Patterns and drivers of maternal personal exposure to PM2.5 in informal settlements in Nairobi, Kenya†
Received
25th May 2023
, Accepted 5th April 2024
First published on 5th April 2024
Abstract
There is limited data on personal exposure to fine particulate matter (PM2.5) for mothers in informal settlements in Africa. Identifying and characterizing the sources of exposure to PM2.5 for this population is key to finding potential solutions for mitigating air pollution exposures. As part of the USAID-funded Kuboresha Afya Mitaani Urban Maternal, Newborn and Child Health project in Nairobi, Kenya, this study aimed to (1) characterize and map environments that contribute to fine particulate matter (PM2.5) exposure for mothers and infants and (2) determine which factors drive exposure to PM2.5. Mothers were enrolled and measured for 24 hours with PM2.5 monitors and GPS loggers in two informal settlements in Nairobi (Dagoretti and Starehe), with complete data received from 73 participants. Two ambient PM2.5 monitors were also installed in each of the respective communities. Time-activity surveys were administered following the monitoring period. Mean daily exposures were 39.9 and 45.5 µg m−3 in Dagoretti and Starehe, respectively, with 60% of samples exceeding the annual WHO Annual Interim-1 Target of 35 µg m−3. Normal daily activities such as sleeping, resting, running errands, and visiting with friends constituted over three-quarters of exposure sampling time. These activities were not associated with elevated PM2.5 exposures, and generally tracked diurnal ambient patterns (morning and evening peaks). Personal exposures, however, did peak higher than ambient in the evenings when cooking was most common, and cooking with wood or charcoal was associated with higher PM2.5 personal exposures (daily mean of 54.2 µg m−3 [n = 16], compared to 40.3 µg m−3 for those who used gas, liquid fuels or electricity [n = 57]). These results suggest that transitioning households to cleaner fuels, such as electricity, LPG, or ethanol, would be the most promising intervention that could rapidly reduce exposures of mothers in informal settlements where biomass use is common. At the same time, larger-scale sectoral efforts are needed to bring ambient PM2.5 concentrations, and thus the overall population exposures, closer to WHO guidelines.
Environmental significance
There is limited data on personal exposure for pregnant women and new mothers in informal settlements in Africa. Identifying and characterizing the sources of exposure for these women is key to finding potential solutions for mitigating air pollution exposures for them and their children. We found that this subpopulation is exposed to daily PM2.5 concentrations above World Health Organization air quality targets and guidelines. Ambient air pollution was likely the largest driver of exposure but requires the greatest sectoral changes. The most important non-ambient driver that could be addressed in the short term is likely use of low-quality cooking fuels. Given the prevalence of charcoal, wood, and kerosene for cooking in urban Africa, this work underscores the need to address these sources in efforts to drive down personal exposures for mothers and their children.
|
1 Introduction
Exposure to air pollution is associated with several adverse maternal and child health outcomes, with recent reviews reporting associations with low birthweight, stillbirth, growth restrictions, preterm birth, and other gestational complications.1–5 Recent studies have also shown associations between exposure to fine particulate matter (PM2.5) and COVID-19 risk,6,7 which is of concern given increased maternal mortality and risk of miscarriage associated with COVID-19.8,9 Childhood morbidity and mortality, primarily from acute lower respiratory infections (ALRI), are also associated with air pollution exposure,10–12 with ALRI being the leading cause of death for children under five.13
These health concerns are of critical importance for mothers and children living in informal settlements, where adverse health outcomes are higher than the national average. In a study of two of Nairobi's informal settlements, the maternal mortality rate was estimated at 709 per 100
000 live births, which is twice the national average (362 per 100
000).14 Neonatal and child mortality is also high, with the neonatal mortality rate estimated at 14.4 per 1000 live births, an infant mortality rate of 39.2 per 1000 live births, and an under-five mortality rate of 79.8 per 1000 live births.14 These health risks, coupled with the risk of air pollutant exposure, puts women and children in these settings at an added disadvantage.
While there is little direct data on personal exposures for mothers in informal settlements in Nairobi, ambient and other exposure studies suggest they are likely exposed to high levels of PM2.5. A review of air pollution studies in Kenya by deSouza,15 for example, found the majority of the studies reported ambient PM2.5 concentrations exceeding the previous WHO 24 hours and annual PM2.5 guidelines (25 and 10 µg m−3, respectively), and those which were associated with informal neighborhoods were often above 100 µg m−3. A recent study with several PM2.5 ambient installations across Nairobi reported 24 hours mean PM2.5 concentration ranging from 22 to 112 µg m−3, with one of the sites close to informal settlements (Mathare) measured at 50 µg m−3.16 Another study in 2017 documented somewhat lower, almost year-long ambient concentrations near informal settlements by Kibera and Viwandani of 23 and 21 µg m−3, respectively.17
These studies have helped characterize the relatively high ambient PM2.5 concentrations in Nairobi, as well as potential associations with land-use zones and pollution sources, such as vehicle emissions; however, they have not provided granular exposure estimates for subpopulations which may be especially impacted by air quality, such as expecting mothers in informal settlements, nor analyses of specific drivers of those exposure estimates. A better understanding of what is driving potentially high exposures for mothers is needed to provide insight into specific interventions or approaches that can help mitigate these exposures. Towards this end, this study aimed to: (1) characterize and map environments that contribute to PM2.5 exposure for mothers in informal settlements in Nairobi, and (2) determine which factors are associated with increased exposure to PM2.5 and prospects for mitigating that exposure through interventions. To address these objectives, we measured location and PM2.5 exposure for new and expecting mothers in two informal settlements in Nairobi, and cross-referenced that data with participant-reported time-activities, in an effort to characterize exposure and potential sources for this population.
2 Methods
2.1 Kuboresha Afya Mitaani (KAM) project
This air quality study was conducted as part of the Kuboresha Afya Mitaani (KAM) implementation research project (also known as the Urban Maternal, Newborn and Child Health (MNCH) project). The KAM project is led by Jacaranda Health with support from Population Council, the Nairobi City County government, Sanergy, and Berkeley Air Monitoring Group, and funded by USAID. The project is applying implementation research to characterize and improve maternal and child health outcomes in informal settlements in Nairobi. The overarching approach is to develop systems that improve quality of care and better health outcomes by integrating often siloed actors, such as the families themselves and care providers/facilities, as well as considering environmental health concerns. The need for better MNCH services is acute in these informal settlements, which have high maternal and child mortality rates.12 Respiratory infections, diarrheal diseases, and malnutrition have contributed to the elevated mortality and morbidity in these communities, which are also largely below the poverty line.18
2.2 Sampling and screening procedure
The site selection and participant recruitment process was designed to make the study population representative of the KAM program, limiting participants to expecting and new mothers aged 15–49 years with babies 0–11 months, living in the selected KAM study villages, and expecting to stay in the same location for the next 6 months. The sampling frame was established through a household listing completed in September 2020 by trained Community Health Volunteers from Dagoretti and Starehe. A total of 6429 eligible households were listed. Participants were pre-screened from the study pool to determine their willingness to enroll and then stratified by neighborhood to ensure geographic distribution across the study location. Of the pre-screened sample, a random sample of 209 households was targeted for participation. The sampling was conducted from February 22, 2021, to March 26, 2021.
Ethical approval for human subjects research was provided by the Population Council's Institutional Review Board (PC IRB 928) and the African Medical and Research Foundation Ethics and Scientific Review Committee (AMREF-ESRC 788/2020). Informed consent was obtained from all women before participating.
2.3 Personal exposure and location monitoring
PurpleAir monitors (model PA-II SD) were used for ambient and personal exposure PM2.5 monitoring and Columbus P-1 GPS loggers were used to log location (Fig. 1). PurpleAir monitors, which employ the Plantower PMS 5003 laser sensors to detect particulate matter, have been used extensively for low-cost monitoring networks,19–22 including in Africa,23–26 and have demonstrated strong performance when corrected for humidity impacts and calibrated against reference-grade monitoring instrumentation.27–29 The PurpleAir monitoring devices recorded data, including relative humidity, at approximately two-minute intervals and the GPS logger recorded every minute. The instruments were secured to a foam insert, which had a USB power supply for the PurpleAirs and was placed within a new mesh-sided backpack for each participant (see Fig. 1). Given potential safety concerns for the participants, extra consideration was given to ensuring that the equipment was discrete, which meant that conspicuous placement of the instrumentation nearer the breathing zone was not practical.
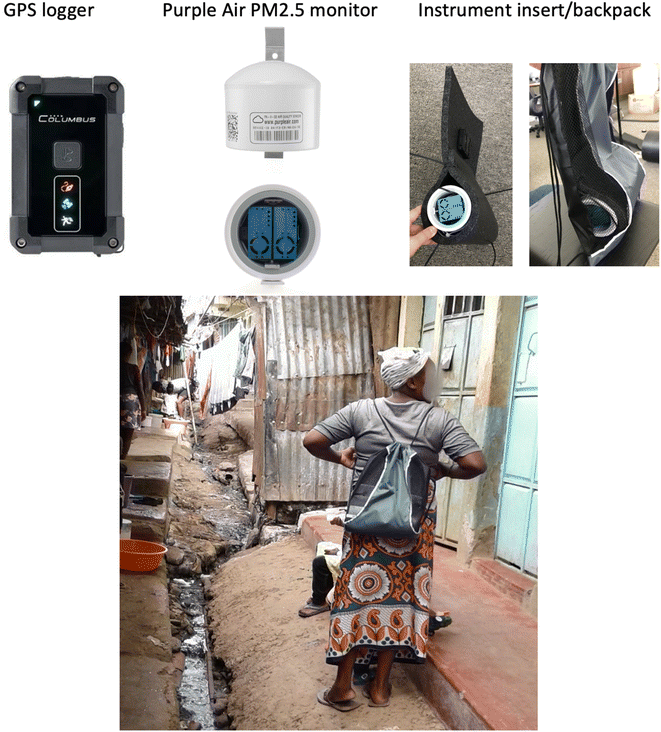 |
| Fig. 1 Instruments used in this study. From top left to right, the Columbus P-1 GPS logger, PurpleAir PA-II-SD, the foam pad used to affix the PurpleAir for insertion into a sampling pack, the mesh-sided backpack used to hold the instrumentation; and shown deployed in the lower panel. | |
In accordance with Giordano et al.30 PurpleAir PM2.5 data were first cleaned by removing all “NA” values; then negative PM2.5 readings on both channels were checked as well as relative humidity readings greater than 100 percent. Neither instance was found in the data. The PurpleAir PM2.5 estimates were corrected for humidity and PM2.5 response via a linear regression with a co-located PurpleAir monitor and real-time federal reference method sampler (BAM 1022, Met One Instruments) at the University of Nairobi that was running at the same time as our field campaign. Co-location data was available from 1420 hours of monitoring. The calibration correction was as follows:
PM2.5_corrected = a × (average of PurpleAir PM2.5 channels [µg m−3]) + b × (relative humidity [%]) + c, |
where
a = 0.58,
b = −0.065, and
c = 9.7157. The regression had a root mean square error of 0.478, and a correlation coefficient (
r) of 0.962, indicating a high degree of correlation between the PurpleAir and BAM 1022 (see a scatter plot in Fig. S1 of the ESI
†). PurpleAir data files with durations of less than 18 hours were not included in the analysis. Zero exposure samples were removed due to short durations, and 14 incomplete days for ambient sampling were excluded, which occurred when the PurpleAir was picked up or visited for battery replacement.
GPS data was used to determine potential pollution hotspots and categorize the participants' location as home vs. away from home. ‘Away from home’ was defined as 100 m or more away from the home location, a large distance range due to measurement uncertainty when GPS loggers operate indoors. A sensitivity analysis with less conservative ranges (50 and 75 m) did not yield substantially different results. While away from the home, recorded GPS speed was used to determine transportation type. Once it was determined that the participant was away from home, individual GPS data points were classified as either static or movement using a method similar to the one outlined by Wu et al.31 GPS data points classified as movement were then reclassified as ‘walking’ if the speed fell below 2 m s−1, as ‘biking’ if the speed was between 2 and 9 m s−1, and as ‘Matatu’ (local minibus) if the speed was above 9 m s−1. These classifications were then checked against participant responses. The analysis only included periods of transportation if the participant reported using that mode of transportation during monitoring.
2.4 Survey and time-activity
Enumerators conducted the surveys in the participant's preferred language (English or Kiswahili) and entered data into the data collection platform Open Data Kit (ODK, https://www.getodk.org/) on Android tablets. Participants took part in two rounds of surveys on either side of the 24 hours monitoring period. First, a pre-sampling survey focused on demographic and socioeconomic information, followed by a post-sampling survey, which recorded all the activities the participant engaged in over the previous day, including transportation to and from locations where these activities took place, with special attention paid to any potential sources of air pollutant exposures.
2.5 Data analysis
All analyses for summary statistics and plots were generated and developed using R 4.2.1. Socioeconomic status analysis (SES) was conducted using the prcomp function from the R 4.1.1 stats package. Assignment to SES groups was based on the households' quintile positioning within the prediction of the first PCA component (household PCA scores and associated quintiles are provided as a csv file in the ESI†). Daily PM2.5 exposures were compared to the WHO annual targets and guidelines (35 and 5 µg m−3, respectively)32 as they were assumed to be chronic and representative of typical of long-term exposures, as has been done for many exposure studies.33–36 The WHO Interim 1 Target of 35 µg m−3 is also the annual standard for Kenya in industrial areas (no target is provided for residential or other environments).37
3 Results
3.1 Sampling and participant characteristics summary
The study team initially intended to sample the exposure of 100 mothers, but a suspension of the data collection campaign due to COVID-19 limited the sample to 78 participants, and data loss due to instrument and data entry errors resulted in a total sample size of 73 mothers (Table 1). The initial sample size target was mainly determined by the resources available, and also informed by the expected confidence intervals of a 100-person sample size. Assuming a coefficient of variation of 108% from another exposure study in Kenya,38 this variability and a 100-person sample size would imply 95% confidence intervals at 20% of the mean estimate. Data collection and sampling in Dagoretti began later than the sampling in Starehe, resulting in a smaller sample size when COVID-19 restrictions forced the suspension of field activities.
Table 1 Sample sizes for data collected at each study subcounty and in each data stream
Study subcounty |
24 hours-personal exposure monitoring samples |
GPS samples |
Pre-sampling survey |
Post-sampling survey |
Starehe |
47 |
47 |
48 |
48 |
Dagoretti |
29 |
30 |
30 |
29 |
Total |
76 |
77 |
78 |
77 |
Samples with valid survey and PurpleAir exposure data |
|
73 |
|
|
|
Table 2 summarizes the basic demographic information collected on the participants (more detailed demographic information is provided in Table S1 of the ESI†). The populations were generally similar between Dagoretti and Starehe, with some small differences suggesting slightly higher socioeconomic status in Starehe (e.g., more television ownership and more participants with higher levels of education). About 22% of the participants had not completed secondary school. The largest difference between the communities was in housing type, with more Starehe residents in apartment-style buildings and more Dagoretti residents in stand-alone homes that shared walls with others. Overall, access to electricity was high in both communities. Income-generating activities for the primary earner were most frequently reported to be casual labor (i.e., construction; 40%), followed by own business (i.e., shop owner, taxi driver; 23%).
Table 2 Demographic characteristics of sample population (more detailed demographic data is presented in Table S1 of the ESI)
|
Dagoretti (n = 28) |
Starehe (n = 45) |
Total (n = 73) |
The 3rd quintile is, by definition, the middle of the 5 quintiles for the entire sample.
|
Age (years)
|
Mean (SD) |
30.0 (5.2) |
28.5 (4.3) |
29.1 (4.7) |
Range |
20.0–45.0 |
22.0–39.0 |
20.0–45.0 |
![[thin space (1/6-em)]](https://www.rsc.org/images/entities/char_2009.gif) |
Access/ownership
|
Electricity |
27 (96.4%) |
42 (93.3%) |
69 (94.5%) |
Radio |
20 (71.4%) |
27 (60.0%) |
47 (64.4%) |
TV |
22 (78.6%) |
28 (62.2%) |
50 (68.5%) |
Mobile phone |
28 (100.0%) |
44 (97.8%) |
72 (98.6%) |
![[thin space (1/6-em)]](https://www.rsc.org/images/entities/char_2009.gif) |
Marital status
|
Divorced |
0 (0.0%) |
2 (4.4%) |
2 (2.7%) |
Living together |
0 (0.0%) |
1 (2.2%) |
1 (1.4%) |
Married |
23 (82.1%) |
31 (68.9%) |
54 (74.0%) |
Separated |
2 (7.1%) |
3 (6.7%) |
5 (6.8%) |
Single (never married) |
2 (7.1%) |
8 (17.8%) |
10 (13.7%) |
Widowed |
1 (3.6%) |
0 (0.0%) |
1 (1.4%) |
![[thin space (1/6-em)]](https://www.rsc.org/images/entities/char_2009.gif) |
Education level
|
College/university (undergraduate) |
2 (7.1%) |
7 (15.6%) |
9 (12.0%) |
No formal education |
0 (0.0%) |
2 (4.4%) |
2 (2.7%) |
Primary complete |
10 (35.7%) |
10 (22.2%) |
20 (27.4%) |
Primary incomplete |
1 (3.6%) |
4 (8.9%) |
5 (6.8%) |
Secondary level complete |
7 (25.0%) |
11 (24.4%) |
18 (24.7%) |
Secondary level incomplete |
6 (21.4%) |
10 (22.2%) |
16 (21.9%) |
Vocational/technical school |
2 (7.1%) |
1 (2.2%) |
3 (4.1%) |
![[thin space (1/6-em)]](https://www.rsc.org/images/entities/char_2009.gif) |
Primary cooking fuel
|
Charcoal |
5 (17.9%) |
3 (6.7%) |
8 (11.0%) |
Electricity |
0 (0.0%) |
1 (2.2%) |
1 (1.4%) |
Kerosene |
3 (10.7%) |
15 (33.3%) |
18 (24.7%) |
LPG |
20 (71.4%) |
26 (57.8%) |
46 (63.0%) |
![[thin space (1/6-em)]](https://www.rsc.org/images/entities/char_2009.gif) |
House type
|
Apartment style home in a single structure/building with many homes |
1 (3.6%) |
25 (55.6%) |
26 (35.6%) |
Multiple structures (compound-style) |
10 (35.7%) |
12 (26.7%) |
22 (30.1%) |
Stand-alone home with no shared walls |
1 (3.6%) |
1 (2.2%) |
2 (2.7%) |
Stand-alone home with shared walls |
16 (57.1%) |
7 (15.6%) |
23 (31.5%) |
![[thin space (1/6-em)]](https://www.rsc.org/images/entities/char_2009.gif) |
Socioeconomic index
|
Mean quintile |
2.9 |
3.1 |
3.0a |
The data on ownership of goods and household construction characteristics were used to calculate a socioeconomic status (SES) score using principal components analysis (PCA). The mean ownership and characteristic fractions are presented by quintiles in Table S2 of the ESI,† and the PCA scores for each household are also provided in the ESI† (see SES_data_with_score_by_hhid.csv). LPG was reported as the most common primary fuel, followed by kerosene and charcoal. About 30% of homes reported using multiple cooking fuel types during the sampling (see Table S3 in the ESI† for the specific fuel mix breakdowns). The specific reported fuel type for a given event was used in the various analyses presented here.
3.2 Exposure and ambient concentrations
Personal PM2.5 exposures and GPS data were collected for 29 participants in Dagoretti, and 46 in Starehe (Table 3). Ambient PM2.5 data was collected for 16 days in Dagoretti, over two administrative wards (Kabiro and Dagoretti North), and 13 days in Starehe, also over two administrative wards (Kiamiako and Kiboro). The two sites in Dagoretti and Starehe were averaged to provide one estimate per administrative ward. More than half the personal exposure samples exceeded WHO Interim 1 guidelines (35 µg m−3) and the variability between participants was high, which we explored using regression models described in later sections. An example of the time series showing the personal exposure, with concurrent ambient data, and the GPS-derived home versus away categorization is shown in Fig. S2 in the ESI.†
Table 3 PurpleAir overall summary table for the personal and ambient samples
Sample type |
Location |
N (days) |
N greater than 35 µg m−3 |
Min PM2.5 (µg m−3) |
Median PM2.5 (µg m−3) |
Mean PM2.5 (µg m−3) |
Max PM2.5 (µg m−3) |
SDa PM2.5 (µg m−3) |
SD is standard deviation.
|
Ambient |
Dagoretti |
16 |
2 |
27.2 |
27.2 |
28.5 |
40.3 |
5.0 |
Ambient |
Starehe |
13 |
9 |
25.5 |
35.8 |
35.3 |
42.9 |
4.9 |
Personal |
Dagoretti |
28 |
13 |
13.6 |
31.8 |
39.9 |
100.6 |
22.9 |
Personal |
Starehe |
45 |
31 |
19.8 |
37.4 |
45.5 |
172.7 |
27.0 |
Ambient concentrations averaged 28.5 µg m−3 (standard deviation = 5.0 µg m−3) in Dagoretti, with only two of the 16 days above WHO Interim 1 guidelines of 35 µg m−3. Ambient concentrations were higher in Starehe, with 9 of the 13 days exceeding the WHO guidance, and a mean of 35.3 µg m−3 (SD = 4.9 µg m−3) (Table 3 and corresponding box plot provided in Fig. S3 of ESI†). Personal exposures were generally higher than the ambient concentrations, with an average of 39.9 µg m−3 (SD = 22.9 µg m−3) for the 28 samples collected in Dagoretti, and 45.5 µg m−3 (SD = 27.0 µg m−3) for the 45 samples collected in Starehe. The median exposures were within 6 µg m−3 of one another in both subcounties, suggesting that the differences in mean exposures were being driven by a few high exposure samples. Fig. 2 shows a comparison of realtime exposures and ambient concentrations averaged over the course of a day. The exposures generally track ambient concentrations, with the exception of evenings, when personal exposures peak to higher concentrations compared to ambient.
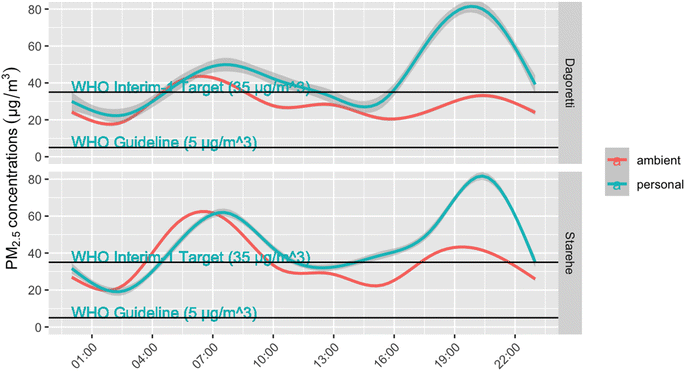 |
| Fig. 2 Smoothed time series data showing the mean values by time of day for all the combined personal and all the combined ambient samples, and by subcounty. Horizontal lines show WHO PM2.5 Annual Interim 1 Target (35 µg m−3) and guideline (5 µg m−3). | |
3.3 Exposure and location
The lower ambient concentrations are corroborated by the personal exposure data linked with the GPS data. Fig. 5 shows that when the data is categorically separated by ‘at home’ vs. ‘away from home’ using the GPS data, there was a pattern of lower exposures away (medians of 24.9 µg m−3 and 25.7 µg m−3 in Dagoretti and Starehe, respectively) versus at home (medians of 33.3 µg m−3 and 38.7 µg m−3 in Dagoretti and Starehe, respectively). All the personal exposure data collected was also categorically separated into 20 m grids and averaged in order to generate a pollution heat map to help us identify potential pollution hot spots over the sampled subcounties; however, there was minimal indication of areas of concern (see Fig. 3 and 4). The 20 m resolution was selected for the pollution heat map because it aligns with the accuracy of the GPS loggers, allowing for a distinction between pollution exposure levels at home and in other locations within the informal settlements of Nairobi.
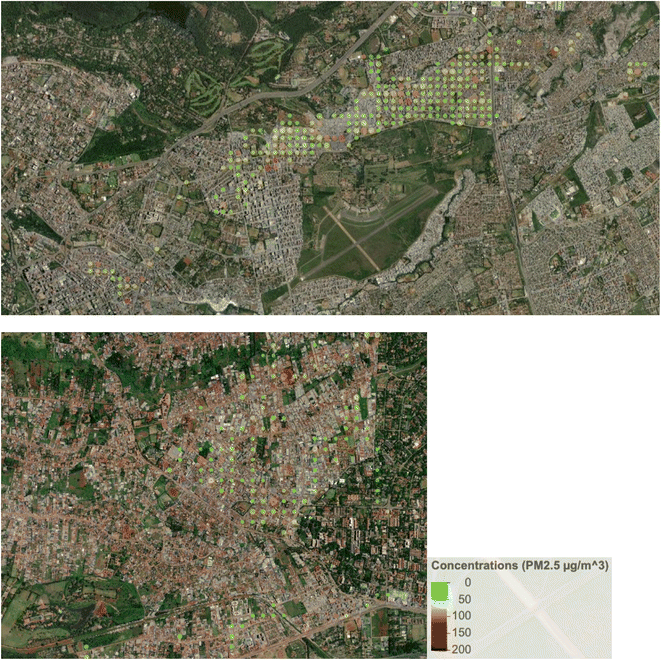 |
| Fig. 3 Average PM2.5 concentrations for the Starehe (top) and Dagoretti subcounties, combining all available data, and averaging it by 20 m grids. | |
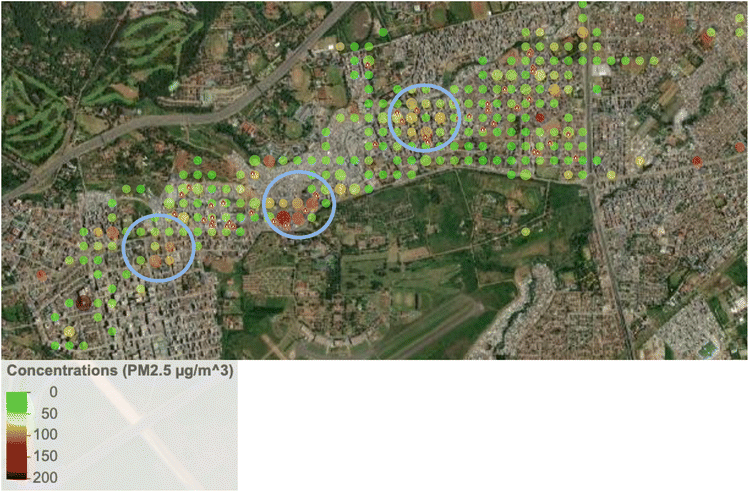 |
| Fig. 4 Potential PM2.5 hotspots in the Starehe subcounty. | |
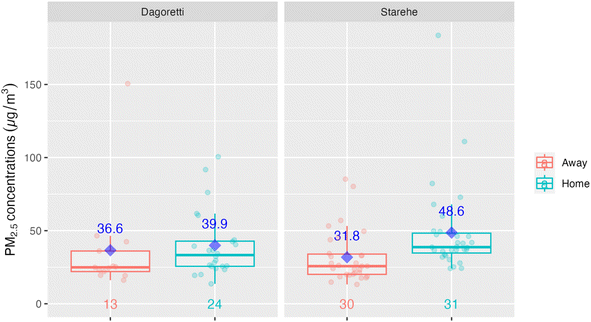 |
| Fig. 5 Daily averages of the PM2.5 concentrations, binned by whether the participant was at home or away from home (as derived from GPS data). Boxes represent the 25th and 75th percentiles, the whiskers are 1.5 times the interquartile range, the center line represents the median, and the numbers at the bottom are the sample sizes. Note: the sample size is of Starehe (N = 31) and Dagoretti (N = 24) combined is lower than the total study sample size (73) due to the samples needing viable GPS and PurpleAir data. | |
3.4 Exposure and activities
Time activity data collected at the end of the sampling period provided time ranges during which participants took part in various activities, such as cooking with a given stove type or using transportation. These data are plotted below in Fig. 6, with the highest exposures being associated with biomass stove use, although relatively few participants relied on these fuels (with the majority relying on LPG or kerosene). ‘Away tasks’ (a catch-all category was used for multiple activities including working, errands, shopping, and visiting friends), and home tasks (chores, resting, sleeping, and errands at home) were respectively grouped as they had similar exposure levels thus, did not provide additional instructive information by disaggregating. The study team also time-weighted the concentrations by activity, and compared to the total exposures for the respective samples. Given this weighting, home tasks, even with relatively low exposures, were associated with the highest contribution per daily exposure sample (70%) as these had the longest associated times (75% of the exposure monitoring time, including sleeping times, for example). As there were not elevated exposures during the home tasks, it is likely that much of the exposure during these times was driven by background or ambient concentrations. On the other hand, cooking with biomass stoves indicated a different pattern with shorter time periods, but higher relative contributions to exposure, suggesting these tasks have a direct effect on increasing exposures. Specifically, cooking with biomass was associated with the second-highest respective contributions towards personal exposures at 17.9%, even though they accounted for only about 11% of the exposure sample times. See Fig. S4 and Table S4 in the ESI† for a detailed breakdown of time-weighted exposure contributions.
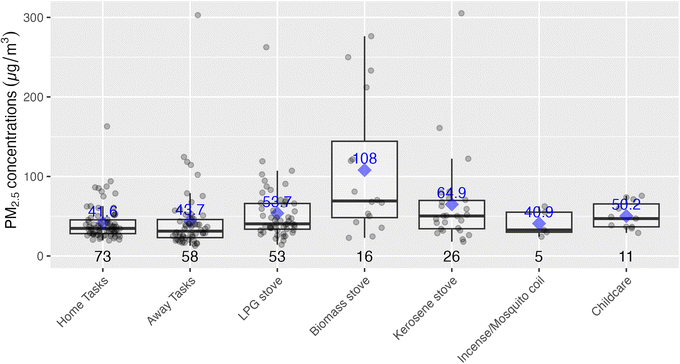 |
| Fig. 6 Activities and the corresponding concentrations during the activity periods. There were no substantial differences by subcounty, so data from the subcounties have been grouped. Boxes represent the 25th and 75th percentiles, the whiskers are 1.5 times the interquartile range, the black line represents the median, and blue diamonds represent the group averages. Numbers at the bottom are the number of events reported for the respective tasks. Biomass includes wood and charcoal stoves. | |
Below Fig. 7 shows an analysis looking specifically at the mode of transportation which suggested that exposures may be lower during walking when compared to riding a bicycle or using a matatu (local minibuses for public transport), although our analysis included relatively small sample sizes for the latter two modes of transport. At the same time, the means and medians for biking and riding in matatus were well above the WHO Annual Interim 1 Target (35 µg m−3), indicating a substantial source of exposure for some members of the study population.
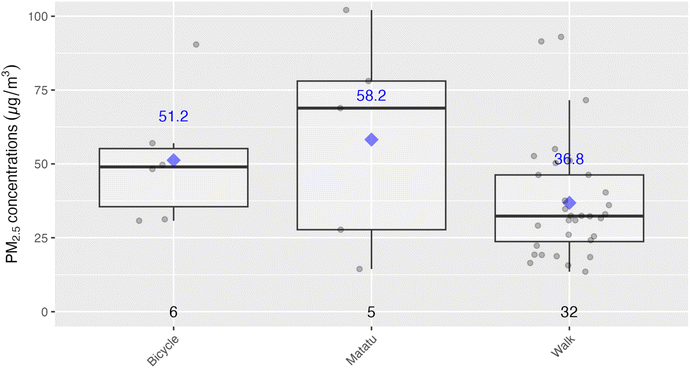 |
| Fig. 7 Exposures during recorded transportation events. Boxes represent the 25th and 75th percentiles, the whiskers are 1.5 times the interquartile range, the black line represents the median, and blue diamonds accompanied by number in blue text represent the group averages. Numbers at the bottom are the sample sizes. | |
4 Discussion
4.1 Exposure context
Overall, personal PM2.5 exposures were relatively high, exceeding the WHO Annual Interim 1 Target of 35 µg m−3 in 57% of samples, and all were higher than the final guideline of 5 µg m−3. The mean exposures (43.9 µg m−3 [SD 22.1, n = 29] in Dagoretti and 44.5 µg m−3 [SD 25.0, n = 46] in Starehe) and ambient concentrations (28.5 µg m−3 [SD 5.0] in Dagoretti and 35.3 µg m−3 [SD 4.9] in Starehe) measured here are in-line with those reported by the recent air pollutant studies in Nairobi. A study in Viwandani, an industrial neighborhood in Nairobi, reported daily PM2.5 exposures ranging from 22.4 to 39.6 µg m−3.39 Mean ambient PM2.5 measurements in Kibera and Viwandani in 2016–17 were reported at 23 and 21 µg m−3, respectively,17 and another measured mean daily roadside and background concentrations of 36.6 and 28.5 µg m−3, respectively.40
The results from these studies and those reported here suggest mothers and children in Nairobi's informal settlements are exposed to PM2.5 concentrations associated with substantial health risks.1–5 That these exposures are on the steepest sections of exposure–response curves for childhood ALRI and low birthweight (among others),10,39–42 also indicates potential for large health gains. For example, reducing mean exposures from ∼45 µg m−3 to the WHO Annual Interim 2 Target of 25 µg m−3, would be estimated to reduce childhood ALRI relative risk from ∼1.4 to less than 1.2,10,40 and increase birthweight by 100 grams,39,41,42 suggesting large potential health benefits could be achieved through interventions which provide even modest air quality improvements.
This study did not uncover any clear indication of hotspots where elevated exposures were associated with specific locations or point sources. The exposure study in Viwandani did report higher exposures for a single participant who traveled through an industrial area,37 but we did not observe any similar patterns. The only mildly suggestive indication of hotspots was noted in Starehe (see Fig. 3 and 4), which could point to locations of concern, but further investigation into potential sources and/or activities in these locations would likely be needed to provide clarity.
Aggregated personal exposures generally tracked with ambient exposures over the course of a day, with Fig. 2 showing the diurnal trend of exposures and ambient concentrations rising during morning and evenings. Exposures above 100 µg m−3 were the most common during these times, helping drive the study means higher than the WHO Interim 1 Target. While this study was not designed to apportion the sources of ambient pollution, other studies have reported vehicle emissions as a dominant source in Nairobi17,40,41and the diurnal PM2.5 patterns observed here are in line with those results.
While traffic and other ambient sources are certainly impacting PM2.5 exposures, mean personal exposures were 35% higher than ambient concentrations, suggesting other sources were adding to the ambient exposure burden. Fig. 2 shows that the largest increase over ambient occurred in the evenings when cooking and other activities are usually occurring at home, and Fig. 6 shows that the highest activity-associated exposures occurred during cooking with biomass. Those participants who used these solid fuels for cooking had a daily mean PM2.5 exposure of 59.7 µg m−3 (n = 16), compared to 40.3 µg m−3 for those who did not (n = 57) (see Fig. S5 in the ESI†). Given that recent estimates indicate 58% of urban Sub-Saharan population relies on biomass as their primary cooking fuel,42 this difference indicates potential for reducing exposure burdens for wood and charcoal users with programs and policies that support transition to clean fuels such as LPG, ethanol, and/or electricity. Promisingly, there are current programs in Nairobi expanding access to LPG and ethanol,43,44 which could be readily focused on mothers in informal settlements if given incentives and support to do so. Cooking with kerosene stoves also suggested slightly elevated exposures and kerosene is also not considered a clean fuel by the WHO,45 and thus should be a target for cleaner fuel interventions.
The other activity-related exposure of interest was walking versus other modes of transportation. Mean PM2.5 exposure during walking was 37 µg m−3, compared to when on a matatu or (58 µg m−3) or a bicycle (51 µg m−3). The sample sizes for matatu and bicycle use were small (n = 5 and 6, respectively), so drawing any strong conclusion is not possible. However, it may be worth further investigation into reasons why biking and riding in matatus had potentially higher exposures compared to walking as there could be mitigation by encouraging a change or modes and/or routes of travel.
4.2 Monitoring approach
A relatively novel approach was used to collect exposure samples on the mothers in this study. PurpleAir monitors, which have traditionally been used for ambient or area monitoring, were adapted and combined with GPS loggers to provide time- and location-resolved data. The backpacks and inserts provided a discrete and safe means to collect these exposure samples and were well-accepted by the participants, which was especially critical during the COVID-19 pandemic.
There were relatively few PurpleAir malfunctions (e.g. power cables being unplugged) and PM2.5 response was able to be adjusted using data from a co-located BAM sampling ambient air in Nairobi. At the same time, aerosol characteristics of the exposure samples certainly differed from and studies have shown that PurpleAir PM2.5 sensitivity changes depending on season and sources;46 and even within homes during different times or activities.47
Overall, the exposure monitoring, while having some limitations in terms of realtime PM2.5 response uncertainty, proved to be relatively inexpensive (each monitoring set was less than $400 total) and well-accepted approach by participants, providing a unique set of exposure data for mothers in informal settlements.
4.3 Limitations
There were several limitations to this study. First, while PM2.5 is generally considered the most critical air pollutant for monitoring and measurement because of its link with health impacts, there are several other air pollutants to which these communities are exposed. Carbon monoxide exposure, which is linked with pre-term babies and low birthweight,48,49 could be important for this study population. CO exposures could be especially high for charcoal users, as CO emissions from charcoal are generally high,50 including from stoves used in Nairobi.51 Other pollutants of interest include black carbon, NO2, SO2, poly-aromatic hydrocarbons (PAHs), and volatile organic carbons, which all have significant household, industrial, and transport sources.52
The methods used in this study helped to identify some of the sources (PM2.5 only) driving exposures for this population by cross-referencing location, activity, and real-time PM2.5 concentrations, but there are additional approaches that could help better resolve the specific sources. Collecting PM2.5 samples on filters would allow for source-apportionment analysis, which could help better assign exposures to specific sources such as biomass smoke, vehicle emissions, road dust, industrial sources, etc. High-resolution proximity measurements, as has been done with Bluetooth beacons,38,53 could also aid in determining sources of exposure. GPS signals can provide a general indication of where people are, but cannot, for example, indicate if a participant is in the same room as a wood stove or diesel generator.
Finally, this study was impacted by COVID-19 restrictions in terms of sample size and ability to collect more exposure-relevant data. For example, we did not measure house volumes or ventilation rates, which can impact exposures, as surveyors did not enter homes and minimized interaction times with participants. While the sample provided a rich set of data, discerning patterns and more sophisticated analysis of factors impacting exposures could potentially elucidate relationships and patterns that were not observed here.
It should also be noted that the sample selected may not have been representative of the KAM project population or other similar populations in informal settlements. While care was taken to stratify the sample across the project geography, use the same criteria as the KAM project (woman's age, and expecting or new mothers) and the participants were randomly selected from a larger pool pre-screened for willingness to enroll, there is a potential for selection bias (we did not collect information on those who declined to participate).
5 Conclusions
The relatively novel approach of adapting low-cost sensors and GPS to monitor mother's exposures in informal settlements yielded unique and potentially important information, highlighting potential sources of air pollutant exposure and corresponding pathways to mitigation. Specifically, the study supports the following conclusions and recommendations:
• The study's results suggest the most promising and practical intervention to reduce exposures in the target population would be to help households using wood and/or charcoal for cooking transition to clean fuels such as LPG, ethanol, or electricity. These households generally have access to electricity and there has been recent research showing the cost-effectiveness of cooking with electricity.54 There are also innovative fuel programs in Nairobi, aiming to overcome cost and convenience barriers with pay-as-you-go LPG smart meters and networks of ethanol dispensing kiosks.43,44 Importantly, these interventions, even if they only provide relatively modest exposure improvements, could result in substantial improvements in maternal and child health as the exposures are on steep sections of estimated exposure–response curves.11,48,55,56
• There is some evidence of other potentially feasible short-term measures to reduce exposures. The study found a few potential exposure hotspots in Starehe. Direct observation and investigation into these locations may provide insight into PM2.5 sources that could be mitigated and/or avoided.
• Over the long-term, lowering exposures to the most protective air quality guidelines will require addressing ambient pollution, as ambient concentrations were likely the greatest contributor to exposures. Interventions to reduce ambient exposures generally require government and/or community-wide efforts. Household air pollution is also a substantial source of ambient air pollution (estimated at 15% in Sub-Saharan Africa57), and thus efforts to transition to cleaner cooking are also fundamental to mitigating ambient air pollution. This study provides evidence that a vulnerable population – mothers and newborns in informal settlements – is being impacted by household and ambient air pollution, and thus can be used in advocacy efforts to relevant decision-makers and stakeholders.
• Further efforts to characterize mothers' and children's exposures and their sources could better inform what interventions would be most effective. Measuring multiple pollutants, source apportionment analysis, high-resolution source proximity measurements, and larger sample sizes would all be beneficial towards this end.
Author contributions
Michael A. Johnson: conceptualization, formal analysis, methodology, project administration, supervision, writing – original draft, writing – review & editing; Timothy Abuya: conceptualization, methodology, project administration, writing – original draft, writing – review & editing; Anneka Wickramanayake: writing – original draft, writing – review & editing; Heather Miller: data curation; formal analysis, writing – original draft, writing – review & editing; Deborah Sambu: data curation, investigation; Daniel Mwanga: data curation, investigation; George Odwe: data curation, investigation; Charity Ndwiga: data curation, investigation; Ricardo Piedrahita: data curation, formal analysis; Madeleine Rossanese: data curation, methodology; Michael J. Gatari: data curation, investigation, resources; Michael R. Giordano: resources, data curation, formal analysis; Daniel M. Westervelt: resources, writing – review & editing; Laura Wotton: conceptualization, methodology; Sathyanath Rajasekharan: conceptualization, methodology, funding acquisition, project administration.
Conflicts of interest
There are no conflicts of interest to declare.
Acknowledgements
Funding for this study was provided by the United States Agency for International Development (Cooperative Agreement no. 7200AA19CA00026). The author's views expressed in this publication do not necessarily reflect the views of the United States Agency for International Development or the United States Government. The authors extend our greatest thanks to the women, mothers, and caregivers in the community who so graciously volunteered information and agreed to participate in this study. We are grateful for the input and guidance provided by Darby Jack of the University of Columbia's Mailman School of Public Health. We also thank Monica Marquez who assisted with data processing, and the contributions of the field team toward the successful completion of this assessment. Ethical human subjects research approvals were provided by the Population Council's Institutional Review Board (PC IRB 928) and the AMREF Ethics and Scientific Review Committee (AMREF ESRC – P788/2020).
References
- R. Ghosh, K. Causey, K. Burkart, S. Wozniak, A. Cohen and M. Brauer, Ambient and household PM2.5 pollution and adverse perinatal outcomes: a meta-regression and analysis of attributable global burden for 204 countries and territories, PLoS Med., 2021, 18, e1003718 CrossRef CAS PubMed.
- P. Klepac, I. Locatelli, S. Korosec, N. Kunzli and A. Kukec, Ambient air pollution and pregnancy outcomes: a comprehensive review and identification of environmental public health challenges, Environ. Res., 2018, 167, 144–159 CrossRef CAS PubMed.
- X. Li, S. Huang, A. Jiao, X. Yang, J. Yun, Y. Wang, X. Xue, Y. Chu, F. Liu, Y. Liu, M. Ren, X. Chen, N. Li, Y. Lu, Z. Mao, L. Tian and H. Xiang, Association between ambient fine particulate matter and preterm birth or term low birth weight: an updated systematic review and meta-analysis, Environ. Pollut., 2017, 227, 596–605 CrossRef CAS PubMed.
- S. M. Melody, J. Ford, K. Wills, A. Venn and F. H. Johnston, Maternal exposure to short-to medium-term outdoor air pollution and obstetric and neonatal outcomes: a systematic review, Environ. Pollut., 2019, 244, 915–925 CrossRef CAS PubMed.
- S. Tsoli, G. B. Ploubidis and O.-I. Kalantzi, Particulate air pollution and birth weight: a systematic literature review, Atmos. Pollut. Res., 2019, 10, 1084–1122 CrossRef CAS.
- X. Zhou, K. Josey, L. Kamareddine, M. C. Caine, T. Liu, L. J. Mickley, M. Cooper and F. Dominici, Excess of COVID-19 cases and deaths due to fine particulate matter exposure during the 2020 wildfires in the United States, Sci. Adv., 2021, 7, eabi8789 CrossRef PubMed.
- O. Milicevic, I. Salom, A. Rodic, S. Markovic, M. Tumbas, D. Zigic, M. Djordjevic and M. Djordjevic, PM2.5 as a major predictor of COVID-19 basic reproduction number in the USA, Environ. Res., 2021, 201, 111526 CrossRef CAS PubMed.
- S. N. Kazemi, B. Hajikhani, H. Didar, S. S. Hosseini, S. Haddadi, F. Khalili, M. Mirsaeidi and M. J. Nasiri, COVID-19 and cause of pregnancy loss during the pandemic: a systematic review, PLoS One, 2021, 16, e0255994 CrossRef CAS PubMed.
- K. G. Sacinti, E. Kalafat, Y. E. Sukur and A. Koc, Increased incidence of first‐trimester miscarriage during the COVID‐19 pandemic, Ultrasound in Obstetrics & Gynecology, 2021, 57, 1013–1014 Search PubMed.
- K. R. Smith, J. P. McCracken, M. W. Weber, A. Hubbard, A. Jenny, L. M. Thompson, J. Balmes, A. Diaz, B. Arana and N. Bruce, Effect of reduction in household air pollution on childhood pneumonia in Guatemala (RESPIRE): a randomised controlled trial, Lancet, 2011, 378, 1717–1726 CrossRef PubMed.
- R. T. Burnett, C. A. Pope III, M. Ezzati, C. Olives, S. S. Lim, S. Mehta, H. H. Shin, G. Singh, B. Hubbell, M. Brauer, H. R. Anderson, K. R. Smith, J. R. Balmes, N. G. Bruce, H. Kan, F. Laden, A. Prüss-Ustün, M. C. Turner, S. M. Gapstur, W. R. Diver and A. Cohen, An Integrated Risk Function for Estimating the Global Burden of Disease Attributable to Ambient Fine Particulate Matter Exposure, Environ. Health Perspect., 2014, 122, 397–403 CrossRef PubMed.
- Impacts on Newborns | State of Global Air, https://www.stateofglobalair.org/health/newborns, accessed 8 February 2024.
- Institute for Health Metrics and Evaluation, GBD Compare | IHME Viz Hub, http://vizhub.healthdata.org/gbd-compare, accessed 30 August 2021.
- D. Beguy, P. Elung'ata, B. Mberu, C. Oduor, M. Wamukoya, B. Nganyi and A. Ezeh, Health & Demographic Surveillance System Profile: The Nairobi Urban Health and Demographic Surveillance System (NUHDSS), Int. J. Epidemiol., 2015, 44, 462–471 CrossRef PubMed.
- P. deSouza, Air pollution in Kenya: a review, Air Qual., Atmos. Health, 2020, 13, 1487–1495 CrossRef.
- C. Kiai, C. Kanali, J. Sang and M. Gatari, Spatial Extent and Distribution of Ambient Airborne Particulate Matter (PM2.5) in Selected Land Use Sites in Nairobi, Kenya, J. Environ. Public Health, 2021, 2021, e4258816 Search PubMed.
- P. deSouza, A Nairobi experiment in using low cost air quality monitors, Clean Air J., 2017, 27, 12–42 Search PubMed.
-
APHRC, Population and health dynamics in Nairobi's informal settlements: report of the Nairobi cross-sectional slums survey (NCSS) 2012, African Population and Health Research Center, Nairobi, 2014 Search PubMed.
- Y. Lu, G. Giuliano and R. Habre, Estimating hourly PM2.5 concentrations at the neighborhood scale using a low-cost air sensor network: a Los Angeles case study, Environ. Res., 2021, 195, 110653 CrossRef CAS PubMed.
- J. Bi, A. Wildani, H. H. Chang and Y. Liu, Incorporating Low-Cost Sensor Measurements into High-Resolution PM2.5 Modeling at a Large Spatial Scale, Environ. Sci. Technol., 2020, 54, 2152–2162 CrossRef CAS PubMed.
- A. Mousavi, Y. Yuan, S. Masri, G. Barta and J. Wu, Impact of 4th of July Fireworks on Spatiotemporal PM2.5 Concentrations in California Based on the PurpleAir Sensor Network: Implications for Policy and Environmental Justice, Int. J. Environ. Res. Public Health, 2021, 18, 5735 CrossRef PubMed.
- G. Raheja, L. Harper, A. Hoffman, Y. Gorby, L. Freese, B. O'Leary, N. Deron, S. Smith, T. Auch, M. Goodwin and D. M. Westervelt, Community-based participatory research for low-cost air pollution monitoring in the wake of unconventional oil and gas development in the Ohio River Valley: empowering impacted residents through community science, Environ. Res. Lett., 2022, 17, 065006 CrossRef.
- C. Malings, D. Westervelt, A. Hauryliuk, A. A. Presto, A. Grieshop, A. Bittner, M. Beekmann and R. Subramanian, Application of Low-Cost Fine Particulate Mass Monitors to Convert Satellite Aerosol Optical Depth Measurements to Surface Concentrations in North America and Africa, Atmospheric Measurement Techniques, 2020, 1–30 Search PubMed.
- G. Raheja, K. Sabi, H. Sonla, E. K. Gbedjangni, C. M. McFarlane, C. G. Hodoli and D. M. Westervelt, A Network of Field-Calibrated Low-Cost Sensor Measurements of PM2.5 in Lomé, Togo, Over One to Two Years, ACS Earth Space Chem., 2022, 6, 1011–1021 CrossRef CAS PubMed.
- C. McFarlane, G. Raheja, C. Malings, E. K. E. Appoh, A. F. Hughes and D. M. Westervelt, Application of Gaussian Mixture Regression for the Correction of Low Cost PM2.5 Monitoring Data in Accra, Ghana, ACS Earth Space Chem., 2021, 5, 2268–2279 CrossRef CAS.
- C. McFarlane, P. K. Isevulambire, R. S. Lumbuenamo, A. M. E. Ndinga, R. Dhammapala, X. Jin, V. F. McNeill, C. Malings, R. Subramanian and D. M. Westervelt, First Measurements of Ambient PM2.5 in Kinshasa, Democratic Republic of Congo and Brazzaville, Republic of Congo Using Field-Calibrated Low-Cost Sensors, Aerosol Air Qual. Res., 2021, 21, 200619 CrossRef.
- I. Stavroulas, G. Grivas, P. Michalopoulos, E. Liakakou, A. Bougiatioti, P. Kalkavouras, K. Fameli, N. Hatzianastassiou, N. Mihalopoulos and E. Gerasopoulos, Field Evaluation of Low-Cost PM Sensors (PurpleAir PA-II) under Variable Urban Air Quality Conditions, in Greece, Atmosphere, 2020, 11, 926 CrossRef CAS.
- K. K. Barkjohn, B. Gantt and A. L. Clements, Development and application of a United States-wide correction for PM2.5 data collected with the PurpleAir sensor, Atmospheric Measurement Techniques, 2021, 14, 4617–4637 CrossRef PubMed.
- C. Malings, R. Tanzer, A. Hauryliuk, S. P. N. Kumar, N. Zimmerman, L. B. Kara, A. A. Presto and R. Subramanian, Development of a general calibration model and long-term performance evaluation of low-cost sensors for air pollutant gas monitoring, Atmospheric Measurement Techniques, 2019, 12, 903–920 CrossRef.
- M. R. Giordano, C. Malings, S. N. Pandis, A. A. Presto, V. F. McNeill, D. M. Westervelt, M. Beekmann and R. Subramanian, From low-cost sensors to high-quality data: a summary of challenges and best practices for effectively calibrating low-cost particulate matter mass sensors, J. Aerosol Sci., 2021, 158, 105833 CrossRef CAS.
- J. Wu, C. Jiang, D. Houston, D. Baker and R. Delfino, Automated time activity classification based on global positioning system (GPS) tracking data, Environ. Health, 2011, 10, 101 CrossRef CAS PubMed.
-
World Health Organization, WHO global air quality guidelines: particulate matter (PM2.5 and PM10), ozone, nitrogen dioxide, sulfur dioxide and carbon monoxide, World Health Organization, Geneva, 2021 Search PubMed.
- M. Johnson, A. Pillarisetti, R. Piedrahita, K. Balakrishnan, J. L. Peel, K. Steenland, L. J. Underhill, G. Rosa, M. A. Kirby, A. Díaz-Artiga, J. McCracken, M. L. Clark, L. Waller, H. H. Chang, J. Wang, E. Dusabimana, F. Ndagijimana, S. Sambandam, K. Mukhopadhyay, K. A. Kearns, D. Campbell, J. Kremer, J. P. Rosenthal, W. Checkley, T. Clasen and L. Naeher, Exposure Contrasts of Pregnant Women during the Household Air Pollution Intervention Network Randomized Controlled Trial, Environ. Health Perspect., 2022, 130, 097005 CrossRef CAS PubMed.
- M. Shupler, P. Hystad, A. Birch, D. Miller-Lionberg, M. Jeronimo, R. E. Arku, Y. L. Chu, M. Mushtaha, L. Heenan, S. Rangarajan, P. Seron, F. Lanas, F. Cazor, P. Lopez-Jaramillo, P. A. Camacho, M. Perez, K. Yeates, N. West, T. Ncube, B. Ncube, J. Chifamba, R. Yusuf, A. Khan, B. Hu, X. Liu, L. Wei, L. A. Tse, D. Mohan, P. Kumar, R. Gupta, I. Mohan, K. G. Jayachitra, P. K. Mony, K. Rammohan, S. Nair, P. V. M. Lakshmi, V. Sagar, R. Khawaja, R. Iqbal, K. Kazmi, S. Yusuf and M. Brauer, Household and personal air pollution exposure measurements from 120 communities in eight countries: results from the PURE-AIR study, The Lancet Planetary Health, 2020, 4, e451–e462 CrossRef PubMed.
- S. N. Chillrud, K. A. Ae-Ngibise, C. F. Gould, S. Owusu-Agyei, M. Mujtaba, G. Manu, K. Burkart, P. L. Kinney, A. Quinn, D. W. Jack and K. P. Asante, The effect of clean cooking interventions on mother and child personal exposure to air pollution: results from the Ghana Randomized Air Pollution and Health Study (GRAPHS), J. Exposure Sci. Environ. Epidemiol., 2021, 1–16 Search PubMed.
- M. Johnson and R. A. Chiang, Quantitative Guidance for Stove Usage and Performance to Achieve Health and Environmental Targets, Environ. Health Perspect., 2015, 123, 820–826 CrossRef CAS PubMed.
- National Environment Management Authority of Kenya, 2014.
- M. Johnson, R. Piedrahita, A. Pillarisetti, M. Shupler, D. Menya, M. Rossanese, S. Delapeña, N. Penumetcha, R. Chartier, E. Puzzolo and D. Pope, Modeling approaches and performance for estimating personal exposure to household air pollution: a case study in Kenya, Indoor Air, 2021, 31, 1441–1457 CrossRef CAS PubMed.
- S. E. West, P. Büker, M. Ashmore, G. Njoroge, N. Welden, C. Muhoza, P. Osano, J. Makau, P. Njoroge and W. Apondo, Particulate matter pollution in an informal settlement in Nairobi: using citizen science to make the invisible visible, Applied Geography, 2020, 114, 102133 CrossRef.
- F. D. Pope, M. Gatari, D. Ng'ang'a, A. Poynter and R. Blake, Airborne particulate matter monitoring in Kenya using calibrated low-cost sensors, Atmos. Chem. Phys., 2018, 18, 15403–15418 CrossRef CAS.
- S. M. Mukaria, R. G. Wahome, M. Gatari, T. Thenya and K. Karatu, Particulate Matter from Motor Vehicles in Nairobi Road Junctions Kenya, Journal of Atmospheric Pollution, 2017, 5, 62–68 Search PubMed.
- O. Stoner, J. Lewis, I. L. Martínez, S. Gumy, T. Economou and H. Adair-Rohani, Household cooking fuel estimates at global and country level for 1990 to 2030, Nat. Commun., 2021, 12, 5793 CrossRef CAS PubMed.
- M. Shupler, M. O'Keefe, E. Puzzolo, E. Nix, R. Anderson de Cuevas, J. Mwitari, A. Gohole, E. Sang, I. Čukić, D. Menya and D. Pope, Pay-as-you-go liquefied petroleum gas supports sustainable clean cooking in Kenyan informal urban settlement during COVID-19 lockdown, Appl. Energy, 2021, 292, 116769 CrossRef CAS PubMed.
-
D. Whitehouse, The Africa Report, 2022 Search PubMed.
-
WHO, WHO Guidelines for Indoor Air Quality: Household Fuel Combustion, World Health Organization, Geneva, 2014 Search PubMed.
- E. Molina Rueda, E. Carter, C. L’Orange, C. Quinn and J. Volckens, Size-Resolved Field Performance of Low-Cost Sensors for Particulate Matter Air Pollution, Environ. Sci. Technol. Lett., 2023, 10(3), 247–253 CrossRef CAS PubMed.
- S. Sankhyan, J. K. Witteman, S. Coyan, S. Patel and M. E. Vance, Assessment of PM2.5 concentrations, transport, and mitigation in indoor environments using low-cost air quality monitors and a portable air cleaner, Environ. Sci.: Atmos., 2022, 2, 647–658 CAS.
- B. J. Wylie, Y. Kishashu, E. Matechi, Z. Zhou, B. Coull, A. I. Abioye, K. L. Dionisio, F. Mugusi, Z. Premji, W. Fawzi, R. Hauser and M. Ezzati, Maternal exposure to carbon monoxide and fine particulate matter during pregnancy in an urban Tanzanian cohort, Indoor Air, 2017, 27, 136–146 CrossRef CAS PubMed.
- A. K. Quinn, I. A. Adjei, K. A. Ae-Ngibise, O. Agyei, E. A. Boamah-Kaali, K. Burkart, D. Carrión, S. N. Chillrud, C. F. Gould, S. Gyaase, D. W. Jack, S. Kaali, P. L. Kinney, A. G. Lee, M. N. Mujtaba, F. B. Oppong, S. Owusu-Agyei, A. Yawson, B. J. Wylie and K. P. Asante, Prenatal household air pollutant exposure is associated with reduced size and gestational age at birth among a cohort of Ghanaian infants, Environ. Int., 2021, 155, 106659 CrossRef CAS PubMed.
- J. Jetter, Y. Zhao, K. R. Smith, B. Khan, T. Yelverton, P. DeCarlo and M. D. Hays, Pollutant Emissions and Energy Efficiency under Controlled Conditions for Household Biomass Cookstoves and Implications for Metrics Useful in Setting International Test Standards, Environ. Sci. Technol., 2012, 46, 10827–10834 CrossRef CAS PubMed.
- M. A. Johnson, C. R. Garland, K. Jagoe, R. Edwards, J. Ndemere, C. Weyant, A. Patel, J. Kithinji, E. Wasirwa, T. Nguyen, D. D. Khoi, E. Kay, P. Scott, R. Nguyen, M. Yagnaraman, J. Mitchell, E. Derby, R. A. Chiang and D. Pennise, In-Home Emissions Performance of Cookstoves in Asia and Africa, Atmosphere, 2019, 10, 290 CrossRef CAS.
- J. Lelieveld, J. S. Evans, M. Fnais, D. Giannadaki and A. Pozzer, The contribution of outdoor air pollution sources to premature mortality on a global scale, Nature, 2015, 525, 367–371 CrossRef CAS PubMed.
- J. Liao, J. P. McCracken, R. Piedrahita, L. Thompson, E. Mollinedo, E. Canuz, O. De Léon, A. Díaz-Artiga, M. Johnson, M. Clark, A. Pillarisetti, K. Kearns, L. Naeher, K. Steenland, W. Checkley, J. Peel, T. F. Clasen and HAPIN Investigators, The use of bluetooth low energy Beacon systems to estimate indirect personal exposure to household air pollution, J. Exposure Sci. Environ. Epidemiol., 2019, 1–11 CrossRef PubMed.
-
N. Rousseau, M. Leach, N. Scott, M. Bricknell, J. Leary, N. Abagi, V. Kumar, E. Brown and S. Batchelor, Overcoming the “Affordability Challenge” associated with the transition to electric cooking, UKAID, London, 2021 Search PubMed.
- K. Steenland, A. Pillarisetti, M. Kirby, J. Peel, M. Clark, W. Checkley, H. H. Chang and T. Clasen, Modeling the potential health benefits of lower household air pollution after a hypothetical liquified petroleum gas (LPG) cookstove intervention, Environ. Int., 2018, 111, 71–79 CrossRef CAS PubMed.
- J. Wieslaw, B. Ivona, F. Elzbieta, P. Agnieszka, J. Ryszard, K. Irena, J. D. Spengler, C. David and F. P. Perera, Estimated Risk for Altered Fetal Growth Resulting from Exposure to Fine Particles during Pregnancy: An Epidemiologic Prospective Cohort Study in Poland, Environ. Health Perspect., 2004, 112, 1398–1402 CrossRef PubMed.
- S. Chowdhury, A. Pillarisetti, A. Oberholzer, J. Jetter, J. Mitchell, E. Cappuccilli, B. Aamaas, K. Aunan, A. Pozzer and D. Alexander, A global review of the state of the evidence of household air pollution’s contribution to ambient fine particulate matter and their related health impacts, Environ. Int., 2023, 173, 107835 CrossRef CAS PubMed.
|
This journal is © The Royal Society of Chemistry 2024 |
Click here to see how this site uses Cookies. View our privacy policy here.