DOI:
10.1039/D1RA03008F
(Review Article)
RSC Adv., 2021,
11, 30426-30447
The exposome paradigm to predict environmental health in terms of systemic homeostasis and resource balance based on NMR data science
Received
18th April 2021
, Accepted 31st August 2021
First published on 13th September 2021
Abstract
The environment, from microbial ecosystems to recycled resources, fluctuates dynamically due to many physical, chemical and biological factors, the profile of which reflects changes in overall state, such as environmental illness caused by a collapse of homeostasis. To evaluate and predict environmental health in terms of systemic homeostasis and resource balance, a comprehensive understanding of these factors requires an approach based on the “exposome paradigm”, namely the totality of exposure to all substances. Furthermore, in considering sustainable development to meet global population growth, it is important to gain an understanding of both the circulation of biological resources and waste recycling in human society. From this perspective, natural environment, agriculture, aquaculture, wastewater treatment in industry, biomass degradation and biodegradable materials design are at the forefront of current research. In this respect, nuclear magnetic resonance (NMR) offers tremendous advantages in the analysis of samples of molecular complexity, such as crude bio-extracts, intact cells and tissues, fibres, foods, feeds, fertilizers and environmental samples. Here we outline examples to promote an understanding of recent applications of solution-state, solid-state, time-domain NMR and magnetic resonance imaging (MRI) to the complex evaluation of organisms, materials and the environment. We also describe useful databases and informatics tools, as well as machine learning techniques for NMR analysis, demonstrating that NMR data science can be used to evaluate the exposome in both the natural environment and human society towards a sustainable future.
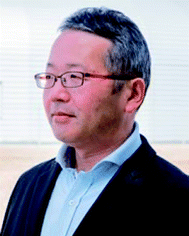 Jun Kikuchi | Jun Kikuchi is a Team Leader of the Environmental Metabolic Analysis Research Team of the RIKEN Center for Sustainable Resource Science, as well as a Visiting Professor of Nagoya University and Yokohama City University. He obtained his PhD from the Tokyo University of Agriculture and Technology, and continues to pursue methodological advancement in NMR analysis ranging from purified proteins to complex metabolites and macromolecular mixture samples. Recently, his research fields have expanded to include complex systems, such as metabolic interactions between a symbiotic microbe and host, naturally collected algae and fish biodiversity, environmental soils, sediments and water systems, and industrial metabolic reactions such as food fermentation, biomass degradation processes and biodegradable polymers. Analysis of these complex systems has led to various data science approaches to compute multiple factors and variables. |
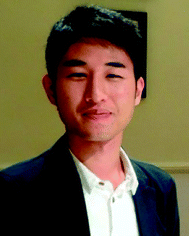 Shunji Yamada | Shunji Yamada is a Postdoctoral Researcher of the Environmental Metabolic Analysis Research Team of the RIKEN Center for Sustainable Resource Science as well as Prediction Science Laboratory, RIKEN Cluster for Pioneering Research, and Data Assimilation Research Team, RIKEN Center for Computational Science. He obtained his PhD from Nagoya University under the supervision of Professor Jun Kikuchi in 2021. Prior to this, he studied NMR data science to evaluate the impact on the immune system of metabolites produced by probiotics under the supervision of Professor Hiroshi Ohno at Yokohama City University from 2010 to 2012. He also worked on drug informatics at Kowa Company, Ltd, from 2012 to 2017. His current research interests include NMR informatics approaches for the analysis of molecular complexity, including materials and the metabolome, towards the dynamic equilibrium of resources and ecosystems. |
1. Introduction
The environment, from microbial ecosystems and materials to macrosystems such as the earth and its inhabitants, fluctuates within certain bounds due to many physical, chemical and biological factors. Within an “environmental system”, fluctuations in these factors at a certain level can be used to evaluate systemic homeostasis.1 For example, a human can be thought of as a human–microbe hybrid or “superorganism”, whose homeostasis (i.e., healthy state) will be affected by (1) intrinsic properties,2 (2) the “exposome” – namely, the totality of human physical, chemical and biological factors such as lifestyle choices (e.g., food, drink and drug intake), and (3) the acquisition of a stable ‘‘healthy’’ symbiotic microbe (the so-called “microbiome”).3 Alterations in this superorganism will be manifested in the metabolic balance within human samples, such as serum, urine and faeces.4 Similarly, natural ecosystems may be conceived of as interconnected environmental and metabolic systems; for example, carbon, nitrogen and phosphorus fluxes are biologically driven through ecosystems on a global scale, and also through biochemical pathways on an organismal or population scale. Therefore, naturally collected samples, including soil, sediment, water, algae, plants, fish and materials, contain much information on homeostatic fluctuations – in other words, the metabolic balance – within an ecosystem of interest.5 With the aim towards a sustainable society, here we propose a shift to a research approach based on the exposome paradigm with a focus on nuclear magnetic resonance (NMR) data science to predict environmental health in terms of systemic homeostasis and resource balance. We first summarize the current status of world population growth and prospects for sustainable development (Section 1), and then outline current technology in NMR data science (Section 2). As examples of the application of NMR data science, we outline issues and proposals across human society in diverse fields such as the natural environment, agriculture, aquaculture, wastewater treatment in the industry, biomass decomposition and biodegradable plastics (Section 3).
1.1 Global human population growth and sustainable development
According to the statistical report of the Food and Agriculture Organization (FAO) of the United Nations, since 2010 the world population and production of agricultural, livestock and marine products has been rising (Fig. 1). In the future, the world population and the number of macro- and microplastics in the environment are expected to increase further.6 Therefore, sustainable development of the world is one of the major challenges of our society. By 2050, we will need to provide affluent lives to an estimated population of over 9 billion and build a sustainable society that takes into account the circulation of natural resources.7 To increase production while lowering the impact on the environment, advances in science and technology to increase production capacity, and reduce or reuse waste from agriculture, aquaculture and industry, are required. Reducing waste is one way to meet demand without increasing production. Food fermentation techniques offer the potential to extend the shelf life of products and create new value-added food ingredients.8 From the viewpoint of waste reuse, energy and polymer materials can be produced from biomass.9 Sustainable diets will need to protect biodiversity and the environment, to optimize natural resources, and to be culturally acceptable, accessible and affordable to various populations, while being safe and nutritious.10 A shift towards lower meat consumption is one strategy to reduce the loss of biodiversity and to offset the effects of climate change,11 but alternative sustainable, non-animal sources of protein must be developed.12 Currently, vegetal sources dominate the global protein supply. From a health perspective, fish, algae and insects offer attractive alternatives to animal protein. Clearly these challenges are complex‚ requiring a focus on both human and earth health. These topics are important, but they require a very broad perspective and have not been considered from a bird's-eye view. In addressing them, “the exposome paradigm-based NMR approach” has many uses that can probe molecular complexity, including samples from natural ecosystems, agriculture, aquaculture and polymer materials development.13
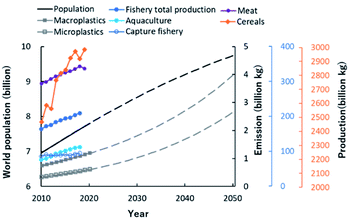 |
| Fig. 1 Global changes in population growth to 2050, and food production and emission of plastics to 2020. Values of world population, productions of meat and cereals were taken from FAOSTAT database (http://www.fao.org/faostat/en/#home) of Food and Agriculture Organization (FAO) of the United Nations. Emissions of macroplastics and microplastics were taken from the paper of Lebreton et al.6 The dotted lines of world population, macroplastics and microplastics are the predicted values. Values of fishery total production, aquaculture production and capture fishery production were taken from databases (http://www.fao.org/fishery/statistics/en) of the FAO Fisheries Division. | |
1.2 Circulation of biological resources and water, and waste recycling in human society
Throughout human history, useful substances such as food, wood and oil have been produced from organisms in the land sphere and the hydrosphere. Between the ecosphere and the humanosphere, it is expected that recycling resources of molecular complexity, such as water, foods, natural resources and plastics, will be necessary to achieve Sustainable Development Goals (Fig. 2). In recent years, our oceans are faced with the problem of red tide due to the inflow of industrial wastewater and pesticides, and the environmental load from aquaculture. Moreover, human society is faced with the need for alternative resources to petroleum and the problem of microplastics due to the outflow of plastics to the environment. NMR will be useful in addressing some of these problems. It can analyse diverse biological and environmental samples and various materials, producing multiple data with inter-institution compatibility that are suitable for data science using informatics technics such as multivariate analysis, machine learning and database creation.13 By coupling NMR with techniques such as inductivity coupled plasma optical emission spectrometry (ICP-OES), thermal analysis and next-generation sequencing (NGS), it is possible to collect data on chemical, physical and microbial factors.14 Furthermore, the ecological environment will be improved by predicting and giving early warning of environmental changes, and by controlling key factors before the balance of the ecosystem is lost due to changes in these factors.15,16
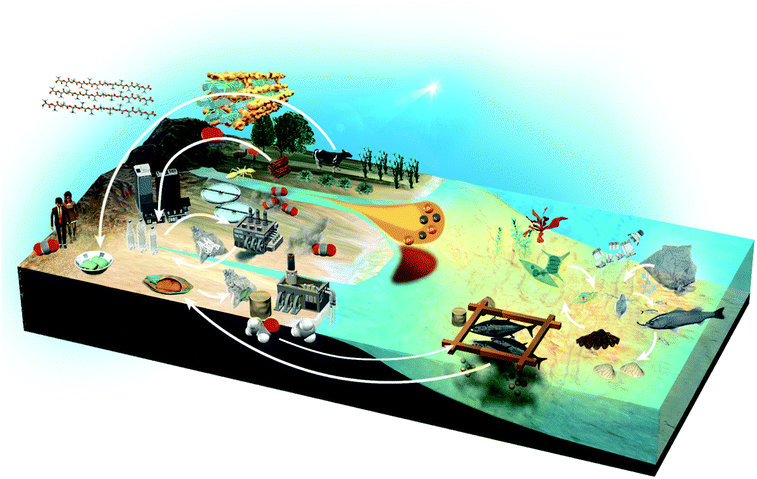 |
| Fig. 2 Recycling of sustainable resources between the ecosphere and humanosphere. Shown is a schematic summary of research broadly aimed at resource circulation of the global environment and human society. It is expected that recycling resources of molecular complexity, such as water, foods, natural resources and plastics will be necessary to achieve Sustainable Development Goals. | |
2. NMR data science approaches
2.1 Investigation of environmental homeostasis
“Exposome changes”, such as human lifestyle choices and environmental alterations in the ecosystem, will be manifested in the metabolic profile of human and environmental samples. NMR-based metabolic balance analysis (i.e., pattern recognition of metabolic profiles based on NMR spectroscopy of complex-mixture samples) can identify potential metabolites as diagnostic biomarkers associated with homeostasis as “environmental health” (Fig. 3). As mentioned in the Introduction, biologically driven carbon flows through ecosystems on a global level, but also through biochemical pathways at the organismal and population levels. The significant increase in carbon emission from soil in the past 40 years is reported to have made a small contribution to global climate change.17 A more direct contribution of human activity to metabolic homeostasis results from resource extraction by humans. For example, overfishing can completely restructure patterns across trophic levels in coastal ecosystems.18 Advances in agricultural/fishery science are providing approaches to overcome food shortages arising from population growth in developing countries.19
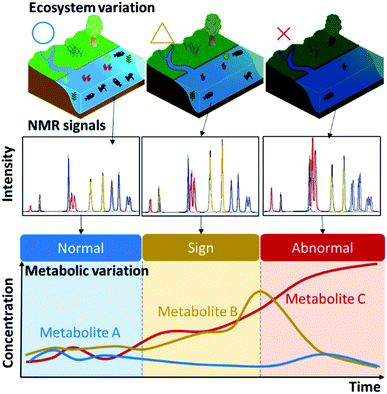 |
| Fig. 3 Conceptual diagram of metabolic profiling using NMR spectra for evaluating homeostasis in ecosystems. Top, the schematic diagram shows collapse of an environmental ecosystem. Middle, the NMR signals of samples from environments can be profiled as a relative intensity change. Bottom, the corresponding variation of three states (normal, sign, and abnormal) are illustrated by hypothetical fluctuations in the concentrations of three metabolites (metabolite A, blue; metabolite B, yellow; and metabolite C, red). | |
Naturally collected samples, including soil, sediment, water, algae and fish, contain much information on homeostatic fluctuations or the metabolic balance of an ecosystem of interest.5 Based on reports of the direct and indirect effects of agriculture, forestry and fisheries on natural ecosystems that produce biomass resources, we propose application of a recent analytical paradigm20–29 to ecosystem research. As a specific example, we will consider changes in homeostasis in the coastal environment. Oceans cover 70% of the earth's surface, but the coastal environment is strongly influenced by human activities.30 In aquaculture, for example, fishery products are grown at high density and fed with large amounts of nitrogen/phosphorus nutrient-rich feed to enable growth in a short period of time,31 but most of the feed is released into the ocean without being metabolized. In a eutrophic environment, this can cause red tides and blue tides, which ultimately have a strong, negative impact on the fishery industry. Therefore, a method for predicting red and blue tides is an important research area. In addition to regular seawater sampling and metabolic profiling, we have developed a method for visualizing which factors are important by mapping various metabolites along with physical factors such as water temperature.14 In addition, it has been shown that fish naturally have different metabolic and microbiota profiles, depending on diet and growing environment, which can be discriminated by modelling based on machine learning.32–34
2.2 NMR techniques
NMR data science involves analytical cycles based on the concept of measurement informatics, which consists of sample preparation,35 NMR measurement,36 database construction, data pre-processing and data analysis (Fig. 4). There are several types of NMR, which facilitate the study of chemical structure and dynamics. NMR can evaluate the components of mixtures from an exposome perspective. In addition, solid-state NMR can evaluate the higher-order structure of solid samples. High-field NMR is used for advanced analysis such as multidimensional NMR and solid-state NMR at the research level, while low-field NMR is used for simple measurements in the field. Regardless of approach, the measured data are stored in databases. As a result, chemical shifts in NMR spectra of samples can be assigned by reference to the spectral data of standard samples stored in databases. In the case of complex mixtures, the data are pre-processed to simplify multi-component signals. The processed data are then evaluated by data-driven analysis. Lastly, based on the evaluation and prediction, sample preparation is optimized through trial-and-error experiments.
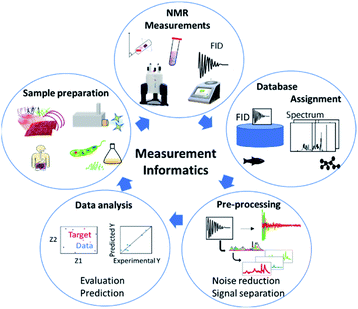 |
| Fig. 4 Strategy for the analysis of complex molecular systems by NMR. Shown is the concept of measurement informatics, which consists of a cycle of sample preparation, NMR measurement, database construction, data pre-processing and data analysis. | |
2.2.1 Solution-NMR. Recently, solution NMR has been applied to metabolic profiling, whereby NMR spectra of mixtures of small biological molecules are subjected to multivariate analysis to identify metabolite biomarkers,37–41 or to evaluate nutrients in food.22,42–44 Innovations in such non-targeted approaches to studying biological systems are important for improving biomass production and for better sustainability of these systems. Although one-dimensional (1D) solution NMR has been often used for metabolic analysis, the analysis of mixtures using traditional 1D NMR spectra is difficult because of signal overlap due to signal splitting caused by spin coupling. Therefore, multidimensional approaches to NMR-based metabolomic analysis have been applied.45 For example, two-dimensional (2D) J-resolved spectroscopy can be used to determine spin–spin coupling constants, which are then used for structural analysis.46 Solution-state multidimensional NMR has been also used to determine correlation signals in homo- and heteronuclear experiments to identify metabolites. In 1H–13C-heteronuclear single quantum coherence (HSQC), metabolites can be identified by comparison to a chemical shift database and previously reported HSQC spectra. To increase identification accuracy, 1H–1H correlation spectroscopy (COSY), 1H–1H totally correlated spectroscopy (TOCSY) and 1H–1H single quantum-double quantum correlation spectroscopy can be used to confirm correlation signals with adjacent atoms. By using HSQC-TOCSY and three-dimensional (3D) HCCH-COSY together, adjacent correlation signals are easily confirmed for annotated metabolites on HSQC spectra.47In solution NMR, solvent suppression is important for evaluating peaks of interest.48 In this regard, pure shift techniques yield a spectrum in which all the J coupling multiplets are collapsed into singlets, while ultra-clean pure shift 1H-NMR has been applied to metabolomics profiling.49 Furthermore, in metabolomic profiling of aqueous samples with 13C natural abundance, 2D real-time BIRD 1H–13C HSQC has been shown to improve spectral resolution in regions affected by spectral overlap.50 To achieve sufficient sensitivity and resolution for a sample of metabolomic interest, acquisition of a 2D spectrum can take from tens of minutes to several hours. To reduce experimental duration, non-uniform sampling and ultrafast NMR have successfully been used in targeted and untargeted metabolomics and lipidomics.51–53 Lastly, fast multi-scan single-shot COSY experiments have been applied to the quantification of major metabolites in biologically relevant samples.54
2.2.2 Solid-state NMR. Solid-state NMR spectroscopy provides information on native structures and dynamics useful for predicting and designing the physical properties of multi-component solid materials. It is being increasingly applied in material and life sciences.5,55 In the characterization of solid-state samples with crystalline, interphase and amorphous domains (i.e., disordered materials), the anisotropy detected by static measurements is useful.56 Many foods are solid or at least semi-solid, with restricted molecular motions relative to pure liquids.57 The most important technique is magic angle spinning (MAS), which uses a defined angle of 54.7° to eradicate the nuclear dipole–dipole interactions, chemical shift anisotropy and variations in magnetic susceptibility that cause line broadening in the NMR spectrum.58 Towards automated acquisition during variable-temperature, static and/or MAS NMR experiments, an external automatic tuning and matching (eATM) robot that can be attached to commercial and/or home-built MAS or static NMR probeheads has been developed.59 In both multidimensional and multinuclear spectroscopy, high-resolution-MAS (HR-MAS) can provide clear and informative molecular characterization of complex heterogeneous systems (e.g., soil organic matter and plant-derived materials) and help to unravel the environmental reactivity of inorganic and organic materials.60Plant biomass, which mainly comprises polysaccharides, is one of the most abundant biomaterials in nature. Determining the structure and composition of plant polysaccharides, which are categorized as storage polysaccharides such as starch61 and structural polysaccharides such as cellulose,62 is therefore one of main challenges of biomass analysis. We have acquired 1D and 2D MAS spectra in a cross-polarization (CP) time series and used peak fitting techniques to establish valid models of crystalline and amorphous cellulose,63,64 showing that matrix polysaccharides are composed of hemicelluloses and pectins. We have also developed methods for the chemical profiling of macroalgae by solution and solid-state NMR, Fourier transform-infrared (FT-IR) spectroscopy and ICP-OES.28 We combined these methods in an integrated analysis of 107 algal samples, using the multi-instrumental data derived to characterize the samples according to their chemical diversity. Correlation analysis yielded chemotaxonomic clusters based on chemical diversity that were consistent with genetic linkages. The integrated analysis also indicated a relationship between alginate and ions including cadmium and arsenic, which are toxic to mammals.21 We have also profiled the cellular biomacromolecules of Euglena gracilis, a flagellated protist that accumulates the polysaccharide paramylon in crystal form and triple-helical β-1,3-glucan as a storage polysaccharide, similar to brown algae.65 For this analysis, we developed a cellular NMR approach using cells of 13C and 15N-labelled E. gracilis without any purification or fractionation steps. The measurements included 2D-/3D-NMR pulse sequences of solid-state NMR (e.g., “incredible natural-abundance double-quantum transfer experiment” [INADEQUATE], second-order Hamiltonian among analogous nuclei plus [SHA+], and 3D dipolar-assisted rotational resonance [DARR]), which required considerable measurement time. To support the more rapid evaluation of macromolecular mixtures, we subsequently developed the webtool InterSpin, which integrates the programs SpinMacro for the assignment of macromolecules, and PeaK SeParation (PKSP) and SENsitivity improvement with Spectral Integration (SENSI) for the separation of broad spectra.66
2.2.3 Time-domain NMR and MRI. Time-domain NMR and relaxometry with low-field and compact NMR instruments has several advantages, including simple sample preparation, easy handling and relatively low costs.67,68 This is a useful approach to evaluate the crystalline state69 and determine carbon chain lengths of fatty acid mixtures.70 As a result, NMR-based relaxometry is widely used in various industries and research fields such as plants,71 point-of-care testing,72 food73,74 and materials.75,76 For the characterization of constituents in complex materials using low-resolution NMR relaxometry data, Laplace inversion using sparse representation methods has been applied.77 Applications of low-field benchtop NMR include continuous-flow process monitoring,78–81 food82 and metabolomics analysis.83 Food evaluation by foodpro84 is also an important technique using benchtop-NMR for taste discrimination in food samples. Benchtop-NMR has been also applied as part of an organic synthesis robot with machine learning to search for new reactivity.85 Overall, a range of challenging wide-line solid-state NMR spectra can be acquired by a maintenance-free, low-cost benchtop/mobile NMR spectrometer.86In spatial encoding and spatial selection methods, several sub-experiments are conducted in parallel in different spatial regions of the sample spectra.87,88 As an example of spatial methods, magnetic resonance imaging (MRI) can be used for the noninvasive evaluation of spatial 1H density, diffusion or relaxation, and chemical shift in biological and other samples. It is also put to practical use in the medical field as a powerful technique for diagnostic imaging. We have observed intact textural features by using 1D MRI and proposed new pulse sequences, collectively named spatial molecular-dynamically ordered spectroscopy (SMOOSY), for NMR measurements driven by diffusion, relaxation and REST encoding.89 In this approach, pseudo-3D SMOOSY spectra similar to MRI spectra are recorded. We have applied SMOOSY to non-invasive imaging of the shrimp body and two heterogeneous systems, showing that pseudo-2D SMOOSY spectral images can be used to assess the different dynamics of compounds at each spatial z-position of the samples.
2.3 NMR-based profiling methods
Because experimentally obtained NMR spectra might include useful and valuable information (relationships between different samples) that is not obvious, data science approaches, such as multivariate statistical analysis90 and machine learning, should be incorporated into the analytical flow of metabolomics studies. Within the analytical flow, data pre-processing steps are very important91 and typically include baseline correction, alignment, binning, normalization and scaling.92 Correction of baseline distortions93 and peak alignment94 are necessary steps in spectral processing because these factors affect intensity values and result in inaccuracy in peak assignment and quantification. In the spectral bucketing or binning approach, the spectrum is split into small bins or buckets containing a range of variations for a specific peak shift, and the intensity of each bucket is calculated as the area under the curve.95 Scaling and normalization are also important in data pre-processing because extreme differences in scales among multiple parameters can obscure the data, leading to inadequate interpretation.96 Typically, an internal standard, such as 4,4-dimethyl-4-silapentane-1-sulfonic acid, is used to normalize datasets. Alternatively, z-scoring uses a standard normal distribution with the average set as 0 and the standard deviation as 1;97 when analysing an entire spectrum, however, z-scoring is problematic in that it also normalizes noise. Lastly, the method of probabilistic quotient normalization is not susceptible to outliers because the median of numerous estimated values is used.98
An essential component of NMR metabolomic studies is multivariate analysis, with methods falling roughly into two categories: unsupervised (e.g., principle component analysis, PCA99) and supervised (e.g., partial least squares, PLS100).101 PCA is the most widely used method for data overview and trend (or cluster) identification in metabolomics, but it is also used to reduce dimensions in dataset pre-processing. Other representative, unsupervised, multivariate analyses include hierarchal clustering,27 k-means clustering102 and self-organizing maps.103 Correlation-based analysis is also widely used in metabolomics studies.104 In addition, market basket analysis, a statistical approach for identifying the co-occurrence of variables in datasets, has been applied to these studies.105
Importantly, in terms of the exposome, metabolomics has great potential in the diagnosis and monitoring of disease using samples such as blood, saliva, urine and faeces. Recently, NMR metabolomic analysis of the human superorganism has provided insight into interactions between the host and intestinal microorganisms during the establishment of immunological homeostasis.106–109 In addition, a Markov blanket-based feature selection method with an ecological–chemical–physical integrated network based on a Bayesian network inference algorithm has provided valuable information on fish home-range, and indicated that chemical and physical characterization of fish muscle can serve as an indicator for fish ecotyping and human impact monitoring.110 Lastly, evaluation of environmental homeostasis, which is growing in importance with the global decline in environmental health, has been evaluated on multiple levels using NMR,26,111–115 while metabolomics analysis has been reported for diverse samples,116–130 including food,131,132 plant,133–137 animal,138 human,92,139 bacterial,140 virus141 and environmental samples.142,143
2.4 NMR databases and analytical tools
In NMR data-driven science, spectrum processing, data pre-processing, data analysis and signal assignment are carried out after NMR data acquisition.144–146 In the standard approach, the samples are prepared, and the data are acquired by 1D or 2D NMR. After analysis, the data are then matched to those in relevant databases to identify biomarkers relevant to the exposome.147 For this purpose, many useful informatics tools have been developed,5,13,148–150 such as NOREVA,151 PhenoMeNal,152 Plasmodesma153 and MetaboAnalyst3.154–156 Solid-state NMR analysis is difficult because the spectra are broad with overlapping peaks.157 To improve sensitivity and resolution, several methods have been developed for spectral separation,66,158 apodization, zero filling, linear prediction, fitting and numerical simulation159 such as covariance analysis,160 SIMPSON,161 SPINEVOLUTION,162 dmfit,163 EASY-GOING deconvolution,164 INFOS,165 Fityk,166 ssNake,167 signal deconvolution methods using short-time FT and non-negative tensor/matrix factorization168 and noise reduction based on PCA.169 Identifying factors related to homeostasis and materials recycling in living organisms and environments can be achieved by using Cytoscape170 to integrate and analyse various sources of information such as genomic, transcriptomic and metabolomic data, environmental factors (chemical and physical) and behaviour. This method has been shown to be useful for clarifying health maintenance,171 factors involved in disease172 and the impact of environmental change,21 as well as for the further development of predictive algorithms.173
NMR is a widely used analytical technique with a growing number of available repositories. As a result, there is a need for an open-data format that will aid long-term storage of NMR data, ease data comparisons and encourage sharing and reuse of NMR data. The NMReDATA database stores chemical shift values, signal integrals, intensities, multiplicities, scalar coupling constants, lists of 2D correlations, relaxation times and diffusion rates related to the raw and spectral data.174 Nmrshiftdb2, an open-data repository for organic structures and their NMR spectra, can import and export NMReDATA.175 Similarly, nmrML serves as a storage format for the MetaboLights data repository,176 while NMR-STAR is the archival format used by the Biological Magnetic Resonance Bank (BMRB),177 the International Repository of Biomolecular NMR Data and the Worldwide Protein Data Bank.178 An NMR database based on linked open data described in Resource Description Framework (RDF) has been developed by the National Bioscience Database Center,179 Protein Data Bank Japan180 and BMRB.181 Constructing databases of compounds enables metabolites to be identified from complex spectra.182 In addition, various databases have been developed for NMR-based metabolomics research,13 such as HMDB,183 MMCD,184 BML-NMR,185 NAPROC-13,186 Mery-B,187 Spektraris188 and SDBS.189 Regarding database and informatics tools for polymers, Polyinfo190 and Polymer Genome191 have been established. Various programs are available for automatically searching NMR peaks of compounds in these databases, such as InterSpin,66 which includes tools for pre-processing of low-resolution NMR and signal assignment of macromolecules; SpinAssign192 for HSQC spectra; SpinCouple193 for 2D J-resolved spectra; and ECOMICS to assign chemical shifts of lignin and hemicellulose.194 Particularly for studies of homeostasis, SpinCouple can quantitatively analyse metabolic changes in a time-course series of samples. Such quantification over time reveals the dynamics of homeostasis not only for humans but also for surrounding environments such as external ecosystems.195 Lastly, the web server COLMAR has been developed for the analysis of complex mixtures and provides several tools.196–201
Python, R and Matlab are the most popular programming language interfaces for data processing and machine learning. Regarding python-based applications, NMRbot improves automated data acquisition and has tools for optimizing experimental parameters on the fly.202 When used in combination with python scientific libraries, nmrglue203 and nmrpy204 provide a highly flexible and robust environment for spectral processing, analysis and visualization; include common utilities such as linear prediction, peak picking and lineshape fitting; and can facilitate reading, writing and conversion of data stored in Bruker, Agilent/Varian, NMRPipe, Sparky, SIMPSON and Rowland NMR Toolkit file formats. The nmrstarlib package has been developed for accessing data stored in the BMRB.205 Other python tools for NMR metabolomics include FOCUS,206 Farseer-NMR207 and KIMBLE.208 There are also many R packages for NMR data handling, pre-processing and analysis,209 such as AlpsNMR,210 speaq211 and PepsNMR.212
2.5 Machine learning in NMR
Machine learning (ML) approaches are integral to artificial intelligence (i.e., systems that imitate human intelligence) and are now used in many scientific fields. The two main types of ML algorithm are supervised learning and unsupervised learning.213 In unsupervised ML, the system learns complex patterns more autonomously and identifies them with the purpose of summarizing, exploring and discovering. This approach requires only a few prior assumptions and little previous knowledge of the data. Four of the most commonly used methods for unsupervised ML in metabolomics data analysis are PCA, k-means clustering, hierarchical clustering and self-organizing map. By contrast, supervised ML trains on an annotated dataset and has a defined output. Its purpose is to determine an association between the response variable and the predictors (often referred to as covariates) and to make accurate predictions. Analyses involving discrete variables (e.g., control group vs. diseased group) are referred to as classification problems, while those involving continuous variables (e.g., metabolite concentration or gene expression level) are known as regression problems. Two of the most widely used methods for supervised ML in metabolomics are partial least squares-discriminant analysis (PLS-DA)214 and support vector machine (SVM). PCA and PLS are both linear methods, but more complex non-linear ML methods, such as random forest (RF),215–217 SVM218–222 with a non-linear kernel, and artificial neural networks (ANNs), may be applicable to NMR-based metabolomics.
Traditionally, the first step in building a predictive model, known as feature selection, is to convert raw data into a form that can be manageably processed. For metabolomics, this would be a matrix of metabolite concentrations. In the second step, the semi-manually extracted data are modelled by using a relatively simple predictive algorithm (e.g., ANN, SVM or PLS). In deep learning, both steps are incorporated into a single algorithm; this requires multiple layers of neurons that are stacked to sequentially deconvolve data from their raw state, abstract latent structure, and then effect prediction. With the shift towards interpretability, PLS has become the standard supervised multivariate method used by the metabolomics community.223 For non-linear ML models, interpretability remains a major challenge; however, the need for high accuracy of classification may overshadow the need for model interpretability.
Deep learning applications in NMR spectroscopy are based predominantly on three types of model: deep neural networks (DNNs), convolutional neural networks (CNNs) and recurrent neural networks (RNNs).224 DNNs are well suited for complex high-dimensional data analysis, including both feature extraction and mapping, CNNs are useful for analysing spatial information, including spectrum reconstruction and denoising, and chemical shift prediction, while RNNs are often used for tasks that require processing of sequential inputs, such as time-domain signals (Fig. 5). Because free induction decay signals and time-series NMR spectra data are sequential, RNNs can provide guidance for processing time-domain data and time change data. We have successfully developed several ML-based analytical approaches, namely, a prediction method for metabolic mixture signals,225,226 DNN-mean decrease accuracy,32 ensemble DNN,33 variable selection for regional feature extraction,34 and methods for evaluation of surface water,14 impact estimation of food intake on mice,227 evaluation of human daily dietary intake228 and relaxometric learning229 in metabolomics studies. DNN has also been used to reconstruct non-uniformly sampled NMR spectra230,231 and for solubility prediction,232 while CNN has been used to remove noise from NMR spectra233 and to reconstruct NMR spectra from non-uniformly sampled data.234 DN-Unet combines structures of encoders–decoders, and CNN can be used to suppress noise in liquid-state NMR spectra to enhance SNR.235 Lastly, NMR-TS automatically identifies a molecule from its NMR spectrum, and discovers candidate molecules whose NMR spectra match the target spectrum by using RNN and density functional theory-computed spectra.236
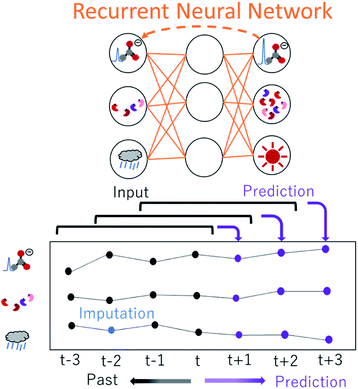 |
| Fig. 5 Conceptual diagram of analysis of time-series data by a recurrent neural network, which is a machine learning method. Examples of time-series data are NMR spectral intensity change of metabolites, microbiome change, and weather change. Completing missing values is an important step in time-series data. | |
Several libraries have been constructed to ease implementation of ML, including MXNet (https://mxnet.apache.org/), PyTorch (https://pytorch.org/), Tensorflow (https://www.tensorflow.org/), scikit-multilearn (http://scikit.ml/), Keras (https://keras.io/), classyfire,237 MetNormalizer,238 Caret239 and mlr.240 By parallelising code to run on graphics processing units (GPUs), ML and simulation may be accelerated, opening new frontiers in NMR data science. GPU computing has been applied to Monte Carlo simulation,241,242 prediction of NMR chemical shifts,243,244 calculating the diffusion tensor for flexible molecules, deep learning for metabolomics,245 de novo pulse sequence design in solid-state NMR,246 reconstruction of non-uniformly sampled NMR spectra230,231 and denoising.235,247 GPU computing is readily available through a workstation-class machine or cloud computing services (e.g., Amazon Web Service, Google Cloud Platform, Microsoft Azure).
3. Application of NMR data science towards global sustainability
3.1 Natural environment
The global environment is a product of the interwoven activity of earth, plants, animals, microorganisms and human society. In terms of the exposome, organic matter and nutrients contained in wastewater from agriculture and human society, as well as minerals from the earth, exert great influence and are essential for healthy biological and chemical (e.g., microbes, organic matters, nutrients and minerals) cycles in nature (Fig. 6a). NMR data science has applications in the integrated analysis of organic, inorganic and microbial data as the environmental exposome related to eutrophication. In this case, organic matter was measured by NMR signal intensities, inorganic elements by abundance measurements using ICP-OES, microbial groups by next-generation sequencing, and nitrogen compounds by elemental analysis.16 Subsequent correlation network analysis revealed that peptides signals from the HSQC spectrum of sediments in eutrophication were correlated with sulfate-reducing bacteria, sulfur, iron and nitrogen compounds (Fig. 6b). The HSQC spectra were analyzed by PCA, and the peptide signal in the sediments associated with eutrophication was related to geographic variation (Fig. 6c). The organic matter that accumulates in estuarine environments and provides habitats for various microorganisms can be analysed by using NMR techniques such as HSQC spectroscopy.248 Dissolved organic matter in water bodies has been extensively investigated by NMR-based approaches. For example, DOM in estuarine environments has been evaluated by 2D correlation spectroscopy using 13C NMR and FT-IR spectra,249 solid- and multidimensional solution-state NMR have determined the chemical composition of river DOM,250 NMR coupled with isotope analysis has revealed refractory proteinaceous compounds in lake DOM,251 while DOM in a shallow aquifer has been characterized by coupling 13C NMR spectroscopy with elemental analysis and ultraviolet-visible absorbance spectroscopy.252 The agglomeration of organic matter in estuarine ecosystems has been evaluated by advanced solid-state NMR, revealing that a fraction of organic matter is analogous in composition to the cell wall components of bacteria.253 Although microbial ecosystems in aquatic environments are often studied by NMR-based metabolomic approaches, concentrations of metabolites tend to be low. Thus, water sampling and pre-treatment are key steps in sample preparation for NMR measurements. In this respect, a method for concentrating metabolites derived from low-density planktonic communities for NMR measurements has been reported.254 In marine environments, the chemical structures and compositions of particulate organic matter in water column and marine sediments have been characterized by solid-state NMR.255,256 For solution-state NMR measurements, a method for the solid-phase extraction of DOM has been proposed,257 and improved guidelines for extraction have been recently reported.258 An NMR-based approach combined with ultrahigh resolution MS has shown that carboxyl-rich alicyclic material is abundant in subfractions of DOM.259 Combined with high performance liquid chromatography measurements, 2D and 3D NMR has demonstrated that oxidized sterols and hopanoids may be dominant in DOM.260 Multidimensional NMR to detect long-range proton–carbon correlations has been used to identify material derived from linear terpenoids in DOM.261 Lastly, the rise in seawater temperature in recent years, coupled with urban industrialization and coastal eutrophication of fertilizer inflow from rural areas, has led to a collapse of the marine microbial ecosystem, resulting in marine damage such as red tides.14 In this regard, we have studied analytically and numerically a stochastic phytoplankton-toxic phytoplankton–zooplankton system.262
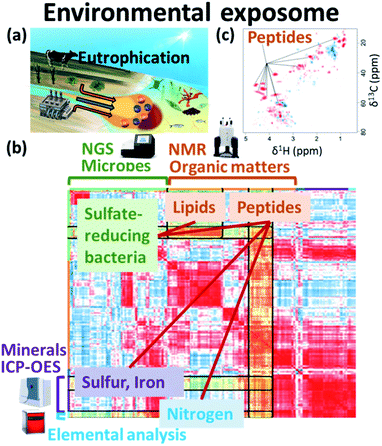 |
| Fig. 6 Integrated analysis of organic, inorganic and microbial data as the environmental exposome related to eutrophication of estuaries. (a) Conceptual diagram of wastewater from agriculture and industry flowing into the estuary. (b) Results of correlation analysis using organic matter measured by NMR, minerals measured by ICP-OES, microbes measured by next-generation sequencing, and nitrogen compounds measured by elemental analysis. (c) The HSQC spectra of geometrically and seasonally variable sediments were analyzed by PCA are shown. The red signal indicates a positive loading factor (PC2) calculated by PCA, while the blue signal is negative. | |
3.2 Agriculture
Plants and algae that live in natural environments are often precious resources that maintain the ecosystem.263–265 In addition to providing food, they are sources of numerous compounds from low molecular weight molecules to polymers, have medicinal value, and provide energy.266–268 NMR can help in targeting of breeding strategies; for instance, NMR has been used to compare eight fleshy fruit species during development and ripening in order to understand the mechanisms that link metabolism to phenotype.269 NMR-based metabolomics has been applied to the study of other foodstuffs, including truffle, kiwifruit, lettuce and sea bass.270 In combination with multivariate analysis, NMR-based metabolomics can be used to characterize differences based on geographical origin and plant varieties. For example, NMR metabolomics has been used to profile tomato variety, fruit development, ripening, quality, geographical origin, and daily and seasonal changes.43,271 ICA and PLS-DA is superior to classical PCA regarding the verification of rice authenticity.272 In addition, lignocelluloses in plants change significantly in response to environmental changes. In addition, a defence mechanism to prevent invasion of microbes hardens cell walls by changing the composition of lignin.273 Therefore, structural characterization of these polymers is important for effective production of biomass as a resource. Solid-state NMR can be used to determine the composition of insoluble polymers and biomass; in this respect, several studies using 13C-labelled Arabidopsis thaliana have been reported.274–279 Lastly, HR-MAS enables the direct application of NMR spectroscopy to semi-solid and gel-like samples,60 can evaluate differences in the chemical composition caused by geographical factors.280 The major advantage of HR-MAS NMR over liquid-state NMR is that there is no extraction step necessary which can lead to the loss of signals from non-soluble metabolites.281
In agriculture, plant growth is affected by exposomes such as the soil, rhizosphere, and weather conditions (Fig. 7a). Relationships among numerous environmental factors can be explored by using NMR-based metabolic profiling with integrated analysis of various measurement data. For example, to examine an experimental agricultural field (“agroecosystem”), a data-driven approach for evaluating the agricultural exposome in terms of the metabolome (an examination of various metabolites using NMR), ionome (looking at the distribution of elements), microbiome (a comprehensive survey of microbial profile), and phenome (an examination of plant phenotypes) has been used (Fig. 7b).282 In this case, NMR spectra of plant and soil detected 13C-labelled bondomers (i.e., succinic acid and proline) metabolized from root absorption of 13C-alanine (Fig. 7c). This network analysis revealed the significant role of organic nitrogen in increasing agricultural crop yield in the agroecosystem.
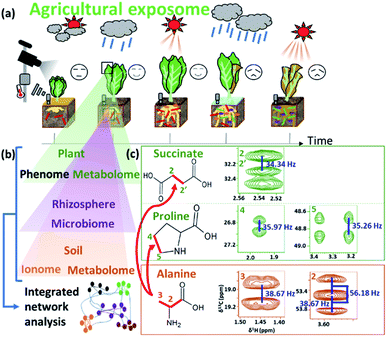 |
| Fig. 7 Data-driven approach for evaluating the agricultural exposome. (a) Conceptual diagram of time-series variables such as the phenome, plant metabolome, rhizosphere microbiome, ionome, soil metabolome, and environment in the agricultural ecosystem. (b) Conceptual diagram of integrated network analysis of multi-omics data including the metabolome, phenome, microbiome and ionome. (c) Regions of interest in HSQC spectra of 13C-labelled compounds (i.e., succinic acid and proline in plant) metabolized from absorbed soil alanine and detected by integrated network analysis. 1JCC values are shown by blue letter. Deducible 13C–13C bondomers are indicated by red lines. | |
Soils contain numerous types of organic matter, ranging from small molecules to highly complex supermolecular structures.283 Microorganisms and their degradation products are also important chemical components of the soil biomass.284 In environmental research, NMR-based approaches have been applied to evaluate and characterize soil organic matter,285 including chemical components and structures. For instance, a solution-state NMR study revealed that biomacromolecules derived from microorganisms and plants are the major components in soil humus,286 a finding supported by another solution-state NMR study that used a solvent system solubilizing 70% of humin (a component of humus).287 Solid-state NMR has also been applied to the characterization of organic matter using various approaches. For example, direct polarization (DP)-MAS, CP-MAS, DP-total suppression of spinning sidebands (TOSS), CP-TOSS and 1H–13C HETCOR have been used to characterize the chemical structures and composition of organic matter in peat,288,289 anaerobically digested biosolids,290 and terra preta and prairie soils.291 NMR-based experiments have also evaluated sorption selectivity and adsorption properties in natural organic matter.292–294 Both solid- and solution-state NMR measurements have been used to characterize the cellulosic supermolecular structures of rice straw and their degradation profiles produced by a microbial community in the paddy field.295 Interestingly, the same chemical components of the rice straw biomass induced distinct metabolic reactions and reaction rates in different supermolecular structures when different key microbes were involved in degrading the plant biomass.295 Moreover, an integrated metabolic dynamics approach using both 1D 1H and 1D 13C NMR spectra with time-course analyses has been proposed for the characterization of soil microbial activities in field environments.296 Another NMR-based metabolomics study has compared agricultural and native state soils.297 Lastly, NMR-based metabolomics has been used to analyse the interactions between soil microbial communities and plants, showing that soil microbial communities and their metabolites change in accordance with physical and chemical properties of the soil that are related to plant growth.23
3.3 Fisheries and aquaculture
Aquaculture provides an increasingly important source of protein for human consumption. In recent years, aquaculture technology has developed, and culture methods have become more intensive; however, there remain several issues, such as the aquaculture environment, feeding and disease. As an example of application of the exposome paradigm to aquaculture, the “muscle quality” of natural fish varies depending on the water temperature and nutrients in the environment where it grows, as well as the plankton and small fish that feed in this environment; as a result, the market value of fish meats also varies greatly.110,298 Thus, “muscle quality” analysis data of natural fishes can be used to determine their origin and will contribute to maintaining and evaluating the market value. To this end, we have focused on the recent progress in NMR instruments not only for high-end laboratory analysis but also for bench-top analysis, and have developed a peak separation method from multi-variate analysis.299 However, if a high-end instrument is used, it is possible to more clearly discriminate changes in chemical composition in the muscle due to the differences in the living environment and food. Therefore, by introducing cutting-edge machine learning calculations, into NMR analysis for evaluating the exposome during fish lifetime, we can either extract important factors (i.e., NMR peaks of metabolites) involved in determining the origin of natural fish (Fig. 8).32 The an improved DNN-based analytical approach that incorporates an importance estimation for each variable using a mean decrease accuracy (DNN-MDA) approach have the best classification accuracy (97.8%) than other examined methods such as PLS, SVM, RF. In addition to this, the DNN-MDA approach facilitate the identification of important variables such as trimethylamine N-oxide, inosinic acid, and glycine, which are characteristic metabolites that contributed to the discrimination of the geographical differences between fish caught in the Kanto region and those caught in other regions. The ensemble DNN (EDNN) approach is applied to metabolomics data of fish muscles collected from Japan coastal and estuarine environments.33 The performance of EDNN regression for fish size based on metabolic profiles is superior to that of DNN, RF, and SVM algorithms.
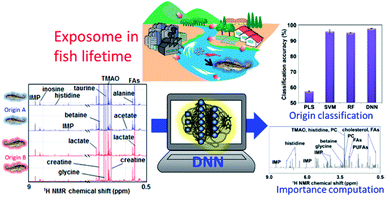 |
| Fig. 8 Data-driven approach for evaluating the exposome during fish lifetime. Several machine-learning algorithms, such as partial least squares (PLS), support vector machine (SVM), random forest (RF), and deep neural network (DNN), can be applied to 1H NMR spectra from gobby muscle to (1) classify fish origins and (2) extract important factors based on features of goby muscle metabolites derived from their habitat environment. In the figure, FAs refers to fatty acids, IMP refers to inosinic acid, PC refers to phosphatidylcholine, PUFAs refers to polyunsaturated fatty acids, and TMAO refers to trimethylamine N-oxide. | |
As another example, fish meal is mainly used for feed in aquaculture, but only about 20% of the nitrogen and phosphorus content is assimilated into fish, while the other 80% enters the environment as residual food and faeces. To lower the environmental load, therefore, the development of both feed300 that does not rely on fish meal and digitization technology for evaluation of ecosystems is required. In addition, nutrition for high trophic species in aquaculture is faced with the problems of sustainable fish and plant-based diets. Insects seem particularly promising for supplementing fish and plant-based diets. In this respect, 1H-NMR metabolomics profiling has been used to compare metabolites in insect diets and in fish plasma, liver and muscle,301 and to evaluate growth performance and feed conversion ratios in fish fed plant-based, commercial, insect, spirulina and yeast diets.302 Aquaculture has been hampered by a scarcity of biological knowledge of the farmed animals and their symbiotic gut bacteria. However, high-throughput NMR-based technologies or “omics” for investigating the genome, transcriptome, proteome, metabolome and microbiome are being gradually applied to aquaculture, and may improve current farming technology. For example, gut microbiota affects both nutrient acquisition and energy homeostasis in the host, and is thought to play an important role in the immune system. Owing to the flow of water through the digestive tract, the gut microbiota of fish and shellfish are especially dependent on the external conditions and change markedly in response to environmental and biological stimuli.303 NMR-based metabolomics can be used to characterize such changes in gut microbiota. For instance, one NMR-based metabolomics study has shown that dietary conditions strongly affect the gut microbiota in fish;24 notably, changes in the feeding of Epinephelus septemfasciatus were found to affect the metabolic and microbiota profiles of faeces. Similarly, in host and symbiotic metabolic analyses of wild yellowfin goby15 and 24 natural fish diversities living in the Kanto and Tohoku region in Japan,34 we found that the microbiota and metabolic profiles of faeces showed clustering trends based on feeding conditions. Thus, as an aggregate of final metabolic products, faeces is affected by diet. Given that the intestinal environment is reflected in the faeces of fish, this type of analysis might be an informative non-invasive technique for use in aquaculture. We have estimated the dietary components of several eel larvae species and the marine environment in the western North Pacific using metagenomic analysis.304 In the future, metabolomic analysis using NMR may lead to an improvement in its early survival during aquaculture. Lastly, another NMR-based metabolite profiling study has evaluated time-series data under different feeding conditions in the rearing environment of leopard coral grouper to identify fish nutritional biorhythms and improve feeding in aquaculture.300
As a radical solution to environmental problems, closed-circulation aquaculture – in which fish and shellfish are cultivated in limited water on land – is drawing attention. By controlling the closed environment, it is possible to attain high growth, modify fish meat components, improve quality, and build an automated system for breeding. In these systems, ammonia is highly toxic and must be monitored and removed; therefore, wastewater treatment is an important issue. Aquaponics, a resource-recycling type of food production system, is an innovative and sustainable hydroponic plant production system using fish waste (ammonia) that can play an important role in the future of environmental and socio-economic sustainability.305 In addition, fish wastewater can be optimized as fertilizer to meet the specific demands of plant species.306
3.4 Wastewater treatment in industry
Just as inadequate removal of feed in the environment causes eutrophication in rivers, lakes and coastal environments, wastewater treatment is also important for environmental health. In other words, inadequate wastewater treatment can be regarded as the “exposome for an aqueous environment”, including the flow of nitrogen and phosphorus downstream (Fig. 9). For example, in practical wastewater treatment process, 31P NMR and machine learning of extreme gradient boosting (XGBoost) method was applied to model the phosphorus release performance of biofilm at different pH.307 The application of NMR spectroscopy to characterization of wastewater has been previously reviewed.308 Here we focus on environmental metabolomics studies of microbial ecosystems utilized in industrial processes such as wastewater treatment and bioremediation. Anaerobic microbial ecosystems are particularly useful in industry because they can degrade supermolecular assemblies into small molecules. To characterize the degradation processes in anaerobic microbial ecosystems, NMR-based metabolomic approaches have been used in conjunction with microbial community analyses. For example, to determine “metabolic sequences” (i.e., metabolic dynamics over time) of anaerobic fermentation, correlation-based analysis has been used to integrate data from metabolic profiling (evaluated by NMR-based metabolomics) with data from microbial community profiling104 (as evaluated by denaturing gradient gel electrophoresis fingerprinting309). This approach enabled the entire set of metabolic processes to be visualized with their responsible factors in an anaerobic microbial ecosystem.104 Stable isotope probing is a useful tool for characterizing and identifying key factors in microbial ecosystems,310 and has been combined with DGGE-NMR analysis to both monitor metabolic variations of microbial ecosystems and capture transient metabolic fluxes with their responsible factors within microbial communities.311 NMR spectroscopy is also well-suited to characterizing cellulose polymer degradation into biogases by anaerobic microbial ecosystems because it can measure solid-, solution- and gas-state compounds.312 Indeed, the degradation of cellulose polymers and production of methane gas via organic compounds in anaerobic microbial ecosystems has been successfully demonstrated using triple-phase (solid-, solution- and gas-state) NMR. The detailed metabolic reactions of degradation processes have been characterized by NMR-based metabolomics combined with metagenomics using stable isotope labelling, identifying key metabolites, enzymes and microbe in the ecosystem.313
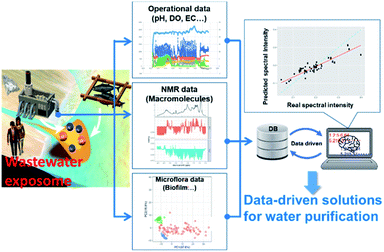 |
| Fig. 9 Data-driven solutions for wastewater treatment. Conceptual diagram of wastewater exhausted from factories and aquaculture as the “exposome for an aqueous environment”. Next, operational data such as pH, dissolved oxygen (DO), and electrical conductivity (EC) are shown as examples of time-series data, as well as NMR spectra of macromolecules and biofilm microflora data analyzed by principal component analysis (PCA). Finally, a conceptual diagram of data-driven analysis for water purification using a database of those data is shown. | |
Regarding operation of wastewater treatment, the main obstacle preventing wider use of membrane bioreactors (MBRs) is membrane fouling (i.e., deterioration of membrane permeability), which increases operating costs. Thus, an understanding of the mechanisms of membrane fouling is important. In the study of membrane fouling, NMR has been used to investigate the nature of membrane foulant (proteinaceous) changes depending on the food-microorganism ratio,314 and seasonal variation (proteinaceous at high temperatures, and polysaccharide-like or humic acid-like substances at low temperatures).315 In these papers, consistent chemical structures have been detected in both the 13C CP-MAS NMR spectra (proteins (55 and 175 ppm), carbohydrates (75 and 105 ppm), aromatic carbons (110–165 ppm)), and the FT-IR spectra (the amido-I, -II bands and aromatic C
C of proteins (1660, 1540, and 1620 cm−1), carbohydrates (1100 cm−1), and humic substances (1400 cm−1)). In an approach to non-invasively elucidate fluid–structure interactions in complex multispecies biofilms, pulsed-field gradient NMR has been used to measure the water diffusion in five different types of biomass aggregate, including sludge flocs, biofilms and granules.316 Because the removal or degradation of particulate organic matter is a crucial part of biological wastewater treatment, particle transport into granular sludge beds has been visualized in 3D by MRI.317 Lastly, NMR has been used to tune the hydrophobicity and fouling-liable properties of polyethersulfone membranes.318
3.5 Biomass degradation
Recycling in an ecosystem occurs through symbiosis between termite gut microbial communities, specific protists and fungi, and soil microbial communities. Plant-derived saccharides are degraded by soil microbial communities and eventually return to the soil.296 Lignocellulose, a high-order structure and sparingly soluble polymer mixture formed from polysaccharide cellulose, hemicellulose and the polymer lignin, is the most abundant biomass on land.319–321 The carbon source produced by trees is decomposed by the intestinal microbial community of termites, is further metabolized to amino acids through nitrogen fixation by the intestinal microorganisms, and eventually becomes the proteins that make up the termite body.322 Furthermore, the decomposition of macromolecular complexes such as lignocellulose (comprising cellulose, hemicellulose and lignin) is an important process in the circulation of various elements. The carbon source from plants and nitrogen in the air is decomposed and metabolized to amino acids via nitrogen fixation by the gut microbes of termites. Carbon and nitrogen circulation via their faeces and remains represents the terrestrial exposome, and enriches and affects the forest and aquatic ecosystem (Fig. 10a).323 Web tools and NMR database for biomass macromolecules are useful for evaluating terrestrial exposome such as plant composition evaluation of ball-milled samples dissolved in dimethyl sulfoxide (DMSO) solvent by solution NMR324 and evaluation of plant cell wall quality by solid-state NMR320 (Fig. 10b). For example, Bm-char in ECOMICS194 can be used to characterize the HSQC spectra of biomass macromolecules measured by solution NMR (Fig. 10c). The database comprises 42 and 17 signals for, respectively, aromatic and aliphatic sites of lignin, and 26 signals for hemicellulosic sites and three uncategorized sites. Biomass samples are characterized by their composition of biomass macromolecules such as lignin and hemicellulose (Fig. 10d). The resulting pie-charts are categorized according to ‘detailed category’ items described on the Bm-Char website: namely ‘syringyl’, ‘syringyl (oxidized alpha-ketone)’, ‘guaiacyl’, ‘guaiacyl (oxidized alpha-ketone), ‘p-hydroxyphenyl’, ‘ferulate’, ‘p-coumarates’, ‘cinnamyl alcohol end group’ and ‘p-hydroxybenzoates’ for aromatic sites of lignin; ‘β-O-4’, ‘β-O-4-S’, ‘β-O-4-H/G’, ‘β-5’, ‘β–β’ and ‘5–5/4-O-β’ for aliphatic sites of lignin; ‘acetylated xylopyranoside’, ‘xylopyranoside’, ‘xylopyranoside + glucopyranoside’, ‘glucopyranoside’, ‘galactopyranoside’, ‘arabinofuranoside’, ‘mannopyranoside’, ‘fucopyranoside’ and ‘methyl-glucuronic acid’ for hemicellulosic sites; and ‘others’. The humus that accumulates in soil after biomass decomposition can adsorb metal elements, which are then taken up by detritus-eating animals that ingest the humus and are responsible for the bioaccumulation of various metals. Plankton and algae such as E. gracilis319 are deeply involved in the cycle of materials and the food chain of aquatic ecosystems. The chemical components contained in seaweeds are used in, for example, foods, feeds, fertilizers and fine chemicals, and the adsorption of metal by polysaccharides is also attracting attention in water treatment processes. However, the composition of seaweeds is known to fluctuate with the seasons and cannot be controlled by humans. To capture the complex seasonal changes in the components of naturally growing seaweeds, it is necessary to comprehensively measure the components over time and evaluate them in an integrated manner.21
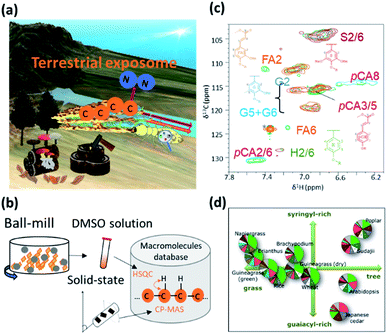 |
| Fig. 10 Characterization of biomass macromolecules as the terrestrial exposome. (a) Conceptual diagram of biomass macromolecules related to carbon and nitrogen circulation as the terrestrial exposome. (b) Development of macromolecules database including solution and solid-state NMR data. In the case of solution NMR, biomass samples are ball-milled and dissolved in DMSO. (c) Overlay of the lignin aromatic region of 2D 1H–13C HSQC spectra of poplar (brown), Japanese cedar (light brown) and Erianthus sp. (green). Lignin signal assignments and their chemical structures are highlighted along with corresponding cross peaks. Assignmented compounds in the figure are the signals of syringyl (S2/6), guaiacyl (G2, G5 + G6), ferulate (FA2, FA6), p-hydroxyphenyl (H2/6), p-coumarate (pCA2/6, pCA3/5, pCA/8). (d) Pie-charts representing the percentage of biomass-related signals of 11 plant samples (i.e., grasses: Erianthus, napier grass, guinea grass, Brachypodium, rice, and wheat, herbs: Arabidopsis, and trees: sudajii, Japanese cedar, and poplar). Pie-charts are categorized according to ‘detailed category’ items described on the Bm-Char website (http://ecomics.riken.jp/biomass/). | |
NMR data science can be applied to investigations of these anaerobic microbial communities. For instance, the microbes responsible for the digestion of plant saccharides into short chain fatty acids (SCFAs) have been investigated in several systems, including animal gut,37,38 waste management,104,312,313 and paddy field soil.295 These symbiont-derived SCFAs have attracted interest because they may be essential for survival of the plant or animal host, which in many cases has evolved to utilize the metabolic activity of their microbial symbionts. SCFAs are also important because they act as electron donors and can reduce heavy metals such as Fe(III) and Mn(IV) under different environmental conditions. Moreover, SCFA-producing bacteria such as Lactobacillus spp. stimulate plant germination, and root and shoot growth in soil ecosystems; thus, SCFAs and the bacteria that produce them are central to the soil environment. On the whole, however, little information is available on the biomass degradation process, especially the dynamics of soil microbial communities and SCFA metabolism. In this respect, metabolic profiling based on NMR represents a powerful tool for the analysis of complex reactions in microbial ecosystems. This technique has been used to characterize total metabolic activity and identify biomarkers in a wide variety of systems.325–327 Furthermore, the introduction of 13C-nuclei to NMR analysis has been a useful technological advance for the resolution of overlapping signals of saccharides that limit 1H-NMR.328 Advantages of the 13C-labelling method also include the characterization of compounds in complex components, the determination of compound structures, and the tracking of microbial metabolic pathways by NMR or other analytical techniques.328 We have previously successfully performed 13C-labelling in plants329,330 and microbial systems,331 as well as metabolic profiling using 2D and 3D NMR techniques.276,332
3.6 Biodegradable plastics
Plastics have been important components of disposable bottles and synthetic fibres; however, the highly stable microplastics333 that result from the discharge of plastic waste into the environment and outflow out from rivers to oceans has led to a worldwide problem of pollution affecting the marine exposome (Fig. 11a).334–337 The degradation process of plastics can be investigated by in vitro experiments conducted in the ocean. Biodegradable plastics, which can decompose in the environment, are a promising solution for the environmental problems caused by disposal of plastics.338–341 Environmental degradation rates and pathways for the major types of thermoplastic polymer have been investigated.342 As alternatives to petroleum resources, microbial products and plant biomass can be used to produce macromolecular materials such as plastics and feedstock.343 For example, polymers such as polylactic acid (PLA),344 poly-ε-caprolactone345 and cellulose62–64,312,313,320,346,347 have been prepared as high-performance materials with various properties. In developing such materials, it is necessary to analyse microbial and plant biomass as complex biochemical systems with multiple macromolecular components. In this respect, NMR spectroscopy can characterize the native structure, components and dynamics of these samples at the atomic level (Fig. 11b).5,55 In addition, time-series NMR measurements produce 1H anisotropic and 13C spectra as descriptors of structure and property during the biodegradation process.
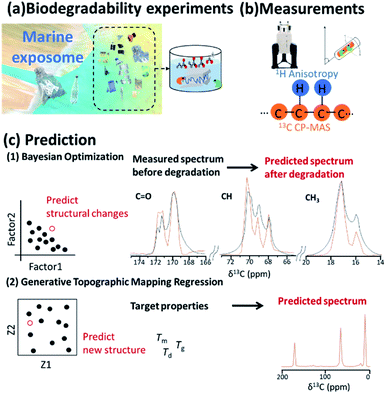 |
| Fig. 11 Data-driven approaches to the development of biodegradable plastics. (a) Plastic pollution of the ocean from human society is a problem affecting the marine exposome. The degradation process of plastics can be investigated by in vitro experiments. (b) NMR measurements of 1H anisotropy and 13C spectra can detect chemical structures and dynamics of solid-state materials. (c) In the development of biodegradable plastics, data-driven scientific methods such as Bayesian optimization and Generative Topographic Mapping Regression (GTMR) can predict (1) NMR spectra after degradation of materials and (2) NMR spectra of materials with target properties. | |
The higher-order structure of materials has a significant influence on their macroscopic properties.348 Traditional trial-and-error design approaches for new materials face significant challenges due to the vast design space. In addition, computational technologies, such as density functional theory225 and molecular dynamics,62 are usually computationally expensive; thus, it is difficult to calculate molecular structures from material properties. To address these problems, ML-assisted materials design is emerging as a breakthrough tool in many areas of science.349 Furthermore, a materials informatics approach has been recently considered for materials design.350 In this approach, “big data” deposited in databases, as well as higher-order structural data obtained during the materials production process,85,190 are used to predict physicochemical properties of new materials. Furthermore, moulding conditions are important in developing a material with desired physical properties. In this regard, NMR, especially low magnetic field NMR which is used for routine material evaluation, produces vast datasets66 that can be used in the prediction of NMR signals to aid in the cycle of developing materials and identifying structures with desired properties. Coupled with NMR measurements, inverse design using ML can be used to design polymers with desired phase behaviour. For example, ML has been used for cloud-point engineering of polymers, whose compositions were determined by 1H NMR.351 NMR chemical shifts are rich in information and encode structural features of the molecules contributing to the physical, chemical and biological properties of a material; thus, they have also been used as a descriptor in quantitative structure–activity/property relationship modelling studies.352 In designing higher-order structures of biodegradable plastics, physical properties such as glass transition, melting and degradation temperatures (Tg, Tm, and Td) related to biodegradability and durability should be controlled (Fig. 11c). Bayesian optimization has been applied in real and virtual degradable experiments of bioplastics.353 From the results of degradation experiments, the weight average molecular weight was obtained as the objective variable, while initial crystallinity, moisture content in the compost environment, degradation period and NMR spectra were used as explanatory variables. These values were subjected to a decomposition degree predictive model consisting of ML. The optimum decomposition degree, various analytical values, and experimental conditions were explored by using a combination approach with a decomposition-degree predictive model and Bayesian optimization. For efficient material design based on the iterative cycle of preparation, measurement of samples and analysis of measured data, GTMR has also been applied to the analysis of CP-MAS spectra to predict 13C NMR spectrum of the material in its solid-state based on its thermophysical properties.168
Microalgae (e.g., Chlamydomonas reinhardtii and Phaeodactylum tricornutum) are also suitable for environmental and biotechnological applications based on the exploitation of solar light.354 Polystyrene is generally considered to be durable and biodegradable, but recently biodegradation of polystyrene by the enterobacteria of mealworms has been reported as a new way to deal with plastic waste.355 Bioderived polyesters such as PLA are interesting alternatives to petrochemical-based plastics.356 PLA is unlikely to hydrolyse in the low temperatures of marine environments; however, the carbonyl group of PLA absorbs UV radiation below 280 nm, making the polymer susceptible to photodegradation in marine environments. Lastly, biodegradation of polyethylene microplastics by the marine fungus Zalerion maritimum has been reported on the basis of FT-IR, NMR and scanning electrom microscope data.357
4. Conclusions and future perspective
In the move towards a sustainable society to meet global population growth, the exposome paradigm based on NMR data science will play an important role in comprehensively evaluating and predicting environmental health in terms of systemic homeostasis and resource balance. Because environmental homeostasis (i.e., environmental health) is largely affected by the “exposome” of all substances over time, NMR approaches have the advantage of being able to characterize fluctuations in molecular complexity in a time-course manner based on a data science approach.13,358 NMR measurements also support diverse samples from polar to non-polar solvent systems, and from small molecules to supermolecular assemblies; they can also be applied to various physicochemical states, including gas, sol, gel and solid samples, facilitating structure, component, interaction, adsorption and diffusion analyses. In particular, solid-state NMR has a unique advantage for the evaluation of higher-order structures and dynamics such as crystalline and amorphous biomass and polymer materials. Because NMR produces vast numbers of data with inter-institution compatibility, it is highly suitable for data science using informatics techniques such as multivariate analysis, ML and database analysis. Many targeted analyses focus on a single compound or type of compound that requires pre-processing procedures for sample preparation, pre-treatment and extraction. In contrast, due to its high reproducibility, quantification and high-throughput, the NMR-based non-targeted approach is superior for analysing both large-scale data from time-series samples in environmental evaluation and multiple samples generated in polymer development and manufacture. With advances in informatics technology for pre-processing of low-resolution NMR data, cost-effective benchtop NMR will become more applicable to on-site assessments of human health, natural environment, agriculture, aquaculture and industry, while artificial intelligence and robotics for automation of research and development will be a key technology of NMR data science. The challenge is to utilize data that are linked across the natural environment and human activities throughout society, and to develop and implement data utilization methods.181
Conflicts of interest
There are no conflicts to declare.
Acknowledgements
The authors' work described in this review was supported, in part, by grants to J. K. from Japan Science for the Promotion of Science (JSPS), as well as the Fisheries Agency, the Agriculture, Forestry and Fisheries Council (AFFRC), the Science and Technology Research Partnership for Sustainable Development (SATREPS) from Japan Science and Technology Agency (JST), the Japan International Cooperation Agency (JICA), and the Strategic Innovation Program (SIP) from Cabinet Office (CAO) of Japan. S. Y. was supported by the RIKEN Junior Research Associate Program, and promotion program for informatics and data science of RIKEN Center for Sustainable Resource Science.
References
- H. K. Reeve and B. Holldobler, Proc. Natl. Acad. Sci. U. S. A., 2007, 104, 9736–9740 CrossRef CAS PubMed.
- J. K. Nicholson, E. Holmes, J. C. Lindon and I. D. Wilson, Nat. Biotechnol., 2004, 22, 1268–1274 CrossRef CAS PubMed.
- R. Vermeulen, E. L. Schymanski, A. L. Barabasi and G. W. Miller, Science, 2020, 367, 392–396 CrossRef CAS PubMed.
- R. Goodacre, J. Nutr., 2007, 137, 259S–266S CrossRef CAS PubMed.
- J. Kikuchi, K. Ito and Y. Date, Prog. Nucl. Magn. Reson. Spectrosc., 2018, 104, 56–88 CrossRef CAS PubMed.
- L. Lebreton, M. Egger and B. Slat, Sci. Rep., 2019, 9, 12922 CrossRef PubMed.
- M. Cole, M. Augustin, M. Robertson and J. Manners, npj Sci. Food, 2018, 2, 14 CrossRef PubMed.
- S. Tomita, K. Saito, T. Nakamura, Y. Sekiyama and J. Kikuchi, PLoS One, 2017, 12, e0182229 CrossRef PubMed.
- M. Li, Y. Pu, F. Chen and A. J. Ragauskas, Nat. Biotechnol., 2021, 60, 189–199 CAS.
- J. Johnston, J. Fanzo and B. Cogill, Adv. Nutr., 2014, 5, 418–429 CrossRef CAS PubMed.
- S. Stoll-Kleemann and U. Schmidt, Reg. Environ. Change, 2017, 17, 1261–1277 CrossRef.
- M. Henchion, M. Hayes, A. Mullen, M. Fenelon and B. Tiwari, Foods, 2017, 6, 53 CrossRef PubMed.
- J. Kikuchi and S. Yamada, Analyst, 2017, 142, 4161–4172 RSC.
- A. Oita, Y. Tsuboi, Y. Date, T. Oshima, K. Sakata, A. Yokoyama, S. Moriya and J. Kikuchi, Sci. Total Environ., 2018, 636, 12–19 CrossRef CAS PubMed.
- F. Wei, K. Sakata, T. Asakura, Y. Date and J. Kikuchi, Sci. Rep., 2018, 8, 3478 CrossRef PubMed.
- T. Asakura, Y. Date and J. Kikuchi, Anal. Chem., 2014, 86, 5425–5432 CrossRef CAS PubMed.
- E. A. Schuur, A. D. McGuire, C. Schadel, G. Grosse, J. W. Harden, D. J. Hayes, G. Hugelius, C. D. Koven, P. Kuhry, D. M. Lawrence, S. M. Natali, D. Olefeldt, V. E. Romanovsky, K. Schaefer, M. R. Turetsky, C. C. Treat and J. E. Vonk, Nature, 2015, 520, 171–179 CrossRef CAS PubMed.
- E. K. Pikitch, Science, 2012, 338, 474–475 CrossRef CAS PubMed.
- D. Butler, Nature, 2008, 453, 8 CrossRef CAS PubMed.
- T. Watanabe, A. Shino, K. Akashi and J. Kikuchi, PLoS One, 2014, 9, e106893 CrossRef PubMed.
- K. Ito, K. Sakata, Y. Date and J. Kikuchi, Anal. Chem., 2014, 86, 1098–1105 CrossRef CAS PubMed.
- Y. Sekiyama, K. Okazaki, J. Kikuchi and S. Ikeda, Metabolites, 2017, 7, 4 CrossRef PubMed.
- T. Ogura, Y. Date, M. Masukujane, T. Coetzee, K. Akashi and J. Kikuchi, Sci. Rep., 2016, 6, 28011 CrossRef PubMed.
- T. Asakura, K. Sakata, S. Yoshida, Y. Date and J. Kikuchi, PeerJ, 2014, 2, e550 CrossRef PubMed.
- T. Komatsu, R. Ohishi, A. Shino, K. Akashi and J. Kikuchi, Metabolites, 2014, 4, 1018–1033 CrossRef PubMed.
- S. Yoshida, Y. Date, M. Akama and J. Kikuchi, Sci. Rep., 2014, 4, 7005 CrossRef CAS PubMed.
- Y. Date, K. Sakata and J. Kikuchi, Polym. J., 2012, 44, 888–894 CrossRef CAS.
- F. Wei, K. Ito, K. Sakata, Y. Date and J. Kikuchi, Anal. Chem., 2015, 87, 2819–2826 CrossRef CAS PubMed.
- J. Kikuchi and T. Komatsu, NMR in Glycoscience and Glycotechnology, The Royal Society of Chemistry, 2017, pp. 369–395 Search PubMed.
- S. P. Seitzinger and L. Phillips, Science, 2017, 357, 350–351 CrossRef CAS PubMed.
- C. J. Stevens, Science, 2019, 363, 578–580 CrossRef CAS PubMed.
- Y. Date and J. Kikuchi, Anal. Chem., 2018, 90, 1805–1810 CrossRef CAS PubMed.
- T. Asakura, Y. Date and J. Kikuchi, Anal. Chim. Acta, 2018, 1037, 230–236 CrossRef CAS PubMed.
- T. Asakura, K. Sakata, Y. Date and J. Kikuchi, Anal. Methods, 2018, 10, 2160–2168 RSC.
- C. Deborde, J. Fontaine, D. Jacob, A. Botana, V. Nicaise, F. Richard-Forget, S. Lecomte, C. Decourtil, K. Hamade, F. Mesnard, A. Moing and R. Molinie, Metabolomics, 2019, 15, 28 CrossRef PubMed.
- J. Kruk, M. Doskocz, E. Jodlowska, A. Zacharzewska, J. Lakomiec, K. Czaja and J. Kujawski, Appl. Magn. Reson., 2017, 48, 1–21 CrossRef CAS PubMed.
- S. Fukuda, H. Toh, K. Hase, K. Oshima, Y. Nakanishi, K. Yoshimura, T. Tobe, J. M. Clarke, D. L. Topping, T. Suzuki, T. D. Taylor, K. Itoh, J. Kikuchi, H. Morita, M. Hattori and H. Ohno, Nature, 2011, 469, 543–547 CrossRef CAS PubMed.
- Y. Furusawa, Y. Obata, S. Fukuda, T. A. Endo, G. Nakato, D. Takahashi, Y. Nakanishi, C. Uetake, K. Kato, T. Kato, M. Takahashi, N. N. Fukuda, S. Murakami, E. Miyauchi, S. Hino, K. Atarashi, S. Onawa, Y. Fujimura, T. Lockett, J. M. Clarke, D. L. Topping, M. Tomita, S. Hori, O. Ohara, T. Morita, H. Koseki, J. Kikuchi, K. Honda, K. Hase and H. Ohno, Nature, 2013, 504, 446–450 CrossRef CAS PubMed.
- J. T. Brindle, H. Antti, E. Holmes, G. Tranter, J. K. Nicholson, H. W. Bethell, S. Clarke, P. M. Schofield, E. McKilligin, D. E. Mosedale and D. J. Grainger, Nat. Med., 2002, 8, 1439–1444 CrossRef CAS PubMed.
- E. Holmes, R. L. Loo, J. Stamler, M. Bictash, I. K. Yap, Q. Chan, T. Ebbels, M. De Iorio, I. J. Brown, K. A. Veselkov, M. L. Daviglus, H. Kesteloot, H. Ueshima, L. Zhao, J. K. Nicholson and P. Elliott, Nature, 2008, 453, 396–400 CrossRef CAS PubMed.
- M. I. Smith, T. Yatsunenko, M. J. Manary, I. Trehan, R. Mkakosya, J. Cheng, A. L. Kau, S. S. Rich, P. Concannon, J. C. Mychaleckyj, J. Liu, E. Houpt, J. V. Li, E. Holmes, J. Nicholson, D. Knights, L. K. Ursell, R. Knight and J. I. Gordon, Science, 2013, 339, 548–554 CrossRef CAS PubMed.
- M. Watanabe, Y. Ohta, S. Licang, N. Motoyama and J. Kikuchi, Food Chem., 2015, 169, 387–395 CrossRef CAS PubMed.
- S. Tomita, T. Nemoto, Y. Matsuo, T. Shoji, F. Tanaka, H. Nakagawa, H. Ono, J. Kikuchi, M. Ohnishi-Kameyama and Y. Sekiyama, Food Chem., 2015, 174, 163–172 CrossRef CAS PubMed.
- S. Tomita, S. Ikeda, S. Tsuda, N. Someya, K. Asano, J. Kikuchi, E. Chikayama, H. Ono and Y. Sekiyama, Magn. Reson. Chem., 2017, 55, 120–127 CrossRef CAS PubMed.
- K. Bingol and R. Bruschweiler, Anal. Chem., 2014, 86, 47–57 CrossRef CAS PubMed.
- J. M. Fonville, A. D. Maher, M. Coen, E. Holmes, J. C. Lindon and J. K. Nicholson, Anal. Chem., 2010, 82, 1811–1821 CrossRef CAS PubMed.
- E. Chikayama, M. Suto, T. Nishihara, K. Shinozaki and J. Kikuchi, PLoS One, 2008, 3, e3805 CrossRef PubMed.
- R. McKay and G. Webb, Annu. Rep. NMR Spectrosc., 2009, 66, 33–76 CrossRef CAS.
- J. M. Lopez, R. Cabrera and H. Maruenda, Sci. Rep., 2019, 9, 6900 CrossRef PubMed.
- I. Timári, C. Wang, A. L. Hansen, G. Costa Dos Santos, S. O. Yoon, L. Bruschweiler-Li and R. Brüschweiler, Anal. Chem., 2019, 91, 2304–2311 CrossRef PubMed.
- E. Martineau, J. Dumez and P. Giraudeau, Magn. Reson. Chem., 2020, 58, 390–403 CrossRef CAS PubMed.
- E. Martineau and P. Giraudeau, Methods Mol. Biol., 2019, 2037, 365–383 CrossRef CAS PubMed.
- B. Féraud, E. Martineau, J. Leenders, B. Govaerts, P. de Tullio and P. Giraudeau, Metabolomics, 2020, 16, 42 CrossRef PubMed.
- T. Jezequel, C. Deborde, M. Maucourt, V. Zhendre, A. Moing and P. Giraudeau, Metabolomics, 2015, 11, 1231–1242 CrossRef CAS.
- M. Eden, Molecules, 2020, 25, 2720 CrossRef CAS PubMed.
- K. Chen, Int. J. Mol. Sci., 2020, 21, 5666 CrossRef CAS PubMed.
- H. Jensen and H. Bertram, Metabolomics, 2019, 15, 44 CrossRef PubMed.
- E. Andrew, A. Bradbury and R. Eades, Nature, 1958, 182, 1659 CrossRef CAS.
- O. Pecher, D. Halat, J. Lee, Z. Liu, K. Griffith, M. Braun and C. Grey, J. Magn. Reson., 2017, 275, 127–136 CrossRef CAS PubMed.
- P. Mazzei and A. Piccolo, Chem. Biol. Technol. Agric., 2017, 4, 11 CrossRef.
- R. Mega, F. Abe, J. S. Kim, Y. Tsuboi, K. Tanaka, H. Kobayashi, Y. Sakata, K. Hanada, H. Tsujimoto, J. Kikuchi, S. R. Cutler and M. Okamoto, Nat. Plants, 2019, 5, 153–159 CrossRef CAS PubMed.
- T. Mori, E. Chikayama, Y. Tsuboi, N. Ishida, N. Shisa, Y. Noritake, S. Moriya and J. Kikuchi, Carbohydr. Polym., 2012, 90, 1197–1203 CrossRef CAS PubMed.
- K. Okushita, T. Komatsu, E. Chikayama and J. Kikuchi, Polym. J., 2012, 44, 895–900 CrossRef CAS.
- K. Okushita, E. Chikayama and J. Kikuchi, Biomacromolecules, 2012, 13, 1323–1330 CrossRef CAS PubMed.
- T. Komatsu, T. Kobayashi, M. Hatanaka and J. Kikuchi, Environ. Sci. Technol., 2015, 49, 7056–7062 CrossRef CAS PubMed.
- S. Yamada, K. Ito, A. Kurotani, Y. Yamada, E. Chikayama and J. Kikuchi, ACS Omega, 2019, 4, 3361–3369 CrossRef CAS PubMed.
- D. Besghini, M. Mauri and R. Simonutti, Appl. Sci., 2019, 9, 1801 CrossRef CAS.
- B. Blumich, J. Magn. Reson., 2019, 306, 27–35 CrossRef PubMed.
- K. Okada, D. Hirai, S. Kumada, A. Kosugi, Y. Hayashi and Y. Onuki, J. Pharm. Sci., 2019, 108, 451–456 CrossRef CAS PubMed.
- E. Nikolskaya and Y. Hiltunen, Appl. Magn. Reson., 2018, 49, 185–193 CrossRef CAS PubMed.
- C. Windt, M. Nabel, J. Kochs, S. Jahnke and U. Schurr, Front. Plant Sci., 2021, 12, 617768 CrossRef PubMed.
- L. Colucci, K. Corapi, M. Li, X. Parada, A. Allegretti, H. Lin, D. Ausiello, M. Rosen and M. Cima, Sci. Transl. Med., 2019, 11, eaau1749 CrossRef PubMed.
- J. van Duynhoven, A. Voda, M. Witek, H. Van As and G. Webb, Annu. Rep. NMR Spectrosc., 2010, 69, 145–197 CrossRef CAS.
- E. Kirtil, S. Cikrikci, M. J. McCarthy and M. H. Oztop, Curr. Opin. Food Sci., 2017, 17, 9–15 CrossRef.
- B. Blumich and K. Singh, Angew. Chem., Int. Ed., 2018, 57, 6996–7010 CrossRef PubMed.
- V. Rantzsch, M. Haas, M. B. Ozen, K. F. Ratzsch, K. Riazi, S. Kauffmann-Weiss, J. K. Palacios, A. J. Muller, I. Vittorias, G. Guthausen and M. Wilhelm, Polymer, 2018, 145, 162–173 CrossRef.
- P. Berman, O. Levi, Y. Parmet, M. Saunders and Z. Wiesman, Concepts Magn. Reson., Part A, 2013, 42, 72–88 CrossRef PubMed.
- P. Sagmeister, J. Poms, J. D. D. Williams and C. O. Kappe, React. Chem. Eng., 2020, 5, 677–684 RSC.
- P. Giraudeau and F. X. Felpin, React. Chem. Eng., 2018, 3, 399–413 RSC.
- D. Bouillaud, V. Heredia, T. Castaing-Cordier, D. Drouin, B. Charrier, O. Goncalves, J. Farjon and P. Giraudeau, Algal Res., 2019, 43, 101624 CrossRef.
- D. Bouillaud, D. Drouin, B. Charrier, C. Jacquemmoz, J. Farjon, P. Giraudeau and O. Goncalves, Process Biochem., 2020, 93, 63–68 CrossRef CAS.
- L. A. Colnago, Z. Wiesman, G. Pages, M. Musse, T. Monaretto, C. W. Windt and C. Rondeau-Mouro, J. Magn. Reson., 2021, 323, 106899 CrossRef CAS PubMed.
- M. Grootveld, B. Percival, M. Gibson, Y. Osman, M. Edgar, M. Molinari, M. Mather, F. Casanova and P. Wilson, Anal. Chim. Acta, 2019, 1067, 11–30 CrossRef CAS PubMed.
- E. Chikayama, R. Yamashina, K. Komatsu, Y. Tsuboi, K. Sakata, J. Kikuchi and Y. Sekiyama, Metabolites, 2016, 6, 36 CrossRef PubMed.
- J. M. Granda, L. Donina, V. Dragone, D. L. Long and L. Cronin, Nature, 2018, 559, 377–381 CrossRef CAS PubMed.
- M. K. Sørensen, N. M. Balsgart, O. Jensen, N. C. Nielsen and T. Vosegaard, ChemPhysChem, 2018, 19, 2985–2988 CrossRef PubMed.
- J. Dumez, Prog. Nucl. Magn. Reson. Spectrosc., 2018, 109, 101–134 CrossRef CAS PubMed.
- J. N. Dumez, J. Magn. Reson., 2021, 323, 106817 CrossRef CAS PubMed.
- K. Ito, Y. Tsuboi and J. Kikuchi, Commun. Chem., 2020, 3, 80 CrossRef CAS.
- B. Worley, S. Halouska and R. Powers, Anal. Biochem., 2013, 433, 102–104 CrossRef CAS PubMed.
- T. Ebbels and R. Cavill, Prog. Nucl. Magn. Reson. Spectrosc., 2009, 55, 361–374 CrossRef CAS.
- A. Smolinska, L. Blanchet, L. Buydens and S. Wijmenga, Anal. Chim. Acta, 2012, 750, 82–97 CrossRef CAS PubMed.
- Y. Xi and D. Rocke, BMC Bioinf., 2008, 9, 324 CrossRef PubMed.
- T. N. Vu and K. Laukens, Metabolites, 2013, 3, 259–276 CrossRef CAS PubMed.
- P. E. Anderson, N. V. Reo, N. J. DelRaso, T. E. Doom and M. L. Raymer, Metabolomics, 2008, 4, 261–272 CrossRef CAS.
- A. Craig, O. Cloareo, E. Holmes, J. Nicholson and J. Lindon, Anal. Chem., 2006, 78, 2262–2267 CrossRef CAS PubMed.
- S. Fukuda, Y. Nakanishi, E. Chikayama, H. Ohno, T. Hino and J. Kikuchi, PLoS One, 2009, 4, e4893 CrossRef PubMed.
- F. Dieterle, A. Ross, G. Schlotterbeck and H. Senn, Anal. Chem., 2006, 78, 4281–4290 CrossRef CAS PubMed.
- H. Hotelling, J. Educ. Psychol., 1933, 24, 498–520 CrossRef.
- S. Wold, M. Sjostrom and L. Eriksson, Chemom. Intell. Lab. Syst., 2001, 58, 109–130 CrossRef CAS.
- Z. Ramadan, D. Jacobs, M. Grigorov and S. Kochhar, Talanta, 2006, 68, 1683–1691 CrossRef CAS PubMed.
- D. M. O. Ogawa, S. Moriya, Y. Tsuboi, Y. Date, A. R. B. Prieto-da-Silva, G. Radis-Baptista, T. Yamane and J. Kikuchi, PLoS One, 2014, 9, e110723 CrossRef PubMed.
- T. Ogura, R. Hoshino, Y. Date and J. Kikuchi, Metabolites, 2016, 6, 7 CrossRef PubMed.
- Y. Date, T. Iikura, A. Yamazawa, S. Moriya and J. Kikuchi, J. Proteome Res., 2012, 11, 5602–5610 CrossRef CAS PubMed.
- Y. Shiokawa, T. Misawa, Y. Date and J. Kikuchi, Anal. Chem., 2016, 88, 2714–2719 CrossRef CAS PubMed.
- M. Li, B. H. Wang, M. H. Zhang, M. Rantalainen, S. Y. Wang, H. K. Zhou, Y. Zhang, J. Shen, X. Y. Pang, M. L. Zhang, H. Wei, Y. Chen, H. F. Lu, J. Zuo, M. M. Su, Y. P. Qiu, W. Jia, C. N. Xiao, L. M. Smith, S. L. Yang, E. Holmes, H. R. Tang, G. P. Zhao, J. K. Nicholson, L. J. Li and L. P. Zhao, Proc. Natl. Acad. Sci. U. S. A., 2008, 105, 2117–2122 CrossRef CAS PubMed.
- H. Sugahara, T. Odamaki, S. Fukuda, T. Kato, J. Z. Xiao, F. Abe, J. Kikuchi and H. Ohno, Sci. Rep., 2015, 5, 13548 CrossRef CAS PubMed.
- H. Morita, H. Toh, S. Fukuda, H. Horikawa, K. Oshima, T. Suzuki, M. Murakami, S. Hisamatsu, Y. Kato, T. Takizawa, H. Fukuoka, T. Yoshimura, K. Itoh, D. J. O'Sullivan, L. L. McKay, H. Ohno, J. Kikuchi, T. Masaoka and M. Hattori, DNA Res., 2008, 15, 151–161 CrossRef CAS PubMed.
- T. Kato, S. Fukuda, A. Fujiwara, W. Suda, M. Hattori, J. Kikuchi and H. Ohno, DNA Res., 2014, 21, 469–480 CrossRef CAS PubMed.
- F. Wei, K. Ito, K. Sakata, T. Asakura, Y. Date and J. Kikuchi, Sci. Rep., 2021, 11, 3766 CrossRef CAS PubMed.
- J. G. Bundy, H. C. Keun, J. K. Sidhu, D. J. Spurgeon, C. Svendsen, P. Kille and A. J. Morgan, Environ. Sci. Technol., 2007, 41, 4458–4464 CrossRef CAS PubMed.
- T. W. Collette, Q. Teng, K. M. Jensen, M. D. Kahl, E. A. Makynen, E. J. Durhan, D. L. Villeneuve, D. Martinovic-Weigelt, G. T. Ankley and D. R. Ekman, Environ. Sci. Technol., 2010, 44, 6881–6886 CrossRef CAS PubMed.
- R. P. Ellis, J. I. Spicer, J. J. Byrne, U. Sommer, M. R. Viant, D. A. White and S. Widdicombe, Environ. Sci. Technol., 2014, 48, 7044–7052 CrossRef CAS PubMed.
- L. M. Samuelsson, B. Bjorlenius, L. Forlin and D. G. Larsson, Environ. Sci. Technol., 2011, 45, 1703–1710 CrossRef CAS PubMed.
- M. L. Whitfield Aslund, H. McShane, M. J. Simpson, A. J. Simpson, J. K. Whalen, W. H. Hendershot and G. I. Sunahara, Environ. Sci. Technol., 2012, 46, 1111–1118 CrossRef CAS PubMed.
- H. Zacharias, M. Altenbuchinger and W. Gronwald, Metabolites, 2018, 8, 47 CrossRef PubMed.
- J. Lindon and J. Nicholson, Annu. Rev. Anal. Chem., 2008, 1, 45–69 CrossRef CAS PubMed.
- E. Riekeberg and R. Powers, F1000Research, 2017, 6, 1148 Search PubMed.
- G. A. Nagana Gowda and D. Raftery, Anal. Chem., 2017, 89, 490–510 CrossRef CAS PubMed.
- A. Vignoli, V. Ghini, G. Meoni, C. Licari, P. Takis, L. Tenori, P. Turano and C. Luchinat, Angew. Chem., Int. Ed., 2019, 58, 968–994 CrossRef CAS PubMed.
- K. Bingol, L. Bruschweiler-Li, D. Li, B. Zhang, M. Xie and R. Bruschweiler, Bioanalysis, 2016, 8, 557–573 CrossRef CAS PubMed.
- P. Takis, V. Ghini, L. Tenori, P. Turano and C. Luchinat, TrAC, Trends Anal. Chem., 2019, 120, 115300 CrossRef.
- J. Marchand, E. Martineau, Y. Guitton, G. Dervilly-Pinel and P. Giraudeau, Curr. Opin. Biotechnol., 2017, 43, 49–55 CrossRef CAS PubMed.
- D. S. Wishart, J. Magn. Reson., 2019, 306, 155–161 CrossRef CAS PubMed.
- P. Giraudeau, Analyst, 2020, 145, 2457–2472 RSC.
- J. L. Markley, R. Brüschweiler, A. S. Edison, H. R. Eghbalnia, R. Powers, D. Raftery and D. S. Wishart, Curr. Opin. Biotechnol., 2017, 43, 34–40 CrossRef CAS PubMed.
- A. H. Emwas, R. Roy, R. T. McKay, L. Tenori, E. Saccenti, G. A. N. Gowda, D. Raftery, F. Alahmari, L. Jaremko, M. Jaremko and D. S. Wishart, Metabolites, 2019, 9, 123 CrossRef CAS PubMed.
- P. Giraudeau, V. Silvestre and S. Akoka, Metabolomics, 2015, 11, 1041–1055 CrossRef CAS.
- H. Ashrafian, V. Sounderajah, R. Glen, T. Ebbels, B. J. Blaise, D. Kalra, K. Kultima, O. Spjuth, L. Tenori, R. Salek, N. Kale, K. Haug, D. Schober, P. Rocca-Serra, C. O'Donovan, C. Steinbeck, I. Cano, P. de Atauri and M. Cascante, Med. Princ. Pract., 2020, 30, 301–310 CrossRef PubMed.
- P. Mercier, M. Lewis, D. Chang, D. Baker and D. Wishart, J. Biomol. NMR, 2011, 49, 307–323 CrossRef CAS PubMed.
- R. Consonni and L. Cagliani, Magn. Reson. Chem., 2019, 57, 558–578 CrossRef CAS PubMed.
- E. Hatzakis, Compr. Rev. Food Sci. Food Saf., 2019, 18, 189–220 CrossRef PubMed.
- C. Deborde, A. Moing, L. Roch, D. Jacob, D. Rolin and P. Giraudeau, Prog. Nucl. Magn. Reson. Spectrosc., 2017, 102, 61–97 CrossRef PubMed.
- H. Kim and R. Verpoorte, Phytochem. Anal., 2010, 21, 4–13 CrossRef CAS PubMed.
- M. Halabalaki, S. Bertrand, A. Stefanou, K. Gindro, S. Kostidis, E. Mikros, L. Skaltsounis and J. Wolfender, Phytochem. Anal., 2014, 25, 350–356 CrossRef CAS PubMed.
- E. Mahrous and M. Farag, J. Adv. Res., 2015, 6, 3–15 CrossRef CAS PubMed.
- J. Schripsema, Phytochem. Anal., 2010, 21, 14–21 CrossRef CAS PubMed.
- J. Pontes, A. Brasil, G. Cruz, R. de Souza and L. Tasic, Anal. Methods, 2017, 9, 1078–1096 RSC.
- S. Lodge, P. Nitschke, T. Kimhofer, J. D. Coudert, S. Begum, S. H. Bong, T. Richards, D. Edgar, E. Raby, M. Spraul, H. Schaefer, J. C. Lindon, R. L. Loo, E. Holmes and J. K. Nicholson, J. Proteome Res., 2021, 20, 1382–1396 CrossRef CAS PubMed.
- B. Zhang and R. Powers, Future Med. Chem., 2012, 4, 1273–1306 CrossRef CAS PubMed.
- M. Vincenzi and M. Leone, Curr. Med. Chem., 2021, 28, 4380–4453 CrossRef CAS PubMed.
- J. G. Bundy, M. P. Davey and M. R. Viant, Metabolomics, 2008, 5, 3 CrossRef.
- M. Bastawrous, A. Jenne, M. Tabatabaei Anaraki and A. J. Simpson, Metabolites, 2018, 8, 35 CrossRef PubMed.
- D. Jacob, C. Deborde and A. Moing, Anal. Bioanal. Chem., 2013, 405, 5049–5061 CrossRef CAS PubMed.
- L. Euceda, G. Giskeodegard and T. Bathen, Scand. J. Clin. Lab. Invest., 2015, 75, 193–203 CrossRef CAS PubMed.
- A. Dona, M. Kyriakides, F. Scott, E. Shephard, D. Varshavi, K. Veselkov and J. Everett, Comput. Struct. Biotechnol. J., 2016, 14, 135–153 CrossRef CAS PubMed.
- I. Garcia-Perez, J. M. Posma, J. I. Serrano-Contreras, C. L. Boulangé, Q. Chan, G. Frost, J. Stamler, P. Elliott, J. C. Lindon, E. Holmes and J. K. Nicholson, Nat. Protoc., 2020, 15, 2538–2567 CrossRef CAS PubMed.
- V. Aguiar-Pulido, W. Huang, V. Suarez-Ulloa, T. Cickovski, K. Mathee and G. Narasimhan, Evol. Bioinf. Online, 2016, 12, 5–16 Search PubMed.
- S. R. Johnson and B. M. Lange, Front. Bioeng. Biotechnol., 2015, 3, 22 Search PubMed.
- J. L. Markley, H. Dashti, J. R. Wedell, W. M. Westler and H. R. Eghbalnia, Methods Mol. Biol., 2019, 2037, 413–427 CrossRef CAS PubMed.
- Q. Yang, Y. Wang, Y. Zhang, F. Li, W. Xia, Y. Zhou, Y. Qiu, H. Li and F. Zhu, Nucleic Acids Res., 2020, 48, W436–W448 CrossRef CAS PubMed.
- K. Peters, J. Bradbury, S. Bergmann, M. Capuccini, M. Cascante, P. de Atauri, T. M. D. Ebbels, C. Foguet, R. Glen, A. Gonzalez-Beltran, U. L. Günther, E. Handakas, T. Hankemeier, K. Haug, S. Herman, P. Holub, M. Izzo, D. Jacob, D. Johnson, F. Jourdan, N. Kale, I. Karaman, B. Khalili, P. Emami Khonsari, K. Kultima, S. Lampa, A. Larsson, C. Ludwig, P. Moreno, S. Neumann, J. A. Novella, C. O'Donovan, J. T. M. Pearce, A. Peluso, M. E. Piras, L. Pireddu, M. A. C. Reed, P. Rocca-Serra, P. Roger, A. Rosato, R. Rueedi, C. Ruttkies, N. Sadawi, R. M. Salek, S. A. Sansone, V. Selivanov, O. Spjuth, D. Schober, E. A. Thévenot, M. Tomasoni, M. van Rijswijk, M. van Vliet, M. R. Viant, R. J. M. Weber, G. Zanetti and C. Steinbeck, GigaScience, 2019, 8, 1–12 CrossRef PubMed.
- L. Margueritte, P. Markov, L. Chiron, J. P. Starck, C. Vonthron-Sénécheau, M. Bourjot and M. A. Delsuc, Magn. Reson. Chem., 2018, 56, 469–479 CrossRef CAS PubMed.
- J. Xia, N. Psychogios, N. Young and D. S. Wishart, Nucleic Acids Res., 2009, 37, W652–W660 CrossRef CAS PubMed.
- J. Xia, R. Mandal, I. V. Sinelnikov, D. Broadhurst and D. S. Wishart, Nucleic Acids Res., 2012, 40, W127–W133 CrossRef CAS PubMed.
- J. Xia, I. V. Sinelnikov, B. Han and D. S. Wishart, Nucleic Acids Res., 2015, 43, W251–W257 CrossRef CAS PubMed.
- K. Schaler, M. Roos, P. Micke, Y. Golitsyn, A. Seidlitz, T. Thurn-Albrecht, H. Schneider, G. Hempel and K. Saalwachter, Solid State Nucl. Magn. Reson., 2015, 72, 50–63 CrossRef PubMed.
- I. Toumi, S. Caldarelli and B. Torrésani, Prog. Nucl. Magn. Reson. Spectrosc., 2014, 81, 37–64 CrossRef CAS PubMed.
- H. Schneider, K. Saalwachter and M. Roos, Macromolecules, 2017, 50, 8598–8610 CrossRef CAS.
- M. Weingarth, P. Tekely, R. Bruschweiler and G. Bodenhausen, Chem. Commun., 2010, 46, 952–954 RSC.
- M. Bak, J. Rasmussen and N. Nielsen, J. Magn. Reson., 2000, 147, 296–330 CrossRef CAS PubMed.
- M. Veshtort and R. Griffin, J. Magn. Reson., 2006, 178, 248–282 CrossRef CAS PubMed.
- D. Massiot, F. Fayon, M. Capron, I. King, S. Le Calve, B. Alonso, J. Durand, B. Bujoli, Z. Gan and G. Hoatson, Magn. Reson. Chem., 2002, 40, 70–76 CrossRef CAS.
- D. Grimminck, B. van Meerten, M. Verkuijlen, E. van Eck, W. Meerts and A. Kentgens, J. Magn. Reson., 2013, 228, 116–124 CrossRef CAS PubMed.
- A. Smith, J. Biomol. NMR, 2017, 67, 77–94 CrossRef CAS PubMed.
- M. Wojdyr, J. Appl. Crystallogr., 2010, 43, 1126–1128 CrossRef CAS.
- S. van Meerten, W. Franssen and A. Kentgens, J. Magn. Reson., 2019, 301, 56–66 CrossRef CAS PubMed.
- S. Yamada, E. Chikayama and J. Kikuchi, Int. J. Mol. Sci., 2021, 22, 1086 CrossRef CAS PubMed.
- Y. Kusaka, T. Hasegawa and H. Kaji, J. Phys. Chem. A, 2019, 123, 10333–10338 CrossRef CAS PubMed.
- B. Demchak, T. Hull, M. Reich, T. Liefeld, M. Smoot, T. Ideker and J. P. Mesirov, F1000Research, 2014, 3, 151 Search PubMed.
- T. J. van den Broek, B. H. Kremer, M. Marcondes Rezende, F. P. Hoevenaars, P. Weber, U. Hoeller, B. van Ommen and S. Wopereis, Genes Nutr., 2017, 12, 5 CrossRef PubMed.
- M. Kim, Y. Nam and H. Shin, BMC Med. Inf. Decis. Making, 2017, 17, 52 CrossRef PubMed.
- M. Luck, G. Bertho, M. Bateson, A. Karras, A. Yartseva, E. Thervet, C. Damon and N. Pallet, PLoS One, 2016, 11, e0166905 CrossRef PubMed.
- M. Pupier, J. M. Nuzillard, J. Wist, N. E. Schlörer, S. Kuhn, M. Erdelyi, C. Steinbeck, A. J. Williams, C. Butts, T. D. W. Claridge, B. Mikhova, W. Robien, H. Dashti, H. R. Eghbalnia, C. Farès, C. Adam, P. Kessler, F. Moriaud, M. Elyashberg, D. Argyropoulos, M. Pérez, P. Giraudeau, R. R. Gil, P. Trevorrow and D. Jeannerat, Magn. Reson. Chem., 2018, 56, 703–715 CrossRef CAS PubMed.
- S. Kuhn and N. Schlorer, Magn. Reson. Chem., 2015, 53, 582–589 CrossRef CAS PubMed.
- D. Schober, D. Jacob, M. Wilson, J. A. Cruz, A. Marcu, J. R. Grant, A. Moing, C. Deborde, L. F. de Figueiredo, K. Haug, P. Rocca-Serra, J. Easton, T. M. D. Ebbels, J. Hao, C. Ludwig, U. L. Günther, A. Rosato, M. S. Klein, I. A. Lewis, C. Luchinat, A. R. Jones, A. Grauslys, M. Larralde, M. Yokochi, N. Kobayashi, A. Porzel, J. L. Griffin, M. R. Viant, D. S. Wishart, C. Steinbeck, R. M. Salek and S. Neumann, Anal. Chem., 2018, 90, 649–656 CrossRef CAS PubMed.
- E. L. Ulrich, H. Akutsu, J. F. Doreleijers, Y. Harano, Y. E. Ioannidis, J. Lin, M. Livny, S. Mading, D. Maziuk, Z. Miller, E. Nakatani, C. F. Schulte, D. E. Tolmie, R. Kent Wenger, H. Yao and J. L. Markley, Nucleic Acids Res., 2008, 36, D402–D408 CrossRef CAS PubMed.
- E. L. Ulrich, K. Baskaran, H. Dashti, Y. E. Ioannidis, M. Livny, P. R. Romero, D. Maziuk, J. R. Wedell, H. Yao, H. R. Eghbalnia, J. C. Hoch and J. L. Markley, J. Biomol. NMR, 2019, 73, 5–9 CrossRef CAS PubMed.
- S. Kawashima, T. Katayama, H. Hatanaka, T. Kushida and T. Takagi, Database, 2018, 2018, bay123 CrossRef PubMed.
- A. Kinjo, H. Suzuki, R. Yamashita, Y. Ikegawa, T. Kudou, R. Igarashi, Y. Kengaku, H. Cho, D. Standley, A. Nakagawa and H. Nakamura, Nucleic Acids Res., 2012, 40, D453–D460 CrossRef CAS PubMed.
- M. Yokochi, N. Kobayashi, E. Ulrich, A. Kinjo, T. Iwata, Y. Ioannidis, M. Livny, J. Markley, H. Nakamura, C. Kojima and T. Fujiwara, J. Biomed. Semant., 2016, 7, 16 CrossRef PubMed.
- A. Alonso, S. Marsal and A. Julià, Front. Bioeng. Biotechnol., 2015, 3, 23 Search PubMed.
- D. S. Wishart, T. Jewison, A. C. Guo, M. Wilson, C. Knox, Y. Liu, Y. Djoumbou, R. Mandal, F. Aziat, E. Dong, S. Bouatra, I. Sinelnikov, D. Arndt, J. Xia, P. Liu, F. Yallou, T. Bjorndahl, R. Perez-Pineiro, R. Eisner, F. Allen, V. Neveu, R. Greiner and A. Scalbert, Nucleic Acids Res., 2013, 41, D801–D807 CrossRef CAS PubMed.
- Q. Cui, I. A. Lewis, A. D. Hegeman, M. E. Anderson, J. Li, C. F. Schulte, W. M. Westler, H. R. Eghbalnia, M. R. Sussman and J. L. Markley, Nat. Biotechnol., 2008, 26, 162–164 CrossRef CAS PubMed.
- C. Ludwig, J. Easton, A. Lodi, S. Tiziani, S. Manzoor, A. Southam, J. Byrne, L. Bishop, S. He, T. Arvanitis, U. Gunther and M. Viant, Metabolomics, 2012, 8, 8–18 CrossRef CAS.
- J. Lopez-Perez, R. Theron, E. del Olmo and D. Diaz, Bioinformatics, 2007, 23, 3256–3257 CrossRef CAS PubMed.
- H. Ferry-Dumazet, L. Gil, C. Deborde, A. Moing, S. Bernillon, D. Rolin, M. Nikolski, A. de Daruvar and D. Jacob, BMC Plant Biol., 2011, 11, 104 CrossRef PubMed.
- J. Fischedick, S. Johnson, R. Ketchum, R. Croteau and B. Lange, Phytochemistry, 2015, 113, 87–95 CrossRef CAS PubMed.
- O. Yamamoto, K. Someno, N. Wasada, J. Hiraishi, K. Hayamizu, K. Tanabe, T. Tamura and M. Yanagisawa, Anal. Sci., 1988, 4, 233–239 CrossRef CAS.
- R. Ma and T. Luo, J. Chem. Inf. Model., 2020, 60, 4684–4690 CrossRef CAS PubMed.
- H. D. Tran, C. Kim, L. Chen, A. Chandrasekaran, R. Batra, S. Venkatram, D. Kamal, J. P. Lightstone, R. Gurnani, P. Shetty, M. Ramprasad, J. Laws, M. Shelton and R. Ramprasad, J. Appl. Phys., 2020, 128, 171104 CrossRef.
- E. Chikayama, Y. Sekiyama, M. Okamoto, Y. Nakanishi, Y. Tsuboi, K. Akiyama, K. Saito, K. Shinozaki and J. Kikuchi, Anal. Chem., 2010, 82, 1653–1658 CrossRef CAS PubMed.
- J. Kikuchi, Y. Tsuboi, K. Komatsu, M. Gomi, E. Chikayama and Y. Date, Anal. Chem., 2016, 88, 659–665 CrossRef CAS PubMed.
- Y. Ogata, E. Chikayama, Y. Morioka, R. Everroad, A. Shino, A. Matsushima, H. Haruna, S. Moriya, T. Toyoda and J. Kikuchi, PLoS One, 2012, 7, e30263 CrossRef CAS PubMed.
- D. Wishart, TrAC, Trends Anal. Chem., 2008, 27, 228–237 CrossRef CAS.
- S. Robinette, F. Zhang, L. Bruschweiler-Li and R. Bruschweiler, Anal. Chem., 2008, 80, 3606–3611 CrossRef CAS PubMed.
- K. Bingol, D. W. Li, L. Bruschweiler-Li, O. A. Cabrera, T. Megraw, F. Zhang and R. Brüschweiler, ACS Chem. Biol., 2015, 10, 452–459 CrossRef CAS PubMed.
- K. Bingol, D. Li, B. Zhang and R. Bruschweiler, Anal. Chem., 2016, 88, 12411–12418 CrossRef CAS PubMed.
- K. Bingol, F. Zhang, L. Bruschweiler-Li and R. Brüschweiler, Anal. Chem., 2012, 84, 9395–9401 CrossRef CAS PubMed.
- K. Bingol, L. Bruschweiler-Li, D. W. Li and R. Brüschweiler, Anal. Chem., 2014, 86, 5494–5501 CrossRef CAS PubMed.
- C. Wang, I. Timári, B. Zhang, D. W. Li, A. Leggett, A. O. Amer, L. Bruschweiler-Li, R. E. Kopec and R. Brüschweiler, J. Proteome Res., 2020, 19, 1674–1683 CrossRef CAS PubMed.
- L. J. Clos, M. F. Jofre, J. J. Ellinger, W. M. Westler and J. L. Markley, Metabolomics, 2013, 9, 558–563 CrossRef CAS PubMed.
- J. Helmus and C. Jaroniec, J. Biomol. NMR, 2013, 55, 355–367 CrossRef CAS PubMed.
- M. Badenhorst, C. Barry, C. Swanepoel, C. van Staden, J. Wissing and J. Rohwer, Processes, 2019, 7, 460 CrossRef CAS.
- A. Smelter, M. Astra and H. N. Moseley, BMC Bioinf., 2017, 18, 175 CrossRef PubMed.
- A. Alonso, M. A. Rodríguez, M. Vinaixa, R. Tortosa, X. Correig, A. Julià and S. Marsal, Anal. Chem., 2014, 86, 1160–1169 CrossRef CAS PubMed.
- J. Teixeira, S. Skinner, M. Arbesu, A. Breeze and M. Pons, J. Biomol. NMR, 2018, 71, 1–9 CrossRef CAS PubMed.
- A. Verhoeven, M. Giera and O. Mayboroda, Anal. Chim. Acta, 2018, 1044, 66–76 CrossRef CAS PubMed.
- J. Stanstrup, C. Broeckling, R. Helmus, N. Hoffmann, E. Mathe, T. Naake, L. Nicolotti, K. Peters, J. Rainer, R. Salek, T. Schulze, E. Schymanski, M. Stravs, E. Thevenot, H. Treutler, R. Weber, E. Willighagen, M. Witting and S. Neumann, Metabolites, 2019, 9, 200 CrossRef CAS PubMed.
- F. Madrid-Gambin, S. Oller-Moreno, L. Fernandez, S. Bartova, M. Giner, C. Joyce, F. Ferraro, I. Montoliu, S. Moco and S. Marco, Bioinformatics, 2020, 36, 2943–2945 CrossRef CAS PubMed.
- C. Beirnaert, P. Meysman, T. Vu, N. Hermans, S. Apers, L. Pieters, A. Covaci and K. Laukens, PLoS Comput. Biol., 2018, 14, e1006018 CrossRef PubMed.
- M. Martin, B. Legat, J. Leenders, J. Vanwinsberghe, R. Rousseau, B. Boulanger, P. Eilers, P. De Tullio and B. Govaerts, Anal. Chim. Acta, 2018, 1019, 1–13 CrossRef CAS PubMed.
- S. Ren, A. A. Hinzman, E. L. Kang, R. D. Szczesniak and L. J. Lu, Metabolomics, 2015, 11, 1492–1513 CrossRef CAS.
- P. Gromski, H. Muhamadali, D. Ellis, Y. Xu, E. Correa, M. Turner and R. Goodacre, Anal. Chim. Acta, 2015, 879, 10–23 CrossRef CAS PubMed.
- L. Breiman, Mach. Learn., 2001, 45, 5–32 CrossRef.
- J. Hochrein, M. S. Klein, H. U. Zacharias, J. Li, G. Wijffels, H. J. Schirra, R. Spang, P. J. Oefner and W. Gronwald, J. Proteome Res., 2012, 11, 6242–6251 CrossRef CAS PubMed.
- C. Strobl, A. L. Boulesteix, A. Zeileis and T. Hothorn, BMC Bioinf., 2007, 8, 25 CrossRef PubMed.
- S. Mahadevan, S. L. Shah, T. J. Marrie and C. M. Slupsky, Anal. Chem., 2008, 80, 7562–7570 CrossRef CAS PubMed.
- Y. Li, H. Zhou, J. Xie, M. Ally, Z. Hou, Y. Xu and Y. Zhang, Evidence-Based Complementary Altern. Med., 2016, 2016, 6012761 Search PubMed.
- J. Heinemann, A. Mazurie, M. Tokmina-Lukaszewska, G. J. Beilman and B. Bothner, Metabolomics, 2014, 10, 1121–1128 CrossRef CAS.
- P. W. T. Krooshof, B. Ustun, G. J. Postma and L. M. C. Buydens, Anal. Chem., 2010, 82, 7000–7007 CrossRef CAS PubMed.
- X. Huang, Q. Xu, Y. Yun, J. Huang and Y. Liang, Chemom. Intell. Lab. Syst., 2015, 146, 365–370 CrossRef CAS.
- K. M. Mendez, D. I. Broadhurst and S. N. Reinke, Metabolomics, 2019, 15, 142 CrossRef PubMed.
- D. C. Chen, Z. Wang, D. Guo, V. Orekhov and X. B. Qu, Chem.–Eur. J., 2020, 26, 10391–10401 CrossRef CAS PubMed.
- K. Ito, Y. Obuchi, E. Chikayama, Y. Date and J. Kikuchi, Chem. Sci., 2018, 9, 8213–8220 RSC.
- K. Ito, X. Xu and J. Kikuchi, Anal. Chem., 2021, 93, 6901–6906 CrossRef CAS PubMed.
- H. Shima, S. Masuda, Y. Date, A. Shino, Y. Tsuboi, M. Kajikawa, Y. Inoue, T. Kanamoto and J. Kikuchi, Nutrients, 2017, 9, 1307 CrossRef PubMed.
- Y. Shiokawa, Y. Date and J. Kikuchi, Sci. Rep., 2018, 8, 3426 CrossRef PubMed.
- Y. Date, F. F. Wei, Y. Tsuboi, K. Ito, K. Sakata and J. Kikuchi, BMC Chem., 2021, 15, 13 CrossRef CAS PubMed.
- D. F. Hansen, J. Biomol. NMR, 2019, 73, 577–585 CrossRef CAS PubMed.
- J. Luo, Q. Zeng, K. Wu and Y. Lin, J. Magn. Reson., 2020, 317, 106772 CrossRef CAS PubMed.
- A. Kurotani, T. Kakiuchi and J. Kikuchi, ACS Omega, 2021, 6, 14278–14287 CrossRef CAS PubMed.
- N. Kobayashi, Y. Hattori, T. Nagata, S. Shinya, P. Guntert, C. Kojima and T. Fujiwara, Bioinformatics, 2018, 34, 4300–4301 CrossRef CAS PubMed.
- X. B. Qu, Y. H. Huang, H. F. Lu, T. Y. Qiu, D. Guo, T. Agback, V. Orekhov and Z. Chen, Angew. Chem., Int. Ed., 2020, 59, 10297–10300 CrossRef CAS PubMed.
- K. Wu, J. Luo, Q. Zeng, X. Dong, J. Chen, C. Zhan, Z. Chen and Y. Lin, Anal. Chem., 2021, 93, 1377–1382 CrossRef CAS PubMed.
- J. Zhang, K. Terayama, M. Sumita, K. Yoshizoe, K. Ito, J. Kikuchi and K. Tsuda, Sci. Technol. Adv. Mater., 2020, 21, 552–561 CrossRef CAS PubMed.
- E. A. Chatzimichali and C. Bessant, Metabolomics, 2016, 12, 16 CrossRef PubMed.
- X. T. Shen, X. Y. Gong, Y. P. Cai, Y. Guo, J. Tu, H. Li, T. Zhang, J. L. Wang, F. Z. Xue and Z. J. Zhu, Metabolomics, 2016, 12, 89 CrossRef.
- M. Kuhn, J. Stat. Software, 2008, 28, 1–26 Search PubMed.
- B. Bischl, M. Lang, L. Kotthoff, J. Schiffner, J. Richter, E. Studerus, G. Casalicchio and Z. M. Jones, J. Mach. Learn. Res., 2016, 17, 1–5 Search PubMed.
- C. A. Waudby and J. Christodoulou, J. Magn. Reson., 2011, 211, 67–73 CrossRef CAS PubMed.
- J. Lapin and A. A. Nevzorov, J. Magn. Reson., 2020, 310, 106641 CrossRef CAS PubMed.
- E. Jonas and S. Kuhn, J. Cheminf., 2019, 11, 50 Search PubMed.
- S. Kang, Y. Kwon, D. Lee and Y. S. Choi, J. Chem. Inf. Model., 2020, 60, 3765–3769 CrossRef CAS PubMed.
- Y. Pomyen, K. Wanichthanarak, P. Poungsombat, J. Fahrmann, D. Grapov and S. Khoomrung, Comput. Struct. Biotechnol. J., 2020, 18, 2818–2825 CrossRef CAS PubMed.
- J. Lapin and A. Nevzorov, J. Magn. Reson., 2020, 317, 106794 CrossRef CAS PubMed.
- P. P. Man, C. Bonhomme and F. Babonneau, Solid State Nucl. Magn. Reson., 2014, 61–62, 28–34 CrossRef CAS PubMed.
- A. J. Simpson, M. J. Salloum, W. L. Kingery and P. G. Hatcher, J. Environ. Qual., 2002, 31, 388–392 CrossRef CAS PubMed.
- H. A. Abdulla, E. C. Minor and P. G. Hatcher, Environ. Sci. Technol., 2010, 44, 8044–8049 CrossRef CAS PubMed.
- E. Kaiser, A. J. Simpson, K. J. Dria, B. Sulzberger and P. G. Hatcher, Environ. Sci. Technol., 2003, 37, 2929–2935 CrossRef CAS PubMed.
- S. J. Goldberg, G. I. Ball, B. C. Allen, S. G. Schladow, A. J. Simpson, H. Masoom, R. Soong, H. D. Graven and L. I. Aluwihare, Nat. Commun., 2015, 6, 6347 CrossRef CAS PubMed.
- N. Mladenov, Y. Zheng, B. Simone, T. M. Bilinski, D. M. McKnight, D. Nemergut, K. A. Radloff, M. M. Rahman and K. M. Ahmed, Environ. Sci. Technol., 2015, 49, 10815–10824 CrossRef CAS PubMed.
- J. D. Mao, L. Tremblay, J. P. Gagne, S. Kohl, J. Rice and K. Schmidt-Rohr, Geochim. Cosmochim. Acta, 2007, 71, 5483–5499 CrossRef CAS.
- R. C. Everroad, S. Yoshida, Y. Tsuboi, Y. Date, J. Kikuchi and S. Moriya, J. Visualized Exp., 2012, 62, e3163 Search PubMed.
- J. I. Hedges, J. A. Baldock, Y. Gelinas, C. Lee, M. Peterson and S. G. Wakeham, Nature, 2001, 409, 801–804 CrossRef CAS PubMed.
- Y. Gelinas, J. A. Baldock and J. I. Hedges, Science, 2001, 294, 145–148 CrossRef CAS PubMed.
- T. Dittmar, B. Koch, N. Hertkorn and G. Kattner, Limnol Oceanogr. Methods, 2008, 6, 230–235 CrossRef CAS.
- Y. Li, M. Harir, M. Lucio, B. Kanawati, K. Smirnov, R. Flerus, B. P. Koch, P. Schmitt-Kopplin and N. Hertkorn, Anal. Chem., 2016, 88, 6680–6688 CrossRef CAS PubMed.
- N. Hertkorn, R. Benner, M. Frommberger, P. Schmitt-Kopplin, M. Witt, K. Kaiser, A. Kettrup and J. I. Hedges, Geochim. Cosmochim. Acta, 2006, 70, 2990–3010 CrossRef CAS.
- G. C. Woods, M. J. Simpson and A. J. Simpson, Water Res., 2012, 46, 3398–3408 CrossRef CAS PubMed.
- B. Lam, A. Baer, M. Alaee, B. Lefebvre, A. Moser, A. Williams and A. J. Simpson, Environ. Sci. Technol., 2007, 41, 8240–8247 CrossRef CAS PubMed.
- H. Liu, C. Dai, H. Yu, Q. Guo, J. Li, A. Hao, J. Kikuchi and M. Zhao, Math. Biosci. Eng., 2021, 18, 4101–4126 Search PubMed.
- H. Haberl, K. H. Erb, F. Krausmann, V. Gaube, A. Bondeau, C. Plutzar, S. Gingrich, W. Lucht and M. Fischer-Kowalski, Proc. Natl. Acad. Sci. U. S. A., 2007, 104, 12942–12947 CrossRef CAS PubMed.
- R. Costanza, B. Fisher, K. Mulder, S. Liu and T. Christopher, Ecol. Econ., 2007, 61, 478–491 CrossRef.
- J. Bruno, K. Boyer, J. Duffy, S. Lee and J. Kertesz, Ecol. Lett., 2005, 8, 1165–1174 CrossRef PubMed.
- M. Balunas and A. Kinghorn, Life Sci., 2005, 78, 431–441 CrossRef CAS PubMed.
- H. F. Ji, X. J. Li and H. Y. Zhang, EMBO Rep., 2009, 10, 194 CrossRef CAS PubMed.
- J. Milledge, B. Smith, P. Dyer and P. Harvey, Energies, 2014, 7, 7194–7222 CrossRef CAS.
- L. Roch, S. Prigent, H. Klose, C. Cakpo, B. Beauvoit, C. Deborde, L. Fouillen, P. van Delft, D. Jacob, B. Usadel, Z. Dai, M. Genard, G. Vercambre, S. Colombie, A. Moing and Y. Gibon, J. Exp. Bot., 2020, 71, 5823–5836 CrossRef CAS PubMed.
- L. Mannina, A. Sobolev and D. Capitani, Electrophoresis, 2012, 33, 2290–2313 CrossRef CAS PubMed.
- A. C. Abreu and I. Fernández, Molecules, 2020, 25, 3738 CrossRef CAS PubMed.
- Y. Monakhova, D. Rutledge, A. Rossmann, H. Waiblinger, M. Mahler, M. Ilse, T. Kuballa and D. Lachenmeier, J. Chemom., 2014, 28, 83–92 CrossRef CAS.
- J. Moura, C. Bonine, J. Viana, M. Dornelas and P. Mazzafera, J. Integr. Plant Biol., 2010, 52, 360–376 CrossRef CAS PubMed.
- M. Dick-Perez, T. Wang, A. Salazar, O. A. Zabotina and M. Hong, Magn. Reson. Chem., 2012, 50, 539–550 CrossRef CAS PubMed.
- M. Okamoto, Y. Tsuboi, E. Chikayama, J. Kikuchi and T. Hirayama, Plant Biotechnol., 2009, 26, 551–560 CrossRef CAS.
- C. Tian, E. Chikayama, Y. Tsuboi, T. Kuromori, K. Shinozaki, J. Kikuchi and T. Hirayama, J. Biol. Chem., 2007, 282, 18532–18541 CrossRef CAS PubMed.
- M. Dick-Perez, Y. Zhang, J. Hayes, A. Salazar, O. A. Zabotina and M. Hong, Biochemistry, 2011, 50, 989–1000 CrossRef CAS PubMed.
- Y. Sekiyama, E. Chikayama and J. Kikuchi, Anal. Chem., 2010, 82, 1643–1652 CrossRef CAS PubMed.
- T. Wang and M. Hong, J. Exp. Bot., 2016, 67, 503–514 CrossRef CAS PubMed.
- E. Song, H. Kim, J. Jeong, H. Chung, H. Kim, E. Bang and Y. Hong, J. Agric. Food Chem., 2016, 64, 3009–3016 CrossRef CAS PubMed.
- D. Augustijn, H. de Groot and A. Alia, Molecules, 2021, 26, 931 CrossRef CAS PubMed.
- Y. Ichihashi, Y. Date, A. Shino, T. Shimizu, A. Shibata, K. Kumaishi, F. Funahashi, K. Wakayama, K. Yamazaki, A. Umezawa, T. Sato, M. Kobayashi, M. Kamimura, M. Kusano, F. Che, M. O'Brien, K. Tanoi, M. Hayashi, R. Nakamura, K. Shirasu, J. Kikuchi and N. Nihei, Proc. Natl. Acad. Sci. U. S. A., 2020, 117, 14552–14560 CrossRef CAS PubMed.
- J. Mao, X. Cao, D. C. Olk, W. Chu and K. Schmidt-Rohr, Prog. Nucl. Magn. Reson. Spectrosc., 2017, 100, 17–51 CrossRef CAS PubMed.
- A. J. Simpson, M. J. Simpson, E. Smith and B. P. Kelleher, Environ. Sci. Technol., 2007, 41, 8070–8076 CrossRef CAS PubMed.
- A. J. Simpson, M. J. Simpson and R. Soong, Environ. Sci. Technol., 2012, 46, 11488–11496 CrossRef CAS PubMed.
- B. P. Kelleher and A. J. Simpson, Environ. Sci. Technol., 2006, 40, 4605–4611 CrossRef CAS PubMed.
- A. J. Simpson, G. X. Song, E. Smith, B. Lam, E. H. Novotny and M. H. B. Hayes, Environ. Sci. Technol., 2007, 41, 876–883 CrossRef CAS PubMed.
- J. D. Mao, B. Xing and K. Schmidt-Rohr, Environ. Sci. Technol., 2001, 35, 1928–1934 CrossRef CAS PubMed.
- J. D. Mao and K. Schmidt-Rohr, Environ. Sci. Technol., 2004, 38, 2680–2684 CrossRef CAS PubMed.
- J. D. Mao, L. S. Hundal, K. Schmidt-Rohr and M. L. Thompson, Environ. Sci. Technol., 2003, 37, 1751–1757 CrossRef CAS PubMed.
- J. D. Mao, R. L. Johnson, J. Lehmann, D. C. Olk, E. G. Neves, M. L. Thompson and K. Schmidt-Rohr, Environ. Sci. Technol., 2012, 46, 9571–9576 CrossRef CAS PubMed.
- C. Lattao, X. Cao, Y. Li, J. Mao, K. Schmidt-Rohr, M. A. Chappell, L. F. Miller, A. L. dela Cruz and J. J. Pignatello, Environ. Sci. Technol., 2012, 46, 12814–12822 CrossRef CAS PubMed.
- X. Cao, C. Lattao, J. J. Pignatello, J. Mao and K. Schmidt-Rohr, Environ. Sci. Technol., 2014, 48, 8645–8652 CrossRef CAS PubMed.
- C. Lattao, X. Cao, J. Mao, K. Schmidt-Rohr and J. J. Pignatello, Environ. Sci. Technol., 2014, 48, 4790–4798 CrossRef CAS PubMed.
- T. Ogura, Y. Date and J. Kikuchi, PLoS One, 2013, 8, e66919 CrossRef CAS PubMed.
- T. Ogura, Y. Date, Y. Tsuboi and J. Kikuchi, ACS Chem. Biol., 2015, 10, 1908–1915 CrossRef CAS PubMed.
- S. Rochfort, V. Ezernieks, P. Mele and M. Kitching, Magn. Reson. Chem., 2015, 53, 719–725 CrossRef CAS PubMed.
- Y. Date, F. Wei, Y. Tsuboi, K. Ito, K. Sakata and J. Kikuchi, BMC Chem., 2021, 15, 13 CrossRef CAS PubMed.
- S. Yamada, A. Kurotani, E. Chikayama and J. Kikuchi, Int. J. Mol. Sci., 2020, 21, 2978 CrossRef CAS PubMed.
- M. Mekuchi, K. Sakata, T. Yamaguchi, M. Koiso and J. Kikuchi, Sci. Rep., 2017, 7, 9372 CrossRef PubMed.
- S. Roques, C. Deborde, L. Guimas, Y. Marchand, N. Richard, D. Jacob, S. Skiba-Cassy, A. Moing and B. Fauconneau, Metabolites, 2020, 10, 83 CrossRef CAS PubMed.
- S. Roques, C. Deborde, N. Richard, L. Sergent, F. Kurz, S. Skiba-Cassy, B. Fauconneau and A. Moing, Metabolomics, 2018, 14, 155 CrossRef PubMed.
- M. Mekuchi, T. Asakura, K. Sakata, T. Yamaguchi, K. Teruya and J. Kikuchi, PLoS One, 2018, 13, e0197256 CrossRef PubMed.
- T. Watanabe, S. Nagai, Y. Kawakami, T. Asakura, J. Kikuchi, N. Inaba, Y. Taniuchi, H. Kurogi, S. Chow, T. Tomoda, D. Ambe and D. Hasegawa, Sci. Rep., 2021, 11, 5488 CrossRef CAS PubMed.
- R. Junge, B. Konig, M. Villarroel, T. Komives and M. H. Jijakli, Water, 2017, 9, 182 CrossRef.
- W. Kloas, R. Gross, D. Baganz, J. Graupner, H. Monsees, U. Schmidt, G. Staaks, J. Suhl, M. Tschirner, B. Wittstock, S. Wuertz, A. Zikova and B. Rennert, Aquac. Environ. Interact., 2015, 7, 179–192 CrossRef.
- S. Shi and G. Xu, Chem. Eng. J., 2019, 369, 694–704 CrossRef CAS.
- E. G. Alves Filho, L. M. Alexandre e Silva and A. G. Ferreira, Magn. Reson. Chem., 2015, 53, 648–657 CrossRef CAS PubMed.
- G. Muyzer, E. C. Dewaal and A. G. Uitterlinden, Appl. Environ. Microbiol., 1993, 59, 695–700 CrossRef CAS PubMed.
- S. Radajewski, P. Ineson, N. R. Parekh and J. C. Murrell, Nature, 2000, 403, 646–649 CrossRef CAS PubMed.
- Y. Date, Y. Nakanishi, S. Fukuda, T. Kato, S. Tsuneda, H. Ohno and J. Kikuchi, J. Biosci. Bioeng., 2010, 110, 87–93 CrossRef CAS PubMed.
- A. Yamazawa, T. Iikura, A. Shino, Y. Date and J. Kikuchi, Molecules, 2013, 18, 9021–9033 CrossRef CAS PubMed.
- A. Yamazawa, T. Iikura, Y. Morioka, A. Shino, Y. Ogata, Y. Date and J. Kikuchi, Metabolites, 2013, 4, 36–52 CrossRef PubMed.
- K. Kimura, N. Yamato, H. Yamamura and Y. Watanabe, Environ. Sci. Technol., 2005, 39, 6293–6299 CrossRef CAS PubMed.
- T. Miyoshi, T. Tsuyuhara, R. Ogyu, K. Kimura and Y. Watanabe, Water Res., 2009, 43, 5109–5118 CrossRef CAS PubMed.
- M. P. Herrling, J. Weisbrodt, C. M. Kirkland, N. H. Williamson, S. Lackner, S. L. Codd, J. D. Seymour, G. Guthausen and H. Horn, Biotechnol. Bioeng., 2017, 114, 2857–2867 CrossRef CAS PubMed.
- F. Ranzinger, M. Matern, M. Layer, G. Guthausen, M. Wagner, N. Derlon and H. Horn, Water Res.: X, 2020, 7, 100050 CAS.
- Z. A. Yi, L. P. Zhu, Y. Y. Xu, Y. F. Zhao, X. T. Ma and B. K. Zhu, J. Membr. Sci., 2010, 365, 25–33 CrossRef CAS.
- T. Komatsu, R. Ohishi, A. Shino and J. Kikuchi, Angew. Chem., Int. Ed., 2016, 55, 6000–6003 CrossRef CAS PubMed.
- T. Komatsu and J. Kikuchi, Anal. Chem., 2013, 85, 8857–8865 CrossRef CAS PubMed.
- N. Akiyoshi, A. Ihara, T. Matsumoto, A. Takebayashi, R. Hiroyama, J. Kikuchi, T. Demura and M. Ohtani, Plant Cell Physiol., 2021, pcab102 CrossRef PubMed.
- G. Tokuda, Y. Tsuboi, K. Kihara, S. Saitou, S. Moriya, N. Lo and J. Kikuchi, Proc. Biol. Sci., 2014, 281, 20140990 Search PubMed.
- Y. Hongoh, V. Sharma, T. Prakash, S. Noda, H. Toh, T. Taylor, T. Kudo, Y. Sakaki, A. Toyoda, M. Hattori and M. Ohkuma, Science, 2008, 322, 1108–1109 CrossRef CAS PubMed.
- H. Kim and J. Ralph, Org. Biomol. Chem., 2010, 8, 576–591 RSC.
- D. I. Schlipalius, N. Valmas, A. G. Tuck, R. Jagadeesan, L. Ma, R. Kaur, A. Goldinger, C. Anderson, J. Kuang, S. Zuryn, Y. S. Mau, Q. Cheng, P. J. Collins, M. K. Nayak, H. J. Schirra, M. A. Hilliard and P. R. Ebert, Science, 2012, 338, 807–810 CrossRef CAS PubMed.
- H. K. Kim, Y. H. Choi and R. Verpoorte, Nat. Protoc., 2010, 5, 536–549 CrossRef CAS PubMed.
- C. A. Sellick, R. Hansen, G. M. Stephens, R. Goodacre and A. J. Dickson, Nat. Protoc., 2011, 6, 1241–1249 CrossRef CAS PubMed.
- K. Bingol, F. Zhang, L. Bruschweiler-Li and R. Bruschweiler, Anal. Chem., 2013, 85, 6414–6420 CrossRef CAS PubMed.
- J. Kikuchi, K. Shinozaki and T. Hirayama, Plant Cell Physiol., 2004, 45, 1099–1104 CrossRef CAS PubMed.
- J. Kikuchi and T. Hirayama, Methods Mol. Biol., 2007, 358, 273–286 CrossRef CAS PubMed.
- Y. Nakanishi, S. Fukuda, E. Chikayama, Y. Kimura, H. Ohno and J. Kikuchi, J. Proteome Res., 2011, 10, 824–836 CrossRef CAS PubMed.
- Y. Sekiyama, E. Chikayama and J. Kikuchi, Anal. Chem., 2011, 83, 719–726 CrossRef CAS PubMed.
- G. X. Wang, D. Huang, J. H. Ji, C. Volker and F. R. Wurm, Adv. Sci., 2021, 8, 2001121 CrossRef CAS PubMed.
- S. B. Borrelle, J. Ringma, K. L. Law, C. C. Monnahan, L. Lebreton, A. McGivern, E. Murphy, J. Jambeck, G. H. Leonard, M. A. Hilleary, M. Eriksen, H. P. Possingham, H. De Frond, L. R. Gerber, B. Polidoro, A. Tahir, M. Bernard, N. Mallos, M. Barnes and C. M. Rochman, Science, 2020, 369, 1515–1518 CrossRef CAS PubMed.
- S. Kubowicz and A. M. Booth, Environ. Sci. Technol., 2017, 51, 12058–12060 CrossRef CAS PubMed.
- C. Rhodes, Sci. Prog., 2018, 101, 207–260 CrossRef PubMed.
- T. Amelia, W. Khalik, M. Ong, Y. Shao, H. Pan and K. Bhubalan, Prog. Earth Planet. Sci., 2021, 8, 12 CrossRef.
- A. K. Mohanty, S. Vivekanandhan, J. M. Pin and M. Misra, Science, 2018, 362, 536–542 CrossRef CAS PubMed.
- L. Filiciotto and G. Rothenberg, ChemSusChem, 2021, 14, 56–72 CrossRef CAS PubMed.
- T. Haider, C. Volker, J. Kramm, K. Landfester and F. Wurm, Angew. Chem., Int. Ed., 2019, 58, 50–62 CrossRef CAS PubMed.
- A. A. Shah, F. Hasan, A. Hameed and S. Ahmed, Biotechnol. Adv., 2008, 26, 246–265 CrossRef CAS PubMed.
- A. Chamas, H. Moon, J. Zheng, Y. Qiu, T. Tabassum, J. Jang, M. Abu-Omar, S. Scott and S. Suh, ACS Sustainable Chem. Eng., 2020, 8, 3494–3511 CrossRef CAS.
- Y. Zhu, C. Romain and C. K. Williams, Nature, 2016, 540, 354–362 CrossRef CAS PubMed.
- S. Inkinen, M. Hakkarainen, A. Albertsson and A. Sodergard, Biomacromolecules, 2011, 12, 523–532 CrossRef CAS PubMed.
- K. Schaler, A. Achilles, R. Barenwald, C. Hackel and K. Saalwachter, Macromolecules, 2013, 46, 7818–7825 CrossRef.
- M. Foston, Curr. Opin. Biotechnol., 2014, 27, 176–184 CrossRef CAS PubMed.
- T. Komatsu and J. Kikuchi, J. Phys. Chem. Lett., 2013, 4, 2279–2283 CrossRef CAS.
- J. Schlagnitweit, M. Tang, M. Baias, S. Richardson, S. Schantz and L. Emsley, J. Magn. Reson., 2015, 261, 43–48 CrossRef CAS PubMed.
- G. Chen, Z. Shen, A. Iyer, U. Ghumman, S. Tang, J. Bi, W. Chen and Y. Li, Polymers, 2020, 12, 163 CrossRef CAS PubMed.
- L. Himanen, A. Geurts, A. Foster and P. Rinke, Adv. Sci., 2020, 7, 1900808 Search PubMed.
- J. Kumar, Q. Li, K. Tang, T. Buonassisi, A. Gonzalez-Oyarce and J. Ye, npj Comput. Mater., 2019, 5, 73 CrossRef.
- R. P. Verma and C. Hansch, Chem. Rev., 2011, 111, 2865–2899 CrossRef CAS PubMed.
- R. Yamawaki, A. Tei, K. Ito and J. Kikuchi, Appl. Sci., 2021, 11, 2820 CrossRef CAS.
- G. Barone, D. Ferizovic, A. Biundo and P. Lindblad, Sustainability, 2020, 12, 10449 CrossRef CAS.
- Y. Yang, J. Yang, W. Wu, J. Zhao, Y. Song, L. Gao, R. Yang and L. Jiang, Environ. Sci. Technol., 2015, 49, 12080–12086 CrossRef CAS PubMed.
- E. Castro-Aguirre, F. Iniguez-Franco, H. Samsudin, X. Fang and R. Auras, Adv. Drug Delivery Rev., 2016, 107, 333–366 CrossRef CAS PubMed.
- A. Paco, K. Duarte, J. da Costa, P. Santos, R. Pereira, M. Pereira, A. Freitas, A. Duarte and T. Rocha-Santos, Sci. Total Environ., 2017, 586, 10–15 CrossRef CAS PubMed.
- J. Kikuchi, K. Ito and Y. Date, Prog. Nucl. Magn. Reson. Spectrosc., 2018, 104, 56–88 CrossRef CAS PubMed.
|
This journal is © The Royal Society of Chemistry 2021 |
Click here to see how this site uses Cookies. View our privacy policy here.