Drivers of variability in disinfection by-product formation potential in a chain of thermally stratified drinking water reservoirs†
Received
27th October 2021
, Accepted 25th February 2022
First published on 5th April 2022
Abstract
Eutrophication, run-off and wastewater inputs to lakes have been identified as significant sources of disinfection by-product (DBPs) precursors, which are suspected carcinogens, in chlor(am)inated water. However, studies addressing the impacts of reservoirs and thermal stratification on DBP precursors are scarce. We conducted a seasonal study along a river–reservoir interconnected system, to investigate the effects of hydraulic residence time (HRT), thermal stratification, and seasonality on the levels and speciation of carbonaceous and nitrogenous DBP formation potential (FP) in source waters. Formation of 4 trihalomethanes (THMs), 4 haloacetonitriles (HANs), 2 haloketones and N-nitrosodimethylamine (NDMA) was measured on filtered lake water. Total THMs (TTHMs) FP was below 93 μg L−1, of which 59–87% of it was trichloromethane (TCM). Formation of dichloroacetonitrile (DCAN), 1,1,1-trichloropropanone (TCP), and NDMA was under 12 μg L−1, 13 μg L−1 and 73 ng L−1, respectively. The FP of the remaining DBPs was under 2 μg L−1. While the effect of depth on DBP FP was insignificant, inter-system and seasonal effects were conspicuous. The most significant variable affecting DBP formation was season, where carbonaceous DBP FP was higher in autumn and summer than in winter. TTHM FP ranged from a 160% median increase in the river upstream of the reservoirs, to a 31% median increase in the last reservoir of the system, from winter to summer. On the contrary, NDMA FP ranged from a 145% median decrease in the river upstream of the reservoirs to an 11% median decrease in the middle reservoir, from winter to summer. TTHMs FP increased from the river upstream of the reservoirs to the last reservoir of the system (40.6% median increase), whereas the opposite trend was also observed for NDMA FP (63% median decrease).
Water impact
This research presents the spatial and seasonal effects on DBP formation potential of an interconnected river-reservoir natural system. Results show that while spatial variability including depth is insignificant, seasonality is the main driver of the observed variability. In particular, carbonaceous DBP FP was higher in autumn and summer than in winter, while the opposite was observed for nitrogen containing DBPs such as NDMA.
|
1 Introduction
Chlorinating drinking water generates disinfection by-products (DBPs) that are suspected to cause cancer,1 reproductive defects,2 and respiratory problems.3 These compounds are formed from unintended reactions between disinfectants, natural dissolved organic matter (DOM) compounds such as humic and fulvic acids4 and algal organic matter,5 anthropogenic DOM from wastewater discharge6,7 and inorganic ions present in water.
In surface waters, DOM is affected by natural factors such as precipitation, droughts, microbial and photolytic processes, as well as anthropogenic factors such as land use, wastewater inputs and global warming,8 making it challenging to manage. While it is possible to control land use and wastewater discharge into drinking water sources, meteorological variables institute seasonal variability in DOM speciation and concentrations, which affects DBP formation potential (FP),9 hence it is challenging to manage.
Seasonality effects on DBP precursor compounds in lakes and reservoirs have been extensively studied and mostly allude to increased concentration of DBP FP when lakes begin warming up. For instance, a study in three reservoirs in Turkey reported an increase in dissolved organic carbon (DOC) and carbonaceous DBP reactivity in fall, with the lowest values in winter;10 a year-long study in Córdoba (Spain) reported a six fold increase in DBP FP in raw water, from spring to summer, for both carbonaceous and nitrogenous DBPs;11 In Quebec, Canada, a study reported increased FP of haloacetonitriles (HANs) and haloketones from spring to summer, followed by a drop in winter.12 Effects of summer thermal stratification on DBP FP have been reported in some studies, although far much less than studies on seasonal effects, but the results are inconclusive. For example, Bukaveckas and co-authors reported that trihalomethane (THM) FP in waters of Taylorsville was highest in the hypolimnion, in summer, compared to the epilimnion and other months, which was attributed to production of precursors from microbial degradation of organic matter.13 Similarly, a study in the Horseshoe–Bartlett reservoir in Arizona by Nguyen and co-authors reported that haloacetic acids (HAAs) FP increased with depth from summer to fall, which was attributed to photodegradation of DOC.14 Yet, a study by Kraus and co-authors on San Luis reservoir in California did not observe any trend with depth on the FP of THMs and HAAs in any season.15 Such reported variability suggests that effects of thermal stratification on DBP FP may be location-specific and thus requires system-specific studies to fully comprehend its implications.
Effects of hydraulic residence time (HRT) on DBP FP have not received much attention yet, but are necessary to be understood, given that nutrients and DOM may be retained and undergo physico-chemical and microbial transformation processes in lakes, which might influence speciation and concentration of DBP FP. Depending on HRT and in-lake processes, reservoirs may act as sources or sinks of both nutrients16,17 and organic matter,18 resulting in varied implications on downstream water uses. Hence, the main objective of this study was to investigate effects of HRT, stratification, and seasonality on the levels and speciation of DBP FP in a chain of three interconnected reservoirs in Catalunya (Spain), to understand the main drivers of DBP FP variability. With this aim, we carried out seasonal sampling campaigns in autumn, winter and summer, in the Ter River and three reservoirs (Sau, Susqueda and Pasteral) to investigate, at the laboratory scale, the FP of a suite of both carbonaceous and nitrogenous DBPs.
The initial hypotheses were (i) nitrogenous DBP FP would be higher in surface than in deep water layers due to higher density of phytoplankton in the euphotic zone in summer, (ii) DBP FP would decrease longitudinally (i.e. Ter > Sau > Susqueda > Pasteral) due to decreasing eutrophication and longer HRT, and (iii) DBP FP would be highest in summer than in other seasons as a result of increased DOM concentration from accelerated microbial activity and algal growth.
2 Methods
2.1 Study sites
Samples were collected in November 2018, February 2019 and July 2019, from Ter River (41°58′48.43′′ N, 2°18′32.79′′ E), Sau (41°58′27.11′′ N, 2°23′3.45′′ E), Susqueda (41°58′45.12′′ N, 2°31′37.99′′ E) and Pasteral (41°59′3.95′′ N, 2°36′4.28′′ E) reservoirs, which lie in the middle stretch of the 200 km long Ter River that originates from the Pyrenees mountain ranges and drains into the Mediterranean Sea (Fig. 1). There was no sampling performed in the spring season (April 2019). From Sau reservoir, water can be withdrawn from any of three available withdrawal depths and transferred to Susqueda reservoir. On the other hand, Susqueda has four withdrawal depths, from which water is alternately drawn and transferred to Pasteral reservoir for eventual abstraction, treatment, and supply to the Barcelona Metropolitan area, that serves over 4 million people. Alternate withdrawal of water from different depths is a well-known practice in reservoir management, carried out to optimize water quality by countering seasonal changes in water quality parameters at different depths.
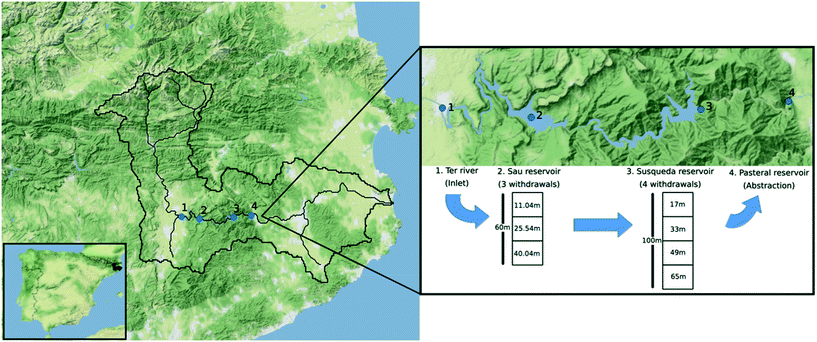 |
| Fig. 1 Map illustrating position of the Ter catchment in Spain, the four sampling locations (Ter River, Sau, Susqueda and Pasteral reservoirs) and water abstraction depths for Sau and Susqueda. | |
Sau and Susqueda reservoirs are deep systems, in which the average depth of Susqueda is twice that of Sau, but both systems have comparable HRT of approximately 3 months. In contrast, Pasteral is shallow, with a mean depth that is five times smaller than Sau and ten times shallower than Susqueda, and an HRT of 0.04 months (Table 1, Fig. 1).
Table 1 Morphometric features of Sau, Susqueda and Pasteral reservoirs
|
Max volume (hm3) |
Max depth (m) |
Surface area (ha) |
Mean water residence time (months) |
Mean depth (m) |
Altitude (masl) |
Data sources: ref. 79–82.
|
Sau |
168.5 |
65 |
570 |
3.6 |
25.2 |
425 |
Susqueda |
233 |
110 |
466 |
3.3 |
50.3 |
351 |
Pasteral |
2 |
5.7 |
35 |
0.04 |
5.7 |
185 |
2.2 Sampling procedure and preservation
Surface water samples were taken from Ter River and Pasteral reservoir by walking to the sites and collecting a grab sample, while samples from Sau and Susqueda were taken by boating to the deepest point of each reservoir to collect four samples from different depths of the water column. Ter River and Pasteral reservoir are too shallow to provide samples from different depths, hence, only surface water could be sampled. All samples for DOM and DBP FP tests were collected in 2.5 L amber glass bottles (prewashed with nitric acid, rinsed and dried in the oven), fully filled with water sample to avoid headspace at the top. On the other hand, samples for nutrients were collected in 50 ml-conical bottom polypropylene tubes (Deltalab, Spain), in sets of filtered and unfiltered samples. The tubes were rinsed with the filtered water sample (0.7 μm Whatman GF/F filter) twice before filling the tubes with samples. All surface water samples were collected by immersing bottles at about 0.5 m from the surface. Sampling depths in Sau and Susqueda reservoirs were determined by targeting locations with sharp changes in temperature, conductivity or turbidity profiles, measured by the SBE 19 plus conductivity, temperature and depth (CTD) profiler (Sea Bird Electronics, USA), resulting into a total of 4 samples collected from each reservoir. Details of depths from which samples were collected, for all the sampling events, are contained in Table 2. Sub-surface samples were collected by using a 5 L depth sampler (UWITEC, Austria), which was rinsed with one sample wash volume before collecting the final sample at each depth. To collect a sample, the sampler was lowered into the water column with the lid open until the desired depth was reached, after which the lid was automatically closed by pulling the rope. The samples were transferred to the laboratory and kept at 4 °C until the day of analysis, which was not later than two days after sampling.
Table 2 Details of depth locations from which samples were collected across all systems and sampling events
Date |
Depth code |
System/actual depth (m) |
Ter |
Sau |
Susqueda |
Pasteral |
2018-11-05 |
1 |
0.5 |
0.5 |
0.5 |
0.5 |
2019-02-04 |
1 |
0.5 |
0.5 |
0.5 |
0.5 |
2019-07-12 |
1 |
0.5 |
0.5 |
0.5 |
0.5 |
2018-11-05 |
2 |
0.5 |
15 |
20 |
0.5 |
2019-02-04 |
2 |
0.5 |
13 |
8 |
0.5 |
2019-07-12 |
2 |
0.5 |
5 |
4.5 |
0.5 |
2018-11-05 |
3 |
0.5 |
30 |
40 |
0.5 |
2019-02-04 |
3 |
0.5 |
30 |
35 |
0.5 |
2019-07-12 |
3 |
0.5 |
15 |
23 |
0.5 |
2018-11-05 |
4 |
0.5 |
40 |
70 |
0.5 |
2019-02-04 |
4 |
0.5 |
42 |
80 |
0.5 |
2019-07-12 |
4 |
0.5 |
30 |
80 |
0.5 |
2.3 Nutrient analysis
Prior to analysis, samples for nitrate, nitrite, ammonium and bromine were filtered through a Whatman 0.2 μm GFF/F filter. Bromide, nitrate, nitrite, phosphate and ammonium were analysed by ionic chromatography, on a DIONEX ICS-5000 ion chromatography system (Thermo Fisher Scientific, USA). DOC was analysed by catalytic oxidation, on a TOC-V CSH analyzer (Shimadzu, Japan). Total phosphorus samples were pre-digested, followed by colorimetric analysis19 on a UV-1800 scanning spectrophotometer (Shimadzu, Japan). Total Kjeldahl nitrogen (TKN) was analysed using the macro-Kjeldahl approach of acid digestion, distillation and eventual quantification.20
2.4 Dissolved organic matter optical properties
DOM absorbance (ultraviolet absorbance, UVA254) was measured on the 8453 UV-vis diode array spectrophotometer (Agilent Technologies, USA) and, thereafter, using the same cuvette (1 cm path length quartz) and sample, fluorescence excitation-emission spectra were measured on the F-7000 fluorescence spectrophotometer (Hitachi, Japan). Excitation wavelength ranged from 200 nm to 449 nm, spaced at 3 nm intervals, whereas emission wavelength ranged from 250 nm to 598 nm, also spaced at 3 nm intervals. Excitation and emission slit widths were set at 5 nm. The resultant absorbances, excitation and emission spectra were processed using an in-house Octave21 code to produce organic matter optical indices such as the humification index (HIX), biological index (BIX) and fluorescence index (FI), which shed light on the sources of DOM present in water. HIX, a measure of DOM maturation (indicated by the extent of aromaticity),22 is calculated as the ratio of peak areas in the emission wavelength ranges of 435–480 nm and 300–345 nm, respectively, both excited at 254 nm. Low HIX values of <4 indicate that DOM is of microbial origin; the middle range of 4–10 indicates a mixture of both humic and biological sources; whereas high values of >16 indicate that DOM is of terrestrial origin.23 On the other hand, BIX, representing the extent of in-reservoir produced DOM, is defined by fluorescence intensity ratios of emissions at 380 nm and 430 nm respectively, both excited at 310 nm.23 High BIX values of >1 suggest that DOM is, predominantly, of microbial origin, whereas low values (≤0.7) indicate low autochthonous DOM production.23 Fluorescence index, which indicates the relative contribution of allochthonous and autochthonous sources to the total DOM pool, is defined by the ratio of emissions at wavelengths of 470 nm and 520 nm respectively, both excited at 370 nm.24 Low FI values of ≤1.4 indicate that DOM is of terrestrial origin, whereas high values of ≥1.9 indicate that DOM is microbially derived.26 Milli-Q blanks, freshly produced just before analyses, were ran at the beginning and after every 10 samples, and subtracted from each sample spectra to correct for Raman scattering,25,27 and inner filter effects were corrected by subtracting UV-visible absorbance spectra from each EEM spectra.23,26,28 Specific ultraviolet absorbance (SUVA254), an indicator of DOM aromaticity,29 was obtained by dividing ultraviolet absorbance data with its corresponding DOC values.
2.5 Disinfection by-product FP tests
Formation of volatile DBPs such as trichloromethane (TCM), bromodichloromethane (BDCM), 1,1-dichloropropanone (DCP), dibromochloromethane (DBCM), 1,1,1-trichloropropanone, tribromomethane (TBM), trichloroacetonitrile (TCAN), trichloronitromethane (TCNM), dichloroacetonitrile and bromochloroacetonitrile (BCAN) was performed following a standard method previously applied by Liu and co-authors30 whereas the N-nitrosodimethylamine (NDMA) FP test followed a standard procedure previously published by Mitch and co-authors.31 A summary of analytical procedure for both classes of DBPs is provided in the ESI† Text S1.
2.6 Statistical analysis
Descriptive statistics.
The nature of systems sampled (e.g. river for which only one sample per date was collected and reservoirs where sampling at several depths was possible) implied an unbalanced sampling design in terms of the number of samples available from each system. As such, data distribution summary statistics of mean, median and standard deviation were used to describe the observed spatial trends in DBP FP and their respective yield.
Principal component analysis (PCA).
In order to explore the potential drivers of variability in DBP FP and nutrients from the measured factors of system (a proxy for HRT), depth and season, PCA was applied to a (27 × 19) data matrix comprising of 27 samples collected from Sau, Susqueda and Pasteral reservoirs and measured for nutrients, DOM optical indices, and DBP FP, across the three seasons and four different depths. The goal was to generate a two-dimensional space from a linear combination of all the measured water quality variables, where sample identities could be projected to observe the clustering pattern of DBP FP, nutrients and a combination of both nutrients and DBP FP.
Correlation analyses among the measured parameters.
Linear associations amongst nutrients, DOM optical indices, and DBP FP results were explored by applying the Spearman rank correlation coefficient test, at type I error rates of α = 0.05, α = 0.01, α = 0.001 and α = 0.0001, in order to determine if some nutrients and DOM optical indices could be used as predictive surrogates for DBP FP. To obtain the correlation coefficients and their statistical significance, the “corstars” R function (written by Guillaume T. Vallet) was applied to the standardized data. The resultant correlation coefficients were classified as either none (0), poor (0.1–0.2), fair (0.3–0.5), moderate (0.6–0.7), very strong (0.8–0.9) or perfect (1).32
All calculations and graphical illustrations were implemented in R33 version 4.40.
3 Results and discussion
3.1 General disinfection by-product FP trends
Formation potential (FP) tests were performed in all samples collected from the four systems. In general, of the six carbonaceous DBPs formed above the limit of detection, TCM formed in largest quantities (maximum of 80 μg L−1), followed by BDCM (maximum of 12.9 μg L−1) and 1,1,1-TCP (maximum of 13.0 μg L−1), while DBCM formed up to 2.5 μg L−1 (ESI† Fig. S1 and S2, Tables S1, S3, S5 and S7). Maximum FP of 1,1-DCP and TBM were 0.8 μg L−1 for both species. TCM FP recorded a maximum of about 80 μg L−1, which is similar to concentrations found in other studies in Yuqiao34 and Qingyuan reservoirs35,36 from China, and other reservoirs in Japan,37 wherein TCM FP also dominated the FP of the carbonaceous DBPs investigated. Within the nitrogen containing DBP family, DCAN FP was the dominating species, measured at a maximum concentration of 11.9 μg L−1, which is consistent with similar results reported by Wang and co-authors36 (ESI† Fig. S1 and S2, Tables S1, S3, S5 and S7). BCAN, TCAN and TCNM formed at maximum quantities of 1.5 μg L−1, 0.2 μg L−1 and 2.0 μg L−1, respectively (above the 0.1 μg L−1 detection limit, ESI† Tables S1, S3, S5 and S7). NDMA, which could be quantified in the low ng L−1 range, formed at a maximum of 72 ng L−1 (ESI† Fig. S1 and S2, Tables S1, S3, S5 and S7). In all the four systems, DCAN FP was measured between 7 and 10 times lower than TCM FP (ESI† Fig. S2, Tables S1, S3, S5 and S7), suggesting that precursor compounds responsible for the formation of carbonaceous DBPs (DOC) were in majority, compared to nitrogenous species (DON). Even though the ranges of spectroscopic indices of HIX (>3 ≤ 15), BIX (≤0.9) and FI (1.56–1.85) (ESI† Fig. S6 and S7, Tables S9–S12), suggest that DOM across the four systems was a mixture of both humic-like and microbial derived, the maxima of HIX, BIX and FI indicate that a larger proportion of DOM was humic in nature,23,38,39 that preferentially forms carbonaceous DBPs.40
3.2 Effect of the system on disinfection by-product FP
Contrary to the initial hypotheses, the median values of TTHM FP increased across the river-reservoirs chain (Fig. 1), ranging from 33 μg L−1 to 90 μg L−1. Specific TTHM also increased across the same river-reservoir continuum, particularly between Ter and Susqueda (ESI† Fig. S5). The opposite trend was hypothesized because reservoirs act as organic matter18 and nutrients16,17 traps, which would imply a reduction in concentration of DBP precursor compounds across the systems. Instead, the results suggested that increasing HRT, particularly in Sau and Susqueda reservoirs, led to the production of more carbonaceous DBP precursors. The increment in precursor concentration was probably not largely a result of additional in-reservoir production (both BIX and FI are below the minimums of 1 and 1.9 respectively, ESI† Fig. S6 and S7, Tables S9–S12) but probably from the biodegradation of terrestrial organic matter,41 with increased aromaticity and molecular weight42,43 that have a higher propensity to form carbonaceous DBPs.44 These observations were supported by an increasing trend observed in medians of UVA254, SUVA254 and HIX (ESI† Fig. S7) and suggests the vital role of HRT in promoting the microbial transformation of complex allochthonous organic matter into more simpler compounds41 which have enhanced reactivity with chlorine. On the other hand, results showed an inverse trend for nitrogenous DBP FP, particularly NDMA, that decreased from a maximum 72 ng L−1 in Ter River to a maximum of 34 ng L−1 in Pasteral (Fig. 2 and S1†). The yield of NDMA also showed similar decreasing trends (ESI† Fig. S5). This finding was consistent with the hypothesis that DBP precursors would decrease from the Ter River to Pasteral reservoir, given that eutrophication decreases across the systems, as nutrients are trapped and mineralized in reservoirs.45 Additionally, wastewater, which is a major precursor source for NDMA46 and HANs47 formation, is discharged into Ter River first and its impact is reduced along the way as the river drains into the reservoir chain. However, results showed a lack of systemic gradient for DCAN FP (median values were roughly similar, Fig. 2), suggesting DCAN precursors (free amino acids and peptides48,49) were recalcitrant, which contradicts other experimental findings,50,51 which reported that solar irradiation and biodegradation, respectively, were effective in degrading both amino acid model compounds and DCAN FP. The difference could be due to the high organic and inorganic turbidity of the investigated systems, which would undermine the effectiveness of solar irradiance. NDMA FP median values were significantly different between Ter and Sau only (median values in Sau, Susqueda and Pasteral reservoirs were roughly similar around 20 ng L−1, Fig. 2), suggesting occurrence of biodegradation of NDMA precursors51 such as secondary, tertiary and quaternary amines,52 which was also demonstrated in a microcosm experiment of Woods and co-authors.53 The sharp drop in NDMA FP between Ter River and Sau reservoir, again, highlighted the role of HRT in NDMA precursor attenuation along the same continuum. Sanchís and co-authors54 investigated NDMA FP along the Llobregat river (Spain) and found that NDMA precursors decreased probably because of natural attenuation. Nevertheless, the current disinfection practice employed at the water works abstracting water from this system is chlorination, that, although is associated with increased carbonaceous DBP formation,55 does not generate NDMA, compared to chloramination.56 Monitoring the formation of DBPs at the drinking water treatment plant (DWTP) was beyond the scope of the study, however, roughly around the winter sampling event (February 2019), Godo-Pla and co-authors investigated the formation of THMs at the same DWTP, with other scientific objectives, and found that TTHMs FP at the output of the DWTP was always below 50 μg L−1.57
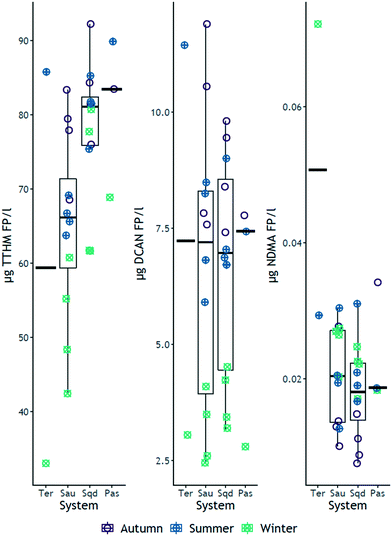 |
| Fig. 2 Box and jitter plots for DBP FPs of TTHMs, DCAN and NDMA, grouped by system and season. The green colored dots are the formation potential values in winter, the blue dots are formation potential values in summer and the violet dots represent formation potential values in autumn. The circles are the actual formation potential data. The horizontal bar on each of the four systems represents the median value for all data of that particular system. | |
3.3 Effect of reservoir depth on disinfection by-product FP
Of the four measured THMs FP, TCM and BDCM and their respective yield, showed marginal trends of increasing FP with increasing depth, mostly in winter, both in Sau and Susqueda reservoirs (ESI† Fig. S1 and S2), whereas the FP was either similar or randomly varied with increasing depth in other seasons (ESI† Fig. S1 and S2). TCP FP and its yield were mostly constant with increasing depth (ESI† Fig. S1 and S2). These findings were unexpected as the initial hypothesis, which is supported by depth trends observed in DOC, UVA254 and HIX (ESI† Fig. S6), predicted a gradient of increasing FP with increasing depth in summer, probably due to photodegradation of precursors in the euphotic zone, and anoxic biodegradation of complex organic matter in the deeper layers in stratified periods13,58 but not in winter when the reservoirs are fully mixed. For nitrogenous species, DCAN FP and its yield increased with increasing depth in autumn, in both reservoirs; remained roughly the same in winter; and decreased with increasing depth in summer (ESI† Fig. S1 and S2), in Sau reservoir only, which was supported by the depth trends observed in BIX in winter and summer (ESI† Fig. S6). Also, in Sau reservoir, NDMA FP decreased from the surface to the metalimnion and remained constant up to the bottom layers in autumn; remained roughly constant in winter; and increased with increasing depth in summer (ESI† Fig. S1), which was supported by SUVA254 (autumn) and BIX depth trends in autumn and winter only (ESI† Fig. S6). On the other hand, in Susqueda reservoir, NDMA FP remained constant with increasing depth in autumn and winter but decreased from the surface to the metalimnion and remained constant up to the bottom water layers in summer (ESI† Fig. S1), which was supported by the BIX depth trend (ESI† Fig. S6), whereas its yield was constant in both reservoirs and across all seasons (ESI† Fig. S2). The initial hypothesis had anticipated a decreasing FP of both DCAN and NDMA, in both reservoirs, with increasing depth in autumn and summer because of the proliferation of phytoplankton in the euphotic zone, which could have contributed more precursors in the upper water layers, as suggested by depth trend observed in BIX (ESI† Fig. S6). Elsewhere in California, Gerecke and Sedlak reported higher NDMA FP in the epilimnion than the hypolimnion of the San Pablo and San Leandro reservoirs, during stratification, which was suspected to originate from atmospheric deposition or photo-transformation of organic matter from the feeding streams.59 The reverse summer trend observed in Sau, in this study, might be due to increased concentration of precursor from anoxic degradation of the sinking particulate organic matter from phytoplankton in the euphotic zone. Overall, the observed marginal depth trends are, in our opinion, minor to have meaningful implications for depth withdrawal management, since the effect of depth on DBP formation was small and not statistically significant.
3.4 Effect of season on disinfection by-product FP
As expected, the FP of TCM and BDCM and their associated yield, were highest in autumn and summer and lowest in winter in all reservoirs (ESI† Fig. S1–S4, Tables S3–S8). The observed autumn increase in carbonaceous DBP FP may be a result of increased DOC concentrations from precipitation meditated terrestrially derived DOM,10 and the increase of DBP FP in summer might be due to higher concentration of organic matter compounds from augmented microbial activity in warmer environments60 and some contribution from autochthonous derived precursor compounds,49,61 both of which are supported by the measured trends in DOC and SUVA254 (autumn, ESI† Fig. S7). On the other hand, TCP FP and its yield did not show any seasonal differences (ESI† Fig. S1–S4, Tables S3–S8). Considering nitrogenous DBPs, the FP and yield for DCAN was also highest in autumn and summer, and lowest in winter, in all the four systems (Fig. 2 and S1–S4†), which could be attributed to increased input of wastewater derived precursors (in autumn high flows), contribution from algal sources in summer,62 as supported by the summer BIX trends and SUVA254 (autumn) trend (Sau and Susqueda, ESI† Fig. S6 and S7) and some contribution from terrestrially derived DOM.63,64 NDMA FP was highest in summer followed by winter and lowest in autumn in both Sau and Susqueda reservoirs (supported by BIX trend in ESI† Fig. S6 and S7); highest in winter and lowest in summer in the Ter River; and highest in autumn, lowest in winter and summer in Pasteral reservoir (Fig. 2 and S1†). In contrast, the yield of NDMA was constant across all seasons, in almost all the systems, except in Pasteral where it was highest in autumn and lowest in winter (ESI† Fig. S2 and S4). The absence of seasonal variations in the yield of NDMA in most of the systems might be due to a lack of relationship between DOC and NDMA. Initial hypotheses had anticipated highest carbonaceous DBP FP in all the systems in autumn due to mobilization of precursors from the catchment (supported by trends in TCM and BDCM FP, ESI† Fig. S1 and S2), as was also reported elsewhere,65 and the highest nitrogenous DBP FP in summer (due to phytoplankton growth66) and autumn (due to increased wastewater inputs67), followed by the lowest FP in winter (due to reservoir turn-over). DCAN FP (in all systems) and, to some extent, NDMA FP (Sau and Susqueda, in summer only) confirmed our expectations (ESI† Fig. S1 and S2). However, high NDMA FP in winter in the Ter, Sau and Susqueda, and lowest values recorded in autumn were unexpected, even though, elsewhere in Korea and Japan, similar findings were reported,68,69 which was attributed to lower biological and photodegradation of precursor compounds due to reduced microbial activity and temperature in winter. Elsewhere in China, Zhou and co-authors60 reported that nitrogenous DBPs in treated water from a closed reservoir on Yangtze River, recorded lowest concentrations in autumn and winter, which was attributed to lowest concentration of precursors in that season. The difference might be related to trophic state and susceptibility to anthropogenic pollution, where the systems investigated in this study (which are usually eutrophic and inundated with wastewater) maintain levels of precursors throughout winter, hence form high nitrogenous DBPs, whereas the Yangtze reservoir is remotely placed and secure from anthropogenic pollution.
In lake systems, high temperature promotes nitrification in the upper layers of the water column, which would lead to depletion of NH3−/NH4+ and a concomitant increase in NO3−; and denitrification in anoxic bottom water layers, which leads to depletion of NO3− and an increase in NH3−/NH4+.70 Also, high water temperature enhances both algal growth rate71 and microbial degradation of dissolved organic matter,72 which, ideally, would lead to depletion of NH3−/NH4+ first, followed by NO3−, as these nutrients are essential in algal metabolism, in the process of in-reservoir dissolved organic matter production and microbial degradation of dissolved organic matter.73,74 Consequently, upper water layers would experience: increased dissolved organic matter concentration from phytoplankton activity; reduced NH3−/NH4+ due to conversion into NO3− and assimilation by phytoplankton in the presence of sunlight and higher temperature. Given that autochthonous dissolved organic matter has been reported to form nitrogenous DBPs,75 one would expect an increase in their formation potential in summer. In the systems under this study (particularly in Sau and Susqueda), NDMA formed the highest concentrations in summer, whereas DCAN recorded highest concentrations in autumn (ESI† Fig. S3), implying that the effect of high summer temperature does not apply to every nitrogenous DBP, as other classes may be influenced by other factors such as hydrology.
3.5 Exploration of drivers of disinfection by-product FP
PCA biplots for the reservoirs DBP FP and their respective yield showed that the two-dimensional projection accounted for a total of 74% of variability in the original dataset, with a clear clustering pattern driven by season (ESI† Fig. S8). Within each season, there was not any other observable clustering pattern, implying that seasonality was the main driver of variability of DBP formation potential, as already described earlier. However, the winter cluster for both FP and yield data showed clear sub-clusters based on system and reservoir depth (ESI† Fig. S8 and S9). Furthermore, the seasonal clustering patterns revealed that TCM FP contributed the only variability appearing in autumn, BDCM FP contributed the most variability in summer and TCP FP contributed most of the variability in winter.
When nutrients were combined with DBP FP data, PCA returned a biplot which accounted for a total of 56% of variability in the original dataset. Although the total explained variance is not super high, the model fit is reasonable because it is above the 50% that other researchers consider as a minimum.76 Just like in the previous case, seasonality was the main driver in the clustering patterns (Fig. 3), in which the winter cluster for both FP and yield data lost the sub-clusters based on system and depth (ESI† Fig. S8 and S9). Most of the summer variability was driven by nitrite, the biological index and BDCM FP, whereas the winter cluster was mostly driven by nitrate, total nitrogen and the HIX (Fig. 3). Other than the correlations suggested by pointing in the same direction between nitrite, BIX and BDCM FP, most of the DBP FP and nutrients pointed in different directions on the combined biplot, suggesting a lack of correlation. The sub-clusters observed within the winter cluster of the yield PCA suggest that the influence of HRT and depth were significant on DOM reactivity towards DBP formation.
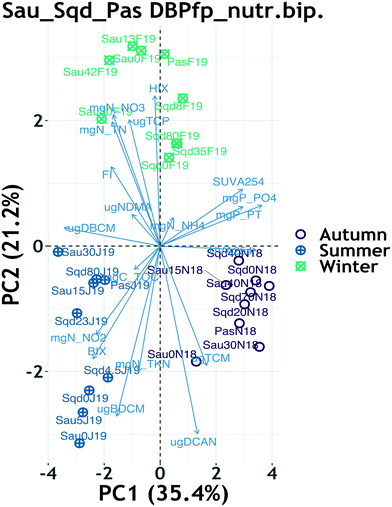 |
| Fig. 3 Principal component analysis biplot for DBP FP + nutrients, excluding a Ter River sample, illustrating clustering by season. Violet color represents samples collected in autumn, green color represents samples collected in winter, whereas the blue color represents samples collected in summer. The blue labeled arrows represent the projection of the measured nutrients, DOM optical indices and DBP FPs on the two-dimensional plots. Samples were coded as: the first 3 letters are an abbreviation of the location (Sau = Sau, Sqd = Susqueda, Pas = Pasteral), followed by depth (m) from which the sample was collected (e.g. 0 = surface, 40…80 m) and, finally, an abbreviation of sampling month and year (e.g. N18 = November 2018, F19 = February 2019, J19 = July 2019). | |
When the Ter sample was included in the PCAs, seasonality patterns were retained in both the FP and yield data (ESI† Fig. S10 and S11), but depth patterns were marginally visible in the FP PCA only, particularly on Sau reservoir data (ESI† Fig. S10) and not in the yield PCA (Fig. S11†).
3.6 Correlation analyses between evaluated parameters
TCM FP and its yield showed positive correlation with UVA254 (fair & fair, r = 0.53, r = 0.56), DOC (fair, r = 0.37) and PO43− (fair & fair, r = 0.40, r = 0.58), whereas the rest of nutrients and other DOM optical indices negatively correlated with TCM as: TN (fair & fair, r = −0.56, r = −0.59) and NO3− (fair & fair, r = −0.59, r = −0.57) (Tables 3 and S13†). In addition, the yield of TCM positively correlated with SUVA254 (moderate, r = 0.60) and TP (fair, r = 0.48), and negatively correlated with BIX (fair, r = −0.59) and FI (fair, r = −0.52) (ESI† Table S13). The significant correlation observed between UVA254 and TCM FP agreed with other findings,77,78 although, in their studies, the correlations were stronger (r = 0.91, p < 0.01), and r = 0.897, p < 0.001, respectively)). On the other hand, BDCM FP positively correlated with DOC (fair, r = 0.54), NO2− (moderate r = 0.68), TKN (fair, r = 0.38) and BIX (fair, r = 0.45) and negatively with PO43− (fair, r = −0.49), TP (fair, r = −0.49), SUVA254 (fair, r = −0.54) and NH4+ (fair, r = −0.52) (Table 3). In addition, the yield of BDCM positively correlated with NO2− (fair r = 0.48) and negatively correlated with DOC (fair, r = −0.44) and HIX (fair, r = −0.46). Correlations with DOC also agreed with findings of Watson and co-authors,78 although, in their study, the correlation was very strong (r = 0.86, p < 0.01). However, the lack of significant correlation between BDCM FP and UVA254, and the negative correlation with SUVA254 were contrary to the findings of Watson and co-authors78 who reported very strong associations (r = 0.90, r = 0.80, p < 0.01, respectively). Probably, the differences might relate to the fact that they were dealing with a one-off analysis of synthetic water, whereas the presently investigated samples were collected from natural systems, over a couple of seasons. TCP FP negatively correlated with PO43− (fair, r = −0.45) and UVA254 (fair, r = −0.50), whereas its yield positively correlated with NO3− (fair, r = 0.45) and negatively correlated with DOC (fair, r = −0.59) and UVA254 (fair, r = −0.49) (ESI† Table S13). Overall, the observed correlations between carbonaceous DBP FP, its associated yield and nutrients appeared less strong to consider nutrients as predictive surrogates for the FP of TCM, BDCM and TCP.
Table 3 Nutrients-DOM optical indices-DBP FP Spearman rank correlation matrix
|
mgN_NH4+ |
mgC_DOC |
mgN_TN |
mgN_TKN |
mgP_PO43− |
mgP_PT |
mgN_NO3− |
mgN_NO2− |
UVA254 |
SUVA254 |
FI |
BIX |
HIX |
μgTCM |
μgBDCM |
μgDCAN |
μgDBCM |
μgTCP |
μgNDMA |
Note: **** = p < 0.0001, *** = p < 0.001, ** = p < 0.01, * = p < 0.05.
|
mgN_NH4+ |
1 |
|
|
|
|
|
|
|
|
|
|
|
|
|
|
|
|
|
|
mgC_DOC |
−0.54** |
1 |
|
|
|
|
|
|
|
|
|
|
|
|
|
|
|
|
|
mgN_TN |
0.27 |
−0.12 |
1 |
|
|
|
|
|
|
|
|
|
|
|
|
|
|
|
|
mgN_TKN |
−0.38* |
0.16 |
−0.35 |
1 |
|
|
|
|
|
|
|
|
|
|
|
|
|
|
|
mgP_PO43− |
0.43* |
−0.21 |
−0.39* |
−0.28 |
1 |
|
|
|
|
|
|
|
|
|
|
|
|
|
|
mgP_PT |
0.51** |
−0.24 |
−0.36 |
−0.18 |
0.87**** |
1 |
|
|
|
|
|
|
|
|
|
|
|
|
|
mgN_NO3− |
0.27 |
−0.17 |
0.97**** |
−0.35 |
−0.37* |
−0.32 |
1 |
|
|
|
|
|
|
|
|
|
|
|
|
mgN_NO2− |
−0.12 |
0.26 |
0.2 |
0.32 |
−0.62*** |
−0.35 |
0.13 |
1 |
|
|
|
|
|
|
|
|
|
|
|
UVA254 |
0.23 |
0.06 |
−0.3 |
−0.3 |
0.87**** |
0.61*** |
−0.3 |
−0.64*** |
1 |
|
|
|
|
|
|
|
|
|
|
SUVA254 |
0.55** |
−0.18 |
−0.15 |
−0.42* |
0.79**** |
0.61*** |
−0.16 |
−0.57** |
0.79**** |
1 |
|
|
|
|
|
|
|
|
|
FI |
−0.03 |
0.14 |
0.49** |
−0.1 |
−0.53** |
−0.33 |
0.49** |
0.38* |
−0.54** |
−0.41* |
1 |
|
|
|
|
|
|
|
|
BIX |
−0.26 |
0.04 |
0.2 |
0.43* |
−0.66**** |
−0.52** |
0.16 |
0.57** |
−0.74**** |
−0.71**** |
0.17 |
1 |
|
|
|
|
|
|
|
HIX |
−0.06 |
0.11 |
0.37* |
−0.31 |
−0.03 |
−0.09 |
0.40* |
−0.28 |
0.16 |
0.13 |
0.34 |
−0.58** |
1 |
|
|
|
|
|
|
μgTCM |
−0.22 |
0.37* |
−0.56** |
0.16 |
0.40* |
0.28 |
−0.59*** |
−0.11 |
0.53** |
0.19 |
−0.3 |
−0.35 |
−0.06 |
1 |
|
|
|
|
|
μgBDCM |
−0.52** |
0.54** |
−0.07 |
0.38* |
−0.49** |
−0.49** |
−0.16 |
0.68**** |
−0.31 |
−0.54** |
0.09 |
0.45* |
−0.24 |
0.41* |
1 |
|
|
|
|
μgDCAN |
−0.1 |
0.41* |
−0.57** |
0.28 |
0.3 |
0.29 |
−0.67**** |
0.26 |
0.31 |
0.1 |
−0.37* |
0.02 |
−0.50** |
0.74**** |
0.54** |
1 |
|
|
|
μgDBCM |
−0.1 |
0.02 |
0.58*** |
0.15 |
−0.87**** |
−0.67**** |
0.56** |
0.72**** |
−0.88**** |
−0.70**** |
0.60*** |
0.69**** |
−0.03 |
−0.54** |
0.40* |
−0.33 |
1 |
|
|
μgTCP |
−0.14 |
−0.05 |
0.2 |
−0.01 |
−0.45* |
−0.25 |
0.3 |
0.09 |
−0.50** |
−0.33 |
0.33 |
0.19 |
0.33 |
−0.41* |
−0.19 |
−0.56** |
0.42* |
1 |
|
μgNDMA |
−0.01 |
−0.31 |
0.36 |
0.09 |
−0.37* |
−0.26 |
0.36 |
0.18 |
−0.44* |
−0.37* |
0.40* |
0.35 |
0 |
−0.25 |
−0.02 |
−0.43* |
0.49** |
0.32 |
1 |
For nitrogenous DBP FP, DCAN FP and its yield positively correlated with DOC (fair, r = 0.41) and negatively correlated with TN (fair & fair, r = −0.57, r = −0.48), NO3− (moderate & fair, r = −0.67, r = −0.58), FI (fair & fair, r = −0.37, r = −0.39) and HIX (fair & moderate, r = −0.50, r = −0.66) (Tables 3 and S13†), implying that none of the measured nutrients and other DOM optical indices could be used as acceptable surrogates to predict DCAN FP. The observed correlation of DCAN FP with DOC agreed with findings of Watson and co-authors78 although their study reported a very strong association (r = 0.80, p < 0.01) plus other strong associations with UVA254 (r = 0.90, p < 0.01) and SUVA254 (r = 0.83, p < 0.01), both of which were absent in this study. On the other hand, NDMA FP positively correlated with the DOM optical index of FI (fair, = 0.40) and negatively correlated with PO43− (fair, r = −0.37), UVA254 (fair, r = −0.44) and SUVA254 (fair, r = −0.37) (Table 3). In addition, the yield of NDMA positively correlated with NO3− (fair, r = 0.45) and TN (fair, r = 0.44) and negatively correlated with DOC (fair, r = −0.55) and UVA254 (fair, r = −0.39). While the present study did not find significant correlations between NDMA FP and DOC, in a USA study, Yang and co-authors77 reported fair and positive correlations for both DOC (r = 0.535, p < 0.001) and UVA254 (r = 0.417, p < 0.001). Instead, in the present study, NDMA FP negatively correlated with UVA254 (fair, r = −0.44) and SUVA254 (fair, r = −0.37), whereas its yield negatively correlated with DOC (fair, r = −0.55) and UVA254 (fair, r = −0.39) (ESI† Table S13). The difference might relate to differences in sampling seasons, as they did not sample in winter.
Associations amongst DBP FP were also explored to identify which species could be used as surrogates for other DBP FP. Results indicated that BDCM FP positively correlated with TCM FP (fair, r = 0.41) (Table 3), which agrees with findings of Watson and co-authors78 (r = 0.92, p < 0.01); TCP FP negatively correlated with DCAN FP (fair, r = −0.56) and TCM FP (fair, r = −0.41) (Table 3). DCAN FP positively correlated with both TCM FP (moderate, r = 0.74) (Table 2), agreeing with findings of Watson and co-authors78 (r = 0.96, p < 0.01) and BDCM (fair, r = 0.54), which also agrees with findings of Watson and co-authors78 (r = 0.89, p < 0.01). NDMA FP negatively correlated with DCAN FP (fair, r = −0.43) (Table 3). The yield of TCM positively correlated with the yield of DCAN (fair, r = 0.57), as did the yield of BDCM (fair, r = 0.54) (ESI† Table S13). The yield of TCP positively correlated with the yield of NDMA (moderate, r = 0.67).The yield of DCAN negatively correlated with the yields of TCP (moderate, r = −0.59) and NDMA (fair, r = −0.43) (ESI† Table S13). These associations suggest that DCAN FP can be expected when THM FP is present but can't be considered suitable proxies for each other.
4 Conclusions
The effect of HRT, depth and season on concentration and speciation of carbonaceous DBP and nitrogenous DBP FP in stratified lakes was investigated. When the three studied factors were considered in isolation, the FP of carbonaceous DBPs increased from Ter across to Pasteral reservoir, while FP of the nitrogenous DBPs (particularly NDMA) decreased across the same spatial scale. However, when the Ter sample (which behaved as an outlier) was not considered, spatial variability was insignificant, as the results seemed to cluster by season, meaning seasonality was the main driver of the observed variability. In particular, carbonaceous DBP FP was higher in autumn and summer than in winter, while the opposite was observed for nitrogen containing DBPs such as NDMA. Additionally, depth was not a significant driver of variability in the FP of DBPs studied.
This study adds a dimension of HRT, depth, and stratification effects on speciation of DBP formation potential, which have been less studied and extends the knowledge base of a few studies that have tackled the influence of seasonality on DBP FP in lakes and reservoirs.
Conflicts of interest
There are no conflicts to declare.
Acknowledgements
This project has received funding from the European Union's Horizon 2020 research and Innovation Programme under the Marie Skłodowska-Curie grant agreement No 722518. The authors thank Generalitat de Catalunya through Consolidated Research Groups ICRA_ENV 2017SGR1124 and ICRA_Tech 2017SGR1318. ICRA researchers thank funding from the CERCA program. MJF acknowledges her Ramón y Cajal fellowship (RyC-2015-17108), from the AEI-MICIU. The authors also wish to acknowledge the invaluable support rendered to them by the laboratory staff at Catalan Institute for Water Research, during the sampling and processing of the samples that culminated into this article.
References
- I. Evlampidou, L. Font-Ribera, D. Rojas-Rueda, E. Gracia-Lavedan, N. Costet, N. Pearce, P. Vineis, J. J. K. Jaakkola, F. Delloye, K. C. Makris, E. G. Stephanou, S. Kargaki, F. Kozisek, T. Sigsgaard, B. Hansen, J. Schullehner, R. Nahkur, C. Galey, C. Zwiener, M. Vargha, E. Righi, G. Aggazzotti, G. Kalnina, R. Grazuleviciene, K. Polanska, D. Gubkova, K. Bitenc, E. H. Goslan, M. Kogevinas and C. M. Villanueva, Trihalomethanes in Drinking Water and Bladder Cancer Burden in the European Union, Environ. Health Perspect., 2020, 128, 017001 CrossRef PubMed.
- J. A. Kaufman, J. M. Wright, A. Evans, Z. Rivera-Núñez, A. Meyer and M. G. Narotsky, Associations Between Disinfection By-Product Exposures and Craniofacial Birth Defects, J. Occup. Environ. Med., 2018, 60, 109–119 CrossRef CAS PubMed.
- A. Florentin, A. Hautemanière and P. Hartemann, Health effects of disinfection by-products in chlorinated swimming pools, Int. J. Hyg. Environ. Health, 2011, 214, 461–469 CrossRef CAS PubMed.
- M. Sillanpää, M. C. Ncibi, A. Matilainen and M. Vepsäläinen, Removal of natural organic matter in drinking water treatment by coagulation: A comprehensive review, Chemosphere, 2018, 190, 54–71 CrossRef PubMed.
- Y. Tang, X. Long, M. Wu, S. Yang, N. Gao, B. Xu and S. Dutta, Bibliometric review of research trends on disinfection by-products in drinking water during 1975–2018, Sep. Purif. Technol., 2020, 241, 116741 CrossRef CAS.
- C. Postigo and S. D. Richardson, Transformation of pharmaceuticals during oxidation/disinfection processes in drinking water treatment, J. Hazard. Mater., 2014, 279, 461–475 CrossRef CAS PubMed.
- C. Postigo, R. Gil-Solsona, M. F. Herrera-Batista, P. Gago-Ferrero, N. Alygizakis, L. Ahrens and K. Wiberg, A step forward in the detection of byproducts of anthropogenic organic micropollutants in chlorinated water, Trends Environ. Anal. Chem., 2021, 32, e00148 CrossRef CAS.
- N. B. Weston, W. P. Porubsky, V. A. Samarkin, M. Erickson, S. E. Macavoy and S. B. Joye, Porewater Stoichiometry of Terminal Metabolic Products, Sulfate, and Dissolved Organic Carbon and Nitrogen in Estuarine Intertidal Creek-bank Sediments, Biogeochemistry, 2006, 77, 375–408 CrossRef CAS.
- M. Valdivia-Garcia, P. Weir, Z. Frogbrook, D. W. Graham and D. Werner, Climatic, Geographic and Operational Determinants of Trihalomethanes (THMs) in Drinking Water Systems, Sci. Rep., 2016, 6, 35027 CrossRef CAS PubMed.
- V. Uyak, K. Ozdemir and I. Toroz, Seasonal variations of disinfection by-product precursors profile and their removal through surface water treatment plants, Sci. Total Environ., 2008, 390, 417–424 CrossRef CAS PubMed.
- M. Serrano, I. Montesinos, M. J. Cardador, M. Silva and M. Gallego, Seasonal evaluation of the presence of 46 disinfection by-products throughout a drinking water treatment plant, Sci. Total Environ., 2015, 517, 246–258 CrossRef CAS PubMed.
- G. Chhipi-Shrestha, M. Rodriguez and R. Sadiq, Unregulated disinfection By-products in drinking water in Quebec: A meta analysis, J. Environ. Manage., 2018, 223, 984–1000 CrossRef CAS PubMed.
- P. A. Bukaveckas, D. McGaha, J. M. Shostell, R. Schultz and J. D. Jack, Internal and external sources of THM precursors in a midwestern reservoir, J. – Am. Water Works Assoc., 2007, 99, 127–136 CrossRef CAS.
- M. L. Nguyen, L. A. Baker and P. Westerhoff, DOC and DBP precursors in western US watersheds and reservoirs, J. – Am. Water Works Assoc., 2002, 94, 98–112 CrossRef CAS.
- T. E. C. Kraus, B. A. Bergamaschi, P. J. Hernes, D. Doctor, C. Kendall, B. D. Downing and R. F. Losee, How reservoirs alter drinking water quality: Organic matter sources, sinks, and transformations, Lake Reservoir Manage., 2011, 27, 205–219 CrossRef CAS.
- X. Ran, A. F. Bouwman, Z. Yu and J. Liu, Implications of eutrophication for biogeochemical processes in the Three Gorges Reservoir, China, Reg. Environ. Change, 2019, 19, 55–63 CrossRef.
- C. Teodoru and B. Wehrli, Retention of sediments and nutrients in the Iron Gate I Reservoir on the Danube River, Biogeochemistry, 2005, 76, 539–565 CrossRef CAS.
- S. J. Parks and L. A. Baker, Sources and transport of organic carbon in an Arizona river-reservoir system, Water Res., 1997, 31, 1751–1759 CrossRef CAS.
- S. H. Jenkins, Standard Methods for the Examination of Water and Wastewater, Water Res., 1982, 16, 1495–1496 CrossRef.
-
R. Baird and L. Bridgewater, Standard methods for the examination of water and wastewater, American Public Health Association, Washington, D.C., 23rd edn, 2017 Search PubMed.
-
J. W. Eaton, D. Bateman, S. Hauberg and R. Wehbring, GNU Octave version 3.8.1 manual: a high-level interactive language for numerical computations, CreateSpace Independent Publishing Platform, 2014, ISBN 1441413006, URL http://www.gnu.org/software/octave/doc/interpreter/ Search PubMed.
- M. Tedetti, P. Cuet, C. Guigue and M. Goutx, Characterization of dissolved organic matter in a coral reef ecosystem subjected to anthropogenic pressures (La Réunion Island, Indian Ocean) using multi-dimensional fluorescence spectroscopy, Sci. Total Environ., 2011, 409, 2198–2210 CrossRef CAS PubMed.
- A. Huguet, L. Vacher, S. Relexans, S. Saubusse, J. M. Froidefond and E. Parlanti, Properties of fluorescent dissolved organic matter in the Gironde Estuary, Org. Geochem., 2009, 40, 706–719 CrossRef CAS.
- J. P. Casas-Ruiz, J. Tittel, D. von Schiller, N. Catalán, B. Obrador, L. Gómez-Gener, E. Zwirnmann, S. Sabater and R. Marcé, Drought-induced discontinuities in the source and degradation of dissolved organic matter in a Mediterranean river, Biogeochemistry, 2016, 127, 125–139 CrossRef CAS.
-
A. M. Hansen, T. E. C. Kraus, B. A. Pellerin, J. A. Fleck, B. D. Downing and B. A. Bergamaschi, Optical properties of dissolved organic matter (DOM): Effects of biological and photolytic degradation, 2016, pp. 1015–1032 Search PubMed.
- J. E. Birdwell and A. S. Engel, Characterization of dissolved organic matter in cave and spring waters using UV–Vis absorbance and fluorescence spectroscopy, Org. Geochem., 2010, 41, 270–280 CrossRef CAS.
-
C. A. Stedmon and R. Bro, Oceanography: Methods Characterizing dissolved organic matter fluorescence with parallel factor analysis: a tutorial, 2008, pp. 572–579 Search PubMed.
- D. N. Kothawala, K. R. Murphy, C. A. Stedmon, G. A. Weyhenmeyer and L. J. Tranvik, Inner filter correction of dissolved organic matter fluorescence, Limnol. Oceanogr.: Methods, 2013, 11, 616–630 CrossRef.
- S. S. Marais, E. J. Ncube, T. A. M. Msagati, B. B. Mamba and T. T. I. Nkambule, Assessment of trihalomethane (THM) precursors using specific ultraviolet absorbance (SUVA) and molecular size distribution (MSD), J. Water Process. Eng., 2019, 27, 143–151 CrossRef.
- P. Liu, M. J. Farré, J. Keller and W. Gernjak, Reducing natural organic matter and disinfection by-product precursors by alternating oxic and anoxic conditions during engineered short residence time riverbank filtration: A laboratory-scale column study, Sci. Total Environ., 2016, 565, 616–625 CrossRef CAS PubMed.
- W. A. Mitch, A. C. Gerecke and D. L. Sedlak, A N-Nitrosodimethylamine (NDMA) precursor analysis for chlorination of water and wastewater, Water Res., 2003, 37, 3733–3741 CrossRef CAS.
- H. Akoglu, User's guide to correlation coefficients, Turk. J. Emerg. Med., 2018, 18, 91–93 CrossRef PubMed.
-
R. CoreTeam, R: A Language and Environment for Statistical Computing, 2017, vol. 2 Search PubMed.
- H. Y. Zhai, X. Z. He, Y. Zhang, T. T. Du, A. S. Adeleye and Y. Li, Disinfection byproduct formation in drinking water sources: A case study of Yuqiao reservoir, Chemosphere, 2017, 181, 224–231 CrossRef CAS PubMed.
- F. Wang, B. Gao, D. Ma, Q. Yue, R. Li and Q. Wang, Reduction of disinfection by-product precursors in reservoir water by coagulation and ultrafiltration, Environ. Sci. Pollut. Res., 2016, 23, 22914–22923 CrossRef CAS PubMed.
- F. Wang, B. Y. Gao, Q. Y. Yue, F. Bu and X. Shen, Effects of ozonation, powdered activated carbon adsorption, and coagulation on the removal of disinfection by-product precursors in reservoir water, Environ. Sci. Pollut. Res., 2017, 24, 17945–17954 CrossRef CAS PubMed.
- H. Sakai, S. Tokuhara, M. Murakami, K. Kosaka, K. Oguma and S. Takizawa, Comparison of chlorination and chloramination in carbonaceous and nitrogenous disinfection byproduct formation potentials with prolonged contact time, Water Res., 2016, 88, 661–670 CrossRef CAS PubMed.
- S. Inamdar, N. Finger, S. Singh, M. Mitchell, D. Levia, H. Bais, D. Scott and P. McHale, Dissolved organic matter (DOM) concentration and quality in a forested mid-Atlantic watershed, USA, Biogeochemistry, 2012, 108, 55–76 CrossRef CAS.
- J. Zhang, T. Maqbool, Y. Qiu, Y. Qin, M. B. Asif, C. Chen and Z. Zhang, Determining the leading sources of N-nitrosamines and dissolved organic matter in four reservoirs in Southern China, Sci. Total Environ., 2021, 771, 145409–145409 CrossRef CAS PubMed.
- C. Fang, X. Yang, S. Ding, X. Luan, R. Xiao, Z. Du, P. Wang, W. An and W. Chu, Characterization of Dissolved Organic Matter and Its Derived Disinfection Byproduct Formation along the Yangtze River, Environ. Sci. Technol., 2021, 55, 12326–12336 CrossRef CAS PubMed.
- A. T. Chow, A. T. O'Geen, R. A. Dahlgren, F. J. Díaz, K.-H. Wong and P.-K. Wong, Reactivity of Litter Leachates from California Oak Woodlands in the Formation of Disinfection By-Products, J. Environ. Qual., 2011, 40, 1607–1616 CrossRef CAS PubMed.
- J. F. Hunt and T. Ohno, Characterization of fresh and decomposed dissolved organic matter using excitation-emission matrix fluorescence spectroscopy and multiway analysis, J. Agric. Food Chem., 2007, 55, 2121–2128 CrossRef CAS PubMed.
- Q. Li, X. Guo, L. Chen, Y. Li, D. Yuan, B. Dai and S. Wang, Investigating the spectral characteristic and humification degree of dissolved organic matter in saline-alkali soil using spectroscopic techniques, Front. Earth Sci., 2017, 11, 76–84 CrossRef CAS.
- C. Y. Hu, H. Z. Zhu, Y. L. Lin, T. Y. Zhang, F. Zhang and B. Xu, Dissolved organic matter fractions and disinfection by-product formation potential from major raw waters in the water-receiving areas of south-to-north water diversion project, China, Desalin. Water Treat., 2015, 56, 1689–1697 CrossRef CAS.
- G. Jossette, B. Leporcq, N. Sanchez and X. Philippon, Biogeochemical mass-balances (C, N, P, Si) in three large reservoirs of the Seine Basin (France), Biogeochemistry, 1999, 47, 119–146 CrossRef CAS.
- S. W. Krasner, The formation and control of emerging disinfection by-products of health concern, Philos. Trans. R. Soc., A, 2009, 367, 4077–4095 CrossRef CAS PubMed.
- T. Bond, Precursors of nitrogenous disinfection by-products in drinking water––A critical review and analysis, J. Hazard. Mater., 2012, 16 Search PubMed.
-
W. Chu, N. Gao, Y. Deng and X. Li, in Recent Advances in Disinfection by-Products, ed. T. Karanfil, B. Mitch, P. Westerhoff and Y. Xie, Amer Chemical Soc, Washington, 2015, vol. 1190, pp. 307–339 Search PubMed.
- W. Chu, D. Yao, Y. Deng, M. Sui and N. Gao, Production of trihalomethanes, haloacetaldehydes and haloacetonitriles
during chlorination of microcystin-LR and impacts of pre-oxidation on their formation, J. Hazard. Mater., 2017, 327, 153–160 CrossRef CAS PubMed.
- W. Qian-Yuan, L. Chao, D. Ye, W. Wen-Long, H. Huang and H. Hong-Ying, Elimination of disinfection byproduct formation potential in reclaimed water during solar light irradiation, Water Res., 2016, 95, 260–267 CrossRef PubMed.
- R. Zhang, F. Wang, W. Chu, C. Fang, H. Wang, M. Hou, R. Xiao and G. Ji, Microbial degradation of typical amino acids and its impact on the formation of trihalomethanes, haloacetonitriles and haloacetamides during chlor(am)ination, Water Res., 2019, 159, 55–64 CrossRef CAS PubMed.
- S. W. Krasner, W. A. Mitch, D. L. McCurry, D. Hanigan and P. Westerhoff, Formation, precursors, control, and occurrence of nitrosamines in drinking water: A review, Water Res., 2013, 47, 4433–4450 CrossRef CAS PubMed.
- G. C. Woods and E. R. V. Dickenson, Natural attenuation of NDMA precursors in an urban, wastewater-dominated wash, Water Res., 2016, 89, 293–300 CrossRef CAS PubMed.
- J. Sanchís, W. Gernjak, A. Munné, N. Catalán, M. Petrovic and M. J. Farré, Fate of N-nitrosodimethylamine and its precursors during a wastewater reuse trial in the Llobregat River (Spain), J. Hazard. Mater., 2021, 4075, 124346 CrossRef PubMed.
- E. H. Goslan, S. W. Krasner, M. Bower, S. A. Rocks, P. Holmes, L. S. Levy and S. A. Parsons, A comparison of disinfection by-products found in chlorinated and chloraminated drinking waters in Scotland, Water Res., 2009, 43, 4698–4706 CrossRef CAS PubMed.
- J. W. A. Charrois, J. M. Boyd, K. L. Froese and S. E. Hrudey, Occurrence of N-nitrosamines in Alberta public drinking-water distribution systems, J. Environ. Eng. Sci., 2007, 6, 103–114 CrossRef CAS.
- L. Godo-Pla, J. J. Rodríguez, J. Suquet, P. Emiliano, F. Valero, M. Poch and H. Monclús, Control of primary disinfection in a drinking water treatment plant based on a fuzzy inference system, Process Saf. Environ. Prot., 2021, 145, 63–70 CrossRef CAS.
- C. Stepczuk, E. M. Owens, S. W. Effler, M. T. Auer and J. A. Bloomfield, A modeling analysis of THM precursors for a eutrophic reservoir, Lake Reservoir Manage., 1998, 14, 367–378 CrossRef CAS.
- A. C. Gerecke and D. L. Sedlak, Precursors of N-mitrosodimethylamine in natural waters, Environ. Sci. Technol., 2003, 37, 1331–1336 CrossRef CAS.
- X. R. Zhou, Y. L. Lin, T. Y. Zhang, B. Xu, W. H. Chu, T. C. Cao and W. Q. Zhu, Speciation and seasonal variation of various disinfection by-products in a full-scale drinking water treatment plant in East China, Water Sci. Technol.: Water Supply, 2019, 19, 1579–1586 CAS.
- E. H. Goslan, C. Seigle, D. Purcell, R. Henderson, S. A. Parsons, B. Jefferson and S. J. Judd, Carbonaceous and nitrogenous disinfection by-product formation from algal organic matter, Chemosphere, 2017, 170, 1–9 CrossRef CAS PubMed.
- T. Bond, J. Huang, M. R. Templeton and N. Graham, Occurrence and control of nitrogenous disinfection by-products in drinking water – A review, Water Res., 2011, 45, 4341–4354 CrossRef CAS PubMed.
- A. T. Chow, S.-T. Lee, A. T. O'Geen, T. Orozco, D. Beaudette, P.-K. Wong, P. J. Hernes, K. W. Tate and R. A. Dahlgren, Litter Contributions to Dissolved Organic Matter and Disinfection Byproduct Precursors in California Oak Woodland Watersheds, J. Environ. Qual., 2009, 38, 2334–2343 CrossRef CAS PubMed.
- Q. Zhang, W. F. Kuang, L. Y. Liu, K. Li, K. H. Wong, A. T. Chow and P. K. Wong, Trihalomethane, haloacetonitrile, and chloral hydrate formation potentials of organic carbon fractions from sub-tropical forest soils, J. Hazard. Mater., 2009, 172, 880–887 CrossRef CAS PubMed.
- E. H. Goslan, D. A. Fearing, J. Banks, D. Wilson, P. Hills, A. T. Campbell and S. A. Parsons, Seasonal variations in the disinfection by-product precursor profile of a reservoir water, J. Water Supply: Res. Technol.--AQUA, 2002, 51, 475–482 CrossRef CAS.
- J. Fang, J. Ma, X. Yang and C. Shang, Formation of carbonaceous and nitrogenous disinfection by-products from the chlorination of Microcystis aeruginosa, Water Res., 2010, 44, 1934–1940 CrossRef CAS PubMed.
- S. W. Krasner, P. Westerhoff, B. Chen, B. E. Rittmann and G. Amy, Occurrence of Disinfection Byproducts in United States Wastewater Treatment Plant Effluents, Environ. Sci. Technol., 2009, 43, 8320–8325 CrossRef CAS PubMed.
- S. Park, S. Jung and H. Kim, Regional and seasonal distributions of N-nitrosodimethylamine (NDMA) concentrations in chlorinated drinkingwater distribution systems in Korea, Water, 2019, 11, 2645 CrossRef CAS.
- B. Zhao, N. Nakada, S. Itai, S. Hanamoto, K. Okumura and H. Tanaka, Diurnal patterns of N-nitrosodimethylamine and formaldehyde behaviors in different seasons in surface water influenced by effluent from sewage treatment plants, J. Hazard. Mater., 2020, 383, 121155 CrossRef CAS PubMed.
- N. Yang, C. Zhang, L. Wang, Y. Li, W. Zhang, L. Niu, H. Zhang and L. Wang, Nitrogen cycling processes and the role of multi-trophic microbiota in dam-induced river-reservoir systems, Water Res., 2021, 206, 117730 CrossRef CAS PubMed.
- M. Lürling, F. Eshetu, E. J. Faassen, S. Kosten and V. L. M. Huszar, Comparison of cyanobacterial and green algal growth rates at different temperatures: Temperature and phytoplankton growth rates, Freshwater Biol., 2013, 58, 552–559 CrossRef.
- Y. Du, F. Chen, K. Xiao, C. Song, H. He, Q. Zhang, Y. Zhou, K. Jang, Y. Zhang, P. Xing, Z. Liu, Y. Zhang and Y. Lu, Water Residence Time and Temperature Drive the Dynamics of Dissolved Organic Matter in Alpine Lakes in the Tibetan Plateau, Global Biogeochem. Cycles, 2021, 35, e2020GB006908 CrossRef CAS.
- E. Asmala, R. Autio, H. Kaartokallio, C. A. Stedmon and D. N. Thomas, Processing of humic-rich riverine dissolved organic matter by estuarine bacteria: effects of predegradation and inorganic nutrients, Aquat. Sci., 2014, 76, 451–463 CrossRef CAS.
- I. Reche, M. L. Pace and J. J. Cole, Interactions of Photobleaching and Inorganic Nutrients in Determining Bacterial Growth on Colored Dissolved Organic Carbon, Microb. Ecol., 1998, 36, 270–280 CrossRef CAS PubMed.
- X. Li, N. R. H. Rao, K. L. Linge, C. A. Joll, S. Khan and R. K. Henderson, Formation of algal-derived nitrogenous disinfection by-products during chlorination and chloramination, Water Res., 2020, 183, 116047 CrossRef CAS PubMed.
- J. C. Watson, Establishing Evidence for Internal Structure Using Exploratory Factor Analysis, Meas. Eval. Couns. Dev., 2017, 50, 232–238 CrossRef.
- L. Yang, D. Kim, H. Uzun, T. Karanfil and J. Hur, Assessing trihalomethanes (THMs) and N-nitrosodimethylamine (NDMA) formation potentials in drinking water treatment plants using fluorescence spectroscopy and parallel factor analysis, Chemosphere, 2015, 121, 84–91 CrossRef CAS PubMed.
- K. Watson, M. J. Farré, F. D. L. Leusch and N. Knight, Using fluorescence-parallel factor analysis for assessing disinfection by-product formation and natural organic matter removal efficiency in secondary treated synthetic drinking waters, Sci. Total Environ., 2018, 640–641, 31–40 CrossRef CAS PubMed.
-
M. A. Puig, J. Armengol, G. Gonzalez, J. Peñuelas, S. Sabater and F. Sabater, Chemical and Biological Changes in the Ter River Induced by a Series of Reservoirs, Regul. Streams, 1987, 373–382.
-
J. Carol, L. Benejam, C. Alcaraz, L. Zamora and E. Navarro, The effects of limnological features on fish assemblages of 14 Spanish reservoirs, 2006, 66–77.
- A. Vila-Gispert, M. G. Fox, L. Zamora and R. Moreno-Amich, Morphological variation in pumpkinseed Lepomis gibbosus introduced into Iberian lakes and reservoirs; adaptations to habitat type and diet?, J. Fish Biol., 2007, 71, 163–181 CrossRef.
- V. Moschini-Carlos, M. Pompêo, P. Y. Nishimura and J. Armengol, Phytoplankton as trophic descriptors of a series of Mediterranean reservoirs (Catalonia, Spain), Fundam. Appl. Limnol., 2018, 191, 37–52 CrossRef.
Footnote |
† Electronic supplementary information (ESI) available. See DOI: 10.1039/d1ew00788b |
|
This journal is © The Royal Society of Chemistry 2022 |