Future greenhouse gas emissions from metal production: gaps and opportunities towards climate goals†
Received
14th July 2021
, Accepted 28th October 2021
First published on 26th November 2021
Abstract
Climate change is an urgent global challenge, and greenhouse gas (GHG) emissions from metal production contribute to a substantial part of total emissions. Metals play an essential role in human life, and their demand will increase with global population and economic growth. Therefore, projecting future GHG emissions associated with metal production and exploring effective measures to alleviate GHG emissions are essential for achieving climate goals. This study projects the future GHG emissions associated with the primary and secondary production of six metals (aluminum, copper, iron, lead, nickel, and zinc) by considering the detailed metal cycles according to the shared socio-economic pathways (SSPs). Additionally, influential factors for GHG emissions in metal cycles are explored using decomposition and sensitivity analyses to reduce future GHG emissions. We show that future GHG emissions from metal production cannot be in line with the climate goal required to maintain the temperature change below 2 °C under any SSP, even though the trends for GHG emissions from metal production are significantly different among the SSPs. Therefore, substantial efforts to reduce GHG emissions are required in addition to the transition to the sustainable socio-economic pathway. From a short-term perspective, lowering the per capita in-use metal stock level and GHG emission intensity of metal production is crucial, especially in middle income groups. From a long-term perspective, improving the recycling rate is a possible step after sufficient in-use metal stocks are accumulated for recycling. To achieve the climate goals for both short- and long-term GHG reductions in metal cycles, a combination of actions on these influential factors without delay is essential.
Broader context
Climate change is an urgent global challenge for the whole society. In particular, metal production requires massive amounts of energy for mining, smelting, and refining, which causes significant amounts of greenhouse gas (GHG) emissions (approximately 10% of global GHG emissions). The predicted increase in future metal demand may get in the way of our climate goal. Here we show that the GHG emission reduction target will not be achieved for metal production under any future socio-economic scenarios (the shared socio-economic pathways: SSPs) that are the basis of climate goal analysis. Therefore, substantial efforts to reduce GHG emissions are required in addition to the transition to the sustainable socio-economic pathway. From a short-term perspective, lowering the saturation value of per capita in-use metal stock and improving GHG emission intensity of metal production could be effective for reducing GHG emissions, especially in the middle income level countries. From a long-term perspective, the improvement of the recycling rate can work after sufficient in-use metal stocks are accumulated for recycling. We conclude that, in addition to the transition to the sustainable socio-economic pathway, implementing multiple measures in parallel with international cooperation is essential for sustainable metal use in line with climate goals.
|
Introduction
Climate change is an urgent global challenge. Reduction in global greenhouse gas (GHG) emissions of 41–72% by 2050 and 78–118% by 2100 compared with the level in 2010 are required to maintain the temperature change below 2 °C relative to pre-industrial levels.1 The current society heavily relies on metals for a variety of purposes, which is predicted to accelerate in the future owing to the global population and economic growth and increasing low-carbon technology adoption.2–7 According to a report by the International Resource Panel, metal production accounts for approximately 10% of global GHG emissions.8 Therefore, the associated GHG emissions and other environmental impacts of metal production are highly concerning in the pursuit of achieving a sustainable society.9–11 Given the significance of the environmental impact of metal production, decoupling the metal demand and associated environmental impacts from economic growth is essential for sustainable development within planetary boundaries.12–16 The growth of metal demand and associated environmental impacts are influenced by various factors related to the metal cycle, production technology, and energy transition, including metal use level, recycling rate, product lifetime, and emission intensities.17,18 Furthermore, socio-economic growth patterns, which determine global population and economic growth, are also relevant factors. In order to figure out the strategy that supports sustainable metal use with lower environmental impacts, it is essential to project the environmental impacts associated with future metal production under probable socio-economic situations and explore effective measures for alleviating the environmental impacts of future metal production.
Several studies have been conducted to estimate future metal demand and quantify environmental impacts, including GHG emissions from metal production.3,13,18–21 However, these previous studies did not fully discuss effective measures to reduce metal demand and associated environmental impacts due to the lack of a detailed analysis of the metal cycle corresponding to different socio-economic situations. Influential parameters or material efficiency strategies to reduce GHG emissions have been explored at economy-wide levels,22 in specific sectors in Germany,23,24 and for the EU and United States steel cycles.25,26 However, global environmental impacts associated with the production of different metals have not been fully analyzed, considering not only material efficiency but also material stock dynamics, varying growth among different income level groups, etc. This results in an insufficient understanding of effective measures to reduce the global environmental impact of metal production in relation to future socio-economic narratives.
Accurately quantifying future situations is highly difficult because of various uncertain factors (e.g., economic/social conditions and development of technologies). In this context, scenario analysis is effective for describing the probable future by quantifying a range of future situations and consequences.27 In conducting scenario analysis, future scenarios play an essential role as a common basis for projecting probable future situations from various perspectives. Shared socio-economic pathways (SSPs) have recently been developed as future scenarios that provide future socio-economic situations.28–30 Quantification of the SSPs in terms of various factors, including energy systems,31 land use,32 and air pollution,33 has been conducted based on their narratives and quantitative descriptions of the SSPs.29,34–38 Some studies estimated future global demand for metals and materials based on the five SSPs.39–41 However, these studies did not quantify the environmental impacts associated with material production or did not fully consider influential factors that affect metal stock dynamics, such as recycling, product lifetime, and per capita stock level.
In this study, we aim to (1) project GHG emissions associated with future global metal production based on the five SSPs and (2) explore influential factors for reducing future GHG emissions in metal cycles under the SSPs. First, future primary/secondary metal production under the SSPs is estimated for six major metals (aluminum, copper, iron, lead, nickel, and zinc) using material flow analysis (MFA). Then, future GHG emissions associated with metal production are quantified by multiplying future metal production and GHG emission intensity for metal production based on the SSPs. Finally, influential factors and effective measures to reduce future GHG emissions are explored using decomposition and sensitivity analyses of influential parameters. This study provides insights into the probable future GHG emissions from the metal cycle in accordance with different SSPs and crucial factors for reducing GHG emissions. This can support the design of future metal management strategies in climate policies.
Methods
Estimation of primary and secondary metal production for 2010–2100
Estimation of metal demand serves as the basis for the projection of GHG emissions from future metal production. Various approaches have been proposed for estimating the future metal demand and can be classified into two approaches: inflow-driven and stock-driven approaches.7 The inflow-driven approach directly estimates future metal flows by modeling metal demand in relation to socio-economic variables such as GDP and urbanization. For example, van Vuuren et al.42 simulated long-term trends in metal consumption based on the intensity of use hypothesis, which assumes that the intensity of use (defined as metal use per GDP) initially rises and then falls with the growth of per capita GDP. As another way of inflow-driven approach, several studies have estimated future metal demands using regression analysis with per capita GDP, urbanization levels, and time as explanatory variables.3,19,43 On the other hand, the stock-driven approach first estimates the future in-use metal stock growth with the relationship between in-use metal stocks and socio-economic variables based on the assumption that the per capita in-use metal stocks do not increase infinitely and will eventually saturate. Then, future metal demands are determined that are required to meet the estimated in-use metal stock growth. This approach has been mainly applied to estimate future metal demands over the last decade.40,44–47 Although it is difficult to determine the most suitable approach to estimate future metal demand, Müller et al.48 suggested that the approach based on the intensity of use hypothesis lacks robustness and proposed the stock-based approach because in-use metal stocks have the physical meaning as products in use, actually providing services to people. Additionally, Schipper et al.39 compared the estimates of future copper demand using both inflow- and stock-driven approaches and suggested that the latter seems suitable for long-term estimations because the former may lead to overestimation due to a lack of stock saturation. Since the long-term estimation of future metal demands is our focus, a stock-driven approach is applied herein.
One of the core elements in the stock-driven approach is the estimation of in-use metal stocks, while the metal cycle controls the in-use metal stocks, as shown in Fig. 1. In previous studies, we estimated past metal flows and stocks of the six target metals for 231 countries and regions for 1900–2010 using a global metal cycle model.49,50 This estimation of metal flows considered all processes of the metal life cycle and international trade based on previous studies.51,52 In this study, we adopt this dynamic MFA to estimate in-use metal stocks for 231 countries until 2010, by updating some parameters from our previous studies.
| 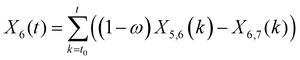 | (1) |
| 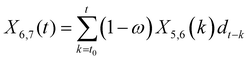 | (2) |
where
X5,6(
t),
X6(
t), and
X5,7(
t) are metal use, in-use metal stock, and waste flow in year
t (kg), respectively (
Fig. 1),
ω is the in-use dissipation rate (%), and
dt is the discard rate determined by the lifetime distribution (%). Historical metal use (
X5,6) was derived from previous studies.
49,50 The end-uses of metals are classified into 4–7 types, and we set the parameter values for each end-use based on previous studies (Tables S1–S6, ESI
†). The in-use metal stocks by country are aggregated into four income level groups (
i.e., high income, upper-middle income, lower-middle income, and low income).
53
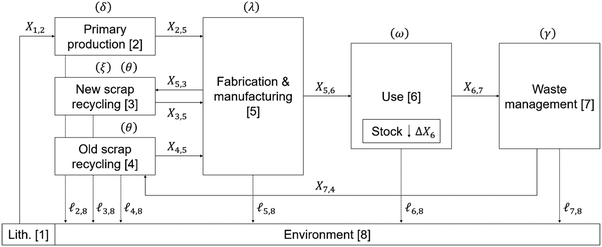 |
| Fig. 1 Metal cycle model covering processes from metal extraction to waste management. X denotes the metal flow or stock; denotes the loss to the environment. | |
In the stock-driven approach, per capita in-use metal stocks are assumed to be saturated at a certain GDP level. To estimate future in-use metal stocks, per capita in-use metal stocks are modeled by applying a logistic curve represented by eqn (3).
| 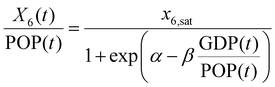 | (3) |
where POP(
t) and GDP(
t) refer to population (billions) and GDP on the basis of purchasing power parity (PPP) (US$2005 (PPP)/cap) in year
t, respectively;
x6,sat is the saturation value of per capita in-use metal stock (kg per cap); and
α and
β are parameters. The saturation value (
x6,sat) is calibrated based on the historical growth of in-use metal stocks in the high income group. Two parameters (
α,
β) are determined for each end-use by fitting the curve to the historical growth of population, GDP, and per capita in-use metal stocks of the four income level groups until 2010, with a boundary condition in which the calculated value of total in-use metal stocks corresponds to the historical result in 2010. Future in-use metal stocks until 2100 are then estimated by applying the future population and GDP for five SSPs to the derived logistic curves. Brief descriptions of SSPs are summarized in
Fig. 2, and the future population and GDP growth for each scenario are shown in Fig. S1 (ESI
†). The five SSPs show diverse transitions of socio-economic conditions due to various factors, including population and economic development, and disparity among the income groups. Some qualitative descriptions of the SSPs have been explored in previous studies,
41,54 but qualitative differences among the SSPs, such as technological innovation and material intensity, are not considered in this study due to their inherent uncertainties. The consideration of these narratively described aspects in the SSPs is partly addressed by the sensitivity analysis described later and should be further explored in future works. Additionally, increases in metal demand due to low-carbon energy technology adoption were not considered in this study. Even though the demand for the six target metals may not significantly increase with the increasing use of low-carbon energy technology compared with lithium and cobalt,
55 considering the effects of low-carbon energy technology adoption on metal demand is also an important future work.
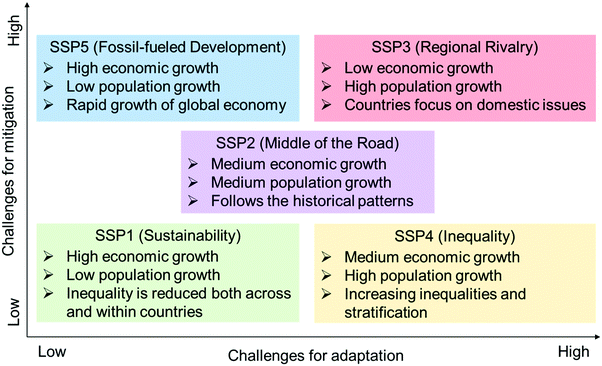 |
| Fig. 2 Overview of the five SSPs (based on O’Neill et al.29). | |
Future metal use was calculated according to the estimated future in-use metal stocks using eqn (4).
| X5,6(t) = X6(t) − X6(t − 1) + X6,7(t) | (4) |
From the yield and collection rates for each process, the metal flows described in Fig. 1 can be calculated according to a previous study.56
| 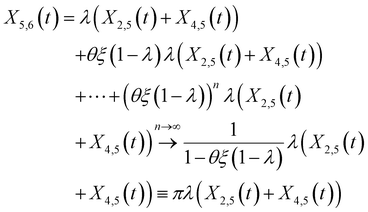 | (5) |
where
λ,
θ,
ξ, and
γ are the manufacturing yield (%), secondary production yield (%), new scrap recovery rate (%), and old scrap collection rate (%), respectively. In
eqn (5), the loop of metals between the fabrication and new scrap recycling processes (
X5,3 and
X3,5) is considered, and π is called new scrap recycling loop factor.
56 Primary metal production (
X2,5) and secondary metal production (
X4,5) can be calculated using
eqn (5) and (6).
Finally, it should be noted that our estimates of future metal production are not predictions but projections by the stock-driven approach. This is based on the assumption that per capita in-use metal stock growth in non-high income groups follows that in a high income group and saturates at the current in-use metal stock level of a high income group. Per capita in-use metal stocks can exceed the saturation level or may decrease in the future. Therefore, it is essential to explore the range of values using sensitivity analysis, as described later.
GHG emissions associated with metal production for 2010–2100
Global GHG emissions associated with future primary and secondary metal production were estimated using eqn (7). | C(t) = kp(t)X2,5(t) + ks(t)X4,5(t) | (7) |
where C(t) is the GHG emissions associated with metal production (kg CO2-eq.), and kp(t) and ks(t) are GHG emission intensities of primary metal production (kg CO2-eq. per kg) and secondary metal production (kg CO2-eq. per kg), respectively.
Regarding the environmental impacts per kg metal production, Van der Voet et al.21 estimated time-series emission intensities using a life cycle assessment database considering ore grade decline, efficiency improvements of metal production, and electricity mixes. In this study, the GHG emission intensities of the primary energy and energy mix for the five SSPs were derived from previous studies.30,31,57–61 The GHG emission intensities of primary and secondary metal production for 2010–2100 (kp, ks) were then estimated based on the GHG emission intensity of primary energy and the energy mix for the SSPs and GHG emissions intensity of metal production estimated by Van der Voet et al.21 Here, note that our analysis of the future GHG emission intensities as well as metal production is based on foreseeable socio-economic conditions and technological development. Therefore, there may be a potential for a significant reduction of future GHG emissions associated with metal production due to technological innovation beyond the foreseeable future situation, especially under the future scenario with technological development (i.e., SSP5). The potential of some specific innovative technologies under development that may reduce GHG emissions of metal production beyond the scenarios based on the SSPs are discussed in the discussion section.
Decomposition analysis
To identify the main factors that contribute to metal demand growth and associated GHG emissions, we adopt the logarithmic mean divisia index (LMDI) approach.62,63 The LMDI is one of the index decomposition analysis (IDA) approaches, which quantify the contribution of factors to changes in indicators such as energy consumption and CO2 emissions.64 The LMDI is recommended among IDA approaches because of its theoretical foundation, adaptability, ease of use and result interpretation, and perfect decomposition.65 It has been adopted to analyze not only CO2 emissions66–68 but also material use-related concerns.69–72
The total GHG emissions associated with metal production calculated using eqn (7) is decomposed as follows:
| 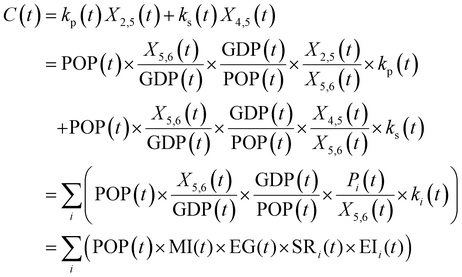 | (8) |
where
Pi(
t) denotes the primary or secondary metal production in year
t (kg);
i denotes the primary or secondary metal; MI(
t) denotes the total metal demand per GDP (
i.e., metal intensity) in year
t (kg per US$2005 (PPP)), EG(
t) denotes the per capita GDP in year
t (US$2005 (PPP) per cap), SR
i(
t) denotes the ratio of primary or secondary metal production to the total demand in year
t (%), and EI
i(
t) denotes the GHG emissions intensity of primary or secondary metal production in year
t (kg CO
2-eq. per kg). The arithmetic change in total GHG emissions associated with metal production Δ
Vtot from year
t1 to year
t0 is decomposed into five factors: the population effect (Δ
VPOP), metal intensity effect (Δ
VMI), economic growth effect (Δ
VEG), secondary metal production ratio effect (Δ
VSR), and emission intensity effect (Δ
VEI).
| ΔVtot = C(t1) − C(t0) = ΔVPOP + ΔVMI + ΔVEG + ΔVSR + ΔVEI | (9) |
In this study, we calculated the contributions of these effects to the total GHG emissions associated with metal production every five years for 2010–2100 using the following equations.63
|  | (10) |
| 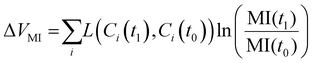 | (11) |
|  | (12) |
|  | (13) |
| 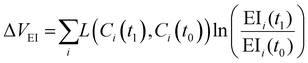 | (14) |
where
Ci(
t) denotes the GHG emissions associated with primary or secondary metal production (kg CO
2-eq.), and
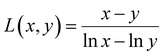
.
Exploration of influential parameters for GHG emissions
We explored the potential to reduce GHG emissions associated with metal production and identified effective measures through the sensitivity analysis of relevant parameters. We varied the parameters, including the saturation value of per capita in-use metal stock, average lifetime, GHG emission intensity, and old scrap collection (recycling) rate, by −10%, −20%, −30%, +10%, +20%, and +30%. Additionally, we estimated GHG emissions for the ultimate scenario assuming a recycling rate of 100% to explore the maximum potential to reduce GHG emissions by recycling. Since the saturation value of per capita in-use metal stock is determined based on the current level of a high income group, changing the saturation value by −10%, −20%, and −30% indicates that the future per capita in-use metal stock of a high income group follows a pattern with lower saturation values than the current level of a high income group. However, it is unrealistic for per capita in-use metal stock levels to rapidly decrease to a new saturation value. Therefore, we assume that the per capita in-use metal stock of a high income group will gradually decrease so that it reaches the lower saturation value in 2050.
Results
Projected metal demand and associated GHG emissions by 2100
The future demand of all target metals is estimated to be larger than the level in 2010 throughout the 21st century and can increase in maximum by 2.3–4.4 times compared with 2010 (Fig. 3). It is clear that the demand growth trends are different among the five SSPs. For most metals, demand rapidly grows until the midcentury and then declines in the late century for SSP1 and SSP5; demand grows until the late century and then declines for SSP2; demand continues to increase for SSP3 and SSP4. SSP1 and SSP5 exhibit the largest metal demand from a short-term perspective (by 2050), while SSP2 and SSP3 exhibit a larger metal demand than these scenarios from a longer-term perspective (by 2100). The different pathways of future metal demand in each SSP result in differences in GHG emissions associated with metal production among the scenarios.
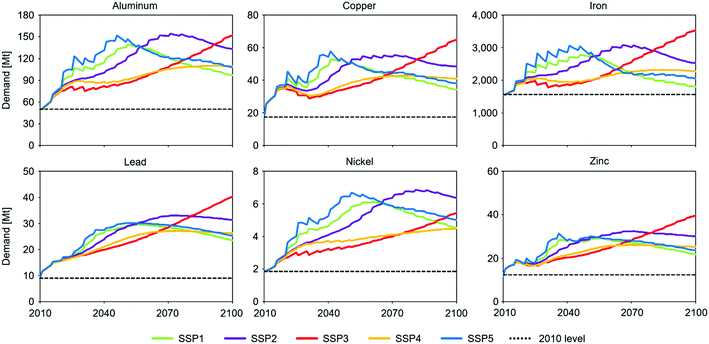 |
| Fig. 3 Future demand of the six metals for 2010–2100 projected for the SSPs. A black dotted line indicates the demand level in 2010. | |
Our projection results of GHG emissions demonstrate the difficulties in achieving climate change goals in terms of GHG emissions associated with metal production. Fig. 4 shows significant differences in the temporal transition of GHG emissions associated with metal production among the five SSPs, but no SSP can achieve the climate goal of maintaining temperature change below 2 °C (RCP2.6) in terms of both annual and cumulative GHG emissions (additional results of future in-use metal stocks, metal production, GHG emission intensity, and GHG emissions for each metal are shown in Fig. S2–S20, ESI†). Although SSP1 exhibits the best performance for the annual GHG emission in 2100, the annual GHG emission in SSP1 increases up to 1.7 times in the early century than that in 2010, resulting in cumulative GHG emissions in 2100 that are 1.9 times higher than the required level as the climate goal. Increasing total GHG emissions from metal production are mainly driven by iron (Fig. S21, ESI†), owning to its largest metal demand and primary production, especially in the early century (Fig. 3 and Fig. S11, ESI†), which is consistent with the result of a previous study.21 Meanwhile, other metals (aluminum, copper, lead, nickel, and zinc) also show a similar temporal trend even though the amounts of GHG emissions associated with their production are relatively lower than that of iron; therefore, there is no significant difference in future GHG emission trends between the six target metals. Furthermore, the results of different income level groups reveal that the middle income groups (both upper and lower) are major contributors to the increase in GHG emissions in the early century under all SSPs (Fig. S22 and S23, ESI†).
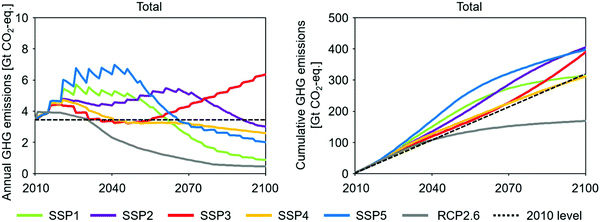 |
| Fig. 4 Total GHG emissions associated with the production of the six metals for 2010–2100 projected for the SSPs (left: annual emission, right: cumulative emission). The gray curve represents the GHG emission pathways aiming to limit the increase of temperature to 2 °C (i.e., RCP2.6), which is estimated assuming a constant share of the GHG emissions associated with metal production to the total GHG emissions in industrial sectors.74 The black dotted line in the right figure indicates cumulative GHG emission assuming the constant annual GHG emission level in 2010. | |
It is evident that SSPs have a significant effect on the GHG emissions associated with metal production. Depending on SSPs, annual and cumulative GHG emissions in 2100 can vary by a maximum of 5.5 and 92.3 Gt CO2-equivalent, respectively (Fig. 4). However, all SSPs exceed the GHG emission level required to achieve the climate goal. Furthermore, SSP4 shows the best performance in terms of cumulative GHG emissions, but describes an undesirable world in which inequality and stratification increase both across and within countries,29 which is against the sustainable development goals.73 This is reflected in the results of per capita in-use metal stocks, which demonstrate the unequal distributions of in-use metal stocks between income level groups under SSP4 (Fig. S3–S8, ESI†). These results suggest that GHG emission reductions associated with metal production cannot lead to the achievement of the goals for sustainable development under the current expected future socio-economic situations described by the SSPs. Further efforts related to other factors (e.g., metal intensity, secondary metal production ratio, and emission intensity) in addition to population and economic growth are required to achieve the climate goal.
Drivers of future GHG emissions associated with metal production
The transition of the annual GHG emissions shown in Fig. 4 is driven by various factors, and decomposition analysis can identify the contribution of each effect on the changes of GHG emissions. The contribution of five effects (population, metal intensity, economic growth, secondary metal use ratio, and emission intensity) on changes in annual GHG emissions compared to those in 2010 is shown as the change over time in Fig. 5 (results for the change in annual GHG emissions every five years are shown in Fig. S24, ESI†). There are major influential effects in all SSPs: economic growth (blue bars in Fig. 5), metal intensity (deep red bars), and secondary metal production ratio (yellow bars). The increase in annual GHG emissions in the early century is mainly attributed to economic growth. Conversely, improvements in the metal intensity (i.e., metal demand per GDP) and secondary metal production ratio are the main contributors to the decrease in GHG emissions. Improvement in metal intensity advances the decoupling of metal demand and economic growth (Fig. S15, ESI†). Thus, metal production-related GHG emissions decrease in most SSPs, on the basis of the assumption that per capita in-use metal stock saturates at a certain economic level (Fig. S2, ESI†). The secondary metal production ratio effect also contributes to the decrease in GHG emissions, which increased in the late century owing to the increase in the in-use metal stock that can be recycled as secondary metal after a certain period of in-use (Fig. S16, ESI†). Effects of the metal intensity and the secondary metal production ratio gradually increase in the late century, except under SSP3. The metal demand in SSP3 increases by 2100 (Fig. 3), whereas the demand in all other SSPs peaks in the early (SSP1 and 5) or late centuries (SSP2 and 4). Therefore, effects of the metal intensity and secondary metal production ratio cannot fully work over time in the case of SSP3. The emission intensity effect (shown as purple bars in Fig. 5) does not significantly contribute to a change in GHG emissions for all SSPs because of relatively low changes over time in the emission intensity for iron production, which accounts for a large part of the total GHG emissions from all metal production (Fig. S17 and S18, ESI†).
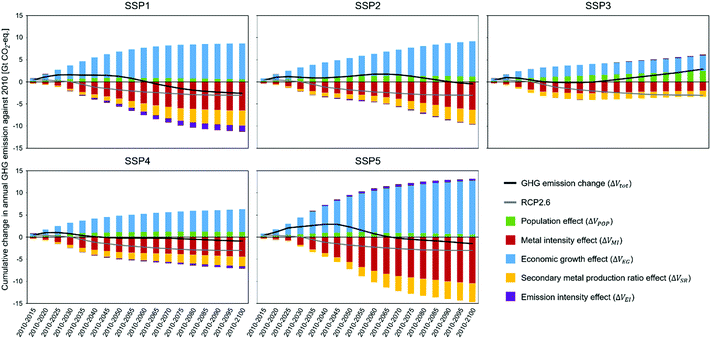 |
| Fig. 5 Decomposition analysis of cumulative changes in the annual GHG emissions associated with metal production compared with the 2010 level. The summation of contributions of the five effects is equal to the cumulative change in GHG emissions. | |
Potential of influential parameters for the reduction of GHG emissions
We demonstrated future projections of GHG emissions associated with metal production and identified the contribution of various factors according to the SSPs. Although the SSPs are archetypes of future scenarios, the parameter settings (stock saturation values, average lifetime, emission intensity of metal production, and recycling rate) may have a range of values that may influence the projection results. Therefore, changing the values of parameters during sensitivity analysis may indicate the potential for reducing GHG emissions.
The sensitivity analysis reveals that the influential parameters for GHG emissions differ depending on the time perspectives. Fig. 6 shows how much cumulative GHG emissions can be reduced in 2050 and 2100 by varying parameters in comparison with the results with the original parameters (Fig. 4), and the temporal transition of GHG emissions over time and annual GHG emission changes for varying parameter settings are shown in Fig. S25–S29 (ESI†). Decreases in saturation value and emission intensity are effective for both mid- and long-term reductions in cumulative GHG emissions for all SSPs. An increase in recycling rate can also contribute to the reductions in both mid- and long-terms; however, its effect is more significant from a long-term perspective. This is because the effect of improving the recycling rate is limited by the amounts of in-use metal stocks and waste flows, which is highlighted by the parameter setting of a 100% recycling rate. Even though the scenario with a 100% recycling rate is based on an extreme and unrealistic assumption, the potential to reduce GHG emissions is limited from a short-term perspective.
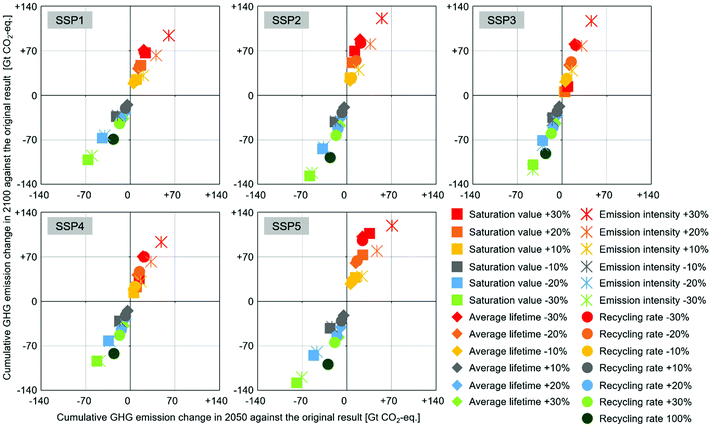 |
| Fig. 6 Cumulative GHG emission changes in 2050 and 2100 compared to the original results, obtained by varying parameters. | |
Our analysis suggests that an action that focuses only on a single parameter is insufficient for achieving the GHG emission reduction goal (Fig. S30, ESI†). Although some cases exhibit the potential to achieve the goal for the annual GHG emission in 2100 (e.g., saturation value −30% with SSP1) (Fig. S30, ESI†), no case presented in Fig. S25–S28 (ESI†) achieves the goal in terms of cumulative GHG emissions until 2100. This suggests that focusing only on annual GHG emissions may lead to misunderstandings and that the consideration of emission pathways and cumulative emissions is significant to discuss measures for achieving the emission reduction targets. Additionally, we explored the effects of varying parameters in different income level groups (Fig. S31 and S32, ESI†). These results show that upper- and lower-middle income groups have a greater potential to reduce GHG emissions by improving the parameters than other groups. This finding implies that taking multiple actions without delay leading to the changes in different parameters in not only developed countries but also developing countries, as well as following the sustainable socio-economic pathway, is essential to achieve lower cumulative GHG emissions in line with climate goals.
Discussion
How can we achieve metal use with lower environmental pressure in line with the climate goal? Primarily, our analysis suggests that the socio-economic situation (i.e., the SSP that we will follow) greatly influences the growth of GHG emissions associated with metal production (Fig. 4). In addition to transitioning to the sustainable socio-economic pathway, decreasing the saturation value of per capita in-use metal stock level and the emission intensity is especially significant for reducing GHG emissions. In this section, we discuss the possibility of improving these parameters in the future.
Decreasing the saturation value of per capita in-use metal stock level means that developed countries go back to their past level. In the case of iron, a 10% lower level of per capita in-use metal stock corresponds to that in a high income group in 2002 (∼9.6 tons per capita), a 20% lower level corresponds to that in 1993 (∼8.5 tons per capita), and a 30% lower level corresponds to that in 1980 (∼7.5 tons per capita) according to our estimates. These results imply that reducing GHG emissions associated with metal production requires ambitious efforts to reduce the per capita metal stock level in a high income group and to saturate the level in other income groups at lower levels. Then, what can we do to reduce the per capita in-use metal stock level? Here, per capita in-use metal stock is decomposed according to a previous study proposing a set of indicators for material stock, flows, and service provisioning:75
|  | (15) |
where the first term on the right side denotes the reciprocal of stock productivity, the second term denotes the reciprocal of the service utilization rate, and the third term denotes the per capita service demand. This decomposition suggests that multiple actions can be taken to reduce the per capita in-use metal stock level. First, stock productivity can be increased by weight saving and the substitution by materials with lower environmental impacts, which are related to technological improvement. Developed countries with higher technological levels can contribute to this factor, and technology transfers to developing countries can be efficient for facilitating the improvement in stock productivity at a global scale. Second, the service utilization rate can be improved by utilizing underused infrastructure and buildings, sharing economy, and product service systems, which are related to systematic improvements.
76–78 This factor basically depends on the actions of each service provider of the metal stocks. Finally, the per capita service demand is related to consumer behavior and living standard, which may be different among income level groups, and may be decreased by inducing a change in lifestyles. From the perspective of equality, increasing this value in developing countries is essential, while saturating it at a moderate level will be required in the future. These points suggest that the efforts of different stakeholders (producers, service providers, and consumers) are essential to reduce the per capita in-use metal stock level. Note that the quantitative analysis of the benefit and feasibility of these actions is beyond the scope of this study, but is an important area for future research.
There are multiple options for reducing the GHG emission intensity associated with metal production, including energy transition, existing technology improvements, and novel approach development, which have been reviewed and examined in previous studies.21,26,49,79,80 Regarding the energy transition, Van der Voet et al.21 examined the emission intensity of metal production under a scenario of a rapid transition towards a renewable energy system. Although some metals exhibit decreasing emission intensity (e.g., that of primary aluminum production can be cut by half in 2050), that of primary iron production, which dominates the GHG emissions associated with metal production (Fig. S21, ESI†), will remain nearly unchanged. This is because primary steel is mainly produced using the blast furnace-basic oxygen furnace (BF-BOF) route, in which the majority of GHG emissions are unrelated to electricity.79 Nevertheless, given that the share of annual GHG emissions of aluminum and secondary iron production will increase in the late century for most SSPs (Fig. S16 and S21, ESI†), an energy transition for reducing the emission intensity of metal production is effective for the long-term reduction of GHG emissions. Furthermore, some innovative steel production technologies premise the energy transition because they depend on electricity rather than fossil fuels.26,79,80 Improving existing production routes (i.e., BF-BOF) has the advantage of utilizing existing plants and thus rapid improvements can be expected, but previous studies suggest a limited potential for reducing the emission intensity.79,80 Therefore, the development of innovative technologies coupled with energy transition is essential to reduce the GHG emission intensity of metal production, particularly in terms of long-term climate goal achievement.
Currently, over 99% of global steel production is covered by existing technologies (i.e., BF-BOF and electric arc furnaces with pig iron, steel scrap, and direct reduced iron), while multiple innovative technologies are under development: top gas recycling, hydrogen reduction, smelt reduction, steel electrolysis, paired straight hearth furnace, hydrogen flash iron-making, and carbon capture and storage.26,79,80 Although these technologies have the potential to dramatically reduce emission intensities associated with steel production, their development and commercialization require time, and thus, prompt improvements in emission intensity are not expected.26,79 As our results suggest that annual GHG emissions associated with metal production will be larger in the early century (Fig. 4), a combination of prompt measures to reduce emission intensity is essential, including the improvement in existing production routes, subsidies to commercialize innovative technologies, and energy transition.
Conclusion
This study quantified the implications of SSPs for global GHG emissions associated with metal production for 2010–2100, and explored effective measures to reduce GHG emissions for achieving climate goals. We show that SSPs have a significant effect on the GHG emissions associated with metal production. Some SSPs exhibit a decrease in annual GHG emissions in the late century. However, due to increasing global metal demand and associated GHG emissions in the early century driven mainly by economic growth in the middle income level groups, the GHG emission reduction target required to maintain a temperature change below 2 °C will not be achieved under any SSP. In addition to SSPs, lowering the saturation value of per capita in-use metal stock and improving emission intensity could be effective for reducing GHG emissions, especially in the middle income level groups. Nevertheless, improving a single parameter is expected to be insufficient for achieving climate goals in terms of cumulative GHG emissions. Therefore, improving several parameters parallelly in not only developed countries but also developing countries, as well as following the sustainable socio-economic pathway (i.e., SSP1), is necessary. Given that parameters such as emission intensity cannot be improved promptly, implementing multiple measures immediately with international cooperation is essential for sustainable metal use in line with the climate goals.
Author contributions
Conceptualization, R. Y., T. W., and M. M.; methodology, R. Y., T. W., and M. M.; formal analysis, R. Y.; investigation, R. Y.; resources, T. W.; writing – original draft, R. Y.; writing – review & editing, R. Y., T. W., and M. M.
Conflicts of interest
There are no conflicts to declare.
Acknowledgements
This research was partly supported by Grant-in-Aid for Early-Career Scientists (JSPS KAKENHI JP20K20014).
References
- IPCC, Climate Change 2014: Mitigation of Climate Change. Contribution of Working Group III to the Fifth Assessment Report of the Intergovernmental Panel on Climate Change, Cambridge University Press, Cambridge, United Kingdom and New York, NY, USA, 2014.
- P. Christmann, Towards a More Equitable Use of Mineral Resources, Nat. Resour. Res., 2018, 27, 159–177 CrossRef.
- A. Elshkaki, T. E. Graedel, L. Ciacci and B. K. Reck, Resource Demand Scenarios for the Major Metals, Environ. Sci. Technol., 2018, 52, 2491–2497 CrossRef CAS PubMed.
- J. Lee, M. Bazilian, B. Sovacool, K. Hund, S. M. Jowitt, T. P. Nguyen, A. Månberger, M. Kah, S. Greene, C. Galeazzi, K. Awuah-Offei, M. Moats, J. Tilton and S. Kukoda, Reviewing the material and metal security of low-carbon energy transitions, Renewable Sustainable Energy Rev., 2020, 124, 109789 CrossRef.
- UNEP, Environmental Risks and Challenges of Anthropogenic Metals Flows and Cycles. A Report of the Working Group on the Global Metal Flows to the international Resource Panel, Paris, France, 2013.
- O. Vidal, B. Goffé and N. Arndt, Metals for a low-carbon society, Nat. Geosci., 2013, 6, 894–896 CrossRef CAS.
- T. Watari, K. Nansai and K. Nakajima, Major metals demand, supply, and environmental impacts to 2100 A critical review, Resour., Conserv. Recycl., 2021, 164, 105107 CrossRef.
- IRP, Global Resources Outlook 2019: Natural Resources for the Future We Want, A Report of the International Resources Panel, United Nations Environment Programme, Nairobi, Kenya, 2019.
- S. H. Farjana, N. Huda, M. A. P. Mahmud and R. Saidur, A review on the impact of mining and mineral processing industries through life cycle assessment, J. Cleaner Prod., 2019, 231, 1200–1217 CrossRef.
-
T. E. Graedel and E. van der Voet, Linkage of Sustainability, MIT Press, Cambridge, 2010 Search PubMed.
- P. Nuss and M. J. Eckelman, Life Cycle Assessment of Metals: A Scientific Synthesis, PLoS One, 2014, 9, e101298 CrossRef PubMed.
- J. Rockström, W. Steffen, K. Noone, Å. Persson, F. S. Chapin III, E. F. Lambin, T. M. Lenton, M. Scheffer, C. Folke, H. J. Schellnhuber, B. Nykvist, C. A. de Wit, T. Hughes, S. van der Leeuw, H. Rodhe, S. Sörlin, P. K. Snyder, R. Costanza, U. Svedin, M. Falkenmark, L. Karlberg, R. W. Corell, V. J. Fabry, J. Hansen, B. Walker, D. Liverman, K. Richardson, P. Crutzen and J. A. Foley, A safe operating space for humanity, Nature, 2009, 461, 472–475 CrossRef.
- H. Schandl, S. Hatfield-Dodds, T. Wiedmann, A. Gaschke, Y. Cai, J. West, D. Newth, T. Baynes, M. Lenzen and A. Owen, Decoupling global environmental pressure and economic growth scenarios for energy use, materials use and carbon emissions, J. Cleaner Prod., 2016, 132, 45–56 CrossRef CAS.
- W. Steffen, K. Richardson, J. Rockström, S. E. Cornell, I. Fetzer, E. M. Bennett, R. Biggs, S. R. Carpenter, W. de Vries, C. Folke, D. Gerten, J. Heinke, G. M. Mace, L. M. Persson, V. Ramanathan, B. Reyers, S. Sörlin and C. A. de Wit, Planetary boundaries: Guiding human development on a changing planet, Science, 2015, 347, 1259855 CrossRef PubMed.
- UNEP, Decoupling natural resource use and environmental impacts from economic growth, A Report of the Working Group on Decoupling to the International Resource panel, 2011.
- T. Watari, K. Nansai and K. Nakajima, Contraction and convergence of in-use metal stocks to meet climate goals, Glob. Environ. Change, 2021, 69, 102284 CrossRef.
- T. E. Graedel, Grand Challenges in Metal Life Cycles, Nat. Resour. Res., 2017, 27, 181–190 CrossRef.
- S. Hatfield-Dodds, H. Schandle, D. Newth, M. Obersteiner, Y. Cai, T. Baynes, J. West and P. Havlik, Assessing global resource use and greenhouse emissions to 2050, with ambitious resource efficiency and climate mitigation policies, J. Cleaner Prod., 2017, 144, 403–414 CrossRef.
- L. Ciacci, T. Fishman, A. Elshkaki, T. E. Graedel, I. Vassura and F. Passarini, Exploring future copper demand, recycling and associated greenhouse gas emissions in the EU-28, Glob. Environ. Change, 2020, 63, 102093 CrossRef.
- K. J. J. Kuipers, L. F. C. M. van Oers, M. Verboon and E. van der Voet, Assessing environmental implications associated with global copper demand and supply scenarios from 2010 to 2050, Glob. Environ. Change, 2018, 49, 106–115 CrossRef.
- E. Van der Voet, L. Van Oers, M. Verboon and K. Kuipers, Environmental Implications of Future Demand Scenarios for Metals: Methodology and Application to the Case of Seven Major Metals, J. Ind. Ecol., 2018, 23, 141–155 CrossRef.
- Y. Lu and H. Schandl, Do sectoral material efficiency improvements add up to greenhouse gas emissions reduction on an economy-wide level?, J. Ind. Ecol., 2021, 25, 523–536 CrossRef CAS.
- S. Pauliuk and N. Heeren, Material efficiency and its contribution to climate change mitigation in Germany: A deep decarbonization scenario analysis until 2060, J. Ind. Ecol., 2021, 25, 479–493 CrossRef CAS.
- S. Pauliuk, T. Fishman, N. Heeren, P. Berrill, Q. Tu, P. Wolfram and E. G. Hertwich, Linking service provision to material cycles: A new framework for studying the resource efficiency–climate change (RECC) nexus, J. Ind. Ecol., 2021, 25, 260–273 CrossRef.
- M. Axelson, S. Oberthür and L. J. Nilsson, Emission reduction strategies in the EU steel industry: Implications for business model innovation, J. Ind. Ecol., 2021, 25, 390–402 CrossRef CAS.
- N. A. Ryan, S. A. Miller, S. J. Skerlos and D. R. Cooper, Reducing CO2 Emissions from U.S. Steel Consumption by 70% by 2050, Environ. Sci. Technol., 2020, 54, 14598–14608 CrossRef CAS PubMed.
- R. J. Swart, P. Raskin and J. Robinson, The problem of the future: sustainability science and scenario analysis, Glob. Environ. Change, 2004, 14, 137–146 CrossRef.
- B. C. O’Neill, E. Kriegler, K. Riahi, K. L. Ebi, S. Hallegatte, T. R. Carter, R. Mathur and D. P. van Vuuren, A new scenario framework for climate change research: the concept of Shared Socioeconomic Pathways, Clim. Change, 2014, 122, 387–400 CrossRef.
- B. C. O’Neill, E. Kriegler, K. L. Ebi, E. Kemp-Benedict, K. Riahi, D. S. Rothman, B. J. van Ruijven, D. P. van Vuuren, J. Birkmann, K. Kok, M. Levy and W. Solecki, The roads ahead: narratives for Shared Socioeconomic Pathways describing world futures in the 21st century, Glob. Environ. Change, 2017, 42, 169–180 CrossRef.
- K. Riahi, D. P. van Vuuren, E. Kriegler, J. Edmonds, B. C. O’Neill, S. Fujimori, N. Bauer, K. Calvin, R. Dellink, O. Fricko, W. Lutz, A. Popp, J. C. Cuaresma, S. Kc, M. Leimbach, L. Jiang, T. Kram, S. Rao, J. Emmerling, K. Ebi, T. Hasegawa, P. Havlik, F. Humpenöder, L. A. Da Silva, S. Smith, E. Stehfest, V. Bosetti, J. Eom, D. Gernaat, T. Masui, J. Rogelj, J. Strefler, L. Drouet, V. Krey, G. Luderer, M. Harmsen, K. Takahashi, L. Baumstark, J. C. Doelman, M. Kainuma, Z. Klimont, G. Marangoni, H. Lotze-Campen, M. Obersteiner, A. Tabeau and M. Tavoni, The Shared Socioeconomic Pathways and their energy, land use, and greenhouse gas emissions implications: An overview, Glob. Environ. Change, 2017, 42, 153–168 CrossRef.
- N. Bauer, K. Calvin, J. Emmerling, O. Fricko, S. Fujimori, J. Hilaire, J. Eom, V. Krey, E. Kriegler, I. Mouratiadou, H. Sytze de Boer, M. van den Berg, S. Carrara, V. Daioglou, L. Drouet, J. E. Edmonds, D. Gernaat, P. Havlik, N. Johnson, D. Klein, P. Kyle, G. Marangoni, T. Masui, R. C. Pietzcker, M. Strubegger, M. Wise, K. Riahi and D. P. van Vuuren, Shared socio-economic pathways of the energy sector – quantifying the narratives, Glob. Environ. Change, 2017, 42, 316–330 CrossRef.
- A. Popp, K. Calvin, S. Fujimori, P. Havlik, F. Humpenöder, E. Stehfest, B. L. Bodirsky, J. P. Dietrich, J. C. Doelmann, M. Gusti, T. Hasegawa, P. Kyle, M. Obersteiner, A. Tabeau, K. Takahashi, H. Valin, S. Waldhoff, I. Weindl, M. Wise, E. Kriegler, H. Lotze-Campen, O. Fricko, K. Riahi and D. P. van Vuuren, Land-use futures in the shared socio-economic pathways, Glob. Environ. Change, 2017, 42, 331–345 CrossRef.
- S. Rao, Z. Klimont, S. J. Smith, R. Van Dingenen, F. Dentener, L. Bouwman, K. Riahi, M. Amann, B. L. Bodirsky, D. P. van Vuuren, L. A. Reis, K. Calvin, L. Drouet, O. Fricko, S. Fujimori, D. Gernaat, P. Havlik, M. Harmsen, T. Hasegawa, C. Heyes, J. Hilaire, G. Luderer, T. Masui, E. Stehfest, J. Strefler, S. van der Sluis and M. Tavoni, Future air pollution in the Shared Socio-economic Pathways, Glob. Environ. Change, 2017, 42, 346–358 CrossRef.
- J. Crespo, Cuaresma, Income projections for climate change research: A framework based on human capital dynamics, Glob. Environ. Change, 2017, 42, 226–236 CrossRef.
- R. Dellink, J. Chateau, E. Lanzi and B. Magné, Long-term economic growth projections in the Shared Socioeconomic Pathways, Glob. Environ. Change, 2017, 42, 200–214 CrossRef.
- L. Jiang and B. O’Neill, Global urbanization projections for the Shared Socioeconomic Pathways, Glob. Environ. Change, 2017, 42, 193–199 CrossRef.
- S. Kc and W. Lutz, The human core of the shared socioeconomic pathways: Population scenarios by age, sex and level of education for all countries to 2100, Glob. Environ. Change, 2017, 42, 181–192 CrossRef PubMed.
- M. Leimbach, E. Kriegler, N. Roming and J. Schwanitz, Future growth patterns of world regions – A GDP scenario approach, Glob. Environ. Change, 2017, 42, 215–225 CrossRef.
- B. W. Schipper, H. C. Lin, M. A. Meloni, K. Wansleeben, R. Heijungs and E. van der Voet, Estimating global copper demand until 2100 with regression and stock dynamics, Resour., Conserv. Recycl., 2018, 132, 28–36 CrossRef.
- R. Yokoi, J. Nakatani and Y. Moriguchi, Calculation of Characterization Factors of Mineral Resources Considering Future Primary Resource Use Changes: A Comparison between Iron and Copper, Sustainability, 2018, 10, 267 CrossRef.
- H. Schandl, Y. Lu, N. Che, D. Newth, J. West, S. Frank, M. Obersteiner, A. Rendall and S. Hatfield-Dodds, Shared socio-economic pathways and their implications for global materials use, Resour., Conserv. Recycl., 2020, 160, 104866 CrossRef.
- D. P. Van Vuuren, B. J. Strengers and H. J. M. De Vries, Long-term perspectives on world metal use—a system-dynamics model, Resour. Policy, 1999, 25, 239–255 CrossRef.
- A. Elshkaki, S. Lei and W. Q. Chen, Material-energy-water nexus Modelling the long term implications of aluminium demand and supply on global climate change up to 2050, Environ. Res., 2020, 181, 108964 CrossRef CAS PubMed.
- H. Hatayama, I. Daigo, Y. Matsuno and Y. Adachi, Outlook of the World Steel Cycle Based on the Stock and Flow Dynamics, Environ. Sci. Technol., 2010, 44, 6457–6463 CrossRef CAS PubMed.
- S. Pauliuk, R. L. Milford, D. B. Müller and J. M. Allwood, The Steel Scrap Age, Environ. Sci. Technol., 2013, 47, 3448–3454 CrossRef CAS PubMed.
- Z. Wen, C. Zhang, X. Ji and Y. Xue, Urban Mining's Potential to Relieve China's Coming Resource Crisis, J. Ind. Ecol., 2015, 19, 1091–1102 CrossRef.
- A. Yoshimura and Y. Matsuno, Dynamic Material Flow Analysis and Forecast of Copper in Global-Scale: Considering the Difference of Recovery Potential between Copper and Copper Alloy, Mater. Trans., 2018, 59, 989–998 CrossRef CAS.
- D. B. Müller, T. Wang and B. Duval, Patterns of iron use in societal evolution, Environ. Sci. Technol., 2011, 45, 182–188 CrossRef.
- T. Watari, K. Nansai, D. Giurco, K. Nakajima, B. McLellan and C. Helbig, Global Metal Use Targets in Line with Climate Goals, Environ. Sci. Technol., 2020, 54, 12476–12483 CrossRef CAS.
- T. Watari and R. Yokoi, International inequality in in-use metal stocks: What it portends for the future, Resour. Policy, 2021, 70, 101968 CrossRef.
- K. Nakajima, I. Daigo, K. Nansai, K. Matsubae, W. Takayanagi, M. Tomita and Y. Matsuno, Global distribution of material consumption: Nickel, copper, and iron, Resour., Conserv. Recycl., 2018, 133, 369–374 CrossRef.
- K. Nansai, K. Nakajima, S. Kagawa, Y. Kondo, S. Suh, Y. Shigetomi and Y. Oshita, Global Flows of Critical Metals Necessary for Low-Carbon Technologies: The Case of Neodymium, Cobalt, and Platinum, Environ. Sci. Technol., 2014, 48, 1391–1400 CrossRef CAS PubMed.
- The World Bank, DataBank, https://databank.worldbank.org/databases, accessed March 2021.
- T. Fishman, N. Heeren, S. Pauliuk, P. Berrill, Q. Tu, P. Wolfram and E. G. Hertwich, A comprehensive set of global scenarios of housing, mobility, and material efficiency for material cycles and energy systems modeling, J. Ind. Ecol., 2021, 25, 305–320 CrossRef.
- B. K. Sovacool, S. H. Ali, M. Bazilian, B. Radley, B. Nemery, J. Okatz and D. Mulvaney, Sustainable minerals and metals for a low-carbon future, Science, 2020, 367, 30–33 CrossRef CAS.
- C. Helbig, A. Thorenz and A. Tuma, Quantitative assessment of dissipative losses of 18 metals, Resour., Conserv. Recycl., 2020, 153, 104537 CrossRef.
- K. Calvin, B. Bond-Lamberty, L. Clarke, J. Edmonds, J. Eom, C. Hartin, S. Kim, P. Kyle, R. Link, R. Moss, H. Mcjeon, P. Patel, S. Smith, S. Waldhoff and M. Wise, The SSP4: A world of deepening inequality, Glob. Environ. Change, 2017, 42, 284–296 CrossRef.
- O. Fricko, P. Havlik, J. Rogelj, Z. Klimont, M. Gusti, N. Johnson, P. Kolp, M. Strubegger, H. Valin, M. Amann, T. Ermolieva, N. Forsell, M. Herrero, C. Heyes, G. Kindermann, V. Krey, D. L. McCollum, M. Obersteiner, S. Pachauri, S. Rao, E. Schmid, W. Schoepp and K. Riahi, The marker quantification of the Shared Socioeconomic Pathway 2: A middle-of-the-road scenario for the 21st century, Glob. Environ. Change, 2017, 42, 251–267 CrossRef.
- S. Fujimori, T. Hasegawa, T. Masui, K. Takahashi, D. S. Herran, H. Dai, Y. Hijioka and M. Kainuma, SSP3: AIM implementation of Shared Socioeconomic Pathways, Glob. Environ. Change, 2017, 42, 268–283 CrossRef.
- E. Kriegler, N. Bauer, A. Popp, F. Humpenöder, M. Leimbach, J. Strefler, L. Baumstark, B. L. Bodirsky, J. Hilaire, D. Klein, I. Mouratiadou, I. Weindl, C. Bertram, J. P. Dietrich, G. Luderer, M. Pehl, R. Pietzcker, F. Piontek, H. Lotze-Campen, A. Biewald, M. Bonsch, A. Giannousakis, U. Kreidenweis, C. Müller, S. Rolinski, A. Schultes, J. Schwanitz, M. Stevanovic, K. Calvin, J. Emmerling, S. Fujimori and O. Edenhofer, Fossil-fueled development (SSP5): An energy and resource intensive scenario for the 21st century, Glob. Environ. Change, 2017, 42, 297–315 CrossRef.
- D. P. van Vuuren, E. Stehfest, D. E. H. J. Gernaat, J. C. Doelman, M. van den Berg, M. Harmsen, H. S. de Boer, L. F. Bouwman, V. Daioglou, O. Y. Edelenbosch, B. Girod, T. Kram, L. Lassaletta, P. L. Lucas, H. van Meijl, C. Müller, B. J. van Ruijven, S. van der Sluis and A. Tabeau, Energy, land-use and greenhouse gas emissions trajectories under a green growth paradigm, Glob. Environ. Change, 2017, 42, 237–250 CrossRef.
- B. W. Ang, F. Q. Zhang and K. H. Choi, Factorizing changes in energy and environmental indicators through decomposition, Energy, 1998, 23, 489–495 CrossRef.
- B. W. Ang, The LMDI approach to decomposition analysis: a practical guide, Energy Policy, 2005, 33, 867–871 CrossRef.
- B. W. Ang, LMDI decomposition approach A guide for implementation, Energy Policy, 2015, 86, 233–238 CrossRef.
- B. W. Ang, Decomposition analysis for policymaking in energy: which is the preferred method?, Energy Policy, 2004, 32, 1131–1139 CrossRef.
- B. W. Ang and B. Su, Carbon emission intensity in electricity production: A global analysis, Energy Policy, 2016, 94, 56–63 CrossRef CAS.
- L. C. Freitas and S. Kaneko, Decomposing the decoupling of CO2 emissions and economic growth in Brazil, Ecol. Econ., 2011, 70, 1459–1469 CrossRef.
- L. C. Liu, Y. Fan, G. Wu and Y. M. Wei, Using LMDI method to analyze the change of China's industrial CO2 emissions from final fuel use: An empirical analysis, Energy Policy, 2007, 35, 5892–5900 CrossRef.
- B. Liu, X. Zheng, Q. Zhang, X. Pan and B. Mclellan, Drivers of global metal footprint during 1995-2013, J. Cleaner Prod., 2020, 256, 120467 CrossRef.
- B. Plank, N. Eisenmenger and A. Schaffartzik, Do material efficiency improvements backfire? Insights from an index decomposition analysis about the link between CO2 emissions and material use for Austria, J. Ind. Ecol., 2021, 25, 511–522 CrossRef CAS.
- F. Pothen and M. Schymura, Bigger cakes with fewer ingredients A comparison of material use of the world economy, Ecol. Econ., 2015, 109, 109–121 CrossRef.
- R. Yokoi, K. Nansai, K. Nakajima, T. Watari and M. Motoshita, Responsibility of consumers for mining capacity: decomposition analysis of scarcity-weighted metal footprints in the case of Japan, iScience, 2021, 24, 102025 CrossRef PubMed.
- UN, Transforming our world: the 2030 Agenda for Sustainable Development, https://sdgs.un.org/publications/transforming-our-world-2030-agenda-sustainable-development-17981, accessed March 2021.
- D. P. van Vuuren, E. Stehfest, M. G. J. den Elzen, T. Kram, J. van Vliet, S. Deetman., M. Isaac, K. K. Goldewijk, A. Hof, A. M. Beltran, R. Oostenrijk and B. van Ruijven, RCP2.6: exploring the possibility to keep global mean temperature increase below 2 °C, Clim. Change, 2011, 109, 95–116 CrossRef.
- H. Tanikawa, T. Fishman, S. Hashimoto, I. Daigo, M. Oguchi, A. Miatto, S. Takagi, N. Yamashita and H. Schandl, A framework of indicators for associating material stocks and flows to service provisioning: Application for Japan 1990–2015, J. Cleaner Prod., 2021, 285, 125450 CrossRef.
- K. Frenken, Political economies and environmental futures for the sharing economy, Philos. Trans. R. Soc., A, 2017, 375, 2016067 CrossRef.
- J. Korhonen, A. Honkasalo and J. Seppälä, Circular Economy: The Concept and its Limitations, Ecol. Econ., 2018, 143, 37–46 CrossRef.
- A. Tukker, Product services for a resource-efficient and circular economy – a review, J. Cleaner Prod., 2015, 97, 76–91 CrossRef.
- Z. Fan and J. Friedmann, Low-carbon production of iron and steel: Technology options, economic assessment, and policy, Joule, 2021, 5, 829–862 CrossRef CAS.
- M. Fischedick, J. Marzinkowski, P. Winzer and M. Weigel, Techno-economic evaluation of innovative steel production technologies, J. Cleaner Prod., 2014, 84, 563–580 CrossRef CAS.
Footnote |
† Electronic supplementary information (ESI) available. See DOI: 10.1039/d1ee02165f |
|
This journal is © The Royal Society of Chemistry 2022 |
Click here to see how this site uses Cookies. View our privacy policy here.