Connecting environmental exposure and neurodegeneration using cheminformatics and high resolution mass spectrometry: potential and challenges†
Received
12th February 2019
, Accepted 2nd July 2019
First published on 5th July 2019
Abstract
Connecting chemical exposures over a lifetime to complex chronic diseases with multifactorial causes such as neurodegenerative diseases is an immense challenge requiring a long-term, interdisciplinary approach. Rapid developments in analytical and data technologies, such as non-target high resolution mass spectrometry (NT-HR-MS), have opened up new possibilities to accomplish this, inconceivable 20 years ago. While NT-HR-MS is being applied to increasingly complex research questions, there are still many unidentified chemicals and uncertainties in linking exposures to human health outcomes and environmental impacts. In this perspective, we explore the possibilities and challenges involved in using cheminformatics and NT-HR-MS to answer complex questions that cross many scientific disciplines, taking the identification of potential (small molecule) neurotoxicants in environmental or biological matrices as a case study. We explore capturing literature knowledge and patient exposure information in a form amenable to high-throughput data mining, and the related cheminformatic challenges. We then briefly cover which sample matrices are available, which method(s) could potentially be used to detect these chemicals in various matrices and what remains beyond the reach of NT-HR-MS. We touch on the potential for biological validation systems to contribute to mechanistic understanding of observations and explore which sampling and data archiving strategies may be required to form an accurate, sustained picture of small molecule signatures on extensive cohorts of patients with chronic neurodegenerative disorders. Finally, we reflect on how NT-HR-MS can support unravelling the contribution of the environment to complex diseases.
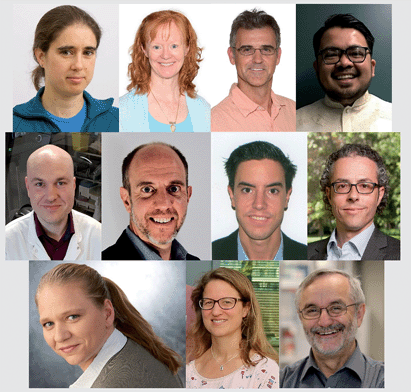 Top: E. L. Schymanski, N. C. Baker, A. J. Williams, R. R. Singh Middle: J.-P. Trezzi, P. Wilmes, P. L. Kolber, R. Krüger Bottom: N. Paczia, C. L. Linster, R. Balling | Associate Professor Emma Schymanski is a Luxembourg National Research Fund (FNR) ATTRACT Fellow and head of the Environmental Cheminformatics group at the Luxembourg Centre for Systems Biomedicine (LSCB), University of Luxembourg. Dr Randolph Singh is a postdoctoral fellow in the same group. Dr Nancy Baker is a consultant at Leidos, Research Triangle Park, USA. Dr Antony Williams is a Computational Chemist, National Center of Computational Toxicology, US EPA. Dr Jean-Pierre Trezzi is postdoctoral fellow affiliated with the Integrated Biobank of Luxembourg, Luxembourg Institute of Health as well as the Eco-Systems Biology group at LCSB, which is headed by Associate Professor Paul Wilmes. Dr med. Pierre Kolber, MD is a member of Neurology department at the Centre Hospitalier de Luxembourg and the Clinical and Experimental Neuroscience Group at LCSB, which is headed by Professor Dr med. Rejko Krüger, also an FNR PEARL Fellow and Director of Transversal Translational Medicine at the Luxembourg Institute of Health. Dr Nicole Paczia, former joint-manager of the Metabolomics Platform at LCSB, now heads the Metabolomics Core facility at the Max Planck Institute in Marburg. Dr Carole Linster is head of the Enzymology and Metabolism Group, LCSB. Professor Rudi Balling is Director of the LCSB. |
Environmental significance
Non-target high resolution mass spectrometry has attracted immense interest regarding the potential for increased characterisation of chemicals and exposures in environmental studies. However, high quality studies remain difficult to perform and often yield fewer successful identifications than desired. We present our multi-disciplinary perspective on tackling potential causes for this discrepancy and the complexity involved in capturing the knowledge needed to investigate the impact of chemicals on human health in the context of long-term diseases. We look at how to mine patient and expert knowledge to find, measure and validate potential neurotoxicants and explore the potential for NT-HR-MS to provide small molecule data signatures in cohorts and build the knowledge required for greater future understanding of environmental impacts on complex diseases.
|
Introduction
Rapid developments in analytical techniques over the last decades have opened up new opportunities such as non-target high resolution mass spectrometry (NT-HR-MS) to explore the impact of humans on the environment,1 as well as the effect of environmental contamination on humans2,3 and other organisms.4,5 With growing accessibility and maturity in NT-HR-MS analytical methods, these are being applied to increasingly complex research questions and hypotheses.6–8 In this perspective article, we take a close look at the challenges in identifying “neurotoxicants” in the context of chronic neurodegenerative diseases and use this case study to explore the potential and limitations of NT-HR-MS to support answering these questions. Ultimately, solving complex challenges such as these will need a systems approach,9,10 requiring knowledge that crosses many disciplines. While the main focus of this article, determining which (relevant) chemicals are present in environmental or biological samples (let alone their mode of action), is already a daunting task, it is a necessary foundation to enable the elucidation of functional effects and generation of hypotheses for a greater understanding of the causes of complex diseases and environmental impacts.
NT-HR-MS, typically coupled with separation techniques such as liquid or gas chromatography, is becoming an increasingly popular approach for the broad screening of complex environmental samples.1 NT-HR-MS can be performed on low sample amounts and reach ppb (or lower) detection limits in many cases, with data acquisition now often able to capture both known and unknown chemicals in a single measurement.11 Ideally in NT-HR-MS, post-acquisition data processing is used to identify (1) the known chemicals, i.e. the “targets” or also “biomarkers” in the context of metabolomics and disease; (2) potential chemicals of interest based on prior knowledge, i.e. lists of chemicals (“suspects”) and (3) the relevant “unknowns” or “non-targets” using some form of prioritisation.1 Data generated in NT-HR-MS can be archived for later use and exploration (also termed retrospective screening).12 While NT-HR-MS has been declared “ready to go”,1 in this article we explore how to gather the knowledge required to take NT-HR-MS to the next level of helping unravel the cause and mechanisms of environmental exposure in the context of late-developing complex diseases, where a lifetime of exposure to thousands of chemicals may potentially impact disease progression. Forming epidemiological connections, however, does not just involve the detection of chemicals of interest, but also gathering information on qualitative aspects and characteristics of potential neurotoxicants, as well as time and duration of exposure.
To date, NT-HR-MS of environmental samples has been commonly directed by substance classes. Several examples include using suspect screening approaches to investigate classes such as pharmaceuticals,13,14 pesticides15,16 or antibiotics.17,18 Further studies are now using publicly-available suspect lists in their screening efforts.19,20 Other early efforts include comprehensive, exploratory characterisation efforts of wastewater that went beyond compound classes.21,22 As NT-HR-MS matures, effect-directed analysis (EDA) studies are beginning to show some level of success with established bioassay endpoints such as mutagenicity.23 The growing popularity of “big data” techniques has seen the expansion into more data exploration-based methods such as virtual EDA, clustering, trend analysis and fingerprinting, summarized elsewhere,1 inspired partially by metabolomics efforts. From a metabolomics perspective, great investments have been made into the detection of biomarkers for certain diseases (discussed further below) and the trend is now towards using these approaches for a more mechanistic understanding of the biology behind the biomarkers,24 primarily using targeted analysis techniques. Yet the conundrum remains: there are orders of magnitude more “unknowns” than “knowns” in both biological and environmental samples,25 despite compound databases now containing over 100 million chemicals and impressive improvements to computational mass spectrometry workflows.26 Additionally, despite greater identification efforts, toxic effects observed in the environment (e.g. in EDA studies) also remain unexplained more often than not,27 indicating that a greater understanding of the entire environmental system is still required.
Currently, the key to successful NT-HR-MS is finding “known unknown” chemicals13 (chemicals documented to exist but unknown upfront to the investigator) of high relevance to the study question in an efficient manner (i.e. via prioritisation).1 The full identification of unknowns is still extremely time consuming and, while it is easy nowadays to get tentative candidates for many detected masses in NT-HR-MS,28,29 candidate selection and validation remains challenging and suspect list/compound database choice has a dramatic influence in the outcome of identification efforts.30 A well-designed suspect screening approach, i.e. searching for a discrete list of chemicals potentially relevant to the study question, is an ideal way to find masses and thus candidates of particular interest quickly. However, there is a delicate balance in suspect screening. Small, carefully validated lists containing tens to a few hundred entries result in few suspect hits ever being found, but if found, are likely highly relevant.15 On the other hand, suspect screening using large databases (containing tens of thousands to millions of entries) for all matching candidates rapidly turns into a non-target identification challenge with multiple matching candidates per mass.28 The current trend in NT-HR-MS is towards compiling very large lists (in the order of tens of thousands of entries) to enable better coverage which is, in effect, closing the gap between suspect screening and typical non-target or unknown identification approaches. A variety of statistical approaches, such as replicates and multiple correction testing, can be used to recognise and reduce the resulting false positives, summarized elsewhere.1 As validation of any exact mass hit (irrespective of the size of the suspect list) in NT-HR-MS is essential,31 additional information must also be used to support the candidate structure. This includes orthogonal analytical evidence (e.g. chromatographic retention behaviour, fragmentation information) as well as so-called metadata, i.e. additional information that may indicate that this chemical is relevant to the study question.7,32 For many years, literature references have been used to prioritise highly interesting candidates33 and these have now been built into many identification approaches.30,34–36 In an environmental context, specialised resources such as the CompTox Chemicals Dashboard,37 the Human Metabolome Database38 (and related resources such as DrugBank39 and T3DB40), and datasets on the NORMAN Suspect List Exchange41,42 offer metadata categories relevant for environmental screening, such as exposure data, activity in in vitro screening bioassays, predictive toxicity values (quantitative structure activity relationships, QSARs), literature counts and occurrence in various matrices of interest. Connecting the metadata associated with mixtures to their individual constituents via the so-called “MS-ready” form now allows screening of mixtures in HR-MS.43,44
While connecting masses detected in environmental samples to “known unknowns” and related metadata is now achievable with NT-HR-MS, connecting chemical exposure to diseases opens up many more challenges. The concept of the “exposome” was first introduced in 2005 by Wild45 with the statement “at its most complete, the exposome encompasses life-course environmental exposures (including lifestyle factors), from the prenatal period onwards”.45 This definition comes with numerous challenges, not least the time frame of the life-course, which are not the focus of this current article. Other articles, including (but not limited to) those cited here, contain further discussions and refinements to the concept.46,47 The “meet-in-the-middle” approach has been introduced “to address the challenge of identifying causal relationships that link exposures and disease outcomes”,48 indicating the need for the connection of exposomics to other “omics” levels such as epigenomics, metabolomics and transcriptomics.48 While biomarkers have resulted in many impressive epidemiological studies, the vast majority of these are based on targeted studies of well-known pollutants; the field is at the cusp of being able to take full advantage of NT-HR-MS and these are the views we wish to present in this perspective.
In the following sections, we explore how to capture more topic-specific metadata and maintain links back to the associated literature and patient knowledge using the chosen case study of neurotoxicants in the context of chronic neurodegenerative diseases. We will then look at what analytical approaches may be needed to capture broad concepts such as “potential neurotoxicants” and explore what may be missing and remain unseen, before moving onto the challenges associated with relating this information back to the study question (e.g. confirming whether potential candidates are responsible for neurotoxicity and the final disease state). Finally, we reflect on how advances in analytical and data technologies could be leveraged and built into long term cohort studies to build a greater understanding of the environmental influences on complex disease states.
Cheminformatics to capture the chemical space of “neurotoxicants”
As mentioned above, the key to successful NT-HR-MS is finding chemicals of high relevance to the study question in an efficient manner (prioritisation). A well-designed suspect screening, i.e. searching for a discrete list of chemicals relevant to the study question, is an ideal way to quickly find masses (features) and thus candidates of particular interest. A number of lists of neurotoxicants were compiled for the purposes of this perspective, summarized in Table 1 and described further in the ESI.† These include small, carefully validated lists explained extensively in the source publications (DNTEFFECTS49 (https://comptox.epa.gov/dashboard/chemical_lists/dnteffects), DNTINVIVO50 (https://comptox.epa.gov/dashboard/chemical_lists/dntinvivo) and HUMANNEUROTOX51 (https://comptox.epa.gov/dashboard/chemical_lists/humanneurotox)), where few suspect hits will ever be found in environmental samples. The DNTPOTNEG50 (https://comptox.epa.gov/dashboard/chemical_lists/dntpotneg) list contains potential negative controls for neurotoxicity which, if found, should not be associated with neurotoxic effects, enabling a second layer of data quality verification of neurotoxicant findings. Two larger lists (NEUROTOXINS52 (https://comptox.epa.gov/dashboard/chemical_lists/neurotoxins) and LITMINEDNEURO53,54 (https://comptox.epa.gov/dashboard/chemical_lists/litminedneuro)) have also been compiled for this perspective. The NEUROTOXINS list was compiled from public resources including ChEBI,55,56 Wikipedia,57,58 T3DB,40 with further details given in the ESI.†
Table 1 A range of lists for performing suspect screening of neurotoxicants, compiled for this perspective. These lists are also available as a single collection on Zenodo62
List code |
Entries and references |
Description |
DNTEFFECTS (https://comptox.epa.gov/dashboard/chemical_lists/dnteffects) |
96 (ref. 49 and 63) |
Chemicals with data demonstrating effects on neurodevelopment |
DNTINVIVO (https://comptox.epa.gov/dashboard/chemical_lists/dntinvivo) |
33 (ref. 50 and 64) |
A (non-exhaustive) list of compounds documented to trigger developmental neurotoxicity (DNT) in at least two different laboratories |
DNTPOTNEG (https://comptox.epa.gov/dashboard/chemical_lists/dntpotneg) |
41 (ref. 50 and 65) |
Suggested potential negative controls for developmental neurotoxicity (DNT) assays. Statins can also be used, see https://comptox.epa.gov/dashboard/chemical_lists/statins |
HUMANNEUROTOX (https://comptox.epa.gov/dashboard/chemical_lists/humanneurotox) |
190 (ref. 51, 66 and 67) |
A set of chemicals identified as potential neurotoxicants by the authors using literature searching, not necessarily active neurotoxicants |
NEUROTOXINS (https://comptox.epa.gov/dashboard/chemical_lists/neurotoxins) |
511 (ref. 52, 62 and 68) |
A list of chemicals reported as neurotoxicants, compiled from public resources (source file with details on Zenodo62) |
LITMINEDNEURO (https://comptox.epa.gov/dashboard/chemical_lists/litminedneuro) |
1243 (ref. 53, 54 and 69) |
Chemicals associated with neurotoxicity compiled through automated literature mining of PubMed using MeSH terms (node C10, subheadings “chemically induced”, “toxicity”, “poisoning”, or “adverse effects”) and associating these with single chemical substances |
LITMINEDNEURO contains chemicals associated with neurotoxicity compiled through systematic literature mining of PubMed59 using Medical Subject Heading (MeSH) terms60 and associating these with single chemical substances (where possible) using previously published methods.61 Articles were identified in which a nervous system disease was annotated with the MeSH node C10 through the MeSH tree with disease subheading “chemically induced” and subheading “toxicity”, “poisoning”, or “adverse effects”. Nerve diseases caused by trauma and manually identified “common English terms” that could not be associated with any specific chemicals (e.g. “particulate matter”, “contrast media”) were omitted. In total 4528 chemicals were identified; all chemicals with 5 or more literature references were registered in the Dashboard and included in the final list. The output of this processing was exported to Microsoft® Excel and is included as ESI† and available on FigShare.53 The CASRN and the CompTox Chemicals Dashboard substance identifier (DTXSID) were included in the spreadsheet for chemicals for which this relationship was captured and associated with MeSH identifiers. The overview tab of this workbook contains 1250 chemicals (1243 unique DTXSIDs) and the co-annotations with 554 nervous system diseases in over 53
000 chemical-disease pairs (“Detail” tab). These relationships were described in 38
192 articles. A batch search of the Dashboard by DTXSID will return all related chemical information needed for generating suspect lists with subject-specific reference scores for disease or effect subsets of this list.
Automated text-mining techniques such as those described here have the advantage of being easy to run. Since they encompass the large and fast-growing PubMed corpus (28 million citations as of October 2018), the approach can identify chemicals that may cause disease and which may not have yet achieved visibility through other means. On the other hand, data extracted through automated text-mining has also not passed through rigorous manual vetting and is likely to contain various types of errors. For instance, some articles discuss more than one chemical and more than one disease, while the algorithms that associate each disease with each chemical may not construct valid pairs. Typing errors and ambiguous synonyms in the original literature may confuse proper mapping of the chemical identities. Additionally, the MeSH annotations do not capture negative results (a chemical not causing a particular neurotoxic effect) and therefore it can be difficult to disambiguate positive from negative reports. For instance, caffeine (https://comptox.epa.gov/dashboard/dsstoxdb/results?search=DTXSID0020232&abbreviation=DNTEFFECTS) is documented with data demonstrating effects on neurodevelopment, yet potentially neuroprotective in the context of Parkinson's disease (PD);70–72 this can be traced via the Excel macro provided in the ESI† in the entry for caffeine. Likewise nicotine is documented in all the neurotoxicant lists in Table 1 (except the negative control list DNTPOTNEG (https://comptox.epa.gov/dashboard/chemical_lists/dntpotneg)), yet smoking may be protective in some cases in the context of Parkinson's disease. For example, non-smoking carriers of the LRRK2 Gly2385Arg gene have increased risk of developing PD;73 while another study (published in German) discusses the balance between neurotoxic and neuroprotective effects of nicotine and smoking.74 Understanding of the context is critical and the interface through the Excel file provided as ESI† is one method to follow-up the literature references for specific chemicals and understand the relevance of the suspect for their particular study question (see examples for nicotine and caffeine above).
Related structures: mapping “chemicals” and “substances”
Reviewing some of the lists in Table 1via the Dashboard reveals several interesting cases where new cheminformatics approaches are needed and already under development to capture less well-defined substance information in the form of discrete chemical structures suitable for NT-HR-MS screening studies. Several examples are given in Fig. 1 and more extensive details given in the ESI.†
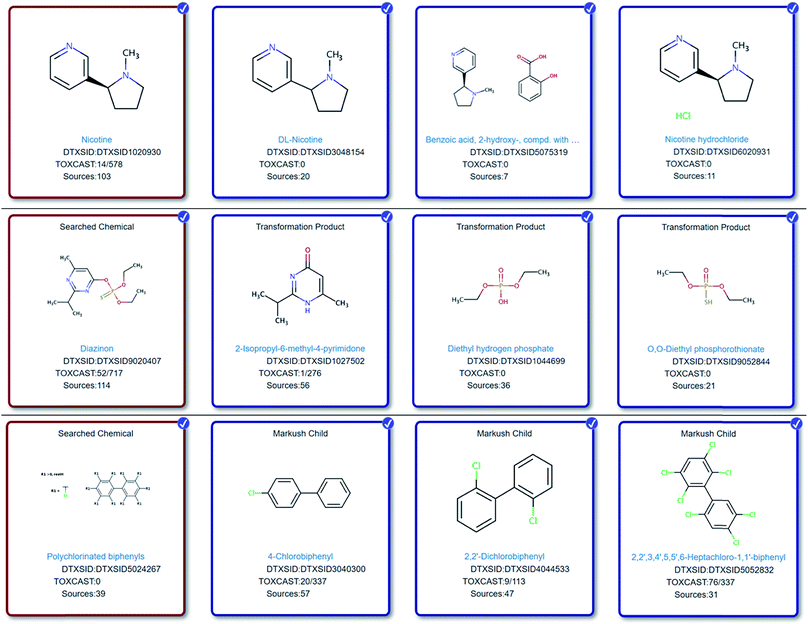 |
| Fig. 1 Top: Nicotine (red box) (https://comptox.epa.gov/dashboard/dsstoxdb/mixture_search?cid=930) and selected mixtures, salts and components. Middle: Diazinon (red box) (https://comptox.epa.gov/dashboard/dsstoxdb/results?search=DTXSID9020407&abbreviation=HUMANNEUROTOX#related-substances) and selected mapped transformation products. Bottom row: The PCB class (red box) (https://comptox.epa.gov/dashboard/dsstoxdb/results?search=PCBs#related-substances) and selected mapped individual PCB compounds. While the generic PCB representation is suboptimal visually, it allows a full, automated enumeration of all members of the PCB class via the ChemAxon Markush Enumeration module to create “Markush child” entries (see label) – an important consideration for upscaling these cheminformatics efforts. All chemicals display the structure, DTXSID, number of assays (active/total) in TOXCAST75 (https://comptox.epa.gov/dashboard/chemical_lists/toxcast) and the data sources. | |
One of the most interesting challenges associated with capturing chemical information for NT-HR-MS related to neurotoxicity (or any potential health impact) is relating what we as humans consume relative to discrete chemical components. For instance, while toxicity testing is most commonly performed on a chemical such as caffeine, patients will instead report coffee (or tea or energy drink) consumption. Coffee, for instance, is documented to contain over 1000 chemical constituents,76 which require a variety of analytical techniques for detection.77 Nicotine is another common example where the chemical tested is often nicotine (or an associated salt or mixture), but patients will instead report e.g. smoking habits. It is well known that nicotine is not the only chemical in cigarettes that may cause detrimental health effects. Several thousand chemicals have been identified in cigarettes78,79 with 599 chemicals listed as additives.80 Capturing such knowledge (e.g. via cross-mapping and adding as lists or related substances in databases) will be increasingly important to help reconcile NT-HR-MS results in the future, yet expand suspect lists even further. The connection of individual chemical structures to mixtures43 and the associated metadata can enable high throughput screening of NT-HR-MS data, including e.g. toxicity and product information,81 which are often associated with mixtures. It also enables better data interpretation downstream. Furthermore, the collection of chemicals into lists combined with well-selected metadata can ensure rapid prioritization by score and can help rapidly pinpoint highly promising candidates amongst hundreds to thousands of possible masses and chemical structures, as demonstrated in Fig. 2. More specific details about how such information can be included in NT-HR-MS studies are given in the ESI.†
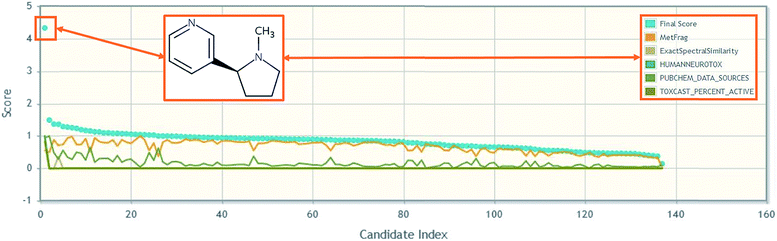 |
| Fig. 2 An example of how metadata can help candidate selection in high throughput NT-HR-MS studies, using nicotine in MetFrag.82 Further details in the ESI.† | |
Capturing patient and medical knowledge
While the preceding sections discuss capturing and exploring the documented chemical and medical knowledge in the context of neurotoxicity and using this during identification in NT-HR-MS, capturing the patient knowledge is an incredibly important part of connecting chemicals to disease. For instance, neurodegeneration in chronic diseases such as Parkinson's disease takes place for many years before the first motor manifestations appear that define the clinical diagnosis of this movement disorder.83 The time span between the diagnosis of PD and the appearance of the initial non-motor symptoms such as depression, REM sleep behavior disorder, hyposmia or chronic constipation (defining the prodromal phase of PD), can range from approximately 5 up to 30 years. Even before this prodromal phase of the disease, neuronal dysfunction and neuronal cell death are already ongoing and might precede the prodromal phase by many years or even decades.84 Thus, in order to analyze the potential neurotoxicants and environmental factors that might lead to neurodegenerative diseases, one has to retrace the exposure of patients long before the diagnosis of the disease, better still before the first neuronal dysfunctions appear. Furthermore, as discussed below, there may no longer be any traces of the chemical to which the patient was exposed in cohort samples and thus the patient's memory of their chemical exposures may be the only documentation that could provide clues to potential causative agents. In the few longitudinal PD patient cohorts that exist, such as the Luxembourg Parkinson's study,85 the first step in analyzing the environmental risk factors leading to neurodegeneration is by using detailed validated patient questionnaires retrospectively trying to trace the patient's professional or leisure activities throughout their entire life (see Fig. 3 for a screenshot from the REDCap86 system (https://www.project-redcap.org/)).
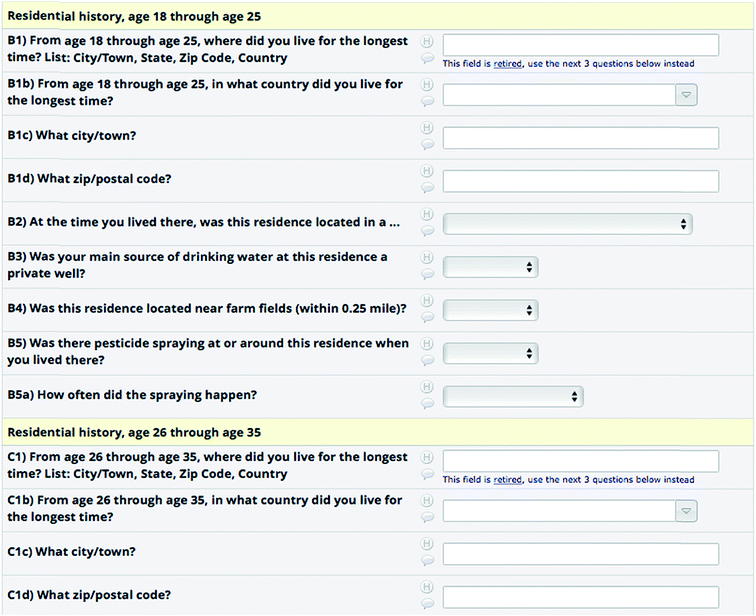 |
| Fig. 3 Screenshot of the “Tanner survey” used to capture patient information, using REDCap (https://www.project-redcap.org/).86,87 | |
These classical epidemiological strategies apply self-reporting questionnaires that are not without flaws, as there is a non-negligible recall bias, especially considering the fact that patients with neurodegenerative diseases are mainly elderly people and to some degree affected by more or less pronounced cognitive defects. Additional confusion can occur due to misunderstanding of the terminology, for instance weed killers approved for household use are not always understood as a herbicide by patients and thus pesticide/herbicide exposure might be neglected by patients in some questionnaires. Nevertheless, these questionnaires can provide first hints towards potential environmental risk factors using the knowledge of the patients themselves. Anecdotal cases such as the one mentioned above are highly subjective and difficult to avoid with a generic survey form as in Fig. 3. However, the association of chemicals with products (e.g. via synonym linking such as aspirin/acetylsalicylic acid (https://comptox.epa.gov/dashboard/dsstoxdb/results?search=aspirin), plus ingredients listing such as the cigarette example above) and products with exposure scores, such as CPDat,81 may help connect what patients report and the information required for chemical analysis. Obtaining chemical and approved product information from authorities and linking this to e.g. agricultural land use can support epidemiological studies87,88 as well as ecological assessments.89 Product information from authorities, while often kept confidential, is essential for improved connections between NT-HR-MS and epidemiology. As such, greater efforts to share this information in the public domain are desperately needed and several authorities are now supportive of information sharing – as evidenced for example by the many lists now available on the CompTox Chemicals Dashboard90 and the NORMAN Suspect Exchange.41,42
Using current knowledge, one can hypothesise that neurotoxicant exposure might precede neurodegenerative diseases such as PD by many decades, potentially during young adulthood or childhood (or even in utero), launching a cascade of malfunctions progressively leading to apoptosis of dopaminergic neurons. This makes finding a representative sample from patients very challenging, as ideally the sample used should contain the neurotoxicants from that initial exposure period, which will also potentially transform over time. See discussion in the next section. Considering PD specifically, the risk for PD due to the usage of hydrocarbon-based compounds such as pesticides, herbicides or fungicides is quite established by now, especially professional pesticide usage.91,92 However, metal-based compounds are also suspected to contribute to an increased risk for PD or atypical parkinsonian syndromes (aPS), such as progressive supranuclear palsy (PSP) – a rapidly progressive aPS with prominent falls and gaze palsy as major clinical hallmarks. Some studies hypothesise a role of toxic metals and metalloids (such as arsenic) in the pathogenesis of PSP.93 Exposure to heavy metals (such as lead) is suggested to be associated with an increased risk for PD. However, difficulties in making differential diagnoses between these disorders can confound these conclusions. A further factor to consider is epigenetics, where epigenetic imprints may reflect exposure in the preceding generation and could add some prior knowledge with respect to the effects observed, if not the actual chemical exposure itself.94 Thus, while surveys can help capture patient knowledge, in the days of “big data” and with the trend towards personalised medicine, it is important to consider how “on the spot” analysis and digital archiving of small molecule data during cohort sample collection may enable a long term generation of a baseline dataset for greater understanding of disease progression and diagnosis in the future.
Available sample matrices
When studying disease-related neurotoxicant exposure, human-derived samples are of primary interest as these depict the pathological changes and can be monitored over time. However, not all of these matrices can be obtained easily and depending on the matrix used, the results can vary significantly. For instance, traces of many compounds are very short-lived in complex matrices like blood, as they are quickly metabolised and excreted by the kidneys. If one analyzes the urine, the window of detection can be delayed (the time for the compound to be filtered out from the blood by the kidneys), but is equally short-lived in the context of long-term diseases. The individual's kidney function, hydration status and metabolism complicate this aspect, as the amount of urine and the frequency of the mictions vary hugely between each individual patient. Not only does the time window of detection vary, but also the concentration of the compound detected in the matrix can vary hugely from one time period to another and if the peak of concentration is missed, the results can become insignificant. Additionally, if one analyzes, for example, the total 24 h diuresis in an attempt not to miss the detection window, the compound can become so diluted that it can falsely render the exposure as insignificant. Furthermore, for those substances where the metabolites are not known or documented, the analyst will have to use additional experiments and data processing approaches to identify potentially relevant metabolites. An impressive recent effort shows the possibility for NT-HR-MS and advanced data processing to provide much broader coverage than the typical targeted urine analysis.95 Yet another very recent effort reports initial developments towards non-invasive drug monitoring via NT-HR-MS analysis of skin.96 However, the transient nature of some compounds and matrices has to be kept in mind while analysing potential neurotoxicants in human samples. Samples containing the compounds for a longer period of time seem to be, in this regard, more valuable. Bone is an interesting matrix for metals exposure and, for example, lead concentration can be measured with 109Cd-excited K-shell X-ray fluorescence, representing a biomarker of cumulative lead exposure.97 Teeth have also been used to estimate long-term exposure to metals and selected organic compounds, with especially recent studies drawing conclusions for various aspects of neurotoxicity.98–101 Hair sampling is a relatively non-invasive longer-term sample, due to the continual release of fat-soluble contaminants that have been proven to provide biomarkers for monitoring human exposures to environmental chemicals such as pesticides and metals.102 In addition, information obtained from hair analysis is more representative of an individual's level of exposure than other matrices such as urine and blood.103 As many (easily available) biological matrices are so transient, linking chemical exposure to affected pathways with e.g. metabolomics approaches will be increasingly relevant.2,3 As recent research has indicated a potential connection between the gut microbiome and disease progression (see text below), there is also increasing interest in faecal samples and the biological, as well as chemical, information contained within.
Apart from human-derived samples, environmental samples can also be studied for the presence of neurotoxicants as surrogates for human samples, knowing that biological matrices can be challenging to access. Wastewater samples, for example, may reveal group exposure to specific contaminants as this matrix is a collection from a population and presents an idea of what the bulk population of a specific area is currently (or was) exposed to.11 As wastewater is released back into the environment after treatment, studying local fish, crustacea or even resting eggs104 may show which specific neurotoxicants have been present over a longer time frame and have bioaccumulative potential. Sediments and soil cores, on the other hand, may give an idea of historical exposure that may have happened over the last few years or decades,105 although degradation and transformation must be taken into account. Even groundwater transects can provide interesting historical trends.7 Such samples will generally reveal only population trends. Dust samples are typically more representative of individual indoor exposure, with many targeted studies now showing health-related conclusions.106 Increasing numbers of non-target studies are being published, including several collaborative and ring trials,29,107 showing this to be a matrix of great interest for future efforts, although high quality data processing is still challenging for routine use.
The quality of the samples of interest, and thus downstream chemical and data analysis, is highly impacted by pre-analytical variations. Ideally, according to biobank protocols, tissue samples should be snap-frozen in liquid nitrogen immediately after sampling. However, for logistical reasons, it is often not feasible to respect these recommendations. Thereby, it is crucial to monitor the pre-analytical variations that can occur after sampling, such as the time between the sampling and the processing of the sample, temperature, pH among others. Especially for biomarker discovery/validation studies, it is crucial to monitor these changes. In this regard, biobanks have established a standard pre-analytical coding for biospecimens to enable standardised documentation of the sample collection and processing to limit pre-analytical variations.108 This becomes crucial in multi-center collection studies. In general, biobanks have stringent protocols for sample preservation and storage, together with strict quality controls. Biobanks typically store biospecimens derived from human individuals, such as blood derivatives (plasma, serum), cerebrospinal fluid, urine, saliva, stool and cells,108 and are all potential matrices for neurotoxicant analyses depending on the study interest. Together with the samples, metadata on medical variables, dietary information, as well as environmental data are collected and made available for research. In terms of creating a digital “snapshot” of small molecule conditions, analysis as close to time of receipt will be a distinct advantage to prevent sample degradation, with the downside of creating large variabilities and batch effects in downstream data analysis. However, with sufficient quality controls and strategies to best capture batch effects to allow correction (standard measurements, internal standards, pooled samples), sophisticated data processing techniques are now available to perform such corrections.
Analysing the chemical space of neurotoxicants
Chemical properties and analytical methods
Not all analytical methods are equally efficient and, as evidenced by the variety in the physico-chemical properties of potential neurotoxicants (see Fig. 4), it is not realistic to expect a “one size fits all” analytical method to perform a full screen of all potential neurotoxicants in a given matrix. A detailed description of the list and the corresponding diversity in physico-chemical properties can be found in the ESI.† Already the choice of solvents during extraction will have an impact on the chemical nature of the analytes to be found in the data acquisition. Multiple extractions as well as measurement protocols have to be applied to enable a comprehensive picture of the possible neurotoxicant composition of the matrix of interest, especially in NT-HR-MS. To create long term records, a minimum of analytical measurements capturing the maximum amount of information possible within the compromise of time, cost and effort will be needed to provide enough information to direct future, more sophisticated analysis on the frozen and archived (physically and digitally) samples. In the next section we explore some of the analytical options available to cover this extraordinarily broad range of contaminants within the category “potential neurotoxicants”. As there are many excellent reviews on analysis,11 including one specifically on PD,109 the text below covers major analytical aspects and decisions that we consider most likely to affect the quality of NT-HR-MS data processing that can be performed, while the ESI† contains some additional discussion.
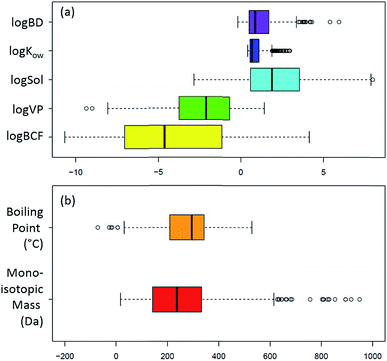 |
| Fig. 4 Box plots showing the wide range of physicochemical properties of the NEUROTOXINS list (https://comptox.epa.gov/dashboard/chemical_lists/neurotoxins),52 according to the OPERA predictions57 and intrinsic properties from the Dashboard. (a) log values of the biodegradation constant (log BD), octanol–water coefficient (log Kow), water solubility in mol L−1 (log Sol), vapour pressure in mm Hg (log VP) and bioconcentration factor (log BCF) plus (b) boiling point (°C) and monoisotopic mass (Da). The monoisotopic mass was cut-off at 1000 Da for display purposes; 11 entries had a mass between 1000 and 5040 Da. | |
When performing potentially long term studies of complex samples, careful sample pre-processing steps are required for the analysis, such as the removal of highly abundant matrix components such as lipids and/or pigments that could interfere with the analysis. However, the use of any sample clean-up technique, as well as the choice of the extraction method, carries the risk of eliminating the actual compounds of interest. Furthermore, careful but simple sample preparation and pre-treatment is required that will enable minimum interferences and appropriate normalisation of samples for data processing and subsequent identification efforts over years of data collection. This is especially challenging for unknowns, as the usual techniques applied to targeted compounds (internal standard, recovery and matrix correction) cannot be applied; furthermore as the structure is not known it is challenging to select closely-matching substances. Comparing potential neurotoxicants in samples (especially e.g. faecal matter110 or house dust) from different groups in cohort studies requires the normalisation of the qualitative or quantitative signal. For instance, the concentration measured in an extract of stool is affected by the properties of the sample (water content, percentage of undigested matter, etc.) beyond even the complexity of wastewater samples, which have been “averaged” in a way throughout the journey to the treatment plant. In the metabolomics context, the retrospective normalisation of the measurement signal to the overall metabolite content (total ion chromatogram or TIC normalisation) or the dry weight of the sample are most common. While the TIC normalisation works only under the (theoretical) assumption that the overall metabolic profile is constant and comparable in all samples of the sequence, the retrospective normalisation to the sample dry weight makes it impossible to adjust sample preparation steps, like the amount of extraction fluid, to achieve best comparability between samples. Such issues make high quality non-targeted data processing and the generation of robust statistics extremely challenging, and will require some dedicated efforts in the coming years, as it is easy for such issues to be inadvertently disregarded, especially in automated workflows.
Measuring the chemical diversity of potential neurotoxicants
In terms of measurement, a large percentage of the neurotoxicants have polarities and ionizable groups that make these molecules amenable to analysis by liquid chromatography high resolution mass spectrometry (LC-HRMS). API techniques like electrospray ionisation (ESI) and atmospheric chemical ionisation (APCI) has dramatically improved compound discovery because of enhanced ionisation efficiency, as well as preventing the need for derivatisation (often the case with GC-MS) that may complicate data interpretation. Analytes that easily lose protons, mainly due to their gas phase acidity, can be analyzed by negative ESI, while strongly basic compounds in the gas phase are ionised with ease in positive mode.111,112 Weaker bases, on the other hand, are better detected with APCI ionisation.113 Some potential neurotoxicants that can be analyzed by APCI are hexamethylmelamine (https://comptox.epa.gov/dashboard/dsstoxdb/results?search=DTXSID4022579), almitrine (https://comptox.epa.gov/dashboard/dsstoxdb/results?search=DTXSID4057899), and diphenylhydantoin (https://comptox.epa.gov/dashboard/dsstoxdb/results?search=DTXSID8020541), which have functional groups that are known to ionise better using APCI (triazine for the first two and phenylurea for the last). Endosulfan (https://comptox.epa.gov/dashboard/dsstoxdb/results?search=DTXSID1020560) has also been shown to be APCI amenable, which provides better precursor ion information compared to EI analysis.114 Another technique that may find more utility in the future is atmospheric pressure photoionisation (APPI) for the analysis of compounds that ionise poorly using the ionisation techniques mentioned thus far.115
Another lesson learned from the neurotoxicant mapping is that many of these compounds are very polar and thus tend to elute at void volume when using reversed phase LC. Because unretained matrix components like macromolecules and salts also come out at or near the void time, hydrophilic molecules tend to suffer from ionisation suppression. Some specific examples include amygdalin (https://comptox.epa.gov/dashboard/dsstoxdb/results?search=DTXSID00897159), stavudine (https://comptox.epa.gov/dashboard/dsstoxdb/results?search=DTXSID1023819), fludarabine (https://comptox.epa.gov/dashboard/dsstoxdb/results?search=DTXSID4039657) and allopurinol (https://comptox.epa.gov/dashboard/dsstoxdb/results?search=DTXSID4022573). Hydrophilic interaction liquid chromatography (HILIC) is often able to retain these compounds better, thus providing better analyte signal integrity. HILIC would potentially open up unique chemical space that has received very little attention in environmental analysis up until recent years.1 Enabling a better separation of compounds of interest will be critical to obtaining sufficient and clean fragmentation information to assist in identification and confirmation, but requires the addition of yet another method to the NT-HR-MS toolbox.
While derivatisation in LC-MS may sometimes be necessary to improve the analytical signal116 and can be used to identify compounds with certain toxicophores,23 complicated data interpretation often ensues. Measurements made with and without derivatisation, followed by customised data processing, may ease this process in the coming years. Another group of neurotoxicants that require special attention are the toxicants with masses above 1000 Da. For these molecules, multiple charging can be expected in NT-HR-MS and care must be taken when doing data analysis. While multiple charges can be detected in many non-target workflows, these are not commonly investigated in great detail and more work needs to be done in the mass spectrometric analysis of these analytes (see also discussion below).
Further inspection of the neurotoxicant list reveals that many entries are either heavy metals or contain heavy metals. Metals like chromium, lead, mercury, nickel, silver, and thallium, organometallics like dimethyl and ethyl mercury, and the metalloid arsenic have all been reported to have neurotoxic effects. However, many of these compounds are neither LC nor GC amenable. For these compounds one needs to perform a spectrophotometric analysis or use an inductively coupled plasma to convert these analytes to the gas phase. Preliminary results in the context of the ENTACT project revealed that some arsenic-containing organometallic compounds can indeed be detected by LC-NT-HR-MS with very informative fragmentation patterns, showing that these should certainly be considered in the interpretation of NT-HR-MS data, although not currently covered by many workflows – this integration will be critical in future years.
Looking beyond small molecules (toxic proteins)
Apart from small molecules, exposure to toxic biomacromolecules (>1000 Da) may also be highly relevant in the context of neurotoxicity. In this context, neurotoxic as well as amyloidogenic (https://en.wikipedia.org/wiki/Amyloid) proteins are of particular relevance as they have been implicated as causal factors in Alzheimer's and Parkinson's diseases.117 In particular, prions (misfolded proteins with infectious properties) have been implicated in triggering amyloid-beta, alpha-synuclein and tau misfolding. Once the misfolding of endogenous proteins occurs, propagation of misfolding may occur through mechanisms similar to those that underlie prion pathogenesis.118,119 Importantly, the causal agent for the initial misfolding may be distant from the brain regions that are ultimately affected by the diseases.120,121 In this context, the enteric nervous system has been implicated.122 Based on these observations, it has been postulated that molecules produced by the gut microbiota may trigger a slowly ascending pathological process.123,124 For instance, the gut microbiota of newly diagnosed Parkinson's disease patients is enriched in known mucus-foraging bacteria.125 Furthermore, apart from other bacterial toxins,126 proteins have been posited to trigger the misfolding of proteins in enteric neurons.127 Thus, a combination of mucus erosion and a resulting exposure to higher levels of microbial amyloidogenic proteins might at least play a role in Parkinson's disease. To obtain pointers to causal mechanisms, it is therefore essential to not only consider small molecules as potential causal agents but also proteins. This means that integrated multi-omic analyses will be highly relevant for resolving potential combinatorial mechanisms involved in neurotoxicity.
As mentioned above, it is possible that protein and peptide signals may be observed in small molecule measurements. This is a challenge that computational workflows will have to tackle increasingly in the future, with respect to the multiple charge states, large masses and also dealing with this information in compatible ways. While multi-omic integration is becoming a common buzzword, the reality is that workflows and formats are still largely incompatible. For instance, the protein structures for download in the Database of Bacterial ExoToxins for Human128 (DBETH, http://www.hpppi.iicb.res.in/btox/) are not in a format compatible with compound databases for small molecules such as the CompTox Chemicals Dashboard or any common small molecule cheminformatics formats. Thus, even obtaining chemical information such as masses and formulas needed for typical suspect or non-target screening workflows is already a challenge with this style of database and better integration will be needed in the future.
Relating chemical analytical results from NT-HR-MS to biology
Biological confirmation of neurotoxic effects
While a critical part of NT-HR-MS is identification and analytical confirmation of suspected chemicals with reference standards (where possible), the results must be related back to the original study question. In environmental studies this is quite often performed using bioassays, as in effect-directed analysis. A recent article contains extensive material discussing approaches for screening neurotoxicity from an ecological standpoint (“econeurotoxicity”) and we refer readers to Legradi et al.129 rather than reproducing a summarised form of this discussion here. The material below provides additional perspectives.
Specific features that distinguish the central nervous system (CNS) from other organs need to be considered when choosing the biological systems, read-outs and methods to test the potential neurotoxic effects of environmental chemicals. These features include the presence of the blood–brain barrier, the high lipid content of the nervous tissue, the high energy requirement of neurons, the particular intercellular signaling system (synaptic transmission), the neural cell structure (long axonal projections), the presence of specific reactive endogenous molecules (e.g. dopamine) and the post-mitotic nature of neuronal cells (making them more sensitive to age-related accumulation of cellular damage130). In addition, the heterogeneity of the brain tissue and the sometimes highly selective susceptibility of certain neuronal cell types to only one or another neurotoxic substance calls for the use of more than one test system to maximise the chances of identifying hazardous effects of a potentially new neurotoxic compound.
A current tendency in the field of (neuro)toxicity testing is to transition from more observational projects using rodents to more mechanistic studies involving the use of in vitro (cellular) test systems.131 The latter include primary neural cells, immortalised neural cell lines and stem cells of non-human or human origin. Given species-specific responses to neurotoxic substances, even between rodents and humans, working with human derived cells (as opposed to cells from non-human origin) maximises the chances for making predictions on neurotoxicity relevant to the human nervous system. However, access to human primary cells is limited, given that they are derived from aborted foetuses or from brain surgery resections. Although less limited, isolation of primary cells from rodents is laborious and requires animal sacrifices for each new experiment. This explains the widespread use of rodent or human cell lines for neurotoxicity testing, even though the physiological relevance of the results generated in these systems are more questionable due to their tumorigenic origin or the immortalisation process applied (e.g. overexpression of oncogenes or telomerase). A very dynamic and expanding field is the use of mouse or human derived embryonic stem cells (ESCs) and of human induced pluripotent stem cells (iPSCs), which have been derived more recently by reprogramming of human somatic cells.132,133 A panoply of protocols have been developed to differentiate iPSCs into different neural cell types.134 Exposing these cells to potential neurotoxicants during the differentiation process allows testing more specifically for developmental neurotoxicity. Finally, rapid progress is ongoing in the development of 3D organoid models of the brain, which are also derived from iPSCs.135–137 Here the aim is to better mimic the complexity of the human brain, or certain parts of it (e.g. midbrain, relevant for Parkinson's disease research), by creating in vitro tissue structures including several cell types in a spatial organisation that reflects closely the physiological situation, but that offer easy access for experimental manipulations.
Common endpoints for in vitro neurotoxicity testing and the analytical approaches used for measuring those endpoints have been reviewed in Schmidt et al.131 with a focus on those methods that allow for high-throughput screening. Briefly, the endpoints can be grouped into viability, morphological and functional read-outs. Viability assays allow testing for neurotoxicity by determining whether one or several types of neural cells are more sensitive to compounds of interest than other cell types. At sub-cytotoxic concentrations, compounds can be further tested for their impact on neural cell morphology or function with endpoints including the monitoring of neurite outgrowth, spontaneous electrical activity of neuronal networks, receptor signaling and cell communication, reactive oxygen species formation, cell migration, mitochondrial transport, calcium storage and release, cell membrane potential, gliosis (i.e. proliferation of glial cells), myelination, network formation, and synaptogenesis. A number of these endpoints, as well as cell viability, can be measured by high-throughput screens based on absorbance, fluorescence or luminescence measurements in multi-well plates. An interesting, more recent development are microelectrode arrays (MEA) that allow for the measurement of extracellular electrical fields of neurons that grow on them. The mean firing rate has been proposed to be the most sensitive parameter for neurotoxicity screening via this method, which allows measurement of the spontaneous electrical activity arising in neural networks at relatively high throughput (24-well MEA plates).138 High-content imaging, i.e. automated fluorescent imaging of fixed or live cells in a high-throughput manner, is a technique that continues to undergo important developments, but has already become a central part of neuroscience and related compound screens.139 Transcriptomics, miRNA profiling and metabolomics are used as analytical tools to characterise molecular changes that occur in response to neurotoxicant exposure. These techniques hold promise for defining molecular signatures that are specific for certain chemicals, providing a read-out that can be more sensitive than morphological changes.140,141
Susceptibility to (neuro)toxicants is defined to a certain extent by the genetic background of each individual. Large cohorts of exposed versus non-exposed individuals are necessary to link genes and pathways with neurotoxicants of interest via genome-wide association studies. A way to circumvent this limitation is to work with model organisms in the lab that are easy to manipulate genetically. Budding yeast can be used for toxicogenomic screens in a highly time and cost-efficient way, given the existence of barcoded genome-wide gene deletion collections.142 Candidate susceptibility genes identified in this simple eukaryotic model organism can subsequently be validated in human cell culture models, if human homologs of the yeast genes exist. Humanised yeast models relevant for Parkinson's disease research have been created through overexpression in the yeast model of the human protein alpha-synuclein143 and have already successfully been used for high-throughput genetic144 and drug screens.145 Similarly, these models could be used to implement neurotoxicity screens and identify compounds that may trigger or advance the onset of PD. Roundworm (Caenorhabditis elegans) and zebrafish (Danio rerio) have emerged as simplified multicellular (non-mammalian) model organisms to be used in gene–environment interaction studies.146 Both organisms have well-characterised nervous systems; additionally, neurodevelopmental processes can be visualised conveniently in vivo given the transparency of C. elegans and zebrafish larvae. Neurotoxicity can also be probed in these animals via behavioural studies using computerised tracking systems, which enable high-throughput screens. Candidate susceptibility genes and/or pathways emerging from studies on these simpler model organisms have to be validated in more focused studies in human cell culture systems such as the ones described above. The challenges faced in the toxicogenomics and toxicogenetics fields are similar in many ways to the pharmacogenomics and pharmacogenetics fields and closer cooperation and collaboration between the fields would be beneficial; especially the environmental and toxicological fields could benefit from greater mathematical modelling and prediction such as the virtual physiological human147 and the Avicenna Alliance (Association for Predictive Medicine).148
Relating chemicals/environment to disease
While the first instance for confirming potential neurotoxic effects is a biological confirmation with relatively simple models such as those described above, the ultimate aim will be to relate the chemical exposure to the disease state in humans. As mentioned above, complex, long-term neurodegenerative disorders can involve a lifetime of exposure and yield multiple symptoms that may arise from vastly different causes, whereas the same causes may yield different symptoms in patients. Thus, it remains to be determined how compounds detected with NT-HR-MS can be integrated into the pathomechanism of the disease. For instance, rotenone (https://comptox.epa.gov/dashboard/dsstoxdb/results?search=DTXSID6021248) (a broad-spectrum insecticide) and paraquat (https://comptox.epa.gov/dashboard/dsstoxdb/results?search=DTXSID3034799) (a widely used herbicide) are shown to induce oxidative stress and cytotoxicity via the activation of microglia NOX2, thus leading to cell death of dopaminergic neurons.149 In other cases, there is clear evidence indicating gene–environment interactions. In one case study, a two-fold difference in PD risk was observed for polymorphic forms of CYP2D6, i.e. the combination of a genetic influence and pesticide exposure was needed for increased risk of developing PD.150 However, for many compounds the biomechanistic role in the disease process is not clear. The world-wide import and export of food, for example, has implications in exposure (and thus source attribution and suspect screening efforts) as the food we consume is no longer typically produced in the local area, but rather internationally in regions with completely different pesticide use and regulations (e.g. 75–95% of pesticide and veterinary antibiotic use associated with food products in Sweden are estimated to occur outside Sweden due to food imports).151,152 An additional challenge is the promiscuity or lack of specificity of many small molecules,153 which may bind to many different proteins with many different affinities, rendering establishing clean cause–effect relationships challenging. As it may also be difficult to predict toxicity for some small molecules, it will be important to discover the relevant effects to warn of certain substances and substance classes. All these factors must be considered in screening studies, requiring worldwide data exchange and a far greater (potential) substance coverage than offered by limited targeted chemical analysis.
It is clear that NT-HR-MS has a lot of potential to provide comprehensive information about potential environmental contaminants and metabolite signatures that may influence disease states. Samples taken and measured now can be archived (both digitally and physically) and screened retrospectively such that chemicals identified in the future could be traced back to determine whether these have, in fact, been present in samples for many years, similar to the NORMAN Early Warning System (NormaNEWS)12 or the daily monitoring of the Rhine river.1 However, to do this for long term diseases such as PD will require an incredible investment into high quality patient cohorts, biobanking of the samples, appropriate analytical measurements, state-of-the-art data analysis, carefully-designed biological validation and cross-disciplinary interpretation of the myriad of ensuing results. Such research extends far beyond a single few-year research project or a single research group, or even a dedicated institution such as the Luxembourg Centre for Systems Biomedicine, but requires international collaboration and support for the open exchange of information (within legal frameworks such as data protection and respecting patient confidentiality etc.).
Conclusions/perspectives
With this perspective we have started a conversation amongst several research groups at one institute and close collaborators to scope the breadth of experience needed to begin to perform a prospective, high quality, comprehensive assessment of potential small molecule neurotoxicants in environmental and biological samples using cheminformatics and non-target high resolution mass spectrometry, and what would be required to link this back to the disease state. Screening the thousands of chemicals potentially involved is a huge task that can be eased significantly by using some carefully-considered cheminformatics, literature-based and data processing methods and we hope users find the resources prepared in this article a useful way to start to explore different approaches for data processing. While we have prepared and described the methods using resources we use and develop, the data files and concepts are transferable to the many excellent workflows and approaches already available. Realistically, non-target MS-based analysis will have to evolve towards a few harmonised methods to ensure sufficient coverage of substances of interest in different contexts. However, compounds not covered by small molecule HR-MS analysis also play a defining role in diseases, such as larger molecules (requiring multi-omics approaches) or metals in salt forms (requiring alternative analysis) and these also need consideration in the bigger picture. Despite the compilation of many chemical lists, it is also clear that these chemicals transform and, although not covered in detail here, the candidate space for NT-HR-MS can and should be extended to screen for predicted transformation products and metabolites of potential neurotoxicants. Another area to explore in future work is a better connection to the concept of disease maps, such as the Parkinson's disease map,154 which can be queried computationally – and thus potentially integrated into mass spectral workflows – via interfaces such as MINERVA.155
While hypothesis generation can begin already from data snapshots on patients and controls at certain time points, in reality the prioritisation and discovery of “driving” neurotoxicants will depend on forming high quality, long term datasets involving cohorts where participants are recruited even before any symptoms are displayed, so that long term trends become clear. Ideally, this would be extended across multiple cohorts to allow hypothesis development in one cohort and replication in another based on chemical-environmental stratification. Alternatively, the increasing development of sensors and portable devices that can be given to patients and the general population open up new opportunities for citizen science, crowd sourcing of data and greater collaborative efforts to form detailed sampling campaigns that would provide complementary information to cohort data. The increasing willingness to share data in open resources such as the Global Natural Products Social Molecular Networking (GNPS)156 repository will open up many new opportunities in the coming years. This includes greater availability of larger public datasets on which to develop machine learning and artificial intelligence approaches to improve identification and classification of relevant chemicals and exposures.157 High resolution mass spectrometry of small molecules is certainly a well-placed technique to start building the knowledge to help answer these extremely complicated questions, assuming the long term support is available to create and maintain such extremely valuable datasets. In the end, however, this will provide only a starting point for further investigations. In addition to hands on biological experiments to elucidate possible exposure routes or gene–environment interactions, additional efforts in computational modeling and simulation using mathematical and mechanistic models will be needed to turn identified chemicals into a greater understanding of an extremely complex system and potential environmental causes of chronic diseases.
Author contributions
ELS conceptualised the perspective upon invitation and coordinated the writing. General contributions: cheminformatics: NCB (literature mining), AJW (registration, lists) and ELS (NT-HR-MS); analytical/samples: RS, JPT, PLK, ELS. Domain-specific knowledge: JPT (biobanking, metabolomics), PLK (medical), RK (clinical/molecular neuroscience), PW (multi-omics), CLL (biology), NP (metabolomics), RB (systems biomedicine). All authors made essential contributions to discussions and material presented in this perspective.
Conflicts of interest
There are no conflicts to declare.
Acknowledgements
ELS is supported by the Luxembourg National Research Fund (FNR) for the ATTRACT project 12341006 and acknowledges additional discussions with Marek Ostaszewski (Bioinformatics Core, LCSB). The Luxembourg cohort, RK and PLK are supported by grants from the Luxembourg National Research Fund (FNR) within the National Centre of Excellence in Research on Parkinson's disease (NCER-PD; FNR/ NCER13/BM/11264123) and the PEARL programme (FNR; FNR/P13/6682797). NP is supported by an FNR Core Junior Grant (C16/BM/11339953). We are grateful to the peer reviewers for their thoughtful comments that have helped refine this manuscript. The views expressed in this paper are those of the authors and do not necessarily reflect the views or policies of the U.S. Environmental Protection Agency. Mention of trade names or commercial products does not constitute endorsement or recommendation for use.
Notes and references
- J. Hollender, E. L. Schymanski, H. P. Singer and P. L. Ferguson, Nontarget Screening with High Resolution Mass Spectrometry in the Environment: Ready to Go?, Environ. Sci. Technol., 2017, 51, 11505–11512 CrossRef CAS.
- D. I. Walker, K. Uppal, L. Zhang, R. Vermeulen, M. Smith, W. Hu, M. P. Purdue, X. Tang, B. Reiss, S. Kim, L. Li, H. Huang, K. D. Pennell, D. P. Jones, N. Rothman and Q. Lan, High-resolution metabolomics of occupational exposure to trichloroethylene, Int. J. Epidemiol., 2016, 45, 1517–1527 CrossRef PubMed.
- D. I. Walker, K. D. Pennell, K. Uppal, X. Xia, P. K. Hopke, M. J. Utell, R. P. Phipps, P. J. Sime, P. Rohrbeck, C. T. M. Mallon and D. P. Jones, Pilot Metabolome-Wide Association Study of Benzo(a)pyrene in Serum From Military Personnel, J. Occup. Environ. Med., 2016, 58, S44–S52 CrossRef CAS.
- Y. Liu, E. S. Richardson, A. E. Derocher, N. J. Lunn, H.-J. Lehmler, X. Li, Y. Zhang, J. Y. Cui, L. Cheng and J. W. Martin, Hundreds of Unrecognized Halogenated Contaminants Discovered in Polar Bear Serum, Angew. Chem., Int. Ed., 2018, 57, 16401–16406 CrossRef CAS.
- Y. Liu, M. Qian, X. Ma, L. Zhu and J. W. Martin, Nontarget Mass Spectrometry Reveals New Perfluoroalkyl Substances in Fish from the Yangtze River and Tangxun Lake, China, Environ. Sci. Technol., 2018, 52, 5830–5840 CrossRef CAS.
- C. M. G. Carpenter, L. Y. J. Wong, C. A. Johnson and D. E. Helbling, Fall Creek Monitoring Station: Highly Resolved Temporal Sampling to Prioritize the Identification of Nontarget Micropollutants in a Small Stream, Environ. Sci. Technol., 2019, 53, 77–87 CrossRef CAS.
- V. Albergamo, J. E. Schollée, E. L. Schymanski, R. Helmus, H. Timmer, J. Hollender and P. de Voogt, Non-target screening reveals time trends of polar micropollutants in a riverbank filtration system, Environ. Sci. Technol., 2019, 53(13), 7584–7594 CrossRef CAS.
- F. Freeling, N. A. Alygizakis, P. C. von der Ohe, J. Slobodnik, P. Oswald, R. Aalizadeh, L. Cirka, N. S. Thomaidis and M. Scheurer, Occurrence and potential environmental risk of surfactants and their transformation products discharged by wastewater treatment plants, Sci. Total Environ., 2019, 681, 475–487 CrossRef CAS.
-
R. J. Bennett and R. J. Chorley, Environmental systems: philosophy, analysis, and control, Princeton University Press, Princeton, N.J, 1978, ISBN: 978-0-691-08217-2 Search PubMed.
-
L. van Bertalanffy, General system theory: foundations, development, applications, Braziller, New York, Rev, 14th edn, 2003, ISBN: 978-0-8076-0453-3 Search PubMed.
- F. Hernández, S. Castiglioni, A. Covaci, P. de Voogt, E. Emke, B. Kasprzyk-Hordern, C. Ort, M. Reid, J. V. Sancho, K. V. Thomas, A. L. N. van Nuijs, E. Zuccato and L. Bijlsma, Mass spectrometric strategies for the investigation of biomarkers of illicit drug use in wastewater, Mass Spectrom. Rev., 2018, 37, 258–280 CrossRef.
- N. A. Alygizakis, S. Samanipour, J. Hollender, M. Ibáñez, S. Kaserzon, V. Kokkali, J. A. van Leerdam, J. F. Mueller, M. Pijnappels, M. J. Reid, E. L. Schymanski, J. Slobodnik, N. S. Thomaidis and K. V. Thomas, Exploring the Potential of a Global Emerging Contaminant Early Warning Network through the Use of Retrospective Suspect Screening with High-Resolution Mass Spectrometry, Environ. Sci. Technol., 2018, 52, 5135–5144 CrossRef CAS.
- H. P. Singer, A. E. Wössner, C. S. McArdell and K. Fenner, Rapid Screening for Exposure to “Non-Target” Pharmaceuticals from Wastewater Effluents by Combining HRMS-Based Suspect Screening and Exposure Modeling, Environ. Sci. Technol., 2016, 50, 6698–6707 CrossRef CAS.
- A. Wössner and H. Singer, S10|SWISSPHARMA|Pharmaceutical List with Consumption Data, Zenodo, 2017 DOI:10.5281/zenodo.2623485.
- C. Moschet, A. Piazzoli, H. Singer and J. Hollender, Alleviating the Reference Standard Dilemma Using a Systematic Exact Mass Suspect Screening Approach with Liquid Chromatography-High Resolution Mass Spectrometry, Anal. Chem., 2013, 85, 10312–10320 CrossRef CAS.
- C. Moschet, S11|SWISSPEST|Swiss Insecticides, Fungicides and TPs, Zenodo, 2017 DOI:10.5281/zenodo.2623741.
- G. K. Paulus, L. M. Hornstra, N. Alygizakis, J. Slobodnik, N. Thomaidis and G. Medema, The impact of on-site hospital wastewater treatment on the downstream communal wastewater system in terms of antibiotics and antibiotic resistance genes, Int. J. Hyg. Environ. Health, 2019, 222, 635–644 CrossRef CAS.
- N. Alygizakis, S6|ITNANTIBIOTIC|Antibiotic List: ITN MSCA ANSWER, Zenodo, 2016 DOI:10.5281/zenodo.2621957.
- Y. Wang, N. Yu, X. Zhu, H. Guo, J. Jiang, X. Wang, W. Shi, J. Wu, H. Yu and S. Wei, Suspect and Nontarget Screening of Per- and Polyfluoroalkyl Substances in Wastewater from a Fluorochemical Manufacturing Park, Environ. Sci. Technol., 2018, 52, 11007–11016 CrossRef CAS.
- N. Yu, H. Guo, J. Yang, L. Jin, X. Wang, W. Shi, X. Zhang, H. Yu and S. Wei, Non-Target and Suspect Screening of Per- and Polyfluoroalkyl Substances in Airborne Particulate Matter in China, Environ. Sci. Technol., 2018, 52, 8205–8214 CrossRef CAS.
- E. L. Schymanski, H. P. Singer, P. Longrée, M. Loos, M. Ruff, M. A. Stravs, C. Ripollés Vidal and J. Hollender, Strategies to Characterize Polar Organic Contamination in Wastewater: Exploring the Capability of High Resolution Mass Spectrometry, Environ. Sci. Technol., 2014, 48, 1811–1818 CrossRef CAS.
- C. Hug, N. Ulrich, T. Schulze, W. Brack and M. Krauss, Identification of novel micropollutants in wastewater by a combination of suspect and nontarget screening, Environ. Pollut., 2014, 184, 25–32 CrossRef CAS PubMed.
- M. Muz, M. Krauss, S. Kutsarova, T. Schulze and W. Brack, Mutagenicity in Surface Waters: Synergistic Effects of Carboline Alkaloids and Aromatic Amines, Environ. Sci. Technol., 2017, 51, 1830–1839 CrossRef CAS.
- C. H. Johnson, J. Ivanisevic and G. Siuzdak, Metabolomics: beyond biomarkers and towards mechanisms, Nat. Rev. Mol. Cell Biol., 2016, 17, 451–459 CrossRef CAS.
- B. Y. L. Peisl, E. L. Schymanski and P. Wilmes, Dark matter in host-microbiome metabolomics: tackling the unknowns–a review, Anal. Chim. Acta, 2018, 1037, 13–27 CrossRef CAS.
- I. Blaženović, T. Kind, J. Ji and O. Fiehn, Software Tools and Approaches for Compound Identification of LC-MS/MS Data in Metabolomics, Metabolites, 2018, 8, 31 CrossRef.
- W. Brack, S. Ait-Aissa, R. M. Burgess, W. Busch, N. Creusot, C. Di Paolo, B. I. Escher, L. Mark Hewitt, K. Hilscherova, J. Hollender, H. Hollert, W. Jonker, J. Kool, M. Lamoree, M. Muschket, S. Neumann, P. Rostkowski, C. Ruttkies, J. Schollee, E. L. Schymanski, T. Schulze, T.-B. Seiler, A. J. Tindall, G. De Aragão Umbuzeiro, B. Vrana and M. Krauss, Effect-directed analysis supporting monitoring of aquatic environments — an in-depth overview, Sci. Total Environ., 2016, 544, 1073–1118 CrossRef CAS.
- E. L. Schymanski, H. P. Singer, J. Slobodnik, I. M. Ipolyi, P. Oswald, M. Krauss, T. Schulze, P. Haglund, T. Letzel, S. Grosse, N. S. Thomaidis, A. Bletsou, C. Zwiener, M. Ibáñez, T. Portolés, R. de Boer, M. J. Reid, M. Onghena, U. Kunkel, W. Schulz, A. Guillon, N. Noyon, G. Leroy, P. Bados, S. Bogialli, D. Stipaničev, P. Rostkowski and J. Hollender, Non-target screening with high-resolution mass spectrometry: critical review using a collaborative trial on water analysis, Anal. Bioanal. Chem., 2015, 407, 6237–6255 CrossRef CAS.
- P. Rostkowski, P. Haglund, R. Aalizadeh, N. Alygizakis, N. Thomaidis, J. B. Arandes, P. B. Nizzetto, P. Booij, H. Budzinski, P. Brunswick, A. Covaci, C. Gallampois, S. Grosse, R. Hindle, I. Ipolyi, K. Jobst, S. L. Kaserzon, P. Leonards, F. Lestremau, T. Letzel, J. Magnér, H. Matsukami, C. Moschet, P. Oswald, M. Plassmann, J. Slobodnik and C. Yang, The strength in numbers: comprehensive characterization of house dust using complementary mass spectrometric techniques, Anal. Bioanal. Chem., 2019, 411(11), 1957–1977 CrossRef CAS.
- E. L. Schymanski, C. Ruttkies, M. Krauss, C. Brouard, T. Kind, K. Dührkop, F. Allen, A. Vaniya, D. Verdegem, S. Böcker, J. Rousu, H. Shen, H. Tsugawa, T. Sajed, O. Fiehn, B. Ghesquière and S. Neumann, Critical Assessment of Small Molecule Identification 2016: Automated Methods, J. Cheminf., 2017, 9, 22 Search PubMed.
- E. L. Schymanski, J. Jeon, R. Gulde, K. Fenner, M. Ruff, H. P. Singer and J. Hollender, Identifying Small Molecules via High Resolution Mass Spectrometry: Communicating Confidence, Environ. Sci. Technol., 2014, 48, 2097–2098 CrossRef CAS.
- L. Bijlsma, M. H. G. Berntssen and S. Merel, A Refined Nontarget Workflow for the Investigation of Metabolites through the Prioritization by in Silico Prediction Tools, Anal. Chem., 2019, 91, 6321–6328 CrossRef CAS.
- J. L. Little, C. D. Cleven and S. D. Brown, Identification of “Known Unknowns” Utilizing Accurate Mass Data and Chemical Abstracts Service Databases, J. Am. Soc. Mass Spectrom., 2011, 22, 348–359 CrossRef CAS.
- C. Ruttkies, E. L. Schymanski, S. Wolf, J. Hollender and S. Neumann, MetFrag relaunched: incorporating strategies beyond in silico fragmentation, J. Cheminf., 2016, 8, 3 Search PubMed.
- Z. Lai, H. Tsugawa, G. Wohlgemuth, S. Mehta, M. Mueller, Y. Zheng, A. Ogiwara, J. Meissen, M. Showalter, K. Takeuchi, T. Kind, P. Beal, M. Arita and O. Fiehn, Identifying metabolites by integrating metabolome databases with mass spectrometry cheminformatics, Nat. Methods, 2018, 15, 53–56 CrossRef CAS.
- Y. Djoumbou-Feunang, A. Pon, N. Karu, J. Zheng, C. Li, D. Arndt, M. Gautam, F. Allen and D. S. Wishart, CFM-ID 3.0: Significantly Improved ESI-MS/MS Prediction and Compound Identification, Metabolites, 2019, 9, 72 CrossRef CAS.
- A. J. Williams, C. M. Grulke, J. Edwards, A. D. McEachran, K. Mansouri, N. C. Baker, G. Patlewicz, I. Shah, J. F. Wambaugh, R. S. Judson and A. M. Richard, The CompTox Chemistry Dashboard: a community data resource for environmental chemistry, J. Cheminf., 2017, 9, 61 Search PubMed.
- D. S. Wishart, Y. D. Feunang, A. Marcu, A. C. Guo, K. Liang, R. Vázquez-Fresno, T. Sajed, D. Johnson, C. Li, N. Karu, Z. Sayeeda, E. Lo, N. Assempour, M. Berjanskii, S. Singhal, D. Arndt, Y. Liang, H. Badran, J. Grant, A. Serra-Cayuela, Y. Liu, R. Mandal, V. Neveu, A. Pon, C. Knox, M. Wilson, C. Manach and A. Scalbert, HMDB 4.0: the human metabolome database for 2018, Nucleic Acids Res., 2018, 46, D608–D617 CrossRef CAS.
- D. S. Wishart, Y. D. Feunang, A. C. Guo, E. J. Lo, A. Marcu, J. R. Grant, T. Sajed, D. Johnson, C. Li, Z. Sayeeda, N. Assempour, I. Iynkkaran, Y. Liu, A. Maciejewski, N. Gale, A. Wilson, L. Chin, R. Cummings, D. Le, A. Pon, C. Knox and M. Wilson, DrugBank 5.0: a major update to the DrugBank database for 2018, Nucleic Acids Res., 2018, 46, D1074–D1082 CrossRef CAS.
- D. Wishart, D. Arndt, A. Pon, T. Sajed, A. C. Guo, Y. Djoumbou, C. Knox, M. Wilson, Y. Liang, J. Grant, Y. Liu, S. A. Goldansaz and S. M. Rappaport, T3DB: the toxic exposome database, Nucleic Acids Res., 2015, 43, D928–D934 CrossRef CAS.
-
NORMAN Network, NORMAN Suspect List Exchange, https://www.norman-network.com/nds/SLE/, accessed 9 June 2019 Search PubMed.
-
NORMAN Network, NORMAN Suspect List Exchange on Zenodo, https://zenodo.org/communities/norman-sle/, accessed 9 June 2019 Search PubMed.
- A. D. McEachran, K. Mansouri, C. Grulke, E. L. Schymanski, C. Ruttkies and A. J. Williams, “MS-Ready” structures for non-targeted high-resolution mass spectrometry screening studies, J. Cheminf., 2018, 10, 45 Search PubMed.
- E. L. Schymanski and A. J. Williams, Open Science for Identifying “Known Unknown” Chemicals, Environ. Sci. Technol., 2017, 51, 5357–5359 CrossRef CAS.
- C. P. Wild, Complementing the genome with an ‘exposome’: the outstanding challenge of environmental exposure measurement in molecular epidemiology, Cancer Epidemiol., Biomarkers Prev., 2005, 14, 1847–1850 CrossRef CAS.
- S. M. Rappaport, Implications of the exposome for exposure science, J. Exposure Sci. Environ. Epidemiol., 2011, 21, 5–9 CrossRef CAS PubMed.
- G. W. Miller and D. P. Jones, The nature of nurture: refining the definition of the exposome, Toxicol. Sci., 2014, 137, 1–2 CrossRef CAS PubMed.
- P. Vineis, Exposomics: mathematics meets biology: Figure 1, Mutagenesis, 2015, gev068 CrossRef.
- W. R. Mundy, S. Padilla, J. M. Breier, K. M. Crofton, M. E. Gilbert, D. W. Herr, K. F. Jensen, N. M. Radio, K. C. Raffaele, K. Schumacher, T. J. Shafer and J. Cowden, Expanding the test set: chemicals with potential to disrupt mammalian brain development, Neurotoxicol. Teratol., 2015, 52, 25–35 CrossRef CAS PubMed.
- M. Aschner, S. Ceccatelli, M. Daneshian, E. Fritsche, N. Hasiwa, T. Hartung, H. Hogberg, M. Leist, A. Li, W. Mundy, S. Padilla, A. Piersma, A. Bal-Price, A. Seiler, R. Westerink, B. Zimmer and P. Lein, Reference compounds for alternative test methods to indicate developmental neurotoxicity (DNT) potential of chemicals: example lists and criteria for their selection and use, ALTEX, 2017, 34, 49–74 Search PubMed.
- P. Grandjean and P. Landrigan, Developmental neurotoxicity of industrial chemicals, Lancet, 2006, 368, 2167–2178 CrossRef CAS.
- N. C. Baker, E. L. Schymanski and A. J. Williams, S43|NEUROTOXINS|Neurotoxicants Collection from Public Resources, Zenodo, 2019 DOI:10.5281/zenodo.2656729.
- N. C. Baker, E. L. Schymanski and A. J. Williams, Literature Neurotoxicants: Excel Macro File, FigShare, 2018 DOI:10.23645/epacomptox.7334603.
- N. C. Baker, E. L. Schymanski and A. J. Williams, S37|LITMINEDNEURO | Neurotoxicants from literature mining PubMed, Zenodo, 2019 DOI:10.5281/zenodo.3242298.
-
EMBL-EBI, Chemical Entities of Biological Interest (ChEBI), https://www.ebi.ac.uk/chebi/, accessed 9 June 2019 Search PubMed.
- J. Hastings, G. Owen, A. Dekker, M. Ennis, N. Kale, V. Muthukrishnan, S. Turner, N. Swainston, P. Mendes and C. Steinbeck, ChEBI in 2016: improved services and an expanding collection of metabolites, Nucleic Acids Res., 2016, 44, D1214–D1219 CrossRef CAS.
-
Wikipedia, Wikipedia Category: Neurotoxins, https://en.wikipedia.org/wiki/Category:Neurotoxins, accessed 9 June 2019 Search PubMed.
-
Wikipedia, Wikipedia Article: Neurotoxin, https://en.wikipedia.org/wiki/Neurotoxin, accessed 9 June 2019 Search PubMed.
-
US National Library of Medicine, PubMed, https://www.nlm.nih.gov/bsd/pubmed.html, accessed 9 June 2019 Search PubMed.
-
US National Library of Medicine, Medical Subject Headings (MeSH), https://meshb.nlm.nih.gov/search, accessed 9 June 2019 Search PubMed.
- N. C. Baker and B. M. Hemminger, Mining connections between chemicals, proteins, and diseases extracted from Medline annotations, J. Biomed. Inf., 2010, 43, 510–519 CrossRef CAS.
- E. L. Schymanski, N. C. Baker and A. J. Williams, Suspect Lists for Neurotoxicant Screening, Zenodo, 2019 DOI:10.5281/zenodo.3243471.
-
US Environmental Protection Agency, Chemical List: Chemicals Demonstrating Effects on Neurodevelopment (DNTEFFECTS), https://comptox.epa.gov/dashboard/chemical_lists/dnteffects, accessed 9 June 2019 Search PubMed.
-
US Environmental Protection Agency, Chemical List: Chemicals Triggering Developmental Neurotoxicity In Vivo (DNTINVIVO), https://comptox.epa.gov/dashboard/chemical_lists/dntinvivo, accessed 9 June 2019 Search PubMed.
-
US Environmental Protection Agency, Chemical List: Potential Negative Controls for DNT Assays (DNTPOTNEG), https://comptox.epa.gov/dashboard/chemical_lists/dntpotneg, accessed 9 June 2019 Search PubMed.
- E. L. Schymanski and A. J. Williams, S24|HUMANNEUROTOX|List of Human Neurotoxins, Zenodo, 2018 DOI:10.5281/zenodo.2648769.
-
US Environmental Protection Agency, Chemical List: Human Neurotoxicants (HUMANNEUROTOX), https://comptox.epa.gov/dashboard/chemical_lists/humanneurotox, accessed 9 June 2019 Search PubMed.
-
US Environmental Protection Agency, Chemical List: Neurotoxicants Collection from Public Resources (NEUROTOXINS), https://comptox.epa.gov/dashboard/chemical_lists/neurotoxins, accessed 9 June 2019 Search PubMed.
-
US Environmental Protection Agency, Chemical List: Neurotoxicants from PubMed (LITMINEDNEURO), https://comptox.epa.gov/dashboard/chemical_lists/litminedneuro, accessed 9 June 2019 Search PubMed.
- H. Checkoway, K. Powers, T. Smith-Weller, G. M. Franklin, W. T. Longstreth and P. D. Swanson, Parkinson's disease risks associated with cigarette smoking, alcohol consumption, and caffeine intake, Am. J. Epidemiol., 2002, 155, 732–738 CrossRef.
- H. Qi and S. Li, Dose-response meta-analysis on coffee, tea and caffeine consumption with risk of Parkinson's disease, Geriatr. Gerontol. Int., 2014, 14, 430–439 CrossRef.
- A. Ascherio, S. M. Zhang, M. A. Hernán, I. Kawachi, G. A. Colditz, F. E. Speizer and W. C. Willett, Prospective study of caffeine consumption and risk of Parkinson's disease in men and women, Ann.
Neurol., 2001, 50, 56–63 CrossRef CAS.
- Y. Miyake, Y. Tsuboi, M. Koyanagi, T. Fujimoto, S. Shirasawa, C. Kiyohara, K. Tanaka, W. Fukushima, S. Sasaki, T. Yamada, T. Oeda, T. Miki, N. Kawamura, N. Sakae, H. Fukuyama, Y. Hirota and M. Nagai, LRRK2 Gly2385Arg polymorphism, cigarette smoking, and risk of sporadic Parkinson's disease: a case–control study in Japan, J. Neurol. Sci., 2010, 297, 15–18 CrossRef CAS.
- R. Erdmann, Neuroleptics and nicotine, Psychiatr Prax., 1995, 22, 223–227 CAS.
- A. M. Richard, R. S. Judson, K. A. Houck, C. M. Grulke, P. Volarath, I. Thillainadarajah, C. Yang, J. Rathman, M. T. Martin, J. F. Wambaugh, T. B. Knudsen, J. Kancherla, K. Mansouri, G. Patlewicz, A. J. Williams, S. B. Little, K. M. Crofton and R. S. Thomas, ToxCast Chemical Landscape: Paving the Road to 21st Century Toxicology, Chem. Res. Toxicol., 2016, 29, 1225–1251 Search PubMed.
-
R. J. Clarke, Coffee Volume 1 Chemistry, Springer, New York, 2013 Search PubMed.
- M. Jeszka-Skowron, A. Zgoła-Grześkowiak and T. Grześkowiak, Analytical methods applied for the characterization and the determination of bioactive compounds in coffee, Eur. Food Res. Technol., 2015, 240, 19–31 CrossRef CAS.
- R. Talhout, T. Schulz, E. Florek, J. van Benthem, P. Wester and A. Opperhuizen, Hazardous compounds in tobacco smoke, Int. J. Environ. Res. Public Health, 2011, 8, 613–628 CrossRef.
- C. Baumung, J. Rehm, H. Franke and D. W. Lachenmeier, Comparative risk assessment of tobacco smoke constituents using the margin of exposure approach: the neglected contribution of nicotine, Sci. Rep., 2016, 6, 35577 CrossRef CAS.
-
Tri-County Cessation Centre, Cigarette Ingredients, https://web.archive.org/web/20160121165220/http:/www.tricountycessation.org/tobaccofacts/Cigarette-Ingredients.html#list, accessed 9 June 2019 Search PubMed.
- K. L. Dionisio, K. Phillips, P. S. Price, C. M. Grulke, A. J. Williams, D. Biryol, T. Hong and K. K. Isaacs, The Chemical and Products Database, a resource for exposure-relevant data on chemicals in consumer products, Sci. Data, 2018, 5, 180125 CrossRef CAS.
- E. L. Schymanski and A. J. Williams, Community Resources Connecting Chemistry and Toxicity Knowledge to Environmental Observations (presentation), Zenodo, 2018 DOI:10.5281/zenodo.3242674.
- S. Lerche, I. Liepelt-Scarfone, G. Alves, P. Barone, S. Behnke, Y. Ben-Shlomo, H. Berendse, D. Burn, R. Dodel, D. Grosset, S. Heinzel, M. Hu, M. Kasten, R. Krüger, W. Maetzler, M. Moccia, B. Mollenhauer, W. Oertel, B. Roeben, U. Sünkel, U. Walter, K. Wirdefeldt and D. Berg, Methods in Neuroepidemiology Characterization of European Longitudinal Cohort Studies in Parkinson's Disease - Report of the JPND Working Group BioLoC-PD, Neuroepidemiology, 2015, 45, 282–297 Search PubMed.
- R. Krüger, J. Klucken, D. Weiss, L. Tönges, P. Kolber, S. Unterecker, M. Lorrain, H. Baas, T. Müller and P. Riederer, Classification of advanced stages of Parkinson's disease: translation into stratified treatments, J. Neural Transm., 2017, 124, 1015–1027 CrossRef.
- G. Hipp, M. Vaillant, N. J. Diederich, K. Roomp, V. P. Satagopam, P. Banda, E. Sandt, K. Mommaerts, S. K. Schmitz, L. Longhino, A. Schweicher, A.-M. Hanff, B. Nicolai, P. Kolber, D. Reiter, L. Pavelka, S. Binck, C. Pauly, L. Geffers, F. Betsou, M. Gantenbein, J. Klucken, T. Gasser, M. T. Hu, R. Balling and R. Krüger, The Luxembourg Parkinson's Study: A Comprehensive Approach for Stratification and Early Diagnosis, Front. Aging Neurosci., 2018, 10, 326 CrossRef.
- P. A. Harris, R. Taylor, R. Thielke, J. Payne, N. Gonzalez and J. G. Conde, Research electronic data capture (REDCap)--a metadata-driven methodology and workflow process for providing translational research informatics support, J. Biomed. Inf., 2009, 42, 377–381 CrossRef.
- B. Ritz and F. Yu, Parkinson's disease mortality and pesticide exposure in California 1984–1994, Int. J. Epidemiol., 2000, 29, 323–329 CrossRef CAS.
- S. Costello, M. Cockburn, J. Bronstein, X. Zhang and B. Ritz, Parkinson's Disease and Residential Exposure to Maneb and Paraquat From Agricultural Applications in the Central Valley of California, Am. J. Epidemiol., 2009, 169, 919–926 CrossRef.
- C. Moschet, I. Wittmer, J. Simovic, M. Junghans, A. Piazzoli, H. Singer, C. Stamm, C. Leu and J. Hollender, How a Complete Pesticide Screening Changes the Assessment of Surface Water Quality, Environ. Sci. Technol., 2014, 48, 5423–5432 CrossRef CAS.
-
US Environmental Protection Agency, CompTox Chemicals Dashboard: Chemical Lists Page, https://comptox.epa.gov/dashboard/chemical_lists, accessed 17 March 2019 Search PubMed.
- A. Elbaz, J. Clavel, P. J. Rathouz, F. Moisan, J.-P. Galanaud, B. Delemotte, A. Alpérovitch and C. Tzourio, Professional exposure to pesticides and Parkinson disease: Professional Pesticide Exposure and PD, Ann. Neurol., 2009, 66, 494–504 CrossRef.
- S. Kab, J. Spinosi, L. Chaperon, A. Dugravot, A. Singh-Manoux, F. Moisan and A. Elbaz, Agricultural activities and the incidence of Parkinson's disease in the general French population, Eur. J. Epidemiol., 2017, 32, 203–216 CrossRef CAS PubMed.
- D. Caparros-Lefebvre, L. I. Golbe, V. Deramecourt, C.-A. Maurage, V. Huin, V. Buée-Scherrer, H. Obriot, B. Sablonnière, F. Caparros, L. Buée and A. J. Lees, A geographical cluster of progressive supranuclear palsy in northern France, Neurology, 2015, 85, 1293–1300 CrossRef.
- L. Mirbahai and J. K. Chipman, Epigenetic memory of environmental organisms: a reflection of lifetime stressor exposures, Mutat. Res., Genet. Toxicol. Environ. Mutagen., 2014, 764–765, 10–17 CrossRef CAS.
- I. Blaženović, T. Kind, M. R. Sa, J. Ji, A. Vaniya, B. Wancewicz, B. S. Roberts, H. Torbašinović, T. Lee, S. S. Mehta, M. R. Showalter, H. Song, J. Kwok, D. Jahn, J. Kim and O. Fiehn, Structure Annotation of All Mass Spectra in Untargeted Metabolomics, Anal. Chem., 2019, 91, 2155–2162 CrossRef.
- A. K. Jarmusch, E. O. Elijah, F. Vargas, A. Bouslimani, R. R. da Silva, M. Ernst, M. Wang, K. K. del Rosario, P. C. Dorrestein and S. M. Tsunoda, Initial Development toward Non-Invasive Drug Monitoring via Untargeted Mass Spectrometric Analysis of Human Skin, Anal. Chem., 2019, 91(13), 8062–8069 CrossRef CAS.
- M. G. Weisskopf, J. Weuve, H. Nie, M.-H. Saint-Hilaire, L. Sudarsky, D. K. Simon, B. Hersh, J. Schwartz, R. O. Wright and H. Hu, Association of Cumulative Lead Exposure with Parkinson's Disease, Environ. Health Perspect., 2010, 118, 1609–1613 CrossRef CAS.
- M. Arora and C. Austin, Teeth as a biomarker of past chemical exposure, Curr. Opin. Pediatr., 2013, 25, 261–267 CrossRef CAS PubMed.
- M. Arora, A. Reichenberg, C. Willfors, C. Austin, C. Gennings, S. Berggren, P. Lichtenstein, H. Anckarsäter, K. Tammimies and S. Bölte, Fetal and postnatal metal dysregulation in autism, Nat. Commun., 2017, 8, 15493 CrossRef CAS PubMed.
- H. Morishita and M. Arora, Tooth-Matrix Biomarkers to Reconstruct Critical Periods of Brain Plasticity, Trends Neurosci., 2017, 40, 1–3 CrossRef CAS.
- E. Velthorst, L. Smith, G. Bello, C. Austin, C. Gennings, A. Modabbernia, N. Franke, S. Frangou, R. Wright, L. de Haan, A. Reichenberg and M. Arora, New Research Strategy for Measuring Pre- and Postnatal Metal Dysregulation in Psychotic Disorders, Schizophr. Bull., 2017, 43, 1153–1157 CrossRef.
- B. M. R. Appenzeller and A. M. Tsatsakis, Hair analysis for biomonitoring of environmental and occupational exposure to organic pollutants: state of the art, critical review and future needs, Toxicol. Lett., 2012, 210, 119–140 CrossRef CAS.
- B. M. R. Appenzeller, E. M. Hardy, N. Grova, C. Chata, F. Faÿs, O. Briand, H. Schroeder and R.-C. Duca, Hair analysis for the biomonitoring of pesticide exposure: comparison with blood and urine in a rat model, Arch. Toxicol., 2017, 91, 2813–2825 CrossRef CAS.
- A. C. Chiaia-Hernandez, R. Ashauer, M. Moest, T. Hollingshaus, J. Jeon, P. Spaak and J. Hollender, Bioconcentration of Organic Contaminants in Daphnia Resting Eggs, Environ. Sci. Technol., 2013, 47, 10667–10675 CrossRef CAS.
- A. C. Chiaia-Hernandez, M. Krauss and J. Hollender, Screening of Lake Sediments for Emerging Contaminants by Liquid Chromatography Atmospheric Pressure Photoionization and Electrospray Ionization Coupled to High Resolution Mass Spectrometry, Environ. Sci. Technol., 2013, 47, 976–986 CrossRef CAS.
- N. Ramírez, M. Z. Özel, A. C. Lewis, R. M. Marcé, F. Borrull and J. F. Hamilton, Exposure to nitrosamines in thirdhand tobacco smoke increases cancer risk in non-smokers, Environ. Int., 2014, 71, 139–147 CrossRef.
- E. M. Ulrich, J. R. Sobus, C. M. Grulke, A. M. Richard, S. R. Newton, M. J. Strynar, K. Mansouri and A. J. Williams, EPA's non-targeted analysis collaborative trial (ENTACT): genesis, design, and initial findings, Anal. Bioanal. Chem., 2019, 411, 853–866 CrossRef CAS.
- S. Lehmann, F. Guadagni, H. Moore, G. Ashton, M. Barnes, E. Benson, J. Clements, I. Koppandi, D. Coppola, S. Y. Demiroglu, Y. DeSouza, A. De Wilde, J. Duker, J. Eliason, B. Glazer, K. Harding, J. P. Jeon, J. Kessler, T. Kokkat, U. Nanni, K. Shea, A. Skubitz, S. Somiari, G. Tybring, E. Gunter, F. Betsou and International Society for Biological and Environmental Repositories (ISBER), Working Group on Biospecimen Science, Standard preanalytical coding for biospecimens: review and implementation of the Sample PREanalytical Code (SPREC), Biopreserv. Biobanking, 2012, 10, 366–374 CrossRef.
- P. Gątarek, M. Pawełczyk, K. Jastrzębski, A. Głąbiński and J. Kałużna-Czaplińska, Analytical methods used in the study of Parkinson's disease, TrAC, Trends Anal. Chem., 2019, 118, 292–302 CrossRef.
- N. Karu, L. Deng, M. Slae, A. C. Guo, T. Sajed, H. Huynh, E. Wine and D. S. Wishart, A review on human fecal metabolomics: Methods, applications and the human fecal metabolome database, Anal. Chim. Acta, 2018, 1030, 1–24 CrossRef CAS.
-
E. M. Thurman, I. Ferrer and A. Fernández-Alba, in Comprehensive Analytical Chemistry, Elsevier, 2005, vol. 43, pp. 369–401 Search PubMed.
-
E. de Hoffmann and V. Stroobant, Mass spectrometry: principles and applications, J. Wiley, Chichester, West Sussex, England; Hoboken, NJ, 3rd edn, 2007, ISBN: 978-0-470-03310-4 Search PubMed.
- E. M. Thurman, I. Ferrer and D. Barceló, Choosing between Atmospheric Pressure Chemical Ionization and Electrospray Ionization Interfaces for the HPLC/MS Analysis of Pesticides, Anal. Chem., 2001, 73, 5441–5449 CrossRef CAS.
- N. Quinete, J. Wang, A. Fernandez, J. Castro and P. R. Gardinali, Outcompeting GC for the detection of legacy chlorinated pesticides: online-SPE UPLC APCI/MSMS detection of endosulfans at part per trillion levels, Anal. Bioanal. Chem., 2013, 405, 5887–5899 CrossRef CAS PubMed.
- H. Hayen and U. Karst, Strategies for the liquid chromatographic–mass spectrometric analysis of non-polar compounds, J. Chromatogr. A, 2003, 1000, 549–565 CrossRef CAS.
- D. M. Butryn, M. S. Gross, L.-H. Chi, A. Schecter, J. R. Olson and D. S. Aga, “One-shot” analysis of polybrominated diphenyl ethers and their hydroxylated and methoxylated analogs in human breast milk and serum using gas chromatography-tandem mass spectrometry, Anal. Chim. Acta, 2015, 892, 140–147 CrossRef CAS.
- S. Majd, J. H. Power and H. J. M. Grantham, Neuronal response in Alzheimer's and Parkinson's disease: the effect of toxic proteins on intracellular pathways, BMC Neurosci., 2015, 16, 69 CrossRef PubMed.
- P. Brundin, R. Melki and R. Kopito, Prion-like transmission of protein aggregates in neurodegenerative diseases, Nat. Rev. Mol. Cell Biol., 2010, 11, 301–307 CrossRef CAS.
- B. Frost and M. I. Diamond, Prion-like mechanisms in neurodegenerative diseases, Nat. Rev. Neurosci., 2010, 11, 155–159 CrossRef CAS PubMed.
- H. Braak, U. Rüb, W. P. Gai and K. Del Tredici, Idiopathic Parkinson's disease: possible routes by which vulnerable neuronal types may be subject to neuroinvasion by an unknown pathogen, J. Neural Transm., 2003, 110, 517–536 CrossRef CAS.
- H. Braak, D. R. Thal, E. Ghebremedhin and K. Del Tredici, Stages of the Pathologic Process in Alzheimer Disease: Age Categories From 1 to 100 Years, J. Neuropathol. Exp. Neurol., 2011, 70, 960–969 CrossRef CAS.
- M. Rao and M. D. Gershon, The bowel and beyond: the enteric nervous system in neurological disorders, Nat. Rev. Gastroenterol. Hepatol., 2016, 13, 517–528 CrossRef CAS.
- S. Bhattacharjee and W. J. Lukiw, Alzheimer's disease and the microbiome, Front. Cell. Neurosci., 2013, 7, 153 Search PubMed.
- F. Scheperjans, The prodromal microbiome: The Prodromal Microbiome, Mov. Disord., 2018, 33, 5–7 CrossRef.
- A. Heintz-Buschart, U. Pandey, T. Wicke, F. Sixel-Döring, A. Janzen, E. Sittig-Wiegand, C. Trenkwalder, W. H. Oertel, B. Mollenhauer and P. Wilmes, The nasal and gut microbiome in Parkinson's disease and idiopathic rapid eye movement sleep behavior disorder: Nose and Gut Microbiome in PD and iRBD, Mov. Disord., 2018, 33, 88–98 CrossRef CAS.
- A. Burton, A Long Shot? Could neurodegenerative disease be caused by a cyanobacterial toxin?, Lancet Neurol., 2013, 12, 1043–1044 CrossRef.
- S. G. Chen, V. Stribinskis, M. J. Rane, D. R. Demuth, E. Gozal, A. M. Roberts, R. Jagadapillai, R. Liu, K. Choe, B. Shivakumar, F. Son, S. Jin, R. Kerber, A. Adame, E. Masliah and R. P. Friedland, Exposure to the Functional Bacterial Amyloid Protein Curli Enhances Alpha-Synuclein Aggregation in Aged Fischer 344 Rats and Caenorhabditis elegans, Sci. Rep., 2016, 6, 34477 CrossRef CAS.
- A. Chakraborty, S. Ghosh, G. Chowdhary, U. Maulik and S. Chakrabarti, DBETH: a Database of Bacterial Exotoxins for Human, Nucleic Acids Res., 2012, 40, D615–D620 CrossRef CAS.
- J. B. Legradi, C. Di Paolo, M. H. S. Kraak, H. G. van der Geest, E. L. Schymanski, A. J. Williams, M. M. L. Dingemans, R. Massei, W. Brack, X. Cousin, M.-L. Begout, R. van der Oost, A. Carion, V. Suarez-Ulloa, F. Silvestre, B. I. Escher, M. Engwall, G. Nilén, S. H. Keiter, D. Pollet, P. Waldmann, C. Kienle, I. Werner, A.-C. Haigis, D. Knapen, L. Vergauwen, M. Spehr, W. Schulz, W. Busch, D. Leuthold, S. Scholz, C. M. vom Berg, N. Basu, C. A. Murphy, A. Lampert, J. Kuckelkorn, T. Grummt and H. Hollert, An ecotoxicological view on neurotoxicity assessment, Environ. Sci. Eur., 2018, 30, 46 CrossRef CAS.
- V. N. Gladyshev, On the cause of aging and control of lifespan: heterogeneity leads to inevitable damage accumulation, causing aging; control of damage composition and rate of accumulation define lifespan, BioEssays, 2012, 34, 925–929 CrossRef.
- B. Z. Schmidt, M. Lehmann, S. Gutbier, E. Nembo, S. Noel, L. Smirnova, A. Forsby, J. Hescheler, H. X. Avci, T. Hartung, M. Leist, J. Kobolák and A. Dinnyés, In vitro acute and developmental neurotoxicity screening: an overview of cellular platforms and high-throughput technical possibilities, Arch. Toxicol., 2017, 91, 1–33 CrossRef CAS.
- K. Takahashi, K. Tanabe, M. Ohnuki, M. Narita, T. Ichisaka, K. Tomoda and S. Yamanaka, Induction of Pluripotent Stem Cells from Adult Human Fibroblasts by Defined Factors, Cell, 2007, 131, 861–872 CrossRef CAS.
- J. Yu, M. A. Vodyanik, K. Smuga-Otto, J. Antosiewicz-Bourget, J. L. Frane, S. Tian, J. Nie, G. A. Jonsdottir, V. Ruotti, R. Stewart, I. I. Slukvin and J. A. Thomson, Induced Pluripotent Stem Cell Lines Derived from Human Somatic Cells, Science, 2007, 318, 1917–1920 CrossRef CAS.
- L. Li, J. Chao and Y. Shi, Modeling neurological diseases using iPSC-derived neural cells: iPSC modeling of neurological diseases, Cell Tissue Res., 2018, 371, 143–151 CrossRef CAS.
- M. A. Lancaster, M. Renner, C.-A. Martin, D. Wenzel, L. S. Bicknell, M. E. Hurles, T. Homfray, J. M. Penninger, A. P. Jackson and J. A. Knoblich, Cerebral organoids model human brain development and microcephaly, Nature, 2013, 501, 373–379 CrossRef CAS.
- A. S. Monzel, L. M. Smits, K. Hemmer, S. Hachi, E. L. Moreno, T. van Wuellen, J. Jarazo, J. Walter, I. Brüggemann, I. Boussaad, E. Berger, R. M. T. Fleming, S. Bolognin and J. C. Schwamborn, Derivation of Human Midbrain-Specific Organoids from Neuroepithelial Stem Cells, Stem Cell Rep., 2017, 8, 1144–1154 CrossRef CAS.
- E. Berger, C. Magliaro, N. Paczia, A. S. Monzel, P. Antony, C. L. Linster, S. Bolognin, A. Ahluwalia and J. C. Schwamborn, Millifluidic culture improves human midbrain organoid vitality and differentiation, Lab Chip, 2018, 18, 3172–3183 RSC.
- A. Vassallo, M. Chiappalone, R. De Camargos Lopes, B. Scelfo, A. Novellino, E. Defranchi, T. Palosaari, T. Weisschu, T. Ramirez, S. Martinoia, A. F. M. Johnstone, C. M. Mack, R. Landsiedel, M. Whelan, A. Bal-Price and T. J. Shafer, A multi-laboratory evaluation of microelectrode array-based measurements of neural network activity for acute neurotoxicity testing, NeuroToxicology, 2017, 60, 280–292 CrossRef CAS.
- J. Delp, A high-throughput approach to identify specific neurotoxicants/developmental toxicants in human neuronal cell function assays, ALTEX, 2018, 235–253 CrossRef.
- A. K. Krug, S. Gutbier, L. Zhao, D. Pöltl, C. Kullmann, V. Ivanova, S. Förster, S. Jagtap, J. Meiser, G. Leparc, S. Schildknecht, M. Adam, K. Hiller, H. Farhan, T. Brunner, T. Hartung, A. Sachinidis and M. Leist, Transcriptional and metabolic adaptation of human neurons to the mitochondrial toxicant MPP+, Cell Death Dis., 2014, 5, e1222 CrossRef CAS.
- L. Smirnova, A. E. M. Seiler and A. Luch, microRNA Profiling as Tool for Developmental Neurotoxicity Testing (DNT): MicroRNA Profiling, Curr. Protoc. Toxicol., 2015, 64, 20.9.1–20.9.22 CrossRef.
- C. M. McHale, M. T. Smith and L. Zhang, Application of toxicogenomic profiling to evaluate effects of benzene and formaldehyde: from yeast to human: Yeast and human toxicogenomic approaches, Ann. N. Y. Acad. Sci., 2014, 1310, 74–83 CrossRef CAS.
- T. F. Outeiro, Yeast Cells Provide Insight into Alpha-Synuclein Biology and Pathobiology, Science, 2003, 302, 1772–1775 CrossRef CAS.
- S. Willingham, Yeast Genes That Enhance the Toxicity of a Mutant Huntingtin Fragment or -Synuclein, Science, 2003, 302, 1769–1772 CrossRef CAS.
- D. F. Tardiff, N. T. Jui, V. Khurana, M. A. Tambe, M. L. Thompson, C. Y. Chung, H. B. Kamadurai, H. T. Kim, A. K. Lancaster, K. A. Caldwell, G. A. Caldwell, J.-C. Rochet, S. L. Buchwald and S. Lindquist, Yeast Reveal a ‘Druggable’ Rsp5/Nedd4 Network that Ameliorates-Synuclein Toxicity in Neurons, Science, 2013, 342, 979–983 CrossRef CAS.
- M. Aschner, E. D. Levin, C. Suñol, J. O. Olopade, K. J. Helmcke, D. S. Avila, D. Sledge, R. H. Ali, L. Upchurch and S. Donerly, Gene–environment interactions: neurodegeneration in non-mammals and mammals, NeuroToxicology, 2010, 31, 582–588 CrossRef CAS.
-
Virtual Physiological Human Institute, Virtual Physiological Human Institute Website, http://www.vph-institute.org/, accessed 20 November 2018 Search PubMed.
-
Avicenna Alliance, Avicenna Alliance, https://avicenna-alliance.com/, accessed 20 November 2018.
- Z. Nayernia, V. Jaquet and K.-H. Krause, New Insights on NOX Enzymes in the Central Nervous System, Antioxid. Redox Signaling, 2014, 20, 2815–2837 CrossRef CAS.
- A. Elbaz, C. Levecque, J. Clavel, J.-S. Vidal, F. Richard, P. Amouyel, A. Alpérovitch, M.-C. Chartier-Harlin and C. Tzourio, CYP2D6 polymorphism, pesticide exposure, and Parkinson's disease: CYP2D6, pesticides, and PD, Ann. Neurol., 2004, 55, 430–434 CrossRef CAS.
-
C. Trimmer, PRINCE final report published, http://www.prince-project.se/prince-final-report-published/, accessed 5 November 2018 Search PubMed.
-
N. Steinbach, V. Palm, C. Cederberg, G. Finnveden, L. Persson, M. Persson, M. Berglund, I. Björk, E. Faure and C. Trimmer, Miljöpåverkan från svensk konsumtion: Nya indikatorer för uppföljning [Environmental Impacts from Swedish Consumption: New Indicators for Follow-up], Final report of the research project PRINCE, Naturvårdsverket, Stockholm, Sweden, 2018 Search PubMed.
- J. Baell and M. A. Walters, Chemistry: Chemical con artists foil drug discovery, Nature, 2014, 513, 481–483 CrossRef CAS.
- K. A. Fujita, M. Ostaszewski, Y. Matsuoka, S. Ghosh, E. Glaab, C. Trefois, I. Crespo, T. M. Perumal, W. Jurkowski, P. M. A. Antony, N. Diederich, M. Buttini, A. Kodama, V. P. Satagopam, S. Eifes, A. Del Sol, R. Schneider, H. Kitano and R. Balling, Integrating pathways of Parkinson's disease in a molecular interaction map, Mol. Neurobiol., 2014, 49, 88–102 CrossRef CAS.
- P. Gawron, M. Ostaszewski, V. Satagopam, S. Gebel, A. Mazein, M. Kuzma, S. Zorzan, F. McGee, B. Otjacques, R. Balling and R. Schneider, MINERVA-a platform for visualization and curation of molecular interaction networks, npj Syst. Biol. Appl., 2016, 2, 16020 CrossRef.
- M. Wang, J. J. Carver, V. V. Phelan, L. M. Sanchez, N. Garg, Y. Peng, D. D. Nguyen, J. Watrous, C. A. Kapono, T. Luzzatto-Knaan, C. Porto, A. Bouslimani, A. V. Melnik, M. J. Meehan, W.-T. Liu, M. Crüsemann, P. D. Boudreau, E. Esquenazi, M. Sandoval-Calderón, R. D. Kersten, L. A. Pace, R. A. Quinn, K. R. Duncan, C.-C. Hsu, D. J. Floros, R. G. Gavilan, K. Kleigrewe, T. Northen, R. J. Dutton, D. Parrot, E. E. Carlson, B. Aigle, C. F. Michelsen, L. Jelsbak, C. Sohlenkamp, P. Pevzner, A. Edlund, J. McLean, J. Piel, B. T. Murphy, L. Gerwick, C.-C. Liaw, Y.-L. Yang, H.-U. Humpf, M. Maansson, R. A. Keyzers, A. C. Sims, A. R. Johnson, A. M. Sidebottom, B. E. Sedio, A. Klitgaard, C. B. Larson, C. A. Boya P., D. Torres-Mendoza, D. J. Gonzalez, D. B. Silva, L. M. Marques, D. P. Demarque, E. Pociute, E. C. O'Neill, E. Briand, E. J. N. Helfrich, E. A. Granatosky, E. Glukhov, F. Ryffel, H. Houson, H. Mohimani, J. J. Kharbush, Y. Zeng, J. A. Vorholt, K. L. Kurita, P. Charusanti, K. L. McPhail, K. F. Nielsen, L. Vuong, M. Elfeki, M. F. Traxler, N. Engene, N. Koyama, O. B. Vining, R. Baric, R. R. Silva, S. J. Mascuch, S. Tomasi, S. Jenkins, V. Macherla, T. Hoffman, V. Agarwal, P. G. Williams, J. Dai, R. Neupane, J. Gurr, A. M. C. Rodríguez, A. Lamsa, C. Zhang, K. Dorrestein, B. M. Duggan, J. Almaliti, P.-M. Allard, P. Phapale, L.-F. Nothias, T. Alexandrov, M. Litaudon, J.-L. Wolfender, J. E. Kyle, T. O. Metz, T. Peryea, D.-T. Nguyen, D. VanLeer, P. Shinn, A. Jadhav, R. Müller, K. M. Waters, W. Shi, X. Liu, L. Zhang, R. Knight, P. R. Jensen, B. O. Palsson, K. Pogliano, R. G. Linington, M. Gutiérrez, N. P. Lopes, W. H. Gerwick, B. S. Moore, P. C. Dorrestein and N. Bandeira, Sharing and community curation of mass spectrometry data with Global Natural Products Social Molecular Networking, Nat. Biotechnol., 2016, 34, 828–837 CrossRef CAS PubMed.
- S. H. Liu, J. F. Bobb, K. H. Lee, C. Gennings, B. Claus Henn, D. Bellinger, C. Austin, L. Schnaas, M. M. Tellez-Rojo, H. Hu, R. O. Wright, M. Arora and B. A. Coull, Lagged kernel machine regression for identifying time windows of susceptibility to exposures of complex mixtures, Biostatistics, 2018, 19, 325–341 CrossRef.
Footnote |
† Electronic supplementary information (ESI) available. See DOI: 10.1039/c9em00068b |
|
This journal is © The Royal Society of Chemistry 2019 |
Click here to see how this site uses Cookies. View our privacy policy here.