DOI:
10.1039/C7RA02056B
(Review Article)
RSC Adv., 2017,
7, 53516-53524
Technological advances in current metabolomics and its application in tradition Chinese medicine
Received
19th February 2017
, Accepted 13th November 2017
First published on 21st November 2017
Abstract
During the last few years, many metabolomics technologies have been established in biomedical research for analyzing the changes of metabolite levels. They have also become important tools in the field of life sciences and are widely applied to discover innovative drugs, providing valuable methods to explore the function and essence of formulas in traditional Chinese medicine (TCM) research. Metabolomics shows major potential to provide a unique perspective for disease diagnosis and elucidate the action mechanism of TCM by measuring endogenous metabolites in biofluids. With the gradual expansion of the application fields of metabolomics, the related number of techniques is increasing day by day. The techniques of choice for the study of various low-molecular-weight metabolic pathways, and for the analysis of metabolites in search of the changes of biomarkers in TCM treatment of disease in biological samples include nuclear magnetic resonance (NMR) spectroscopy, chromatography coupled with mass spectrometry, and so forth. These techniques have been proven to be powerful tools for the detection of metabolites and biomarkers. Here, we will introduce the newly used analytical techniques for metabolomics, and metabolomics in TCM is also summarized.
1 Introduction
Metabolomics aims to characterize and quantify all small molecular metabolites in complex biological specimens including serum, plasma, urine, etc.1 Metabolomics is applied for rapid metabolite identification in global profiling of different living systems, because of improvements in the detection capabilities of current instrumentation techniques used in biomedical research. Since then, the technique has been greatly improved by coupling mass spectrometers to chromatography, such as liquid chromatography (LC), gas chromatography (GC), and capillary electrophoresis (CE), and various types of mass analyzers including magnetic or electric sector, time-of-flight (TOF), quadrupole (Q), ion trap (IT), and Fourier transform ion cyclotron resonance (FTICR) mass spectrometers.2,3 Due to the diverse properties of metabolites and the complexity of the metabolome, there is no single analytical platform that can be used for the detection of all metabolites in biological samples. A variety of techniques such as chromatography coupled with mass spectrometry4–6 and NMR spectroscopy are available in metabolomics at present.7,8 This is because these techniques can provide excellent resolution to determine individual molecular species. These are valuable tools for the screening of metabolites and biomarkers, and they are extremely beneficial in terms of the identification and analysis of interest.
Based on the compatibility theory of Chinese medical science, traditional Chinese medicine (TCM) is usually prescribed in combination of several medicinal herbs at a certain mass ratio, playing a key role in treatment of disease and the clinical prevention for centuries in China.9,10 TCM treats diseases with multi-component acting on multi-target simultaneously through multiple pathways, generating a range of actions that manifest as a comprehensive overall effect. The sources of these active constituents are complicated, ranging from original compounds of the prescription to their metabolites of the drugs in vivo after administration11,12 (Fig. 1). The identification of metabolites and absorbed constituents plays a critical role in elucidating mechanism of action and the therapeutic material basis. Thus, technique is important for reliable and practical analysis of the chemical constituents of TCM and the detection of potential biomarkers associated with TCM treatment. The findings demonstrate that the efficiency of ultra performance liquid chromatography (UPLC), the accuracy of Q-TOF-MS, and the sensitivity and quantitation ability of Qtrap-MS provide a method for the efficient and comprehensive chemical characterization and quality control of complex TCM.13 It is necessary and valuable to develop a reliability sensitivity analysis approach for the detection and identification of metabolites and absorbed constituents of TCM. It might pave the way for further investigations into the mechanisms of action of TCM.
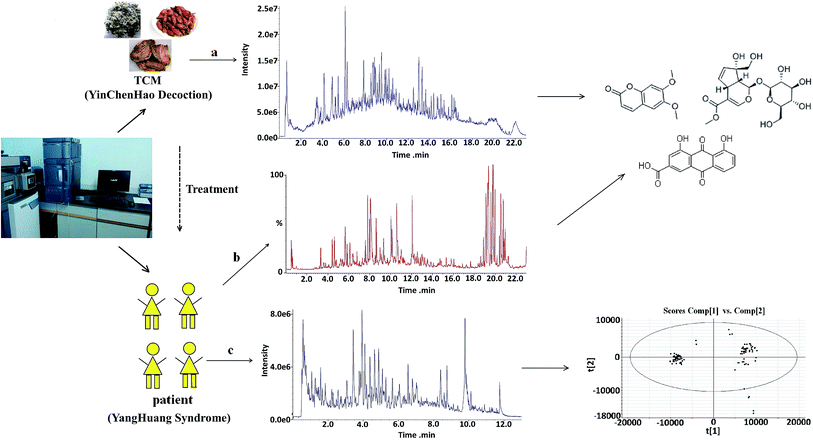 |
| Fig. 1 The application of metabolomics technology in TCM. YinChenHao Decoction (YCHD), a well-known TCM formula, is used for the treatment of YangHuang syndrome. A metabolomics approach and the multivariate statistical methods have been developed for identification and screening of original compounds and their metabolites of YCHD. Based on the same instrumental conditions, potential biomarkers associated with YangHuang syndrome and with YCHD treatment were detected and identified. (a) Original compounds of YCHD in vitro. (b) Original compounds and their metabolites of YCHD in vivo after administration. (c) Metabolomics-based studies on YangHuang syndrome. | |
2 Analytical technology
Over the past twenty years, an explosion of technological developments has been inspired by renewed interest in metabolic research to study metabolism. Chromatography-mass spectrometry and nuclear magnetic resonance (NMR) spectroscopy are the two most commonly used analytical technologies in metabolomics.14
2.1 NMR spectroscopy
NMR spectroscopy, a powerful technique, can be applied to elucidate the structures of organic compounds. NMR-based metabolomics analysis of biofluids accurately and quickly, without the need for initial separation or processing, has found wide applications, for example, in diagnosis of disease,15 physiological evaluation,16 characterization of animal models of disease,17 drug safety assessment,18,19 and drug therapy monitoring.20 The major strengths of NMR spectroscopy are its efficiency and ability to measure analytes. Recently, improvements have included stop-flow chromatography samples, lower instrument cost, and higher spectral resolution.21 This extremely important experimental technique is based on magnetic nuclear spin of 1H, 13C, 15N, 119Sn, and so on, and among them 1H and 13C-NMR spectroscopy are the common analytical technologies used for metabolomics primarily.22 A large amount of metabolic data can be generated by simple 1H-NMR experiments, which provides a surprising detailed to explore the biochemical events throughout the organism and study species differences based on toxicological biomarkers.23 The application of 13C-NMR raises the possibility of better separation between experimental groups and easier identification of metabolites using multivariate analyses, owing to better spectral dispersion.24 In the study of small molecule mixtures, two dimensional NMR (2D-NMR) spectra provides the benefit of more detailed structural information, which is particularly relevant to detect novel chemotypes.25 There are a series of 2D-NMR experiments commonly used in metabolomics, such as 2D J-resolved NMR, 2D and 3D diffusion ordered spectroscopy (DOSY), 2D 1H–1H correlation spectroscopy (COSY), 2D 1H–13C heteronuclear NMR, etc.26 Although, 2D-NMR experiments are relatively less sensitive compared to 1D experiments, the additional information available from 1H–13C cross peaks are invaluable for spectral annotation/quantitation, avoiding the peak overlaps that usually clutter the 1D spectrum.27 These technologies have commonality and own their features (Fig. 2), and thus it is essential to choose an appropriate analytical technology for the specific class of analyte of interest in the biomatrix.
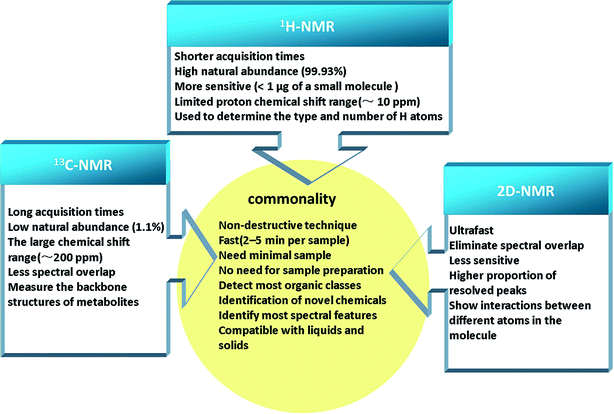 |
| Fig. 2 The commonality and features between 1H-NMR, 13C-NMR and 2D-NMR. | |
2.2 Chromatography-mass spectrometry
Mass spectrometry is a recommended approach for metabolomics research at present because it can directly and simultaneously analyze many compounds.28,29 The efficacy of MS in the study of endogenous metabolites stems from its proven success in disease studies,30–32 pharmacokinetics33,34 and drug-metabolite analysis.35 MS (especially Q-TOF) also helps to identify previously uncharacterized metabolites.13,36,37 MS in quantitative analysis of small molecules has been established, unlike its application to proteomics. Due to the complexity of metabolites and hundreds of metabolites therein, high-resolution MS has combined with advanced and high-throughput separation techniques, but not exclusively, to perform these measurements. MS provides an additional and completely independent method for the identification of compounds. By coupling chromatography to MS, the sensitivity of mass spectrometry has been widely used, and among them liquid chromatography-mass spectrometry (LC-MS) has already been applied to metabolomics studies of seminal plasma.38 Although these technologies each have their own strengths and weaknesses (Table 1), numerous studies have shown how they may be used to complement each other. Gas chromatography coupled with mass spectrometry (GC-MS) has been recognized as a primary tool for small molecule analysis,39 and is still used to detect various metabolic disorders today.40 Recent interest in metabolomics stems from the ability to carry out more comprehensive metabolome analysis using new liquid chromatography combined with mass spectrometry (LC-MS), which has the potential to discover and identify both disease and pharmaceutical biomarkers, exploring the hidden secrets of the biochemical processes of tissues and cells.41,42 Advances in LC-MS are very impressive, with recent developments in UPLC, the speed of analysis is further improved. Recent developments in analytical separation sciences have led to the emergence of technologies based on MS and UPLC, demonstrating ever improving sensitivity of mass measurements and resolution of metabolite species.43 In the field of metabolomics, today capillary electrophoresis-mass spectrometry (CE-MS), as a particularly useful complementary analytical technique, has been unitized to separate and detect ionic compounds based on the different migration rates of charged metabolites.6,44 In view of these advances, this current metabolomics technology can allow the extent of capability to become better diagnostic and therapeutic tools.
Table 1 The strengths and weaknesses of hyphenated MS techniques
|
Strengths |
Weaknesses |
Ref. |
GC-MS |
Good sensitivity (LOD = 0.5 μM) |
Sample not recoverable |
81–85 |
Suitable sample volume (0.1–0.2 mL) |
Sample derivatization is required |
Superb separation reproducibility wide dynamic range |
Separation is required |
Detects some inorganic and most organic molecules |
Long analysis time (20–40 min per sample) |
Can be mostly automated |
Not compatible with solids |
Novel compound identification is difficult |
LC-MS |
Great sensitivity (LOD = 0.5 nM) |
Sample not recoverable |
60 and 85–89 |
Very flexible technology |
Not very quantitative |
Detects some inorganic and most organic molecules |
Time-consuming sample preparation procedures |
Small sample volume (10–100 μL) |
Long analysis time (15–40 min per sample) |
Direct infusion |
Lower reproducibility and poor separation resolution |
Has the potential to detect the largest portion of metabolome |
Less-robust instrumentation |
Can be mostly automated |
Not compatible with gases |
Novel compound identification is difficult |
CE-MS |
Good sensitivity (LOD = 0.5 fM) |
Sample not recoverable |
43, 90 and 91 |
Smaller sample volume (1–50 nL) |
Relatively low sample throughputs |
High resolution |
Low separation reproducibility |
Polar ionogenic metabolites |
Not compatible with gases and solids |
Short analysis time (1–20 min per sample) |
Relatively less-robust instrumentation |
Can be mostly automated |
Poor migration time reproducibility |
Detects most organic, inorganic molecules, and biological macromolecules |
Low solvent consumption |
Can be done without separation (direct injection) |
3 Technological advances in metabolomics
Metabolomics, a new emerging discipline, evaluates the concentration of different metabolites in complex biological samples to understand the ongoing metabolism. Metabolites are the end products of a variety of metabolic pathways, which are involved in the occurrence of disease, and can be used as biomarkers for the evaluation of diagnosis, treatment, and prognosis of disease.45 At the forefront of methodological innovation is an approach called ‘‘non-targeted’’ or ‘‘discovery’’ metabolomics. In order to comprehensive analysis of the metabolome at the systems level, non-targeted metabolomics attempts to measure a largely undefined set of metabolites in a given biological specimen. In view of its potential comprehensive coverage, non-targeted metabolomics is often the first choice of experiments for investigators to pursue a metabolic research question.46
A new opportunity to discover biomarkers in complex diseases has been provided by metabolomics, which may improve the clinical course and provide pathological understanding of the disease, beyond the traditional technology.47 The potential of this approach for clinical diagnostics is enormous, since only minimal biological preparation is required. Recently, metabolomics-based studies on disease such as cancer have been used to screen plasma, urine, and tumor tissue from control populations and cancer patients.48–52 The first discovery of metabolic changes in cancer occurred almost a century ago. Recently altered metabolism has been identified as a key marker of cancer and metabolism-focused research has received renewed attention.50 Dereplication based on hyphenated techniques has been extensively applied in metabolomics, thereby recognizing disease with the metabolic relationship. Over the past decade, metabolomics research has provided the necessary advanced methods to identify changing metabolite levels, leading to rapid progress in disease biomarker discovery.53–55 Diagnostic cancer biomarkers detected by metabolomics have gained much attention in the field of clinical cancer research, to further understand its complex heterogeneity, to indicate changes in metabolic biomarkers during therapeutic intervention and to explore pathways involving cancer that can be used for new targets.56 We take the colon cancer as an example to demonstrate the metabolomics used within the field of colorectal cancer (CRC).
Denkert et al. reported a cluster of paired colorectal cancer and normal mucosa samples were studied by GC-TOF/MS, which detected 206 metabolites. Compared to normal colon mucosa, intermediates of lipids and the TCA cycle were decreased in colorectal cancer, while pyrimidines, purines, amino acids, and urea cycle metabolites were generally upregulated.57 Different types of samples were discriminated by the NMR spectrum, in this case between serum samples from patients with cancer and those from healthy volunteers. Identification of metabolites indicated upregulated levels of pyruvate, lactate and ketone bodies in patients with cancer.50 Qiu et al. used GC-MS coupled with a multivariate statistics technique to profile urine metabolites from healthy controls and patients with CRC. Discovery of abnormal glutamate metabolism and histamine metabolism only in the patient urine, and discovery of abnormal polyamine metabolism only in the urine samples of rats. This study demonstrated the metabolomic variations associated with CRC, and thus providing supplementary information to tissue and serum metabonomics to elucidate fully the underlying metabolic mechanisms of CRC.58
Biomarkers were found to be performed using FTICR-MS. Comprehensive metabolomic analyses showed that all three independent sets of patients with CRC had significantly reduced levels of hydroxylated, polyunsaturated ultra long-chain fatty acids compared to healthy subjects.59 Multivariate analysis of the GC peak areas was performed to visualise clusters within cases, discriminating the volatile metabolites between healthy individuals and cancer patients. It was well achieved to discriminate within cancer groups and between control and cancer groups.60 Cheng et al. investigated a second urinary metabonomic of a larger set of CRC patients and healthy controls studied by GC-TOF/MS and UPLC-qTOF-MS. Their findings confirmed different urinary metabolic footprints of patients with CRC, which were characterized by the changes in the levels of metabolites obtained from gut microbial-host co-metabolism. A set of metabolite markers composed of 2-aminobutyrate, myristate, citrate, putrescine, p-cresol, kynurenate, and hippurate was selected to distinguish between CRC patients and healthy subjects.61 We explored a variety of analytical technologies applied in the study of CRC metabolomics or in specific metabolites associated with CRC research. Early studies using NMR and GC-MS showed that characterization of tumor cells with aerobic glycolysis, up-regulation of purine metabolism for DNA synthesis, and protein synthesis. LC, CE, and GC, each in conjunction with MS, promise to advance the field and allow exploration of metabolic pathways using cancer cells. Technology improvement is required for conducting studies to determine better biomarkers and potential therapeutic targets to treat or prevent CRC.62
4 Application in TCM
Metabolomics technology platforms for disease research can offer more concise, direct, effective and rapid methods. In particular, metabolomics can serve as powerful tools for insight into the essence of Chinese medicine syndromes (CMS) disease, promoting personalized TCM. Metabolomics is a powerful technology that provides holistic metabolic profiles which is the assemblage of individuals and the dynamic change of an individual at a particular time point similar to the holistic and dynamic nature of TCM.63 Robust metabolomic approaches have played a key role in traditional herbal medicine; particular focusing on the past successes in metabonomics applications will help biomarkers discovery in TCM research. In light of the advantages of metabolomics, many studies have applied this approach to explore the effect of Chinese herbal medicines and the mechanisms of CMS disease.63,64
Up to now, there are several analytical technologies for the determination of metabolites in biological samples after oral administration of TCM. Blood plasma after oral administration of TCM contains hundreds even thousands of low-molecular-weight compounds that vary widely in stabilities and concentrations and are typically noncovalently bound to proteins.65 However, it is quite difficult to find the metabolites for TCMs by mass spectrometric data acquired automatically, due to the high background noise of biological samples, the absorbed compounds at low concentration and the shortage of reference standards.66–68 Therefore, the development of better technologies to find a way out of the difficulty is challenging.
4.1 Application of NMR and GC-MS in TCM
In order to explore the field of medicine especially Tradition Chinese Medicine (TCM) we should make the best of technology to develop metabonomics. As a case study, Jiang et al. explained the protective mechanisms of TCM, Xue–Sai–Tong injection, against myocardial ischemia/reperfusion (I/R) injury by the combination of 1H-NMR metabolomics and biochemical factors analysis. By comparing the metabolic characteristics of serum samples, it was found that 9 metabolites altered by I/R injury were restored to normal status (sham operation) after XST treatment. Pathway analysis showed the metabolic changes were mainly involved in citrate cycle, glycolysis, and pyruvate metabolism.69 Xiaoyaosan (XYS) which is one of the most famous TCM formulas in China has been prescribed to treat mental disorders. Tian et al. monitored the changes of metabolites in patients treated with XYS and determined changes in those metabolites concentrations respectively with GC-MS. In this study, 33 healthy volunteers and 25 depressed patients were recruited. By analyzing the urine metabolites of the healthy controls compared with depressed patients before and after treatment, five metabolites have been identified as therapeutic and potential disease biomarkers of Xiaoyaosan and depression.70 An empirical TCM prescription of Quzhuotongbi decoction (QZTBD) had no serious adverse effects in the treatment of hyperuricemia. Chen et al. developed the serum metabolic profiling of rats by a GC-MS approach, and further, explored the different mechanism of urate-lowering therapy by evaluating the effects of allopurinol and QZTBD on metabolic profiles.71
4.2 Application of UPLC-MS in TCM
Recently, the rapid and efficient technique of ultra-performance liquid chromatography-electrospray ionization quadrupole time-of-flight tandem mass spectrometry (UPLC-Q-TOF-MS) has been applied to analyze and identify the chemical constituents in TCM.13,72–74 This technique has particular advantages over conventional analytical methods in showing sensitivity or low concentration of metabolites and rapid extraction of target metabolites.75 Among the well-known TCM formulas, one named Zhi-zi-chi decoction (ZZCD). Characterization of the potential metabolites and bioactive constituents of ZZCD was performed by an UPLC-triple TOF-MS/MS approach comprehensively, which detected 109 potential bioactive compounds. After oral administration of ZZCD, 100 compounds were found in the rat biofluids, including 61 original ZZCD compounds and 39 metabolites under the same instrumental conditions.75 Senkyunolide I (SEI), a bioactive phthalides of Ligusticum chuanxiong Hort, was effectively analyzed and detected the metabolites in rats after oral administration and their chemical constituents with 1D and 2D-NMR and UPLC/Q-TOF-MS. They determined structures and obtained the major pathways of SEI metabolism in vivo by this method, which helped to identify unknown compounds and elucidated the mechanism of action of SEI.76 Traditional Chinese formula of Fangji Huangqi Tang (FHT) was screened and characterized the multiple constituents in vitro, and the potential bioactive components in the serum of rats in vivo with UHPLC-ESI-Q-TOF-MS.77 Zi Shen Wan (ZSW), a TCM formula, has been widely used in treatment of prostatitis and infection diseases. Li et al. indicated that UHPLC-MS in conjunction with MassLynx software and multivariate data processing approach could be used to rapidly screen and comprehensive analyze chemical constituents in vitro and prototype components or metabolites in vivo of TCM.78
5 Conclusion and perspective
Metabolomics applications as a methodology for insight into human disease continue to expand rapidly. Metabolomics has the potential to improve understanding disease, diagnosing disease, personalized medicine, risk stratification, monitoring the success of drug discovery and disease treatments. In particular, the technology platform of metabolomics is playing a key role in studying in-depth research of the constituents of TCM in vivo and in vitro. Over the years, chromatography-mass spectrometry and NMR have demonstrated themselves as powerful techniques for detecting and annotating diverse metabolite classes and have become necessary tools for metabolomics analysis in numerous organisms. Nowadays, a variety of conventional MS-based multiclass analyses are replaced by metabolomics approaches, providing excellent combinations of analytical and bioinformatics tools, and can offer comprehensive information on a large number of metabolites in any particular system. NMR allows a much better resolution of peaks since they are spread along an additional dimension.26,79 The current databases commonly store hundreds of metabolites, while a single organism contains several thousand diverse metabolites. Thus, a database-dependent approach has a significant limitation when it comes to determining of the entire metabolome of a complex biological system. A new approach, named SUMMIT MS/NMR, is well suited for high-throughput applications for the discovery of new metabolites in biological and biomedical mixtures, overcoming the need of experimental MS and NMR metabolite databases.80 Although the rapid development of chromatography-mass spectrometry and NMR has great prospects, there are many challenges in metabolomics applications, including complexity, high upfront costs, lack of user-friendliness, and the complexity of the science and the associated regulatory processes. For chemist/biochemists, it is important to open up new avenues to identify potentially unknown metabolites and overcome the obstacle and bottleneck in finding novel metabolites in complex biological mixtures.
Conflicts of interest
The authors declare no competing financial interests.
Acknowledgements
This work was supported by grants from the Key Program of Natural Science Foundation of State (Grant No. 81430093, 81373930, 81302905, 81673586), National Key Subject of Drug Innovation (Grant No. 2015ZX09101043-005, 2015ZX09101043-011), TCM State Administration Subject of Public Welfare of (Grant No. 2015468004), Specialized Research Fund for the Doctoral Program of Higher Education (20132327130001, 20122327120006), Application Technology and Development of Youth Talents Project in Harbin (2014RFQXJ116), University Nursing Program for Young Scholars with Creative Talents in Heilongjiang Province (UNPYSCT-2015118).
References
- J. K. Nicholson and J. C. Lindon, Systems biology: Metabonomics, Nature, 2008, 455(7216), 1054–1056 CrossRef CAS PubMed.
- G. T. Carter, NP/MS since 1970: from the basement to the bench top, Nat. Prod. Rep., 2014, 31(6), 711 RSC.
- J. S. Dickschat, Capturing volatile natural products by mass spectrometry, Nat. Prod. Rep., 2014, 31(6), 838–861 RSC.
- Y. Zhao, C. Zhao and Y. Li, et al., Study of metabolite differences of flue-cured tobacco from different regions using a pseudotargeted gas chromatography with mass spectrometry selected-ion monitoring method, J. Sep. Sci., 2014, 37(16), 2177–2184 CrossRef CAS PubMed.
- Y. Chang, C. Zhao and Z. Zhu, et al., Metabolic profiling based on LC/MS to evaluate unintended effects of transgenic rice with cry1Ac and sck genes, Plant Mol. Biol., 2012, 78(4–5), 477–487 CrossRef CAS PubMed.
- Y. Suzuki, T. Fujimori and K. Kanno, et al., Metabolome analysis of photosynthesis and the related primary metabolites in the leaves of transgenic rice plants with increased or decreased Rubisco content, Plant, Cell Environ., 2012, 35(8), 1369–1379 CrossRef CAS PubMed.
- D. Capitani, A. P. Sobolev and A. Tomassini, et al., Peach fruit: metabolic comparative analysis of two varieties with different resistances to insect attacks by NMR spectroscopy, J. Agric. Food Chem., 2013, 61(8), 1718–1726 CrossRef CAS PubMed.
- M. van Doorn, J. Vogels and A. Tas, et al., Evaluation of metabolite profiles as biomarkers for the pharmacological effects of thiazolidinediones in type 2 diabetes mellitus patients and healthy volunteers, Br. J. Clin. Pharmacol., 2007, 63(5), 562–574 CrossRef CAS PubMed.
- T. Y. Lee, H. H. Chang and M. Y. Wu, et al., Yin-Chen-Hao-Tang ameliorates obstruction-induced hepatic apoptosis in rats, J. Pharm. Pharmacol., 2007, 59(4), 583–590 CrossRef CAS PubMed.
- L. Wu, H. Li and S. Z. Zheng, et al., Da-Huang-Fu-Zi-Tang attenuates liver injury in rats with severe acute pancreatitis, J. Ethnopharmacol., 2013, 150(3), 960–966 CrossRef PubMed.
- Y. M. Lao, J. G. Jiang and L. Yan, Application of metabonomic analytical techniques in the modernization and toxicology research of traditional Chinese medicine, Br. J. Pharmacol., 2009, 157(7), 1128–1141 CrossRef CAS PubMed.
- X. Wang, H. Sun and A. Zhang, et al., Potential role of metabolomics approaches in the area of traditional Chinese medicine: as pillars of the bridge between Chinese and Western medicine, J. Pharm. Biomed. Anal., 2011, 55(5), 859–868 CrossRef CAS PubMed.
- X. Wang, A. Zhang and H. Sun, et al., Discovery and development of innovative drug from traditional medicine by integrated chinmedomics strategies in the post-genomic era, TrAC, Trends Anal. Chem., 2016, 76, 86–94 CrossRef CAS.
- A. Zhang, H. Sun and X. Wang, Mass spectrometry-driven drug discovery for development of herbal medicine, Mass Spectrom. Rev., 2016, 9999, 1–14 Search PubMed.
- M. E. Bollard, E. G. Stanley and J. C. Lindon, et al., NMR-based metabonomic approaches for evaluating physiological influences on biofluid composition, NMR Biomed., 2005, 18(3), 143–162 CrossRef CAS PubMed.
- J. T. Brindle, H. Antti and E. Holmes, et al., Rapid and noninvasive diagnosis of the presence and severity of coronary heart disease using 1H-NMR-based metabonomics, Nat. Med., 2002, 8(12), 1439–1444 CrossRef CAS PubMed.
- L. Zhao, H. Gao and F. Lian, et al., (1)H-NMR-based metabonomic analysis of metabolic profiling in diabetic nephropathy rats induced by streptozotocin, Am. J. Physiol., 2011, 300(4), F947–F956 CrossRef CAS PubMed.
- M. Coen, E. Holmes and J. C. Lindon, et al., NMR-based metabolic profiling and metabonomic approaches to problems in molecular toxicology, Chem. Res. Toxicol., 2008, 21(1), 9–27 CrossRef CAS PubMed.
- J. L. Griffin, Metabonomics: NMR spectroscopy and pattern recognition analysis of body fluids and tissues for characterisation of xenobiotic toxicity and disease diagnosis, Curr. Opin. Chem. Biol., 2003, 7(5), 648–654 CrossRef CAS PubMed.
- J. C. Lindon, E. Holmes and M. E. Bollard, et al., Metabonomics technologies and their applications in physiological monitoring, drug safety assessment and disease diagnosis, Biomarkers, 2004, 9(1), 1–31 CrossRef CAS PubMed.
- E. J. Want, B. f. Cravatt and G. Siuzdak, The expanding role of mass spectrometry in metabolite profiling and characterization, ChemBioChem, 2005, 6(11), 1941–1951 CrossRef CAS PubMed.
- K. E. Hollinshead, D. S. Williams and D. A. Tennant, et al., Probing Cancer Cell Metabolism Using NMR Spectroscopy, Adv. Exp. Med. Biol., 2016, 899, 89–111 CrossRef CAS PubMed.
- G. L. Gall, NMR Spectroscopy of Biofluids and Extracts, Methods Mol. Biol., 2014, 1277, 29–36 Search PubMed.
- C. Clendinen, B. Leemcmullen and C. M. Williams, et al., 13C NMR Metabolomics: Applications at Natural Abundance, Front. Plant Sci., 2014, 86(18), 9242–9250 CAS.
- F. C. Schroeder, D. M. Gibson and A. C. Churchill, et al., Differential Analysis of 2D NMR Spectra: New Natural Products from a Pilot-Scale Fungal Extract Library, Angew. Chem., Int. Ed., 2007, 46(6), 901–904 CrossRef CAS PubMed.
- E. A. Mahrous and M. A. Farag, Two dimensional NMR spectroscopic approaches for exploring plant metabolome: A review, J. Adv. Res., 2015, 6(1), 3 CrossRef CAS PubMed.
- A. Dubey, A. Rangarajan and D. Pal, et al., Chemical Shifts to Metabolic Pathways: Identifying Metabolic Pathways Directly from a Single 2D NMR Spectrum, Anal. Chem., 2015, 87(24), 12197–12205 CrossRef CAS PubMed.
- A. Zhang, H. Sun and Y. Han, et al., Urinary metabolic biomarker and pathway study of hepatitis B virus infected patients based on UPLC-MS system, PLoS One, 2013, 8(5), e64381 CAS.
- A. Zhang, H. Sun and G. Yan, et al., Urinary metabolic profiling identifies a key role for glycocholic acid in human liver cancer by ultra-performance liquid-chromatography coupled with high-definition mass spectrometry, Clin. Chim. Acta, 2013, 418, 86–90 CrossRef CAS PubMed.
- A. H. Zhang, H. Sun and Y. Han, et al., Ultraperformance liquid chromatography-mass spectrometry based comprehensive metabolomics combined with pattern recognition and network analysis methods for characterization of metabolites and metabolic pathways from biological data sets, Anal. Chem., 2013, 85(15), 7606–7612 CrossRef CAS PubMed.
- X. Wang, A. Zhang and G. Yan, et al., UHPLC-MS for the analytical characterization of traditional Chinese medicines, TrAC, Trends Anal. Chem., 2014, 63, 180–187 CrossRef CAS.
- A. Zhang, H. Sun and S. Dou, et al., Metabolomics study on the hepatoprotective effect of scoparone using ultra-performance liquid chromatography/electrospray ionization quadruple time-of-flight mass spectrometry, Analyst, 2013, 138(1), 353–361 RSC.
- K. Se-Hyung, K. Do-Hoon and B. Ji-Yeong, et al., Effects of CYP2C9 genetic polymorphisms on the pharmacokinetics of celecoxib and its carboxylic acid metabolite, Arch. Pharmacal Res., 2016, 1–9 Search PubMed.
- W. Xiong, J. Zhao and L. Wang, et al., UPLC-MS/MS method for the determination of tobacco-specific biomarker NNAL, tamoxifen and its main metabolites in rat plasma, Biomed. Chromatogr., 2017, 31(6), 1–34 CrossRef PubMed.
- Y. Liu, S. Zhou and M. Assaf, et al., Impact of Renal Impairment on the Pharmacokinetics of Apremilast and Metabolite M12, Clin. Pharmacol. Drug Dev., 2016, 5(6), 469–479 CrossRef CAS PubMed.
- T. Rousu and A. Tolonen, Characterization of cyanide-trapped methylated metabonates formed during reactive drug metabolite screening in vitro, Rapid Commun. Mass Spectrom., 2011, 25(10), 1382–1390 CrossRef CAS PubMed.
- H. Jiang, J. m. Song and P. f. Gao, et al., Metabolic characterization of the early stage of hepatic fibrosis in rat using GC-TOF/MS and multivariate data analyses, Biomed. Chromatogr., 2017, 31(6), 1–8 CrossRef PubMed.
- J. Vitku, L. Sosvorova and T. Chlupacova, et al., Differences in bisphenol A and estrogen levels in the plasma and seminal plasma of men with different degrees of infertility, Physiol. Res., 2015, 64, 1–9 Search PubMed.
- N. W. Kwiecien, D. J. Bailey and M. J. P. Rush, et al., High-Resolution Filtering for Improved Small Molecule Identification via GC/MS, Anal. Chem., 2015, 87(16), 8328–8335 CrossRef CAS PubMed.
- S. J. Park, I. H. Jeong and B. S. Kong, et al., Disease Type- and Status-Specific Alteration
of CSF Metabolome Coordinated with Clinical Parameters in Inflammatory Demyelinating Diseases of CNS, PLoS One, 2016, 11(11), e0166277 Search PubMed.
- Y. Jiang, B. Mistretta and S. Elsea, et al., Simultaneous determination of plasma total homocysteine and methionine by liquid chromatography-tandem mass spectrometry, Clin. Chim. Acta, 2017, 464, 93–97 CrossRef CAS PubMed.
- H. Huang, T. T. Tong and Y. Lee-Fong, et al., LC-MS Based Sphingolipidomic Study on A2780 Human Ovarian Cancer Cell Line and its Taxol-resistant Strain, Sci. Rep., 2016, 6, 34684 CrossRef CAS PubMed.
- A. Zhang, H. Sun and P. Wang, et al., Modern analytical techniques in metabolomics analysis, Analyst, 2012, 137(2), 293–300 RSC.
- R. Ramautar, G. W. Somsen and G. J. D. Jong, CE-MS for metabolomics: Developments and applications in the period 2012–2014, Electrophoresis, 2015, 36(1), 212–224 CrossRef CAS PubMed.
- H. Chu, H. Sun and G. Yan, et al., Metabolomics analysis of health functions of Physalis pubescens L. using by ultra-performance liquid chromatography/electrospray ionization quadruple time-of-flight mass spectrometry, World J. Tradit. Chin. Med., 2015, 1(3), 1–12 CrossRef.
- Y. Ma, P. Zhang and F. Wang, et al., An integrated proteomics and metabolomics approach for defining oncofetal biomarkers in the colorectal cancer, Ann. Surg., 2012, 255(4), 720–730 CrossRef PubMed.
- A. Zhang, H. Sun and G. Yan, et al., Metabolomics for Biomarker Discovery: Moving to the Clinic, BioMed Res. Int., 2015, 2015, 354671 Search PubMed.
- L. Deng, H. Gu and J. Zhu, et al., Combining NMR and LC/MS Using Backward Variable Elimination: Metabolomics Analysis of Colorectal Cancer, Polyps, and Healthy Controls, Anal. Chem., 2016, 88(16), 7975–7983 CrossRef CAS PubMed.
- X. Liu, Z. Ser and J. W. Locasale, Development and quantitative evaluation of a high-resolution metabolomics technology, Anal. Chem., 2014, 86(4), 2175–2184 CrossRef CAS PubMed.
- Q. Liang, C. Wang and B. Li, et al., Lipidomics Analysis Based on Liquid Chromatography Mass Spectrometry for Hepatocellular Carcinoma and Intrahepatic Cholangiocarcinoma, RSC Adv., 2015, 5, 63711–63718 RSC.
- I. Bertini, S. Cacciatore and B. V. Jensen, et al., Metabolomic NMR fingerprinting to identify and predict survival of patients with metastatic colorectal cancer, Cancer Res., 2012, 72(1), 356–364 CrossRef CAS PubMed.
- Q. Liang, C. Wang and H. Wu, et al., Metabolite fingerprint analysis of cervical cancer using LC-QTOF/MS and multivariate data analysis, Anal. Methods, 2014, 6, 3937 RSC.
- Q. Liang, H. Liu and X. Li, et al., High-throughput metabolomics analysis discovers salivary biomarkers for predicting mild cognitive impairment and Alzheimer's disease, RSC Adv., 2016, 6, 75499–75504 RSC.
- Q. Liang, H. Liu and Y. Jiang, et al., Discovering lipid phenotypic changes of sepsis-induced lung injury using high-throughput lipidomic analysis, RSC Adv., 2016, 6, 38233–38237 RSC.
- Q. Liang, H. Liu and H. Xing, et al., UPLC-QTOF/MS based metabolomics reveals metabolic alterations associated with severe sepsis, RSC Adv., 2016, 6, 43293–43298 RSC.
- R. D. Beger, A review of applications of metabolomics in cancer, Metabolites, 2013, 3(3), 552–574 CrossRef CAS PubMed.
- C. Denkert, J. Budczies and W. Weichert, et al., Metabolite profiling of human colon carcinoma – deregulation of TCA cycle and amino acid turnover, Mol. Cancer, 2008, 7(1), 185–189 CrossRef PubMed.
- Y. Qiu, G. Cai and M. Su, et al., Urinary metabonomic study on colorectal cancer, J. Proteome Res., 2010, 9(3), 1627–1634 CrossRef CAS PubMed.
- S. A. Ritchie, P. W. Ahiahonu and D. Jayasinghe, et al., Reduced levels of hydroxylated, polyunsaturated ultra long-chain fatty acids in the serum of colorectal cancer patients: implications for early screening and detection, BMC Med., 2010, 8, 13 CrossRef PubMed.
- C. L. Silva, M. Passos and J. S. Camara, Investigation of urinary volatile organic metabolites as potential cancer biomarkers by solid-phase
microextraction in combination with gas chromatography-mass spectrometry, Br. J. Cancer, 2011, 105(12), 1894–1904 CrossRef CAS PubMed.
- Y. Cheng, G. Xie and T. Chen, et al., Distinct urinary metabolic profile of human colorectal cancer, J. Proteome Res., 2012, 11(2), 1354–1363 CrossRef CAS PubMed.
- M. D. Williams, R. Reeves and L. S. Resar, et al., Metabolomics of colorectal cancer: past and current analytical platforms, Anal. Bioanal. Chem., 2013, 405(15), 5013–5030 CrossRef CAS PubMed.
- H. Sun, A. Zhang and X. Wang, Potential role of metabolomic approaches for Chinese medicine syndromes and herbal medicine, Phytother. Res., 2012, 26(10), 1466–1471 CAS.
- Y. N. Song, G. B. Zhang and Y. Y. Zhang, et al., Clinical Applications of Omics Technologies on ZHENG Differentiation Research in Traditional Chinese Medicine, J. Evidence-Based Complementary Altern. Med., 2013, 2013(3), 243 Search PubMed.
- Y. Liang, G. Wang and L. Xie, et al., Recent development in liquid chromatography/mass spectrometry and emerging technologies for metabolite identification, Curr. Drug Metab., 2011, 12(4), 329–344 CrossRef CAS PubMed.
- M. Zhu, H. Zhang and W. G. Humphreys, Drug metabolite profiling and identification by high-resolution mass spectrometry, J. Biol. Chem., 2011, 286(29), 25419–25425 CrossRef CAS PubMed.
- R. Cho, Y. Huang and J. C. Schwartz, et al., MS(M), an efficient workflow for metabolite identification using hybrid linear ion trap Orbitrap mass spectrometer, J. Am. Soc. Mass Spectrom., 2012, 23(5), 880–888 CrossRef CAS PubMed.
- C. Chen and S. Kim, LC-MS-based Metabolomics of Xenobiotic-induced Toxicities, Comput. Struct. Biotechnol. J., 2013, 4(4), 1–10 Search PubMed.
- M. Jiang, X. Zhao and L. Wang, et al., Integrating candidate metabolites and biochemical factors to elucidate the action mechanism of Xue-sai-tong injection based on 1 H NMR metabolomics, J. Chromatogr. B: Anal. Technol. Biomed. Life Sci., 2016, 1026, 87–96 CrossRef CAS PubMed.
- J. S. Tian, G. J. Peng and Y. F. Wu, et al., A GC-MS urinary quantitative metabolomics analysis in depressed patients treated with TCM formula of Xiaoyaosan, J. Chromatogr. B: Anal. Technol. Biomed. Life Sci., 2016, 1026, 227–235 CrossRef CAS PubMed.
- C. Jiao, Z. Jia and S. Wei, et al., Effect of a traditional Chinese medicine prescription Quzhuotongbi decoction on hyperuricemia model rats studied by using serum metabolomics based on gas chromatography–mass spectrometry, J. Chromatogr. B: Anal. Technol. Biomed. Life Sci., 2015, 1026, 272–278 Search PubMed.
- S. Yanga, T. Meng and Y. Lei, et al., Analysis of E. rutaecarpa alkaloids constituents in vitro and in vivo by UPLC-Q-TOF-MS combined with diagnostic fragment, J. Anal. Methods Chem., 2016, 2016(5), 1–10 Search PubMed.
- H. Sun, H. Wang and A. Zhang, et al., Chemical Discrimination of Cortex Phellodendri amurensis and Cortex Phellodendri chinensis by Multivariate Analysis Approach, Pharmacogn. Mag., 2016, 12(45), 41 CrossRef CAS PubMed.
- S. Li, S. Liu and Z. Pi, et al., Chemical profiling of Fufang-Xialian-Capsule by UHPLC-Q-TOF-MS and its antioxidant activity evaluated by in vitro method, J. Pharm. Biomed. Anal., 2017, 289–301 CrossRef PubMed.
- W. Feng, Q. Dong and M. Liu, et al., Screening and identification of multiple constituents and their metabolites of Zhi-zi-chi decoction in rat urine and bile by UPLC-Q-TOF-MS/MS, Biomed. Chromatogr., 2017, 31(10), 1–34 CrossRef PubMed.
- Q. Ma, C. Ma and F. Wu, et al., Preparation and structural determination of four metabolites of senkyunolide I in rats using ultra performance liquid chromatography/quadrupole-time-of-flight tandem mass and nuclear magnetic resonance spectra, BMC Complementary Altern. Med., 2016, 16(1), 504 CrossRef PubMed.
- X. Wang, X. Liu and X. Xu, et al., Screening and identification of multiple constituents and their metabolites of Fangji Huangqi Tang in rats by ultra-high performance liquid chromatography coupled with quadrupole time-of-flight tandem mass spectrometry basing on coupling data processing te, J. Chromatogr. B: Anal. Technol. Biomed. Life Sci., 2015, 985, 14–28 CrossRef CAS PubMed.
- X. N. Li, A. Zhang and H. Sun, et al., Rapid discovery of absorbed constituents and metabolites in rat plasma after the oral administration of Zi Shen Wan using high-throughput UHPLC-MS with a multivariate analysis approach, J. Sep. Sci., 2016, 39(24), 4700–4711 CrossRef CAS PubMed.
- J. Marchand, E. Martineau and Y. Guitton, et al., Multidimensional NMR approaches towards highly resolved, sensitive and high-throughput quantitative metabolomics, Curr. Opin. Biotechnol., 2016, 43, 49 CrossRef PubMed.
- K. Bingol, L. Bruschweilerli and C. Yu, et al., Metabolomics beyond spectroscopic databases: a combined MS/NMR strategy for the rapid identification of new metabolites in complex mixtures, Anal. Chem., 2015, 87(7), 3864 CrossRef CAS PubMed.
- Y. Chen, J. Zhang and L. Guo, et al., A characteristic biosignature for discrimination of gastric cancer from healthy population by high throughput GC-MS analysis, Oncotarget, 2016, 7(52), 87496–87510 Search PubMed.
- X. Xiong, X. Sheng and D. Liu, et al., A GC/MS-based metabolomic approach for reliable diagnosis of phenylketonuria, Anal. Bioanal. Chem., 2015, 407(29), 8825–8833 CrossRef CAS PubMed.
- X. M. Liu, R. Li and S. Z. Chen, et al., Screening of Inherited Metabolic Disorders in Infants with Infantile Spasms, Cell Biochem. Biophys., 2015, 72(1), 61–65 CrossRef CAS PubMed.
- L. Han, F. Han and J. Ye, et al., Spectrum analysis of common inherited metabolic diseases in Chinese patients screened and diagnosed by tandem mass spectrometry, J. Clin. Lab. Anal., 2015, 29(2), 162–168 CrossRef CAS PubMed.
- D. S. Wishart, Emerging applications of metabolomics in drug discovery and precision medicine, Nat. Rev. Drug Discovery, 2016, 15(7), 473–484 CrossRef CAS PubMed.
- M. J. Gouveia, P. J. Brindley and L. L. Santos, et al., Mass spectrometry techniques in the survey of steroid metabolites as potential disease biomarkers: a review, Metab., Clin. Exp., 2013, 62(9), 1206–1217 CrossRef CAS PubMed.
- X. Wang, I. Davis and A. Liu, et al., Improved separation and detection of picolinic acid and quinolinic acid by capillary electrophoresis-mass spectrometry: application to analysis of human cerebrospinal fluid, J. Chromatogr. A, 2013, 1316(18), 147–153 CrossRef CAS PubMed.
- M. A. Al-Ghobashy, S. A. Hassan and D. H. Abdelaziz, et al., Development and validation of LC-MS/MS assay for the simultaneous determination of methotrexate, 6-mercaptopurine and its active metabolite 6-thioguanine in plasma of children with acute lymphoblastic leukemia: Correlation with genetic polymorphism, J. Chromatogr. B: Anal. Technol. Biomed. Life Sci., 2016, 1038, 88–94 CrossRef CAS PubMed.
- G. J. Patti, O. Yanes and G. Siuzdak, Innovation: Metabolomics: the apogee of the omics trilogy, Nat. Rev. Mol. Cell Biol., 2012, 13(13), 263–269 CrossRef CAS PubMed.
- Y. Suzuki, T. Fujimori and K. Kanno, et al., Metabolome analysis of photosynthesis and the related primary metabolites in the leaves of transgenic rice plants with increased or decreased Rubisco content, Plant, Cell Environ., 2012, 35(8), 1369–1379 CrossRef CAS PubMed.
- R. Ramautar, G. W. Somsen and G. J. de Jong, CE-MS for metabolomics: developments and applications in the period 2010-2012, Electrophoresis, 2015, 32(1), 52–65 CrossRef PubMed.
|
This journal is © The Royal Society of Chemistry 2017 |
Click here to see how this site uses Cookies. View our privacy policy here.