DOI:
10.1039/D0SC04321D
(Review Article)
Chem. Sci., 2021,
12, 830-840
Can we predict materials that can be synthesised?
Received
6th August 2020
, Accepted 25th November 2020
First published on 9th December 2020
Abstract
The discovery of materials is an important element in the development of new technologies and abilities that can help humanity tackle many challenges. Materials discovery is frustratingly slow, with the large time and resource cost often providing only small gains in property performance. Furthermore, researchers are unwilling to take large risks that they will only know the outcome of months or years later. Computation is playing an increasing role in allowing rapid screening of large numbers of materials from vast search space to identify promising candidates for laboratory synthesis and testing. However, there is a problem, in that many materials computationally predicted to have encouraging properties cannot be readily realised in the lab. This minireview looks at how we can tackle the problem of confirming that hypothetical materials are synthetically realisable, through consideration of all the stages of the materials discovery process, from obtaining the components, reacting them to a material in the correct structure, through to processing into a desired form. In an ideal world, a material prediction would come with an associated ‘recipe’ for the successful laboratory preparation of the material. We discuss the opportunity to thus prevent wasted effort in experimental discovery programmes, including those using automation, to accelerate the discovery of novel materials.
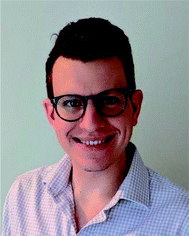 Filip T. Szczypińsk | Filip Szczypiński obtained his Master's degree in Natural Sciences in 2014 from the University of Cambridge, where he investigated the synthesis and host-guest binding inside large metal–organic cages. For his PhD, he studied synthetic information molecules under the supervision of Prof. Chris Hunter. Currently, Filip is working with Dr Kim Jelfs at Imperial College London on the modelling of new porous organic molecular materials, where he combines his synthetic experience with computational tools to predict new materials that can be synthesised. |
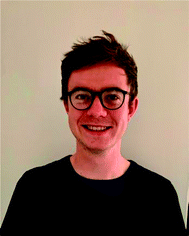 Steven Bennett | Steven Bennett obtained his Master's degree in Chemistry in 2018 from University College London (UCL). In October 2018, he joined the Jelfs Group at Imperial College London to work on the computational discovery of synthetically accessible functional materials, namely porous organic cages. This work is supported by a PhD studentship from the Leverhulme Trust via the Leverhulme Centre for Functional Materials, which aims to develop his interest in sustainable, advanced material development. |
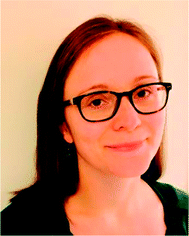 Kim E. Jelfs | Kim Jelfs is a Reader in Computational Materials Chemistry and Royal Society University Research Fellow at the Department of Chemistry at Imperial College London, UK. Kim carried out her PhD modelling zeolite crystal growth at UCL, before working as a post-doctoral researcher at the University of Liverpool with Prof. Andy Cooper FRS. Kim began her independent research at Imperial College in 2013 and her research focuses upon the use of computer simulations to assist in the discovery of supramolecular materials. |
1 Introduction
Advances in materials and technology have always accompanied human progress. However, the fast growth of industry is a double-edged sword that has created many new global challenges, such as resource scarcity, waste, and pollution. The discovery of new, cheap, and sustainable materials with tailored properties can help us address humanity's current and future challenges. Materials can be simple systems made from single molecules or a small number of (inorganic) elements, or more complex systems processed into a variety of forms, including crystals, amorphous structures, thin films, and devices with multiple materials assembled together. Materials discovery is frustratingly slow, with frequent incremental improvements and much rarer leaps to new materials classes with ground-breaking properties.
There is considerable discussion of the potential for automation to revolutionise materials science so that new materials can enter the market sooner than the current 40 year timescale from laboratory discovery to industrialisation.1 The design and synthesis processes in materials discovery can be accelerated with autonomous workflows, such as the use of data repositories, automation, and parallelisation.2 Recently, a mobile robotic chemist was used to autonomously explore a large chemical space in search for an improved hydrogen-production catalyst.3 Such an autonomous screening strategy was employed as many materials are impossible to design rationally due to their extremely high multi-scale complexity. Great efforts and resources are wasted on the synthesis of systems that do not yield materials with interesting properties. The chemical space of drug-like organic molecules alone, which are in theory potential material building blocks, is estimated to be between 1023 and 1060 possible compounds.4,5 Moreover, most materials are not built from organic molecules alone and for inorganic materials, the number of potential elemental compositions and stoichiometries is practically infinite, but many are not stable, or their preparation conditions are not known. Even with automation, it is not possible to adequately search the chemical space of materials, and many material syntheses or property characterisation techniques are not simply automatable due to complex multi-step protocols or specialist offline equipment being required.
In recent years, we are increasingly turning to computation to assist in accelerating material discovery across broad classes of materials and applications. The Holy Grail of materials prediction is inverse design (see Fig. 1), where appropriate materials and their components are designed based upon knowledge of only the desired material properties. The capability to achieve inverse design would be equivalent to the retrosynthetic analysis that can be carried out for molecular organic synthesis. The unpredictability of component assembly into the final material form means that we cannot reliably provide retrosynthetic pathways to optimal materials from first principles. Thus, rather than design materials, we must typically screen viable materials that have targeted properties. A computational screening process usually starts with large libraries of precursors, predicts how they will assemble into a material and finally calculates their properties using computational chemistry tools or data-driven models such as machine learning. The significant challenge of the structure prediction stage means that this is often skipped, or assumptions are made based on commonly occurring structural motifs. The computed material properties of the candidates can be used to select the experimental target for laboratory synthesis and testing.
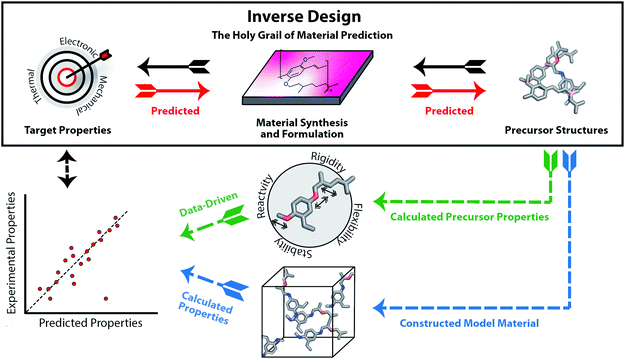 |
| Fig. 1 Inverse design (top), as opposed to a typical computational-experimental discovery workflow (below). Ideally, the precursor structures could be designed directly from the desired properties, considering the synthesis and formulation of the material. Instead, typically researchers start from databases of precursors used to construct molecules in silico and calculate the properties of the modelled material using quantum chemistry or machine learning techniques (blue route). Sometimes, data-driven approaches can be used to predict the material properties directly from the precursor structures or properties (green route). The main question stays the same: can both the precursors and the material in its correct form to achieve the desired properties actually be synthesised? | |
Computational screening is still more time- and resource-efficient than a synthetic screening. Machine learning is also beginning to influence and accelerate the structure–property prediction, but the lack of sufficient training data inhibits generation of predictive models, especially for organic materials.6 Molecules can be encoded for machine learning in numerous ways: from simple molecular formulae that do not convey any connectivity, through 2D chemical graphs, to full spatial coordinate descriptors at a single molecule level. Beyond representations of the material's components, it is often important to encode the broader environment of the solid-state packing and the development of representations in general is an active area of research. Which specific prediction task is being tackled will heavily influence the choice of representation and the correct selection can be critical to any potential prediction success.
A key question remains: can screened or designed materials actually be synthesised, and how? As Jansen and Schön argue, we are never designing materials but merely searching for thermodynamically viable minima on the potential energy landscape.7 It is far easier to predict materials with good properties than it is to predict materials with good properties that can be synthetically realised, rather than remaining “hypothetical”. Furthermore, even if synthetically realised in solution, many materials need to be processed into other forms, such as thin films and membranes. It is additionally challenging to predict whether the calculated properties are achieved in the material's final form.
This minireview aims to highlight the major challenges in the prediction of materials that can actually be synthesised in the laboratory. We try to follow an experimentalists' thought process – what precursors to use, are they available, how to combine them to form a material, what is the desired form – and discuss the recent computational advances that can guide or complement each of those stages.
2 Harnessing the power of the literature
Most researchers currently embark on a new material discovery project by exploring the primary form of scholarly communication: published scientific articles. A corresponding literature-based data extraction should also play an important role in automated and autonomous materials discovery. Albeit fragmented into multiple research items, most synthetic procedures are highly prescriptive, and hence in theory suitable for automated extraction using natural language processing methods.8,9 Extraction of chemical data and its automated association with the relevant chemical entities as well as details of the physical measurements (“metadata”) allow for easy creation of massive chemical databases.10 Generated databases can be subsequently enriched by simulations in order to create new data used for materials prediction. Such a workflow has recently been successfully applied in the prediction of dye pairs suitable for solar-cell applications.11 Natural language processing has been used on a corpus of inorganic materials synthesis literature containing the synthesis conditions for various metal oxides. In conjunction with machine learning, those data allowed the prediction of synthesis outcomes in unseen materials systems.12,13 The approaches described above require manual labelling of large datasets and hard-coding complicated language grammar rules, making their implementation extremely labour-intensive. Unsupervised and semi-supervised machine learning have been recently used to by-pass that problem, allowing one to reconstruct not only flowcharts of possible synthetic procedures, but also to capture latent chemical knowledge such as the prediction of promising material candidates years prior their publication.14–16
However, the quality of the datasets and the predictive power of literature-based models can only be as good as the original literature data. Many technical details and conditions are often omitted from reported procedures, which rely on the experience of the experimentalist and hidden instrument settings. Many reactions and processes might indeed be extremely sensitive to changes in room temperature and ambient humidity throughout the year, let alone different countries. Standardisation of experimental procedures and the resulting measurements across the fields of chemistry and material science would greatly simplify the generation of data-driven models and their transferability across various fields. Furthermore, the notable absence of unsuccessful experiments from the scientific literature causes bias in the datasets and thus reduces the reliability of the resulting knowledge. With the advent of pre-print servers and journals focusing on scientific validity instead of perceived research impact, we anticipate that in the near future we will see more accurate computational models trained on a wider range of publicly available experimental results.
3 Accessibility and availability of components
The first experimental step of bottom-up material synthesis always involves reacting some type of precursor components. Inorganic materials are typically obtained from commercially available or relatively simple elemental compounds, alloys, and salts. While some elements might be scarce, precursors for most naturally occurring elements are readily available and thermodynamically-driven reactions are relatively insensitive to the exact nature of the inorganic building blocks. For those reasons, tailored precursors are rarely used in inorganic materials discovery, especially when using automated synthesis platforms. The vast synthesis search space for inorganic materials is further discussed in Section 4.
For organic and metal–organic materials, the synthesis of the organic precursors might be the most time and resource-heavy part of the development process, and small changes in the structure of the organic building blocks might lead to drastic changes in their reactivity. There are millions of commercially available, albeit not necessarily cheap, organic compounds and a practically infinite space of possible molecules that can be enumerated. This provides a great advantage when searching for desired properties in organic materials. However, the synthesis of the organic precursors might be prohibitively difficult or even impossible, thus yielding “ideal” materials “unsynthesisable”. Many libraries of organic precursors contain either potentially biologically active molecules (often of high complexity and not viable for large scale material synthesis, e.g. the ZINC database)17,18 or algorithmically- and machine-learning-generated structures through artificial transformations (hence not necessarily synthetically feasible at all, e.g. the GDB family).19
It is therefore crucial to consider precursor accessibility for material synthesis when assessing whether the material can be experimentally realised. Precursor synthesisability can be used as a filter to remove unsynthesisable materials, but even better, it could provide synthetic chemists with a synthetic protocol (or a retrosynthetic analysis) from commercially available building blocks to the final material. To address this issue, there have been attempts to provide metrics of the synthetic accessibility for organic compounds, albeit focused on their synthesis for drug discovery. These metrics broadly fit into four separate categories: (a) focusing on cheminformatic features related to fragment complexity, (b) built using the available reaction data reported in the chemical literature, (c) based on expert chemists' scoring, and (d) following retrosynthetic planning tools (see Fig. 2).
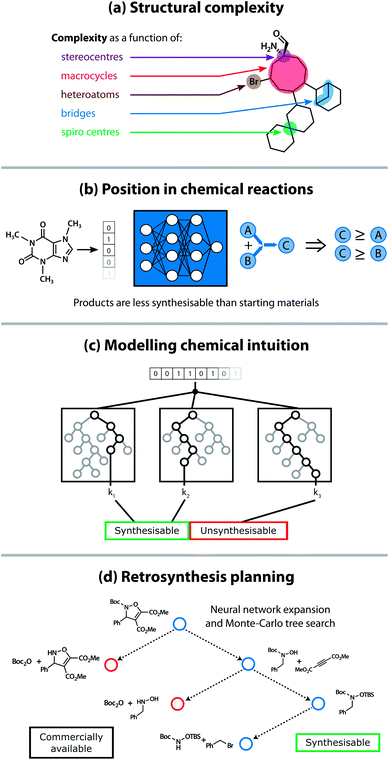 |
| Fig. 2 Four approaches used to calculate the synthetic accessibility of organic compounds: (a) as function of the number of complex structural motifs present in the molecule; (b) deep neural networks trained on extensive reaction databases (reported and proprietary); (c) modelling the decision making process of expert chemists (the “chemical intuition”); (d) by identifying viable reaction pathways using automated retrosynthesis planning tools. | |
Early attempts at modelling the synthetic accessibility of organic molecules focused on a quantitative analysis of the underlying complexity of a molecule based on which structural motifs were present.20,21 However, the assumption that complexity corresponds to synthetic accessibility is not always valid. To mitigate the issues associated with this complexity-based approach, analyses of molecular fragments found in large compound libraries were incorporated into some scoring methods. Fragments that commonly appeared in commercially available compound databases were considered more synthetically accessible than those that appeared infrequently.22–24 Separately, the chemical intuition of expert chemists has been modelled statistically to elucidate the key molecular features that influence a chemist's decision making process.25,26
More recently, extensive reaction databases have paved the way for data-driven synthetic accessibility scores and automated retrosynthesis planning tools to emerge. The synthetic complexity score by Coley et al. used the Reaxys databases, comprised of 12 million reactant–product pairs, to train a neural network on a pairwise ranking task.27 Molecules commonly seen as products of reactions in this database were ranked as more synthetically complex than reactants. One significant development in retrosynthesis planning tools utilised the Monte Carlo tree search algorithm, in combination with a neural network to generate viable retrosynthetic disconnections.28 Natural language processing techniques, such as the transformer neural network architecture,29 in addition to reinforcement learning techniques,30 have also been shown to be effective algorithms for performing a retrosynthetic analysis. However, non-ML techniques such as identifying pathways to similar compounds and hard-coding reaction rules have also performed well.31,32 While computer-assisted retrosynthesis methods are now frequently able to find routes to desirable precursors, it is often time-consuming to fully explore the generated reaction tree and many state-of-the-art models have limited or commercial-only access, making them somewhat unsuitable for high-throughput screening methods.
None of the aforementioned synthetic accessibility scores are perfect, but they can greatly simplify the materials discovery process when acting as a filter for unreasonable precursors. Material synthesis often requires precursors that are cheap and accessible on a large scale, and these heuristic methods could be used to identify precursors that fit these criteria, although not originally developed for this purpose. These methods have been shown to increase the number of experimentally viable candidates when incorporated into objective functions of generative algorithms.33 Such methods may in the future be incorporated into prediction workflows, providing a full synthetic scheme from simple and commercially available building blocks to final materials.
4 Material synthesis
There are two major materials synthesis routes: direct synthesis of a framework material, e.g. inorganic oxides, zeolites, metal–organic frameworks (MOFs), covalent organic frameworks (COFs) – be they crystalline or amorphous – or solid-phase formulation of molecular materials (e.g. porous organic cages). Challenges for framework materials are: do the reactive groups react in the way we want them to, can we predict the solid-phase structure of the resulting material, and is that the form that gives the desired properties? In the case of molecular materials, the corresponding challenges are the following: do the reactive groups react in the way that we would want them to create discrete molecular units and can we predict the structure of those units, (e.g. the correct topology – for organic cages formed from a condensation reaction between a tritopic and a ditopic building blocks, there are six commonly observed cage topologies)34 and can we predict the packing of those units in the solid-phase? The two prediction steps are crucial in this case, as the properties of the resulting material will depend on the intrinsic properties of the discrete units and the extrinsic properties originating from solid-phase packing.
The formulation of solid-state materials carries other challenges than those present in synthetic chemistry. While molecular reactions commonly happen at homogeneous equilibria with all reactants present at finite amount, formulation into the solid-state always involves heterogeneous equilibrium and may often proceed until one or more of the condensed phases fully disappear. The crystal (or amorphous) solid-state structure can provide chemists with valuable insight into many properties of the target materials. Therefore, solid-phase structure prediction is invaluable in predicting new materials. Solid-phase structure prediction is a global optimisation problem, where one is trying to generate the thermodynamic structure based on the chemical composition of the material.35 The two major considerations are the efficient exploration of the multidimensional energy landscape, and then the correct ranking of the relative energies of the resulting spatial arrangements.36 We shall focus on crystal structure prediction (CSP) of crystalline materials, but significant advances have also been made in amorphous structure prediction.37,38
While it is tempting to derive a common theory unifying solid-phase structure prediction for organic and inorganic materials, the challenges in those fields are different and hence they must advance in parallel. We will discuss the different prediction aspects related to inorganic and organic (and hybrid metal–organic) materials below, as outlined in Fig. 3.
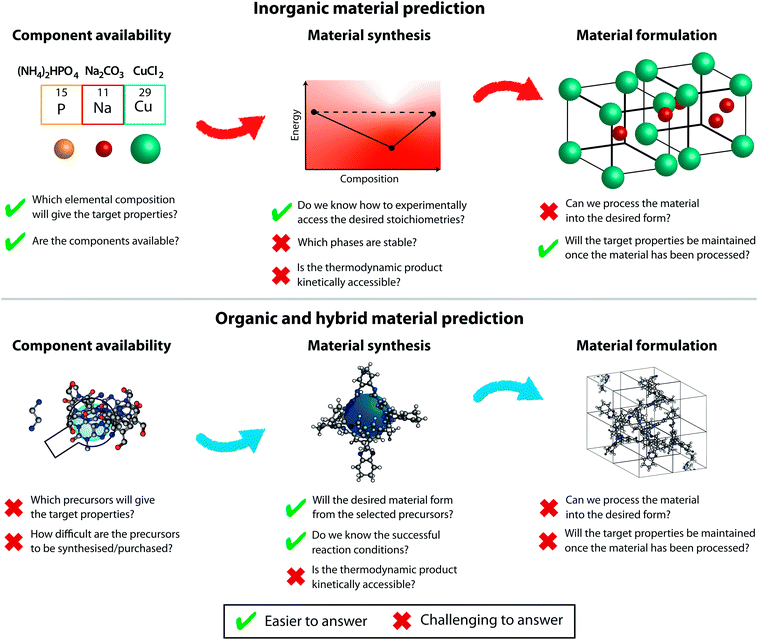 |
| Fig. 3 The challenges to be considered at various stages of prediction for organic materials (top) and inorganic materials (bottom). There are additional considerations at the device level that are not considered in this figure. | |
Inorganic materials
Inorganic materials are most often mixtures of metals or their oxides with a practically infinite continuum of possible elemental compositions.39 Since availability of the inorganic precursors is generally not the limiting factor, the composition possibilities are endless and structure prediction is thus challenging. Indeed, not all possible binary systems were even partially experimentally studied under normal conditions. When looking at the more complex ternary and quaternary structures, the vast majority of the possible compound space remains unexplored.40
Inorganic CSP in its simplest form is based on structure sampling followed by (normally) ab initio energy calculations.41–43 Other methods used to effectively explore the configurational space include simulated annealing,44,45 basin hopping,46 minima hopping,47 metadynamics,48 and evolutionary techniques.36,49–51 Such CSP techniques require high-level ab initio calculations for reliable relative energy ranking to correctly identify local minima and predict, at least theoretically, the existence of (meta)stable inorganic structures. Various further simplifications have been implemented, such as the application of common formulae per unit cell or the use of robust building blocks like inorganic anions.52 Complementary CSP approaches to the computationally expensive ab initio methods harvest the large amount of data gathered in the Inorganic Crystal Structure Database53 and the Cambridge Structural Database.54 Recently, Hegde and Aykol et al. encoded thermodynamic stability of inorganic materials as a phase diagram network. Such a graph-theoretic approach allows one to uncover unknown relationships between materials, such as the discovery of novel materials as absences of expected nodes in thus derived networks.55
Machine learning can be applied directly to crystallographic data “allowing prediction of novel structures”56–59 or to the ab initio energy landscape,38,60–62 thus providing much faster yet accurate potentials used for structure prediction. In particular, generative machine learning models allow one to generate materials with target properties rather than predict the properties of candidate materials. Such approaches have been used to generate novel structures of binary and ternary oxides.63,64 Furthermore, data-mining approaches focus on the extrapolation from the structures that have already been explored and might not be useful for prediction of completely new classes of materials.
Mixed oxides are of high interest due to their applications in the molecular electronics and energy materials. A major issue in the prediction of such materials is the relative stability of possible phases, as unstable phases are by default not synthesisable. Buckeridge et al. reported software that can be used to test the thermodynamic stability of multi-ternary materials.56 Their algorithm significantly speeds up the analysis of the thermodynamic stability of a material, which is normally a very slow process in the case of ternary and quaternary systems. Density functional theory calculations have also been successfully used to obtain reaction enthalpies for complex oxides with large unit cells and doped oxides from binary oxides, which correctly identified the structures obtained experimentally.65–67
Porous materials find application in molecular separations, catalysis and sorption. The most industrially widespread microporous inorganic materials are zeolites and zeo-types: crystalline structures formed by XO4 tetrahedra (where X = Si, Al, P, etc.). All possible zeolitic frameworks have been enumerated mathematically, or built bottom-up from secondary building units, providing the basis for the prediction of novel materials.68,69 These materials have then been assessed for their relative thermodynamic stability, which would correlate with their ease of synthetic access.70 To access hypothetical structures, organic templates have also been successfully computationally screened.71
In summary, accurate prediction of the relative stability of different phases in inorganic materials can help researchers filter out non-viable candidates, hence allowing for the identification of promising materials that can actually be synthesised. However, two different yet similar phases can both be locally stable and the desired form might not be obtained upon first attempt, as the relative thermodynamic stability does not imply that the structure can actually be accessed since other factors, such as kinetic barriers or unknown synthetic routes, might inhibit their experimental realisation. Looking on the bright side, thermodynamic stability at least focuses chemists' efforts in hopeful areas.
Organic and hybrid materials
If the organic precursors for organic and hybrid materials are readily available and stable (which is a prediction challenge in itself, as discussed in Section 3), the synthesis of the material is typically a result of a relatively simple condensation reaction between building blocks. Generally, a single topology of the product is anticipated, but researchers should always expect formation of beautiful and unexpected structures, especially if slight variations from the perfect precursor geometry are present.72,73 We have recently enumerated common topologies observed in porous organic cages34 and other groups have reported typical structures of metal–organic frameworks.74 The underlying assumption is that the material synthesis is under thermodynamic control and hence the resulting topologies can be predicted based on their formation energies and hence relative thermodynamic stability.75 However, kinetic traps can always thwart these necessary assumptions.76,77
With the plethora of chemically reasonable organic building blocks, the possibilities for (metal-)organic materials are endless. The diversity of small molecular backbones with identical reactive units means that common synthetic techniques can be used to obtain the material from a nearly infinite sea of precursors. These established protocols make it low-effort to synthetically screen tens and hundreds of potential materials, especially when robotic automation is exploited.3,78,79 However, it also limits the diversity of the materials explored as the candidates tend to cluster in discrete areas of the chemical space. Furthermore, autonomous use of established synthetic protocols leads to a large number of unsuccessful reactions. This can be due to incorrect experimental conditions that do not favour the format ion of the stable compound as predicted from computational discovery, which could otherwise have been obtained if the appropriate reaction conditions (e.g. solvent or temperature) had been identified with trial-and-error. Machine learning algorithms can aid the discovery of optimal experimental conditions from sets of failed experiments.80 Reporting of unsuccessful reactions would greatly improve the accuracy and availability of such predictions.
As in the case of inorganic materials, the final material properties do not solely depend on the properties of their subunits. Prediction of the material's solid-phase structure is crucial for accurate calculation of bulk material properties. In general, organic CSP starts with the identification of several low-energy molecular conformations, which are then trialled in thousands of different packing arrangements.81 These are then (accurately) ranked by their energy, with again the presumption that a lower energy structure is more likely to form experimentally. While the exploration of the crystal structure space is algorithmically similar to that of inorganic CSP, the multiple possible conformations of organic molecules (as opposed to placement of single atoms or ions) greatly expand the search space. Furthermore, organic solid-phase structures often result from a balance of numerous weak interactions, rather than strong and predictable bonding patterns. Hence, weak interactions need to be correctly accounted for theoretically when attempting organic CSP.82
Large-scale CSP has afforded new molecular semiconductors, which showed good charge-transfer properties related to their packing arrangements.83 In the field of porous molecular materials, CSP leads to the possibility to tune the overall porosity of the material through different crystallisation strategies of the already porous subunits.84,85 A combination of CSP and property calculations allowed for the generation of energy–structure–function maps of porous molecular materials, aiding the discovery of materials for gas storage and selective mixture separations.86 Recently, CSP has been coupled with automated in silico construction of molecular structures in a discovery workflow that afforded new multi-component organic cage pots.87
In the field of MOFs and COFs, many frameworks have been enumerated, either from mathematically enumerated topologies or from a unit-based assembly,74 as described for zeolites. However, thus far, little work has been done to assess their thermodynamic stability or kinetic inertness – indeed molecular dynamics calculations have suggested that many are not.88 This is due to the difficulty in performing DFT level calculations to assess the relative energies of 100
000s of structures. There are large databases of experimentally reported MOFs with solvent and disorder removed, so as to be immediately suitable for molecular simulations.89–91 Hence, assumptions can be made as to which analogous new frameworks can be made within the field of reticular chemistry, but this would limit one to relatively simple modifications.
Of course, the fact that a material has a desired arrangement in the crystalline solid-state is no guarantee that the material can be processed into a desired powder, thin film, membrane, etc. and still deliver the properties for the target application. Furthermore, for many applications, such as solar cells, there is the requirement to fabricate the device with control of interfaces, grain boundaries, and macroscopic structure to maximise or maintain desired properties. These material formulation features are rarely, if ever, factored into a (computational) materials discovery process, and largely rely on the experience of the experimental scientist.
5 Conclusions and outlook
The large space of material compositions, structures, and formulations offers great opportunities in the discovery of potentially useful materials. On the other hand, the ability to synthetically deliver a desired material is limited by the large space of different outcomes, both in terms of structural composition and formulation possibilities. There is thus inevitably a large amount of wasted synthetic effort and cost at every stage of the current process. The inclusion of consideration of the ability to realise a material into the computational prediction process can revolutionise the discovery process. We need to consider whether the material components can be made or easily obtained, whether the synthesis will be successful (in multiple senses), whether the desired structure will form, whether the material can be formulated correctly, and all of this while reaching the targeted properties.
While new capabilities such as automation and artificial intelligence hold promise for materials discovery, it is also important to ensure that computational chemists or material scientists have sufficient insight into the experimental processes involved for the materials they are modelling. Bridging the gap between experimental and computational researchers through fostering close collaborations and fused research programmes is vital. Prediction of new materials would greatly benefit from more standardised experimental procedures that make data mining easier. More detailed and relevant metadata will aid automated prediction of successful experimental conditions. In particular, reporting of unsuccessful synthetic attempts will provide more balanced datasets, which will improve accuracy of computational models and speed up the discovery process. Close discussions and feedback loops between computational predictions and experimental outcomes can direct modelling efforts towards more fruitful and trustworthy results. Those more relevant models will in turn further stimulate experimental material discovery. If we can get better at predicting materials that can be quickly synthetically realised, it will overcome a major bottleneck in the discovery process, offering the potential to accelerate materials discovery beyond the current 40 year timescale.
Conflicts of interest
There are no conflicts to declare.
Acknowledgements
We thank Dr Rebecca Greenaway for useful discussions. K. E. J. thanks the Royal Society for a Royal Society University Research Fellowship. K. E. J. and F. T. S. thank the Leverhulme Trust for a Leverhulme Trust Research Project Grant. S. B. thanks the Leverhulme Research Centre for Functional Materials Design for a Ph.D. studentship. We acknowledge funding from the European Research Council under FP7 (CoMMaD, ERC Grant No. 758370).
Notes and references
- K. Alberi, M. B. Nardelli, A. Zakutayev, L. Mitas, S. Curtarolo, A. Jain, M. Fornari, N. Marzari, I. Takeuchi, M. L. Green, M. Kanatzidis, M. F. Toney, S. Butenko, B. Meredig, S. Lany, U. Kattner, A. Davydov, E. S. Toberer, V. Stevanovic, A. Walsh, N. G. Park, A. Aspuru-Guzik, D. P. Tabor, J. Nelson, J. Murphy, A. Setlur, J. Gregoire, H. Li, R. Xiao, A. Ludwig, L. W. Martin, A. M. Rappe, S. H. Wei and J. Perkins, The 2019 materials by design roadmap, J. Phys. D: Appl. Phys., 2019, 52, 013001 CrossRef
.
- H. S. Stein and J. M. Gregoire, Progress and prospects for accelerating materials science with automated and autonomous workflows, Chem. Sci., 2019, 10, 9640–9649 RSC
.
- B. Burger, P. Maffettone, V. Gusev, C. Aitchison, Y. Bai, W. Xiaoyan, R. S. Sprick and A. I. Cooper, A Mobile Robotic Researcher, Nature, 2020, 583(7815), 237–241 CrossRef CAS
.
- P. G. Polishchuk, T. I. Madzhidov and A. Varnek, Estimation of the size of drug-like chemical space based on GDB-17 data, J. Comput.-Aided Mol. Des., 2013, 27, 675–679 CrossRef CAS
.
- P. Ertl, Cheminformatics analysis of organic substituents: Identification of the most common substituents, calculation of substituent properties, and automatic identification of drug-like bioisosteric groups, J. Chem. Inf. Comput. Sci., 2003, 43, 374–380 CrossRef CAS
.
-
S. Bennett, A. Tarzia, M. A. Zwijnenburg and K. E. Jelfs, Artificial Intelligence Applied to the Prediction of Organic Materials, Royal Society of Chemistry, Cambridge, 2020, ch. 12 Search PubMed
.
- M. Jansen and J. C. Schön, Rational development of new materials — putting the cart before the horse?, Nat. Mater., 2004, 3, 838 CAS
.
- L. Hawizy, D. M. Jessop, N. Adams and P. Murray-Rust, ChemicalTagger: A tool for semantic text-mining in chemistry, J. Cheminf., 2011, 3, 1–13 Search PubMed
.
- D. M. Lowe and R. A. Sayle, LeadMine: A grammar and dictionary driven approach to entity recognition, J. Cheminf., 2015, 7, 1–9 Search PubMed
.
- M. C. Swain and J. M. Cole, ChemDataExtractor: A Toolkit for Automated Extraction of Chemical Information from the Scientific Literature, J. Chem. Inf. Model., 2016, 56, 1894–1904 CrossRef CAS
.
- J. M. Cole, A Design-to-Device Pipeline for Data-Driven Materials Discovery, Acc. Chem. Res., 2020, 53, 599–610 CrossRef CAS
.
- E. Kim, K. Huang, A. Saunders, A. McCallum, G. Ceder and E. Olivetti, Materials Synthesis Insights from Scientific Literature via Text Extraction and Machine Learning, Chem. Mater., 2017, 29, 9436–9444 CrossRef CAS
.
- E. Kim, Z. Jensen, A. Van Grootel, K. Huang, M. Staib, S. Mysore, H. S. Chang, E. Strubell, A. McCallum, S. Jegelka and E. Olivetti, Inorganic Materials Synthesis Planning with Literature-Trained Neural Networks, J. Chem. Inf. Model., 2020, 60, 1194–1201 CrossRef CAS
.
- H. Huo, Z. Rong, O. Kononova, W. Sun, T. Botari, T. He, V. Tshitoyan and G. Ceder, Semi-supervised machine-learning classification of materials synthesis procedures, npj Comput. Mater., 2019, 5, 1–7 CrossRef
.
- L. Weston, V. Tshitoyan, J. Dagdelen, O. Kononova, A. Trewartha, K. A. Persson, G. Ceder and A. Jain, Named Entity Recognition
and Normalization Applied to Large-Scale Information Extraction from the Materials Science Literature, J. Chem. Inf. Model., 2019, 59, 3692–3702 CrossRef CAS
.
- V. Tshitoyan, J. Dagdelen, L. Weston, A. Dunn, Z. Rong, O. Kononova, K. A. Persson, G. Ceder and A. Jain, Unsupervised word embeddings capture latent knowledge from materials science literature, Nature, 2019, 571, 95–98 CrossRef CAS
.
- J. J. Irwin and B. K. Shoichet, ZINC - A free database of commercially available compounds for virtual screening, J. Chem. Inf. Model., 2005, 45, 177–182 CrossRef CAS
.
- T. Sterling and J. J. Irwin, ZINC 15 - Ligand Discovery for Everyone, J. Chem. Inf. Model., 2015, 55, 2324–2337 CrossRef CAS
.
- L. Ruddigkeit, R. Van Deursen, L. C. Blum and J. L. Reymond, Enumeration of 166 billion organic small molecules in the chemical universe database GDB-17, J. Chem. Inf. Model., 2012, 52, 2864–2875 CrossRef CAS
.
- S. H. Bertz, The First General Index of Molecular Complexity, J. Am. Chem. Soc., 1981, 103, 3599–3601 CrossRef CAS
.
- H. W. Whitlock, On the structure of total synthesis of complex natural products, J. Org. Chem., 1998, 63, 7982–7989 CrossRef CAS
.
- P. Ertl and A. Schuffenhauer, Estimation of synthetic accessibility score of drug-like molecules based on molecular complexity and fragment contributions, J. Cheminf., 2009, 1, 1–11 Search PubMed
.
- K. Boda and A. P. Johnson, Molecular complexity analysis of de novo designed ligands, J. Med. Chem., 2006, 49, 5869–5879 CrossRef CAS
.
- K. Boda, T. Seidel and J. Gasteiger, Structure and reaction based evaluation of synthetic accessibility, J. Comput.-Aided Mol. Des., 2007, 21, 311–325 CrossRef CAS
.
- Y. Takaoka, Y. Endo, S. Yamanobe, H. Kakinuma, T. Okubo, Y. Shimazaki, T. Ota, S. Sumiya and K. Yoshikawa, Development of a method for evaluating drug-likeness and ease of synthesis using a data set in which compounds are assigned scores based on chemists' intuition, J. Chem. Inf. Comput. Sci., 2003, 43, 1269–1275 CrossRef CAS
.
- R. P. Sheridan, N. Zorn, E. C. Sherer, L. C. Campeau, C. Chang, J. Cumming, M. L. Maddess, P. G. Nantermet, C. J. Sinz and P. D. O'Shea, Modeling a crowdsourced definition of molecular complexity, J. Chem. Inf. Model., 2014, 54, 1604–1616 CrossRef CAS
.
- C. W. Coley, L. Rogers, W. H. Green and K. F. Jensen, SCScore: Synthetic Complexity Learned from a Reaction Corpus, J. Chem. Inf. Model., 2018, 58, 252–261 CrossRef CAS
.
- M. H. S. Segler, M. Preuss and M. P. Waller, Planning chemical syntheses with deep neural networks and symbolic AI, Nature, 2018, 555, 604–610 CrossRef CAS
.
- P. Schwaller, T. Laino, T. Gaudin, P. Bolgar, C. A. Hunter, C. Bekas and A. A. Lee, Molecular Transformer: A Model for Uncertainty-Calibrated Chemical Reaction Prediction, ACS Cent. Sci., 2019, 5, 1572–1583 CrossRef CAS
.
- J. S. Schreck, C. W. Coley and K. J. M. Bishop, Learning Retrosynthetic Planning through Simulated Experience, ACS Cent. Sci., 2019, 5, 970–981 CrossRef CAS
.
- T. Klucznik, B. Mikulak-Klucznik, M. P. McCormack, H. Lima, S. Szymkuć, M. Bhowmick, K. Molga, Y. Zhou, L. Rickershauser, E. P. Gajewska, A. Toutchkine, P. Dittwald, M. P. Startek, G. J. Kirkovits, R. Roszak, A. Adamski, B. Sieredzińska, M. Mrksich, S. L. J. Trice and B. A. Grzybowski, Efficient Syntheses of Diverse, Medicinally Relevant Targets Planned by Computer and Executed in the Laboratory, Chem, 2018, 4, 522–532 CAS
.
- C. W. Coley, L. Rogers, W. H. Green and K. F. Jensen, Computer-Assisted Retrosynthesis Based on Molecular Similarity, ACS Cent. Sci., 2017, 3, 1237–1245 CrossRef CAS
.
- W. Gao and C. W. Coley, The Synthesizability of Molecules Proposed by Generative Models, J. Chem. Inf. Model., 2020 DOI:10.1021/acs.jcim.0c00174
.
- V. Santolini, M. Miklitz, E. Berardo and K. E. Jelfs, Topological landscapes of porous organic cages, Nanoscale, 2017, 9, 5280–5298 RSC
.
- A. R. Oganov, C. J. Pickard, Q. Zhu and R. J. Needs, Structure prediction drives materials discovery, Nat. Rev. Mater., 2019, 4, 331–348 CrossRef
.
- A. R. Oganov, A. O. Lyakhov and M. Valle, How evolutionary crystal structure prediction works-and why, Acc. Chem. Res., 2011, 44, 227–237 CrossRef CAS
.
- L. J. Abbott, K. E. Hart and C. M. Colina, Polymatic: A generalized simulated polymerization algorithm for amorphous polymers, Theor. Chem. Acc., 2013, 132, 1–19 Search PubMed
.
- V. L. Deringer, N. Bernstein, A. P. Bartók, M. J. Cliffe, R. N. Kerber, L. E. Marbella, C. P. Grey, S. R. Elliott and G. Csányi, Realistic Atomistic Structure of Amorphous Silicon from Machine-Learning-Driven Molecular Dynamics, J. Phys. Chem. Lett., 2018, 9, 2879–2885 Search PubMed
.
- M. Jansen, A Concept for Synthesis Planning in Solid-State Chemistry, Angew. Chem., Int. Ed., 2002, 41, 3746–3766 CrossRef CAS
.
- P. Villars, K. Brandenburg, M. Berndt, S. LeClair, A. Jackson, Y. H. Pao, B. Igelnik, M. Oxley, B. Bakshi, P. Chen and S. Iwata, Binary, ternary and quaternary compound former/nonformer prediction via Mendeleev number, J. Alloys Compd., 2001, 317–318, 26–38 CrossRef CAS
.
- C. M. Freeman, J. M. Newsam, S. M. Levine and C. R. A. Catlow, Inorganic crystal structure prediction using simplified potentials and experimental unit cells: Application to the polymorphs of titanium dioxide, J. Mater. Chem., 1993, 3, 531–535 RSC
.
- M. U. Schmidt and U. Englert, Prediction of crystal structures, J. Chem. Soc., Dalton Trans., 1996, 2077–2082 RSC
.
- C. J. Pickard and R. J. Needs,
Ab initio random structure searching, J. Phys.: Condens. Matter, 2011, 23(5), 053201 CrossRef
.
- J. Pannetier, J. Bassas-Alsina, J. Rodriguez-Carvajal and V. Caignaert, Prediction of crystal structures from crystal chemistry rules by simulated annealing, Nature, 1990, 346, 343–345 CrossRef CAS
.
- J. C. Schön and M. Jansen, First Step Towards Planning of Syntheses in Solid-State Chemistry: Determination of Promising Structure Candidates by Global Optimization, Angew. Chem., Int. Ed., 1996, 35, 1286–1304 CrossRef
.
- D. J. Wales and J. P. K. Doye, Global optimization by basin-hopping and the lowest energy structures of Lennard-Jones clusters containing up to 110 atoms, J. Phys. Chem. A, 1997, 101, 5111–5116 CrossRef CAS
.
- S. Goedecker, Minima hopping: An efficient search method for the global minimum of the potential energy surface of complex molecular systems, J. Chem. Phys., 2004, 120, 9911–9917 CrossRef CAS
.
- R. Martoňák, A. Laio and M. Parrinello, Predicting Crystal Structures: The Parrinello-Rahman Method Revisited, Phys. Rev. Lett., 2003, 90, 075503 CrossRef
.
- A. R. Oganov and C. W. Glass, Crystal structure prediction using ab initio evolutionary techniques: Principles and applications, J. Chem. Phys., 2006, 124, 244704 CrossRef
.
- T. S. Bush, C. R. A. Catlow and P. D. Battle, Evolutionary programming techniques for predicting inorganic crystal structures, J. Mater. Chem., 1995, 5, 1269 RSC
.
- S. M. Woodley, P. D. Battle, J. D. Gale and C. R. A. Catlow, The prediction of inorganic crystal structures using a genetic algorithm and energy minimisation, Phys. Chem. Chem. Phys., 1999, 1, 2535–2542 RSC
.
- G. Férey, Hybrid porous solids: past, present, future, Chem. Soc. Rev., 2008, 37, 191–214 RSC
.
- A. Belsky, M. Hellenbrandt, V. L. Karen and P. Luksch, New developments in the Inorganic Crystal Structure Database (ICSD): accessibility in support of materials research and design, Acta Crystallogr., Sect. B: Struct. Sci., 2002, 58, 364–369 CrossRef
.
- C. R. Groom, I. J. Bruno, M. P. Lightfoot and S. C. Ward, The Cambridge Structural Database, Acta Crystallogr., Sect. B: Struct. Sci., Cryst. Eng. Mater., 2016, 72, 171–179 CrossRef CAS
.
- V. I. Hegde, M. Aykol, S. Kirklin and C. Wolverton, The phase stability network of all inorganic materials, Sci. Adv., 2020, 6, eaay5606 CrossRef CAS
.
- J. Buckeridge, D. O. Scanlon, A. Walsh and C. R. A. Catlow, Automated procedure to determine the thermodynamic stability of a material and the range of chemical potentials necessary for its formation relative to competing phases and compounds, Comput. Phys. Commun., 2014, 185, 330–338 CrossRef CAS
.
- K. T. Butler, D. W. Davies, H. Cartwright, O. Isayev and A. Walsh, Machine learning for molecular and materials science, Nature, 2018, 559, 547–555 CrossRef CAS
.
- S. A. Lopez, B. Sanchez-Lengeling, J. de Goes Soares and A. Aspuru-Guzik, Design Principles and Top Non-Fullerene Acceptor Candidates for Organic Photovoltaics, Joule, 2017, 1, 857–870 CrossRef CAS
.
- S. Curtarolo, G. L. W. Hart, M. B. Nardelli, N. Mingo, S. Sanvito and O. Levy, The high-throughput highway to computational materials design, Nat. Mater., 2013, 12, 191–201 CrossRef CAS
.
- S. Curtarolo, D. Morgan, K. Persson, J. Rodgers and G. Ceder, Predicting Crystal Structures with Data Mining of Quantum Calculations, Phys. Rev. Lett., 2003, 91, 135503 CrossRef
.
- A. Jain, Y. Shin and K. A. Persson, Computational predictions of energy materials using density functional theory, Nat. Rev. Mater., 2016, 1, 1–13 Search PubMed
.
- V. L. Deringer, D. M. Proserpio, G. Csányi and C. J. Pickard, Data-driven learning and prediction of inorganic crystal structures, Faraday Discuss., 2018, 211, 45–59 RSC
.
- J. Noh, J. Kim, H. S. Stein, B. Sanchez-Lengeling, J. M. Gregoire, A. Aspuru-Guzik and Y. Jung, Inverse Design of Solid-State Materials via a Continuous Representation, Matter, 2019, 1, 1370–1384 CrossRef
.
- S. Kim, J. Noh, G. H. Gu, A. Aspuru-Guzik and Y. Jung, Generative Adversarial Networks for Crystal Structure Prediction, ACS Cent. Sci., 2020, 6, 1412–1420 CrossRef CAS
.
- M. S. Dyer, C. Collins, D. Hodgeman, P. A. Chater, A. Demont, S. Romani, R. Sayers, M. F. Thomas, J. B. Claridge, G. R. Darling and M. J. Rosseinsky, Computationally Assisted Identification of Functional Inorganic Materials, Science, 2013, 340, 847–852 CrossRef CAS
.
- C. Collins, M. S. Dyer, A. Demont, P. A. Chater, M. F. Thomas, G. R. Darling, J. B. Claridge and M. J. Rosseinsky, Computational prediction and experimental confirmation of B-site doping in YBa2Fe3O8, Chem. Sci., 2014, 5, 1493–1505 RSC
.
- C. A. Tzitzeklis, J. K. Gupta, M. S. Dyer, T. D. Manning, M. J. Pitcher, H. J. Niu, S. Savvin, J. Alaria, G. R. Darling, J. B. Claridge and M. J. Rosseinsky, Computational Prediction and Experimental Realization of p-Type Carriers in the Wide-Band-Gap Oxide SrZn1−xLixO2, Inorg. Chem., 2018, 57, 11874–11883 CrossRef CAS
.
- O. D. Friedrichs, A. W. M. Dress, D. H. Huson, J. Klinowski and A. L. Mackay, Systematic enumeration of crystalline networks, Nature, 1999, 400, 644–647 CrossRef CAS
.
- C. Mellot-Draznieks, S. Girard, G. Férey, J. C. Schön, Z. Cancarevic and M. Jansen, Computational design and prediction of interesting not-yet-synthesized structures of inorganic materials by using building unit concepts, Chem.–Eur. J., 2002, 8, 4102–4113 CrossRef CAS
.
- M. A. Zwijnenburg, S. T. Bromley, M. D. Foster, R. G. Bell, O. Delgado-Friedrichs, J. C. Jansen and T. Maschmeyer, Toward understanding the thermodynamic viability of zeolites and related frameworks through a simple topological model, Chem. Mater., 2004, 16, 3809–3820 CrossRef CAS
.
- D. W. Lewis, D. J. Willock, C. R. A. Catlow, J. M. Thomas and G. J. Hutchings, De novo design of structure-directing agents for the synthesis of microporous solids, Nature, 1996, 382, 604–606 CrossRef CAS
.
- D. Fujita, Y. Ueda, S. Sato, N. Mizuno, T. Kumasaka and M. Fujita, Self-assembly of tetravalent Goldberg polyhedra from 144 small components, Nature, 2016, 540, 563–566 CrossRef CAS
.
- W. M. Bloch, J. J. Holstein, B. Dittrich, W. Hiller and G. H. Clever, Hierarchical Assembly of an Interlocked M8L16 Container, Angew. Chem., Int. Ed., 2018, 57, 5534–5538 CrossRef CAS
.
- C. E. Wilmer, M. Leaf, C. Y. Lee, O. K. Farha, B. G. Hauser, J. T. Hupp and R. Q. Snurr, Large-scale screening of hypothetical metal-organic frameworks, Nat. Chem., 2012, 4, 83–89 CrossRef CAS
.
- V. Abet, F. T. Szczypiński, M. A. Little, V. Santolini, C. D. Jones, R. Evans, C. Wilson, X. Wu, M. F. Thorne, M. J. Bennison, P. Cui, A. I. Cooper, K. E. Jelfs and A. G. Slater, Inducing Social Self-Sorting in Organic Cages To Tune The Shape of The Internal Cavity, Angew. Chem., Int. Ed., 2020, 59, 16755–16763 CrossRef CAS
.
- S. Lee, A. Yang, T. P. Moneypenny and J. S. Moore, Kinetically Trapped Tetrahedral Cages via Alkyne Metathesis, J. Am. Chem. Soc., 2016, 138, 2182–2185 CrossRef CAS
.
- A. Yang, J. S. Moore, T. J. Woods, T. P. Moneypenny, N. P. Walter, Y. Zhang and D. L. Gray, Product Distribution from Precursor Bite Angle Variation in Multitopic Alkyne Metathesis: Evidence for a Putative Kinetic Bottleneck, J. Am. Chem. Soc., 2018, 140, 5825–5833 CrossRef
.
- R. L. Greenaway, V. Santolini, M. J. Bennison, B. M. Alston, C. J. Pugh, M. A. Little, M. Miklitz, E. G. B. Eden-Rump, R. Clowes, A. Shakil, H. J. Cuthbertson, H. Armstrong, M. E. Briggs, K. E. Jelfs and A. I. Cooper, High-throughput discovery of organic cages and catenanes using computational screening fused with robotic synthesis, Nat. Commun., 2018, 9, 2849 CrossRef CAS
.
- S. Steiner, J. Wolf, S. Glatzel, A. Andreou, J. M. Granda, G. Keenan, T. Hinkley, G. Aragon-Camarasa, P. J. Kitson, D. Angelone and L. Cronin, Organic synthesis in a modular robotic system driven by a chemical programming language, Science, 2019, 363, eaav2211 CrossRef CAS
.
- S. M. Moosavi, A. Chidambaram, L. Talirz, M. Haranczyk, K. C. Stylianou and B. Smit, Capturing chemical intuition in synthesis of metal-organic frameworks, Nat. Commun., 2019, 10, 1–7 CrossRef
.
- S. L. Price, Predicting
crystal structures of organic compounds, Chem. Soc. Rev., 2014, 43, 2098–2111 RSC
.
- A. J. Cruz-Cabeza, S. M. Reutzel-Edens and J. Bernstein, Facts and fictions about polymorphism, Chem. Soc. Rev., 2015, 44, 8619–8635 RSC
.
- J. Yang, S. De, J. E. Campbell, S. Li, M. Ceriotti and G. M. Day, Large-Scale Computational Screening of Molecular Organic Semiconductors Using Crystal Structure Prediction, Chem. Mater., 2018, 30, 4361–4371 CrossRef CAS
.
- A. G. Slater and A. I. Cooper, Function-led design of new porous materials, Science, 2015, 348, aaa8075 CrossRef
.
- T. Hasell and A. I. Cooper, Porous organic cages: Soluble, modular and molecular pores, Nat. Rev. Mater., 2016, 1(9), 16053 CrossRef CAS
.
- A. Pulido, L. Chen, T. Kaczorowski, D. Holden, M. A. Little, S. Y. Chong, B. J. Slater, D. P. McMahon, B. Bonillo, C. J. Stackhouse, A. Stephenson, C. M. Kane, R. Clowes, T. Hasell, A. I. Cooper and G. M. Day, Functional materials discovery using energy-structure-function maps, Nature, 2017, 543, 657–664 CrossRef CAS
.
- R. L. Greenaway, V. Santolini, A. Pulido, M. A. Little, B. M. Alston, M. E. Briggs, G. M. Day, A. I. Cooper and K. E. Jelfs, From Concept to Crystals via Prediction: Multi-Component Organic Cage Pots by Social Self-Sorting, Angew. Chem., Int. Ed., 2019, 58, 16275–16281 CrossRef CAS
.
- L. Bouëssel Du Bourg, A. U. Ortiz, A. Boutin and F. X. Coudert, Thermal and mechanical stability of zeolitic imidazolate frameworks polymorphs, APL Mater., 2014, 2(12), 124110 CrossRef
.
- Y. G. Chung, J. Camp, M. Haranczyk, B. J. Sikora, W. Bury, V. Krungleviciute, T. Yildirim, O. K. Farha, D. S. Sholl and R. Q. Snurr, Computation-Ready, Experimental Metal–Organic Frameworks: A Tool To Enable High-Throughput Screening of Nanoporous Crystals, Chem. Mater., 2014, 26, 6185–6192 CrossRef CAS
.
- Y. G. Chung, E. Haldoupis, B. J. Bucior, M. Haranczyk, S. Lee, H. Zhang, K. D. Vogiatzis, M. Milisavljevic, S. Ling, J. S. Camp, B. Slater, J. I. Siepmann, D. S. Sholl and R. Q. Snurr, Advances, Updates, and Analytics for the Computation-Ready, Experimental Metal–Organic Framework Database: CoRE MOF 2019, J. Chem. Eng. Data, 2019, 64, 5985–5998 CrossRef CAS
.
- P. Z. Moghadam, A. Li, S. B. Wiggin, A. Tao, A. G. P. Maloney, P. A. Wood, S. C. Ward and D. Fairen-Jimenez, Development of a Cambridge Structural Database Subset: A Collection of Metal-Organic Frameworks for Past, Present, and Future, Chem. Mater., 2017, 29, 2618–2625 CrossRef CAS
.
|
This journal is © The Royal Society of Chemistry 2021 |
Click here to see how this site uses Cookies. View our privacy policy here.