Long-term changes in precipitable water vapour over India derived from satellite and reanalysis data for the past four decades (1980–2020)†
Received
24th October 2022
, Accepted 6th February 2023
First published on 7th February 2023
Abstract
India has a tropical monsoon climate with significant regional variability in rainfall and temperature, where precipitation is closely connected to precipitable water vapour (PWV). Here, the satellite and reanalysis data are considered to study the spatial and temporal changes of PWV over India in 1980–2020. We have also analysed its potential drivers such as precipitation, surface temperature and evapotranspiration during the same period. The distribution of annual PWV depicts the highest values over the east coast (40–50 mm) and lowest in Western Himalaya (<10 mm). The seasonal distribution shows highest PWV during monsoon (June–July–August–September, about 40–65 mm). Similarly, the monthly cycle of PWV shows the lowest amount in January, which gradually increases with time until it peaks in July, and then decreases thereafter. Inter-annual changes show a peak in 1997–1998, which can be attributed to the strong El Niño event (Nino 3.4 region), during which the increase in temperature leads to more evapotranspiration and thus, enhanced PWV. Among the sources and sinks, evapotranspiration (0.6–0.9), precipitation (0.7–0.9) and surface temperature (0.5–0.6) are highly correlated with PWV across the regions in India. The PWV trends in India are found to be significantly positive (0.6–0.9 mm per year), which can be linked to the recent increase in surface temperature and thus, the increase in atmospheric moisture. This is a concern for regional climate change as PWV is directly connected to water vapour and, thus, to temperature and climate.
Environmental significance
Precipitable water vapour (PWV) is considered as one of the most important constituents of the atmosphere that can influence regional climate and weather processes. The PWV variability over Indian regions is very diverse due to its different geographical conditions. Here, we examine the long-term changes in PWV and its associated drivers to understand regional climate processes, which have not been discussed before. The increase in atmospheric temperature due to enhanced anthropogenic greenhouse emissions causes more evapotranspiration that leads to a high amount of PWV, which further influences precipitation and the regional climate.
|
1. Introduction
Precipitable water vapour (PWV) is the amount of water that would result from condensing a column of water vapour, which extends from the surface to the top of the atmosphere. It is considered as one of the most important constituents of the atmosphere that can influence regional climate and weather processes.1–4 Most of the water vapour is present in the lower troposphere that contributes to PWV, and its amount is generally controlled by surface temperature.5–7 It also plays an important role in precipitation processes, the hydrological cycle and weather events in the lower part of the atmosphere.5,8–10 In addition, changes in PWV are strongly connected to the global radiation budget, which can influence the structure of atmospheric temperature and characteristics of droughts and rainfall.11,12
As per the IPCC (2021)13 report, the Earth's temperature has been steadily increasing at the local, regional and global scales since 1950. Various studies have been conducted to quantify the PWV changes in different regions across the latitudes. For instance, Ross and Elliott (2001)14 found an increasing trend in PWV over the Northern Hemisphere during 1973–1995, except for Europe, and about 3% dec−1 over the islands located in the western tropical Pacific, East China and North America. Trenberth et al. (2005)9 used Special Sensor Microwave Imager (SSM/I) data over the oceans from 1988 to 2003 and estimated a positive trend in PWV of about 1.3 ± 0.3% dec−1. Mieruch et al. (2008)15 examined PWV on a global scale using data from the Scanning Imaging Absorption Spectrometer for Atmospheric Chartography (SCHIMACHY) and Global Ozone Monitoring Experiment (GOME) for the period 1996–2006. Their findings revealed negative trends in PWV over Central America, the northwest United States, the Amazon, central Africa and the Arabian Peninsula, but increasing trends in East Europe, Siberia, Oceania and Greenland. Bengtsson (2004)16 showed that the global PWV increased at a rate of 0.36 mm dec−1, using the ERA-40 reanalysis for the 1979–2001 period. Furthermore, Chen and Liu (2016)17 reported that global PWV has been increasing significantly at a rate of 1.31
±
0.47% dec−1 during the period 1992–2014. A similar increase in global PWV (about 1% dec−1) is also observed by Allen et al. (2022)18 for the period 1988–2014.
In the Indian subcontinent, a first-time PWV study was conducted in 2004 based on the global positioning system (GPS) and Moderate Resolution Imaging Spectroradiometer (MODIS) satellite measurements.19 Due to different geographical and climatic conditions, the PWV variability across Indian regions is very diverse. For example, Jade et al. (2019)19 showed that the interannual variation of PWV is about 9–22% for southern India, 9–19% for Himalaya and about 3–10% for northeast India. Mishra (2019)20 showed a positive trend of about 1.69% dec−1 in PWV for the period 1980–2018 in India. Similarly, Jindal et al. (2020)21 found positive trends in PWV at different locations in India (about 1.663% per year and 0.580% per year over Hyderabad and Delhi, respectively) during the period 2003–2011. Patel and Kuttippurath (2022)22 found that the water vapour is increasing significantly over India in recent decades, with values of 0.1–0.2 kg per m2 per year. Therefore, a dedicated study on the long-term changes in PWV and associated drivers is needed for India to understand regional climate change. We analyse the PWV distribution over India using satellite and reanalysis data. We also examine the correlation of PWV with its key drivers such as evapotranspiration, precipitation and surface temperature for the period 1980–2020.
2. Data and methods
The PWV distribution over India shows very high spatial and temporal variability. As a result, it is important to consider various measurements to understand the distribution of PWV across the regions. These data include ground-based radiosonde and GPS measurements, microwave and infrared satellite observations, lidars, and reanalysis data such as Modern-Era Retrospective Analysis for Research and Applications version 2 (MERRA-2) and the European Centre for medium range weather forecast reanalysis (ERA5). Here, we use satellite (MODIS), reanalysis (ERA5 and MERRA-2), ground-based (IMD) and model data (TerraClimate) to investigate long-term changes in PWV over India. In addition, the changes in PWV are also investigated over homogeneous temperature regions of India (source IITM Pune: https://www.tropmet.res.in). The regions are West Coast (WC), East Coast (EC), Western Himalaya (WH), Interior Peninsular (IP), Northeast (NE), North Central (NC) and Northwest (NW), as shown in Fig. 1. The homogeneous regions are defined based on the spatial and temporal distribution of mean, maximum and minimum surface air temperatures across the country. In addition, climate and geographical conditions are also considered for the delineation of homogeneous regions, e.g. the tropical climate (EC, WC and IP), humid subtropical (NC), arid and semiarid (NW), cold (WH) and high rainfall regions such as NE.23
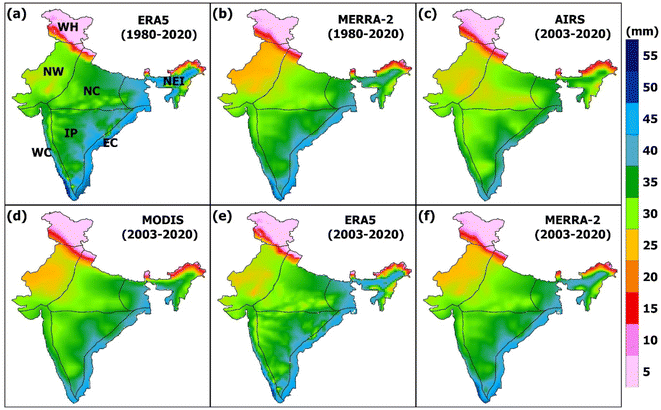 |
| Fig. 1 The climatology of PWV for India derived from (a) ERA5 (1980–2020), (b) MERRA-2 (1980–2020), (c) AIRS (2003–2020), (d) MODIS (2003–2020), (e) ERA5 (2003–2020) and (f) MERRA-2 (2003–2020). Different temperature homogeneous regions of India such as WC (West Coast), EC (East Coast), IP (Interior Peninsular), NE (North East), NC (North Central), NW (North West) and WH (Western Himalaya) are also marked. | |
2.1 PWV from MODIS, ERA5, MERRA-2 and AIRS
The MODIS is an instrument on the earth observation satellites (EOSs) Terra and Aqua. Terra's orbit around the Earth crosses the equator from north to south in the morning, while Aqua crosses the equator from south to north in the afternoon. The Terra MODIS and Aqua MODIS scan the entire Earth’s surface and collect data in 36 spectral bands every two days. PWV is derived using an algorithm based on the observations of water vapour attenuation (near-IR solar radiation) reflected by the Earth’s surface.24,25 PWV is determined using the transmittance after extracting variation in surface reflection by using a ratio of two channels of atmospheric windows (0.865 and 1.24 m) and three water absorption channels (0.905, 0.936 and 0.940 m). Finally, PWV is calculated from the transmittance using a lookup table and radiative transfer calculations. As per Gao and Kaufman (2003),25 the MODIS PWV retrieved using the above-mentioned algorithm has an error of 5–10%. Here, MODIS level 3 PWV (1° × 1°) data are used for the period from 2003 to 2020.
The MODIS Terra PWV data for Indian regions are validated using three ground-based GPS measurements located in Bangalore, Hyderabad and Kanpur.26 According to the study, MODIS PWV has a higher correlation with the GPS (91%) at these stations, with a systematic bias during wet and dry months. Kumar et al. (2013)27 showed that MODIS PWV has a correlation coefficient of about 0.98 with the GPS data in Varanasi and Kanpur for the period 2007–2008. Joshi et al. (2013)28 conducted a similar study with the Almora GPS station data in the Central Himalaya (altitude of 1259 msl) for the period 2009–2011 and found a correlation of 91% with annual and 62–87% with seasonal data. Furthermore, the bias in PWV during these periods was 4.53 mm, with a root mean square error (RMSE) of 4.06, but higher values were estimated for the monsoon season. In another study, it is observed that the MODIS underestimates the PWV measurements made in summer at a high altitude (4325 m) trans-Himalaya GPS station due to dry terrain there.29
The PWV data of ERA5 are made with microwave and infrared sensors such as the Advanced Microwave Scanning Radiometer, global precipitation measurements, microwave imagers, SSM/I and several other water vapour observations. The ERA5 data are available from January 1979 to date. In CY41R2 of the ECMWF Integrated Forecast System, 4D-Variational data assimilation and model forecasts are used for the assimilation of ERA5 data, with 137 hybrid sigma-pressure levels in the vertical and the top level at 0.01 hPa. The ERA5 reanalysis incorporates water vapour from high-quality observations such as the most recent multi-satellite sounders, imagers and ground-based data.30 Here, PWV data from ERA5 are used for the period 1980–2020.
Kannemadugu et al. (2022)31 used ERA5 and 18 GPS station data to analyse PWV over India for the period from March 2013 to February 2014. They concluded that ERA5 PWV is consistent with GPS measurements, with a correlation coefficient ranging from 0.93 to 0.99, indicating that ERA5 can accurately capture the observed PWV variability. They also found that the bias between ERA5 and GPS PWV was in the range of 0.24–0.49 cm. Also, the ERA5 data overestimate GPS PWV at most stations. A recent study by Wang et al. (2020)32 evaluated the PWV from five reanalysis datasets with ground-based observations. They found that PWV derived from ERA5 has higher accuracy, with a RMSE of 1.84 mm. The latest atmospheric reanalysis data produced by the Global Modeling and Assimilation Office (GMAO) for the modern satellite era is MERRA-2. The reanalysis incorporates observation types that were not previously considered for MERRA and has additional updates to the Goddard Earth Observing System (GEOS) model.33,34 Unlike MERRA, all MERRA-2 data are computed at the same latitude and longitude resolution of 0.625° × 0.5°. The MERRA-2 PWV data are based on many additional satellites, new microwave sounders and hyperspectral infrared instruments such as the Atmospheric Infrared Sounder,35 SSM/I and TIROS Operational Vertical Sounder. It also assimilates reprocessed versions of some of the satellite observations used in MERRA. We use the MERRA-2 PWV data for the period 1980–2020.
In addition, PWV from the Atmospheric Infrared Sounder (AIRS) is also analysed for the period 2003–2020. It is a hyperspectral infrared sounder on board the Aqua satellite, which was launched in 2002. It uses infrared channels for water vapour observations.36 It is sensitive up to 260 hPa in the latitude range 40° S–40° N and has 2378 channels with a spectral resolution of k/dk = 1200, where k represents the wavelength. It is widely used in global water vapour studies.22,37
2.2 Precipitation, temperature and evapotranspiration data
The IMD data are taken to analyse the potential drivers and sinks of PWV such as surface air temperature and precipitation. The correlation between PWV and drivers is estimated to understand their inter-relationship. The IMD currently operates approximately 550 surface observatories across the country, from which the daily surface temperature and precipitation measurements are collected.38,39 The IMD National Data Centre compiles, digitizes, performs quality control analysis and archives this information. The IMD daily gridded temperature and precipitation data are available at a spatial resolution of 1° × 1° and 0.25° × 0.25°, respectively, for the period 1951–2020. Here, the correlation analysis is performed between the aforementioned parameters and PWV for the 1980–2020 period. In addition, precipitation data from the global precipitation climatology project (GPCP) are also analysed for the period 1980–2020. Evapotranspiration is one of the major factors that affect the distribution and trend of PWV over land regions. Here, the monthly mean evapotranspiration data are considered from TerraClimate, which was calculated using the Penman Montieth methods.40 To compute the correlation with PWV, the monthly mean evapotranspiration data at 4 km spatial resolution are used from 1980 to 2020.
2.3 Computation of mean bias error and root mean square error
MODIS PWV values are considered to assess the accuracy of two reanalysis datasets, ERA5 and MERRA-2. In this case, statistical metric RMSE and mean bias error (MBE) are employed to evaluate the reanalysis data. The following formulae are used:
where PWVRi and PWVMi are reanalysis and MODIS measurements, respectively, and N is the number of observations.
2.4 Comparison of ERA5 and MERRA-2 PWV with AIRS and MODIS
Both AIRS and MODIS can measure the PWV with high accuracy of several millimetres, except during monsoon seasons when cloud cover prevents AIRS and MODIS from capturing the complete column of water vapour. As a result, a comparison is conducted to evaluate the PWV obtained from ERA5 and MERRA-2 using the AIRS and MODIS observations over India for the common period of 2001–2020. Fig. S1† shows the MBE and RMSE between ERA5/MERRA-2 derived and AIRS/MODIS PWV. MERRA-2 with respect to MODIS data shows a smaller MBE of 1–2 mm, compared to 1–4 mm with ERA 5 in NC India, and the corresponding RMSE is also lower in MERRA-2 (2 mm) than that in ERA5 (4 mm). Furthermore, both data show high values of MBE (5–7 mm, in the upper regions of NEI) and RMSE (8–10 mm) in NEI. These higher values of MBE and RMSE indicate the underestimation of MODIS PWV. This can be attributed to the cloud cover during the monsoon season in India. Similar MBE and RMSE are also found in EC, WC and NW India. In sum, both reanalyses show similar distributions of MBE and RMSE in India, but the biases are slightly higher in ERA5. Similar distributions of MBE and RMSE are also observed with AIRS data (e.g. a MBE of about 2–4 mm in NC, IP and WC), but values are slightly higher than that with MODIS. We have also computed the correlation coefficient of ERA5 with AIRS and MODIS (Fig. S2†), and observed that ERA5 data show positive relation with both satellite datasets, with comparatively higher values in ERA5 and AIRS. As ERA5 and MERRA-2 show high positive correlation with satellite measurements and are available for a longer period, we have computed the trend using these reanalysis data.
2.5 Estimation of trends in PWV and other data
The annual and seasonal distribution and trends of PWV are analysed using the monthly averaged data from ERA5 and MERRA-2 over the period 1980–2020. Here, the trends in PWV are calculated for the period 1980–2020 using linear regression and its statistical significance is computed at the 95% confidence interval (CI). Similarly, the trends in other variables such as temperature, precipitation and evapotranspiration are calculated using the same methodology. The seasons are defined as winter (January–February; JF), pre-monsoon (March–April–May; MAM), monsoon (June–July–August–September; JJAS) and post-monsoon (October–November–December; OND). To study the connection of PWV with its drivers, an analysis is carried out for the period 1980–2020 using Pearson's correlation.
3. Results and discussion
3.1 Climatology of PWV
The climatology of PWV over India derived from the 40 year ERA5 and MERRA-2 reanalysis data are shown in Fig. 1a and b. The distribution of PWV throughout India shows clear geographical differences. For instance, high PWV values are found in the East Coast (EC), southern West Coast (WC) and in the North East (NE) (ranging from 40 to 50 mm) as analysed from the ERA5 data. The high PWV in the coastal areas are related to the water vapour transport from the Bay of Bengal and Arabian Sea. Low PWV (<10 mm) is observed in the high-altitude Western Himalaya (WH) and sub-Himalaya areas. In MERRA-2, similar PWV distributions are found in EC and WC with comparable values as found in ERA5. We have also analysed the annual mean climatology of PWV for the period 2003–2020, which is the common data period for AIRS, MODIS, ERA5 and MERRA-2, and is shown in Fig. 1c–f. The PWV from reanalysis data overestimates that of MODIS and AIRS, about 5 mm in both coastal regions. The NE regions show higher PWV in the reanalyses than in the satellite data. The PWV distribution in NW, NC and WH are similar in all four datasets. Our findings are coherent with that of Barman et al. (2017),41 where they find low values of PWV in the hilly and very high values in the coastal and NE regions. Chakraborty et al. (2019)42 also found heterogeneity in the distributions of PWV across India, with high values in the coastal and NE regions, and low values in NW arid and Himalayan regions. To understand the variability in PWV over India, we have also computed its annual mean standard deviation (SD) using all four datasets considered (Fig. S3†). It is observed that variability in PWV is very small in the hilly and high in WC regions, as revealed by all four datasets. MODIS shows slightly higher values of SD over India, particularly in EC, WC, NC and IP. The variability in the annual mean PWV can be attributed to the seasonal cycle of PWV. During summer, the south-west monsoon brings a significant amount of moisture, which results in wet conditions across India.
3.2 PWV variability
3.2.1 Monthly and seasonal variability.
The monthly mean distribution of PWV throughout the regions derived from all four datasets is shown in Fig. 2. The ERA5 data reveal the highest PWV in June–September (>50 mm) and lowest (<30 mm) in December–February. The highest PWV of 55–58 mm is found over NC and NE in June–August, but very high values are found over EC in all months. During the winter months (December–January) small PWV values are observed across the regions, with very low values in WH, about 5 mm. This is due to the comparatively smaller temperature and lower surface water availability (e.g., sources of evapotranspiration) there in that period. A similar inference can be made for WH, where dry conditions are observed because of lower temperatures throughout the year. A similar monthly cycle is also observed in the other three datasets across all regions. For instance, MERRA-2 shows high values in monsoon months and smaller values in the winter months of December–February in all regions. PWV derived from both reanalyses is consistent with MODIS observations, except in WH, where MODIS underestimates the other three datasets. It can be due to the high-altitude, where MODIS is unable to capture the entire column of PWV. On the other hand, AIRS underestimates the other three datasets in all regions, except in WH during June–September, owing to the cloud cover, where it fails to capture the entire column of water vapour.37
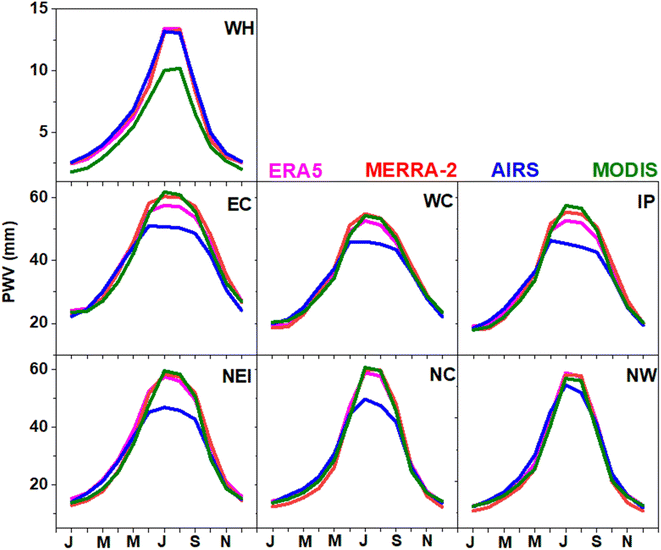 |
| Fig. 2 Monthly distribution of PWV derived from the ERA5 (1980–2020), MERRA-2 (1980–2020), AIRS (2001–2020) and MODIS (2003–2020) data in the temperature homogeneous regions of India. The x-axis entries are months and the first letters of alternate months are shown (e.g., J for January and N for November). | |
There is also seasonal variability in PWV distribution in India, as shown in Fig. 3. A clear seasonal cycle is observed in PWV in all four datasets, with high values in monsoon and low values in winter. There is a gradual increase of PWV from 5–15 mm in winter to 50–65 mm in monsoon, except in WH (where values are <10 mm), as revealed by ERA5. Small PWV in winter is primarily due to the low temperature and relatively dry atmosphere during the period. In pre-monsoon, PWV is slightly higher than that in winter in all regions, due to the evapotranspiration driven by enhanced thermal heating.43 The highest values are observed in monsoon, as mentioned above, due to the transport of moisture from nearby oceans by the winds (see wind vector in Fig. 3). The transport of moisture during monsoon is acting as a source of precipitation there. On the other hand, it is observed that transport of moisture in other seasons is not as prominent as it is in monsoon. Similarly, in post-monsoon, relatively high values are found in EC (30–45 mm) and WC (20–40 mm) as compared to those in other regions. In brief, the changes in PWV are primarily due to the regional evapotranspiration driven by surface air temperature, except in the monsoon season.10,22 MERRA-2 data also show a similar seasonal distribution across the regions, except in NW, where they show slightly lower values of PWV than that in ERA5 during pre-monsoon. In addition, the reanalysis PWV values are consistent with the satellite measurements across all regions and seasons (except monsoon in AIRS), albeit with differences in their values due to different time periods.
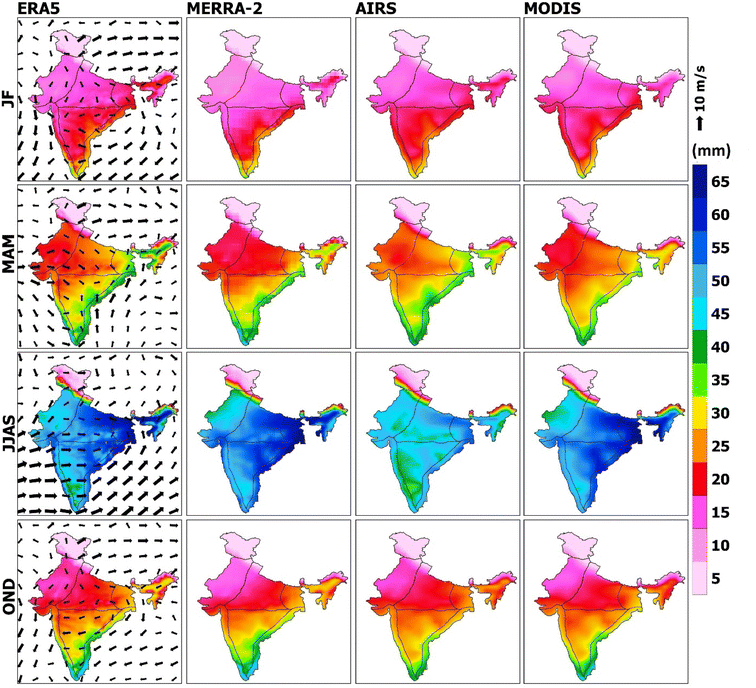 |
| Fig. 3 Distribution of PWV in different seasons as analysed from the ERA5 (1980–2020), MERRA-2 (1980–2020), AIRS (2003–2020) and MODIS (2001–2020) data in India. Wind vectors are overlaid for the respective seasons. | |
3.2.2 Interannual variability.
The interannual variability of seasonal mean PWV over different regions as found in the ERA5 and MERRA-2 data is depicted in Fig. 4. PWV is gradually increasing during the monsoon season in all regions. Despite the strong El Niño event in 1997–1998, a sharp peak is observed in PWV owing to the positive monsoon anomaly.44,45 Slingo and Annamalai (2000)46 also found that precipitation in India was almost 2% higher than its average during this period. On the other hand, it can also be attributed to the increase in temperature due to El Niño, which increases evapotranspiration and thus, the higher PWV there. We also analysed the temporal evolution of annual averaged precipitation over India using GPCP data and found an increase in precipitation during the years 1997–1998 (Fig. S4†).
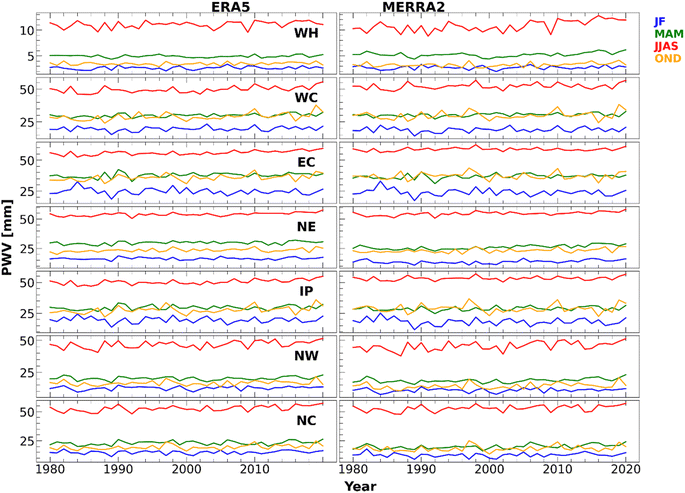 |
| Fig. 4 Temporal evolution of PWV over India in different seasons over the temperature homogeneous regions as analysed from the ERA5 and MERRA-2 data for the period 1980–2020. | |
Similarly, a drop in PWV is observed in WH, NW and NC during monsoon in 2009. Conversely, high variability is observed in the coastal regions (EC and WC) and IP during winter. The coastal regions and IP also show very high variability in post-monsoon. Among the regions, seasonal variability is relatively small in NE. MERRA-2 also shows an analogous interannual variability as observed in ERA5. For instance, the drop in WH, NW and NC during monsoon in 2009 and the relatively small interannual change in NE are also replicated in the ERA5 data.
Fig. S5† illustrates the interannual variability of the annual average PWV over India derived from all four datasets. All datasets show similar change with time in all regions, except in WH. PWV is gradually increasing in all regions, particularly from 2000 onwards. Changes in PWV over WH since 2000 are much higher than those in other regions, as observed in MERRA-2 data. In contrast, MODIS data show slightly lower PWV values, with a gradual decrease in WH. Similarly, both satellite measurements show lower values than that of reanalysis in NE, although a gradual increase has been observed from 2000 to date. It can be attributed to the mountainous topography of NE, where the temperature differences are higher due to the shadow of the mountains. There is a drop in PWV during 1991–1992, which is consistent with the decline in surface temperature owing to the Mount Pinatubo volcanic eruption in that period.47 Similarly, there is an increase in PWV in all four datasets in year 2010 over WH, EC and IP. This can be due to the La Niña event that must have brought moisture to the Indian land regions.
3.3 Drivers of PWV
Time evolution of drivers of PWV over India in different seasons from 1980 to 2020 is depicted in Fig. S6.† Here, we have taken the potential sources, drivers and sinks such as evapotranspiration, surface temperature and precipitation. In general, precipitation over India is highest (6–8 mm) during the monsoon season, and the PWV values are correspondingly higher (35–40 mm) with respect to the rainfall in each season. As the surface temperature increases, the evapotranspiration also increases, which makes more PWV in the atmosphere.
The correlation analysis for these variables with PWV in different seasons for the period 1980–2020 is shown in Fig. 5. It is observed that the PWV and evapotranspiration are positively correlated in most regions during all seasons, but they are negatively correlated (−0.6 to −0.9) in most parts of NE, EC and WC regions. Furthermore, PWV and precipitation are positively correlated in all regions across the seasons. In the case of PWV and surface temperature, we observe noticeable changes in correlation over different regions. Also, both are positively correlated in the hilly regions and some areas of WC in winter. However, during pre-monsoon, a negative correlation is found over most regions, except in the hilly areas and NEI; indicating the dry atmosphere there. Similarly, a negative correlation is observed during the monsoon season, which could be due to the moisture transport by the winds from nearby oceans during this season. A strong positive correlation with temperature is found in WC, NE and WH during monsoon. Likewise, a positive correlation with temperature is found in the northern NE in post-monsoon due to the enhanced evapotranspiration with the increase in temperature. The NE region has vast vegetation cover and high amounts of surface water and precipitation, which can lead to high evapotranspiration there. Similarly, in WH, the temperature increase can make more moisture in the atmosphere through evaporation of snow or ice.
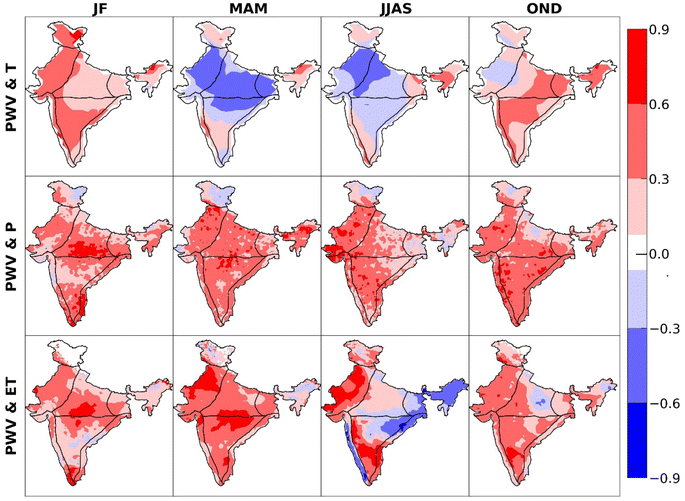 |
| Fig. 5 Correlation between PWV and surface temperature (T, top), precipitation (P, middle) and evapotranspiration (ET, bottom) in India derived from the ERA5, IMD and TerraClimate data for the period 1980–2020. | |
3.4 Trends in PWV
Trends in PWV computed using the ERA5 data are shown in Fig. 6 for the period 1980–2020. The hatched regions represent the statistical significance of the estimated trends at 95% CI. The trend in annual PWV is positive throughout the regions (up to 0.09 mm per year) in the ERA5 data. A positive trend of about 0.03 mm per year is found in WH. The trends derived for each season are also shown in Fig. 6. In winter, statistically insignificant negative trends with values ranging from −0.06 to −0.01 mm per year are found in IP and EC. In pre-monsoon, regions of NE, EC and WC show significant positive trends. The southern part of both coastal regions shows significant positive trends, about 0.09–0.15 mm per year. Also, significant positive trends are observed in most of India in the monsoon season. The western NC and the southern NW have higher trends, around 0.13 mm per year in this season. In post-monsoon, significant positive trends are observed over the entire India, except for WH, northern NW and NE, with values of about 0.09–0.12 mm per year. Our findings are consistent with that of Patel and Kuttippurath (2022)22 and Mishra (2020),20 where they reported that water vapour is significantly increasing over India, with high values in monsoon. Furthermore, Chakraborty et al. (2019)42 also found that PWV is increasing across India during monsoon and pre-monsoon seasons.
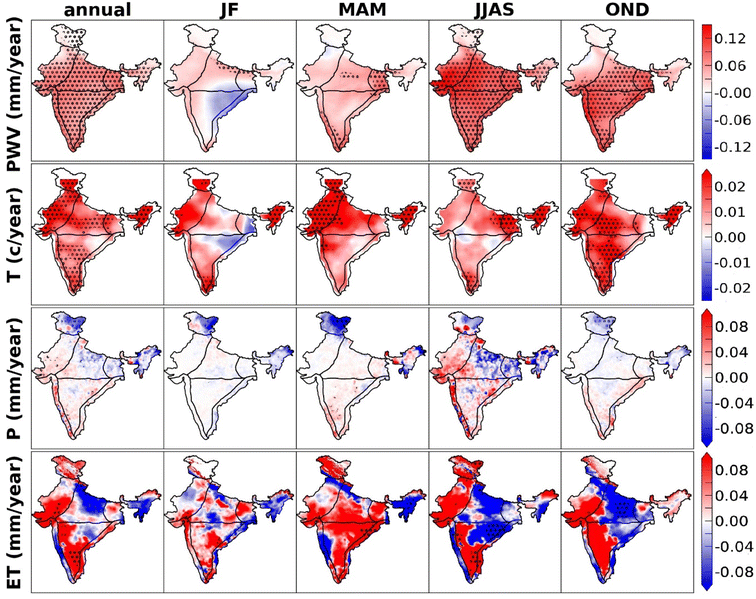 |
| Fig. 6 The annual and seasonal trends in PWV (ERA5), surface temperature (IMD), precipitation (IMD) and evapotranspiration (TerraClimate) for the period 1980–2020. The hatched regions represent the statistically significant trends at 95% CI. | |
Surface temperature is one of the key drivers of PWV. Trends in surface temperature along with PWV, evapotranspiration and precipitation are presented in Fig. 6. A significant positive trend in the annual mean temperature is found throughout India (0.01–0.04 °C per year), except for regions near EC. Due to enhanced greenhouse emissions, surface temperature is increasing at a faster rate.13 As a result, more evapotranspiration would make enhanced PWV in the atmosphere. During winter, an insignificant trend is estimated in PWV and temperature, except for NE and IP. Despite the increase in temperature (0.04–0.05 °C per year), the trend is very small in PWV in NW during pre-monsoon, as it is a dry desert region. There is a significant increase in annual mean evapotranspiration, with values higher than 0.06 mm per year, except in some regions in WC, NC and NEI with negative trends.
In general, pre-monsoon is associated with dry conditions in India where comparatively less moisture is observed in the atmosphere. In monsoon, temperature is relatively lower than that in pre-monsoon. The positive trend in PWV in this season is mainly due to higher evapotranspiration,43 where surface water is available through precipitation. We also observe that evapotranspiration is increasing in PI, WH and NWI, with values > 0.08 mm per year. However, there is a negative trend in evapotranspiration during monsoon in WC and NW. Despite a negative trend in evapotranspiration during monsoon, PWV is increasing there. This could be attributed to the moisture transport by southwest monsoon winds from nearby oceans to the land regions. During post-monsoon a positive trend is observed in temperature over IP (0.01–0.02 °C per year), NW (0.01–0.02 °C per year) and NE (0.01–0.03 °C per year), consistent with the PWV trends there. Similarly, the annual precipitation in India is also increasing in some regions (0.01–0.06 mm per year),48,49 suggesting more PWV in the atmosphere. Since all data and reanalysis have limitations, the analysis presented in this work is also not free from those uncertainties. Therefore, computed statistical uncertainties are to be taken care of when interpreting trend estimates.
4. Conclusions
Our study reveals that the annual mean PWV in India is generally characterised by three high PWV regions: EC adjacent to the Bay of Bengal, southern WC near the Arabian Sea (40–50 mm) and the highly vegetated NE India. Low PWV regions are located in WH, with values less than 5 mm. PWV is highest in monsoon, followed by post-monsoon and pre-monsoon, and lowest in winter. In post-monsoon, the southern EC shows higher values which could be attributed to the northeast monsoon winds with substantial moisture from the Bay of Bengal. However, the moisture is transported from the Indian Ocean to the Indian land regions during south west monsoon, whereas the dry airflow from the Arabian Desert results in low atmospheric moisture over India, except in southern EC during the northeast monsoon. Furthermore, the southwest monsoon brings plenty of moisture from the Bay of Bengal to PI. The annual PWV trends are significantly positive in all regions over the last four decades. However, a higher PWV trend (0.3–1.5 mm per year) is found during monsoon throughout the regions, except in WH. This higher trend is due to the increasing temperature during the period, which led to more water vapour in the atmosphere through evapotranspiration. The annual mean surface temperature is also increasing significantly over India with values ranging from 0.01 to 0.02 °C per year. Similarly, both temperature and PWV show increasing trends in post-monsoon. In accordance with PWV and temperature distributions in India, there is also an increasing trend in the annual precipitation. Furthermore, the monsoon season has the highest positive trend of precipitation of all seasons. Therefore, the increase in surface temperature due to global warming and climate change make more water vapour in the atmosphere. As PWV has an important role in Earth's climate system, our findings have wider implications for regional weather processes and climate change. This study also provides insight on future studies that would critically examine the importance of PWV in precipitation processes, development of Earth's system models and regional warming.
Data availability
MERRA 2 data are available at: https://disc.gsfc.nasa.gov/, ERA5 data are available at: https://cds.climate.copernicus.eu/#!/search?text=ERA5%26type=dataset, MODIS data are available at: https://giovanni.gsfc.nasa.gov/giovanni/, IMD data are available at: https://www.imdpune.gov.in/ClimPredLRFNew/GridedDataDownload.html, and TerraClimate data for evapotranspiration are available at: https://www.climatologylab.org/terraclimate.html.
Author contributions
SS: methodology, data analysis, visualization, writing – original draft. VKP: methodology, data analysis, visualization, review & editing of the original draft. JK: conceptualization, methodology, supervision, visualization, review & editing of the original draft.
Conflicts of interest
The authors declare no conflict of interest associated with this study.
Acknowledgements
We thank the Director, Indian Institute of Technology Kharagpur (IIT Kgp), the Chairman CORAL IIT Kgp and the Ministry of Education (MoE) for facilitating the study. SS and VKP acknowledge the support from MoE and IIT KGP. We thank GES-DISC and GIOVANNI for MERRA-2 and MODIS data. We also acknowledge the Copernicus Atmospheric Data Store and National centers for environmental information for ERA5 reanalysis and radiosonde data, respectively. We also thank the Climatology Lab for TerraClimate data.
References
- J. T. Kiehl and K. E. Trenberth, Earth's annual global mean energy budget, Bull. Am. Meteorol. Soc., 1997, 78, 197–208 CrossRef.
- T. Wagner, S. Beirle, M. Grzegorski and U. Platt, Global trends (1996–2003) of total column precipitable water observed by Global Ozone Monitoring Experiment (GOME) on ERS2 and their relation to near-surface temperature, J. Geophys. Res.: Atmos., 2006, 111, D12102 CrossRef.
-
C. Ahrens and P. Samson, Extreme Weather and Climate, Brooks Cole, United States of America, 2011, p. 1 Search PubMed.
- F. Alshawaf, K. Balidakis, G. Dick, S. Heise and J. Wickert, Estimating trends in atmospheric water vapour and temperature time series over Germany, Atmos. Meas. Tech., 2017, 10, 3117–3132 CrossRef.
- I. M. Held and B. J. Soden, Water vapor feedback and global warming, Annu. Rev. Environ. Resour., 2000, 25, 441–475 Search PubMed.
-
G. Myhre, Coauthors, Anthropogenic and Natural Radiative Forcing. Climate Change. The Physical Science Basis, ed. T. F. Stocker, et al., Cambridge University Press, 2013, pp. 659–740 Search PubMed.
- L. Zhang, L. Wu and B. Gan, Modes and mechanisms of global water vapour variability over the twentieth century, J. Clim., 2013, 26(15), 5578–5593 CrossRef.
- K. E. Trenberth, A. Dai, R. M. Rasmussen and D. B. Parsons, The changing character of precipitation, Bull. Am. Meteorol. Soc., 2003, 84, 1205–1217 CrossRef.
- K. E. Trenberth, J. Fasullo and L. Smith, Trends and variability in column-integrated atmospheric water vapor, Clim. Dyn., 2005, 7–8, 741–758 CrossRef.
- B. Radhakrishna, T. N. Rao and K. Saikranthi, Spatial Coherence of Water Vapor and Rainfall over the Indian Subcontinent during Different Monsoon Seasons, J. Hydrometeorol., 2019, 20, 45–58 CrossRef.
- R. Wang, Y. Fu, T. Xian, F. Chen, R. Yuan, R. Li and G. Liu, Evaluation of Atmospheric Precipitable Water Characteristics and Trends in Mainland China from 1995 to 2012, J. Clim., 2017, 30, 8673–8688 CrossRef.
- Y. Zhang, J. Xu, N. Yang and P. Lan, Variability and Trends in Global Precipitable Water Vapor Retrieved from COSMIC Radio Occultation and Radiosonde Observations, Atmosphere, 2018, 9, 174 CrossRef.
-
IPCC Climate Change, the Physical Science Basis. Contribution of Working Group I to the Sixth Assessment Report of the Intergovernmental Panel on Climate Change, Cambridge University Press, 2021 Search PubMed.
- R. J. Ross and W. P. Elliott, Radiosonde-based Northern Hemisphere tropospheric water vapor trends, J. Clim., 2001, 14, 1602–1612 CrossRef.
- S. Mieruch, S. Noel, H. Bovensmann and J. P. Burrows, Analysis of global water vapour trends from satellite measurements in the visible spectral range, Atmos. Chem. Phys., 2008, 8, 491–504 CrossRef CAS.
- L. Bengtsson, S. Hagemann and K. I. Hodges, Can climate trends be calculated from reanalysis data, J. Geophys. Res.: Atmos., 2004, 109, D11111 CrossRef.
- B. Chen and Z. Liu, Global water vapor variability and trend from the latest 36 years (1979 to 2014) data of ECMWF and NCEP reanalyses, radiosonde, GPS, and microwave satellite, J. Geophys. Res.: Atmos., 2016, 121, 442–462 CrossRef.
- R. P. Allan, K. M. Willett, V. O. John and T. Trent, Global changes in water vapor 1979–2020, J. Geophys. Res.: Atmos., 2022, 127, D036728 Search PubMed.
- S. Jade, T. S. Shrungeshwara and B. Anil, Water vapor study using MODIS and GPS data at 64 continuous GPS stations (2002–2017) in indian subcontinent, J. Atmos. Sol.-Terr. Phys., 2019, 196, 105138 CrossRef.
- A. K. Mishra, Variability of integrated precipitable water over India in a warming climate, Meteorol. Appl., 2020, 27, 1869 CrossRef.
- P. Jindal, P. K. Thapliyal, M. V. Shukla, S. K. Sharma and D. Mitra, Trend analysis of atmospheric temperature, water vapour, ozone, methane and carbon-monoxide over few major cities of India using satellite data, J. Earth Syst. Sci., 2020, 129, 60 CrossRef CAS.
- V. K. Patel and J. Kuttippurath, Significant increase in water vapour over India and Indian Ocean: Implications for tropospheric warming and regional climate forcing, Sci. Total Environ., 2022, 838, 155885 CrossRef CAS.
- K. R. Kumar, K. K. Kumar and G. B. Pant, Diurnal asymmetry of surface temperature trends over India, Geophys. Res. Lett., 1994, 21, 677–680 CrossRef.
- Y. J. Kaufman and B. C. Gao, Remote sensing of water vapor in the near IR from EOS/MODIS, IEEE Trans. Geosci. Remote Sens., 1992, 30, 871–884 CrossRef.
- B. C. Gao and Y. J. Kaufman, Water vapor retrievals using Moderate Resolution Imaging Spectrometer (MODIS) near-infrared channels, J. Geophys. Res.: Atmos., 2003, 108, 4389 Search PubMed.
- A. K. Prasad and R. P. Singh, Validation of MODIS terra, AIRS, NCEP/DOE AMIP-II reanalysis- 2, and AERONET sun photometer derived integrated precipitable water vapor using ground-based GPS receivers over India, J. Geophys. Res.: Atmos., 2009, 114, D05107 CrossRef.
- S. Kumar, A. K. Singh, K. A. Prasad and R. P. Singh, Variability of GPS derived water vapor and comparison with MODIS data over the Indo-Gangetic plains, Phys. Chem. Earth, 2013, 55, 11–18 CrossRef.
- S. Joshi, K. Kumar, B. Pande and M. C. Pant, GPS-derived precipitable water vapour and its comparison with MODIS data for Almora, central Himalaya, India, Meteorol, Atmos. Phys., 2013, 120, 177–187 CrossRef.
- S. S. Ningombam, S. Jade, T. S. Shrungeshwara and H. J. Song, Validation of water vapor retrieval from moderate resolution imaging spectro-radiometer (MODIS) in near infrared channels using GPS data over IAO-Hanle, in the trans-Himalayan region, J. Atmos. Sol.-Terr. Phys., 2016, 137, 76–85 CrossRef.
- H. Hersbach,
et al., The ERA5 global reanalysis, Q. J. R. Meteorol. Soc., 2020, 146, 1999–2049 CrossRef.
- H. B. S. Kannemadugu, K. Ranganathan, B. Gharai and M. V. R. Seshasai, GNSS-GPS derived integrated water vapor and performance assessment of ERA-5 data over India, J. Atmos. Sol.-Terr. Phys., 2022, 227, 105807 CrossRef.
- S. Wang, T. Xu, W. Nie, C. Jiang, Y. Yang, Z. Fang, M. Li and Z. Zhang, Evaluation of Precipitable Water Vapor from Five Reanalysis Products with Ground-Based GNSS Observations, Remote Sens., 2020, 12, 1817 CrossRef.
- M. M. Rienecker, M. J. Suarez, R. Gelaro, R. Todling, J. Bacmeister, E. Liu, M. G. Bosilovich, D. Schubert, L. Takacs, G. K. Kim, S. Bloom, J. Chen, D. Collins, A. Conaty, A. D. Silva, W. Gu, J. Joiner, R. D. Koster, R. Lucchesi, A. Molod, T. Owens, S. Pawson, P. Pegion, C. R. Redder, R. Reichle, F. R. Robertson, A. G. Ruddick, M. Sienkiewicz and J. Woollen, MERRA- NASA modern-era retrospective analysis for research and applications, J. Clim., 2011, 24, 3624–3648 CrossRef.
- A. Molod, L. Takacs, M. Suarez and J. Bacmeister, Development of the GEOS-5 atmospheric general circulation model: Evolution from MERRA to MERRA2, Geosci. Model Dev., 2015, 8, 1339–1356 CrossRef.
- R. Jacola, R. Knuteson, T. August, T. Hultberg, S. Ackerman and H. Revercomb, A global assessment of NASA AIRS v6 and EUMETSAT IASI v6 precipitable water vapor using ground-based GPS SuomiNet stations, J. Geophys. Res.: Atmos., 2016, 121(15), 8925–8948 CrossRef.
- H. H. Aumann, M. T. Chanhine, C. Gautier, M. Goldberg, E. Kalnay, L. MCMillin, H. Revercomb, P. W. Rosenkranz, W. L. Smith, D. Staelin, L. Strow and J. Susskind, AIRS/AMSU/HSB on the AQUA mission: Design, science objectives data products and processing systems, IEEE Trans. Geosci. Remote Sens., 2003, 41, 410–417 CrossRef.
- T. J. Hearty, A. Savtchenko, B. Tian, E. Fetzer, Y. L. Yung, M. Theobald, B. Vollmer, E. Fishbein and Y. I. Won, Estimating sampling biases and measurement uncertainties of AIRS/AMSU-A temperature and water vapor observations using MERRA reanalysis, J. Geophys. Res.: Atmos., 2014, 119, 2725–2741 CrossRef CAS.
- A. K. Srivastava, M. Rajeevan and S. R. Kshirsagar, Development of High Resolution Daily Gridded Temperature Data Set (1969–2005) for the Indian Region, Atmos. Sci. Lett., 2009, 10, 249–254 Search PubMed.
- D. S. Pai, S. Latha, M. Rajeevan, O. P. Sreejith, N. S. Satbhai and B. Mukhopadhyay, Development of a new high spatial resolution (0.25° X 0.25°) Long period (1901–2010) daily gridded rainfall data set over India and its comparison with existing data sets over the region, Mausam, 2014, 65, 1–18 CrossRef.
-
J. T. Abatzoglou, S. Z. Dobrowski, S. A. Parks and K. C. Hegewisch, TerraClimate, A High-Resolution Global Dataset of Monthly Climate and Climatic Water Balance from 1958–2015, Sci. Data, 2018, vol. 5 Search PubMed.
- P. Barman, S. Jade, A. Kumar and J. Wangshimenla, Interannual, spatial, seasonal, and diurnal variability of precipitable water vapour over northeast India using GPS time series, Int. J. Remote Sens., 2017, 38, 391–411 CrossRef.
- R. Chakraborty, M. V. Ratnam and S. G. Basha, Long-term trends of instability and associated parameters over the Indian region obtained using a radiosonde network, Atmos. Chem. Phys., 2019, 19, 3687–3705 CrossRef CAS.
- S. Goroshi, R. Pradhan, R. P. Singh, K. K. Singh and J. S. Parihar, Trend analysis of evapotranspiration over India: Observed from long-term satellite measurements, J. Earth Syst. Sci., 2017, 126, 113 CrossRef.
- H. S. Chaudhari, S. Pokhrel, S. Mohanty and S. K. Saha, Seasonal prediction of Indian summer monsoon in NCEP coupled and uncoupled model, Theor. Appl. Climatol., 2013, 114, 459–477 CrossRef.
- T. V. L. Kumar, K. K. Rao, R. Uma and K. Aruna, The role of El Niño southern oscillation on the patterns of cycling rates observed over India during the monsoon, J. Water Clim. Chang., 2014, 5, 696–706 CrossRef.
- J. M. Slingo and H. Annamalai, 1997: the El Niño of the Century and the Response of the Indian Summer Monsoon, Mon. Weather Rev., 2000, 128, 1778–1797 CrossRef.
- K. E. Trenberth and L. Smith, The Mass of the Atmosphere: A Constraint on Global Analyses, J. Clim., 2005, 18(6), 864–875 CrossRef.
- P. J. Nair, A. Chakraborty, H. Varikoden, P. A. Francis and J. Kuttippurath, The local and global climate forcings induced inhomogeneity of Indian rainfall, Sci. Rep., 2018, 8, 6026 CrossRef CAS PubMed.
- J. Kuttippurath, S. Murasingh, P. A. Stott, B. Balan Sarojini, M. K. Jha, P. Kumar, P. J. Nair, H. Varikoden, S. Raj, P. A. Francis and P. C. Pandey, Observed rainfall changes in the past century (1901–2019) over the wettest place on Earth, Environ. Res. Lett., 2021, 16, 1748–9326 Search PubMed.
|
This journal is © The Royal Society of Chemistry 2023 |
Click here to see how this site uses Cookies. View our privacy policy here.