8, ESI†). Table 1 shows a list of significantly different metabolites after 24 hours of incubation. The list covers metabolites which were determined by targeted relative quantification (using 13C internal standardization) together with metabolites discovered by untargeted data evaluation. Accordingly, their identity confirmation is based on the accurate mass, isotopologue pattern and spectral library match (MS2; mzCloud database), which is considered a level 2 structure confirmation.66 Upon drug exposure, the sensitive cell models revealed a pronounced metabolic perturbation, while the resistant cells showed only subtle changes (see multivariate statistical evaluation Fig. S9–S12 (ESI†) and Table 1).
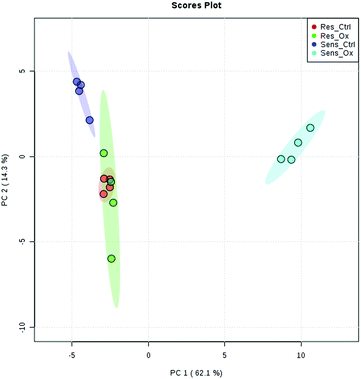 |
| Fig. 6 Principal component analysis of sensitive and resistant HCT116 control and sensitive and resistant HCT116 incubated with oxaliplatin 5 μM for 24 h. A clear separation could be observed between the sensitive and the resistant line as well as between the sensitive control and sensitive cells incubated with oxalipatin, while resistant cells with and without drug exposure were not separated. Method: UPLC BEH Amide at pH 4 in positive and negative mode using a reduced data matrix considering only features which were identified (level 1, level 2). | |
Table 1 List of metabolites which showed significant differences (p < 0.05) in a HCT116 cell extract (N = 6 biological replicates) after incubation with oxaliplatin 5 μM for 24 h in targeted (internal standardization, Tukey's HSD test) and untargeted metabolomics (without internal standardization, MS2 library match, Benjamini–Hochberg correction). Column: UPLC BEH Amide pH 4 in positive and negative mode. The p value is the probability of obtaining an effect equal to or more extreme than the one observed considering the null hypothesis is true. The null hypothesis is said to be disproven if the effect observed is so important, and consequently the p value is so low, that either an exceptionally rare chance has occurred or the theory is not true.67 On this table, the p value is expressed in logarithm base 10. The fold change (FC) value is the ratio between two quantities. On this table, FC is expressed in logarithm base 2
Targeted |
Compound |
Polarity |
Pathways |
RT (min) |
Resistant control vs. sensitive control |
Sensitive control vs. sensitive oxaliplatin |
Resistant control vs. resistant oxaliplatin |
−log 10 (p) |
log 2 (FC) |
−log 10 (p) |
log 2 (FC) |
−log 10 (p) |
log 2 (FC) |
The separation of the different isomers was not possible with this chromatographic method. If different peaks were partially separated, they were integrated as one single peak.
Only one biological replicate was detected in the resistant line.
|
Alaninea |
Pos |
Alanine, aspartate and glutamate metabolism |
4.64 |
2.86 |
−0.38 |
3.26 |
0.44 |
— |
— |
Cysteine and methionine metabolism |
Taurine and hypotaurine metabolism |
Selenoamino acid metabolism |
Alpha-ketoglutarate |
Neg |
Alanine, aspartate and glutamate metabolism |
3.13 |
— |
— |
4.97 |
0.85 |
— |
— |
TCA cycle |
D-Glutamine and D-glutamate metabolism |
Butanoate metabolism |
Histidine metabolism |
Lysine biosynthesis |
Ascorbate and aldarate metabolism |
AMPa |
Pos/Neg |
Purine and pyrimidine metabolism |
5.62 |
— |
— |
3.66 |
1.34 |
— |
— |
Nitrogen metabolism |
Argininosuccinic acid |
Pos |
Alanine, aspartate and glutamate metabolism |
6.83 |
6.12 |
−1.21 |
3.80 |
0.73 |
— |
— |
Arginine and proline metabolism |
Asparagine |
Neg |
Alanine, aspartate and glutamate metabolism |
5.47 |
4.16 |
−0.48 |
3.40 |
0.46 |
— |
— |
Nitrogen metabolism |
Aspartate |
Neg |
Alanine, aspartate and glutamate metabolism |
5.99 |
4.53 |
−0.48 |
— |
— |
— |
— |
|
|
Glycine, serine and threonine metabolism |
|
|
|
|
|
|
|
|
|
Nitrogen metabolism |
|
|
|
|
|
|
|
|
|
Arginine and proline metabolism |
|
|
|
|
|
|
|
|
|
Cysteine and methionine metabolism |
|
|
|
|
|
|
|
|
|
Nicotinate and nicotinamide metabolism |
|
|
|
|
|
|
|
|
|
Histidine metabolism |
|
|
|
|
|
|
|
|
|
Lysine biosynthesis |
|
|
|
|
|
|
|
|
|
Pantothenate and CoA biosynthesis |
|
|
|
|
|
|
|
|
|
Beta-alanine metabolism |
|
|
|
|
|
|
|
Carnitine |
Pos |
Lysine degradation |
6.64 |
2.69 |
−0.29 |
2.96 |
0.35 |
— |
— |
ABC transporters |
Fatty acid oxidation |
Choline |
Pos |
Glycine, serine and threonine metabolism |
2.01 |
3.58 |
−0.73 |
— |
— |
— |
— |
Glycerophospholipid metabolism |
Cystathionine |
Pos |
Nitrogen metabolism |
6.64 |
6.81 |
0.73 |
— |
— |
— |
— |
Cysteine and methionine metabolism |
Glycine, serine and threonine metabolism |
Fructose-1,6-bisphosphate |
Pos/Neg |
Phenylalanine, tyrosine and tryptophan biosynthesis |
7.35 |
— |
— |
3.15 |
1.31 |
2.78 |
1.11 |
Fumarate |
Neg |
Alanine, aspartate and glutamate metabolism |
3.15 |
6.56 |
−0.79 |
7.86 |
1.32 |
— |
— |
TCA cycle |
Arginine and proline metsbolism |
Butanoate metabolism |
Nicotinate and nicotinamide metabolism |
Phenylalanine metabolism |
Tyrosine metabolism |
GDP |
Pos |
Purine and pyrimidine metabolism |
7.62 |
— |
— |
2.31 |
0.45 |
— |
— |
Glutamate |
Pos/Neg |
Arginine biosynthesis |
5.55 |
— |
— |
4.82 |
0.53 |
— |
— |
Alanine, aspartate and glutamate metabolism Arginine and proline metsbolism |
|
|
|
|
|
|
|
Nitrogen metabolism |
|
|
|
|
|
|
|
D-Glutamine and D-glutamate metabolism |
|
|
|
|
|
|
|
Glutathione metabolism |
|
|
|
|
|
|
|
Histidine metabolism |
|
|
|
|
|
|
|
Glutamine |
Pos/Neg |
Arginine biosynthesis |
5.35 |
4.53 |
−0.53 |
3.03 |
0.42 |
— |
— |
Alanine, aspartate and glutamate metabolism |
|
|
|
|
|
|
|
Purine metabolism |
|
|
|
|
|
|
|
Pyrimidine metabolism |
|
|
|
|
|
|
|
D-Glutamine and D-glutamate metabolism |
|
|
|
|
|
|
|
Nitrogen metabolism |
|
|
|
|
|
|
|
Arginine and proline metsbolism |
|
|
|
|
|
|
|
Glutathione oxidized |
Pos |
Cysteine and methionine metabolism |
7.55 |
— |
— |
3.37 |
4.84 |
— |
— |
Hexose phospatea |
Pos/Neg |
Glycolisis/gluconeogenesis |
6.51 |
— |
— |
3.25 |
0.96 |
— |
— |
Galactose metabolism |
Pentose and glucoronate interconversions |
Untargeted |
Compound |
Polarity |
Pathways |
RT (min) |
mZCloud results (match) |
Resistant control vs. sensitive control |
Sensitive control vs. sensitive oxaliplatin |
Resistant control vs. resisitant oxaliplatin |
−log 10 (p) |
log 2 (FC) |
−log 10 (p) |
log 2 (FC) |
−log 10 (p) |
log 2 (FC) |
Arginine |
Pos |
Arginine and proline metabolism |
6.03 |
4 (81) |
2.28 |
−0.53 |
— |
— |
— |
— |
D-Arginine and D-ornithine metabolism |
Acetyl-carnitine |
Pos |
Fatty acid oxidation |
2.47 |
4 (99) |
5.01 |
−0.67 |
— |
— |
— |
— |
Acetyl-β-methylcholine |
Pos |
N/A |
2.12 |
4 (92) |
2.75 |
−0.32 |
— |
— |
— |
— |
N8-Acetylspermidine |
Pos |
N/A |
5.16 |
3 (81) |
— |
— |
6.09 |
−1.29 |
— |
— |
Acetylcholine |
Pos |
Glycerophospholipid metabolism |
2.68 |
2 (98) |
3.2 |
−0.48 |
— |
— |
— |
— |
Palmitoylcarnitine |
Pos |
Fatty acid oxidation |
0.95 |
1 (96) |
—b |
−1.32 |
6.02 |
−1.11 |
— |
— |
Fatty acid metabolism |
|
|
|
|
|
|
|
|
4-Acetamidobutanoic acid |
Pos |
Arginine and proline metabolism |
1.37 |
1 (99) |
4.07 |
−0.78 |
— |
— |
— |
— |
Nicotinic acid adenine dinucleotide |
Pos |
Nicotinate and nicotinamide metabolism |
7.03 |
1 (93) |
3.71 |
0.52 |
4.74 |
0.75 |
— |
— |
Glutathione (reduced) |
Neg |
Cysteine and methionine metabolism |
5.97 |
3 (79) |
2.57 |
−0.44 |
2.62 |
0.49 |
— |
— |
Glutathione metabolism |
The classic hallmark of tumor cell metabolism is the Warburg effect, which was found to be frequently accompanied by altered Krebs cycle, glutaminolysis, fatty acid and lipid metabolism and nucleotide synthesis.68,69 In a seminal study, metabolomics profiles as obtained across the NCI-60 panel pointed towards a positive correlation between all these “Warburg-like” phenotypes and platinum chemosensitivity.29 However, this study did not include oxaliplatin and is based on the correlation of untreated transcriptomics and the metabolomics data with the cell viability data. In contrast, our study includes simultaneous measurements of drug uptake and metabolomics. Parental HCT116 cells differ in glycolysis compared to the oxaliplatin resistant HCT116 cells. Pyruvate levels are higher in sensitive cells (Fig. S6, ESI†), and are strongly influenced upon drug incubation in this cell model (Fig. S9 and Table S10, ESI†). Additionally, the metabotype of the oxaliplatin sensitive HCT116 compared to the resistant model revealed differences regarding the intermediates of the Krebs cycle (i.e., fumarate, succinate and malate) confirming altered activity (Table 1 and Fig. S6, ESI†). All three metabolites are higher in sensitive cells compared to the resistant cells. Glutamate involved in glutaminolysis and purine synthesis via the folate cycle shows lower abundance in sensitive cells than resistant cells in the first 4 and 8 h. The low glutamate levels in sensitive cells are accompanied by low alpha-ketoglutarate (AKG) levels, the metabolite linking glutamate to the Krebs cycle. The biochemical basis for this increase of AKG in resistant cells might be explained by altered generation of carbon derived from amino acid catabolism and amino acid synthesis or by altered glutamine uptake from the media. Thus, our data suggest that the acquired resistance against oxaliplatin is accompanied by an overall altered energy metabolism. Moreover, the low glutamate level in sensitive cells is accompanied by the low cystathionine levels linked to glutathione biosynthesis (Fig. S6, ESI†). Glutathione decreases significantly during oxaliplatin incubation which can be inferred from the Pearson correlation data treatment addressing the differential impact of exposure time (see Tables S10–S13, ESI†) on metabolites and pathways. This same fact can be observed in Table 1 and Fig. S9 (ESI†). The involvement of glutathione in platinum drug efflux and its association to drug resistance has been discussed controversially in the past.70 Whereas Mohn et al.71 did not observe any glutathione alteration upon oxaliplatin incubation in in vitro studies of the colorectal cancer line HCT-8, others have shown variations.17 Finally, Fig. 7 gives the comparative metabolomic data on palmitoyl-carnitine, N1,N12-diacetylspermine and N8-acetylspermidine discovered by non-targeted analysis (level 1 and 2 identification, based on spectral library match). The long chain acyl-carnitine is a key metabolite of fatty acid catabolism, more specifically of fatty acid oxidation.72 This pathway is an essential source of NADH, FADH2, NADPH and ATP. Fatty acids are conjugated with carnitine for translocation into the mitochondria. Palmitoyl-carnitine has been reported to change the activity of several enzymes and transporters localized in the mitochondrial membrane and has been shown to stimulate caspase activity.73,74 Resistant cells showed consistently lower levels of palmitoyl-carnitine over 24 hours of incubation time, which were not affected by the drug uptake. In contrast, in sensitive cells, the levels decreased with time in the control samples while drug uptake resulted in the opposite trend (Fig. 7). There is growing interest in the role of fatty acid metabolism in cancer progression and metastasis,75 but little is known about its role in platinum drug efficacy and resistance. Increased levels of fatty acid oxidation have been linked to apoptosis.76 Already a decade ago, Riedel et al.77 observed that fatty acid metabolism played a role in cisplatin-resistance. More recently, a positive correlation between fatty acid oxidation and malignant phenotypes including metastasis, therapeutic resistance to cisplatin and relapse was found.78 Our data (see also Table 1) indicate that in the case of oxaliplatin not only the energy metabolism but also the fatty acid metabolism might be decisive for drug sensitivity. Hence, future studies should address the enzymes of the related pathways as potential drug targets reversing resistance. As shown by the metallomics approach, resistance was accompanied by differential drug uptake, which could be explained by differential transport. Related to fatty acid metabolism (see Table 1), interestingly, it has been shown that the organic cation/carnitine transporter (OCTN1) plays a role in oxaliplatin accumulation, which is in agreement with the differential platinum uptake found in sensitive versus resistant cells. It was found that (OCTN1)-mediated uptake of oxaliplatin might contribute to its neuronal accumulation and treatment-limiting neurotoxicity.63,64N8-Acetlyspermidine and N1,N12-diacetylspermine are metabolites of polyamine catabolism. Interestingly, polyamine metabolites were discussed as potential biomarkers for colon cancer and oxidative damage (as ROS is produced by polyamine catabolism). Recently, it has become increasingly clear that polyamine catabolism can play a dominant role in drug response, apoptosis and response to stressful stimuli.79 In the case of platinum drugs, polyamine catabolism was associated with renal toxicity.
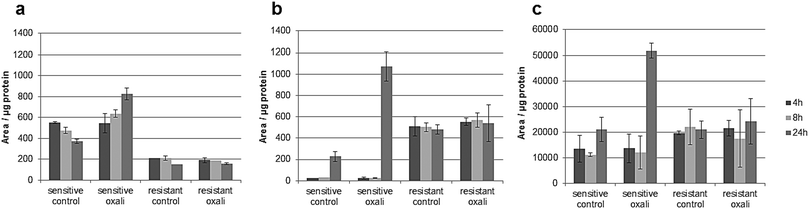 |
| Fig. 7 Relative area normalized to the protein content upon incubation time with 5 μM oxaliplatin in untargeted metabolomics in positive mode of (a) palmitoylcarnitine. Low values were observed for the resistant line whereas higher values were observed in the sensitive line which increased upon incubation time. (b) N1,N12-Diacetylspermine. No differences could be observed in the resistant line whereas high values were observed in the sensitive line upon incubation time. (c) N8-Acetylspermidine. No differences could be observed in the resistant line whereas high values were observed in the sensitive line upon incubation time. Column: UPLC BEH Amide at pH 4. | |
Conclusion
In this preclinical study, colon cancer resistance towards oxaliplatin was studied for the first time by an integrated metallomics/metabolomics approach. Previous studies have mainly focused on cisplatin and little is known about oxaliplatin in the context of the metabolome. Our study compared treated and untreated samples of colon cancer cells allowing not only a static comparison of the altered metabolism in resistant versus sensitive cells, but also whether the treatment induces (or not) diverse metabolic pertubations in the different cells. We found that the oxaliplatin sensitive HCT116 cell line displayed a metabolic phenotype which differed most significantly in hallmarks of energy metabolism, sulfur metabolism, fatty acid metabolism and polyamine metabolites. From metallomics data, it could be inferred that the resistance in the HCT116 model was accompanied by a different drug transport mechanism. It could be speculated that the sensitive cells were less protected against oxaliplatin, since glutathione levels and other intermediates of the sulfur metabolism were lower than in the resistant cells. Furthermore, polyamine catabolism was significantly dysregulated upon exposure to oxaliplatin which is known to increase the ROS burden to the cell.
In summary, the introduced methods revealed novel insights and provided valid tools for generating new hypotheses regarding resistance towards oxaliplatin. E.g., future studies will investigate whether inhibition of fatty acid oxidation has an effect on drug sensitivity and resistance and could potentially serve for reversing platinum resistance.
Conflicts of interest
There are no conflicts to declare.
References
- J. Favier, J. Brière and N. Burnichon,
et al., The Warburg Effect Is Genetically Determined in Inherited Pheochromocytomas, PLoS One, 2009, 4, 1–12 CrossRef PubMed.
-
G. G. Harrigan and R. Goodacre, Introduction: Metabolic profiling: pathways in drug discovery, in Metabolic Profiling: Its Role in Biomarker Discovery and Gene Function Analysis, ed. G. G. Harrigan and R. Goodacre, 2003, pp. 1–8 Search PubMed.
-
G. Kroemer, D. Mumberg, H. Keun, B. Riefke, T. Steger-Hartmann and K. Petersen, Using metabolomics to monitor anticancer drugs, in Oncogenes Meets Metabolism. From Deregulated Genes to a Broader Understanding of Tumour Physiology, ed. G. Stock and C. Klein, 2007, pp. 55–78 Search PubMed.
- D. Nagrath, C. Caneba, T. Karedath and N. Karedath, Bellance Metabolomics for mitochondrial and cancer studies, Biochim. Biophys. Acta, 2011, 1807, 650–663 CrossRef CAS.
- R. Beger, A Review of Applications of Metabolomics in Cancer, Metabolites, 2013, 3, 552–574 CrossRef CAS.
- L. B. Sullivan, D. Y. Gui and M. G. Van Der Heiden, Altered metabolite levels in cancer: implications for tumour biology and cancer therapy, Nat. Rev. Cancer, 2016, 16, 680–693 CrossRef CAS PubMed.
-
E. G.
Armitage and A. D. Southam, Monitoring cancer prognosis, diagnosis and treatment efficacy using metabolomics and lipidomics, Metabolomics, 2016, 12, 1–15 CrossRef PubMed.
- M. Frédérich, B. Pirotte, M. Fillet and P. DeTullio, Metabolomics as a Challenging Approach for Medicinal Chemistry and Personalized Medicine, J. Med. Chem., 2016, 59, 8649–8666 CrossRef PubMed.
- R. A. Cairns, I. S. Harris and T. W. Mak, Regulation of cancer cell metabolism, Nat. Rev. Cancer, 2011, 11 Search PubMed.
- L. Kelland, The resurgence of platinum-based cancer chemotherapy, Nat. Rev. Cancer, 2007, 7, 573–584 CrossRef CAS PubMed.
- D. Shaloam and P. B. Tchounwou, Cisplatin in cancer therapy: molecular mechanisms of action, Eur. J. Pharmacol., 2014, 740, 364–378 CrossRef PubMed.
-
I. Pötsch, D. Baier, B. K. Keppler and W. Berger, Challenges and Chances in the Preclinical to Clinical Translation of Anticancer Metallodrugs, in Metal-based anticancer agents, ed. A. Cassini, A. Vessières and S. M. Meier-Menches, The Royal Society of Chemistry, 2019, ch. 12, pp. 308–347 Search PubMed.
- J. S. Brown, R. Sundar and J. Lopez, Combining DNA damaging therapeutics with immunotherapy: more haste, less speed, Br. J. Cancer, 2018, 118, 312–324 CrossRef CAS PubMed.
- N. D. Eljack, H. Y. Ma, J. Drucker, C. Shen, T. W. Hambley, E. J. New, T. Friedrich and R. J. Clarke, Mechanisms of cell uptake and toxicity of the anticancer drug cisplatin, Metallomics, 2014, 6, 2126–2133 RSC.
- D. Gibson, Metal Anticancer Compounds processing The mechanism of action of platinum anticancer agents—what do we really know about it?, Dalton Trans., 2009, 10681–10689 RSC.
- T. Alcindor and N. Beauger, Oxaliplatin: a review in the era of molecularly targeted therapy, Curr. Oncol., 2011, 18, 18–25 CAS.
- L. Manzano, C. Bug and S. Bystrup, Tumor-Related Molecular Mechanisms of Oxaliplatin Resistance, Mol. Cancer Ther., 2015, 14, 1767–1776 CrossRef PubMed.
- K. O. Alfarouk, C. M. Stock and S. Taylor,
et al., Resistance to cancer chemotherapy: failure in drug response from ADME to P-gp, Cancer Cell Int., 2015, 1–13 CAS.
- L. M. Poisson, A. Munkarah and H. Madi,
et al., A metabolomic approach to identifying platinum resistance in ovarian cancer, J. Ovarian Res., 2015, 8, 1–14 CrossRef CAS PubMed.
- M. Groessl and C. G. Hartinger, Anticancer metallodrug research analytically painting the “omics” picture-current developments and future trends, Anal. Bioanal. Chem., 2013, 405, 1791–1808 CrossRef CAS PubMed.
- P. M. Bruno, Y. Liu and G. Y. Park,
et al., A subset of platinum-containing chemotherapeutic agents kills cells by inducing ribosome biogenesis stress, Nat. Med., 2017, 23, 461–471 CrossRef CAS PubMed.
- X. Zhu, Y. Cui and J. Tang,
et al., Serum Metabolic Profile Alteration Reveals Response to Platinum-Based Combination Chemotherapy for Lung Cancer: Sensitive Patients Distinguished from Insensitive ones, Sci. Rep., 2017, 7, 1–11 CrossRef.
- M. J. Slifker, X. Ma and M. S. Kurzer,
et al., Estrogen metabolism in the human lung: impact of tumorigenesis, smoke, sex and race/ethnicity, Oncotarget, 2017, 8, 106778–106789 Search PubMed.
- Y. Ni, G. Xie and W. Jia, Metabonomics of human colorectal cancer: new approaches for early diagnosis and biomarker discovery, J. Proteome Res., 2014, 13, 3857–3870 CrossRef CAS PubMed.
- M. Schwaiger, E. Rampler, G. Hermann, W. Miklos, W. Berger and G. Koellensperger, Anion-Exchange Chromatography Coupled to High-Resolution Mass Spectrometry: A Powerful Tool for Merging Targeted and Non-targeted Metabolomics, Anal. Chem., 2017, 89, 7667–7674 CrossRef CAS PubMed.
- T. Pivetta, S. Vascellari and E. Pinna,
et al., Mass spectrometric discrimination of phospholipid patterns in cisplatin-resistant and -sensitive cancer cells, Rapid Commun. Mass Spectrom., 2018, 33, 97–106 Search PubMed.
- T. Ezaki, S. Nishiumi, T. Azuma and M. Yoshida, Metabolomics for the early detection of cisplatin-induced nephrotoxicity, Toxicol. Res., 2017, 6, 843–853 RSC.
- L. Von Stechow, A. Ruiz-aracama, B. Van De Water, A. Peijnenburg and E. Danen, Identification of Cisplatin-Regulated Metabolic Pathways in Pluripotent Stem Cells, PLoS One, 2013, 8, 1–13 CrossRef.
- R. Cavill, A. Kamburov and J. K. Ellis,
et al., Consensus-phenotype integration of transcriptomic and metabolomic data implies a role for metabolism in the chemosensitivity of tumour cells, PLoS Comput. Biol., 2011, 7, 1–12 CrossRef PubMed.
- G. J. Patti, O. Yanes and G. Siuzdak, Metabolomics: the apogee of the omics trilogy, Nature Reviews, Mol. Cell. Biol., 2012, 13, 263–269 CAS.
- K. Dettmer, N. Nürnberger, H. Kaspar, M. A. Gruber, M. F. Almstetter and P. J. Oefner, Metabolite extraction from adherently growing mammalian cells for metabolomics studies: optimization of harvesting and extraction protocols, Anal. Bioanal. Chem., 2011, 399, 1127–1139 CrossRef CAS PubMed.
- C. Coman, F. A. Solari, R. Ahrends, A. Hentschel, A. Sickmann and R. P. Zahedi, Simultaneous Metabolite, Protein, Lipid Extraction (SIMPLEX): A Combinatorial Multimolecular Omics Approach for Systems Biology, Mol. Cell. Proteomics, 2016, 5, 1453–1466 CrossRef PubMed.
- E. S. Nakayasu, C. D. Nicora and A. C. Sims,
et al., MPLEx: a Robust and Universal Protocol, mSystems, 2016, 1, 1–14 CrossRef PubMed.
- S. C. Sapcariu, T. Kanashova, D. Weindl, J. Ghelfi, G. Dittmar and K. Hiller, Simultaneous extraction of proteins and metabolites from cells in culture, MethodsX, 2014, 1, 74–80 CrossRef PubMed.
- K. Ortmayr, S. Hann and G. Koellensperger, Complementing reversed-phase selectivity with porous graphitized carbon to increase the metabolome coverage in an on-line two-dimensional LC-MS setup for metabolomics, Analyst, 2015, 5, 3465–3473 RSC.
- K. Ortmayr, T. J. Causon, S. Hann and G. Koellensperger, Trends in Analytical Chemistry Increasing selectivity and coverage in LC-MS based metabolome analysis, Trends Anal. Chem., 2016, 82, 358–366 CrossRef CAS.
- J. Ivanisevic, Z. Zhu and L. Plate,
et al., Toward ‘ Omic Scale Metabolite Profiling: A Dual Separation – mass Spectrometry Approach for Coverage of Lipid and Central Carbon Metabolism, Anal. Chem., 2013, 16, 6876–6884 CrossRef PubMed.
- L. Jiang and M. Snyder, Optimized Analytical Procedures for the Untargeted Metabolomic Profiling of Human Urine and Plasma by Combining Hydrophilic Interaction ( HILIC) and Reverse-Phase Liquid Chromatography (RPLC) – Mass Spectrometry, Mol. Cell. Proteomics, 2015, 14, 1684–1695 CrossRef PubMed.
-
M. Cuykx, W. Jiang, A. Covaci and T. Centre, Hilic – UHPlc – MS as a Tool for Metabolomics Study, The applications book, 2016, vol. 40 Search PubMed.
- K. Spagou, H. Tsoukali, N. Raikos, H. Gika, I. D. Wilson and G. Theodoridis, Hydrophilic interaction chromatography coupled to MS for metabonomic/metabolomic studies, J. Sep. Sci., 2010, 33, 716–727 CrossRef CAS PubMed.
-
I. Kohler, R. J. E. Derks and M. Giera, The Rise of Hydrophilic Interaction Chromatography in Untargeted Clinical Metabolomics, 2016, http://www.chromatographyonline.com/rise-hydrophilic-interaction-chromatography-untargeted-clinical-metabolomics.
- H. Bi, K. W. Krausz, S. K. Manna, F. Li, C. H. Johnson and F. J. Gonzalez, Optimization of harvesting, extraction, and analytical protocols for UPLC-ESI-MS-based metabolomic analysis of adherent mammalian cancer cells, Anal. Bioanal. Chem., 2013, 405, 5279–5289 CrossRef CAS PubMed.
- G. Hermann, M. Schwaiger, P. Volejnik and G. Koellensperger,
13C-labelled yeast as internal standard for LC-MS/MS and LC high resolution MS based amino acid quantification in human plasma, J. Pharm. Biomed. Anal., 2018, 155, 329–334 CrossRef CAS PubMed.
- H. C. M. T. Prinsen, B. G. M. Schiebergen-Bronkhorst and M. W. Roeleveld,
et al., Rapid quantification of underivatized amino acids in plasma by hydrophilic interaction liquid chromatography (HILIC) coupled with tandem mass-spectrometry, J. Inherited Metab. Dis., 2016, 39, 651–660 CrossRef CAS PubMed.
- A. Teleki, A. Sánchez-Kopper and R. Takors, Alkaline conditions in hydrophilic interaction liquid chromatography for intracellular metabolite quantification using tandem mass spectrometry, Anal. Biochem., 2015, 475, 4–13 CrossRef CAS PubMed.
- S. Neubauer, C. Haberhauer-Troyer and K. Klavins,
et al., U13C cell extract of Pichia pastoris – a powerful tool for evaluation of sample preparation in metabolomics, J. Sep. Sci., 2012, 35, 3091–3105 CrossRef CAS PubMed.
- K. Klavins, S. Neubauer and A. Al Chalabi,
et al., Interlaboratory comparison for quantitative primary metabolite profiling in Pichia pastoris, Anal. Bioanal. Chem., 2013, 405, 5159–5169 CrossRef CAS PubMed.
- N. G. Mahieu, X. Huang, Y. J. Chen and G. J. Patti, Credentialing features: a platform to benchmark and optimize untargeted metabolomic methods, Anal. Chem., 2014, 86, 9583–9589 CrossRef CAS PubMed.
- S. A. Abramkin, U. Jungwirth and S. M. Valiahdi,
et al., {(1R,2R,4R)-4-Methyl-1,2-cyclohexanediamine}oxalatoplatinum(II): A Novel Enantiomerically Pure Oxaliplatin Derivative Showing Improved Anticancer Activity in vivo, J. Med. Chem., 2010, 7356–7364 CrossRef CAS.
- C. S. Dam, I. H. Lambert, B. Gammelgaard and S. Stürup, Distribution of platinum between nuclear and cytosolic fractions-can subcellular fractionation be performed quantitavely?, J. Pharm. Biomed. Anal., 2019, 165, 82–89 CrossRef CAS.
- M. Corte-Rodríguez, M. Espina and L. M. Sierra,
et al., Quantitative evaluation of cellular uptake, DNA incorporation and adduct formation in cisplatin sensitive and resistant cell lines: comparison of different Pt-containing drugs, Biochem. Pharmacol., 2015, 98, 69–77 CrossRef PubMed.
- Y. Wu and L. Li, Sample normalization methods in quantitative metabolomics, J. Chromatogr. A, 2015, 1430, 80–95 CrossRef.
- N. Bordag, U. Rennefahrt and J. Nachtigall,
et al., Fast Sampling of Adherent Cell Cultures for Optimal Metabolomics Results, Metabolomics: Open Access, 2016, 6, 1–4 Search PubMed.
- R. J. Deberardinis and N. S. Chandel, Fundamentals of cancer metabolism, Sci. Adv., 2016, 2, 1–18 Search PubMed.
- M. Schwaiger, H. Schoeny, Y. El Abiead, G. Hermann, E. Rampler and G. Koellensperger, Merging metabolomics and lipidomics into one analytical run, Analyst, 2019, 144, 220–229 RSC.
- C. R. Kowol, B. K. Keppler, W. Berger, P. Heffeter, U. Jungwirth and C. G. Hartinger, Anticancer Activity of Metal Complexes: Involvement of Redox Processes, Antioxid. Redox Signaling, 2011, 15, 1085–1127 CrossRef PubMed.
- L. Galvez, M. Rusz, M. A. Jakupec and G. Koellensperger, Heart-cut 2DSEC-RP-LC-ICP-MS as a screening tool in metal-based anticancer research, J. Anal. At. Spectrom., 2019, 34, 1279–1286 RSC.
- S. R. Alberts, R. K. Ramanathan and B. P. Findlay,
et al., A Randomized Controlled Trial of Fluorouracil Plus Leucovorin, Irinotecan, and Oxaliplatin Combinations in Patients With Previously Untreated Metastatic Colorectal Cancer, J. Clin. Oncol., 2003, 22, 23–30 Search PubMed.
- B. Stordal, N. Pavlakis and R. Davey, Oxaliplatin for the treatment of cisplatin-resistant cancer: a systematic review, Cancer Treat. Rev., 2007, 33, 347–357 CrossRef CAS PubMed.
- P. Heffeter, U. Jungwirth and M. Jakupec,
et al., Resistance against novel anticancer metal compounds: differences and similarities, Drug Resist. Updates, 2008, 11, 1–16 CrossRef CAS PubMed.
- B. Englinger, C. Pirker, P. Heffeter, A. Terenzi, C. R. Kowol, B. K. Keppler and W. Berger, Metal Drugs and the Anticancer Immune Response, Chem. Rev., 2019, 119, 1519–1624 CrossRef CAS PubMed.
-
U. Jungwirth, Thesis: Pre-clinical investigations of novel platinum- and gallium-based anticancer drugs, 2012, http://repositorium.meduniwien.ac.at/obvumwhs/download/pdf/2712451?originalFilename=true.
- H. Burger, W. J. Loos, K. Eechoute, J. Verweij, R. H. J. Mathijssen and E. A. C. Wiemer, Drug transporters of platinum-based anticancer agents and their clinical significance, Drug Resist. Updates, 2011, 14, 22–34 CrossRef CAS PubMed.
- N. N. Jong, T. Nakanishi, J. J. Liu, I. Tamai and M. J. McKeage, Oxaliplatin transport mediated by organic cation/carnitine transporters OCTN1 and OCTN2 in overexpressing human embryonic kidney 293 cells and rat dorsal root ganglion neurons, J. Pharmacol. Exp. Ther., 2011, 338, 537–547 CrossRef CAS PubMed.
- H. Burger, A. Zoumaro-Djayoon, A. W. M. Boersma, J. Helleman, E. M. J. J. Berns, R. H. J. Mathijssen, W. J. Loos and E. A. C. Wiemer, Differential transport of platinum compounds by the human organic cation transporter hOCT2 (hSLC22A2), Br. J. Pharmacol., 2010, 159, 898–908 CrossRef CAS.
- L. W. Sumner, A. Amberg and D. Barrett,
et al., Proposed minimum reporting standards for chemical analysis, Metabolomics, 2007, 3, 211–221 CrossRef CAS PubMed.
- D. J. Biau, B. M. Jolles and R. Porcher, P Value and the Theory of Hypothesis Testing, Clin. Orthop. Relat. Res., 2010, 468, 885–892 CrossRef PubMed.
- P. P. Hsu and D. M. Sabatini, Cancer cell metabolism: Warburg and beyond, Cell, 2008, 134, 703–707 CrossRef CAS PubMed.
- S. Mazurek, C. B. Boschek, F. Hugo and E. Eigenbrodt, Pyruvate kinase type M2 and its role in tumor growth and spreading, Semin. Cancer Biol., 2005, 15, 300–308 CrossRef CAS.
- Y. Kasherman, S. Sturup and D. Gibson, Is glutathione the major cellular target of cisplatin? A study of the interactions of cisplatin with cancer cell extracts, J. Med. Chem., 2009, 52, 4319–4328 CrossRef CAS PubMed.
- C. Mohn, H. G. Häcker, R. A. Hilger, M. Gütschow and U. Jaehde, Defining the role of MRP-mediated efflux and glutathione in detoxification of oxaliplatin, Pharmazie, 2013, 68, 622–627 CAS.
- N. Longo, M. Frigeni, M. Pasquali, B. Biophys and A. Author, Carnitine transport and fatty acid oxidation h, Biochim. Biophys. Acta, 2016, 1863, 2422–2435 CrossRef CAS.
- M. A. B. Melone, A. Valentino, S. Margarucci, U. Galderisi, A. Giordano and G. Peluso, The carnitine system and cancer metabolic plasticity review-article, Cell Death Dis., 2018, 9, 1–12 CrossRef CAS.
- M. C. Mutomba, H. Yuan and M. Konyavko,
et al., Regulation of the activity of caspases by L-carnitine and palmitoylcarnitine, FEBS Lett., 2000, 478, 19–25 CrossRef CAS PubMed.
- A. van Weverwijk, N. Koundouros, M. Iravani, M. Ashenden, Q. Gao, G. Poulogiannis, U. Jungwirth and C. M. Isacke, Metabolic adaptability in metastatic breast cancer by AKR1B10-dependent balancing of glycolysis and fatty acid oxidation, Nat. Commun., 2019, 10, 1–13 CrossRef CAS PubMed.
- D. G. Tang, E. La, J. Kern and J. P. Kehrer, Fatty Acid Oxidation and Signaling in Apoptosis, Biol. Chem., 2002, 383, 425–442 CAS.
- R. F. Riedel, A. Porrello and E. Pontzer,
et al., A genomic approach to identify molecular pathways associated with chemotherapy resistance, Mol. Cancer Ther., 2008, 7, 3141–3149 CrossRef CAS PubMed.
- C. Y. Kuo and D. K. Ann, When fats commit crimes: fatty acid metabolism, cancer stemness and therapeutic resistance, Cancer Commun., 2018, 38, 1–12 CrossRef PubMed.
- R. A. Casero, T. Murray Stewart and A. E. Pegg, Polyamine metabolism and cancer: treatments, challenges and opportunities, Nat. Rev. Cancer, 2018, 18, 681–695 CrossRef PubMed.
Footnote |
† Electronic supplementary information (ESI) available. See DOI: 10.1039/c9mt00141g |
|
This journal is © The Royal Society of Chemistry 2019 |