Combining predictive and analytical methods to elucidate pharmaceutical biotransformation in activated sludge†
Received
19th April 2023
, Accepted 16th July 2023
First published on 4th August 2023
Abstract
While man-made chemicals in the environment are ubiquitous and a potential threat to human health and ecosystem integrity, the environmental fate of chemical contaminants such as pharmaceuticals is often poorly understood. Biodegradation processes driven by microbial communities convert chemicals into transformation products (TPs) that may themselves have adverse ecological effects. The detection of TPs formed during biodegradation has been continuously improved thanks to the development of TP prediction algorithms and analytical workflows. Here, we contribute to this advance by (i) reviewing past applications of TP identification workflows, (ii) applying an updated workflow for TP prediction to 42 pharmaceuticals in biodegradation experiments with activated sludge, and (iii) benchmarking 5 different pathway prediction models, comprising 4 prediction models trained on different datasets provided by enviPath, and the state-of-the-art EAWAG pathway prediction system. Using the updated workflow, we could tentatively identify 79 transformation products for 31 pharmaceutical compounds. Compared to previous works, we have further automatized several steps that were previously performed by hand. By benchmarking the enviPath prediction system on experimental data, we demonstrate the usefulness of the pathway prediction tool to generate suspect lists for screening, and we propose new avenues to improve their accuracy. Moreover, we provide a well-documented workflow that can be (i) readily applied to detect transformation products in activated sludge and (ii) potentially extended to other environmental studies.
Environmental significance
Transformation products (TPs) of micropollutants in the environment are, like their parent compounds, a potential threat to human and ecosystem health, but their environmental impact is generally not well understood. Identification and characterization of TPs are crucial to understand their fate in the environment, in particular for pharmaceuticals for which no TP study is required for market approval. Here, we propose an updated workflow for TP identification using computational prediction of suspect TPs followed by HRMS screening in activated sludge experiments. By applying our workflow to 31 pharmaceuticals, we tentatively identified 79 TPs. We compare our results to previously published workflows to highlight recent advances in analytical and computational method development and to provide guidance for future TP identification efforts.
|
Introduction
The fate of an anthropogenic chemical in the environment is to a large extent determined by its intrinsic capability to be biotransformed by microorganisms. Biodegradation leads to the transient or permanent presence of transformation products (TPs), which can, like their parent compounds, be characterized by their behavior in the environment in terms of persistence, mobility, toxicity, and their ability to bioaccumulate. In certain cases, TPs have been found to be more persistent, mobile and/or toxic than their parent compound,1–3 which further highlights the importance of considering TPs in the environmental risk assessment of chemicals. Biodegradation studies identifying half-lives and biotransformation products are mandatory for certain classes of chemicals, i.e., pesticides.4,5 For pharmaceuticals, in contrast, only the characterization of human metabolites is required by regulation in the European Union,6 leading to a knowledge gap regarding the fate of active pharmaceutical ingredients (APIs) in the environment. As most APIs reach wastewater treatment plants (WWTP), understanding their fate in activated sludge is primordial. However, the identification of TPs is challenging because (i) the TP structures are not known in advance, and (ii) often no analytical standards are available to confirm the exact structure.
Helbling et al. (2010) first addressed systematically the issue of TP identification.7 To detect previously unknown degradation products of micropollutants in activated sludge, the authors presented a workflow combining computational and analytical approaches: (i) automatic generation of a suspect list of potential TPs for each compound, (ii) spiking activated sludge reactors with parent compounds, and (iii) screening the sludge samples for suspected TPs using liquid chromatography coupled to high-resolution tandem mass spectrometry (LC-HR-MSMS). In the first step (i), expert-curated biochemical transformation rules were iteratively applied to a chemical structure of interest to predict biodegradation pathways involving potential TPs. Typical pathway prediction tools are PathPred,8 BNICE,9 RetroPathRL10 or the University of Minnesota Pathway Prediction System (UM-PPS).11 The UM-PPS, which was used in Helbling et al.,7 is specifically designed for biodegradation studies and can prioritize likely over less likely biotransformations using prioritization rules (also called relative reasoning rules) to yield biochemically plausible biotransformation pathways and corresponding TPs.12 In the third step (iii), the generated suspect list was then used to extract single ion chromatograms for matching masses, which were further analyzed for peak formation over time, isotopic fit and shared fragments between parent compound and TPs.13 Still today, the main challenges of this approach are the high number of false positives in the suspect list leading to a low prediction precision, i.e., a low number of correctly predicted TPs per total number of predicted TPs, and the need for individual inspection of the extracted ion chromatogram (XIC), and MS and MS/MS spectra for each candidate. Without reference standards, considerable efforts are still needed for the resolution of TPs' isomeric structures and TP quantification, such as the development of advanced identification workflows and even the development of novel approaches (e.g., machine learning models) to predict ionization efficiencies that can improve the detection of more candidate TPs and the estimation of their concentration.14
In the past years, this workflow was applied and modified by different research groups to identify TPs in samples from biotransformation experiments. In particular, the prediction methods and underlying biodegradation databases have evolved to yield more accurate TP predictions: in 2012, the University of Minnesota Biodegradation/Biocatalysis Database and Pathway Prediction System (UM-BBD/PPS)15 was moved to Eawag and renamed to EAWAG-BBD/PPS, while keeping its original pathway prediction tool (PPS) and biodegradation data obtained from pure or enrichment cultures (BBD). In 2015, Wicker et al. re-implemented the EAWAG-BBD/PPS platform as enviPath, and the original BBD database was transferred to the new platform as EAWAG-BBD data package.16 In 2017, Latino et al. collected soil biodegradation data for 317 pesticides from regulatory reports and compiled them into the EAWAG-SOIL data package.17 The latest data addition to enviPath is EAWAG-SLUDGE, which contains biodegradation data for 91 micropollutants in activated sludge collected from scientific literature (https://envipath.org/package/7932e576-03c7-4106-819d-fe80dc605b8a). Compared to its predecessors, enviPath not only holds more data, but also provides an improved pathway prediction system where the expert-curated reaction prioritization rules were replaced with a machine-learning algorithm that learns the relative reasoning rules directly from the data.18,19
On the analytical side, new solutions emerged that facilitate the identification of TPs, in particular to decrease the workload of manually investigating mass matches for long suspect lists: different automated tools (Sieve, Compound Discoverer™ by Thermo Fisher Scientific™, among others) now address this issue by peak prioritization based on intensity, isotopic pattern, mass defect, time course of peak formation and predicted retention time (RT) by quantitative structure retention relationships (QSRR).20,21 Furthermore, the interpretation of MS spectra is facilitated by spectral library search (e.g., MassBank,22 NIST,23 mzCloud24) and in silico fragmentation tools (e.g., Mass Frontier, SIRIUS,25 CFM,26 MetFrag27).
These recent developments require a systematic analysis of previous studies to form an accurate picture of the current state-of-the-art in TP identification in biodegradation experiments, and to benchmark the performance of newly available tools against the original methods. To address this need, we (i) provide an overview of previous publications on TP identification in activated sludge or wastewater, (ii) present an updated, partially automated workflow for TP identification (Fig. 1), (iii) apply it to elucidate biotransformation processes of 42 pharmaceuticals, for many of which no TPs have been reported before, in a batch experiment with activated sludge, and (iv) evaluate the accuracy of five different TP prediction algorithms to guide future applications.
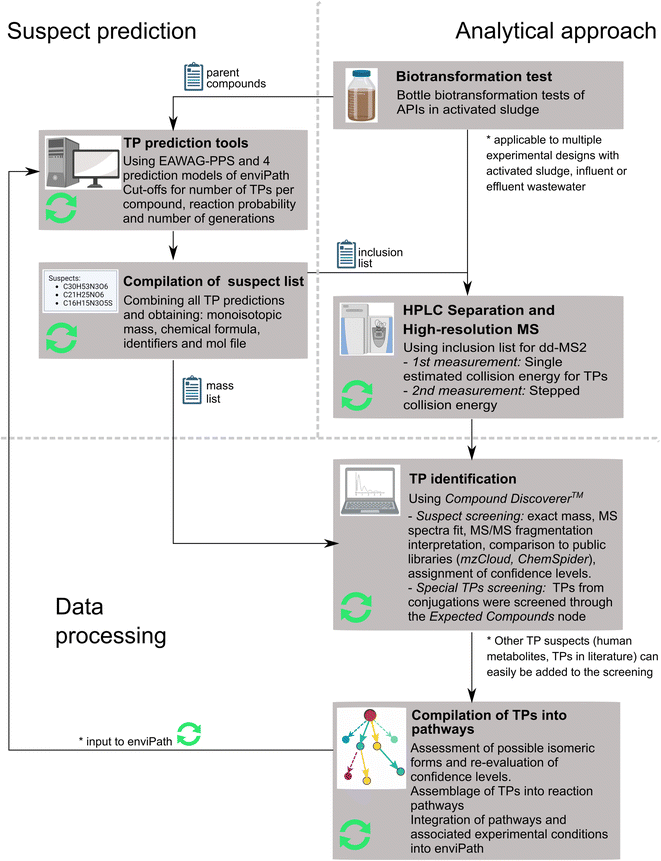 |
| Fig. 1 Workflow for TP identification in a biotransformation experiment starting from a suspect list (icons from BioRender). Main steps of the workflow are in bold text followed by the specific procedure applied in this study. Green circular arrows indicate updates from the workflow of Helbling et al. | |
Methods
Literature search
The objective of the literature search was to collect all publications on TP identification experiments in activated sludge or samples from wastewater treatment plants (WWTP) that used pathway prediction to generate suspect lists. The search terms “biotransformation”, “sludge” or “waste water”, “pathway prediction system” or “in silico metabolism prediction” or the name of a prediction system (e.g., “Pathpred”) or “suspect screening” were used in Reaxys (https://www.reaxys.com, last accessed 29/08/2022) and Clarivate Web of Science (https://www.webofscience.com, last accessed 01/09/2022). Furthermore, a Scopus (https://www.scopus.com, last accessed 02/09/2022) search for citation of the articles by Helbling et al. (2010)7 or Wicker et al. (2015)16 was performed. For each relevant article presenting results on TP identification, we extracted (i) the number of predicted and identified TPs, (ii) the substance class, (iii) the initially spiked concentration of test chemicals (if applicable), (iv) the pathway prediction method, (v) the experimental setup, (vi) the analytical method, and (vii) whether the TP identification was solely based on a suspect list (suspect screening) or whether additional TPs were identified by comparing full-scan MS data from different time points to detect emerging metabolites (non-target screening). Reviews were analyzed separately to identify general trends in analytical and computational methodologies.
TP identification workflow
The overall workflow for suspect TP identification included six steps (Fig. 1): (i) predicting TPs using pathway prediction tools, (ii) compiling a suspect list and annotating structures with MS-relevant information, (iii) performing biotransformation experiments, (iv) analyzing samples using liquid chromatography coupled to high-resolution tandem mass spectrometry, (v) identifying TPs from HR-MS data (including suspect screening and assignment of confidence levels), (vi) compiling identified TPs into pathways. Each step is described in detail in the next subsections. Compared to the original workflow proposed by Helbling et al.,7 the following steps were updated (Fig. 1, see green circular arrows): (i) suspect and mass list generation, (ii) second LC-MS measurement with stepped collision energy, (iii) spectral library search within Compound Discoverer, (iv) assignment of confidence levels according to Schymanski et al.,28 (v) prediction of conjugation reactions using Compound Discoverer, (vi) feedback of curated TP pathways into enviPath.
TP prediction tools
Suspect lists were obtained from EAWAG-PPS and enviPath. For enviPath, 4 pathway prediction models were trained on different combinations of the publicly available enviPath data packages EAWAG-BBD, EAWAG-SOIL, and EAWAG-SLUDGE to study the effect of adding different training data sets on the prediction performance. The following machine-learning models were trained using the respective data packages from EAWAG for provide different purposes: (i) ML-ECC-BBD was trained on pathway data in EAWAG-BBD and considered the standard, reference model. (ii) ML-ECC-BBD + SOIL was trained on pathway data from both EAWAG-BBD and EAWAG-SOIL to study the effect of biasing the model towards biodegradation in soil. (iii) ML-ECC-BBD + SLUDGE was trained on EAWAG-BBD and EAWAG-SLUDGE to study the effect of biasing the model towards biodegradation in activated sludge. (iv) ML-ECC-BBD + SOIL + SLUDGE was trained on all three data packages to see if including a maximum number of training pathways increases model performance. Table 1 shows the size and composition of the training sets used for the different models. All models used MACCS fingerprints as molecular descriptors and were trained using multi-label Ensemble Classifier Chains (ECC). Further details on the training of relative reasoning models can be found elsewhere.16
Table 1 Training sets used to build relative reasoning models for pathway prediction in enviPath
Model |
Number of reactions (pathways) in training data |
EAWAG-BBD |
EAWAG-SOIL |
EAWAG-SLUDGE |
Total |
ML-ECC-BBD |
1480 (220) |
— |
— |
1480 (220) |
ML-ECC-BBD + SOIL |
1480 (220) |
2447 (317) |
— |
3927 (537) |
ML-ECC-BBD + SLUDGE |
1480 (220) |
— |
355 (91) |
1835 (311) |
ML-ECC-BBD + SOIL + SLUDGE |
1480 (220) |
2447 (317) |
355 (91) |
4282 (628) |
For the TP prediction, EAWAG-PPS was run in batch mode using relative reasoning for three iterations with a neutral aerobic likelihood cut-off. The enviPath TP prediction was also run in batch mode (for details, see Methods section on “Data availability”), with a cut-off at 50 TPs per parent compound. The search algorithm employed a greedy pathway search in a weighted network, where the nodes are compounds and the edges are biotransformation reactions weighted with the predicted probability of the reaction to happen, given available data and competing reactions. The reaction probability pedge is obtained from the ML-based relative reasoning algorithm. For a child node n generated during the pathway search, the probability pnode,n is calculated as the product of pedge,n−1→n of the reaction producing the TPs with the probability of its direct parent node (pnode,n−1). During the search, the nodes are expanded in order of decreasing combined probability until the maximum of 50 TPs is reached, or no more TPs with a combined probability greater than zero are available for further expansion. The node and reaction probabilities are reported for each predicted TP, indicating their probability to be observed experimentally given the underlying relative reasoning model. The pathway search algorithms used by EAWAG-PPS and enviPath are illustrated in Fig. S1 (ESI-I).†
Compilation of suspect list
Python (version 3.6.13) scripting was used to combine the TPs predicted by the five different models into one suspect list, and to determine their monoisotopic mass, chemical formula, InChIKey, CAS number and structure as mol file using the Python libraries RDKit (version 2020.09) and PubChemPy (version 1.0.4). Some TPs were predicted for several parent compounds, in which case they were merged in the suspect list used for screening but counted separately in the method evaluation and comparison. From the suspect list, we extracted the charged masses for HRMS measurements (inclusion list), and the formulae and Molfiles for TP identification in Compound Discoverer (mass list).
Experimental setup of sludge reactors
The experimental setup of the sludge reactors was adapted from Gulde et al.29 In short, sludge-seeded and aerated bottle reactors were spiked with the mixture of 46 selected compounds at an initial concentration of 8 μg L−1 (details in ESI-I Table S3†). The APIs were selected based on commercial availability, expected measurability using HPLC-HRMS/MS, and predictability of the corresponding TPs. The selected substances show a wide range of structural moieties and diversity in their functional groups. Only irbesartan,30 valsartan,7,31–34 metformin35 and hydrochlorothiazide34 were previously investigated in biotransformation experiments in activated sludge or wastewater samples. Further, olanzapine, mirtazapine, rivastigmine, aliskiren, atazanavir, efavirenz and rosuvastatin were screened for in waste water samples.36–38 The environmental fate of the remaining 35 APIs has not been investigated to the best of our knowledge. Control experiments were used to reveal abiotic degradation, sorption processes, and matrix background (ESI-I Table S4†). The airflow of half of the reactors was augmented with CO2 to assess biotransformation at pH 6 in addition to the native pH of approximately 7.5. Additionally, reactors were run at two levels of total suspended solids (TSS): dilute (DB, TSS = 0.6 g L−1) and high biomass (HB, TSS = 7.1 g L−1). Samples were taken from biotransformation reactors at time points 0 (triplicate), 2 h, 4 h, 9 h, 24 h, 30 h (triplicate), 48 h, 54 h and 72 h and were centrifuged. The aqueous phase was transferred to an LC-MS amber vial and stored at −20 °C until analysis. Two calibration curves were used, one in nanopure water and one in sludge matrix, at concentrations of 0.05, 0.1, 0.2, 0.5, 1, 2, 5 and 8 μg L−1. A more detailed description of the experimental setup of the sludge reactors can be found in the ESI-I, Section S3.†
HPLC-HRMS/MS analysis
The samples of the biotransformation experiments were measured using an HPLC-HRMS/MS (QExactivePlus, Thermo Fisher Scientific, Waltham, MA, USA) approach. For the HPLC separation, a standard method adopted from Achermann et al. was used.34 More details can be found in the ESI-I (Section S4).† In a first measurement, mass spectra were acquired in full-scan in positive and negative ionization modes and then a data-dependent top 5 analysis (ddMS2-top5) was used. The [M + H]+ and [M − H]− masses of parent APIs and predicted TPs were included in the inclusion list. A single normalized collision energy (NCE) for each compound in the inclusion list was calculated by an empirical formula (eqn (1)).39 In a second measurement, samples from four time points (0 h, 2 h, 24 h and 72 h) were re-measured with a stepped NCE approach where fragments from 3 different collision energies (15, 35 and 60) were simultaneously collected, thus improving the chances of obtaining relevant MS2 spectra for structure elucidation of suspect TPs. For the second measurement, TPs arising from conjugation reactions predicted by Compound Discoverer™ during the analysis of the first measurement were added to the inclusion list. The HRMS settings are further detailed in the ESI-I (Section S4.2).† | Normalized collision energy [NCE] = mass[u] × (−0.41) + 160 | (1) |
TP identification
The Compound Discoverer™ software (Thermo Scientific™, Version 3.2) was used for TP suspect screening. The procedure included compound detection, comparison to suspect mass list, in silico prediction of fragments and (spectral) library search (mzCloud, ChemSpider), described in more detail in the ESI-I (Section S5†). The entries of plausible candidates were reviewed manually based on peak shape, isotopic pattern and chromatographic area evolution over time and comparison to controls. Confidence levels were reported according to Schymanski et al.:28 1 (confirmed structure by reference standard), 2a (probable structure by spectral library match), 2b (probable structure by diagnostic evidence), 3 (tentative candidate with reasonable MS2), 4 (unequivocal molecular formula found), 5 (exact mass found). Finally, molecular structures were drawn based on structural evidence.
Compound Discoverer™ was further used to identify TPs resulting from conjugation reactions. N-Acetylation and N-succinylation were shown to be highly relevant for primary and secondary amines,40 but their prediction is beyond the scope of biodegradation tools, which focus on the breakage (and not formation) of molecular bonds. Conjugation reactions (acetylation, formylation, fumarylation, malonylation and succinylation) were therefore predicted using the Expected Compounds nodes of Compound Discoverer. In addition, we also screened literature to find TPs reported in previous studies. While we did not include TPs arising from conjugation reactions and TPs reported in literature in the suspect list, we still searched for their presence in the LC-HRMS measurements. These TPs were analyzed separately to avoid interfering with our evaluation of TP prediction methods and are therefore discussed separately as manual suspects.
Comparison of prediction methods
To evaluate and compare the performance of the different TP prediction methods, we calculated how many TPs we would have found by applying each method separately. For each method, we determined the precision according to eqn (2). Next, we wanted to know if we could have obtained a better performance in terms of precision if we had stopped the prediction algorithm earlier. To answer this question, we generated smaller suspect lists by only keeping TPs that would have been obtained with a given cut-off threshold, and we evaluated the number of correctly predicted TPs and the precision of these reduced suspect list. By varying the cut-off threshold for the number of generations for all methods, we obtained the prediction performance for TPs generated in 1, 2 and 3 generations. We further varied the cut-off threshold for the maximum number of TPs to predict from 1 to 50. As EAWAG-PPS does not support setting a threshold for the maximum number of TPs, the analysis of TP ranks was performed for enviPath methods only. The analysis was implemented in Python (see Data availability section for details). |  | (2) |
Results and discussion
EAWAG-PPS is the most popular TP prediction tool
To assess the current state-of-the-art in suspect screening of TPs in wastewater or activated sludge systems, we performed a literature search for the timespan between 2010 and 2022, and we found 27 publications that used predicted TPs to screen samples (Table 2 and ESI-I Table S1†). The most widely used tools for generating suspect lists were UM-PPS and EAWAG-PPS, which were applied in 7 and 12 studies, respectively. PathPred8 (2 studies, both in combination with EAWAG-PPS) and MetabolitePredict41 (2 studies, one in combination with EAWAG-PPS) were also applied, even though these tools are not specific to biodegradation and represent general biochemistry and human metabolism. Each one of Metaprint2D,42 O3-PPS (specific to ozonation reactions)43 and Metabolitepilot (commercial software) were used in one study only. From this review, we conclude that the UM-PPS and its successor EAWAG-PPS are the most popular tools for TP prediction in activated sludge, as both tools combined were used in 89% of the studies considered.
Table 2 Articles on TP identification in activated sludge or wastewater using predicted suspect listsa
Year |
Authors |
Substance class |
Number of compounds tested |
Experimental setup |
Prediction tool |
Number of TPs found |
Reference |
API = active pharmaceutical ingredients, Pe = pesticides, MP = micropollutants, BR = batch reactor, WW = waste water sample.
|
2010 |
Helbling et al. |
API, Pe |
12 |
BR |
UM-PPS |
26 |
7
|
2010 |
Helbling et al. |
MP, Pe, API |
30 |
BR |
UM-PPS |
53 |
31
|
2010 |
Kern et al. |
API, Pe |
8 |
BR |
UM-PPS |
12 |
13
|
2011 |
Prasse et al. |
API |
2 |
BR |
UM-PPS |
9 |
44
|
2013 |
Müller et al. |
API |
1 |
BR |
UM-PPS |
2 |
54
|
2014 |
Huntscha et al. |
MP |
3 |
BR |
UM-PPS |
13 |
55
|
2015 |
Letzel et al. |
API |
5 |
BR and WW |
EAWAG-PPS |
6 |
33
|
2015 |
Kosjek et al. |
API |
1 |
BR |
UM-PPS |
9 |
56
|
2015 |
Gago-Ferrero et al. |
API |
173 |
WW |
MetabolitePredict |
47 |
36
|
2016 |
Gulde et al. |
API, Pe, MP |
19 |
BR |
EAWAG-PPS, metaprint2D |
144 |
40
|
2016 |
Beretsou et al. |
API |
1 |
BR |
EAWAG-PPS, MetabolitePredict |
14 |
45
|
2016 |
Letzel et al. |
API |
1 |
BR and WW |
EAWAG-PPS |
4 |
30
|
2018 |
Kosjek et al. |
API |
1 |
BR |
EAWAG-PPS |
11 |
57
|
2018 |
Achermann et al. |
MP |
93 |
BR |
EAWAG-PPS |
75 |
34
|
2019 |
Zumstein and Helbling |
API |
6 |
BR |
EAWAG-PPS |
16 |
58
|
2020 |
Gornik et al. |
API |
1 |
BR |
EAWAG-PPS |
10 |
59
|
2020 |
Trenholm et al. |
MP |
3 |
BR |
EAWAG-PPS |
9 |
60
|
2020 |
Wang et al. |
Pe, API |
60 |
WW |
EAWAG-PPS |
57 |
61
|
2020 |
Wu et al. |
API |
1 |
BR |
EAWAG-PPS, PathPred |
4 |
62
|
2021 |
Kinyua et al. |
MP |
2 |
BR |
EAWAG-PPS, MetabolitePredict |
10 |
75
|
2021 |
Cai et al. |
Pe |
2 |
BR |
EAWAG-PPS, PathPred |
10 |
63
|
2021 |
Choi et al. |
MP |
1 |
WW |
EAWAG-PPS |
29 |
64
|
2021 |
Martínez-Piernas et al. |
API |
20 |
WW |
EAWAG-PPS |
18 |
65
|
2021 |
Psoma et al. |
API |
4 |
BR |
EAWAG-PPS |
22 |
35
|
2021 |
Gulde et al. |
MP |
87 |
BR and WW |
O3-PPS |
83 |
38
|
2021 |
Zhang et al. |
Pe |
30 |
WW |
Metabolitepilot™ |
20 |
37
|
2022 |
Rich et al. |
MP |
40 |
BR |
EAWAG-PPS |
46 |
66
|
The most common analytical method is LC-HRMS (Q-TOF and Orbitrap technologies, 14 and 12 studies, respectively). Bottle incubations are the most common experimental setup (14 studies), followed by WWTP influent and effluent sampling (8 studies). Most authors combine suspect and non-target screening using LC-MS techniques. In some cases, the analytical method was extended by an NMR spectroscopy approach44 or by the use of HILIC in addition to reverse-phase columns to improve retention and separation of hydrophilic compounds and – in some cases – isomers.45 Most common substance classes are pharmaceuticals (18), pesticides (5) or just micropollutants (4) in general. Even though enviPath is publicly available since 2016, it has not been used so far to predict biodegradation pathways in wastewater samples, but it has been applied for TP prediction in soil and surface water samples.46,47 To evaluate the overall success of suspect screening across biodegradation studies, we compared their performance in terms of detected TPs per parent compounds. As some studies only looked at very few parent compounds and performed the TP screening in greater detail, we only looked at studies with more than 10 parent compounds for a fair comparison with the workflow presented here. The eight studies that fulfilled these criteria had an average ratio of found TPs per parent compound of 1.5, ranging between 0.3 and 5.3. Finally, it should be noted that our search may have missed relevant articles that did not contain our search terms in the title or abstract.
The search also revealed five relevant articles that review available tools for pathway prediction from three different angles: (i) metabolite prediction methods for drug metabolism,48,49 (ii) pathway prediction methods in the context of pathway design for metabolic engineering,50 and (iii) TP prediction for environmental contaminants.51–53 Comprehensive overviews of existing tools for field-specific applications are hence available from the indicated reviews and are therefore not further discussed here. Interestingly, some of the tools such as PathPred and EAWAG-PPS/enviPath were mentioned across scientific fields, while others were exclusively applied in their field of origin. Also, Sveshnikova et al. point out that only few predictive biochemistry frameworks are being actively maintained and continuously applied in experimental work,50 which is crucial to ensure reproducibility and continued evaluation of the prediction method. Out of the prediction tools applied to TP prediction in activated sludge, only UM-PPS/EAWAG-PPS/enviPath, PathPred and MetabolitePredict are actively maintained. Out of these, only UM-PPS/EAWAG-PPS/enviPath are specific to microbial biodegradation prediction. As these tools are also the most widely applied methods for TP prediction in the context of environmental chemistry, they are the focus of our study.
Thousands of potential TPs predicted by EAWAG-PPS and enviPath
Based on the results from the literature search, we focused on EAWAG-PPS and its successor platform enviPath to generate suspect lists and to evaluate their respective performances in correctly predicting TPs. We chose EAWAG-PPS as a benchmark and compared it to the four enviPath models trained on different data packages. The enviPath models were trained on four different combinations of the following data packages: EAWAG-BBD containing 220 pathways, EAWAG-SOIL containing 317 pathways, and EAWAG-SLUDGE containing 91 pathways. Models were trained on BBD only, BBD + SOIL, BBD + SLUDGE, and BBD + SOIL + SLUDGE packages (Table 1).
To obtain a suspect list, we applied the five pathway prediction models to the 46 pharmaceuticals. All the prediction systems combined generated a total of 5570 TPs, out of which 348 (6.25%) TPs were predicted by all methods. The EAWAG-PPS predicted an average of 47 TPs per compound, ranging from four to 441 TPs. For example, fingolimod only has two hydroxyl moieties acting as reactive sites, resulting in four predicted TPs. In contrast, naloxegol features a long polyethylene glycol chain that can be cleaved at alternative reactive sites according to reaction rules, leading to 441 predicted TPs. The four enviPath models were limited to a maximum of 50 TP per compound, which was reached for almost all compounds. One of the exceptions is metformin, for which the enviPath pathway expansion converged at three TPs, meaning that no more reactions occurred according to the available biotransformation and relative reasoning rules. However, metformin may be a special case, as this small molecule only has a few reactive sites and a particular structure that may not be well represented in the training data.
Biodegradation behavior observed for 34 compounds
A total of 42 out of the 46 spiked compounds were detected in the bottle reactors using the Compound Discoverer workflow. Acalabrutinib, ceritinib and orlistat were filtered out by the Compound Discoverer workflow due to low intensity of m/z ions and could only be found by manual exploration of the chromatograms and mass spectra in the raw files of sludge samples or in freshly spiked calibration samples. Ridaforolimus was detected only in pure aqueous standards at 1 mg L−1. This behaviour could be explained by low ionization efficiencies, instability of the API or rapid losses such as volatilization or sorption to glass and/or plastic materials. We therefore excluded these four APIs from further analysis.
Six other APIs, atovaquone, clotrimazol, efavirenz, mometasone, nilotinib and regorafenib were detected in the samples from the sludge reactors; however, in the biotransformation reactors no clear degradation trend was observed over the time course of the experiment, and in the sorption control reactors these APIs show a decrease in the area by at least one order of magnitude from time-point 0 h to 24 h (ESI-II, Sections S4.2, S4.3, S4.5, S4.7, S4.9 and S4.10†). All these six compounds have a (predicted) soil adsorption coefficient log
Koc between 3 and 5.5 (ESI-I Table S3†), which would be consistent with noticeable losses by sorption to sludge. Substantial sorption to soil organic material hinders microbial biotransformation, and hence the formation of TPs, due to low bioavailability.67 Mometasone and nilotinib were also dissipated abiotically in the high pH abiotic controls (ESI-II, Sections S4.7 and S4.9). Finally, atomoxetine, duloxetine, mirtazapine, rivastigmine and terbinafine, all APIs with amine moieties, show non-linear kinetics in the biotransformation reactors at high pH (ESI-II, Sections S3.4, S3.11, S3.19, S3.26 and S3.29†), which could indicate that some level of ion-trapping occurred in parallel to biotransformation.68 For the remaining 31 pharmaceuticals, we obtained clear trends of decreasing concentration over time (for details, see ESI-II†). However, we proceeded with TP identification for all APIs, independently of their biotransformation behavior.
Suspect screening identifies 67 TPs
A total of 79 TPs were tentatively identified, out of which 67 were found with the help of the suspect list and twelve additional TPs were tentatively identified using the list of manual suspects (see Methods section for details). TPs were found for 31 parent compounds. Confidence levels were assigned to the TPs according to Schymanski et al. during the screening process (Fig. 2).28 The structures of only seven TPs (9%) were confirmed with a reference standard (level 1) and one additional TPs (1.3%) showed a good match with the spectral library mzCloud (level 2a). Diagnostic evidence (level 2b) was found for the structures of eleven TPs (14%). Most TPs (56, 71%) were reported with tentative structures (level 3) and for four (5%) the MS2 spectra were not conclusive (level 4). Levels 3 and 4 include TPs for which several possible isomeric structures were considered possible. For example, Clp_TP_3 is the oxidation product of clopidogrel. Hydroxylation, N-oxidation, S-oxidation or oxidative N-dealkylation are plausible reaction mechanisms for the observed modification to the chemical formula, but not enough structural evidence was found to determine a specific structure and its corresponding reaction mechanism (Fig. 2). Three TPs (Val_TP_5, level 4; Val_TP_7, level 1; and Val_TP_12, level 3) were assigned to both valsartan and irbesartan, since they could originate from both parents and the experimental setup did not allow for distinguishing their origin. These three TPs were counted double in the results, as they could originate from both parent compounds. The confidence levels depend on the availability of reference standards and database spectra, as well as on the quality of reported and measured MS2 data. For 34 TPs, the best fragmentation was achieved using a stepped collision energy approach, where the analyte is exposed to three different collision energies for each data-dependent scan.
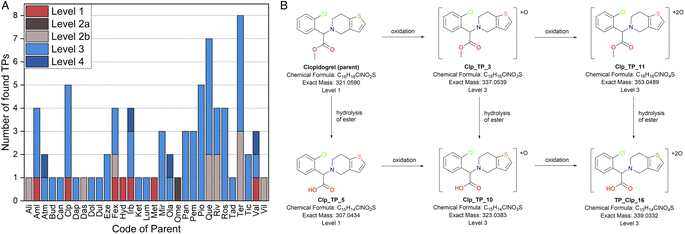 |
| Fig. 2 Confidence levels in TPs and their translation to pathways. (A) Number and confidence levels of found TPs for each parent API. Full names of the APIs can be found in Table 3. (B) Suggested biodegradation pathway for clopidogrel. Brackets around the compound structure indicate that the exact modification site is unknown. | |
In a next step, tentatively identified TPs were manually assembled into pathways with the help of the suspect lists, which contain information on the biotransformation that is responsible for the formation of each TP (ESI-II†). In the manually drawn pathways given in ESI-II,† ambiguous isomeric structures were reported as a general structure with possible modifications on specific moieties. All the resulting pathways and associated experimental parameters have also been made available on enviPath, where they were integrated into the EAWAG-SLUDGE package (https://envipath.org/package/7932e576-03c7-4106-819d-fe80dc605b8a). Because enviPath requires unambiguous structural information for compounds, ambiguous isomeric structures are represented by all possible alternative structures, which were merged into a single compound entry in the EAWAG-SLUDGE package. Finally, CAS numbers were found for 27 TPs (34%), out of which 21 TPs (27%) have been previously reported in the context of their parent compound. Of these, 13 (16%) TPs have been found in sludge or waste-water in previous studies (the 3 common TPs of valsartan and irbesartan are counted double). Therefore, 54 TPs associated with 24 APIs are reported here for the first time.
Our suspect screening resulted in a ratio of 1.5 tentatively identified TPs per parent compound, which is similar to the average ratio found in other studies with more than 10 parent compounds (1.5 found TPs per parent) (Table 3 and ESI-I Table S1†). It should be recognized that this similar ratio was obtained in this work despite performing no systematic non-target screening, and despite operating at low API and, consequently, TP concentrations. For example, the study with the highest ratio of found TPs per parent (5.3) involved non-target screening at a spike concentration of 120 μg L−1. Increasing the concentration could improve the chances of observing TPs, but it would not represent the real WWTP influent concentration of most APIs,69,70 and degradation kinetics vary at different initial spiked or unspiked concentrations of micropollutants.71 Thus, the conditions used here are likely more conducive to identify biotransformation pathways from activated sludge experiments that are relevant to full-scale WWTPs.
Table 3 Predicted and found TPs for each of the 42 parent compounds
Parent compound |
Abbreviation |
Total predicted TPs |
TPs found from suspect list |
TPs found from manual suspectsa |
Overall Precision |
TPs that were not predicted by any of the evaluated prediction methods but found in literature or using Compound Discoverer's conjugation reaction prediction are here called manual suspects.
TP count without duplicate TPs from irbesartan and valsartan.
|
Aliskiren fumarate |
Ali |
231 |
1 |
0 |
0.43% |
Amlodipine besylate |
Aml |
78 |
1 |
3 |
1.28% |
Atazanavir sulfate |
Ata |
144 |
0 |
0 |
0.00% |
Atomoxetine |
Atm |
100 |
1 |
1 |
1.00% |
Atovaquone |
Ato |
84 |
0 |
0 |
0.00% |
Budesonide |
Bud |
85 |
0 |
1 |
0.00% |
Canagliflozin hydrate |
Can |
124 |
1 |
0 |
0.81% |
Clopidogrel bisulfate |
Clp |
110 |
5 |
0 |
4.55% |
Clotrimazol |
Clo |
68 |
0 |
0 |
0.00% |
Dapagliflozin |
Dap |
144 |
1 |
0 |
0.69% |
Dasatinib |
Das |
156 |
1 |
0 |
0.64% |
Dienogest |
Die |
94 |
0 |
0 |
0.00% |
Dolutegravir sodium |
Dol |
147 |
1 |
0 |
0.68% |
Duloxetine |
Dul |
89 |
0 |
1 |
0.00% |
Efavirenz |
Efa |
64 |
0 |
0 |
0.00% |
Ezetimibe |
Eze |
85 |
2 |
0 |
2.35% |
Fexofenadine |
Fex |
111 |
4 |
0 |
3.60% |
Fingolimod hydrochloride |
Fin |
84 |
0 |
0 |
0.00% |
Hydrochlorothiazide |
Hyd |
118 |
0 |
1 |
0.00% |
Irbesartan |
Irb |
129 |
4 |
0 |
3.10% |
Keto-desogestrel |
Ket |
108 |
1 |
0 |
0.93% |
Lumiracoxib |
Lum |
94 |
1 |
0 |
1.06% |
Metformin hydrochloride |
Met |
3 |
0 |
1 |
0.00% |
Mirtazapine |
Mir |
149 |
3 |
0 |
2.01% |
Mometasone furoate |
Mom |
148 |
0 |
0 |
0.00% |
Naloxegol |
Nal |
472 |
0 |
0 |
0.00% |
Nilotinib |
Nil |
174 |
0 |
0 |
0.00% |
Olanzapine |
Ola |
137 |
2 |
0 |
1.46% |
Omeprazole |
Ome |
117 |
1 |
0 |
0.85% |
Panobinostat lactate |
Pan |
80 |
3 |
0 |
3.75% |
Pemetrexed |
Pem |
160 |
3 |
0 |
1.88% |
Pioglitazone hydrochloride |
Pio |
109 |
4 |
1 |
3.67% |
Quetiapine fumarate |
Que |
141 |
7 |
0 |
4.96% |
Regorafenib |
Reg |
74 |
0 |
0 |
0.00% |
Rivastigmine hydrochloride |
Riv |
87 |
3 |
1 |
3.45% |
Rosuvastatin calcium |
Ros |
130 |
4 |
0 |
3.08% |
Tadalafil |
Tad |
95 |
1 |
0 |
1.05% |
Terbinafine hydrochloride |
Ter |
138 |
6 |
2 |
4.35% |
Ticagrelor |
Tic |
136 |
2 |
0 |
1.47% |
Valsartan |
Val |
120 |
3 |
0 |
2.50% |
Vildagliptin |
Vil |
88 |
1 |
0 |
1.14% |
Vorinostat |
Vor |
59 |
0 |
0 |
0.00% |
Total |
|
5064 |
67 (64b) |
12 |
1.35% |
enviPath model trained on BBD + SOIL performed best
To evaluate the performance of the different pathway prediction models, we compared their total number of correctly predicted TPs and we found that enviPath models performed best, predicting around 50 identified TPs, while EAWAG-PPS only predicted 43 correctly (Fig. 3). Out of the four enviPath models, those including additional biodegradation data from soil and/or sludge performed slightly better, indicating that additional data can improve model performance. We then traced back which TPs were predicted by which method and found that 22 (32.8%) of all TPs were predicted by all prediction methods. Another twelve (17.9%) of TPs found were correctly predicted by all enviPath methods, which hints at their similarity in predicting TPs. In other words, suspect screening could identify roughly half of the TPs by using any of the enviPath methods. However, some of the TPs were exclusively predicted by one method. Most notably, the EAWAG-PPS exclusively predicted five (7.5%) identifiable TPs that were not covered by any enviPath method. Thus, combining multiple prediction methods leads to the most comprehensive suspect list.
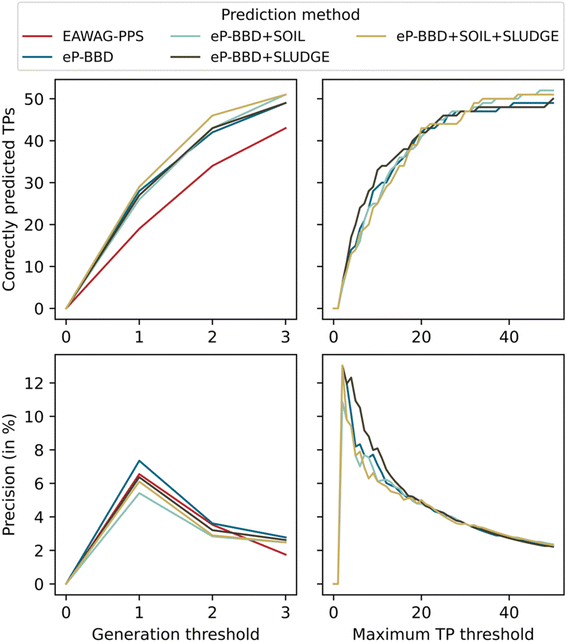 |
| Fig. 3 Influence of the models' prediction parameters on the precision and the number of correctly predicted TPs. Top: correctly predicted TPs, bottom: precision in percent. eP: enviPath. | |
However, a long suspect lists increases the manual workload, and it is therefore crucial to balance the number of detected TPs with the number of suspects to search for. The prediction precision indicates the number of found TPs per predicted TP and can be used as a metric to describe the efficiency of the prediction method. The overall precision of the TP prediction was found to be 1.35%, meaning that more than one in hundred predicted TPs was correctly predicted (Table 3). As the number of predicted TPs is comparable for all substances (except for metformin), the precision mainly reflects the number of correctly predicted TPs. The precision varied for different APIs: for some compounds, such as quetiapine, the precision was as high as 5%, indicating that this compound has many stable transformation products and its structural features were well represented in the training data of the pathway prediction models, therefore leading to a high number of correctly predicted TPs. All models performed similarly with a prediction precision between 2 and 2.6%, with enviPath models generally performing better than EAWAG-PPS (Table 4). The model trained on the BBD and SOIL packages had the best overall performance regarding the number of TPs found (53) and, consequently, also precision (2.58%).
Table 4 Performance comparison of prediction methods
Prediction method |
Found TPs |
Predicted TPs |
Prediction precision |
EAWAG-PPS |
42 |
2080 |
2.02% |
enviPath-BBD |
49 |
2052 |
2.39% |
enviPath-BBD + SOIL |
53 |
2051 |
2.58% |
enviPath-BBD + SLUDGE |
51 |
2052 |
2.49% |
enviPath-BBD + SOIL + SLUDGE |
50 |
2051 |
2.44% |
It should be noted that these low values for precision represent a worst-case scenario, as the suspect list can be further filtered to increase the precision. For example, removing compounds with a mass below the quantification limit of the analytical method (100 g mol−1) slightly increases the prediction precision of the suspect list from 1.35 to 1.37%. If a small suspect list is required, the precision can be further increased by adapting the parameters of the pathway search: In EAWAG-BBD, the generation threshold can be set to 1, 2 or 3, and in enviPath the maximum number of TPs to predict can be defined. However, limiting the number of generations or TPs to predict comes at the cost of losing correctly predicted TPs. To characterize this trade-off, we analyzed the effect of different thresholds for these two parameters on the precision and the number of correctly predicted TPs. For the number of generations, the threshold analysis showed that the precision peaks at the first generation for all methods (5.4–7.3%), where EAWAG-PPS correctly predicts 19 TPs and the enviPath models between 26 and 29 TPs (Fig. 3). Regarding the threshold of the maximum numbers of TPs to predict, the precision peaks between 10.9 and 13.0% if only the top 2 TPs are predicted. The number of correctly predicted TPs reaches a plateau at a threshold of 30 predicted TPs, beyond which the workload increases but not many more TPs are identified. This characterization of the trade-off between precision and correctly predicted TPs can be used as a guide to select the parameters that are best suited to the objective and the resources of a suspect screening project. To give a practical example, the workload of manual TP confirmation can be cut in half by setting the maximum TP threshold to 25, while still obtaining 86.3–92% of correctly predicted TPs at the maximal threshold explored here (50).
Observed TPs can be explained by 24 biotransformation rules
A total of 114 different biotransformation rules were applied to predict potential TPs. Interestingly, 24 of these rules were sufficient to predict the biodegradation pathways leading to the overall 45 well-defined and 34 ambiguous TP structures found (Fig. 4, ESI-II Section S3.1†). The products of oxygenation reactions (+O) turned out to be the most challenging to assign a well-defined structure to due to the multitude of possible isomers. For example, the use of the oxidative N-dealkylation rule (bt0063) only lead to well-defined structures in 48% of the cases, because the resulting TPs could not be distinguished from other possible oxidation products. The prediction of hydroxylation of methylene (bt0242) only lead to ambiguous structures for the same reason. Elucidating structures from these kinds of reactions would be especially important, because 70% of all found reactions belong to this category. Resolving the structures of TPs that resulted from hydration (+H2O) or hydrolysis (+H2O–X) was less challenging and lead to well-defined structures in 85% of the cases due to few plausible reaction sites or characteristic cleavage moieties. Desaturation-type reactions (−H2) were only predicted and found for the oxidation of primary (bt0001) and secondary alcohols (bt0002). The type of reaction could be determined through the atomic modifications relative to the precursor molecule, but the site of transformation was only identifiable in 62% of the cases. The beta-oxidation process (bt0337) was observed once and was not considered in Fig. 4, because it does not fit into any of the proposed categories.
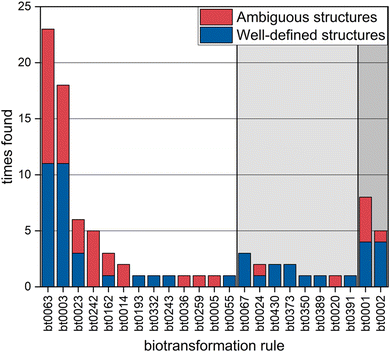 |
| Fig. 4 Comparison of biotransformation rules leading either to well-defined or ambiguous structures. The rules were categorized into monooxygenation (+O, white), hydrolysis and hydration (+H2O, light gray) and desaturation (−H2, dark gray). | |
Complementary approaches reveal and fill knowledge gaps in TP prediction models
Careful analysis of the time trends in chromatogram areas revealed TP-like behavior for several unidentified compounds, indicating that not all formed TPs were predicted by the employed pathway prediction methods. To identify the structures of analytes with TP behavior, we searched literature for known TPs, and we predicted conjugation reactions. APIs are particularly prone to undergo conjugation, as they often contain primary and secondary amines. However, this type of transformation is not covered by any of the TP prediction methods analyzed here, because they all focus exclusively on catabolic reactions. As a result, we tentatively identified four TPs were that underwent either N-acetylation or N-succinylation. For conjugation reactions, the MS2 spectra are closely related to those of the parent because they share the same molecular backbone, thus facilitating TP identification. Therefore, screening for conjugates can help identify additional TPs by considering reaction classes that are beyond the scope of the TP prediction tools.
Another eight TPs were either previously reported in literature or derived by expert logic (e.g., suspected hydroxylation when observing corresponding mass signature and TP-like behavior over time). Three of them were previously reported in literature and reference standards were available to the authors, but they were neither predicted nor part of any of the used databases. For example, the TP guanylurea of metformin was not predicted, even though it is known to literature.72 These cases highlight the importance of expanding the databases towards more diversity in terms of chemical structure, application class, and biodegradation environment. In the particular case of pharmaceuticals, it could be helpful to also consider metabolites produced by human metabolism or human microbiomes, because of the potential overlap of degradation mechanisms present in human and wastewater systems. For example, the only detected TP of aliskiren was not predicted by any TP prediction model but reported to also occur in human metabolism.52 Computational tools for drug metabolite prediction could therefore be applied to complement environmental TP prediction with prediction tools for human drug metabolism (e.g., Metabolitepredict,41 NICEdrug.ch,73 Biotransformer 3.0 (ref. 74)).
Conclusion
We present an updated workflow to identify TPs in activated sludge biodegradation experiments using suspect screening. We applied the workflow to 46 pharmaceutical substances and tentatively identified 79 TPs for 31 parent compounds. Of these, 66 (83%) are TPs reported for the first time in activated sludge, and only 13 TPs have previously been reported in similar or wastewater studies. We further compared our workflow with a comprehensive list of similar studies, and we discussed limitations of the analytical and computational methodology.
This workflow was applied to a specific biotransformation experiment and achieved a good ratio of found TPs per parent despite having an initial spiked concentration of 8 μg L−1 only, which is more than an order of magnitude lower than the concentrations of the original experiment conducted by Helbling et al.7 and the majority of studies reviewed here. Regarding the analytical methods, 15 out of the 27 analyzed studies complemented suspect screening with non-target screening to detect more TPs. Since conjugation reactions are not currently predicted by the EAWAG-PPS or enviPath, we suggest to complement the suspect list with TPs formed by acetylation, formylation, fumarylation, malonylation and/or succinylation. Another approach to detect more TPs would be to perform a systematic literature review on each parent compound to expand the suspect list towards TPs found in environmental biodegradation studies or mammalian metabolism.
Although our prediction precision is comparable to the precision reported by other studies and sufficient to perform a successful suspect screening, a higher precision would decrease the manual effort required to verify mass spectra. A systematic approach to improve the precision of the TP prediction methods would involve the collection of more high-quality biodegradation data to better cover the chemical diversity of organic micropollutants, and hence to increase the prediction accuracy of the machine learning models. However, if resources are limited, predicting 30 TPs per parent compound with the currently available models will achieve reasonable predictions without any significant loss in sensitivity. Currently, the training data sets for BBD, SOIL and SLUDGE together contain 623 degradation pathways, which only represents a small fraction of the chemical compound space. The combination of all these and the incorporation of the EAWAG-PPS led to the most comprehensive suspect list.
To share our results with the scientific community in a computer readable format, we enriched the EAWAG-SLUDGE data package with the newly obtained biodegradation pathways for 34 pharmaceuticals in activated sludge, thus feeding our learnings back into the design-build-test-learn cycle to evolve towards robust biotransformation prediction tools adapted to different environmental situations. As data acquisition is crucial to develop better models, future work will focus on improving the integration of the prediction platform enviPath with MS screening tools and on facilitating systematic and standardized data upload to enviPath. We hope that our work can guide TP identification efforts in the future and encourage researchers to share biodegradation data openly to improve prediction models.
Disclaimer
This manuscript only reflects the authors' views and the JU is not responsible for any use that may be made of the information it contains.
Data availability
The biotransformation pathways were uploaded to the enviPath database and integrated into the publicly accessible EAWAG-SLUDGE package available at https://envipath.org/package/7932e576-03c7-4106-819d-fe80dc605b8a. Results are further detailed in the ESI-I and II (Supplementary_Information_I.docx and Supplementary_Information_II-TP_data.docx).† Raw MS output can be obtained from the authors upon reasonable request. All scripts used to predict TPs, create suspect lists, and analyze data are publicly available at https://github.com/FennerLabs/TP_predict. The TP prediction uses the enviPath platform and therefore requires the installation of the enviPath python API (enviPath-python version 0.2.0, https://github.com/enviPath/enviPath-python). Detailed instructions can be found in the README file of the git repository. This resource also provides the code to convert the output of the enviPath pathway prediction and EAWAG-PPS into suspect lists that are compatible with the Compound Discoverer software.
Author contributions
CC, KF and JH designed the study. CC and LT performed sludge experiments, LC-MS measurements and analysis in Compound Discoverer. LT and JH performed data conversion and analysis. JH predicted transformation products. LT, CC, KF and JH wrote the manuscript. KF reviewed all the TP structural assignments and acquired the funding.
Conflicts of interest
There are no conflicts of interest to declare.
Acknowledgements
CC, JH and KF are members of the Prioritisation and Risk Evaluation of Medicines In the EnviRonment (PREMIER). PREMIER has received funding from the Innovative Medicines Initiative 2 Joint Undertaking under grant agreement no. 875508. This Joint Undertaking receives support from the European Union's Horizon 2020 Research and Innovation Programme and EFPIA.
References
- A. B. A. Boxall, C. J. Sinclair, K. Fenner, D. Kolpin and S. J. Maund, When synthetic chemicals degrade in the environment, Environ. Sci. Technol., 2004, 38, 368A–375A CrossRef CAS PubMed.
- T. Reemtsma, U. Berger, H. P. H. Arp, H. Gallard, T. P. Knepper, M. Neumann, J. B. Quintana and P. de Voogt, Mind the Gap: Persistent and Mobile Organic Compounds—Water Contaminants That Slip Through, Environ. Sci. Technol., 2016, 50, 10308–10315 CrossRef CAS PubMed.
- M. J. Andrés-Costa, K. Proctor, M. T. Sabatini, A. P. Gee, S. E. Lewis, Y. Pico and B. Kasprzyk-Hordern, Enantioselective transformation of fluoxetine in water and its ecotoxicological relevance, Sci. Rep., 2017, 7, 15777 CrossRef PubMed.
-
Regulation (EC) No. 1107/2009 of the European Parliament and of the Council of 21 October 2009 Concerning the Placing of Plant Protection Products on the Market and Repealing Council Directives 79/117/EEC and 91/414/EEC
Search PubMed.
-
Regulation (EU) No. 528/2012 of the European Parliament and of the Council of 22 May 2012 Concerning the Making Available on the Market and Use of Biocidal Products
Search PubMed.
-
Directive 2001/83/EC of the European Parliament and of the Council of 6 November 2001 on the Community Code Relating to Medicinal Products for Human Use
Search PubMed.
- D. E. Helbling, J. Hollender, H.-P. E. Kohler, H. Singer and K. Fenner, High-Throughput Identification of Microbial Transformation Products of Organic Micropollutants, Environ. Sci. Technol., 2010, 44, 6621–6627 CrossRef CAS PubMed.
- Y. Moriya, D. Shigemizu, M. Hattori, T. Tokimatsu, M. Kotera, S. Goto and M. Kanehisa, PathPred: an enzyme-catalyzed metabolic pathway prediction server, Nucleic Acids Res., 2010, 38, W138–W143 CrossRef CAS PubMed.
- V. Hatzimanikatis, C. Li, J. A. Ionita, C. S. Henry, M. D. Jankowski and L. J. Broadbelt, Exploring the diversity of complex metabolic networks, Bioinformatics, 2005, 21, 1603–1609 CrossRef CAS PubMed.
- M. Koch, T. Duigou and J.-L. Faulon, Reinforcement Learning for Bioretrosynthesis, ACS Synth. Biol., 2020, 9, 157–168 CrossRef CAS PubMed.
- L. B. M. Ellis, D. Roe and L. P. Wackett, The University of Minnesota Biocatalysis/Biodegradation Database: the first decade, Nucleic Acids Res., 2006, 34, D517–D521 CrossRef CAS PubMed.
- K. Fenner, J. Gao, S. Kramer, L. Ellis and L. Wackett, Data-driven extraction of relative reasoning rules to limit combinatorial explosion in biodegradation pathway prediction, Bioinformatics, 2008, 24, 2079–2085 CrossRef CAS PubMed.
- S. Kern, K. Fenner, H. P. Singer, R. P. Schwarzenbach and J. Hollender, Identification of Transformation Products of Organic Contaminants in Natural Waters by Computer-Aided Prediction and High-Resolution Mass Spectrometry, Environ. Sci. Technol., 2009, 43, 7039–7046 CrossRef CAS PubMed.
- E. Palm and A. Kruve, Machine Learning for Absolute Quantification of Unidentified Compounds in Non-Targeted LC/HRMS, Molecules, 2022, 27, 1013 CrossRef CAS PubMed.
- J. Gao, L. B. M. Ellis and L. P. Wackett, The University of Minnesota Biocatalysis/Biodegradation Database: Improving Public Access, Nucleic Acids Res., 2010, 38, D488–D491 CrossRef CAS PubMed.
- J. Wicker, T. Lorsbach, M. Gütlein, E. Schmid, D. Latino, S. Kramer and K. Fenner, enviPath – the environmental contaminant biotransformation pathway resource, Nucleic Acids Res., 2015, gkv1229 Search PubMed.
- D. A. R. S. Latino, J. Wicker, M. Gütlein, E. Schmid, S. Kramer and K. Fenner, Eawag-Soil in enviPath: a new resource for exploring regulatory pesticide soil biodegradation pathways and half-life data, Environ. Sci.: Processes Impacts, 2017, 19, 449–464 RSC.
- J. Wicker, K. Fenner, L. Ellis, L. Wackett and S. Kramer, Predicting biodegradation products and pathways: a hybrid knowledge- and machine learning-based approach, Bioinformatics, 2010, 26, 814–821 CrossRef CAS PubMed.
- J. Y. C. Tam, T. Lorsbach, S. Schmidt and J. S. Wicker, Holistic evaluation of biodegradation pathway prediction: assessing multi-step reactions and intermediate products, J. Cheminf., 2021, 13, 63 Search PubMed.
- J. Stanstrup, S. Neumann and U. Vrhovšek, PredRet: Prediction of Retention Time by Direct Mapping between Multiple Chromatographic Systems, Anal. Chem., 2015, 87, 9421–9428 CrossRef CAS PubMed.
- P. Bonini, T. Kind, H. Tsugawa, D. K. Barupal and O. Fiehn, Retip: Retention Time Prediction for Compound Annotation in Untargeted Metabolomics, Anal. Chem., 2020, 92, 7515–7522 CrossRef CAS PubMed.
- H. Horai, M. Arita, S. Kanaya, Y. Nihei, T. Ikeda, K. Suwa, Y. Ojima, K. Tanaka, S. Tanaka, K. Aoshima, Y. Oda, Y. Kakazu, M. Kusano, T. Tohge, F. Matsuda, Y. Sawada, M. Y. Hirai, H. Nakanishi, K. Ikeda, N. Akimoto, T. Maoka, H. Takahashi, T. Ara, N. Sakurai, H. Suzuki, D. Shibata, S. Neumann, T. Iida, K. Tanaka, K. Funatsu, F. Matsuura, T. Soga, R. Taguchi, K. Saito and T. Nishioka, MassBank: a public repository for sharing mass spectral data for life sciences, J. Mass Spectrom., 2010, 45, 703–714 CrossRef CAS PubMed.
-
NIST Standard Reference Database 1A
Search PubMed.
-
HighChem LLC, Advanced Mass Spectral Database (mzCloud), https://www.mzcloud.org/ Search PubMed.
- K. Dührkop, M. Fleischauer, M. Ludwig, A. A. Aksenov, A. V. Melnik, M. Meusel, P. C. Dorrestein, J. Rousu and S. Böcker, SIRIUS 4: a rapid tool for turning tandem mass spectra into metabolite structure information, Nat. Methods, 2019, 16, 299–302 CrossRef PubMed.
- F. Allen, R. Greiner and D. Wishart, Competitive fragmentation modeling of ESI-MS/MS spectra for putative metabolite identification, Metabolomics, 2015, 11, 98–110 CrossRef CAS.
- S. Wolf, S. Schmidt, M. Müller-Hannemann and S. Neumann,
In silico fragmentation for computer assisted identification of metabolite mass spectra, BMC Bioinf., 2010, 11, 148 CrossRef PubMed.
- E. L. Schymanski, J. Jeon, R. Gulde, K. Fenner, M. Ruff, H. P. Singer and J. Hollender, Identifying Small Molecules via High Resolution Mass Spectrometry: Communicating Confidence, Environ. Sci. Technol., 2014, 48, 2097–2098 CrossRef CAS PubMed.
- R. Gulde, D. E. Helbling, A. Scheidegger and K. Fenner, pH-Dependent Biotransformation of Ionizable Organic Micropollutants in Activated Sludge, Environ. Sci. Technol., 2014, 48, 13760–13768 CrossRef CAS PubMed.
-
T. Letzel, S. Grosse, W. Schulz, T. Lucke, A. Kolb, M. Sengl and M. Letzel, in Assessing Transformation Products of Chemicals by Non-target and Suspect Screening – Strategies and Workflows Volume 1, American Chemical Society, 2016, vol. 1241, pp. 85–101 Search PubMed.
- D. E. Helbling, J. Hollender, H.-P. E. Kohler and K. Fenner, Structure-Based Interpretation of Biotransformation Pathways of Amide-Containing Compounds in Sludge-Seeded Bioreactors, Environ. Sci. Technol., 2010, 44, 6628–6635 CrossRef CAS PubMed.
- S. Kern, R. Baumgartner, D. E. Helbling, J. Hollender, H. Singer, M. J. Loos, R. P. Schwarzenbach and K. Fenner, A tiered procedure for assessing the formation of biotransformation products of pharmaceuticals and biocides during activated sludge treatment, J. Environ. Monit., 2010, 12, 2100–2111 RSC.
- T. Letzel, A. Bayer, W. Schulz, A. Heermann, T. Lucke, G. Greco, S. Grosse, W. Schüssler, M. Sengl and M. Letzel, LC–MS screening techniques for wastewater analysis and analytical data handling strategies: sartans and their transformation products as an example, Chemosphere, 2015, 137, 198–206 CrossRef CAS PubMed.
- S. Achermann, P. Falås, A. Joss, C. B. Mansfeldt, Y. Men, B. Vogler and K. Fenner, Trends in Micropollutant Biotransformation along a Solids Retention Time Gradient, Environ. Sci. Technol., 2018, 52, 11601–11611 CrossRef CAS PubMed.
- A. K. Psoma, N. I. Rousis, E. N. Georgantzi and N. S. Thomaidis, An integrated approach to MS-based identification and risk assessment of pharmaceutical biotransformation in wastewater, Sci. Total Environ., 2021, 770, 144677 CrossRef CAS PubMed.
- P. Gago-Ferrero, E. L. Schymanski, A. A. Bletsou, R. Aalizadeh, J. Hollender and N. S. Thomaidis, Extended Suspect and Non-Target Strategies to Characterize Emerging Polar Organic Contaminants in Raw Wastewater with LC-HRMS/MS, Environ. Sci. Technol., 2015, 49, 12333–12341 CrossRef CAS PubMed.
- Y. Zhang, H. Zhang, J. Wang, Z. Yu, H. Li and M. Yang, Suspect and target screening of emerging pesticides and their transformation products in an urban river using LC-QTOF-MS, Sci. Total Environ., 2021, 790, 147978 CrossRef CAS PubMed.
- R. Gulde, M. Rutsch, B. Clerc, J. E. Schollée, U. von Gunten and C. S. McArdell, Formation of transformation products during ozonation of secondary wastewater effluent and their fate in post-treatment: from laboratory- to full-scale, Water Res., 2021, 200, 117200 CrossRef CAS PubMed.
- J. E. Schollée, E. L. Schymanski, S. E. Avak, M. Loos and J. Hollender, Prioritizing Unknown Transformation Products from Biologically-Treated Wastewater Using High-Resolution Mass Spectrometry, Multivariate Statistics, and Metabolic Logic, Anal. Chem., 2015, 87, 12121–12129 CrossRef PubMed.
- R. Gulde, U. Meier, E. L. Schymanski, H.-P. E. Kohler, D. E. Helbling, S. Derrer, D. Rentsch and K. Fenner, Systematic Exploration of Biotransformation Reactions of Amine-Containing Micropollutants in Activated Sludge, Environ. Sci. Technol., 2016, 50, 2908–2920 CrossRef CAS PubMed.
- Q. Wang and R. Xu, MetabolitePredict: a de novo human metabolomics prediction system and its applications in rheumatoid arthritis, J. Biomed. Inf., 2017, 71, 222–228 CrossRef PubMed.
- L. Carlsson, O. Spjuth, S. Adams, R. C. Glen and S. Boyer, Use of historic metabolic biotransformation data as a means of anticipating metabolic sites using MetaPrint2D and Bioclipse, BMC Bioinf., 2010, 11, 362 CrossRef PubMed.
- S. Lim, C. S. McArdell and U. von Gunten, Reactions of aliphatic amines with ozone: kinetics and mechanisms, Water Res., 2019, 157, 514–528 CrossRef CAS PubMed.
- C. Prasse, M. Wagner, R. Schulz and T. A. Ternes, Biotransformation of the Antiviral Drugs Acyclovir and Penciclovir in Activated Sludge Treatment, Environ. Sci. Technol., 2011, 45, 2761–2769 CrossRef CAS PubMed.
- V. G. Beretsou, A. K. Psoma, P. Gago-Ferrero, R. Aalizadeh, K. Fenner and N. S. Thomaidis, Identification of biotransformation products of citalopram formed in activated sludge, Water Res., 2016, 103, 205–214 CrossRef CAS PubMed.
- B. Jiao, Y. Zhu, J. Xu, F. Dong, X. Wu, X. Liu and Y. Zheng, Identification and ecotoxicity prediction of pyrisoxazole transformation products formed in soil and water using an effective HRMS workflow, J. Hazard. Mater., 2022, 424, 127223 CrossRef CAS PubMed.
- K. Rocco, C. Margoum, L. Richard and M. Coquery, Enhanced database creation with in silico workflows for suspect screening of unknown tebuconazole transformation products in environmental samples by UHPLC-HRMS, J. Hazard. Mater., 2022, 440, 129706 CrossRef CAS PubMed.
- J. Kirchmair, M. J. Williamson, J. D. Tyzack, L. Tan, P. J. Bond, A. Bender and R. C. Glen, Computational Prediction of Metabolism: Sites, Products, SAR, P450 Enzyme Dynamics, and Mechanisms, J. Chem. Inf. Model., 2012, 52, 617–648 CrossRef CAS PubMed.
- P. Piechota, M. T. D. Cronin, M. Hewitt and J. C. Madden, Pragmatic Approaches to Using Computational Methods To Predict Xenobiotic Metabolism, J. Chem. Inf. Model., 2013, 53, 1282–1293 CrossRef CAS PubMed.
- A. Sveshnikova, H. MohammadiPeyhani and V. Hatzimanikatis, Computational tools and resources for designing new pathways to small molecules, Curr. Opin. Biotechnol., 2022, 76, 102722 CrossRef CAS PubMed.
- A. A. Bletsou, J. Jeon, J. Hollender, E. Archontaki and N. S. Thomaidis, Targeted and non-targeted liquid chromatography-mass spectrometric workflows for identification of transformation products of emerging pollutants in the aquatic environment, TrAC, Trends Anal. Chem., 2015, 66, 32–44 CrossRef CAS.
- L. Chibwe, I. A. Titaley, E. Hoh and S. L. M. Simonich, Integrated Framework for Identifying Toxic Transformation Products in Complex Environmental Mixtures, Environ. Sci. Technol. Lett., 2017, 4, 32–43 CrossRef CAS PubMed.
- A. K. Singh, M. Bilal, H. M. N. Iqbal and A. Raj, Trends in predictive biodegradation for sustainable mitigation of environmental pollutants: recent progress and future outlook, Sci. Total Environ., 2021, 770, 144561 CrossRef CAS PubMed.
- E. Müller, W. Schüssler, H. Horn and H. Lemmer, Aerobic biodegradation of the sulfonamide antibiotic sulfamethoxazole by activated sludge applied as co-substrate and sole carbon and nitrogen source, Chemosphere, 2013, 92, 969–978 CrossRef PubMed.
- S. Huntscha, T. B. Hofstetter, E. L. Schymanski, S. Spahr and J. Hollender, Biotransformation of Benzotriazoles: Insights from Transformation Product Identification and Compound-Specific Isotope Analysis, Environ. Sci. Technol., 2014, 48, 4435–4443 CrossRef CAS PubMed.
- T. Kosjek, N. Negreira, M. L. de Alda and D. Barceló, Aerobic activated sludge transformation of methotrexate: identification of biotransformation products, Chemosphere, 2015, 119, S42–S50 CrossRef CAS PubMed.
- T. Kosjek, N. Negreira, E. Heath, M. López de Alda and D. Barceló, Aerobic activated sludge transformation of vincristine and identification of the transformation products, Sci. Total Environ., 2018, 610–611, 892–904 CrossRef CAS PubMed.
- M. T. Zumstein and D. E. Helbling, Biotransformation of antibiotics: exploring the activity of extracellular and intracellular enzymes derived from wastewater microbial communities, Water Res., 2019, 155, 115–123 CrossRef CAS PubMed.
- T. Gornik, A. Kovacic, E. Heath, J. Hollender and T. Kosjek, Biotransformation study of antidepressant sertraline and its removal during biological wastewater treatment, Water Res., 2020, 181, 115864 CrossRef CAS PubMed.
- R. A. Trenholm, B. J. Vanderford, N. Lakshminarasimman, D. C. McAvoy and E. R. V. Dickenson, Identification of Transformation Products for Benzotriazole, Triclosan, and Trimethoprim by Aerobic and Anoxic-Activated Sludge, J. Environ. Eng., 2020, 146, 04020094 CrossRef CAS.
- Y. Wang, K. Fenner and D. E. Helbling, Clustering micropollutants based on initial biotransformations for improved prediction of micropollutant removal during conventional activated sludge treatment, Environ. Sci.: Water Res. Technol., 2020, 6, 554–565 RSC.
- G. Wu, J. Geng, Y. Shi, L. Wang, K. Xu and H. Ren, Comparison of diclofenac transformation in enriched nitrifying sludge and heterotrophic sludge: transformation rate, pathway, and role exploration, Water Res., 2020, 184, 116158 CrossRef CAS PubMed.
- W. Cai, P. Ye, B. Yang, Z. Shi, Q. Xiong, F. Gao, Y. Liu, J. Zhao and G. Ying, Biodegradation of typical azole fungicides in activated sludge under aerobic conditions, J. Environ. Sci., 2021, 103, 288–297 CrossRef CAS PubMed.
- Y. Choi, J. Jeon and S. D. Kim, Identification of biotransformation products of organophosphate ester from various aquatic species by suspect and non-target screening approach, Water Res., 2021, 200, 117201 CrossRef CAS PubMed.
- A. B. Martínez-Piernas, P. Plaza-Bolaños and A. Agüera, Assessment of the presence of transformation products of pharmaceuticals in agricultural environments irrigated with reclaimed water by wide-scope LC-QTOF-MS suspect screening, J. Hazard. Mater., 2021, 412, 125080 CrossRef PubMed.
- S. L. Rich, M. T. Zumstein and D. E. Helbling, Identifying Functional Groups that Determine Rates of Micropollutant Biotransformations Performed by Wastewater Microbial Communities, Environ. Sci. Technol., 2022, 56, 984–994 CrossRef CAS PubMed.
- K. Fenner, C. Screpanti, P. Renold, M. Rouchdi, B. Vogler and S. Rich, Comparison of Small Molecule Biotransformation Half-Lives between Activated Sludge and Soil: Opportunities for Read-Across?, Environ. Sci. Technol., 2020, 54, 3148–3158 CrossRef CAS PubMed.
- R. Gulde, S. Anliker, H.-P. E. Kohler and K. Fenner, Ion Trapping of Amines in Protozoa: A Novel Removal Mechanism for Micropollutants in Activated Sludge, Environ. Sci. Technol., 2018, 52, 52–60 CrossRef CAS PubMed.
- G. Castro, M. Ramil, R. Cela and I. Rodríguez, Identification and determination of emerging pollutants in sewage sludge driven by UPLC-QTOF-MS data mining, Sci. Total Environ., 2021, 778, 146256 CrossRef CAS PubMed.
- M. Zhang, J. Shen, Y. Zhong, T. Ding, P. D. Dissanayake, Y. Yang, Y. F. Tsang and Y. S. Ok, Sorption of pharmaceuticals and personal care products (PPCPs) from water and wastewater by carbonaceous materials: a review, Crit. Rev. Environ. Sci. Technol., 2022, 52, 727–766 CrossRef CAS.
- R. Tian, M. Posselt, K. Fenner and M. S. McLachlan, Increasing the Environmental Relevance of Biodegradation Testing by Focusing on Initial Biodegradation Kinetics and Employing Low-Level Spiking, Environ. Sci. Technol. Lett., 2023, 10, 40–45 CrossRef CAS.
- L. J. Tassoulas, A. Robinson, B. Martinez-Vaz, K. G. Aukema and L. P. Wackett, Filling in the Gaps in Metformin Biodegradation: a New Enzyme and a Metabolic Pathway for Guanylurea, Appl. Environ. Microbiol., 2021, 87, e03003–e03020 CrossRef CAS PubMed.
- H. MohammadiPeyhani, A. Chiappino-Pepe, K. Haddadi, J. Hafner, N. Hadadi and V. Hatzimanikatis, NICEdrug.ch, a workflow for rational drug design and systems-level analysis of drug metabolism, eLife, 2021, 10, e65543 CrossRef CAS PubMed.
- D. S. Wishart, S. Tian, D. Allen, E. Oler, H. Peters, V. W. Lui, V. Gautam, Y. Djoumbou-Feunang, R. Greiner and T. O. Metz, BioTransformer 3.0—a web server for accurately predicting metabolic transformation products, Nucleic Acids Res., 2022, gkac313 Search PubMed.
- J. Kinyua, A. K. Psoma, N. I. Psoma, M. Nika, A. Covaci, A. L. N. van Nuijs and N. S. Τhomaidis, Investigation of Biotransformation Products of p-Methoxymethylamphetamine and Dihydromephedrone in Wastewater by High-Resolution Mass Spectrometry, Metabolites, 2021, 11(2), 66 CrossRef CAS PubMed.
Footnotes |
† Electronic supplementary information (ESI) available. See DOI: https://doi.org/10.1039/d3em00161j |
‡ These authors contributed equally: Leo Trostel, Claudia Coll. |
|
This journal is © The Royal Society of Chemistry 2023 |
Click here to see how this site uses Cookies. View our privacy policy here.