Characterizing metals in particulate pollution in communities at the fenceline of heavy industry: combining mobile monitoring and size-resolved filter measurements†
Received
10th April 2023
, Accepted 9th August 2023
First published on 10th August 2023
Abstract
Exposures to metals from industrial emissions can pose important health risks. The Chester-Trainer-Marcus Hook area of southeastern Pennsylvania is home to multiple petrochemical plants, a refinery, and a waste incinerator, most abutting socio-economically disadvantaged residential communities. Existing information on fenceline community exposures is based on monitoring data with low temporal and spatial resolution and EPA models that incorporate industry self-reporting. During a 3 week sampling campaign in September 2021, size-resolved particulate matter (PM) metals concentrations were obtained at a fixed site in Chester and on-line mobile aerosol measurements were conducted around Chester-Trainer-Marcus Hook. Fixed-site arsenic, lead, antimony, cobalt, and manganese concentrations in total PM were higher (p < 0.001) than EPA model estimates, and arsenic, lead, and cadmium were predominantly observed in fine PM (<2.5 μm), the PM fraction which can penetrate deeply into the lungs. Hazard index analysis suggests adverse effects are not expected from exposures at the observed levels; however, additional chemical exposures, PM size fraction, and non-chemical stressors should be considered in future studies for accurate assessment of risk. Fixed-site MOUDI and nearby mobile aerosol measurements were moderately correlated (r ≥ 0.5) for aluminum, potassium and selenium. Source apportionment analyses suggested the presence of four major emissions sources (sea salt, mineral dust, general combustion, and non-exhaust vehicle emissions) in the study area. Elevated levels of combustion-related elements of health concern (e.g., arsenic, cadmium, antimony, and vanadium) were observed near the waste incinerator and other industrial facilities by mobile monitoring, as well as in residential-zoned areas in Chester. These results suggest potential co-exposures to harmful atmospheric metal/metalloids in communities surrounding the Chester-Trainer-Marcus Hook industrial area at levels that may exceed previous estimates from EPA modeling.
Environmental significance
Toxic trace metal/metalloids are emitted into the atmosphere as byproducts of many industrial operations, including petroleum refining, smelting, and waste incineration. In some highly industrialized areas residential communities are located immediately adjacent to emission sources, raising exposure concerns. Our results indicated that heavy metals levels in a fenceline study area are underestimated by EPA models. Heavy metals of concern were observed predominantly in fine particulate matter (PM), the size which penetrates deepest into the lungs, with important implications for health. Source apportionment analysis indicated general combustion as a major metals emission source. These results demonstrate the importance of novel measurement approaches to complement regulatory monitoring in accurately characterizing toxic exposures for fenceline communities.
|
1. Introduction
Particulate matter (PM), a complex mix of chemical components suspended in air as small particles, is a major contributor to excess mortality and global disease burden.1,2 Atmospheric metals are generally found in the condensed form due to the low vapor pressure of most metal compounds.3 Particulate metals in the atmosphere originate from both biogenic sources, such as natural mineral dust and wildfires, and anthropogenic sources, including fossil fuel refining and combustion, vehicle emissions, and waste incineration.4,5
Fossil fuel emissions generally are metals-enriched compared to other PM sources,5,6 and the metals fraction of PM has been recognized as a significant source of PM toxicity.7 Transition metals in PM, especially when acidic,8 have been associated with oxidative stress and mortality.7,9–11 Increases in mortality rates have been linked with exposure to PM lead12 and arsenic,13 among other metal/metalloids. Adverse health effects associated with inhalation of atmospheric metals and their compounds include neurotoxicity (lead, manganese, mercury), cancer (arsenic, cadmium, chromium, nickel), pulmonary and renal damage (cadmium), and respiratory irritation (lead, manganese, mercury, arsenic, cadmium, chromium, nickel).14 Pulmonary deposition of particulate matter varies by aerodynamic diameter, with smaller particles generally depositing deeper in the lungs to induce toxic effects; PM sizes under 1 μm are associated with alveolar zone deposition.15,16
The US Environmental Protection Agency (EPA) Risk-Screening Environmental Indicators (RSEI) estimates that nickel, cobalt, and chromium exposure constitute as much as 10, 16, and 20% of the total RSEI score (incorporating toxicity weight, exposed population, and estimated dose) from petroleum emissions nationwide, respectively.17 However, to accurately characterize community-level exposures and health risks, size-resolved empirical data on PM constituents like metals are needed. RSEI health risk estimates are based in part on Toxic Release Inventory (TRI) data, which currently rely on industry self-report,18 include only a limited group of metals, and do not incorporate information on particle size. The EPA provides modeled metals values through the Air Toxics Screening Assessment (AirToxScreen) at the census tract level throughout the US. Regulatory monitoring provides valuable long-term PM data, but typically at low spatial, size, and/or temporal resolution. Air metals measurements in PM for most US communities are based on multi-day filter sampling of PM2.5, PM10, and/or total suspended particles (TSP).
Polluting industries have been increasingly attracted to the Chester-Trainer-Marcus Hook area in Pennsylvania following industrial booms during the 20th century and ensuing economic and population declines.19 The area is currently home to a refining and chemical complex, one of the largest waste incinerators in the United States, and smaller chemical industries concentrated along the Delaware River waterfront. Many of these industrial facilities are located adjacent to residential communities. Although situated in one of the wealthiest counties of Pennsylvania, this area is among the most financially distressed and polluted in the state and has been the focus of decades-long environmental justice campaigns.20,21 The Pennsylvania Department of Environmental Protection (PA DEP) provides 1 in 6 day gravimetric PM and composition measurements at two regulatory monitoring locations in the industrialized area along the Chester-Trainer-Marcus Hook waterfront.
In this study, integrated PM sampling of 10 discrete size fractions was leveraged to investigate the size distribution of metals in PM, a key determinant of PM health impacts. Combining this established measurement technique with spatially distributed lower cost sensors and mobile monitoring provides a promising approach to capturing fine concentration gradients adjacent to fenceline communities.22 Using these complementary advanced online and offline mass spectrometry techniques provides more information than each technique individually, and demonstrates the utility of fenceline community measurements. This work augments existing modeling and regulatory data on PM metal concentrations in the Chester-Trainer-Marcus Hook, PA area.
2. Methods
2.1 Sampling campaign
A 3 week field campaign was conducted as part of the Hazardous Air Pollutant Monitoring and Assessment Project (HAP-MAP) study in September 2021 in Chester, Trainer, and Marcus Hook, Pennsylvania. The study included fixed-site measurements at Widener University from a fourth-floor chemistry laboratory, time-resolved mobile measurements by an Aerodyne Research Inc. Mobile Laboratory (AML) throughout the domain shown in Fig. 1, and low-cost PM sensors from Quant-AQ Modulair-PM. The fixed site, representing a polluted urban background approximately 1 to 4 miles removed from the densely industrialized waterfront, included a micro-orifice uniform deposit impactor (MOUDI, Model 100NR, TSI, Shoreview, MN, US). An Aerodyne soot particle aerosol mass spectrometer (SP-AMS) was deployed in the AML (Aerodyne, Billerica, MA, US).
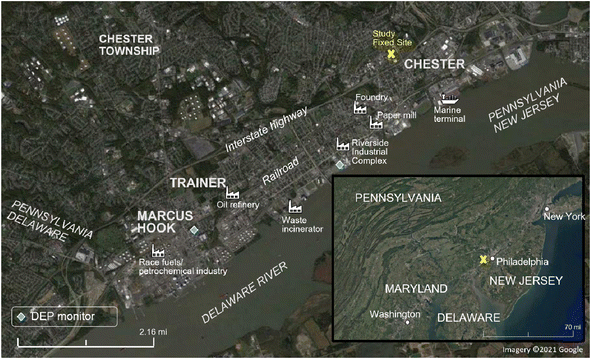 |
| Fig. 1 Map of study area labeled with measurement sites, industrial emission sources and locations of DEP monitors. Imagery ©2021 Google. | |
2.2 Reagents and standards
Optima conc. HNO3 (67–69%, Thermo Fisher Scientific, Waltham, MA, US), Optima conc. HF (47–51%, Thermo Fisher Scientific), Optima conc. HCl (32–35%, Thermo Fisher Scientific), MeOH suitable for HPLC (≥99.9%, Sigma Aldrich, Burlington, MA, US), and 18.2 MΩ cm ultrapure water from a Millipore-Sigma system (Sigma Aldrich) were used in all ICP-MS analyses in this work.
The following analytical standards were used for quantification by ICP-MS: a 10 ppm 32-element standard stock (ICP-MSCS-M-100, High Purity Standards, North Charleston, SC, US), Hg (10 mg L−1, SPEX, Metuchen, NJ, US), Si (1000 mg L−1, Sigma Aldrich), Ti (100 mg L−1, CPI International, Santa Rosa, CA, US), W (1000 mg L−1, Ricca Chemical, Arlington, TX, US), Sn (1000 mg L−1, Inorganic Ventures, Christiansburg, VA, US), Gd (1000 mg L−1, Elemental Scientific, Omaha, NE, US), and K (1000 mg L−1, LGC Standards, Teddington, UK).
The standard reference material used was SRM 1648a (Urban Air Particulate Matter from National Institutes of Standards and Technologies, Rockville, MD, US). Quality control spikes for several analytes were prepared from standards from different manufacturers than calibration standards, where possible: QCS-21 (100 mg L−1, High Purity Standards), Hg (10 mg L−1, SPEX), Si (1000 mg L−1, Sigma Aldrich), Ag (100 mg L−1, CPI International), Ba (1000 mg L−1, Ricca Chemical), U (1000 mg L−1, Ricca Chemical), Si (50 mg L−1, Hach, Loveland, CO, US), W (1000 mg L−1, Ricca Chemical), Sn (1000 mg L−1, Ricca Chemical). The internal standard solution used was LIS8-100 (Bi, Ge, In, Li-6, Rh, Sc, Tb, Y at 100 mg L−1, LGC Standards).
2.3 Fixed-site MOUDI filter collection and preparation
Ten PM size fractions with size cuts at aerodynamic particle diameters corresponding to 0.056, 0.10, 0.18, 0.32, 0.56, 1.0, 1.78, 3.16, 6.9, 18 μm were collected via MOUDI at the fixed site. A flow rate of approximately 30 standard liters per minute (SLPM) was maintained by rotary vane pump. Exact flow rates were recorded before and after each MOUDI run by a TSI flow meter (4000 series, TSI Inc, Shoreview MN, US), with high flow HEPA filter on the inlet to prevent contamination of filters, and averaged for air volume calculations. Attachment of the HEPA filter had a negligible effect on flow rate. For air sampling from the fixed site, the room window was opened and a wood composite board placed in the opening with a 22 mm hole drilled to accommodate the ¾ inch (∼20 mm) outer diameter (OD) copper inlet tubing.
Particulate matter samples were collected on 37 mm, 2 μm pore size PTFE membrane disc filters (R2PJ037, Pall, Port Washington, NY, US). Filters were weighed pre- and post-sampling in a temperature- and humidity-controlled environment at 21 ± 3 °C and 35 ± 5% relative humidity on a microbalance after equilibrating for at least 24 hours. Filters were transported to and from the fixed site in clean Petri dishes.
A total of 12 MOUDI runs were completed with varying integration times: 24 h (n = 6 sampling periods), 48 h (n = 5) and 96 h (n = 1), in addition to 2 field blank runs collected by connecting a HEPA filter to the inlet instead of copper tubing. To reduce contamination, MOUDI filter holders were cleaned in between runs using reagent-grade ethanol followed by ultrapure water, and filters were handled with tweezers by the polyethylene support rings in a clean laboratory fume hood.
Filters were separated from polyethylene support rings prior to acid digestion by cutting using a clean stainless steel razor and custom-made epoxy filter holder, consisting of a solid base and a top plate with a 37 mm diameter circular hole. Filters were mounted on clean weigh paper (Whatman, Maidstone, UK), replaced between each sample, to prevent cross-contamination via the epoxy base. The razor and filter holder were cleaned with ethanol and ultrapure water between each sample. For each MOUDI run, 4 to 6 analytical blank filters were removed from their packaging in the laboratory and cut out using the same procedures as samples. Filters were cut out in a HEPA class 2 positive pressure clean air hood.
Filter samples, SRM 1648 powder aliquots, and analytical filter blanks were acid-digested using a Mars 5 Xpress microwave system (CEM Corp, Matthews, NC, US) in a pressurized, two-stage, ramp-to-temperature method with a maximum temperature of 160 °C and a hold time of 10 minutes. Two milliliters of a 97.6% conc. HNO3, 2.4% conc. HF solution (v/v) were added to each sample in perfluoroalkoxy (PFA) microvessels (CEM Corp.) before microwaving. In between digestion runs, the reusable microvessels were cleaned by microwaving with conc. HNO3 using the same microwave program used for samples. Acid reagents were combined immediately before each digestion. After completion of the digestion, the sample was diluted to 5 mL by adding 3 mL of 0.5% HCl in ultrapure water. All handling of acids and acid digests was carried out in a biological safety cabinet using HF-resistant gloves and strict safety precautions.
2.4 Elemental analysis of fixed-site MOUDI samples by ICP-MS
Elemental analysis of the digested fixed-site MOUDI filter samples was conducted by Agilent 8900 ICP-MS/MS (Agilent Technologies, Santa Clara, CA, US) equipped with an octopole reaction system (ORS), 400 μL min−1 MicroMist nebulizer (Glass Expansion, Pocasset, MA, US), and Peltier-cooled (2 °C) Scott double-pass spray chamber (Agilent Technologies). Argon (Airgas US, LLC) was used as the plasma, dilution (0.20 L min−1), and carrier (1.0 L min−1) gases. The instrument was tuned daily to achieve acceptable oxide ratios (CeO/Ce < 0.5%), doubly-charged ratios (Ba++/Ba < 2%) and signal fluctuation (<3% relative standard deviation) in each run with hot plasma conditions (RF power 1600 W, sampling depth 9 mm).
Twenty-eight metal and metalloid isotopes (Na32, Mg24, Al27, Si28, K39, Ca44, Ti47, V51, Cr52, Mn55, Fe56, Co59, Ni60, Cu63, Zn66, As75, Se80, Sr88, Mo95, Ag107, Cd111, Sn118, Sb121, Ba138, W182, Pb208, Th232, U238) were determined in two ORS gas modes: O2 with mass shift (Ca, V, Cr, Fe, Ni, As, Se, Sb; 30%) and He (remainder of analytes; 4 mL min−1). All filter acid-digest samples were analyzed in one replicate each over three runs with randomized sample order. Aqueous external calibration was employed because no matrix effects were detected in preliminary analyses. Samples and standards were diluted using a reagent solution containing 0.5% (v/v) HCl and 1 μg L−1 internal standards to give a final HNO3 concentration of 4% for introduction into the ICP-MS.
Quantification was achieved using two calibration curves: a main curve prepared from a multi-element standard and a supplemental curve prepared from single-element solutions for additional elements of interest. For the main calibration curve, 6 calibration solutions were prepared from the multi-element standard ICP-MSCS-M-100 at levels ranging from 1 to 1000 μg L−1. The supplemental standard curve consisted of 5 levels ranging in concentration from 1 to 100 μg L−1 prepared from 7 individual calibration stock solutions. Three additional high silicon standard solutions containing 1, 20 and 50 mg L−1 silicon were included in each run to reach the concentration range present in samples. All calibration standards were prepared fresh daily. Rh103 was used as the internal standard for all analytes in He mode, and In115 for O2 mode (no mass shift). Calibration curves were processed using 1/y weighting.
One to two SRM 1648a acid digests and one to three multi-elemental QC spikes, matrix-matched to samples using blank filter acid digests, were analyzed in each run. A single-element silicon matrix-matched spike and aqueous multi-element spike were included in one run. For all analytes for which data are presented, excluding Th, known target concentrations were available for the SRM and/or spike. Accuracy for 25 of 29 analytes was within ±25% of certified or reference values in the SRM and/or of the spike target values in all three runs; for Se, Ni, and Sn, accuracy was within ±35% in one run (Table S1 ESI†), and for Si, which was excluded from quantitative analysis, ±75% in one run. Only total metals were included in this analysis; no speciation or valence state determinations were performed.
2.5 SP-AMS mobile monitoring analysis
Mobile on-line chemical composition and sizing measurements of metals in aerosols were conducted by SP-AMS mounted in the AML.23 Similar approaches to metals measurement by SP-AMS have been used by a small number of previous studies.24–33 The SP-AMS combines conventional high resolution Aerodyne AMS measurements of real-time, size-resolved composition of nonrefractory, aerosol particles with the ability to measure refractory particles.34 Nonrefractory aerosol particles are thermally vaporized at 600 °C using a resistively heated tungsten vaporizer while refractory particles are vaporized by a 1064 nm intracavity, continuous wave laser vaporizer. The resulting vapor is ionized by electron ionization at 70 eV and ions are detected in a high-resolution mass spectrometer. Data rate was 1 to 20 seconds with real-time viewing during collection allowing for identification of plumes and route corrections as needed. A standard aerodynamic lens was used for limiting particles to smaller than 1 μm in vacuum aerodynamic diameter.35 Data was processed in standard AMS analysis software packages including high resolution analysis. Inspection of high-resolution fitting identified acceptable mass spectral fits for 15 elements of interest (Al, As, Cd, Cr, Cu, Fe, K, Mn, Ni, Pb, Sb, Se, Sn, Sr, V) which were examined in further SP-AMS data analysis. The mobile laboratory containing the SP-AMS went on ∼8 h daily drives along a planned route to conduct mobile measurements, with times shifting daily to cover most hours of the day. For the remaining 16 hours per day, the AML was parked near the fixed site (∼885 m away) taking stationary measurements. Therefore, for approximately 8 hours per day on days of mobile measurements MOUDI samples were not co-measured by the SP-AMS.
Given the known challenges in quantification of refractory species, such as metals, due to difficulty in vaporization and ionization with the SP-AMS laser,24 SP-AMS data were treated as semi-quantitative. For all 15 elements of interest, correlations were explored between average SP-AMS measurements (corresponding to PM1) obtained overnight with the AML parked near the fixed site and fixed-site MOUDI PM1 metals concentrations averaged over the same time periods.
2.6 Data processing and statistical analysis
2.6.1 Fixed-site MOUDI data processing.
Elemental solution concentration Csol (ng mL−1) was blank-corrected and converted to air concentration Cair (ng m−3) using eqn (1): | 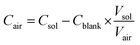 | (1) |
where Vsol is the volume of the acid digest (5 mL), Cblank is the mean concentration of the analytical blank filters in the run, and Vair is the air volume sampled in m3. Limit of detection (LOD) was calculated as 3 × σ where σ is the standard deviation of all analytical filter blanks analyzed (n = 33) excluding extreme outliers as identified by the generalized extreme studentized deviate test. For comparison with toxicological values only, concentrations below the LOD were imputed as
without blank filter correction, and negative values (detectable but negative after blank subtraction) were replaced with zero. Imputation of negative values resulted in the same estimate as the zero replacement approach used for 22 analytes, and overestimates of less than 2% for Mg, Al, Cr, and Ag; the zero replacement approach was selected as the preferred approach for handling negative values as it provided the more conservative (lower) estimate. The proportions of measurements below the LOD measurements for each MOUDI sample integration time are shown in Fig. S1 ESI.† Non-parametric statistical tests were used for statistical analyses of fixed-site MOUDI data, which were found to be log-normally distributed.
2.6.2 Mapping of SP-AMS mobile monitoring data.
Raw mobile SP-AMS metal measurements recorded in ions per second were aggregated by taking the mean over 50 m × 50 m (250 m2) grid cells excluding grid cells with fewer than 3 measurements during unique measurement hours throughout the campaign. For the purpose of qualitative hotspot identification and spatial signal comparison, based on previous work,22 3 was chosen as the minimum number of passes for which the mean gives a reliable estimate of signal intensity in a given grid cell throughout the campaign, while minimizing the exclusion of potential hotpots. Spatial analysis and mapping of mobile data was carried out in R software (v4.1, Vienna, Austria). Zoning data, used in investigating proximity of mobile AMS signals to residential areas, were derived from the Delaware County, PA, Mapping and Data portal (https://portal-dcpd.opendata.arcgis.com/, data updated in June, 2021).
2.6.3 Source apportionment.
Positive matrix factorization (PMF)36,37 was conducted on fixed-site MOUDI data using each size fraction (n = 10) at each time (n = 12) as a data point for a total of 120 unique samples for PMF analysis. A model error of 10% and minimum error equivalent to the lowest observed standard deviation in the dataset multiplied by 3 (3 × 10−5 ng m−3) were applied in PMF. Standard deviation of 3 instrumental replicates multiplied by 3 was used to calculate the associated error matrix for each analyte. Silicon was excluded from PMF analysis because of lower analytical accuracy compared to other analytes; further, as the most abundant analyte, the high absolute error for Si greatly influenced the PMF solution. The PMF solution was chosen based on the ratio of the observed to expected Q, where Q refers to the sum of the weighed squared residuals of the fit. PMF was conducted using the PET tool in Igor Pro software (v8.04, WaveMetrics, Lake Oswego, OR, US).36
Agglomerative hierarchical clustering analysis (HCA) was used as a complementary approach to PMF to investigate clustering of size-resolved metals concentrations measured by ICP-MS. Particle size distributions of metals were averaged over the campaign. HCA and Wilcoxon tests were conducted in R software with level of significance of 95%.
Wind direction data during the campaign was derived from the National Oceanic and Atmospheric Administration meteorological station at the Philadelphia Airport, approximately 5 miles east of the fixed site. Data were obtained through the Worldmet package (v.0.9.5) in R.38 Wind roses were created using the Openair package (v.2.12) in R.39 The predominant wind direction during the campaign in the study area was from the S/SW (32% of the time), followed by W/NW (28%), N/NE (21%), and E/SE (19%). Meteorological analysis could not be used to identify emission sources because of the variability of wind during each MOUDI sampling period, an inadequate number of samples per wind direction, and the clustering of many emission sources to the S/SW of the fixed site. Wind roses showing wind direction and wind speed for each day of MOUDI sampling are shown in Fig. S2 ESI.†
2.6.4 Comparisons with regulatory data, models, and toxicity values.
Differences in air concentration of metals between fixed-site MOUDI measurements, PA DEP data, and AirToxScreen data (census tracts entirely or mostly within a 10
000 m radius of the fixed site, the distance from a chemical emission source considered “fenceline” by EPA40) were evaluated using the Wilcoxon rank-sum test with level of significance of 95%. AirToxScreen data used was derived from the most recent assessment which represented annual averages for 2019 estimated using methods described on the EPA website.41 Briefly, AirToxScreen estimates ambient concentrations at the census tract level based on National Emissions Inventory (NEI) emissions data using chemical transport and dispersion models. Limitations include the use of emission inventories and models rather than observed data, which requires assumptions about air toxics emissions, and limited spatial resolution.
Because the MOUDI does not include a PM2.5 cut-point, 1.78 μm was used as an approximate cutoff for the MOUDI for fine PM comparisons. PA DEP data included TSP, PM2.5, and PM10 measurements for up to 7 elements (Be, As, Cd, Cr, Pb, Mn, Ni) from three Delaware County monitors (Chester, Marcus Hook, and Swarthmore) between the years 2017 and 2020, which included several temporal measurement gaps. PA DEP-reported Be concentrations were shown as zero for all three sites over this time period. Because of low PA DEP data precision, non-exact p-values were computed by the Wilcoxon rank-sum test in comparisons with PA DEP data. For AirToxScreen, data on modeled concentrations in TSP were compared to fixed-site MOUDI data from this study for compounds of Pb, Ni, Mn, Co, Cd, Sb, and inorganic As including arsine.
Concentrations obtained by ICP-MS were compared with corresponding toxicity values from US public health agencies using a hazard quotient approach. While the current study duration was only 3 weeks, chronic toxicity values were used based on comparisons of our measurements with regulatory measurements by PA DEP, which did not display strong long-term temporal trends between 2010 and 2020 (for six elements of interest in PM2.5 and TSP, R2 < 0.18) (Section 3.4, Fig. S3 ESI†).
When available, chronic exposure values published by the US EPA (reference concentration (RfC) from Integrated Risk Information System, IRIS; or rolling 3 month average primary/secondary standard from the National Ambient Air Quality Standard, NAAQS) were used. In the absence of EPA chronic values, chronic Agency for Toxic Substances and Disease Registry (ATSDR) values were prioritized, followed by chronic California Office of Environmental Health Hazard Assessment (OEHHA) values. For many elements, particularly transition metals for which numerous oxidation states and compounds of health concern may exist, attempts were made to include the toxicity value corresponding to the form or compound most likely present in the study area; when multiple forms or compounds were likely to be present, the most protective (lowest) value was selected. For each identified toxicity value, information on the source and numerical value was extracted, as well as the target organ or system (e.g., cardiovascular effects) upon which the toxicity value was based.
The median concentration of each element was divided by its corresponding toxicity value to generate a hazard quotient. Hazard quotients less than or equal to 1 indicate that adverse health effects are not expected to occur, whereas values above 1 indicate that adverse health effects are possible following exposure to the chemical. For elements with toxicity values based on a common target organ or system (e.g., respiratory effects), hazard indices were calculated by summing up each of the relevant hazard quotients. Hazard indices are interpreted in the same way as hazard quotients.
3. Results and discussion
3.1 Size distribution and source apportionment based on fixed-site MOUDI data
Size distributions of metals from fixed-site MOUDI analysis fell into three groups based on concentrations across size fractions: larger particle fraction with diameters >1.78 μm only (Na, Mg, Al, Ca, Ti, Cr, Mn, Fe, Co, Sr, Zr, Ba, U), smaller particle fraction with diameters less than 1 μm (As, Se), and both larger and smaller particle fractions (K, V, Ni, Cu, Zn, Mo, Ag, Cd, Sn, Sb, W, Pb, Th) (Fig. 2).
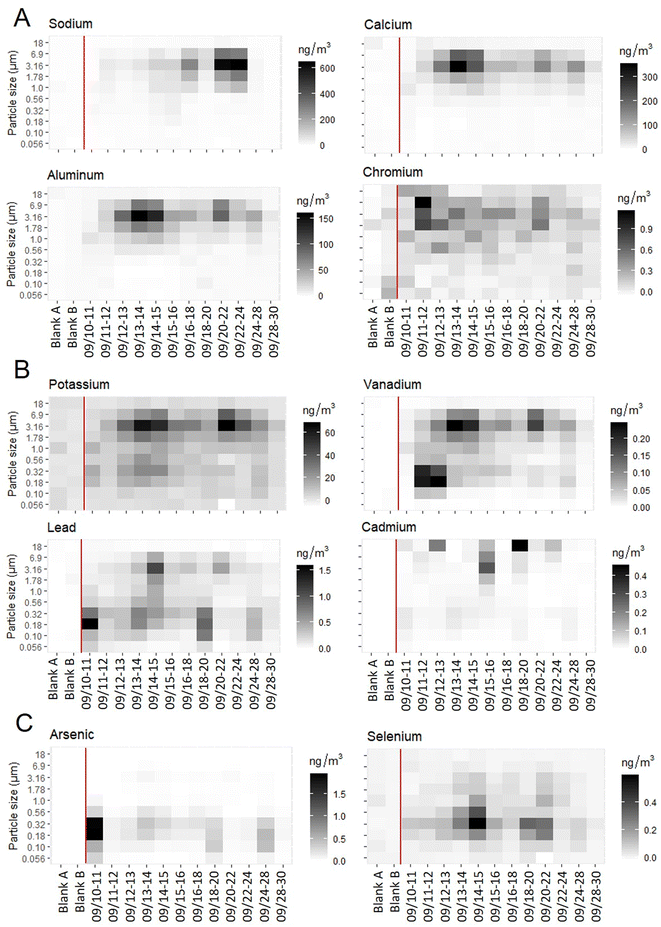 |
| Fig. 2 Fixed-site MOUDI data for selected elements throughout the HAP-MAP campaign, including field blanks, showing information on temporal changes and PM size. (A) Examples of elements enriched in the >1.78 μm PM fraction; (B) elements enriched both in <1 μm and >1.78 μm fractions; (C) elements enriched in the <1 μm PM fraction. Vertical red lines delineate field blank measurements from samples. | |
Based on average analyte concentrations by size fraction throughout the HAP-MAP campaign, HCA results suggested the presence of four elemental clusters. Clusters 1, 2, and 4 were earth-abundant elements Na, Ca, Al, Fe, Mg, and K; cluster 3 was a group of 21 elements including all the trace elements (Fig. S4 ESI†).
A four-factor PMF solution of fixed-site MOUDI data was selected. The ratio of the observed to expected Q fell sharply as the number of factors was increased from 1 to 3, then exhibited a gradual decrease with 4 or more factors (Fig. S5 ESI†). This trend in Q, combined with information about the PM size distributions (Fig. 2), suggests that 4 factors provide the simplest reasonable solution.
Elements were grouped into the four PMF factors representing sea salt (factor 1), mineral dust (factor 2), general combustion (factor 3), and non-exhaust vehicle (factor 4) sources (Fig. 3). Factor names were derived from the metal signatures present. The sea salt factor consists mainly of sodium and magnesium in the coarse PM fraction, two tracers of sea salt; the mass ratio Mg/Na for the sea salt factor was found to be 0.13 (molar ratio 0.12), similar to previous studies.42,43 Sea salt PM likely was derived from the Delaware River, which is brackish near the study area, or farther transport from the Atlantic 111 kilometers east, to the E/SE of the fixed measurement site. Long-distance transport of ocean-sourced aerosol is not uncommon; marine aerosol was observed to contribute significantly to urban aerosol in Paris, a continental city.44 Accordingly, the highest concentrations in the sea salt factor are observed when wind is predominantly from the east. The mineral dust factor was dominated by calcium and aluminum in the coarse fraction, which are two tracers for mineral dust (e.g., road and construction dust).45 The general combustion factor includes potassium and most of the trace elements measured in this study, and is the only factor that consists of mostly fine PM (PM1.78). Major industrial sources of combustion emissions are present in the study area, frequently upwind of the fixed site (Fig. 1 and S3 ESI†). In addition to fossil fuel combustion and refining, nearly all non-essential, trace, and crustal/major metals can be found in waste processed by incinerators, including K, Zn, and Pb.46,47 Non-exhaust vehicle emissions included mainly Ba, as well as Cu, Cr, Fe, Sr, Sb, and others in coarse size fractions. High traffic volume was present in the study area, with the interstate highway and minor highways close to the waterfront (Fig. 1). Ba in coarse particles has been associated with non-exhaust traffic emissions (brake and tire)48 as have Cu49 and Sb50 as discussed further in Section 3.3.
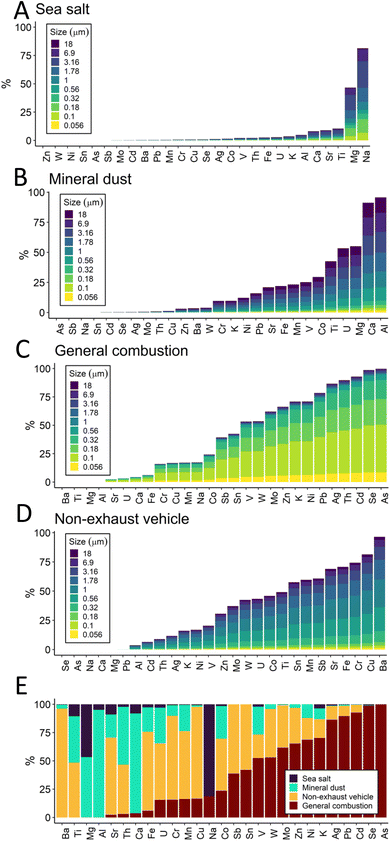 |
| Fig. 3 PMF results: percent contributions of each element's total contribution to PM by PM size fraction for the (A) sea salt, (B) mineral dust, (C) general combustion, (D) non-exhaust factors. (E) Distribution of each element among PMF factors. | |
Metals size distribution results indicate that trace elements were predominantly found in the fine fraction in the fixed site MOUDI samples. Source apportionment analysis by PMF suggested that many elements were attributed primarily to one of four emission sources. Notably, the trace elements Se, As, Pt, Cd, Pb, and Ag were all at least 75% contained within the general combustion factor; Al and Ca were almost entirely accounted for by the mineral dust factor; Na was mainly assigned to sea salt factor; and Ba and Cu were assigned more than 75% to non-exhaust vehicle emissions. The PMF results are largely consistent with the HCA analysis which grouped trace elements together distinct from crustal/major elements. K was grouped into the general combustion PMF factor along with trace elements, but was not included in the trace element cluster in HCA; this difference reflects the similarity in temporal patterns between K and the trace elements which are captured by PMF.
Our observation that fine PM associated with combustion emissions includes trace metals of health concern suggests the potential for metals deposition in the lower respiratory tract. Toxic trace elements As, Pb, Cd, Ni, Cr, and others showed the highest concentrations observed in the fine PM size fractions 0.18 and 0.32 μm. Consistent with our findings, previous studies have also found non-essential trace metals and K predominantly in, or spatially correlated with, fine PM.51–54
3.2 Comparison of mobile SP-AMS and fixed-site MOUDI measurements
Coefficients for Spearman correlations between SP-AMS mobile ions per second for metal species and ICP-MS PM1 filter concentrations were at least 0.5 for Al (r = 0.6), K (r = 0.9) and Se (r = 0.5) (Fig. 4). Correlation coefficients 0.4 or lower were observed for the 12 remaining analytes common to both techniques.
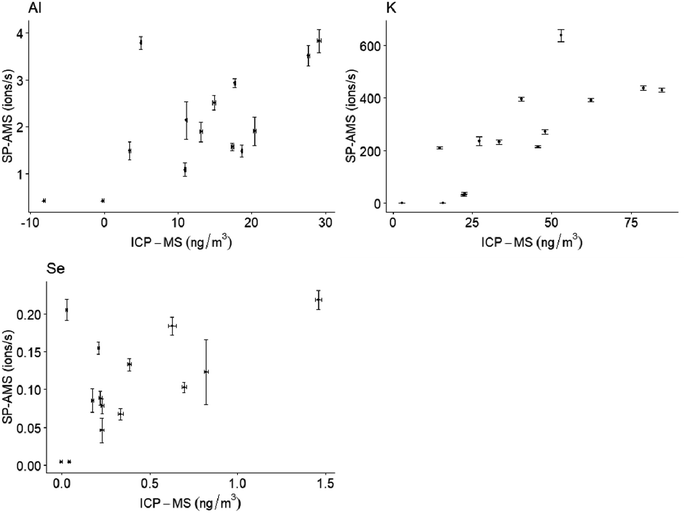 |
| Fig. 4 Scatter plots of average K, Se, and Al signals measured by SP-AMS as a function of concentrations determined by ICP-MS during equivalent sampling periods for PM1. Error bars represent the standard deviation of 3 instrumental replicates (ICP-MS) and of all measurements over the sampling period (SP-AMS). | |
Potassium measurements by SP-AMS are influenced by surface ionization, as previously noted.32 The relationship observed here between fixed-site MOUDI and SP-AMS measurements for K is specific to the instrument operating parameters used in this study and is therefore not expected for other instruments or measurements. These results from a subset of elements demonstrate the potential of combining fixed-site filter sampling with mobile measurements as a quantitation approach in future studies. Additional more refractory metals may have exhibited weaker relationships with ICP-MS measurements due to difficulty in vaporization and ionization with the SP-AMS laser.24 The ∼8 hours of non-overlapping sampling time per day may also have introduced discrepancy between the techniques for some of these analytes. Comparison of PM2.5 and PM10 measurements at the MOUDI measurement site versus the stationary SP-AMS site, using QuantAQ MODULAIR-PM instrumentation, suggests similar mean concentrations and temporal patterns throughout the campaign, albeit with some high concentration spikes at the MOUDI fixed site (Fig. S6 ESI†). Still, the horizontal and vertical distances between the stationary SP-AMS and the MOUDI measurements (fixed) sites may have contributed to some of the differences observed. Further work is needed to refine calibration and quantification approaches of mobile metals monitoring data.
3.3 Spatial distribution of metal/metalloids based on SP-AMS mobile monitoring
Maps showing qualitative spatial distributions of SP-AMS measurements for 4 elements of health concern are shown in Fig. 5. For Cd, hotspots were observed throughout the study domain, including near the Marcus Hook liquid natural gas (LNG) and race fuel plant and the waste incinerator. Hotspots of Sb, As, and V were also observed near the waste incinerator, as well as several elevated signals in Chester downtown to the NW of multiple chemical plants. Additional As hotspots near the LNG/race fuels plant, and V and Sb hotspots along the high-traffic interstate 95 corridor, were observed. Elevated signals of all four elements overlapped areas zoned as residential. The elemental maps also suggested concentrated elevated signals in Chester to the NW of the city's multiple chemical plants. These results indicate downtown Chester and the area adjacent to the waste incinerator as locations of potential interest for additional study of metals, e.g., potential sites for stationary monitors. AML elemental maps for the remaining 11 analytes investigated are available in Fig. S7 ESI.†
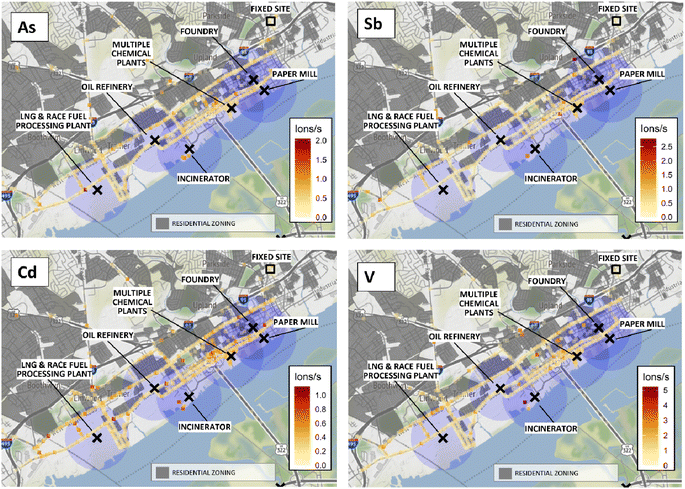 |
| Fig. 5 Spatial distribution of 4 metal/metalloids across study area, throughout the 1 month campaign, based on mobile SP-AMS measurements. Means of 3 or more measurements per 50 m × 50 m grid cell are shown. Residential zoning is shown by gray fill; light blue circles represent 1000 m buffers around TRI facilities (x). Symbol colors represent ions/s. | |
Fossil fuel combustion is known to generate PM containing many trace and non-essential metals including V, As, and Se55,56 in fine PM.49 Consistent with the general combustion PMF factor, higher intensities were also observed for trace elements Cd, Sb, V, and As near the waste incinerator. Note that maps represent PM1 only, and therefore crustal/major elements in coarse fractions are not represented.
3.4 Comparison of fixed-site MOUDI measurements with EPA model data and regulatory monitor data
EPA AirToxScreen-estimated concentrations of metals in total PM were significantly lower (p < 0.01) than HAP-MAP fixed-site measurements for Sb, As, Cr, Co, Pb, Mn, and Cd, and higher for Ni (p < 0.001) (Fig. 6; numerical values for MOUDI measurements and AirToxScreen estimates shown in Table S2 ESI†). AirToxScreen-modeled Sb concentrations were significantly higher in the industrial area along the waterfront compared to the rest of the 6-mile-radius domain around the fixed site (p < 0.001). For most elements determined, PA DEP monitoring site measurements were reported to a single integer significant figure, and many measurements were reported as 0 (e.g., As, Cd, Ni in Fig. 6 and S8 ESI†). The average TSP Mn concentration reported by PA DEP at the Chester monitoring site was higher than that obtained in this study (p < 0.05). For metals concentrations in fine PM, there were no significant differences between HAP-MAP fixed-site MOUDI data (PM1.78) and PA DEP data (PM2.5) except for As, for which all but one reported values from all three Delaware County monitoring sites were 0 ng m−3 during the 2017–2020 period (Fig. S2 and S8 ESI†).
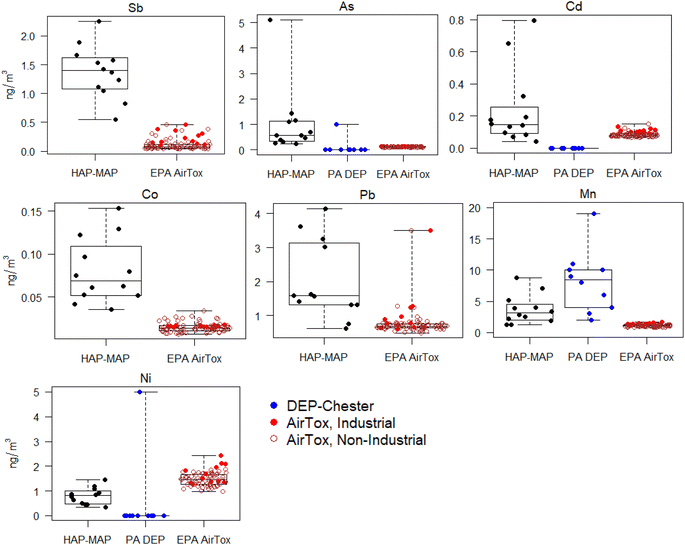 |
| Fig. 6 Comparison of HAP-MAP 2021 (fixed-site MOUDI data, this study), AirToxScreen 2017, and PA DEP 2017-2020 reports (Chester monitoring site; no data available from other sites for this time period) for 7 metal/metalloids in TSP. “AirTox, Industrial”: census tracts between Chester and Marcus Hook along the Delaware River. “AirTox, Non-Industrial”: census tracts in 6-mile radius of Widener University excluding waterfront. Error bars represent temporal (one month for fixed-site MOUDI data, 3 years for PA DEP data) and spatial (Delaware County census tracts for AirToxScreen data) variability. | |
Previous investigations have found both high and low biases in TRI data, which are self-reported by facilities and incorporated into AirToxScreen models.18 Our data suggests that at least for several metals, the modeled values in the Chester-Trainer-Marcus Hook area may be underestimates. For Pb and As, a previous study also found underestimation on the US national scale from the 2011 AirToxScreen (then known as the National Air Toxics Assessment) suggested to be caused by missing anthropogenic emission sources for these elements.52
Higher TSP Mn concentrations reported by DEP compared to this study could be due to low-level Mn contamination in quartz filters or spectral polyatomic interferences in ICP-MS. PA DEP monitoring data are collected using federal regulatory methods that necessarily have certain limitations. Specifically, PM is collected on quartz filters which have been reported to contain higher background metals levels than the PTFE filters used in our measurements.57 PM2.5 and PM10 samples are analyzed by X-ray fluorescence spectrometry, which typically has poorer detection limits and sensitivity compared to ICP-MS, the gold standard elemental analysis technique for trace analysis. Data on size resolution beyond PM2.5, PM10, and TSP are not available, and monitoring occurs 1-in-6 days or less frequently, with several extended gaps in coverage (Fig. S2 ESI†). One key advantage of DEP monitoring data, however, is its long-term temporal coverage over a 10 year period before the present study campaign.
3.5 Comparison of fixed-site MOUDI measurements with health-based toxicity values
In total, relevant toxicity values were found for 13 of the 28 elements determined by ICP-MS for HAP-MAP. Table S3 ESI† shows comparisons of concentrations obtained by HAP-MAP fixed-site and mobile analysis with toxicity values derived from US governmental and California state agencies. None of the estimated hazard quotients exceeded 1, indicating that no adverse health effects are expected as a result of exposure to any of the assessed elements alone, though toxicity values were available for fewer than half of the elements measured. All hazard quotients were below 0.05, with the exceptions of Cr, Mn, and Ti. The hazard quotient for Cr was calculated as 0.14 using the ATSDR chronic MRL for hexavalent Cr; however, Cr measured by ICP-MS was not speciated and cannot be assumed to be solely in the most toxic hexavalent form. Based on the identified endpoints associated with each toxicity value, hazard indices were calculated for four target organs/systems. No adverse health effects are expected as a result of combined exposure to this subset of analytes and chemical exposures: the hazard index was 0.33 for respiratory effects, 0.17 for renal effects, 0.11 for neurological effects, and 0.04 for cardiovascular effects. Additionally, the hazard indices for respiratory and renal effects likely overestimate risk associated with chromium exposure as a result of the aforementioned uncertainty regarding speciation.
These results should be interpreted in light of several additional limitations. Because fewer than half of the elements included in the fixed-site MOUDI analysis had available toxicity values, potential risks associated with the majority of measured elements remain unquantified. In addition, the hazard index approach assumes that combined chemicals have additive effects, though synergistic or antagonistic interactions among elements are possible.58 Moreover, the industrial facilities in southeastern Pennsylvania emit additional contaminants (e.g., volatile organic compounds) that are known to affect several of the same target organs or systems as the elements examined in the current study;14,17 while the elements included in this analysis may not pose risks in isolation, they may contribute to adverse health effects when considered in the context of additional chemical exposures. Trace metals with known toxicity (e.g., Pb, As) were also observed in the fine PM fraction in this study, the size fraction that penetrates deepest into the lungs to pose more severe systemic health effects.7 Available toxicity values do not distinguish by particle size and are based on total suspended particle concentrations. Lastly, fenceline communities tend to be comprised of low-income and minority populations who are burdened by social and economic stressors that may increase susceptibility to chemical exposures. Research suggests that current approaches to risk assessment may not adequately account for potential variation in human susceptibility and thus may fail to protect vulnerable populations.59
4. Conclusions
The presence of trace metals in emissions from a wide range of industries is well established, and many of these metals pose human health risks. In Chester-Trainer-Marcus Hook, PA, current data on metals do not provide a comprehensive picture of potential fenceline exposures due to low spatiotemporal resolution, incorporation of industry self-report, and limitations of regulatory methods. In this study, state-of-the-art analytical methods were employed as a new lens to characterize PM metals in heavily industrialized communities and identify potential metals emissions sources. Mobile measurements of metals identified hotspots near several industrial facilities, some overlapping with residential-zoned areas. While urban background levels measured at the fixed site were below current regulatory levels, levels of most metals were higher than US AirToxScreen estimates. AirToxScreen is a key tool used to make decisions about hazardous air pollutant exposures for fenceline communities in the US, making accuracy of estimates critical, especially for the most highly exposed members of the population living near industrial facilities.
PM varies greatly in size and composition by emission source and region. Health effects are determined by the size distribution and the mixture of chemical components present, and cannot be understood based on toxicology of single species,5 highlighting the importance of investigating PM in a local context. The data obtained in this study and investigated by size, spatially and by source apportionment approaches support previous findings that toxic trace elements are emitted by industrial combustion sources. These elements were observed in the smallest PM fractions, which penetrate most deeply into the lungs, with major implications for health. This work illustrates the importance of combining mobile and size-integrated analytical approaches to monitor metals and quantify exposures, protect the health of fenceline communities, and advance the evidence base for environmental justice.
Author contributions
MWT, investigation, formal analysis, methodology, writing – original draft; ECF and BSW, investigation; ESR, investigation, methodology, writing – review & editing; AAC, formal analysis, methodology, writing – review & editing; RS, investigation, writing – review & editing; CG, writing – review & editing; TY, supervision, project administration; SVB, project administration, writing – review & editing; TB, funding acquisition, supervision, writing – review & editing; KK, supervision, resources, writing – review & editing; KEN, funding acquisition, supervision, writing – review & editing; ANM, supervision, resources, validation, writing – review & editing; PFD, funding acquisition, conceptualization, resources, supervision, validation, writing – review & editing. All authors reviewed the final manuscript.
Conflicts of interest
There are no conflicts to declare.
Acknowledgements
The authors acknowledge support from Bloomberg Philanthropies (Grant ID 2021-100480). AAC was supported by NIEHS Training Grant T32ES007141. CG was supported by the Johns Hopkins University Education and Research Center for Occupational Safety and Health (ERC) from the National Institute for Occupational Safety and Health (NIOSH), under Grant No. 5 T42 OH 008428. The authors thank all operators of the Aerodyne Mobile Laboratory who worked on the sampling campaign.
References
- C. Liu, R. Chen, F. Sera, A. M. Vicedo-Cabrera, Y. Guo, S. Tong, M. S. Z. S. Coelho, P. H. N. Saldiva, E. Lavigne, P. Matus, N. Valdes Ortega, S. Osorio Garcia, M. Pascal, M. Stafoggia, M. Scortichini, M. Hashizume, Y. Honda, M. Hurtado-Díaz, J. Cruz, B. Nunes, J. P. Teixeira, H. Kim, A. Tobias, C. Íñiguez, B. Forsberg, C. Åström, M. S. Ragettli, Y.-L. Guo, B.-Y. Chen, M. L. Bell, C. Y. Wright, N. Scovronick, R. M. Garland, A. Milojevic, J. Kyselý, A. Urban, H. Orru, E. Indermitte, J. J. K. Jaakkola, N. R. I. Ryti, K. Katsouyanni, A. Analitis, A. Zanobetti, J. Schwartz, J. Chen, T. Wu, A. Cohen, A. Gasparrini and H. Kan, Ambient particulate air pollution and daily mortality in 652 cities, N. Engl. J. Med., 2019, 381(8), 705–715, DOI:10.1056/nejmoa1817364.
-
World Health Organization, WHO Global Air Quality Guidelines: Particulate Matter (PM 2.5 and PM 10), Ozone, Nitrogen Dioxide, Sulfur Dioxide and Carbon Monoxide, 2021 Search PubMed.
- D. Salcedo, A. Laskin, V. Shutthanandan and J. L. Jimenez, Feasibility of the detection of trace elements in particulate matter using online high-resolution aerosol mass spectrometry, Aerosol Sci. Technol., 2012, 46(11), 1187–1200, DOI:10.1080/02786826.2012.701354.
- S. Giannoukos, C. P. Lee, M. Tarik, C. Ludwig, S. Biollaz, H. Lamkaddam, U. Baltensperger, A. S. Henry Prevot and J. Slowik, Real-time detection of aerosol metals using online extractive electrospray ionization mass spectrometry, Anal. Chem., 2020, 92(1), 1316–1325, DOI:10.1021/acs.analchem.9b04480.
- P. Maciejczyk, L. C. Chen and G. Thurston, The role of fossil fuel combustion metals in PM2.5 air pollution health associations, Atmosphere, 2021, 12(9), 1–34, DOI:10.3390/atmos12091086.
- V. B. Vouk and W. T. Piver, Metallic elements in fossil fuel combustion products: amounts and form of emissions and evaluation of carcinogenicity and mutagenicity, Environ. Health Perspect., 1983, 47, 201–225, DOI:10.1289/ehp.8347201.
- F. J. Kelly and J. C. Fussell, Size, source and chemical composition as determinants of toxicity attributable to ambient particulate matter, Atmos. Environ., 2012, 60, 504–526, DOI:10.1016/j.atmosenv.2012.06.039.
- T. Fang, H. Guo, L. Zeng, V. Verma, A. Nenes and R. J. Weber, Highly acidic ambient particles, soluble metals, and oxidative potential: a link between sulfate and aerosol toxicity, Environ. Sci. Technol., 2017, 51(5), 2611–2620, DOI:10.1021/acs.est.6b06151.
- A. J. Ghio, Biological effects of utah valley ambient air particles in humans: a review, J. Aerosol Med., 2004, 17(2), 157–164, DOI:10.1089/0894268041457200.
- R. T. Burnett, J. Brook, T. Dann, C. Delocla, O. Philips, S. Cakmak, R. Vincent, M. S. Goldberg and D. Krewski, Association between particulate- and gas-phase components of urban air pollution and daily mortality in eight Canadian cities, Inhalation Toxicol., 2000, 12(sup4), 15–39, DOI:10.1080/08958370050164851.
- M. Lippmann, K. Ito, J. S. Hwang, P. Maciejczyk and L. C. Chen, Cardiovascular effects of nickel in ambient air, Environ. Health Perspect., 2006, 114(11), 1662–1669, DOI:10.1289/ehp.9150.
- B. Ostro, W. Y. Feng, R. Broadwin, S. Green and M. Lipsett, The effects
of components of fine particulate air pollution on mortality in California: results from calfine, Environ. Health Perspect., 2007, 115(1), 13–19, DOI:10.1289/ehp.9281.
- M. Franklin, P. Koutrakis and J. Schwartz, The role of particle composition on the association between PM2.5 and mortality, Epidemiology, 2008, 19(5), 680–689 CrossRef PubMed.
-
ATSDR, Toxicological Profiles, https://www.atsdr.cdc.gov/toxprofiledocs/index.html Search PubMed.
- L. Zhou, E. Kim, P. K. Hopke, C. O. Stanier and S. Pandis, Advanced factor analysis on pittsburgh particle size-distribution data, Aerosol Sci. Technol., 2004, 38(Suppl. 1), 118–132, DOI:10.1080/02786820390229589.
- J. Schwartz, D. W. Dockery and L. M. Neas, Is daily mortality associated specifically with fine particles?, J. Air Waste Manage. Assoc., 1996, 46(10), 927–939, DOI:10.1080/10473289.1996.10467528.
-
United States Environmental Protection Agency, EasyRSEI Dashboard, 2020 Search PubMed.
-
L. Younes, A. Shaw, and A. Kofman, How We Created the Most Detailed Map Ever of Cancer-Causing Industrial Air Pollution, ProPublica, 2021 Search PubMed.
-
C. Mele, Race and the Politics of Deception: The Making of an American City, NYU Press, New York, 2017 Search PubMed.
- S. Foster, Justice from the ground up: distributive inequities, grassroots resistance, and the transformative politics of the environmental justice movement, Calif. Law Rev., 1998, 86(4), 775, DOI:10.2307/3481140.
-
K. Cooper, ‘We Want to Live. They Want to Burn Trash’: Chester Residents Raise Environmental Racism Concerns over Incinerator with Covanta, WHYY, 2021 Search PubMed.
- S. E. Chambliss, C. P. R. Pinon, K. P. Messier, B. LaFranchi, C. R. Upperman, M. M. Lunden, A. L. Robinson, J. D. Marshall and J. S. Apte, Local- and regional-scale racial and ethnic disparities in air pollution determined by long-term mobile monitoring, Proc. Natl. Acad. Sci. U. S. A., 2021, 118(37) DOI:10.1073/pnas.2109249118.
- T. B. Onasch, A. Trimborn, E. C. Fortner, J. T. Jayne, G. L. Kok, L. R. Williams, P. Davidovits and D. R. Worsnop, Soot particle aerosol mass spectrometer: development, validation, and initial application, Aerosol Sci. Technol., 2012, 46(7), 804–817, DOI:10.1080/02786826.2012.663948.
- E. S. Cross, A. Sappok, E. C. Fortner, J. F. Hunter, J. T. Jayne, W. A. Brooks, T. B. Onasch, V. W. Wong, A. Trimborn, D. R. Worsnop and J. H. Kroll, Real-time measurements of engine-out trace elements: application of a novel soot particle aerosol mass spectrometer for emissions characterization, J. Eng. Gas Turbines Power, 2012, 134(7), 1–10, DOI:10.1115/1.4005992.
- S. Carbone, T. Onasch, S. Saarikoski, H. Timonen, K. Saarnio, D. Sueper, T. Rönkkö, L. Pirjola, A. Haÿrinen, D. Worsnop and R. Hillamo, Characterization of trace metals on soot aerosol particles with the SP-AMS: detection and quantification, Atmos. Meas. Tech., 2015, 8(11), 4803–4815, DOI:10.5194/amt-8-4803-2015.
- J. C. Corbin, A. A. Mensah, S. M. Pieber, J. Orasche, B. Michalke, M. Zanatta, H. Czech, D. Massabò, F. Buatier De Mongeot, C. Mennucci, I. El Haddad, N. K. Kumar, B. Stengel, Y. Huang, R. Zimmermann, A. S. H. Prévôt and M. Gysel, Trace metals in soot and PM2.5 from heavy-fuel-oil combustion in a marine engine, Environ. Sci. Technol., 2018, 52(11), 6714–6722, DOI:10.1021/acs.est.8b01764.
- L. H. Rivellini, M. G. Adam, N. Kasthuriarachchi and A. K. Y. Lee, Characterization of carbonaceous aerosols in Singapore: insight from black carbon fragments and trace metal ions detected by a soot particle aerosol mass spectrometer, Atmos. Chem. Phys., 2020, 20(10), 5977–5993, DOI:10.5194/acp-20-5977-2020.
- S. Carbone, H. J. Timonen, A. Rostedt, M. Happonen, T. Rönkkö, J. Keskinen, J. Ristimaki, H. Korpi, P. Artaxo, M. Canagaratna, D. Worsnop, F. Canonaco, A. S. H. Prévôt, R. Hillamo and S. Saarikoski, Distinguishing fuel and lubricating oil combustion products in diesel engine exhaust particles, Aerosol Sci. Technol., 2019, 53(5), 594–607, DOI:10.1080/02786826.2019.1584389.
- S. Saarikoski, H. Timonen, S. Carbone, H. Kuuluvainen, J. V. Niemi, A. Kousa, T. Rönkkö, D. Worsnop, R. Hillamo and L. Pirjola, Investigating the chemical species in submicron particles emitted by city buses, Aerosol Sci. Technol., 2017, 51(3), 317–329, DOI:10.1080/02786826.2016.1261992.
- J. Enroth, S. Saarikoski, J. Niemi, A. Kousa, I. Ježek, G. Močnik, S. Carbone, H. Kuuluvainen, T. Rönkkö, R. Hillamo and L. Pirjola, Chemical and physical characterization of traffic particles in four different highway environments in the helsinki metropolitan area, Atmos. Chem. Phys., 2016, 16(9), 5497–5512, DOI:10.5194/acp-16-5497-2016.
- H. Timonen, F. Mylläri, P. Simonen, M. Aurela, M. Maasikmets, M. Bloss, H. L. Kupri, K. Vainumäe, T. Lepistö, L. Salo, V. Niemelä, S. Seppälä, P. I. Jalava, E. Teinemaa, S. Saarikoski and T. Rönkkö, Household solid waste combustion with wood increases particulate trace metal and lung deposited surface area emissions, J. Environ. Manage., 2021, 293 DOI:10.1016/j.jenvman.2021.112793.
- S. Collier, L. R. Williams, T. B. Onasch, C. D. Cappa, X. Zhang, L. M. Russell, C. L. Chen, K. J. Sanchez, D. R. Worsnop and Q. Zhang, Influence of emissions and aqueous processing on particles containing black carbon in a polluted urban environment: insights from a soot particle-aerosol mass spectrometer, J. Geophys. Res. Atmos., 2018, 123(12), 6648–6666, DOI:10.1002/2017JD027851.
- P. T. Nilsson, A. C. Eriksson, L. Ludvigsson, M. E. Messing, E. Z. Nordin, A. Gudmundsson, B. O. Meuller, K. Deppert, E. C. Fortner, T. B. Onasch and J. H. Pagels,
In situ characterization of metal nanoparticles and their organic coatings using laser-vaporization aerosol mass spectrometry, Nano Res., 2015, 8(12), 3780–3795, DOI:10.1007/s12274-015-0877-9.
- P. F. DeCarlo, J. R. Kimmel, A. Trimborn, M. J. Northway, J. T. Jayne, A. C. Aiken, M. Gonin, K. Fuhrer, T. Horvath, K. S. Docherty, D. R. Worsnop and J. L. Jimenez, Field-deployable, high-resolution, time-of-flight aerosol mass spectrometer, Anal. Chem., 2006, 78(24), 8281–8289, DOI:10.1021/ac061249n.
- P. S. K. Liu, R. Deng, K. A. Smith, L. R. Williams, J. T. Jayne, M. R. Canagaratna, K. Moore, T. B. Onasch, D. R. Worsnop and T. Deshler, Transmission efficiency of an aerodynamic focusing lens system: comparison of model calculations and laboratory measurements for the aerodyne aerosol mass spectrometer, Aerosol Sci. Technol., 2007, 41(8), 721–733, DOI:10.1080/02786820701422278.
- I. M. Ulbrich, M. R. Canagaratna, Q. Zhang, D. R. Worsnop and J. L. Jimenez, Interpretation of organic components from positive matrix factorization of aerosol mass spectrometric data, Atmos. Chem. Phys., 2009, 9(9), 2891–2918, DOI:10.5194/acp-9-2891-2009.
- P. Paatero and U. Tapper, Positive matrix factorization: a non-negative factor model with optimal utilization of error estimates of data values, Environmetrics, 1994, 5(2), 111–126, DOI:10.1002/env.3170050203.
-
D. Carslaw, Worldmet: Import Surface Meteorological Data from NOAA Integrated Surface Database (ISD), 2021 Search PubMed.
- D. C. Carslaw and K. Ropkins, Openair – an R package for air quality data analysis, Environ. Model. Software, 2012, 27–28, 52–61, DOI:10.1016/j.envsoft.2011.09.008.
-
United States EPA, TSCA Screening Level Approach for Assessing Ambient Air and Water Exposures to Fenceline Communities, US EPA, 2022 Search PubMed.
-
United States Environmental Protection Agency, AirToxScreen Assessment Methods, https://www.epa.gov/AirToxScreen/airtoxscreen-assessment-methods Search PubMed.
- K. Sellegri, J. Gourdeau, J. P. Putaud and S. Despiau, Chemical composition of marine aerosol in a mediterranean coastal zone during the FETCH experiment, J. Geophys. Res. Atmos., 2001, 106(D11), 12023–12037, DOI:10.1029/2000JD900629.
- K. Hara, K. Osada, M. Yabuki and T. Yamanouchi, Seasonal variation of fractionated sea-salt particles on the antarctic coast, Geophys. Res. Lett., 2012, 39(17), 1–5, DOI:10.1029/2012GL052761.
- M. Crippa, I. El Haddad, J. G. Slowik, P. F. Decarlo, C. Mohr, M. F. Heringa, R. Chirico, N. Marchand, J. Sciare, U. Baltensperger and A. S. H. Prévôt, Identification of marine and continental aerosol sources in Paris using high resolution aerosol mass spectrometry, J. Geophys. Res. Atmos., 2013, 118(4), 1950–1963, DOI:10.1002/jgrd.50151.
- S. Gallavardin, U. Lohmann and D. Cziczo, Analysis and differentiation of mineral dust by single particle laser mass spectrometry, Int. J. Mass Spectrom., 2008, 274(1–3), 56–63, DOI:10.1016/j.ijms.2008.04.031.
- W. P. Linak and J. O. L. Wendt, Toxic metal emissions from incineration: mechanisms and control, Prog. Energy Combust. Sci., 1993, 19(2), 145–185, DOI:10.1016/0360-1285(93)90014-6.
- M. Kasahara, K. C. Choi and K. Takahashi, Source contribution of atmospheric aerosols in Japan by chemical mass balance method, Atmos. Environ., Part A, 1990, 24(3), 457–466, DOI:10.1016/0960-1686(90)90002-5.
- S. L. Goddard, K. R. Williams, C. Robins and R. J. C. Brown, Determination of antimony and barium in UK air quality samples as indicators of non-exhaust traffic emissions, Environ. Monit. Assess., 2019, 191(11) DOI:10.1007/s10661-019-7774-8.
- R. O. Gonzalez, S. Strekopytov, F. Amato, X. Querol, C. Reche and D. Weiss, New insights from zinc and copper isotopic compositions into the sources of atmospheric particulate matter from two major European cities, Environ. Sci. Technol., 2016, 50(18), 9816–9824, DOI:10.1021/acs.est.6b00863.
- H. Hagino, M. Oyama and S. Sasaki, Laboratory testing of airborne brake wear particle emissions using a dynamometer system under urban city driving cycles, Atmos. Environ., 2016, 131, 269–278, DOI:10.1016/j.atmosenv.2016.02.014.
- X. Li, L. Wang, Y. Wang, T. Wen, Y. Yang, Y. Zhao and Y. Wang, Chemical composition and size distribution of airborne particulate matters in Beijing during the 2008 olympics, Atmos. Environ., 2012, 50, 278–286, DOI:10.1016/j.atmosenv.2011.12.021.
- J. W. Xu, R. V. Martin, B. H. Henderson, J. Meng, Y. B. Öztaner, J. L. Hand, A. Hakami, M. Strum and S. B. Phillips, Simulation of airborne trace metals in fine particulate matter over North America, Atmos. Environ., 2019, 214, 116883, DOI:10.1016/j.atmosenv.2019.116883.
- S. Mbengue, L. Y. Alleman and P. Flament, Size-distributed metallic elements in submicronic and ultrafine atmospheric particles from urban and industrial areas in Northern France, Atmos. Res., 2014, 135–136, 35–47, DOI:10.1016/j.atmosres.2013.08.010.
- W. Birmili, A. G. Allen, F. Bary and R. M. Harrison, Trace metal concentrations and water solubility in size-fractionated atmospheric particles and influence of road traffic, Environ. Sci. Technol., 2006, 40(4), 1144–1153, DOI:10.1021/es0486925.
- M. Rojas-Lemus, N. López-Valdez, P. Bizarro-Nevares, A. González-Villalva, M. Ustarroz-Cano, A. Zepeda-Rodríguez, F. Pasos-Nájera, I. García-Peláez, N. Rivera-Fernández and T. I. Fortoul, Toxic effects of inhaled vanadium attached to particulate matter: a literature review, Int. J. Environ. Res. Public Health, 2021, 18(16) DOI:10.3390/ijerph18168457.
- E. Beceiro-González, E. González-Soto, P. López-Mahía and D. Prada-Rodríguez, Total arsenic and selenium levels in atmospheric particulate matter of La Coruna (Spain), Sci. Total Environ., 1997, 208(3), 207–211, DOI:10.1016/S0048-9697(97)00296-9.
- T. Berg, O. Røyset and E. Steinnes, Blank values of trace elements in aerosol filters determined by ICP-MS, Atmos. Environ., Part A, 1993, 27(15), 2435–2439, DOI:10.1016/0960-1686(93)90411-Q.
-
Agency for Toxic Substances and Disease Registry, Interaction Profiles for Toxic Substances, https://www.atsdr.cdc.gov/interactionprofiles/index.html, accessed March, 2023 Search PubMed.
- J. R. Varshavsky, S. D. G. Rayasam, J. B. Sass, D. A. Axelrad, C. F. Cranor, D. Hattis, R. Hauser, P. D. Koman, E. C. Marquez, R. Morello-Frosch, C. Oksas, S. Patton, J. F. Robinson, S. Sathyanarayana, P. M. Shepard and T. J. Woodruff, Current practice and recommendations for advancing how human variability and susceptibility are considered in chemical risk assessment, Environ. Health, 2023, 21(133) DOI:10.1186/s12940-022-00940-1.
|
This journal is © The Royal Society of Chemistry 2023 |
Click here to see how this site uses Cookies. View our privacy policy here.